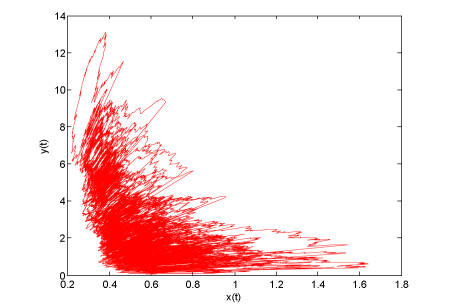
This paper reveals dynamical behaviors in the stochastic multimolecule oscillatory reaction model with Poisson jumps. First, this system is proved to have a unique global positive solution via the Lyapunov technique. Second, the existence and uniqueness of general random attractors for its stochastic homeomorphism flow is proved by the comparison theorem, and meanwhile, a criterion for the existence of singleton sets is obtained. Finally, numerical simulations are used to illustrate the predicted random attractors.
Citation: Yongchang Wei, Zongbin Yin. Long-time dynamics of a stochastic multimolecule oscillatory reaction model with Poisson jumps[J]. AIMS Mathematics, 2022, 7(2): 2956-2972. doi: 10.3934/math.2022163
[1] | Ranran Liu, Hui Liu, Jie Xin . Random attractors for stochastic discrete long wave-short wave resonance equations driven by fractional Brownian motions. AIMS Mathematics, 2021, 6(3): 2900-2911. doi: 10.3934/math.2021175 |
[2] | Ruonan Liu, Tomás Caraballo . Random dynamics for a stochastic nonlocal reaction-diffusion equation with an energy functional. AIMS Mathematics, 2024, 9(4): 8020-8042. doi: 10.3934/math.2024390 |
[3] | Kaihong Zhao . Attractor of a nonlinear hybrid reaction–diffusion model of neuroendocrine transdifferentiation of human prostate cancer cells with time-lags. AIMS Mathematics, 2023, 8(6): 14426-14448. doi: 10.3934/math.2023737 |
[4] | Ailing Ban . Asymptotic behavior of non-autonomous stochastic Boussinesq lattice system. AIMS Mathematics, 2025, 10(1): 839-857. doi: 10.3934/math.2025040 |
[5] | Xintao Li . Wong-Zakai approximations and long term behavior of second order non-autonomous stochastic lattice dynamical systems with additive noise. AIMS Mathematics, 2022, 7(5): 7569-7594. doi: 10.3934/math.2022425 |
[6] | Xin Liu . Stability of random attractors for non-autonomous stochastic p-Laplacian lattice equations with random viscosity. AIMS Mathematics, 2025, 10(3): 7396-7413. doi: 10.3934/math.2025339 |
[7] | Rou Lin, Min Zhao, Jinlu Zhang . Random uniform exponential attractors for non-autonomous stochastic Schrödinger lattice systems in weighted space. AIMS Mathematics, 2023, 8(2): 2871-2890. doi: 10.3934/math.2023150 |
[8] | Xintao Li, Yunlong Gao . Random uniform attractors for fractional stochastic FitzHugh-Nagumo lattice systems. AIMS Mathematics, 2024, 9(8): 22251-22270. doi: 10.3934/math.20241083 |
[9] | Abdelkader Moumen, Amin Benaissa Cherif, Fatima Zohra Ladrani, Keltoum Bouhali, Mohamed Bouye . Fourth-order neutral dynamic equations oscillate on timescales with different arguments. AIMS Mathematics, 2024, 9(9): 24576-24589. doi: 10.3934/math.20241197 |
[10] | Dan-Dan Dai, Wei Zhang, Yu-Lan Wang . Numerical simulation of the space fractional (3+1)-dimensional Gray-Scott models with the Riesz fractional derivative. AIMS Mathematics, 2022, 7(6): 10234-10244. doi: 10.3934/math.2022569 |
This paper reveals dynamical behaviors in the stochastic multimolecule oscillatory reaction model with Poisson jumps. First, this system is proved to have a unique global positive solution via the Lyapunov technique. Second, the existence and uniqueness of general random attractors for its stochastic homeomorphism flow is proved by the comparison theorem, and meanwhile, a criterion for the existence of singleton sets is obtained. Finally, numerical simulations are used to illustrate the predicted random attractors.
Mathematical models which depict dynamics of substrates have played an important role in understanding multimolecule reaction problems. E. E. Sel'kov [21] proposed a kinetic model of an open monosubstrate enzyme reaction with substrate inhibition and product activation. Its mechanism can be simplified as
[ˉA0]k1→A1,A1k2→0(output),pA1+qA2k3→(p+q)A2,A2k4→0(output), |
to describe the evolution of reaction concentrations of the two reactants A1 and A2. Here, the concentration of ˉA0 is assumed to be constant in time (and space). By the law of mass action and the law of mass conservation, the time evolution of reaction concentrations of A1 and A2 can be described by
{dxtdt=k1ˉx0−k2xt−k3xptyqt,dytdt=k3xptyqt−k4yt, | (1.1) |
where ˉx0 is the concentration of ˉA0 and ki(i=1,⋯,4) are kinetic parameters. Herein, all parameters involved in system (1.1) are assumed to be positive constants.
