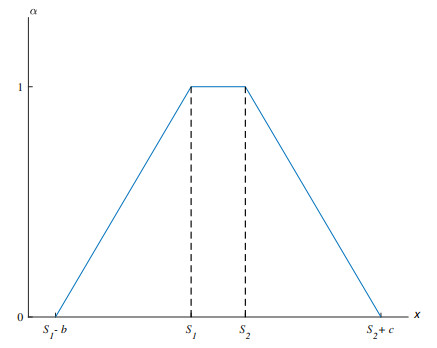
In this paper, we develop a Malliavin calculus approach for hedging a fixed strike lookback option in fuzzy space. Due to the uncertainty in financial markets, it is not accurate to describe the problems of option pricing and hedging in terms of randomness alone. We consider a fuzzy pricing model by introducing a fuzzy stochastic differential equation with Skorohod sense. In this way, our model simultaneously involves randomness and fuzziness. A well-known hedging strategy for vanilla options is so-called Δ-hedging, which is usually derived from the Itô formula and some properties of partial differentiable equations. However, when dealing with some complex path-dependent options (such as lookback options), the major challenge is that the payoff function of these options may not be smooth, resulting in the estimates are computationally expensive. With the help of the Malliavin derivative and the Clark-Ocone formula, the difficulty will be readily solved, and it is also possible to apply this hedging strategy to fuzzy space. To obtain the explicit expression of the fuzzy hedging portfolio for lookback options, we adopt the Esscher transform and reflection principle techniques, which are beneficial to the calculation of the conditional expectation of fuzzy random variables and the payoff function with extremum, respectively. Some numerical examples are performed to analyze the sensitivity of the fuzzy hedging portfolio concerning model parameters and give the permissible range of the expected hedging portfolio of lookback options with uncertainty by a financial investor's subjective judgment.
Citation: Kefan Liu, Jingyao Chen, Jichao Zhang, Yueting Yang. Application of fuzzy Malliavin calculus in hedging fixed strike lookback option[J]. AIMS Mathematics, 2023, 8(4): 9187-9211. doi: 10.3934/math.2023461
[1] | Min-Ku Lee, Jeong-Hoon Kim . Closed-form approximate solutions for stop-loss and Russian options with multiscale stochastic volatility. AIMS Mathematics, 2023, 8(10): 25164-25194. doi: 10.3934/math.20231284 |
[2] | Min-Ku Lee, Jeong-Hoon Kim . Pricing vanilla, barrier, and lookback options under two-scale stochastic volatility driven by two approximate fractional Brownian motions. AIMS Mathematics, 2024, 9(9): 25545-25576. doi: 10.3934/math.20241248 |
[3] | Zhidong Guo, Xianhong Wang, Yunliang Zhang . Option pricing of geometric Asian options in a subdiffusive Brownian motion regime. AIMS Mathematics, 2020, 5(5): 5332-5343. doi: 10.3934/math.2020342 |
[4] | Xinyi Wang, Chunyu Wang . Pricing geometric average Asian options in the mixed sub-fractional Brownian motion environment with Vasicek interest rate model. AIMS Mathematics, 2024, 9(10): 26579-26601. doi: 10.3934/math.20241293 |
[5] | Xiaodong Zhang, Junfeng Liu . Solving a class of high-order fractional stochastic heat equations with fractional noise. AIMS Mathematics, 2022, 7(6): 10625-10650. doi: 10.3934/math.2022593 |
[6] | Yao Fu, Sisi Zhou, Xin Li, Feng Rao . Multi-assets Asian rainbow options pricing with stochastic interest rates obeying the Vasicek model. AIMS Mathematics, 2023, 8(5): 10685-10710. doi: 10.3934/math.2023542 |
[7] | Hua Zhao, Yue Xin, Jinwu Gao, Yin Gao . Power-barrier option pricing formulas in uncertain financial market with floating interest rate. AIMS Mathematics, 2023, 8(9): 20395-20414. doi: 10.3934/math.20231040 |
[8] | Chao Yue, Chuanhe Shen . Fractal barrier option pricing under sub-mixed fractional Brownian motion with jump processes. AIMS Mathematics, 2024, 9(11): 31010-31029. doi: 10.3934/math.20241496 |
[9] | Guiwen Lv, Ping Xu, Yanxue Zhang . Pricing of vulnerable options based on an uncertain CIR interest rate model. AIMS Mathematics, 2023, 8(5): 11113-11130. doi: 10.3934/math.2023563 |
[10] | Ming Yang, Yin Gao . Pricing formulas of binary options in uncertain financial markets. AIMS Mathematics, 2023, 8(10): 23336-23351. doi: 10.3934/math.20231186 |
In this paper, we develop a Malliavin calculus approach for hedging a fixed strike lookback option in fuzzy space. Due to the uncertainty in financial markets, it is not accurate to describe the problems of option pricing and hedging in terms of randomness alone. We consider a fuzzy pricing model by introducing a fuzzy stochastic differential equation with Skorohod sense. In this way, our model simultaneously involves randomness and fuzziness. A well-known hedging strategy for vanilla options is so-called Δ-hedging, which is usually derived from the Itô formula and some properties of partial differentiable equations. However, when dealing with some complex path-dependent options (such as lookback options), the major challenge is that the payoff function of these options may not be smooth, resulting in the estimates are computationally expensive. With the help of the Malliavin derivative and the Clark-Ocone formula, the difficulty will be readily solved, and it is also possible to apply this hedging strategy to fuzzy space. To obtain the explicit expression of the fuzzy hedging portfolio for lookback options, we adopt the Esscher transform and reflection principle techniques, which are beneficial to the calculation of the conditional expectation of fuzzy random variables and the payoff function with extremum, respectively. Some numerical examples are performed to analyze the sensitivity of the fuzzy hedging portfolio concerning model parameters and give the permissible range of the expected hedging portfolio of lookback options with uncertainty by a financial investor's subjective judgment.
