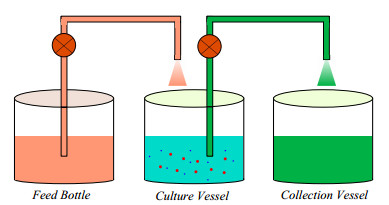
A chemostat is a laboratory device (of the bioreactor type) in which organisms (bacteria, phytoplankton) develop in a controlled manner. This paper studies the asymptotic properties of a chemostat model with generalized interference function and Poisson noise. Due to the complexity of abrupt and erratic fluctuations, we consider the effect of the second order Itô-Lévy processes. The dynamics of our perturbed system are determined by the value of the threshold parameter C⋆0. If C⋆0 is strictly positive, the stationarity and ergodicity properties of our model are verified (practical scenario). If C⋆0 is strictly negative, the considered and modeled microorganism will disappear in an exponential manner. This research provides a comprehensive overview of the chemostat interaction under general assumptions that can be applied to various models in biology and ecology. In order to verify the reliability of our results, we probe the case of industrial waste-water treatment. It is concluded that higher order jumps possess a negative influence on the long-term behavior of microorganisms in the sense that they lead to complete extinction.
Citation: Yassine Sabbar, José Luis Diaz Palencia, Mouhcine Tilioua, Abraham Otero, Anwar Zeb, Salih Djilali. A general chemostat model with second-order Poisson jumps: asymptotic properties and application to industrial waste-water treatment[J]. AIMS Mathematics, 2023, 8(6): 13024-13049. doi: 10.3934/math.2023656
[1] | Yongchang Wei, Zongbin Yin . Long-time dynamics of a stochastic multimolecule oscillatory reaction model with Poisson jumps. AIMS Mathematics, 2022, 7(2): 2956-2972. doi: 10.3934/math.2022163 |
[2] | Yue Dong, Xinzhu Meng . Stochastic dynamic analysis of a chemostat model of intestinal microbes with migratory effect. AIMS Mathematics, 2023, 8(3): 6356-6374. doi: 10.3934/math.2023321 |
[3] | Ramkumar Kasinathan, Ravikumar Kasinathan, Dumitru Baleanu, Anguraj Annamalai . Well posedness of second-order impulsive fractional neutral stochastic differential equations. AIMS Mathematics, 2021, 6(9): 9222-9235. doi: 10.3934/math.2021536 |
[4] | Xiuxian Chen, Zhongyang Sun, Dan Zhu . Mean-variance investment and risk control strategies for a dynamic contagion process with diffusion. AIMS Mathematics, 2024, 9(11): 33062-33086. doi: 10.3934/math.20241580 |
[5] | Kung-Chi Chen, Kuo-Shing Chen . Pricing green financial options under the mixed fractal Brownian motions with jump diffusion environment. AIMS Mathematics, 2024, 9(8): 21496-21523. doi: 10.3934/math.20241044 |
[6] | Jiali Wu, Maoning Tang, Qingxin Meng . A stochastic linear-quadratic optimal control problem with jumps in an infinite horizon. AIMS Mathematics, 2023, 8(2): 4042-4078. doi: 10.3934/math.2023202 |
[7] | Noorah Mshary, Hamdy M. Ahmed, Ahmed S. Ghanem . Existence and controllability of nonlinear evolution equation involving Hilfer fractional derivative with noise and impulsive effect via Rosenblatt process and Poisson jumps. AIMS Mathematics, 2024, 9(4): 9746-9769. doi: 10.3934/math.2024477 |
[8] | Jie Ling, Mingwei Lin, Lili Zhang . Medical waste treatment scheme selection based on single-valued neutrosophic numbers. AIMS Mathematics, 2021, 6(10): 10540-10564. doi: 10.3934/math.2021612 |
[9] | Gunduz Caginalp . Fat tails arise endogenously from supply/demand, with or without jump processes. AIMS Mathematics, 2021, 6(5): 4811-4846. doi: 10.3934/math.2021283 |
[10] | Chunwei Wang, Jiaen Xu, Shujing Wang, Naidan Deng . An investment risk model with bilateral jumps. AIMS Mathematics, 2024, 9(1): 2032-2050. doi: 10.3934/math.2024101 |
A chemostat is a laboratory device (of the bioreactor type) in which organisms (bacteria, phytoplankton) develop in a controlled manner. This paper studies the asymptotic properties of a chemostat model with generalized interference function and Poisson noise. Due to the complexity of abrupt and erratic fluctuations, we consider the effect of the second order Itô-Lévy processes. The dynamics of our perturbed system are determined by the value of the threshold parameter C⋆0. If C⋆0 is strictly positive, the stationarity and ergodicity properties of our model are verified (practical scenario). If C⋆0 is strictly negative, the considered and modeled microorganism will disappear in an exponential manner. This research provides a comprehensive overview of the chemostat interaction under general assumptions that can be applied to various models in biology and ecology. In order to verify the reliability of our results, we probe the case of industrial waste-water treatment. It is concluded that higher order jumps possess a negative influence on the long-term behavior of microorganisms in the sense that they lead to complete extinction.
A chemostat (chemical environment that remains static) is a bioreactor in which fresh medium is continuously added, while culture fluid typically containing remaining nutrients, metabolic end products, and specific microorganisms is continuously removed to the same speed to absolutely keep the culture constant in volume. By adjusting the rate at which the medium is included to the bioreactor, the specific growth rate of the microorganism can be easily controlled within certain limits [1,2]. In industrial application, a standard chemostat is purely a laboratory instrument of three containers that function to multiply and increase microorganisms in an environment (see Figure 1). In its customary form, the outlet of the supply tank is the inlet of the culture vessel, while the outlet of the latter is the inlet of the collection bottle. The first vial contains all the nutritional needs that contribute to the development and reproduction of microorganisms. The content of this bowl is pumped into the 2nd at a constant rate. The microorganisms feed on the nutrients in the feeder and live in the culture vessel which is well agitated so that all organisms retain similar access to the nutrients [3]. The contents of the culture vessel are then pipetted into a third vessel containing the nutrient sources, bacteria and appendages produced by these microorganisms [4].
Broadly speaking, chemostats are the familiar representation of the natural biological competition that occurs when two or more groups compete for the same food supply [5]. It performs a crucial role in environmental modeling [1], interactions between populations [2] and other domains. To better characterize and describe the mechanism of a single-organism chemostat, we consider these two ordinary differential equations (ODEs):
dN(t)=(Q0(N⋆−N(t))−ΘG(N(t))M(t))dt,dM(t)=(G(N(t))M(t)−Q1M(t))dt, |
where N(t) and M(t) are respectively the densities of nourishing elements and microbial organisms at time t. N⋆ is the alimentation flux rate, Q0 is the washout ratio for N(t), Q1 is the elimination average combining alleviation ratio and bacterial death rate, Θ indicates the highest rate of nutrient depletion and also the rate of reproduction for M(t), G refers to the functional response simulating nutrient utilization by bacteria. Generally, one can find in the literature several studies that have treated and analyzed chemostat systems with various shapes of G (uptake function). In Table 1, we assort some popular kinds of G and we offer some references for each case.
Name | Expression | Sources |
Lotka Volterra | G(N(t))=N(t) | [6,7] |
Michaelis Menten (Monod) | G(N(t))=N(t)c+N(t), (c>0) | [8,9] |
Sigmoidal | G(N(t))=N2(t)(c1+N(t))(c2+N(t)), (c1,c2>0) | [10,11,12] |
Monod Haldane | G(N(t))=N(t)m1+N(t)+m2N2(t), (m1,m2>0) | [13,14,15] |
Fundamentally, uninterrupted environmental disturbances adversely affect microbiological cultures and inevitably make it difficult to predict their behavior and attitude [16,17,18,19,20,21,22]. In particular, in the chemostatic situation, even if the empirical results (typically observed according to theoretical standards) relatively resemble the asymptotic behavior of the deterministic model, we cannot ignore the variations that can naturally occur under the operational conditions [23,24,25,26]. Thus, some researchers have incorporated the effect of extrinsic fluctuations in chemostat systems by using white noises [27,28]. These systems are only probabilistic models with standard Brownian motions (SBMs) and therefore their solution is continuous [29,30,31,32]. Unluckily, this approach is not totally adequate to characterize some unforeseen external perturbations such as changes in lab conditions [26,33,34,35,36,37]. Hence, we will investigate to the Lévy effect which are known for their powerful ability to properly formulate the said randomness [38,39,40,41,42]. By taking into consideration this general frame, the authors in [26] integrated linear Lévy noises and the Michaelis-Menten response in the one-organism chemostat system which takes the following shape:
{dN(t)=(Q0(N⋆−N(t))−ΘN(t)c+N(t)M(t))dt+ϱ11N(t)dB1(t)+∫Hκ11(u)N(t−)K(dt,du),dM(t)=(ΘN(t)c+N(t)M(t)−Q1M(t))dt+ϱ21M(t)dB2(t)+∫Hκ21(u)M(t−)K(dt,du), | (1.1) |
where N(t−) and M(t−) are respectively the left limits of N(t) and M(t). Let ΩE,P≡(Ω,E,{Et}t≥0,P) be a filtered probability space with {Et}t≥0 satisfying the habitual conditions. For (1.1), B1≡B1(t) and B2≡B2(t) are independent SBMs defined on ΩE,P with their associated intensities ϱ11,ϱ21>0. N(⋅,⋅) is a Poisson random measure with finite characteristic measure χ(⋅) on a measurable subset H of (0,∞), where χ(H)<∞. The associated compensated Poisson random measure K is expressed by
K(dt,du)=−χ(du)dt+N(dt,du), |
which is an {Et}-martingale. Furthermore, it is supposed that N is independent to SBMs B1, B2; and the functions κ11, κ21 are continuous on H such that κi1(u)>−1, u∈H, (i=1,2). In [26], the authors examined the leverage of the discrete-time delay and linear Lévy noises on the continuation and suppression of the single microorganism M. In a particular case, when κ11(u)=κ21(u)=0, the authors in [20], studied the asymptotic behavior of a one organism in an irregular environment. They indicated that white noises have passive impacts on the organism perseverance.