By taking the change of variables
X=k1/(p+q1)3x,Y=k1/(p+q−1)3y,α=k2,β=k4,δ=k1k1/(p+q−1)3ˉx0, |
and by rescaling with ˉx=δ(q−1)/pβ−q/pX, ˉy=δ−1βY and τ=δ1+(q−1)/pβ−q/pt, system (1.1) can be simplified as
{dˉxtdt=1−aˉxt−ˉxptˉyqt,dˉytdt=b(ˉxptˉyqt−ˉyt), | (1.2) |
where a=αδ−1−(q−1)/pβq/p, b=δ−1−(q−1)/pβ1+q/p and t is used to replace τ in common practice. When parameters a=0,p=n, and q=2, Zhang [27] proved that system (1.2) has a unique positive stable solution and further verified that system (1.1) produced stable limit cycles from Hopf bifurcations as n<b≪(3+2√2)n. Furthermore, Tang and Zhang [23] verified that system (1.2) occurs Bogdanov-Takens Bifurcation and obtains the corresponding universal unfolding. In addition, there are a lot of works which studied the dynamics of multimolecule oscillatory reactions, a paradigm of non-equilibrium dynamics [9,10,17,22,24].
Apparently, the above deterministic description of the chemical reaction model is insufficient since stochastic fluctuations in the concentrations of reactive species are inevitable. Nicolis and Prigogine [18] treated the chemical Brusselator as a Markov jump process, namely, the Markov jump Brusselator, which takes the discrete particle structure of the physico-chemical processes involved into account, but lump most of the microscopic information to "internal fluctuations". Thus, they obtained a mesoscopic model in terms of a Markov jump process (xt,yt) in state space R2+, where transition probabilities are described by the so-called master equation (or Kolmogorov's second equation). Interestingly, Nicolis and Prigogine showed that the Markovian process can be simplified as a Poissonian one if the transition events follow an exponential distribution. There have also existed studies on this non-Gaussian noise [4,5,13,25]. Besides, Yang et al. [26] proposed a reasonable, stochastic multimolecule reaction model driven by the Gaussian noise in the well-stirred case, they further established the criterions of the end of the reaction and continuous reaction conditions. Furthermore, Huang et al. [11] studied the permanence of the reaction and estimated the polynomial convergence rate of the transition probability to an invariant probability measure of stochastic system (1.1).
Given that deterministic system (1.1) has an oscillatory behavior, a natural issue is whether oscillations still exist in the stochastic counterpart of this system (i.e., the deterministic multimolecule reaction model is subjected to noise). Owing to the representative of the stochastic multimolecule reaction model, its random attractors are of particular interesting. Meanwhile, Lyapunov exponents play an important role since they can be used to detect the structure of the attractors [1,2]. By the stochastic bifurcation theory, Arnold et al. [2] investigated Lyapunov exponents of the Brusselator under Gaussian noise and its random attractors and bifurcation behavior using a numerical method. Schenk-Hoppé [20] proved the existence of random attractors in the random Duffing-van der Pol system and estimated the corresponding Lyapunov exponents.
In reality, the chemical reactions are usually subject to the same random factors such as temperature, humidity and other extrinsic influences [8]. Inspired by this fact, it is more plausible to introduce random perturbations into system (1.1) by replacing the kinetic parameters k2 and k4 by
−k2dt→−k2dt+σdBt+∫Uγ1(u)˜Nq(dt,du),and−k4dt→−k4dt+σdBt+∫Uγ2(u)˜Nq(dt,du) |
where ˜Nq(dt,du) denotes a compensated Poisson random measure corresponding to a Poisson measure Nq(dt,du) with characteristic measure dtν(du) on the product space [0,∞)×U, and ν is a finite characteristic measure, i.e., ν(U)<∞, and further assume that Bt and Nq(dt,du) are independent. Therefore, the following stochastic multimolecule oscillatory system (1.1) with Poisson jumps as q=1 and p=2 is given by
{dxt=(k1ˉx0−k2xt−k3x2tyt)dt+σxtdBt+∫Uγ1(u)xt−˜Nq(dt,du),dyt=(k3x2tyt−k4yt)dt+σytdBt+∫Uγ2(u)yt−˜Nq(dt,du), | (1.3) |
where xt−=lims↑txs.
We point out that the abundant dynamics of the stochastic multimolecule reaction model with the Gaussian noise have been revealed [2,6,7]. Meanwhile, note that we considered a stochastic low concentration trimolecular oscillatory chemical system with jumps and established the existence of the corresponded random attractors [25], but it is unknown whether random attractors are uniqueness in the stochastic multimolecule oscillatory reaction model with Poisson jumps. Furthermore, if they are unique, what is their structure? The present paper will address these questions and find new dynamical phenomena.
First, we establish the existence-uniqueness theorem of the positive solution of the stochastic multimolecule oscillatory reaction model with Poisson jumps. Then, we derive a sufficient set of conditions that ensure the existence and uniqueness of general random attractors in system (1.3). Moreover, we give the estimation of Lyapunov exponents, and obtain a singleton set random attractor by the estimated Lyapunov exponents. Finally, we introduce some numerical simulations to support the main results.
The rest of this paper is organized as follows. In Section 2, some preliminaries and definitions are formulated. Moreover, the global positive solutions of the stochastic multimolecule oscillatory reaction model with Poisson jumps are established. In Section 3, random attractors and Singleton sets for its stochastic homeomorphism flow are investigated. In Section 4, Numerical simulations are given.
In this section, we recall several basic concepts and definitions which will be needed throughout the paper.
Consider the following d-dimensional stochastic differential equations with Poisson jumps
dzt=A(zt)dt+B(zt)dW(t)+∫UC(zt−,u)˜Nq(dt,du), | (2.1) |
with the initial condition z0∈Rd. Assume that f∈C2(Rd), then the infinitesimal generator of the process zt to (2.1) is
Lf(z)=d∑i=1Ai(z)∂∂zif(z)+12d∑i,j=1[BT(z)B(z)]ij∂2∂zi∂zjf(x)+∫U[f(˜C(z,u))−f(z)−d∑i=1Ci(z,u)∂∂zif(z)]ν(du), |
where ˜C(z,u)=z+C(z,u), T denotes transiposition, and denote Rd+={x∈Rd:xi>0,i=1,2,⋯,d}.