In the financial market, volatility varies from time to time, so uncertain information is more common than certain information. The uncertainty of this information may come from the natural variability of the market or the lack of information such that people are unable to fully comprehend the trend of the market. The traditional option pricing model is derived by stochastic differential equations, but it is not suitable for conveying nonrandom uncertainties. For instance, statements such as "the stock price went up approximately 6%" and "the interest rate of the bond is almost 10%-20%" are hardly accounted for by randomness alone. The concept of the fuzzy set was first introduced by Zadeh[1] such that the value in the interval [0, 1] can be used to represent the degree of agreement between the discussed object and the fuzzy concept, which greatly improves the accuracy of the description of the problem. Fuzzy theory is applicable in the investigation of numerous engineering and economic problems. There are two main methods to study option pricing in a fuzzy environment. One is to regard the parameters of the financial market as fuzzy numbers and fuzzify the parameters in the stochastic model (see, e.g. [2,3]), and the results of this method do not involve any theory of fuzzy stochastic processes. The other method regards the pricing model as fuzzy stochastic differential equations and derives the α-cut of the fuzzy option price by the definition of fuzzy random variable expectations (see, e.g. [4,5,6]). These two approaches lead to two different results. We will use the latter method to study option hedging in a fuzzy environment because it avoids fuzzing every parameter in the model, especially if there are too many parameters.
The theories of fuzzy stochastic differential equations can be found in [7,8,9]. As parts of fuzzy stochastic differential equations, fuzzy random variables and fuzzy stochastic processes play fundamental roles. The notion of a fuzzy random variable that combines randomness and fuzziness has been introduced by Kwakernaak [10] and Puri [11]. There are many different perspectives to consider fuzzy stochastic differential equations. The main problem leading to this phenomenon is the various concepts of the fuzzy stochastic Itô integral, whose crisp counterpart is a notion of the classical stochastic Itô integral. In [12] the fuzzy stochastic Itô integral is regarded as a fuzzy set of the space of square integrable random vectors, and in [13], we can find a proof of the existence of solutions with respect to fuzzy stochastic differential equations. On the other hand, in [14,15,16,17,18,19], the diffusion term of the fuzzy stochastic differential equation is a fuzzy random variable, and the solutions are the fuzzy non anticipating stochastic processes driven by the Wiener process. However, given the fuzzy stochastic Itô integral driven by 1-dimension Brownian motion only with the single-valued in these works, there are not many crucial properties that are needed in our applications, so we give an explanation of the necessity that the integrand is not single-valued in Section 4.2. In order to construct the fuzzy stochastic differential equation in the Skorohod sense, the fuzzy stochastic integral with anticipating integrands is studied in this study. The Skorohod (anticipating) integral, which turns out to be an extension of the traditional Ito integral, allows integrands to not always be modified. In this paper, we treat the pricing model as a Skorohod fuzzy stochastic differential equation. We limit ourselves to adjusted linear coefficients for the sake of simplicity.
The financial market usually adopts the methods of Δ-hedging to hedge a contingent claim. The method of Δ-hedging is to apply the Itô formula to the payoff function and uses the definition of a self-financing portfolio and conclusions about partial differential equations to obtain the hedging portfolio. However, satisfying the differentiability conditions is difficult if these contingent claims have a complex payoff function. The other approach of Malliavin calculus requires that the payoff function F is square integrable, and the Malliavin derivative of F satisfies some specific conditions to remedy the deficiency of Δ-hedging. Research on Malliavin calculus in fuzzy spaces is still in its infancy. In [20] shows the definitions of first order fuzzy Malliavin calculus and obtains the sensitive analysis of European options Greeks. To date, there are few studies on options hedging in the fuzzy environment. In [21] a methodology is proposed for hedging European options with fuzzy parameters. In [22] presents the optimal fuzzy hedging portfolio strategy with options, maximizing the expected value of the portfolio and minimizing its value-at-risk. From the perspective of complementing the field, our research is meaningful and contributory. In this paper, we will give the definition of the nth fuzzy Malliavin derivative ˜Dn and its adjoint operator ˜δn (also called divergence operator). In terms of Wiener chaos expansion, the fuzzy Clark-Ocone formula is proposed, which makes options hedging accessible in fuzzy space.
To obtain an expression for the hedging portfolio for fixed strike lookback options, we develop a probabilistic method. The expectations of fuzzy random variables mentioned in the current manuscript are proposed by Yoshida et al. [23]. The primary reason lookback options are more complicated to price and hedge than barrier options is that lookback options have extremes (maximum or minimum) in both the payout amount and the payoff condition, which leads to more complicated expectations calculations. For this problem, we adopt the Esscher transform and the reflection principle of Brownian motion in fuzzy space. The Esscher transform introduced by [24] is applied to determine an equivalent martingale measure in the financial market. The contributions in [25] make the Esscher transform an efficient technique for option pricing. The reflection principle is usually used in pricing barrier options, which plays a crucial role in eliminating extremes. We also practice this principle to lookback options pricing and hedging since the extreme exists in the payoff. By the properties of the reflection principle, the cumulative distribution function of the Brownian motion and its maximum (or minimum) can be explicitly computed.
This paper is organized as follows. Section 2 introduces some notions about fuzzy theories, including fuzzy numbers, fuzzy random variables, and fuzzy stochastic processes. We also study the fuzzy stochastic integral with anticipating integrands. In Section 3, we define the fuzzy Malliavin derivative and its adjoint operator, and the fuzzy Clark-Ocone formula is presented successively. In Section 4, we develop a pricing model based on the fuzzy stochastic differential equation in the sense of Skorohod. By the fuzzy Clark-Ocone formula, we hedge fixed strike lookback options in fuzzy space. The analytical solutions of the hedging portfolio are obtained by the Esscher transform and reflection principle. Section 5 performs some numerical simulation. We analyze the sensitivity of hedging portfolios to different parameters. We also consider the rational expected hedging portfolio of the lookback options and financial investors' permissible range of expected prices.
This section mainly introduces some theories of fuzzy sets and fuzzy stochastic processes. We also describe the construction of a fuzzy Itô integral. The reader can refer to [26,27,28] for more details.
Definition 2.1. (Metric of Hausdorff) Denote K(R) the family of all nonempty, bounded, compact and convex subsets of R. The Hausdorff metric dH is defined as follows
dH(A,B)=max{supa∈Ainfb∈B|a−b|,supb∈Binfa∈A|a−b|}. | (2.1) |
Let L2(R) denote the set of R-valued stochastic processes. If f∈L2(R), then the norm of f as follows
‖f‖L2(R)=[E(∫T0‖f(t,ω)‖pds)]1p<∞. |
Definition 2.2. (Fuzzy number) Let F(R) denote the family of all the fuzzy sets
u:R⟼[0,1], |
such that [u]α∈K(R) for every α∈[0,1], in which [u]α is a α-level set can be expressed as [u]α={x∈R:u(x)≥α}=[uLα,uUα] for α∈(0,1] and [u]0:supu=cl{x∈R:˜u(x)>0}. u(x) is interpreted as the degree of membership of x in the fuzzy set u. If u is normal, convex and upper semicontinuous, we call u a fuzzy number.