When severely handling with chemostatic processes, other components must be taken into consideration, such as the acute sensitivity of microorganisms to nutrient concentrations and the mutual interference between chemostatic components [43,44,45]. Therefore, it should be stated that the choice of interaction function influences the prediction of behavior [11,12,46,47]. For this reason, this study puts forward a new chemostat model with an interaction function that takes into consideration many familiar interactions examples (Table 2). Hence, we formulate our system in the following structure:
{dN(t)=(Q0(N⋆−N(t))−ΘΦ(N(t),M(t))M(t))dt+ϱ11N(t)dB1(t)+∫Hκ11(u)N(t−)K(dt,du),dM(t)=(ΘΦ(N(t),M(t))M(t)−Q1M(t))dt+ϱ21M(t)dB2(t)+∫Hκ21(u)M(t−)K(dt,du). | (1.2) |
Name | Expression | |
Interaction type |
||
Interaction type |
||
Interaction type |
||
Beddington-DeAngelis | ||
Crowley-Martin |
We presume that the general interference response Φ∈C2(R+×R+,R+) verifies these hypotheses:
(a) Φ(0,M)=0, ∀M≥0.
(b) ∃A>0 satisfying ∂Φ(N,M)∂N≤A, for all N,M≥0.
(c) Φ is increasing in N and decreasing in M.
(d) Φ follows the uniformly continuous property at M=0: limM→0supN>0{|Φ(N,M)−Φ(N,0)|}=0.
The properties (a), (b), (c) and (d) are easily satisfied by the classic examples listed in the Table 2.
Based on the fact that copious amounts of extrinsic fluctuations (physical conditions) can strongly affect chemostat dynamics, in this research we offer new insight into modeling these environmental complexities. Massive pollution, domestic and industrial sewage discharges, sudden climatic fluctuations, excessive temperatures and human intervention represent the frequent abrupt and unstable changes that affect ecological competition and lead to interruptions in the industrial chemostat process. For this cause, we aim to provide an alternative framework that adequately explains the said laboratory fluctuations [48,49]. Implementing Taylor expansion and second-order jumps, we suggest a highly perturbed model that extends the aforementioned studies and provides a general framework for randomness. This form of noise has not been addressed before in the literature due to a lack of modeling knowledge and some analytical complexities. So, the current study offers a major enhancement and generalization of the papers [20] and [26]. Explicitly, we presume the practical and real situation of the chemostat mechanism by assuming the nonlinearity of perturbations and raising the order of Lévy noises to the second one (quadratic) in the system (1.2) as follows:
{dN(t)=(Q0(N⋆−N(t))−ΘΦ(N(t),M(t))M(t))dt+dPH,21(t),dM(t)=(ΘΦ(N(t),M(t))M(t)−Q1M(t))dt+dPH,22(t), | (1.3) |
where
dPH,21(t)=(ϱ11N(t)+ϱ12N2(t))dB1(t)+∫H(κ11(u)N(t−)+κ12(u)N2(t−))K(dt,du),dPH,22(t)=(ϱ21M(t)+ϱ22M2(t))dB2(t)+∫H(κ21(u)M(t−)+κ22(u)M2(t−))K(dt,du). |
Here, ϱ11, ϱ21 denote the white noise intensities of the first order (linear), and ϱ12, ϱ22 stand for that of the second order (quadratic). The Lévy intensities κ11(u), κ21(u), κ12(u), κ22(u) are positive and continuous functions.
Setting R2,⋆+={(x,y):x>0,y>0} and supposing that ∫Hκ2kℓ(u)χ(du)<∞; k,ℓ=1,2. By this assumption, and similar reasoning as in ([33], Theorem 1), we deduce that for (N(0),M(0))∈R2,⋆+, the corresponding solution (N(t),M(t))∈R2,⋆+.
When analyzing a mathematical formulation that characterizes the long-run behavior of an organism, our main preoccupation is to know if it will end or will last. Since our stochastic model (1.3) is perturbed by a new version of perturbations, threshold analysis is considered an intriguing and important issue. Moreover, nonlinear random perturbations are a rich topic that arouses the interest of many researchers on account of their real-world significance and practicality [22,50,51,52]. As far as we know, there is no existing study that attempts to present and treat chemostat systems with quadratic Lévy noises and a general interference function. In this research, we adopt this general framework and we analyze the system (1.3) by discussing the stationarity and disappearance properties of M. So, our problematic is to find the sufficient and necessary condition for these two asymptotic characteristics. Technically, this study offers a new and general scope for treating perturbed models driven by quadratic Lévy jumps in biology. We present the sill among the ergodicity and disappearance of M by the use of the probabilistic comparison theorem, the exponential inequality for martingales, the Feller property and other mathematical tools. The obtained threshold value C⋆0 is regarded to be sufficient for having an excellent view of the long-time chemostat process. Specifically, if C⋆0>0, we have the existence and uniqueness of an ergodic stationary distribution, while the extinction happens when C⋆0<0.
The remaining parts of this research are ordered in the following arrangement: In Section 2, we present some long-run characteristics of an auxiliary chemostat equation with quadratic jumps, then we introduce the sill C⋆0 of our model. In Section 3, we demonstrate that C⋆0 is the real threshold of our system (1.3). In Section 4, we give an application of waste-water treatment to affirm and clarify our theoretical outcomes.
This section is devoted to the dynamical analysis of a boundary chemostat equation in the case of M(t)=0 (absence of the microorganism). Because of that reason, we utilize the following auxiliary equation with quadratic Lévy jumps:
{dΨ(t)=Q0(N⋆−Ψ(t))dt+(ϱ11Ψ(t)+ϱ12Ψ2(t))dB1(t)+∫H(κ11(u)Ψ(t−)+κ12(u)Ψ2(t−))K(dt,du),Ψ(0)=N(0)>0. | (2.1) |
Remark 2.1. For any positive initial value, Eq (2.1) has a solution which is: positive, unique and global [41].
Remark 2.2. Via the well-known probabilistic comparison theorem [53], one can infer that Ψ(t)≥N(t) for all t∈[0,∞) almost surely (abbreviated as a.s.).
To move forward, the next lemma is needed.
Lemma 2.1. The solution Ψ of (2.1) satisfies two main results.
1) For all p∈(0,1], ∃˜m independent of Ψ(0) satisfying
lim supt→∞E{p−1(1+Ψ)p}≤˜m<∞. | (2.2) |
2) Ψ(t) has the unique ergodic stationary distribution πΨ(⋅), and
limt→∞1t∫t0Ψ(s)ds=∫∞0xπΨ(dx)=N⋆. | (2.3) |
Proof. For the convenience of the reader, we split the proof into two stages.