Let (Ω,F,P) be a complete probability space. Define the shift operator
(θtω)(⋅)=ω(t+⋅)−ω(t),foranyt∈R,ω∈Ω, |
it is easy to verify that
θ0=idΩ,θt∘θs=θt+s,s,t∈R, |
and (t,ω)→θtω is B(R)⊗F/F-measurable. Therefore, {θt}t∈R is a group of measurable transformations of (Ω,F,P). Meanwhile, P is {θt}-invariant, i.e.,
P(θt−1A)=P(A),foranyA∈F,t∈R. |
Let {θt}t∈R be ergodic, then (Ω,F,P,{θt}t∈R) is a metric dynamical system.
Definition 2.1. A function φ:R×Ω×Rd→Rd is called a measurable random dynamical system over the metric dynamical system (Ω,F,P,{θt}t∈R), if φ is B(R)⊗F⊗B(Rn)/B(Rn)-measurable and if φ(t,ω) is càdlàg in t and forms a càdlàg cocycle over θt, i.e., for any s,t∈R and ω∈Ω
φ(0,ω)=idRd,φ(t+s,ω)=φ(t,θsω)∘φ(s,ω). | (2.2) |
Remark 2.1. According to Arnold [1], a RDS induces a skew product flow of measurable maps
Θt:Ω×Rd→Ω×Rd,(ω,z)→(θtω,φ(t,ω)z). |
The flow property Θt+s=Θt∘Θs follows from (2.2).
Definition 2.2. A family random closed set A(ω)ω∈Ω map Ω into nonempty closed subsets of Rd such that for any fixed x∈Rd, ω↦d(x,A(ω)):=inf{d(x,y)|y∈A(ω)} is F/B([0,∞])-measurable. Here we make the convention d(x,∅)=∞.
Definition 2.3. [19] Let φ be a càdlàg random dynamical system on Rd and D be an IC-system, the set A∈D is called a random attractor with domain of attraction D if
(i) A is invariant and compact, i.e., φ(t,ω)A(ω)=A(θtω) for all t∈R;
(ii) A attracts set C∈D, i.e., limt→∞d(φ(t,θ−tω)C(θ−tω)|A(ω))=0, where d(A|B)=supx∈Ainfy∈Bd(x,y) is the Hausdorff semimetric.
Next, the following theorem shows that the solutions of system (1.3) is positive and global. Throughout this paper, we assume that the coefficient satisfies
(H0):γi(u)>−1,i=1,2,foru∈U. |
(H1):2σ2+∫U[max(γ21(u),γ22(u))−2]ν(du)<2min{k2,k4},foru∈U. |
Theorem 2.1. Let Assumptions (H0) and (H1) hold, then there exists a unique positive solution (xt,yt) of system (1.3) for any initial value (x0,y0)∈R2+, t≥0 and the solution (xt,yt)∈R2+ almost surely.
Proof. As the drift coefficient of system (1.3) is locally Lipschitz continuous, it follows from Mao [15] that there exists a unique local solution (xt,yt) for any initial value (x0,y0)∈R2+ and t∈[0,τe], where τe is the explosion time. Now one shows that τe=∞, a.s. Let n0>0 be so sufficiently large that x0,y0 all lies within the interval [1n0,n0] which proves the solution is global. For each n≥n0, define a stopping time
τn=inf{t∈[0,τe):min{xt,yt}≤1normax{xt,yt}≥n}, |
clearly, τn is increasing as n↑∞. Set τ∞=limn→∞τn, hence τ∞≤τe, a.s. and it is sufficient to check that τ∞=∞. Define a C2-function V:R2+→R+∪{∞} by
V(x,y)=(x−1−logx)+(y−1−logy)+l1(x+y)22,l1>0, |
and it is easy to know that V(x,y)≥0 for any (x,y)∈R2+. Let T>0 be arbitrary, for any 0<t<τn∧T and (x,y)∈R2+, applying Itô's formula to system (1.3), one obtains
dV(x,y)=I1+I2+I3+σ(x+y−2)dBt, | (2.3) |
where
I1=k1ˉx0+k2+k4+σ2−k1ˉx0x+(l1k1ˉx0−k2)x+(l1k1ˉx0−k4)y∫U[γ1(u)+γ2(u)−log(1+γ1(u))−log(1+γ2(u))]ν(du), | (2.4) |
I2=(k3−l1k2−l1k4)xy+(l1σ2−l1k2−k3)x2+(l1σ2−l1k4)y2+l12∫U[(γ1(u)x+γ2(u)y)2−(x+y)2]ν(du) | (2.5) |
and
I3=∫U[γ1(u)x+γ2(u)x−log(1+γ1(u))−log(1+γ2(u))+l1(x+y)(γ1(u)x+γ2(u)y)+l12(γ1(u)x+γ2(u)y)2]˜Nq(dt,du). |
For (2.4), one gets
I1≤I4+(l1k1ˉx0−k2)x+(l1k1ˉx0−k4)y, | (2.6) |
where I4=k1ˉx0+k2+k4+σ2+∫U[γ1(u)+γ2(u)−log(1+γ1(u))−log(1+γ2(u))]ν(du) and there is some positive constant l2 such that I4≤l2.