Definition 2.3. (Metric on F(R)) Assume C,D∈F(R) are two fuzzy numbers, α∈[0,1], then a metric between them is defined as follows
d∞(C,D)=supα∈[0,1]dH([C]α,[D]α). |
Then d∞:F(R)×F(R)→[0,∞), and (F(R),d∞) is a complete metric space.
Definition 2.4. (Fuzzy arithmetic operations) Let a and b be two fuzzy numbers. Then a⊕b, a∘b and ab are also fuzzy numbers, for all α∈[0,1], their α-level sets are:
(a⊕b)α=[aLα+bLα,aUα+bUα],
(a∘b)α=[min{aLαbLα,aLαbUα,aUαbLα,aUαbUα},max{aLαbLα,aLαbUα,aUαbLα,aUαbUα}].
If α-level set bα does not contain 0, then we have(ab)α=[min{aLαbLα,aLαbUα,aUαbLα,aUαbUα}],max{aLαbLα,aLαbUα,aUαbLα,aUαbUα}].
Due to the particularity of the difference between two fuzzy numbers, which is closely related to the differentiability, we will discuss it in the Section 3.
Definition 2.5. (Fuzzy random variable) Let (Ω,A,P) be a probability space. For all α∈[0,1], if [X]α:Ω→K(R) is an A-measurable function, then a map X:Ω→F(R) is called a fuzzy random variable.
For any metric ds in F(R), let Bds be a σ-algebra generated by the topology induced by the metric ds, then we can consider a fuzzy random variable if and only if the mapping u:(Ω,A)→(F(R),Bds) is A|Bds measurable, with the Skorohod metric ds (definition in [29]).
Let X is an integrally bounded fuzzy random variable, the expectation of fuzzy random variable is proposed by Yoshida et al. [23]. In what follows we recall this definition. For every α∈[0,1] and x∈R, the expectation E(X) of the fuzzy random variable X is defined by a fuzzy number
E(X)(x)=supα∈[0,1]min{α,1E(X)α(x)}, |
where
E(X)α=[∫ΩXLαdP(ω),∫ΩXUαdP(ω)]. |
Definition 2.6. (Fuzzy stochastic process) Let us consider a fuzzy family {X(t),t∈[0,T]} on probability space (Ω,A,{At}t∈[0,T],P), {At}t∈[0,T] is an increasing and right continuous family of sub-σ-algebras of A. For every t∈[0,T], a fuzzy random variable X(t,⋅) can expressed as X:Ω→F(R). Then we call X={X(t)}t∈[0,T]:[0,T]×Ω→F(R) a fuzzy stochastic process.
If almost all trajectories X(⋅,ω):[0,T]→F(R) are d∞-continuous functions, then we call that the fuzzy stochastic process X is d∞-continuous. A fuzzy stochastic process f is adapted if and only if for every α∈[0,1], [f]α is measurable with respect to the σ-algebra N, which can be defined as follows
N:={M∈B([0,T])⊗At:Mt∈At for each t∈[0,T]}, |
where Mt={ω:(t,ω)∈M}, B([0,T]) denotes the Borel σ-algebra of subsets of [0,T]. If there exists a real-valued stochastic process h∈Lp([0,T]×Ω,N;R) such that ‖|[X(t,ω)]0|‖≤h(t,ω), a fuzzy stochastic process X={X(t,ω):t∈[0,T]} is called Lp-integrably bounded.
Definition 2.7. ([30] Fuzzy Aumann Type Lebesgue Integral) Denote the set of all adapted and Lp-integrably bounded fuzzy stochastic processes by Lp([0,T]×Ω,N;F(R)). Assume that X∈Lp([0,T]×Ω,N;F(R)), and for α∈[0,1], there exists ΩXα∈A and P(ΩXα)=1 such that for each ω∈ΩXα
∫T0‖Xα(t,ω)‖pdt<∞. |
Considering a fuzzy stochastic process Xα∈Lp([0,T]×Ω,N;F(R)), for any α∈[0,1], we define the Aumann type Lebesgue integral Lα,t(Xα)(ω) by
Lα,t(Xα)(ω)={{∫t0Xα(s)ds},forω∈ΩXα,{0},forω∉ΩXα. |
To correlatethe the Skorohod integral with the fuzzy stochastic integral concerning the wiener process W, let us briefly review some definition of Malliavin calculus in crisp case. Let S denote the set of all smooth random variables of the form
F=f(X(h1),⋯,X(hn)). |
Where n≥1, X(hi),i=1,⋯,n are Gaussian random variables, hi belongs to a real separable Hilbert space H, then f:R→F(R) is an infinitely differentiable function and all the partial derivatives of f have at most polynomial growth. For simplicity, we consider the 1th Malliavin derivative
DF=m∑i=1∂nf∂xii⋯∂xin(X(h1),⋯X(hm))hi. |
Let D1,2 denote the closure of S with respect to the norm
‖F‖D1,2=(E[|F|2]+E[‖DF‖2H])12. |
We denote the adjoint of operator D by δ. The Dom δ is the subset of L2[[0,T]×Ω] composed of those square integrable random variables h such that
|E[⟨DnF,h⟩H]|≤c√E[F2]. |
For h∈Domδ, δ(h) is also called Skorohod integral with the form
δ(h)=∫T0h(t)dWt. |
Skorohod integral is the extension of the Itô integral for anticipating processes. Particularly, the operator δ restricted to L2([0,T]×Ω) coincides with the Itô stochastic integral.
Let U be a real separable Hilbert space, then the space SU is the collection of all smooth U-valued random elements of the type h=∑ni=1Fivi, where Fi∈S, vi∈U. One can extend the domain of D to the space D1,2(U), which is defined as the closure of SU with respect to the norm
‖h‖2D1,2(U)=(E[‖h‖2U]+E[‖Dh‖2H⊗U])12. |
If U=L2([0,T]), then D1,2(L2([0,T])) coincides with the class of processes h∈L2([0,T]×Ω) such that h(t)∈D1,2 for almost all t.
Next, let us recall some notations of fuzzy stochastic integral. Denote L2([0,T]×Ω,N,λ×P;F(R)) by L2N(λ×P), λ is the Lebesgue measure on ([0,T],B([0,T])). Let {F(t)}t∈[0,T] be a set-valued stochastic process with values in K(R). It means that there exists a mapping F:[0,T]×Ω→K(R), we call F is adapted if it is N-measurable. Considering a set
S2N(F)={f∈L2N(λ×P):f∈F}. |
Let F:[0,T]×Ω→K(R) is L2N(λ×P)-integrably bound, then by Kuratowski and Ryll-Nardzewski Selection Theorem (see e.g.[32]) it follows that S2N(F,λ×P)≠∅.