Part I. We let the Lyapunov function
V(Ψ)=p−1(1+Ψ)p. |
By Itô differential operator LV of (2.1), we get
LV(Ψ)=(1+Ψ)p−1(Q0N⋆−Q0Ψ)+0.5(p−1)(1+Ψ)p−2(ϱ11Ψ+ϱ12Ψ2)2+∫H(p−1((1+Ψ)+κ11(u)Ψ+κ12(u)Ψ2)p−p−1(1+Ψ)p−(1+Ψ)p−1(κ11(u)Ψ+κ12(u)Ψ2))χ(du). |
Clearly, we have for all 0<p≤1, that
∫H[(1+κ11(u)Ψ1+Ψ+κ12(u)Ψ21+Ψ)p−1−p(κ11(u)Ψ1+Ψ+κ12(u)Ψ21+Ψ)]χ(du)≤0. |
Then, LV(Ψ)≤Q0(1+N⋆)⏟=v2(1+Ψ)p−1−Q0⏟=1pv1(1+Ψ)p. Consequently
LV(Ψ)≤−v1V(Ψ)+v2. | (2.4) |
According to the proof of Lemma 2.3 in [54], we get
lim supt→∞E{p−1(1+Ψ)p}≤v2v1=˜m<∞. |
Part II. Based on the theory presented in [55], in order to check the ergodicity of Ψ(t), it is enough to prove the existence of a non-negative function V and v1,v2>0 such that
LV(Ψ)≤−v1V(Ψ)+v2. |
From the inequality (2.4), Theorem 6.3 in [55], and ergodic theorem [56], we deduce that Ψ(t) has a unique ergodic stationary distribution (named as πΨ), and
limt→∞1t∫t0Ψ(s)ds=∫∞0xπΨ(dx). |
To find this limit, we simply take the expectation on both sides of (2.1), then we have
0=limt→∞EΨ(t)t=Q0N⋆−Q0limt→∞1t∫t0EΨ(s)ds=Q0N⋆−Q0Elimt→∞1t∫t0Ψ(s)ds=Q0N⋆−Q0E∫∞0xπΨ(dx). | (2.5) |
Therefore
Q0N⋆−Q0E∫∞0xπΨ(dx)=Q0N⋆−Q0∫∞0xπΨ(dx)=0. |
Consequently, we get
limt→∞1t∫t0Ψ(s)ds=∫∞0xπΨ(dx)=N⋆. |
Remark 2.3. Lemma 2.1 plays an intrinsic role to substantiate the outcomes of this study.
Remark 2.4. Set ϱ⋆=max{ϱ211,ϱ221}, κ⋆(u)=max{κ11(u),κ21(u)} and κ⋆(u)=min{κ11(u),κ21(u)}. To highlight the value of the result (2.5), we compare it with that in [34]. According to [41], we can get the results in [34] without considering the following assumption:
Q0−0.5(p−1)ϱ⋆−1p∫H((1+κ⋆(u))p−1−pκ⋆(u))χ(du)>0,p>1, | (2.6) |
which is widely used to demonstrate that
1) limt→∞Ψ(t)t=0 a.s.
2) limt→∞1t∫t0Ψ(s)dB1(s)=0, and limt→∞1t∫t0Ψ2(s)dB1(s)=0 a.s.
3) limt→∞1t∫t0∫H(κ11(u)Ψ(s−)+κ12(u)Ψ2(s−))K(ds,du)=0 a.s.
In this study, we offer an alternative approach without any extra restrictions on parameters like (2.6).
Remark 2.5. Note that the result exhibited in Lemma 2.1 is a generalization of that presented in Remarks 2 and 3 of [41].
Remark 2.6. By using the properties of Φ and Lemma 2.1, we conclude that
∫∞0Φ(x,0)πΨ(dx)≤A∫∞0xπΨ(dx)=limt→∞At∫t0Ψ(s)ds=AN⋆<∞. |
By using Remark 2.6, the threshold value of (1.3) is given in the following structure
C⋆0=Θ∫∞0Φ(x,0)πΨ(dx)−Q1−0.5ϱ221−∫H(κ21(u)−ln(1+κ21(u)))χ(du). |
Remark 2.7. Note that in the case of the functional response type 1, the threshold is
C⋆,10=Θ∫∞0xπΨ(dx)−Q1−0.5ϱ221−∫H(κ21(u)−ln(1+κ21(u)))χ(du). |
Since the expression of πΨ is unknown, Lemma 2.1 gives the exact value of the sill value which is presented as:
C⋆,10=ΘN⋆−Q1−0.5ϱ221−∫H(κ21(u)−ln(1+κ21(u)))χ(du). |
In the next part, we shall show that C⋆0 is the sill among the stationarity and the disappearance of M.
This subsection introduces a new approach to verify the stationarity (and of course the ergodic property) of our system. This method is presented by the following interesting lemma.
Lemma 3.1. [Limited possibilities lemma [57]] Consider a stochastic process M∈Rn that satisfy the Feller property, then one of the following cases occurs: theexistence and uniqueness of an ergodic stationary distribution, or
limt→∞supˆζ1t∫t0∫RnP(x;s,D)ˆζ(dx)ds=0,forallclosedandboundedsubsetD⊂Rn, | (3.1) |
where the supremum is taken over all initial distributions ˆζ on Rn and P(x;s,D) is the probability for M belongs to D with M(0)=x∈Rn.
Theorem 3.1. For any initial data (N(0),M(0))∈R2,⋆+, if C⋆0>0, the solution (N(t),M(t)) to (1.3) has the ergodic property and admits a unique stationary distribution π⋆(⋅).
Proof. From (Lemma 3.2 [58]), we easily verify that the solution of (1.3) has the Feller property. The main purpose of next part is to prove that (3.1) is impossible. Via using Itô's formula, we have
L(−lnM(t))=−ΘΦ(N(t),M(t))+Q1+0.5(ϱ21+ϱ22M(t))2−∫H(ln(1+κ21(u)+κ22(u)M(t))−(κ21(u)+κ22(u)M(t)))χ(du)=−ΘΦ(Ψ(t),0)+Q1+0.5ϱ221+∫H(κ21(u)−ln(1+κ21(u)))χ(du)+ΘΦ(Ψ(t),0)−ΘΦ(N(t),M(t))−ΘΦ(N(t),0)+ΘΦ(N(t),0)+ϱ21ϱ22M(t)+0.5ϱ222M2(t)+∫H[κ22(u)M(t)−ln(1+κ22(u)M(t)1+κ21(u))]χ(du). |
Then, we get
L(−lnM(t))≤−ΘΦ(Ψ(t),0)+Q1+0.5ϱ221+∫H(κ21(u)−ln(1+κ21(u)))χ(du)+ΘA(Ψ(t)−N(t))+(ϱ21ϱ22+∫Hκ22(u)χ(du))M(t)+0.5ϱ222M2(t)+ΘΦ(N(t),0)−ΘΦ(N(t),M(t)). | (3.2) |
On the other hand, we have