For (2.5), one obtains
I2=−[l1k2+l1k4−k3−l1∫U(γ1(u)γ2(u)−2)ν(du)]xy−[l1(k2−σ2−12∫U(γ21(u)−2)ν(du))+k3]x2−l1[k4−σ2−12∫U(γ22(u)−2)ν(du)]y2. | (2.7) |
Moreover, by (H0) and (H1), one chooses some positive constant l1 such that the following inequality
l1k2+l1k4−l1∫U(γ1(u)γ2(u)−2)ν(du)>k3 |
hold. Therefore, according to (2.6) and (2.7), there exists a positive constant l3 such that
I1+I2≤l3,foranyx,y∈R+,0<t<τn∧T. | (2.8) |
Integrating (2.3) from 0 to τn∧T and taking expectation on both sides give
EV(xτn∧T,yτn∧T)≤V(x0,y0)+E∫τn∧T0l3dt≤V(x0,y0)+l3T. | (2.9) |
Meanwhile, note that
V(xτn∧T,yτn∧T)≥2min{l1n2+n−1−logn,l1n2+1n−1−log1n}:=2l4. | (2.10) |
It then follows from (2.9) and (2.10) that
V(x0,y0)+l3T≥EV(xτn∧T,yτn∧T)≥EV(xτn,yτn)I{τn≤T}≥3l4P(τn≤T), | (2.11) |
where I{τn≤T} is the indicator function of the set {τn≤T}. By the similar proof in [16], it gives
P(τ∞=∞)=1. |
Therefore, the stochastic flow (xt,yt) of system (1.3) is global to forward, and also implies that P{xt≥0,yt≥0foranyt≥0}=1.
Remark 2.2. Note that Bt has independent increments, ˜Nq(dt,du) is a stationary compensated Poisson random measure, and the coefficients of system (1.3) are independent of t, it is easy to know that (xt,yt) is a homogeneous Markov process. Moreover, it follows from Arnold [1] that system (1.3) can generate a stochastic dynamical system.
Remark 2.3. Define
Nq([t,0),B)=♯{t≤s<0,q(s)∈B},foranyB∈B(U∖{0}), |
where ♯ denotes the cardinality of a set. Therefore, the compensated Poisson random measure on (−∞,0]×U can be expressed by
˜Nq([t,0),du)=Nq([t,0),du)+ν(du)t,t∈(−∞,0]. |
From Qiao [19] and Kager [12], it follows that {q(t),t<0} is a stationary {F0t}t≤0−adapted Poisson point process with values in U∖{0}. Moreover, it follows from Arnold [1] that the solution zt to system (1.3) is Ft-measurable for any t∈R.
In this section, we show mainly the existence and uniqueness of tempered random attractors of system (1.3). First, we state some essential lemma which will be crucial for our analysis in the following content. Consider the following stochastic differential equations
{dzt=(a1zt+a2)dt+b1ztdBt+∫U(c1(u)zt−+c2(u))˜Nq(dt,du),zt=z0,ast=0, | (3.1) |
where c1(u) and c2(u) be bounded functions with c1(u)>−1.
Lemma 3.1. There exists a θt-invariant set ˜Ω∈F of Ω of full P measure such that for any ω∈˜Ω and ∫U[log(1+c1(u))−c1(u)]ν(du)<12b21−a1,
(i) the random variable |z(ω)| is tempered.
(ii) the mapping
(t,ω)→¯zt(ω)=∫t−∞H(t,s)(a2−∫Uc1(u)c2(u)1+c1(u)ν(du))ds+∫t−∞∫UH(t,s)c2(u)1+c1(u)˜Nq(ds,du) |
is a stationary solution of system (3.1) with continuous trajectories and
H(t,s)=exp[(a1−12b21+∫U[log(1+c1(u))−c1(u)]ν(du))(t−s)−b1(Bs−Bt)−∫st∫Ulog(1+c1(u))˜Nq(ds,du)]. | (3.2) |
Proof. (i) By the variation of constant formula [4], the solution of system (3.1) is explicitly expressed by
φ(t,ω)z0=Φ(t)[z0+∫t0Φ−1(s)(a2−∫Uc1(u)c2(u)1+c1(u)ν(du))ds+∫t0∫UΦ−1(s)c2(u)1+c1(u)˜Nq(ds,du)], | (3.3) |
where
Φ(t)=exp[(a1−12b21+∫U[log(1+c1(u))−c1(u)]ν(du))t+b1Bt+∫t0∫Ulog(1+c1(u))˜Nq(ds,du)]. | (3.4) |
Denote
I5=∫t0∫Ulog(1+c1(u))˜Nq(ds,du),I6=∫t0∫Uc2(u)1+c1(u)˜Nq(ds,du). |
Note that
⟨I5⟩(t)=∫t0∫Ulog2(1+c1(u))ν(du)ds≤l5t,⟨I6⟩(t)=∫t0∫U(c2(u)1+c1(u))2ν(du)ds≤l6t, |
where l5 and l6 are positive constants.
According to the strong law of large numbers [14], one obtains
limt→∞1t∫t0∫Ulog(1+c1(u))˜Nq(ds,du)=limt→∞1t∫t0∫Uc2(u)1+c1(u)˜Nq(ds,du)=0,a.s. |
Since limt→∞Btt=0,a.s. and a1−12b21+∫U[log(1+c1(u))−c1(u)]ν(du)<0, one has the following integrals:
∫0−∞Φ−1(t)(a2−∫Uc1(u)c2(u)1+c1(u)ν(du))dt<∞,a.s., |
∫0−∞∫UΦ−1(t)c2(u)1+c1(u)˜Nq(dt,du)<∞,a.s. |
Meanwhile, (3.1) is affine with stable linear part. Thus (3.3) and Arnold [1] imply that for any z0∈R, the unique invariant measure μ of (3.1) is the Dirac measure supported by
z(ω)=limt→∞φ(−t,ω)−1z0=∫0−∞Φ−1(t)(a2−∫Uc1(u)c2(u)1+c1(u)ν(du))dt+∫0−∞∫UΦ−1(t)c2(u)1+c1(u)˜Nq(dt,du), |
where
Φ(t)=exp[(a1−12b21+∫U[log(1+c1(u))−c1(u)]ν(du))t+b1Bt+∫t0∫Ulog(1+c1(u))˜Nq(ds,du)]. |
Therefore, it follows from Arnold [1] that limt→∞log+|z(θtω)|t=0, ω∈˜Ω. That is, the random variable |z(ω)| is tempered.