Considering a set-valued functional stochastic integral of F with respect to the standard Brownian motion Wt, we mean the set
∫t0F(s)dWs={∫t0f(s)dWs:f∈S2N(F,λ× P),t∈[0,T]} |
is called the set-valued stochastic integral of F with respect to the wiener process W. Let a process h in class D1,2(L2[0,T]), then we give an assumption:
(C1) For f∈S2N(F), E[∫T0(∫T0(Dsft)2ds)p2dt]<∞, p>2.
Proposition 2.8. (Proposition 3.15 of [31]) Let F:[0,T]×Ω→K(R) be L2-integrally set-valued mapping, and S2N(F)⊂D1,2. We assume the (C1) is hold, then we have the following conclusions
(a) S2N(F) is a closed, convex, bounded, weakly compact and decomposable subset of L2([0,T]×Ω),
(b) ∫t0F(s)dWs is a bounded closed, weakly compact and convex subset of L2(Ω,At,P,R+).
Theorem 2.9. (Theorem 3.1 of [33]) Let C⊂R be a nonempty set and let {Cα:α∈[0,1]} be a family of subsets of C such that
(1) C0=C.
(2) C0⊃Cα⊃Cβ for 0≤α≤β.
(3) If αn↗α then Cα=∩n=NCαn.
Then there exists u∈F(R) such that [u]α=Cα for any α∈(0,1], and [u]0⊂C0. Moreover
u(x)={supx∈Cα,ifx∈C,0,ifx∉C. |
On the other hand, for u∈F(R) the family of sets C0=[u]0, Cα=[u]α, α∈(0,1] satisfies conditions (1) and (2).
Theorem 2.10. Let Fn:[0,T]×Ω→K(R) is a sequence of decreasing and adapted set-valued stochastic processes such that F1 is L2N(λ×P)- integrably bounded and F1⊃F2⊃⋯⊃F. Where ⋂n≥1Fn=F, then for every t∈[0,T], it holds
⋂n≥1∫t0Fn(s)dWs=∫t0F(s)dWs. |
As mentioned above, let us start discussing the fuzzy-valued stochastic integrals driven by 1-dimension Brownian motion.
Definition 2.11. (Fuzzy-valued stochastic integrals) According to Proposition 2.8, Theorems 2.9 and 2.10, we can conclude that for every t∈[0,T] and α∈[0,1], there exists a fuzzy set (X(t,F)) such that (X(t,F))α=∫t0(F(s))αdWs. Then for an anticipating and LP-integrably bounded fuzzy stochastic process F, a family of fuzzy sets {X(t,F)}∈F(L2(Ω,At,P;R)), which can be denoted by
X(t,F)=∫t0F(s)∘dWs, | (2.2) |
In this way, the fuzzy Itô integral obtained under the assumption is bounded, which is the crucial property needed in applications.
The fuzzy Malliavin derivative and divergence operator in the sense of the stochastic gradient are defined in this section. In the following part, we put forth a crucial theory known as the fuzzy Clark-Ocone formula that offers theoretical backing for lookback options hedging.
Definition 3.1. (Generalized Hukuhara difference) Let x,y∈F(R), we define the generalized Hukuhara difference of x and y if there exist a fuzzy number z such that the following conditions are satisfied
(i)x=y+z,(ii)y=x+(−z). |
Condition (i) corresponds to the conception of Hukuhara difference. The generalized Hukuhara difference of x and y is denoted by z=x⊖ghy. For any α∈[0,T], if [x]α and [y]α are two fuzzy closed intervals, the gH-difference is given by
[x]α⊖gh[y]α=[min{xLα−yLα,xUα−yUα},max{xLα−yLα,xUα−yUα}]. |
However, the gH-difference for some pairs of fuzzy number are undefined. For instance, if A=(1,2,3), B=(4,5), A⊖gHB is undefined. Fortunately, the generalized difference proposed in [34] solves this problem. The generalized difference x⊖gy is defined levelwise as
[x⊖gy]α=conv⋃β≥α([x]β⊖gH[y]β), |
where conv denotes the convex hull.
Definition 3.2. ([35] Generalized differentiable) Assume a fuzzy-number-valued function F:(a,b)→F(R) is generalized differentiable, then for x0∈(a,b), the following limit
limh→0F(x0+h)⊖gF(x0)h |
exists and equals to a fuzzy number. We denote this fuzzy number by F′g(x) and call F generalized differentiable at x0.
Next, let us start with the definition of the fuzzy Malliavin derivative. Denote by ˜S the set of all fuzzy smooth random variables of the form
F=f(X(h1),⋯,X(hn)). | (3.1) |
Where X(hi),i=1,⋯,n, are fuzzy Gaussian random variables with the form of X(hi)=∫T0hi(t)∘dW(t), hi∈H, H is a real separable Hilbert space. Assume that f:R→F(R) is an infinitely differentiable function and all the partial derivatives of f have the most polynomial growth.
Let H⊙n is a separable Hilbert space generated by the H⊙n-valued fuzzy random variables f that are square integrable and symmetric. Denote the symmetric tensor product by ⊙, fs is called the symmetrization of f. In order to express the meaning of "symmetric", we introduce the following equation
fs(t1,⋯,tn)=1n!∑σf(tσ1,⋯,tσn), |
where σ=(σ1,⋯σn) is permutations of (1,⋯,n).
Definition 3.3. (Fuzzy Malliavin derivative) Let F:Ω→F(R) is given by (3.1), n≥1 is an integer. Assume f is generalized differentiable, {X(hi)} is a centered Gaussian family, then the Malliavin derivative of F in L2[Ω×H⊙n;F(R)] is defined by
˜DnF=m∑i1,⋯,in=1∂nf∂xii⋯∂xin(X(h1),⋯X(hm))hi1⊗⋯⊗hin, | (3.2) |
where "⊗" denotes the tensor product. In particular, ˜D1F can be simply denoted as ˜DF. For h∈H, it holds
⟨DF,f⟩H=limε→01ε[f(X(h1)+ε⟨h1,f⟩H,…,X(hm)+ε⟨hm,f⟩H)−F], |
it means that ˜DF can be regarded as a direction derivative.