L(lnΨ(t)−lnN(t))≤(Q0N⋆Ψ(t)−Q0N⋆N(t))+ΘΦ(N(t),M(t))M(t)N(t)−0.5((ϱ11+ϱ12Ψ(t))2−(ϱ11+ϱ12N(t))2)+∫H[ln(1+κ11(u)+κ12(u)Ψ(t)1+κ11(u)+κ12(u)N(t))−κ12(u)(Ψ(t)−N(t))]χ(du)≤ΘAM(t)−ϱ11ϱ12(Ψ(t)−N(t))−(Ψ(t)−N(t))∫H[κ12(u)(κ11(u)+κ12(u)N(t))1+κ11(u)+κ12(u)N(t)]χ(du)≤ΘAM(t)−ϱ11ϱ12(Ψ(t)−N(t)). | (3.3) |
We combine (3.2) and (3.3), then
L(−lnM(t)+AΘϱ11ϱ12(lnΨ(t)−lnN(t)))≤−ΘΦ(Ψ(t),0)+Q1+0.5ϱ221+∫H(κ21(u)−ln(1+κ21(u)))χ(du)+(ϱ21ϱ22+∫Hκ22(u)χ(du))M(t)+A2Θ2ϱ11ϱ12M(t)+0.5ϱ222M2(t)+ΘΦ(N(t),0)−ΘΦ(N(t),M(t))=−Θ∫∞0Φ(x,0)πΨ(dx)+Q1+0.5ϱ221+∫H(κ21(u)−ln(1+κ21(u)))χ(du)+Θ(∫∞0Φ(x,0)πΨ(dx)−Φ(Ψ(t),0))+(A2Θ2ϱ11ϱ12+ϱ21ϱ22+∫Hκ22(u)χ(du))M(t)+0.5ϱ222M2(t)+ΘΦ(N(t),0)−ΘΦ(N(t),M(t)). |
Choose a positive value s verifying s≥1Q1(A2Θ2ϱ11ϱ12+ϱ21ϱ22+∫Hκ22(u)χ(du)), and define
V(t)=−lnM(t)+AΘϱ11ϱ12(lnΨ(t)−lnN(t))+sM(t). |
Then, we have
LV(t)≤−Θ∫∞0Φ(x,0)πΨ(dx)+Q1+0.5ϱ221+∫H(κ21(u)−ln(1+κ21(u)))χ(du)+Θ(∫∞0Φ(x,0)πΨ(dx)−Φ(Ψ(t),0))+ΘΦ(N(t),0)−ΘΦ(N(t),M(t))+0.5ϱ222M2(t)+sΘΦ(N(t),M(t))M(t)≤−C⋆0+Θ(∫∞0Φ(x,0)πΨ(dx)−Φ(Ψ(t),0))+Θ(Φ(N(t),0)−Φ(N(t),M(t)))+0.5ϱ222M2(t)+sΘAN(t)M(t). |
By Itô's formula to p−1(1+N)p and p−1Mp for p∈(0,1), gives
L((1+N(t))pp)=(1+N(t))p−1(Q0N⋆−Q0N(t)−ΘΦ(N(t),M(t))M(t))+0.5(p−1)(1+N(t))p−2(ϱ11N(t)+ϱ12N2(t))2+∫H(((1+N(t))+κ11(u)N(t)+κ12(u)N2(t))pp−(1+N(t))pp−(1+N(t))p−1(κ11(u)N(t)+κ12(u)N2(t)))χ(du)≤Q0N⋆−0.5(1−p)ϱ212Np+2(t), |
and
L(Mp(t)p)=Mp−1(t)(ΘΦ(N(t),M(t))M(t)−Q1M(t))+0.5(p−1)Mp−2(t)(ϱ21M(t)+ϱ22M2(t))2+∫H((M(t)+κ11(u)M(t)+κ12(u)M2(t))pp−Mp(t)p−Mp(t)(κ11(u)+κ12(u)M(t)))χ(du)≤ΘAN(t)Mp(t)−(Q1+0.5(1−p)ϱ221)Mp(t)−(1−p)ϱ21ϱ22Mp+1(t)−0.5(1−p)ϱ222Mp+2(t)≤ΘAp+1Np+1(t)+pΘAp+1Mp+1(t)−0.5(1−p)ϱ222Mp+2(t). |
Consider the function ˜V(N(t),M(t))=DV(t)+(1+N(t))pp+Mp(t)p, where D>0 is a sufficiently large number such that −DC⋆0+B≤−2, and
B=max{sup(N,M)∈R2,⋆+{ΘAp+1Np+1−0.25(1−p)ϱ212Np+2+pΘAp+1Mp+1−0.25(1−p)ϱ222Mp+2+Q0N⋆},1}. |
Since ˜V(N,M) gets the lower bound at (N_,M_) in R2+, we let the Lyapunov function
˜V(N(t),M(t))=DV(t)+(1+N(t))pp+M(t)pp−˜V(N_,M_). |
Then, one can see that
L˜V(N(t),M(t))≤−DC⋆0+DsΘAN(t)M(t)+DΘ(Φ(N(t),0)−Φ(N(t),M(t)))+0.5Dϱ222M2(t)−0.25(1−p)ϱ212Np+2(t)−0.25(1−p)ϱ222Mp+2(t)+Q0N⋆+ΘAp+1Np+1(t)−0.25(1−p)ϱ212Np+2(t)+pΘAp+1Mp+1(t)−0.25(1−p)ϱ222Mp+2(t)+DΘ(∫∞0Φ(x,0)πΨ(dx)−Φ(Ψ(t),0))=g(N(t),I(t))+DΘ(∫∞0Φ(x,0)πΨ(dx)−Φ(Ψ(t),0)). |
We consider the following bounded domain
Dϵ,ϵ⋆={(N,M)∈R2,⋆+|ϵ≤N≤ϵ−1,ϵ⋆≤M≤ϵ−1⋆}, |
with 0<ϵ<1, ϵ⋆=min{ϵ,ϵ0} and ϵ0 will be accurately chosen in the following. In the set R2,⋆+∖Dϵ,ϵ⋆, let ϵ sufficiently small satisfying
DΘAϵ(s+1)+pD(sΘAϵ+0.5ϱ222)2+p(2D(sΘAϵ+0.5ϱ222)0.25(1−p)(2+p)ϱ222)2p≤1, | (3.4) |
Dϵ(sΘA+0.5ϱ222ϵ)+pDsΘAϵ2+p(2DsΘAϵ0.25(1−p)(2+p)ϱ212)2p<1, | (3.5) |
−DC⋆0+Z−0.25(1−p)ϱ212ϵ−(2+p)≤−1, | (3.6) |
−DC⋆0+Z−0.25(1−p)ϱ222ϵ−(2+p)≤−1, | (3.7) |
where
Z=sup(N,M)∈R2,⋆+{0.5DsΘAN2+0.5D(sΘA+ϱ222)M2+Q0N⋆+ΘAp+1Np+1−0.25(1−p)ϱ212Np+2+pΘAp+1Mp+1−0.25(1−p)ϱ222Mp+2}. |
Next, we split R2,⋆+∖Dϵ,ϵ⋆ into
Dϵ,1={(N,M)∈R2,⋆+|0<N<ϵ},Dϵ⋆,2={(N,M)∈R2,⋆+|0<M<ϵ⋆},Dϵ,3={(N,M)∈R2,⋆+|N>ϵ−1},Dϵ⋆,4={(N,M)∈R2,⋆+|M>ϵ−1⋆}. |
Plainly, Dcϵ,ϵ⋆=R2,⋆+∖Dϵ,ϵ⋆=Dϵ,1∪Dϵ⋆,2∪Dϵ,3∪Dϵ⋆,4. Next, we check the inequality
g(N,M)≤−1, | (3.8) |
First case. For any (N,M)∈Dϵ,1, we utilize NM≤ϵM≤ϵ(1+M2) and (3.4) to obtain that
g(N(t),M(t))≤−DC⋆0+DsΘAϵ+DΘAϵ+DsΘAϵM2(t)+0.5Dϱ222M2(t)−0.25(1−p)ϱ222Mp+2(t)+Q0N⋆+ΘAp+1Np+1(t)−0.25(1−p)ϱ212Np+2(t)+pΘAp+1Mp+1(t)−0.25(1−p)ϱ222Mp+2(t)≤−DC⋆0+B+DsΘAϵ+DΘAϵ+pD(sΘAϵ+0.5ϱ222)2+p(2D(sΘAϵ+0.5ϱ222)0.25(1−p)(2+p)ϱ222)2p≤−1. |
Second case. Φ(N(t),M(t)) is uniformly continuous at M=0. Hence, assumption (d) implies that ∃ϵ0>0 satisfying 0<M≤ϵ0,
DsΘAϵ+0.5Dϱ222ϵ2+pDsΘAϵ2+p(2DsΘAϵ0.25(1−p)(2+p)ϱ212)2p⏟<1(viacondition(3.5))+DΘ(Φ(N(t),0)−Φ(N(t),M(t)))<1. |
Thus, if M<ϵ⋆=min{ϵ,ϵ0}, NM≤ϵN≤ϵ(1+N2), we get from (3.5) that
g(N(t),I(t))≤−DC⋆0+DsΘAϵ+DsΘAϵN2(t)+0.5Dϱ222ϵ2+DΘ(Φ(N(t),0)−Φ(N(t),M(t)))−0.25(1−p)ϱ212Np+2(t)+Q0N⋆+ΘAp+1Np+1(t)−0.25(1−p)ϱ212Np+2(t)+pΘAp+1Mp+1(t)−0.25(1−p)ϱ222Mp+2(t)≤−DC⋆0+B+DsΘAϵ+0.5Dϱ222ϵ2+pDsΘAϵ2+p(2DsΘAϵ0.25(1−p)(2+p)ϱ212)2p+DΘ(Φ(N(t),0)−Φ(N(t),M(t)))≤−1. |
Third case. When (N,M)∈Dϵ,3, we use the classical inequality NM≤N22−1+M22−1, then
g(N(t),M(t))≤−DC⋆0+0.5DsΘAN2(t)+DΘAN(t)−0.25(1−p)ϱ212Np+2(t)+0.5DsΘAM2(t)+0.5Dϱ222M2(t)+Q0N⋆+ΘAp+1Np+1(t)−0.25(1−p)ϱ212Np+2(t)+pΘAp+1Mp+1(t)−0.25(1−p)ϱ222Mp+2(t)≤−DC⋆0+Z−0.25(1−p)ϱ212ϵ−(2+p)≤−1, |
that can be deduced by (3.6).