(ii) It is easy to see that H(t,s)=Φ(t)Φ−1(s), then one has
ˉzt(ω)=Φ(t)[∫t−∞Φ−1(s)(a2−∫Uc1(u)c2(u)1+c1(u)ν(du))ds+∫t−∞∫UΦ−1(s)c2(u)1+c1(u)˜Nq(ds,du)]=Φ(t)(z(ω)+∫t0Φ−1(s)(a2−∫Uc1(u)c2(u)1+c1(u)ν(du))ds+∫t0∫UΦ−1(s)c2(u)1+c1(u)˜Nq(ds,du)]=φ(t,ω)z(ω),a.s. | (3.5) |
Besides, denote s=r+t, then H(t,s) can be written as
H(t,s)=exp[−(a1−12b21+∫U[log(1+c1(u))−c1(u)]ν(du))r−b1(Br+t−Bt)−∫r+tt∫Ulog(1+c1(u))˜Nq(ds,du)]=exp[−(a1−12b21+∫U[log(1+c1(u))−c1(u)]ν(du))r−b1ˆBr−∫r0∫Ulog(1+c1(u))˜Nˆq(dt,du)]=Φ−1(r) | (3.6) |
where ˆBr=Br+t−Bt and ˆqr=qr+t−qt.
Therefore,
ˉzt(ω)=∫0−∞Φ−1(t)(a2−∫Uc1(u)c2(u)1+c1(u)ν(du))dt+∫0−∞∫UΦ−1(t)c2(u)1+c1(u)˜Nˆq(dt,du)=z(θtω). | (3.7) |
According to Schenk-Hoppé [20], (3.5) and (3.7) imply that ˉzt(ω) is a measurable stable stationary solution of system (3.1).
Moreover, it follows from Arnold [1] that ¯zt(ω) are continuous trajectories with respect to (t,ω), ω∈˜Ω.
The following theorem is established for the unique tempered random attractor of system (1.3).
Theorem 3.1. If the assumption (H0) and (H1) are satisfied. Further assume that for any u∈U
(H2)ˆk=min{k2,k4},ˆγ(u)=γ1(u)∧γ2(u),ˇγ(u)=γ1(u)∨γ2(u) |
and
(H3)∫U2[log(1+ˇγ(u))−ˆγ(u)]ν(du)<σ2+2ˆk. |
Then the random dynamical system generated by system (1.3) has the unique tempered random attractor A(ω)ω∈Ω with domain of attraction D(A) containing the universe of sets Cl(U), generated by
U={(D(ω))ω∈Ω|D(ω)⊂R2+isatemperedrandomset}. |
Furthermore, the random attractor A(ω)ω∈Ω of system (1.3) is measurable with respect to F0−∞=σ{Bt,Nq([t,0),B);t≤0}.
Proof. Define V(x,y)=(x+y)2, applying Itô's formula, one obtains
V(xt,yt)=V(x0,y0)+∫t0[2(xs+ys)(k1ˉx0−k2xs−k4ys)+σ2x2s+σ2y2s]ds+∫t02σ(xs+ys)2dBs+∫t0∫U[(xs+γ1(u)xs+ys+γ2(u)ys)2−(xs+ys)2−2(xs+ys)(xs+γ1(u)xs+ys+γ2(u)ys)]ν(du)ds+∫t0∫U[(xs−+γ1(u)xs−+ys−+γ2(u)ys−)2−(xs−+ys−)2]˜Nq(ds,du)=V(x0,y0)+∫t0[2(xs+ys)(k1ˉx0−k2xs−k4ys)+σ2x2s+σ2y2s]ds+2σ∫t0(xs+ys)2dBs−2∫t0∫U(xs+ys)(xs+γ1(u)xs+ys+γ2(u)ys)]ν(du)ds+∫t0∫U[(xs−+γ1(u)xs−+ys−+γ2(u)ys−)2−(xs−+ys−)2]Nq(ds,du). |
By the comparison theorem [5] and (H2), it follows that for any (x0,y0)∈R2+
V(xt,yt)≤V(x0,y0)+∫t0[(−2ˆk+σ2−2∫Uˆγ(u)ν(du))(xs+ys)2−2σ2xsys+2k1¯x0(xs+ys)]ds+∫t02σ(xs+ys)2dBs+∫t0∫U(2+ˇγ(u))ˇγ(u)(xs−+ys−)2Nq(ds,du)=V(x0,y0)+∫t0[I7V(xs,ys)+I8]ds+∫t02σV(xs,ys)dBs+∫t0∫UI9(u)V(xs−,ys−)˜Nq(ds,du) | (3.8) |
where I7=−2ˆk+σ2+∫U[2ˇγ(u)+ˇγ2(u)−2ˆγ(u)]ν(du), I8=−2σ2xsys+2k1(xs+ys)ˉx0 and I9(u)=(2+ˇγ(u))ˇγ(u).
According to I8, it follows that there exists a positive constant l7 such that I8≤l7.