Assume that the fuzzy random variable FG∈˜S can be represented in the form of (3.1), denote by N(x) the density of the standard normal distribution on R, that is
N(x)=(2π)−m2exp(−12m∑i=1xi). |
Hence
E[⟨˜D(FG),h⟩]H=∫Rm∂f(x)∂x1N(x)dxi⋯dxm=∫Rmx1f(x)N(x)dxi⋯dxm=E[X(h)FG]. | (3.3) |
It means that operator ˜Dn is closable. Let ˜Dn,2 denote the closure of ˜S with respect to the norm
‖F‖˜Dn,2=(E[|F|2]+E[‖DF‖2H]+⋯+E[‖DpF‖2H⊗n])12. |
˜Dn,2 is also called the domain of the operator ˜Dn in L2(Ω;F(R)).
We suppose that the separable Hilbert space H is an L2 space of the form H=L2([0,T],B,μ), where μ is a σ-finite atomless measure on a measurable space ([0,T],B). The derivative of a fuzzy random variable F∈˜Dn,2 will be a fuzzy stochastic process due to the identification between L2[Ω×H⊙n;F(R)] and L2[[0,T]⊗n×Ω,N;F(R)]. For n≥1, the derivative ˜DnF={˜Dnt1,⋯,tnF,ti∈T} is a μn×P measurable function. Specially, DtF is defined almost everywhere with respect to the measure μ×P.
Lemma 3.4. (Chain rule) Let F and Ψ∈C1(F(R)) be a generalized differentiable fuzzy function. Assume that F is a fuzzy random variable whose components are elements of ˜D1,2, and g′(F)˜DtF∈L2(P×λ), then we have the chain rule
˜Dt(Ψ(F))=Ψ′(F)˜DtF. | (3.4) |
Proof. For any direction γ∈Ω we have
˜Dγ(Ψ(F))=liml→01l[g(F(ω+lγ))⊖gΨ(F(ω))]=liml→01l[Ψ(F(ω)+l˜DγF)⊖gΨ(F(ω))]=1lΨ′(F(ω))l˜DγF=Ψ′(F)˜DγF=∫T0Ψ′(F)˜DtFγ(t)dt. |
So the proof is completed.
Definition 3.5. (Duality formula) We denote the adjoint of operator ˜Dn by ˜δn, and Dom ˜δn is represented as the subset of L2[Ω×H⊙n;F(R)] composed of those random variables h such that
|E[⟨˜DnF,h⟩H]|≤c√E[F2], |
where F∈˜S, c>0 is a constant. For F∈˜Dn,2, if h∈Dom˜δn, then ˜δn(h) is the unique element of L2[Ω;F(R)] characterized by the following duality formula
E[F˜δn(h)]=E[⟨˜DnF,h⟩H]. | (3.5) |
The operator ˜δn is called the divergence operator of order n.
Combining computations (3.3) and (3.5), {it is easy to} deduce that ˜δ(h)=X(h) when G=1, we call ˜δ(h) is the fuzzy Skorohod stochastic integral of h.
Let G be another real separable Hilbert space, then the space ˜SG is the collection of all smooth G-valued fuzzy random elements of the type F=∑ni=1Fivi, where Fi∈˜S, vi∈G. For k≥1, the kth Malliavin derivative of any F∈˜SG can be expressed as ˜DkF=∑ni=1˜DkFi⊗vi.
Theorem 3.6. Let G=H, and denote the class of H-valued smooth fuzzy random variables by ˜SH, for all h∈˜SH such that h can be written as ∑ni=1Fivi, where Fi∈˜S, vi∈H. Let ˜δ(h)∈˜D1,2, h∈SH⊂Dom˜δn, then we have
˜D˜δ(h)⊖g˜δ(˜Dh)=h. | (3.6) |
Proof. For all g,f∈˜D1,2, u∈H, according to (3.5), we get
E[g˜δ(f)]=E[⟨˜Dg,fu⟩H]=E[g(˜δ(u)f⊖g⟨˜Df,u⟩H)]. |
Let g=1, it has ˜δ(fu)=˜δ(u)f−⟨˜Df,u⟩H. Then we can deduce that
˜δ(h)=˜δ(n∑i=1fiui)=n∑i=1(fiX(ui)⊖g⟨˜Dfi,ui⟩H), |
such that
˜D˜δ(h)=⨁n=1(X(ui)˜Dfi⊕fiui⊖g⟨˜D2fi,ui⟩H), |
and
˜δ(˜Dh)=n∑i=1(X(ui)˜Dfi⊖g⟨˜D2fi,ui⟩H). |
Subtract ˜D˜δ(h) and ˜δ(˜Dh), then we complete this proof.
Fix k≥1 is an integer, we can know H⊗n=H⊗n−k⊗H⊗k. For h∈H⊗n which can be written as h=∑ni=1fiui, where fi∈H⊗n−k and ui∈H⊗k, such that ˜δn(f) be a H⊗n−k-valued fuzzy random variable
˜δn(f)=˜δn−k(˜δk(f)). |
It means that ˜δk(h)∈L2(Ω,H⊗n−k;F(R)) and for ˜δn(f)∈˜D1,2, we can easily deduce the following equation by Theorem 3.6 and induction
˜D˜δn(f)=n˜δn−1(f). | (3.7) |
In order to prove the Clark-Ocone formula in fuzzy space, we first recall some definitions and lemmas.
Definition 3.7. (Fuzzy iterated Itô integral) Let f∈L2([0,T]⊙n;F(R)) be symmetric, then the fuzzy iterated Itô integral is defined as follows
In(f)=∫[0,T]nf(t1,⋯,tn)∘dWt1⋯dWtn=n!∫T0∫tn0⋯∫t20f(t1,⋯,tn)∘dWt1⋯dWtn=n!Jn(f). |
It can be seen that the n-fold iterated integral In(f)=˜δn(f).
Theorem 3.8. (Wiener chaos expansion) Suppose that F∈L2(Ω;F(R)) is a fuzzy random variable defined as (3.1), for some unique collection of kernels fn∈H⊙n, F can be expanded as
F=⨁n=0In(fn), |
where f0=E[F], and I0 is an identity mapping such that I0(f0)=E[F].
Proof. Assume that Mn is the closed subset of L2(Ω;F(R)) composed by the fuzzy random variables of the type Hn(X(f)), {Hn}n≥0 denotes the Hermite orthogonal polynomials. Thence by property of Hermite polynomials, Mn is orthogonal to Mm when n≠m, M0=F(R). With the help of Theorem 2.2.4 in [36], we know that the linear space Mn is dense in L2(Ω;F(R)). It means that
L2(Ω;F(R))=M0⊕M1⊕⋯⊕Mn. |
We can know that Hn(X(f))=In(fn) by means of In(f)=˜δn(f), so we can regard Mn as a dense subspace of L2(Ω;F(R)) generated by class In(fn), then the proof is completed.