Fourth case. For any (N,M)∈Dϵ⋆,4, by the arguments in case 3, we obtain from (3.7)
g(N(t),M(t))≤−DC⋆0+0.5DsΘAN2(t)+DΘAN(t)−0.25(1−p)ϱ222Mp+2(t)+0.5DsΘAM2(t)+0.5Dϱ222M2(t)+Q0N⋆+ΘAp+1Np+1(t)−0.25(1−p)ϱ212Np+2(t)+pΘAp+1Mp+1(t)−0.25(1−p)ϱ222Mp+2(t)≤−DC⋆0+Z−0.25(1−p)ϱ222ϵ−(2+p)≤−1. |
Hence, (3.8) is obtained. Besides, we can prove that ∃˜a>0 verifying g(N,M)≤˜a, for all (N,M)∈R2,⋆+. Thus,
−E(˜V(N(0),M(0)))≤E(˜V(N(t),M(t)))−E(˜V(N(0),M(0)))=∫t0E(L˜V(N(s),M(s)))ds≤∫t0E(g(N(s),M(s)))ds+DΘE(∫t0∫∞0Φ(x,0)πΨ(dx)ds−∫t0Φ(Ψ(s),0)ds). |
The ergodic property of Ψ(t) gives
0≤lim inft→∞1t∫t0(Eg(N(s),M(s))1{(N(s),M(s))∈Dcϵ,ϵ⋆}+Eg(N(s),M(s))1{(N(s),M(s))∈Dϵ,ϵ⋆})ds≤lim inft→∞1t∫t0(−P((N(s),M(s))∈Dcϵ,ϵ⋆)+˜aP((N(s),M(s))∈Dϵ,ϵ⋆))ds=−1+(1+˜a)lim inft→∞1t∫t0P((N(s),M(s))∈Dϵ,ϵ⋆)ds. |
Therefore,
lim inft→∞1t∫t0P((N(s),M(s))∈Dϵ,ϵ⋆)ds≥11+˜a>0. |
Which means
lim inft→∞1t∫t0P((N(0),I(0));s,Dϵ,ϵ⋆)ds≥11+˜a>0,∀(N(0),I(0))∈R2,⋆+. |
Theorem 3.2. Let (N(t),M(t)) be the solution of (1.3) for (N(0),M(0))∈R2,⋆+. If C⋆0<0, then (N(t),M(t)) follows:
lim supt→∞t−1lnM(t)≤C⋆0<0a.s., |
which means that M will exponentially extinct with probability 1. Moreover, N(t) converges weakly to the stationary distribution πΨ of Ψ(t).
Proof. The proof is achieved through the following parts.
Part I. Itô's formula implies
dlnM(t)=(ΘΦ(N(t),M(t))−Q1−0.5(ϱ21+ϱ22M(t))2+∫H(ln(1+κ21(u)+κ22(u)M(t))−(κ21(u)+κ22(u)M(t)))χ(du))dt+(ϱ21+ϱ22M(t))dB2(t)+∫Hln(1+κ21(u)+κ22(u)M(t−))K(dt,du). |
From Remark (2.2), we obtain
dlnM(t)≤(ΘΦ(Ψ(t),0)−Q1−0.5(ϱ21+ϱ22M(t))2+∫H(ln(1+κ21(u)+κ22(u)M(t))−(κ21(u)+κ22(u)M(t)))χ(du))dt+(ϱ21+ϱ22M(t))dB2(t)+∫Hln(1+κ21(u)+κ22(u)M(t−))K(dt,du). |
We integrate from 0 to t and divide by t on both sides of the last inequality, then
lnM(t)−lnM(0)t≤Θt∫t0Φ(Ψ(s),0)ds−Q1−0.5ϱ221−∫H(κ21(u)−ln(1+κ21(u)))χ(du)−ϱ21ϱ22t∫t0M(s)ds−0.5ϱ222t∫t0X2(s)ds+1t∫t0ϱ21dB2(s)+1t∫t0∫H[ln(1+κ22(u)M(s)1+κ21(u))−κ22(u)M(s)]χ(du)ds+1t∫t0ϱ22M(s)dB2(s)+1t∫t0∫Hln(1+κ21(u))K(ds,du)+1t∫t0∫Hln(1+κ22(u)M(s−)1+κ21(u))K(ds,du). |
We let G1(t)=∫t0ϱ21dB2(s) and G2(t)=∫t0∫Hln(1+κ21(u))K(ds,du). It is easy to show that their quadratic variations are given by
⟨G1(t),G1(t)⟩=ϱ221tand⟨G2(t),G2(t)⟩=t∫H(ln(1+κ21(u)))2χ(du). |
By employing the strong large numbers theorem for local martingales [59], we obtain
t−1G1(t)→0a.s.andt−1G2(t)→0a.s.,ast→∞. |
Now, we apply the exponential inequality for martingales described in [59], then
P{sup0≤t≤n[∫t0ϱ22M(s)dB2(s)−0.5∫t0ϱ222X2(s)ds−∫t0∫H((κ22(u)M(s)1+κ21(u))+ln(1+κ22(u)M(s)1+κ21(u)))χ(du)ds+∫t0∫Hln(1+κ22(u)M(s−)1+κ21(u))K(ds,du)]≥2lnn}≤1n2. |
Borel-Cantelli Lemma [59] stated that for almost ω∈Ω, we have the existence of an integer nω>0 such that for all n≥nω, t∈[n−1,n)⊆R+ a.s.,
2lnn+0.5∫t0ϱ222X2(s)ds+∫t0∫H((κ22(u)M(s)1+κ21(u))−ln(1+κ22(u)M(s)1+κ21(u)))χ(du)ds≥∫t0ϱ22M(s)dB2(s)+∫t0∫Hln(1+κ22(u)M(s−)1+κ21(u))K(ds,du). |
Consequently, for all n≥nω, t∈[n−1,n)⊆R+ a.s., we established that
lnM(t)−lnM(0)t≤[Θt∫t0Φ(Ψ(s),0)ds−Q1−0.5ϱ221−∫H(κ21(u)−ln(1+κ21(u)))χ(du)]−ϱ21ϱ22t∫t0M(s)ds+1t∫t0∫H((κ22(u)M(s)1+κ21(u))−κ22(u)M(s))χ(du)ds+G1(t)t+G2(t)t+2lnnn−1. |
We take the superior limit on both sides of the last result, then
lim supt→∞lnM(t)t≤Θ∫∞0Φ(x,0)πΨ(dx)−Q1−0.5ϱ221−∫H(κ21(u)−ln(1+κ21(u)))χ(du)⏟C⋆0<0. |
Since the exponential extinction implies the stochastic extinction [59], so limt→∞M(t)=0 a.s. and the microorganism in the chemostat of (1.3) almost sure to be extinct.
Part II. From Part I, we deduce that for r>0, ∃t0 and Ωr⊂Ω verify P(Ωr)>1−r and
Φ(N,M)M≤Φ(N,0)M≤ArN. |
Hence,
(Q0(N⋆−N(t))−ΘArN(t))dt+dPH,21(t)≤dN(t)≤(Q0(N⋆−N(t)))dt+dPH,21(t), |
indicates that the distribution of N(t) converges weakly to the πΨ(⋅). The proof of the extinction theorem is finished.
Now, we give a real example of the stochastic model (1.3). Our fundamental purpose is to belay the accuracy of the obtained results and to highlight the complex long-run attitude of chemostat mechanisms. Lastly, we will give a short discussion about our outcomes.
Through waste-water handling, we can turn sewage into treated water that is reused in daily activities. In general, waste-water is full of bacterial contaminants, chemicals, and other toxins that can result from industrial production and standard human activities. Its treatment process aims to reduce pollutants to reasonable levels and make the water safe to discharge back into the environment. Figure 2 presents a classical configuration for a biological interaction that is used in the waste-water treatment. A detailed description can be found in [2].
Now, we investigate the bacteria reproduction in the waste-water treatment process by (1.3) with Beddington-DeAngelis interference function. Specifically, we suppose that the bacteria reproduction is presented by the M equation, and reproduce through the consumption of a substrate species N. The purpose is to monitor the substrate and bacteria density of waste-water generated by industry activities. For this reason, we study the asymptotic dynamics of the model processed by Nguyen et al. in [60], that is
{dN(t)=(=Q0⏞1θ(N⋆−N(t))−ΘN(t)M(t)1+mNN(t)+mMM(t))dt+dPH,21(t),dM(t)=(ΘYN(t)M(t)1+mNN(t)+mMM(t)−(Qd+1+Rθ)⏟=Q1M(t))dt+dPH,22(t),N(0)=10,M(0)=4.5. | (4.1) |
Here, we apply the algorithm presented in [61] to discretize (4.1). We consider the values listed in Tables 3 (obtained from [2]) and 4, we consider two different scenarios of waste-water operation under unexpected and higher order fluctuations.