Let φ(t,ω)(x0,y0) be the stochastic dynamical systems generated by system (1.3) and ψ(t,ω)V(x0,y0) be generated by the following system
V(xt,yt)=V(x0,y0)+∫t0(I7V(xs,ys)+l7)ds+∫t02σV(xs,ys)dBs+∫t0∫UI9(u)V(xs−,ys−)˜Nq(ds,du). | (3.9) |
Applying the variation of constant formula to system (3.9), one immediately gets
ψ(t,ω)V(x0,y0)=Φ1(t){V(x0,y0)+∫t0l7Φ−11(s)ds} | (3.10) |
where
Φ1(t)=exp[(I7−2σ2+∫U[log(1+I9(u))−I9(u)]ν(du))t+2σBt+∫t0∫Ulog(1+I9(u))˜Nq(ds,du)]. |
Thus, it follows from (3.8) that the associated stochastic system (3.9) has the following dominating solution ψ, i.e.,
V(φ(t,ω)(x0,y0))≤ψ(t,ω)V(x0,y0). | (3.11) |
By Lemma 3.1 and (H3), one shows that ψ(t,ω) has the unique invariant measure μ supported by
z1(ω)=∫0−∞l7Φ−11(t)dt. | (3.12) |
It follows from Lemma 3.1 (i) that z1(ω)≥0, and it attracts any points with exponential speed. Therefore, it is easy to verify that ψ(t,θ−tω)z1(θ−tω)→z1(ω) as t→∞ for any z1(ω) with e−ϵtz1(θ−tω)→0, ϵ>0.
Let η(⋅):Ω→R+, η(ω)=sup{z|z∈I(ω)} with limt→∞log+η(θtω)t=0. Define the universe of sets by
U1={I(ω)⊂R+isatemperedrandomset}. | (3.13) |
From Schenk-Hoppé[20], there exists a ϵ>0 such that e−ϵtz(θ−tω)→0 as t→∞. Meanwhile, it is clear to see that U1 is closed under inclusion and Ω×{z}∈U1 for any deterministic point {z}⊂R+.
From the definition of U1 and the above same reference, it follows that the random set [0,(1+ϵ)z1(ω)] is absorbing w.r.t. the universe U1. Note further that for any 0<x<y
ψ(t,ω,0)>0andψ(t,ω)x<ψ(t,ω)y. |
According to the proof of Lemma 3.1 (ii), one obtains by (3.10) and (3.12)
ψ(t,θ−tω)(1+ϵ)z1(θ−tω)≤(1+ϵ)z1(ω), | (3.14) |
that is, the random set [0,(1+ϵ)z1(ω)] is forward invariant for ψ w.r.t. the universe U1.
Similar to the proof of (3.14), one gets
e−ϵtz1(θ−tω)→0,ast→∞, |
for any ϵ>0. Therefore, [0,(1+ϵ)z1(ω)]∈U1.
Let B(ω) be a compact set of R2+ defined by
B(ω)=V−1([0,(1+ϵ)z1(ω)]),foranyϵ>0. |
It is easy to see that B(ω) is non-empty and bounded if the pre-images of sets is bounded.
Next, one proves that the family {B(ω)}ω∈Ω satisfies the conditions of Schenk-Hoppé [20].
In fact, it follows from Schenk-Hoppé [20] that B(ω) is measurability and forward invariant, i.e.,
ψ(t,θ−tω)B(θ−tω))⊂B(ω). |
Moreover, the definition of U implies that the variable η(ω)=sup{√x2+y2:v=(x,y)∈U} grows sub-exponentially. For any C∈U, note that V(x,y)=(x+y)2≤2|v|2, thus one further proves that the random variable satisfies sup{v:v∈V(C(ω))}≤2η(ω) which grows sub-exponentially, that is, V(C)∈U1.
Similarly, one gets that there exists a t(ω,V(C)) such that for any t≥t(ω,C)
V(φ(t,θ−tω)C(θ−tω))⊂ψ(t,θ−tω)V(C(θ−tω))⊂[0,(1+ϵ)z1(ω)]=V(B(ω)), | (3.15) |
Meanwhile, from the fact that z1(θ−tω)≤z1(ω) and the set [0,(1+ϵ)z1(ω)] absorbing any set in U1, which implies the absorbtion of any set w.r.t. φ in U.
For any C∈U, there exists a random variable ϑ(ω)>0 such that C(ω)⊂D(ϑ(ω))={(x,y)∈R2+,√x2+y2≤ϑ(ω)}. It is easy to see that D(ˆbρ(ω))∈U and D(ˆbρ(ω)) is a neighborhood of C(ω) for any ˆb>1.
Note that z1(ω) is F0−∞ measurable, so is A(ω). Therefore, it gives the complete proof in Schenk-Hoppé [20].
Remark 3.1. Compared to Wei [25], this paper considers the compensated Poisson random measure and further establishes the unique attractor for the stochastic multimolecule oscillatory reaction model.
Based on Theorem 3.1, the following theorem obtains the singleton sets random attractor of system (1.3).
Theorem 3.2. Let (H0)−(H2) hold, if the following assumption
(H4):k1ˉx0<σ2and∫U2[log(1+ˇγ(u))−ˆγ(u)]ν(du)<2ˆk |
hold, then the random dynamical system generated by system (1.3) has the unique tempered random attractor A(ω)={(0,0)} with domain of attraction D(A) containing the universe of sets Cl(U), which is given by
U={(D(ω))ω∈Ω|D(ω)⊂R2+isatemperedrandomset}. |
In particular, for any (x,y)∈R2+, the solutions φ(t,θ−tω)(x0,y0) and φ(t,ω)(x0,y0) tend to {(0,0)} with exponential speed as t→∞.
Proof. Define a function V(x,y)=(x+y)2. According to the proof of Theorem 3.1, one gets
dV(xt,yt)≤[I7V(xt,yt)+I8]dt+2σV(xt,yt)dBt+∫UI9(u)V(xt−,yt−)˜Nq(dt,du) | (3.16) |
where I7=−2ˆk+σ2+∫U[2ˇγ(u)+ˇγ2(u)−2ˆγ(u)]ν(du), I8=−2σ2xsys+2k1(xs+ys)ˉx0 and I9(u)=(2+ˇγ(u))ˇγ(u).