By (3.7), we can deduce ˜DIn(fn)=nIn−1(fn), so that for F∈˜D1,2, it holds
˜DF=⨁n≥1nIn−1(fn), | (3.8) |
where fn∈H⊙n. From the perspective of Wiener chaos expansion in term of fuzzy iterated integrals, we also defined the fuzzy Malliavin derivative.
Theorem 3.9. (Fuzzy Clark-Ocone formula) Let F∈˜D1,2 be AT measurable and Wt is a 1-dimension Brownian motion. Then we have
F=E[F]⊕∫T0E[˜DtF|At]∘dWt. |
Proof. Following the Proposition 3.11 in [37], for fn∈H⊙n, we can know that E[In(fn)|AG]=In(fn1G), where G is a Borel set in [0,T]. AG is a completed σ-algebra generated by fuzzy random variables of the form (3.1). Then we can deduce that
∫T0E[˜DtF|At]∘dWt=∫T0E[⨁n≥1nIn−1(fn)|At]∘dWt=∫T0⨁n≥1nIn−1(fn⋅1⊗(n−1)[0,T](t,⋅))∘dWt=∫T0⨁n≥1n(n−1)!Jn−1(fn⋅1⊗(n−1)[0,T](t,⋅))∘dWt=⨁n≥1In(fn)=F⊖gE[F], |
where In−1(fn(t,⋅)) is means that only n−1 fuzzy variables in the kernel fn are integrated out, while the others act as free parameters.
In this section, we will construct a portfolio to replicate a fixed strike lookback option in fuzzy space. Let us denote the fuzzy notation by "∼", it means {for} a fuzzy random variable ˜X, its crisp counterpart is a random variable X. Then we will use the theories of fuzzy Malliavin calculus proved above, and explicitly calculated hedging portfolio ˜β in a probabilistic approach.
To build our pricing model, we need to introduce some theories of fuzzy stochastic differential equations in the Skorohod sense firstly. Considering a fuzzy stochastic differential equation, we restrict ourselves to adapted linear coefficients for simplicity of the exposition
˜Xt=X0⊕∫t0σs˜Xs∘dWs⊕∫t0b(s,˜Xs)∘ds, | (4.1) |
where X0 is a fuzzy random variable, σ∈L2[0,T]. Let S2N(F)⊂˜Dn,2(L2[0,T])⊂Dom˜δ, and assume that b is a fuzzy random function satisfies the following assumption:
(C2) Let ˜b:[0,T]×R×Ω→F(R) is a measurable function such that there exists an integrable function γt on [0,T], γt≥0, a constant L>0, and a set N1∈A of probability one, for every x,y∈F(R), t∈[0,T], it holds
d∞(˜b(t,x,ω),˜b(t,y,ω))≤γ(t)d2∞(x,y); |
∫T0γ(t)dt≤L; |
d∞(˜b(t,x,ω),{0})≤L. |
Then consider two transformations
˜At(ω)s=ωs+∫t∧s0σudu,˜Bt(ω)s=ωs−∫t∧s0σudu, |
such that ˜At(ω)s˜Bt(ω)s=˜Bt(ω)s˜At(ω)s=Identity. ˜λt=exp(∫t0σdWs−12∫t0σ2ds) can be defined as a Radon-Nikodym derivative, then E[Y(Bt)˜λt]=E[Y] holds for any fuzzy random variable Y∈L1(Ω;F(R)) by Girsanov's theorem. Then we can call (4.1) a fuzzy stochastic differential equation in the Skorohod sense, For x∈F(R) and ω∈Ω, we have the following equation
˜Zt(ω,x)=x∘∫t0˜λ−1s(At(ω))b(s,˜λs(As(ω))Zs(ω,x),As(ω))∘ds. |
Theorem 4.1. For a fixed initial condition ˜X0∈LP(Ω;F(R)), p>2, and assumptions (C1),(C2) are held, we define
˜Xt=˜λt∘Zt(A(t),˜X(0,A(t))). | (4.2) |
Then the process ˜X={˜Xt,t∈[0,T]} satisfies 1[0,t]σ˜Xt∈Dom ˜δ. X is the unique solution of (4.1), ˜X∈L2([0,T]×Ω;F(R)). The proof of the solution's existence and uniqueness can be found in [31,38].
Considering a market consisting of two assets: a risk-less asset of price Bt and a stock of price ˜St, and replicate a fixed strike lookback option ˜F with the self-financing strategy in fuzzy space. The dynamic of the model takes the following form
˜St=˜S0⊕∫t0r˜St∘dt⊕∫t0σ˜St∘dWt, | (4.3) |
and the dynamic of Bt is
dBt=rBtdt,B0=1, |
where risk-free rate r and volatility σ are regarded as positive constants, Wt is a standard Brownian motion. Let pricing model (4.3) be consistent with the principle of fuzzy stochastic differential equation in the Skorohod sense, then the fuzzy Itô integral ∫t0σ˜St∘dWtis bounded. According to Theorem 4.1 we have
Zt(ω,x)=˜S0⊕r∫t0Zs(ω,˜S0)∘ds. | (4.4) |
We denote Zt(ω,˜S0) by Zt in order to simplify the notation. Then we can solve (4.3) as follows
{(ZLt)α=ert(SL0)α,(ZUt)α=ert(SU0)α. |
By (4.2) we know that the fuzzy model has the unique solution
˜St=˜S0∘ertexp(∫t0σdWs−12∫t0σ2ds), | (4.5) |
it can be solved as
{(SLt)α=˜λtert(˜SL0)α,(SUt)α=˜λtert(˜SU0)α. |
Let ˜ηt be the number of non-risky assets and ˜βt be the number of stocks owned by the investor at time t, we call the couple ˜ϕt=(˜ηt,˜βt) is a fuzzy portfolio with the value process
dV(˜ϕt)=˜ηtdBt⊕˜βt∘ d˜St. | (4.6) |
We can express ˜ηt as ˜ηt=V(˜ϕt)⊖g˜βt∘˜StBt, then (4.6) can be written as
dV(˜ϕt)=rV(˜ϕt)∘dt⊕σ˜βt∘˜St∘dWt. |
By observing the above formula, the issue of hedging is equivalent to the question about finding a suitable ˜βt such that these two opposite positions offset. Plainly, for a fixed strike lookback option ˜F at maturity time T, exist the following equation
V(˜ϕT)=˜F. | (4.7) |
Considering the discounted prices of this portfolio
e−rTV(˜ϕT)=V(˜ϕ0)⊕∫T0e−rtσβt∘˜StdWt, | (4.8) |
assume that ˜F∈˜D1,2, then apply the fuzzy Clark-Ocone formula of Theorem 3.9 to ˜H=e−rT˜F with the following form
˜H=E[˜H]⊕∫TtE[˜Dt˜H|At]dWt . | (4.9) |
By (4.7) and the correspondence of (4.8) and (4.9), it holds E[˜H]=V(˜ϕ0), and
e−r(t−T)σ⊕˜βt⊕˜St=E[˜Dt˜F|At]. | (4.10) |
Observing the above formulas, if the fuzzy stochastic Itô integrals involved in (2.2) having only single-valued integrands will lead to the result in (4.10) is identical to crisp case, so this embody the importance of making the integrand a fuzzy number.