Parameters | Domain | Units | Scenario 1 | Scenario 2 |
day | 1 | 7 | ||
mg of cells |
8 | 8 | ||
mg of cells |
60 | 60 | ||
mg of cells |
60 | 60 | ||
Dimensionless | 0.7 | 0.7 | ||
1/day | 0.06 | 0.06 | ||
– | ||||
– | mg of cells |
15 | 15 |
Intensity | Scenario 1 | Scenario 2 |
0.013 | 0.01 | |
0.04 | 0.02 | |
0.02 | 0.02 | |
0.03 | 0.01 | |
0.01 | 0.07 | |
0.04 | 0.01 | |
0.015 | 0.015 | |
0.013 | 0.011 |
We take the values of the detreministc coefficients from Table 3 (column Scenario 1) and the values of noise intensities from Table 4 (column Scenario 1). By assuming that χ(H)=1 and for T>0 sufficiently large, the sill C⋆0 is calculated as follows:
C⋆0=∫∞0ΘYx1+mNxπΨ(dx)−(Qd+1+Rθ)−0.5ϱ221−∫H(κ21(u)−ln(1+κ21(u)))χ(du)=limT→∞1T∫T0ΘYΨ(s)1+mNΨ(s)ds−(Qd+1+Rθ)−0.5ϱ221−∫H(κ21(u)−ln(1+κ21(u)))χ(du)≈−0.9980<0. |
From Theorem 3.2, we deduce that M goes to extinction and N persists almost surely. Figure 3 confirm this result.
Let us choose the parameters from 5th column of Table 3 and 3rd column of Table 4. Then, we get
C⋆0=∫∞0ΘYx1+mNxπΨ(dx)−(Qd+1+Rθ)−0.5ϱ221−∫H(κ21(u)−ln(1+κ21(u)))χ(du)=limT→∞1T∫T0ΘYΨ(s)1+mNΨ(s)ds−(Qd+1+Rθ)−0.5ϱ221−∫H(κ21(u)−ln(1+κ21(u)))χ(du)≈0.4446>0. |
Hence, by Theorem 2.3 that there exists a unique ergodic stationary distribution of (4.1) (see Figure 4). This result indicates that the bacteria persists in the waste-water regulation process. We also sketch experimental two-dimensional density of (N(t),M(t)) in Figure 5.
To highlight the impact of second-order noise on the chaotic dynamics of the industrial chemostat, we merely present Figures 6. In the latter, we can clearly show that taking higher-order disturbances into account can radically alter the behavior asymptotic of microorganisms and can switch its persistence to its state of extinction. This means that a large amount of noise helps severely reduce the growth of bacteria and inhibit the chemostat process. In other words, at a given value of quadratic jump intensities, a dynamic bifurcation occurs and various scenarios can occur. Moreover, it can be deduced that loud noises have a negative impact on the dynamics of bacteria and the extinction time is proportional to the intensity values.
Determining the hydraulic retention time θ is an intrinsic factor for the operation of a waste-water treatment process. To accommodate the high hydraulic load, the station must control the wash-out time. In fact, sewage flow rates throughout the day have a large variance, and the drainage system must adjust this difference. If the residence time is less than a critical value, denoted by θ⋆0 (to find it, we solve the equation C⋆0(θ)=0), then the sewage flow is too fast for bacteria to grow and increase, existing cells are flushed out rapidly than they can compound [60]. Consequently, the bacteria become extinct. From Figure 7, we conclude that C⋆0 is an increasing function of θ and we have the result appearing in Table 5. Under specified conditions, controlling θ⋆0 is the theoretical approach to enable the plant to handle with the higher hydraulic loading and avoid the disappearance of bacteria (Scenario 1) under the faster flow of the untreated effluent.
Scenario | Critical value |
1 | 1.9888 |
2 | 2.7450 |
During this research, we have suggested and processed a novel shape of the chemostat model which joints two hypotheses: general interaction function and quadratic Lévy noises. This merger provides a comprehensive overview of the intermingling among organisms and nutrients in a highly perturbed environment. Then we have the following points:
⋆ The investigated model has a threshold behavior using some dynamical properties of the Eq (2.1) perturbed by quadratic Lévy noises.
⋆ By Theorem 3.1, have that the model has a stationarity and ergodicity point.
⋆ In Theorem 3.2, the extinction scenario is investigated, also the weak convergence of nutrient distribution to that of the boundary auxiliary Eq (2.1).
⋆ As a practical example, we have treated the waste-water operation under Lévy perturbations.
The strong point of this study is that we succeeded in obtaining the sufficient and necessary condition of the ergodicity and the extinction without imposing any additional hypotheses or constraints on the model parameters. As limitations of this study, we can mention the following two open problems:
⋆ What happens in the case of C⋆0=0?
⋆ Can we give an analytical method to process the model (1.3) in the case of negative Lévy intensities?
We will do our best in the future to address these issues.
This work has been financed by the CEU San Pablo University, grant number MGI22AOQ.
The corresponding author states that there is no conflict of interest.
[1] | H. L. Smith, P. Waltman, The theory of the chemostat: dynamics of microbial competition, Cambridge: Cambridge university press, 1995. |
[2] | W. Nazaroff, L. Alvarez-Cohen, Environmental engineering science, New York: John Wiley and Sons, 2001. |
[3] |
A. Novick, L. Szilard, Experiments with the chemostat on spontaneous mutations of bacteria, Proc. Natl. Acad. Sci., 36 (1950), 708–719. https://doi.org/10.1073/pnas.36.12.708 doi: 10.1073/pnas.36.12.708
![]() |
[4] |
A. Novick, L. Szilard, Description of the chemostat, Science, 112 (1950), 715–716. https://doi.org/10.1126/science.112.2920.715 doi: 10.1126/science.112.2920.715
![]() |
[5] |
S. Pavlou, I. G. Kevrekidis, Microbial predation in a periodically operated chemostat: a global study of the interaction between natural and externally imposed frequencies, Math. Biosci., 108 (1992), 1–55. https://doi.org/10.1016/0025-5564(92)90002-E doi: 10.1016/0025-5564(92)90002-E
![]() |
[6] |
F. Y. Wang, G. P. Pang, S. W. Zhang, Analysis of a Lotka-Volterra food chain chemostat with converting time delays, Chaos Soliton. Fract., 42 (2009), 2786–2795. https://doi.org/10.1016/j.chaos.2009.03.189 doi: 10.1016/j.chaos.2009.03.189
![]() |
[7] |
G. P. Pang, F. Y. Wang, L. S. Chen, Study of Lotka-Volterra food chain chemostat with periodically varying dilution rate, J. Math. Chem., 43 (2008), 901–913. https://doi.org/10.1007/s10910-007-9263-5 doi: 10.1007/s10910-007-9263-5
![]() |
[8] | J. Monod, La technique de culture continue: theorie et applications, Ann. Inst. Pasteur., 79 (1950), 390–410. |
[9] | J. Monod, Recherches sur la croissance des cultures bacteriennes, Hermann, 1942. |
[10] |
G. S. K. Wolkowicz, Z. Q. Lu, Global dynamics of a mathematical model of competition in the chemostat: general response functions and differential death rates, SIAM J. Appl. Math., 52 (1992), 222–233. https://doi.org/10.1137/0152012 doi: 10.1137/0152012
![]() |
[11] |
B. T. Li, Global asymptotic behavior of the chemostat: general response functions and different removal rates, SIAM J. Appl. Math., 59 (1998), 411–422. https://doi.org/10.1137/S003613999631100X doi: 10.1137/S003613999631100X
![]() |
[12] |
B. Tang, G. Wolkowicz, Mathematical models of microbial growth and competition in the chemostat regulated by cell-bound extracellular enzymes, J. Math. Biol., 31 (1992), 1–23. https://doi.org/10.1007/BF00163841 doi: 10.1007/BF00163841
![]() |
[13] |