Moreover, denote
Ω1={(x,y)|x+y≥1,x≥0,y≥0},Ω2={(x,y)|x+y<1,x≥0,y≥0}, |
then R2+=Ω1∪Ω2. For (x,y)∈Ω1, one has
dV(xt,yt)≤(I7+2k1ˉx0)V(xt,yt)dt+2σV(xt,yt)dBt+∫UI9(u)V(xt−,yt−)˜Nq(dt,du). |
For (x,y)∈Ω2, by (H4), it is easy to verify that I8≤0. Therefore, one further obtains by the comparison theorem for any (x,y)∈R2+
dV(xt,yt)≤(I7+2k1ˉx0)V(xt,yt)dt+2σV(xt,yt)dBt+∫UI9(u)V(xt−,yt−)˜Nq(dt,du). | (3.17) |
According to Lemma 3.1, (3.17), (H0)–(H2) and (H4), the top Lyapunov exponent λ1 of system (1.3) can be bounded by
λ1=limt→∞log(x2t+y2t)2t≤limt→∞logV(xt,yt)2t≤12(I7−2σ2+2k1ˉx0+∫U[log(1+I9(u))−I9(u)]ν(du))+limt→∞log|V(x0,y0)|2t+limt→∞2σBt2t+limt→∞∫t0∫UI9(u)˜Nq(dt,du)2t=12(−2ˆk−σ2+2k1ˉx0+∫U2[log(1+ˇγ(u))−ˆγ(u)]ν(du))<0. |
From Lemma 3.1, one gets that the stochastic dynamical system generated by the following stochastic system
dzt=(I7+2k1ˉx0)V(xt,yt)dt+2σV(xt,yt)dBt+∫UI9(u)V(xt−,yt−)˜Nq(dt,du) |
has the unique invariant measure δ0. Similar to the proof of Theorem 3.1, it shows that A(ω)={(0,0)}=V−1(0,0) is an attractor.
In particular, for any (x0,y0)∈R2+, the solutions φ(t,θ−tω)(x0,y0) and φ(t,ω)(x0,y0) tend to A(ω) with exponential speed as t→∞.
In this section, we introduce mainly some examples and numerical simulations to support the main results.
Example 4.1. Let us illustrate the conditions of the unique tempered random attractor for Theorem 3.1. Choosing
k1=1.1,k2=2.5,ˉx0=2.2,k3=2.15,k4=0.65,σ=0.5,γ1(u)=γ2(u)=0.55andν(U)=0.65, |
implies that the conditions (H0)−(H3) are satisfied. It follows from Theorem 3.1 that system (1.3) has a unique random attractor. Applying the Infinitesimal method [28] and [3] to simulate (1.3), the simulated of system (1.3) is shown Figure 1. Meanwhile, the numerical simulations of the different samples xt and yt are shown as in Figure 2 (a) and (b) .
Example 4.2. Let us illustrate the conditions of the singleton set random attractor for Theorem 3.2. Choosing
k1=0.1,k2=1.5,ˉx0=0.2,k3=1.15,k4=0.1,σ=0.2γ1(u)=γ2(u)=0.35andν(U)=0.75, |
implies that the conditions (H0)−(H2) and (H4) are satisfied. then system (1.3) has a singleton set random attractor by Theorem 3.2. Moreover, the simple simulated orbit of system (1.3) is as shown in Figure 3.
Note that we established the existence of the corresponded random attractors in a stochastic low concentration trimolecular oscillatory chemical system[25], but the uniqueness is unknown. Based on this fact, we considered a stochastic multimolecule oscillatory reaction model with Poisson jumps in this paper. We have not only obtained that the multimolecule oscillatory reaction model with Poisson jumps has a unique global positive solution, but also importantly obtained the existence and uniqueness of random attractors in the stochastic multimolecule oscillatory reaction model, by using a technique similar to the Lyapunov's direct method.
This work is partly supported through the National Natural Science Foundation of China (No.11871118) and National Natural Science Foundation of China (No.11801096).
All authors declare no conflicts of interest in this paper.