Considering a call lookback option with fixed strike price K, the payoff at maturity time T is ˜F=(˜MTS−K)+, where ˜MTS=maxt∈[0,T]˜St. Then the Eq (4.10) with the following form
e−r(t−T)σ⊕˜βt⊕˜St=E[˜Dt(˜MTS−K)+|At]. | (4.11) |
Theorem 4.2. Assume that ˜St∈˜D1,2, for 0≤t≤T, then the fuzzy Malliavin derivative of ˜MTS can be calculated as
˜Dt˜MTS=σ˜MTS. |
Proof. In order to obtain ˜Dt˜MTS, we need to compute ˜Dt˜Ss firstly, 0≤t≤s, By Taylor expansion, we can know eσWs→∑∞k=0(σWs)kk! in L2(Ω;F(R)). Since ∑∞k=0(σWs)kk!∈˜S, we have
˜Dt(n∑k=0(σWs)kk!)=n∑k=0˜Dt((σWs)kk!)=n∑k=0σkk!˜Dt((Ws)k)=n∑k=0σkk!k(Ws)k−11{0≤t≤s}=σn−1∑k=0(σWs)kk!1{0≤t≤s}→σeσWs1{0≤t≤s}whenn→∞. |
So we can solve ˜Dt˜Ss as follows
˜Dt˜Ss=exp(r−σ22)˜S0∘n−1∑k=0(σWs)kk!σ1{0≤t≤s}→e(r−σ22)˜S0∘σeσWs1{0≤t≤s}whenn→∞=σ˜Ss1{0≤t≤s}. |
Then we can obtain ˜Dt˜MTS=σ˜MTS from the crisp case in Corollary 1 of [39].
It is easy to see (˜MTS−K)+ can be expressed equivalently as φ(˜MTS)=max(˜MTS,K)−K. When φ(˜MTS) is a Lipschitz function and φ(˜MTS)∈˜D1,2, according to the chain rule in Lemma 3.4 we can deduce that
˜Dt(˜MTS−K)+=˜Dt˜MTS1{˜MTS≥K}. |
So (4.11) can be written as
e−r(t−T)σ˜βt∘˜St=E[σ˜MTS1{˜MTS>K}|At]. | (4.12) |
We will adopt a completely probabilistic approach to calculate hedging portfolio ˜βt. To begin with, we need to recall some useful lemmas.
Considering the crisp case of Eq (4.3) that can also be called the differential form of B-S model
St=S0+∫t0rStdt+∫t0σStdWt. |
Let Xt=lnStS0, then we have Xt=(r−12σ2)dt+σWt. Denote supt∈[0,T]Xt=MTX and k=lnKS0.
Lemma 4.3. (Reflection principle) We assume that Wt is a standard Brownian motion, P is the cumulative distribution function, and N is the standard normal distribution. We denote MTW=supt∈[0,T]Wt, then we have the following reflection principle
P(Wt≤x,MTW≥y)=P(Wt≤2y−x), |
where x≤y, y>0. It implies that
P(XT≤x,MTX≥y)=eξyP(XT≤x−2y)=eξyN(x−2y−uTσ√T). |
By Lemma 4.3, the joint probability density function XT and MTX can be written as follows
∂P(MTX≤k,XT≤k)∂y=∂(P(XT≤y)−P(MTX>y,XT≤y))∂y=∂P(XT≤y)∂y−∂eξyP(XT≤−y)∂y=∂P(XT≤y)∂y−ξeξyF(XT≤−y)−eξy∂P(XT≤−y)∂y. | (4.13) |
Lemma 4.4. ([40] Esscher transform) For a risk-neutral measure Q, the process ecXTEQ[ecXT] can be regarded a Radon-Nikodym derivative such that
EQ1[Y]=EQ[YecXTEQ[ecXT]], |
where Y is a random variable which is a real-valued function of {Xt,0≤t≤T}, then a new probability measure Q1 is called the Esscher measure concerning parameter c. In order to express conveniently, we denote EQ1[Y]=E[Y;c]. Under Esscher measure of parameter c, the diffusion coefficient is still σ, but the drift coefficient is changed from u to u+cσ2. For ξ=2uσ2, we have
E[e−ξXT]=e(−ξ)uT+12(−ξ)2σ2T=1, |
the cumulative distribution function of XT is
E[I{XT<k}]=N(lnKS0−uTσ√t), |
thence for a constant c, it holds
E[ecX(T)I{X(T)>k}]=E[ecX(T)]E[I{X(T)>k]ecX(T)E[ecX(T)]]=exp(cuT+c2σ2T2)P(XT>k;c)=exp(cuT+c2σ2T2)N(−lnKS0+(u+cσ2)Tσ√T). | (4.14) |
Theorem 4.5. Assume the underlying asset price follows the fuzzy stochastic differential equation as shown in (4.3), for every α∈[0,1], we obtain the analytical expression for hedging portfolio of lookback options as follows
(˜βL0)α=(2−ξ1+ξ)N(ln(˜SL0)αK+(r+σ22)Tσ√T)+e−rTξ(K(˜SU0)α)1+ξ1+ξN(ln(˜SL0)αK−(r−σ22)Tσ√T), |
and
(˜βU0)α=(2−ξ1+ξ)N(ln(˜SU0)αK+(r+σ22)Tσ√T)+e−rTξ(K(˜SL0)α)1+ξ1+ξN(ln(˜SU0)αK−(r−σ22)Tσ√T), |
where ξ=2uσ2,u=r−σ22. The other notations in the above equation are the same as previously mentioned.