A. Rapaport, J. Harmand, Biological control of the chemostat with nonmonotonic response and different removal rates, Math. Biosci. Eng., 5 (2008), 539–547. https://doi.org/10.3934/mbe.2008.5.539 doi: 10.3934/mbe.2008.5.539
![]() |
[14] |
F. Mazenc, M. Malisoff, Stabilization of a chemostat model with haldane growth functions and a delay in the measurements, Automatica, 46 (2010), 1428–1436. https://doi.org/10.1016/j.automatica.2010.06.012 doi: 10.1016/j.automatica.2010.06.012
![]() |
[15] |
J. F. Andrews, A mathematical model for the continuous culture of microorganisms utilizing inhibitory substrates, Biotechnol. Bioeng., 10 (1968), 707–723. https://doi.org/10.1002/bit.260100602 doi: 10.1002/bit.260100602
![]() |
[16] |
Y. Sabbar, A. Zeb, N. Gul, D. Kiouach, S. Rajasekar, N. Ullah, et al., Stationary distribution of an sir epidemic model with three correlated brownian motions and general lévy measure, AIMS Mathematics, 8 (2023), 1329–1344. https://doi.org/10.3934/math.2023066 doi: 10.3934/math.2023066
![]() |
[17] |
Y. Sabbar, D. Kiouach, S. P. Rajasekar, Acute threshold dynamics of an epidemic system with quarantine strategy driven by correlated white noises and Lévy jumps associated with infinite measure, International Journal of Dynamics and Control, 11 (2023), 122–135. https://doi.org/10.1007/s40435-022-00981-x doi: 10.1007/s40435-022-00981-x
![]() |
[18] |
Y. Sabbar, M. Yavuz, F. Ozkose, Infection eradication criterion in a general epidemic model with logistic growth, quarantine strategy, media intrusion, and quadratic perturbation, Mathematics, 10 (2022), 4213. https://doi.org/10.3390/math10224213 doi: 10.3390/math10224213
![]() |
[19] |
A. Din, A. Khan, Y. Sabbar, Long-term bifurcation and stochastic optimal control of a triple-delayed ebola virus model with vaccination and quarantine strategies, Fractal Fract., 6 (2022), 578. https://doi.org/10.3390/fractalfract6100578 doi: 10.3390/fractalfract6100578
![]() |
[20] |
D. L. Zhao, S. L. Yuan, Sharp conditions for the existence of a stationary distribution in one classical stochastic chemostat, Appl. Math. Comput., 339 (2018), 199–205. https://doi.org/10.1016/j.amc.2018.07.020 doi: 10.1016/j.amc.2018.07.020
![]() |
[21] |
M. M. Gao, D. Q. Jiang, T. Hayat, A. Alsaedi, Threshold behavior of a stochastic Lotka-Volterra food chain chemostat model with jumps, Physica A, 523 (2019), 191–203. https://doi.org/10.1016/j.physa.2019.02.029 doi: 10.1016/j.physa.2019.02.029
![]() |
[22] |
X. J. Lv, X. Z. Meng, X. Z. Wang, Extinction and stationary distribution of an impulsive stochastic chemostat model with nonlinear perturbation, Chaos Soliton. Fract., 110 (2018), 273–279. https://doi.org/10.1016/j.chaos.2018.03.038 doi: 10.1016/j.chaos.2018.03.038
![]() |
[23] |
A. Khan, Y. Sabbar, A. Din, Stochastic modeling of the Monkeypox 2022 epidemic with cross-infection hypothesis in a highly disturbed environment, Math. Biosci. Eng., 19 (2022), 13560–13581. https://doi.org/10.3934/mbe.2022633 doi: 10.3934/mbe.2022633
![]() |
[24] |
Y. Sabbar, A. Khan, A. Din, Probabilistic analysis of a marine ecological system with intense variability, Mathematics, 10 (2022), 2262. https://doi.org/10.3390/math10132262 doi: 10.3390/math10132262
![]() |
[25] |
Y. Sabbar, A. Khan, A. Din, D. Kiouach, S. P. Rajasekar, Determining the global threshold of an epidemic model with general interference function and high-order perturbation, AIMS Mathematics, 7 (2022), 19865–19890. https://doi.org/10.3934/math.20221088 doi: 10.3934/math.20221088
![]() |
[26] |
D. L. Zhao, S. L. Yuan, H. D. Liu, Stochastic dynamics of the delayed chemostat with Levy noises, Int. J. Biomath., 12 (2019), 1950056. https://doi.org/10.1142/S1793524519500566 doi: 10.1142/S1793524519500566
![]() |
[27] |
X. F. Zhang, R. Yuan, A stochastic chemostat model with mean-reverting Ornstein-Uhlenbeck process and Monod-Haldane response function, Appl. Math. Comput., 394 (2021), 125833. https://doi.org/10.1016/j.amc.2020.125833 doi: 10.1016/j.amc.2020.125833
![]() |
[28] |
Z. W. Cao, X. D. Wen, H. S. Su, L. Y. Liu, Q. Ma, Stationary distribution of a stochastic chemostat model with Beddington-Deangelis functional response, Physica A, 554 (2020), 124634. https://doi.org/10.1016/j.physa.2020.124634 doi: 10.1016/j.physa.2020.124634
![]() |
[29] |
Y. Sabbar, D. Kiouach, S. P. Rajasekar, S. E. A. El-Idrissi, The influence of quadratic Lévy noise on the dynamic of an SIC contagious illness model: new framework, critical comparison and an application to COVID-19 (SARA-CoV-2) case, Chaos Soliton. Fract., 159 (2022), 112110. https://doi.org/10.1016/j.chaos.2022.112110 doi: 10.1016/j.chaos.2022.112110
![]() |
[30] |
D. Kiouach, Y. Sabbar, The long-time behaviour of a stochastic SIR epidemic model with distributed delay and multidimensional Levy jumps, Int. J. Biomath., 15 (2022), 2250004. https://doi.org/10.1142/S1793524522500048 doi: 10.1142/S1793524522500048
![]() |
[31] |
D. Kiouach, Y. Sabbar, Developing new techniques for obtaining the threshold of a stochastic SIR epidemic model with 3-dimensional Levy process, Journal of Applied Nonlinear Dynamics, 11 (2022), 401–414. https://doi.org/10.5890/JAND.2022.06.010 doi: 10.5890/JAND.2022.06.010
![]() |
[32] |
D. Kiouach, Y. Sabbar, S. E. A. El-idrissi, New results on the asymptotic behavior of an SIS epidemiological model with quarantine strategy, stochastic transmission, and Levy disturbance, Math. Method. Appl. Sci., 44 (2021), 13468–13492. https://doi.org/10.1002/mma.7638 doi: 10.1002/mma.7638
![]() |
[33] |
X. H. Zhang, K. Wang, Stochastic SIR model with jumps, Appl. Math. Lett., 26 (2013), 867–874. https://doi.org/10.1016/j.aml.2013.03.013 doi: 10.1016/j.aml.2013.03.013
![]() |
[34] |
Y. L. Zhou, W. G. Zhang, Threshold of a stochastic SIR epidemic model with Levy jumps, Physica A, 446 (2016), 204–216. https://doi.org/10.1016/j.physa.2015.11.023 doi: 10.1016/j.physa.2015.11.023
![]() |
[35] |
D. Kiouach, Y. Sabbar, Developing new techniques for obtaining the threshold of a stochastic SIR epidemic model with 3-dimensional Levy process, Journal of Applied Nonlinear Dynamics, 11 (2022), 401–414. https://doi.org/10.5890/JAND.2022.06.010 doi: 10.5890/JAND.2022.06.010
![]() |
[36] |
D. Kiouach, Y. Sabbar, The long-time behaviour of a stochastic SIR epidemic model with distributed delay and multidimensional Levy jumps, Int. J. Biomath., 15 (2022), 2250004. https://doi.org/10.1142/S1793524522500048 doi: 10.1142/S1793524522500048
![]() |
[37] |
D. Kiouach, Y. Sabbar, Dynamic characterization of a stochastic SIR infectious disease model with dual perturbation, Int. J. Biomath., 14 (2021), 2150016. https://doi.org/10.1142/S1793524521500169 doi: 10.1142/S1793524521500169
![]() |
[38] | I. I. Gihman, A. V. Skorohod, Stochastic differential equations, Berlin Heidelberg: Springer, 1972. |
[39] |
Y. Cheng, F. M. Zhang, M. Zhao, A stochastic model of HIV infection incorporating combined therapy of HARRT driven by Levy jumps, Adv. Differ. Equ., 2019 (2019), 321. https://doi.org/10.1186/s13662-019-2108-2 doi: 10.1186/s13662-019-2108-2
![]() |
[40] |
Y. Cheng, M. T. Li, F. M. Zhang, A dynamics stochastic model with HIV infection of CD4+ T-cells driven by Levy noise, Chaos Soliton. Fract., 129 (2019), 62–70. https://doi.org/10.1016/j.chaos.2019.07.054 doi: 10.1016/j.chaos.2019.07.054