[1] | L. Arnold, Random dynamical systems, Berlin: Springer, 1998. doi: 10.1007/978-3-662-12878-7. |
[2] | L. Arnold, G. Bleckert, K. R. Schenk-Hoppe, The stochastic Brusselator: parametric noise destroys Hopf bifurcation, In: Stochastic dynamics, Berlin: Springer, 1999, 71–92. doi: 10.1007/0-387-22655-9-4. |
[3] |
V. Bally, D. Talay, The law of the euler scheme for stochastic differential equations, Probab. Th. Rel. Fields, 104 (1996), 43–60. doi: 10.1007/BF01303802. doi: 10.1007/BF01303802
![]() |
[4] |
J. Bao, X. Mao, G. Yin, C. Yuan, Completive Lotak-Volterra population dynamics with jump, Nonlinear Anal. Theor., 74 (2011), 6601–6616. doi: 10.1016/j.na.2011.06.043. doi: 10.1016/j.na.2011.06.043
![]() |
[5] |
J. Bao, C. Yuan, Comparison theorem for stochastic differential delay equations with jumps, Acta Appl. Math., 116 (2011), 119. doi: 10.1007/s10440-011-9633-7. doi: 10.1007/s10440-011-9633-7
![]() |
[6] |
T. Biancalani, D. Fanelli, F. Di Patti, Stochastic Turing patterns in the Brusselator model, Phys. Rev. E, 81 (2010), 046215. doi: 10.1103/PhysRevE.81.046215. doi: 10.1103/PhysRevE.81.046215
![]() |
[7] | D. A. Dawson, Galerkin approximation of nonlinear Markov processes, In: Statistics and related topics, North Holland: Amsterdam, 1981,317–339. |
[8] |
N. T. Dieu, N. H. Du, G. Yin, Classification of asymptotic behavior in a stochastic SIR model, SIAM J. Appl. Dyn. Syst., 15 (2016), 1062–1084. doi: 10.1137/15M1043315. doi: 10.1137/15M1043315
![]() |
[9] |
A. A. Golovin, B. J. Matkowski, V. A. Volpert, Turing pattern formation in the Brusselator model with superdiffusion, SIAM J. Appl. Math., 69 (2008), 251–272. doi: 10.1137/070703454. doi: 10.1137/070703454
![]() |
[10] | B. D. Hassard, N. D. Kazarinoff, Y. H. Wan, Theory and applications of Hopf bifurcation, Cambridge: Cambridge University Press, 1981. |
[11] |
Z. Huang, J. Cao, G. Long, Long-time behavior of stochastic multimolecular reaction model, Physica A, 503 (2018), 331–344. doi: 10.1016/j.physa.2018.02.164. doi: 10.1016/j.physa.2018.02.164
![]() |
[12] |
G. Kager, M. K. R. Scheutzow, Generation of one-sided random dynamical systems by stochastic differential equations, Electron. J. Probab., 2 (1997), 1–17. doi: 10.1214/EJP.v2-22. doi: 10.1214/EJP.v2-22
![]() |
[13] |
G. Li, Q. Yang, Y. Wei, Dynamics of stochastic heroin epidemic model with lévy jumps, J. Appl. Anal. Comput., 8 (2018), 998–1010. doi: 10.11948/2018.998. doi: 10.11948/2018.998
![]() |
[14] |
P. Lipster, A strong law of large numbers for local martingales, Stachastics, 3 (1980), 217–218. doi: 10.1080/17442508008833146. doi: 10.1080/17442508008833146
![]() |
[15] | X. Mao, Stochastic differential equations and their applications, Horwood: Chinester, 2007. doi: 10.1533/9780857099402. |
[16] |
X. Mao, G. Marion, E. Renshaw, Environmental noise suppresses explosion in population dynamics, Stoch. Proc. Appl., 97 (2002), 95–110. doi: 10.1016/S0304-4149(01)00126-0. doi: 10.1016/S0304-4149(01)00126-0
![]() |
[17] | J. Nee, A note for the solution of the Brusselator equation, Contemporary Analysis and Applied Mathematics, 1 (2013), 49–59. |
[18] | G. Nicolis, I. Prigogine, Self-organization in nonequilibrium systems, New York: Wiley, 1997. |
[19] |
H. Qiao, J. Duan, Topological equivalence for discontinuous random dynamical systems and applications, Stoch. Dyn., 14 (2014), 1350007. doi: 10.1142/S021949371350007X. doi: 10.1142/S021949371350007X
![]() |
[20] |
K. R. Schenk-Hoppé, Random attractors-general properties, existence and applications to stochastic bifurcation theory, Discrete Cont. Dyn. Syst., 4 (1998), 99–130. doi: 10.3934/dcds.1998.4.99. doi: 10.3934/dcds.1998.4.99
![]() |
[21] |
E. E. Sel'kov, Self-oscillations in glycolysis. 1. A simple kinetic model, Eur. J. Biochem., 4 (1968), 79–86. doi: 10.1111/j.1432-1033.1968.tb00175.x. doi: 10.1111/j.1432-1033.1968.tb00175.x
![]() |
[22] |
M. Sun, Y. Tan, L. Chen, Dynamical behaviors of the brusselator system with impulsive input, J. Math. Chem., 44 (2008), 637–649. doi: 10.1007/s10910-008-9362-y. doi: 10.1007/s10910-008-9362-y
![]() |
[23] |
Y. Tang, W. Zhang, Bogdanov-Takens bifurcation of a polynomial differential system in biochemical reaction, Comput. Math. Appl., 48 (2004), 869–883. doi: 10.1016/j.camwa.2003.05.012. doi: 10.1016/j.camwa.2003.05.012
![]() |
[24] |
J. J. Tyson, J. C. Light, Properties of two-component bimolecular and trimolecular chemical reaction systems, J. Chem. Phys., 59 (1973), 4164. doi: 10.1063/1.1680609. doi: 10.1063/1.1680609
![]() |
[25] |
Y. Wei, Q. Yang, Dynamics of the stochastic low concentration trimolecular oscillatory chemical system with jumps, Commun. Nonlinear Sci. Numer. Simulat., 59 (2018), 396–408. doi: 10.1016/j.cnsns.2017.11.019. doi: 10.1016/j.cnsns.2017.11.019
![]() |
[26] |
Y. Yang, Y. Zhao, D. Jiang, The dynamics of the stochastic multi-molecule biochemical reaction model, J. Math. Chem., 52 (2014), 1477–1495. doi: 10.1007/s10910-014-0324-2. doi: 10.1007/s10910-014-0324-2
![]() |
[27] |
W. Zhang, Existence of closed orbits for a differential equation model concerning multimolecule, Appl. Math. Mech., 14 (1993), 589–596. doi: 10.1007/BF02451369. doi: 10.1007/BF02451369
![]() |
[28] |
X. Zou, K. Wang, Numerical simulations and modeling for stochastic biological systems with jumps, Commun. Nonlinear Sci. Numer. Simulat., 19 (2014), 1557–1568. doi: 10.1016/j.cnsns.2013.09.010. doi: 10.1016/j.cnsns.2013.09.010
![]() |