![]() |
[41] |
N. T. Dieu, T. Fugo, N. H. Du, Asymptotic behaviors of stochastic epidemic models with jump-diffusion, Appl. Math. Model., 86 (2020), 259–270. https://doi.org/10.1016/j.apm.2020.05.003 doi: 10.1016/j.apm.2020.05.003
![]() |
[42] |
N. Privault, L. Wang, Stochastic SIR Levy jump model with heavy tailed increments, J. Nonlinear Sci., 31 (2021), 15. https://doi.org/10.1007/s00332-020-09670-5 doi: 10.1007/s00332-020-09670-5
![]() |
[43] |
G. J. Butler, G. S. K. Wolkowicz, A mathematical model of the chemostat with a general class of functions describing nutrient uptake, SIAM J. Appl. Math., 45 (1985), 138–151. https://doi.org/10.1137/0145006 doi: 10.1137/0145006
![]() |
[44] |
Q. L. Dong, W. B. Ma, M. J. Sun, The asymptotic behavior of a chemostat model with crowley-martin type functional response and time delays, J. Math. Chem., 51 (2013), 1231–1248. https://doi.org/10.1007/s10910-012-0138-z doi: 10.1007/s10910-012-0138-z
![]() |
[45] |
H. X. Li, J. H. Wu, Y. L. Li, C. A. Liu, Positive solutions to the unstirred chemostat model with crowley-martin functional response, AIMS Mathematics, 23 (2018), 2951–2966. https://doi.org/10.3934/dcdsb.2017128 doi: 10.3934/dcdsb.2017128
![]() |
[46] |
L. Wang, D. Q. Jiang, Ergodic property of the chemostat: a stochastic model under regime switching and with general response function, Nonlinear Analysis: Hybrid Systems, 27 (2018), 341–352. https://doi.org/10.1016/j.nahs.2017.10.001 doi: 10.1016/j.nahs.2017.10.001
![]() |
[47] |
L. Wang, D. Q. Jiang, A note on the stationary distribution of the stochastic chemostat model with general response functions, Appl. Math. Lett., 73 (2017), 22–28. https://doi.org/10.1016/j.aml.2017.04.029 doi: 10.1016/j.aml.2017.04.029
![]() |
[48] |
Y. Sabbar, A. Khan, A. Din, M. Tilioua, New method to investigate the impact of independent quadratic alpha-stable Poisson jumps on the dynamics of a disease under vaccination strategy, Fractal Fract., 7 (2023), 226. https://doi.org/10.3390/fractalfract7030226 doi: 10.3390/fractalfract7030226
![]() |
[49] |
Y. Sabbar, A. Zeb, D. Kiouach, N. Gul, T. Sitthiwirattham, D. Baleanu, et al., Dynamical bifurcation of a sewage treatment model with general higher-order perturbation, Results Phys., 39 (2022), 105799. https://doi.org/10.1016/j.rinp.2022.105799 doi: 10.1016/j.rinp.2022.105799
![]() |
[50] |
Q. Liu, D. Q. Jiang, T. Hayat, B. Ahmed, Periodic solution and stationary distribution of stochastic SIR epidemic models with higher order perturbation, Physica A, 482 (2017), 209–217. https://doi.org/10.1016/j.physa.2017.04.056 doi: 10.1016/j.physa.2017.04.056
![]() |
[51] |
Q. Liu, D. Q. Jiang, Stationary distribution and extinction of a stochastic SIR model with nonlinear perturbation, Appl. Math. Lett., 73 (2017), 8–15. https://doi.org/10.1016/j.aml.2017.04.021 doi: 10.1016/j.aml.2017.04.021
![]() |
[52] |
Q. Liu, D. Q Jiang, T. Hayat, B. Ahmed, Stationary distribution and extinction of a stochastic predator-prey model with additional food and nonlinear perturbation, Appl. Math. Comput., 320 (2018), 226–239. https://doi.org/10.1016/j.amc.2017.09.030 doi: 10.1016/j.amc.2017.09.030
![]() |
[53] |
S. G. Peng, X. H. Zhu, Necessary and sufficient condition for comparison theorem of 1-dimensional stochastic differential equations, Stoch. Proc. Appl., 116 (2006), 370–380. https://doi.org/10.1016/j.spa.2005.08.004 doi: 10.1016/j.spa.2005.08.004
![]() |
[54] |
N. T. Dieu, D. H. Nguyen, N. H. Du, G. Yin, Classification of asymptotic behavior in a stochastic SIR model, SIAM J. Appl. Dyn. Syst., 15 (2016), 1062–1084. https://doi.org/10.1137/15M1043315 doi: 10.1137/15M1043315
![]() |
[55] |
F. B. Xi, Asymptotic properties of jump-diffusion processes with state-dependent switching, Stoch. Proc. Appl., 119 (2009), 2198–2221. https://doi.org/10.1016/j.spa.2008.11.001 doi: 10.1016/j.spa.2008.11.001
![]() |
[56] | Y. A. Kutoyants, Statistical inference for ergodic diffusion processes, London: Springer, 2004. https://doi.org/10.1007/978-1-4471-3866-2 |
[57] | L. Stettner, On the existence and uniqueness of invariant measure for continuous time Markov processes, Brown University, 1986. |
[58] |
J. Y. Tong, Z. Z. Zhang, J. H. Bao, The stationary distribution of the facultative population model with a degenerate noise, Stat. Probabil. Lett., 83 (2013), 655–664. https://doi.org/10.1016/j.spl.2012.11.003 doi: 10.1016/j.spl.2012.11.003
![]() |
[59] | X. R. Mao, Stochastic differential equations and applications. Chichester: Elsevier, 2007. |
[60] |
D. H. Nguyen, N. N. Nguyen, G. Yin, General nonlinear stochastic systems motivated by chemostat models: complete characterization of long time behavior, optimal controls, and applications to wastewater treatment, Stoch. Proc. Appl., 130 (2020), 4608–4642. https://doi.org/10.1016/j.spa.2020.01.010 doi: 10.1016/j.spa.2020.01.010
![]() |
[61] |
N. B. Liberati, E. Platen, Strong approximations of stochastic differential equations with jumps, J. Comput. Appl. Math., 205 (2007), 982–1001. https://doi.org/10.1016/j.cam.2006.03.040 doi: 10.1016/j.cam.2006.03.040
![]() |
1. | Yassine Sabbar, Exploring threshold dynamics of a behavioral epidemic model featuring two susceptible classes and second-order jump–diffusion, 2024, 186, 09600779, 115216, 10.1016/j.chaos.2024.115216 |
Name | Expression | Sources |
Lotka Volterra | G(N(t))=N(t) | [6,7] |
Michaelis Menten (Monod) | G(N(t))=N(t)c+N(t), (c>0) | [8,9] |
Sigmoidal | G(N(t))=N2(t)(c1+N(t))(c2+N(t)), (c1,c2>0) | [10,11,12] |
Monod Haldane | G(N(t))=N(t)m1+N(t)+m2N2(t), (m1,m2>0) | [13,14,15] |
Name | Expression | |
Interaction type |
||
Interaction type |
||
Interaction type |
||
Beddington-DeAngelis | ||
Crowley-Martin |
Parameters | Domain | Units | Scenario 1 | Scenario 2 |
day | 1 | 7 | ||
mg of cells |
8 | 8 | ||
mg of cells |
60 | 60 | ||
mg of cells |
60 | 60 | ||
Dimensionless | 0.7 | 0.7 | ||
1/day | 0.06 | 0.06 | ||
– | ||||
– | mg of cells |
15 | 15 |
Intensity | Scenario 1 | Scenario 2 |
0.013 | 0.01 | |
0.04 | 0.02 | |
0.02 | 0.02 | |
0.03 | 0.01 | |
0.01 | 0.07 | |
0.04 | 0.01 | |
0.015 | 0.015 | |
0.013 | 0.011 |
Scenario | Critical value |
1 | 1.9888 |
2 | 2.7450 |
Name | Expression | Sources |
Lotka Volterra | G(N(t))=N(t) | [6,7] |
Michaelis Menten (Monod) | G(N(t))=N(t)c+N(t), (c>0) | [8,9] |
Sigmoidal | G(N(t))=N2(t)(c1+N(t))(c2+N(t)), (c1,c2>0) | [10,11,12] |
Monod Haldane | G(N(t))=N(t)m1+N(t)+m2N2(t), (m1,m2>0) | [13,14,15] |
Name | Expression | |
Interaction type |
||
Interaction type |
||
Interaction type |
||
Beddington-DeAngelis | ||
Crowley-Martin |
Parameters | Domain | Units | Scenario 1 | Scenario 2 |
day | 1 | 7 | ||
mg of cells |
8 | 8 | ||
mg of cells |
60 | 60 | ||
mg of cells |
60 | 60 | ||
Dimensionless | 0.7 | 0.7 | ||
1/day | 0.06 | 0.06 | ||
– | ||||
– | mg of cells |
15 | 15 |
Intensity | Scenario 1 | Scenario 2 |
0.013 | 0.01 | |
0.04 | 0.02 | |
0.02 | 0.02 | |
0.03 | 0.01 | |
0.01 | 0.07 | |
0.04 | 0.01 | |
0.015 | 0.015 | |
0.013 | 0.011 |
Scenario | Critical value |
1 | 1.9888 |
2 | 2.7450 |