Proof. We consider a crisp expectation E[MTX1{MTS>K}|At] which can be solved by (4.14) as follows
E[eMTX1{MTX>K}]=∫y>key∂P(MTX≤y)∂ydy=∫y>key∂P(MTX≤y,XT≤y)∂ydy=∫y>key∂P(XT≤y)∂ydy−ξ∫y>ke(1+ξ)yP(XT≤−y)dy−∫y>ke(1+ξ)y∂P(XT≤−y)∂ydy. | (4.15) |
We will calculate it separately. The first term of (4.15) can be calculated as
∫y>key∂P(XT≤y)∂ydy=E[eXT1{XT>k}]=ertN(−k+(r+σ22)Tσ√T). | (4.16) |
The second term of (4.15) can be solved by integration by parts and Esscher transform as follows
−ξ∫y>ke(1+ξ)yP(XT≤−y)dy=−ξ1+ξe(1+ξ)yP(XT≤−y)|y=∞y=k+ξ1+ξ∫y>ke(1+ξ)y∂P(XT≤−y)∂ydy=ξ1+ξe(1+ξ)kN(−k−uTσ√T)+ξ1+ξ∫y>ke(1+ξ)y∂P(−XT≥y)∂ydy=ξ1+ξe(1+ξ)kN(−k−uTσ√T)−ξ1+ξE[e−(1+ξ)XT]E[1[−XT>k];−(1+ξ)]=ξ1+ξ(e(1+ξ)kN(−k−(r−σ22)Tσ√T)−erTN(−k+(r+σ22)Tσ√T)). | (4.17) |
The last identity of (4.15) can be solved by the same technique with the following form
∫y>key∂P(XT≤y)∂ydy=E[eXT1{XT>k}]=erTN(−k+(r+σ22)Tσ√t). | (4.18) |
Then the hedging portfolio in the crisp case β0=er(t−T)σ−1(St)−1EQ[(σMTS1{MTS>K}] can be solved explicitly as follows
β0=(2−ξ1+ξ)N(lnS0K+(r+σ22)Tσ√T)+e−rTξ(KS0)1+ξ1+ξN(lnS0K−(r−σ22)Tσ√T), | (4.19) |
from the definition of fuzzy expectation of fuzzy random variables, the fuzzy hedging portfolio in (4.12) can be written as
{(˜βL0)α=e−rTσ−1(˜SL0)−1αEQ[σ(˜MTS)Lα1(˜MTS)Lα>K}],(˜βU0)α=e−rTσ−1(˜SU0)−1EQ[σ(˜MTS)Uα1(˜MTS)Uα>K}]. | (4.20) |
Combine Eqs (4.15)–(4.19), the proof of Theorem 4.5 is completed.
In this section, we will perform some numerical simulations to illustrate the theoretical results obtained in the previous sections. First of all, let us introduce the construction of a trapezoidal fuzzy number (see Figure 1).
For a fuzzy stochastic process {˜St}t∈[0,T], then its membership function takes the following form
˜St(x)={x−(S1−b)b,x∈[S1−b,S1),1,x∈[S1,S2),(S2+c)−xc,x∈[S2,S2+c),0, else , |
where [S1,S2] is called tolerance interval, bt>0 is the left-width, ct>0 is the right-width. Assumed that the trapezoidal fuzzy numbers ˜St are symmetrical, it means bt=ct. Following [41], we can get the α-level set of ˜St with the form of (˜St)α=[(˜SLt)α,(˜SUt)α]=[S1−(1−α)bt,S2+(1−α)ct].
Let us consider a fixed strike lookback options with the payoff ˜F=(˜MTS−K)+. We assume S1=32, S2=34, K=28, T=1, σ=0.2, r=0.05, and left and right width b=c=5. To simplify the notation, we will write β0=β, b0=b, c0=c. Table 1 shows the α-level closed intervals of the fuzzy hedging portfolio ˜β. For α=0.95, the hedging portfolio ˜β lies in the closed interval [1.4063, 1.6130], which means that financial investors can choose any value from this interval as their later portfolio to hedge target options if they are comfortable with this belief degree 0.95. From another aspect of view, a risk aversion investor may pick the value of ˜β with a higher belief degree. On the contrary, risk adventurer investors may be acceptable to a relatively low belief degree and select the corresponding interval if they think it is reasonable.
α | [˜βLα,˜βUα] |
0.90 | [1.3833, 1.6318] |
0.91 | [1.3880, 1.6281] |
0.92 | [1.3926, 1.6243] |
0.93 | [1.3972, 1.6206] |
0.94 | [1.4018, 1.6168] |
0.95 | [1.4063, 1.6130] |
0.96 | [1.4109, 1.6093] |
0.97 | [1.4154, 1.6055] |
0.98 | [1.4199, 1.6016] |
0.99 | [1.4244, 1.5978] |
1.00 | [1.4289, 1.5940] |
In this subsection, we will analyze the sensitivity of the left and right points of the α-cut of the fuzzy hedging portfolio of F with respect to the parameters r, σ and T. For this purpose, we take a control variable approach, changing one parameter at a time and keeping the other parameters constant. One thing to note is that the experiments were done at α=0.95 in this subsection.
Figure 2 shows the left and right points of the α-cut of the hedging portfolio ˜β against some model parameters. (a) reflects the relation of risk-free interest rate r and ˜βα, we find that the function of ˜βα with respect to of risk -free interest rate r is not monotonous. With the increases of r, the value of ˜βα firstly increases and then decreases but generally tends to be stable. (b) shows the relation of volatility σ and ˜βα, it is also not a monotonous function, but the overall trend is decreasing. (c) embodies the longer the maturity time, the smaller hedging portfolio ˜βα. Through the sensitivity analysis of the above parameters, we know that volatility is the biggest factor in the choice of hedge portfolio, which can be suspected by the fact that the greater volatility, the greater the price of the underlying asset, so we only need a smaller number of the portfolio.
In order to obtain the financial investors' subjective judgment about hedging portfolios, Yoshida in [4] proposes a kind of utility function method which can represent the investors' satisfaction with the portfolios that can be traded in the current market. Next, let us recall this definition. Considering a fuzzy goal by a fuzzy set ψ:R→[0,1], which is a continuous and increasing function with ψ(0)=0 and limx→∞ψ(x)=1. Then we can get the α-cut ψα=[ψ−α,∞] for every α∈[0,1]. The fuzzy expectation of fuzzy values ˜β which is given in Section 2.1 can be defined as
E(˜β)=∫R+˜β(x)d˜m(x)=supx∈R+min{˜β(x),φ(x)}, | (5.1) |
where ˜m is the possibility measure generated by density ψ and ∫d˜m denotes Sugeno integral. The fuzzy expectation (5.1) implies the degree of financial investors' satisfaction regarding fuzzy portfolio ˜β. Then the fuzzy goal ψ(x) means a kind of utility function for expected portfolio x in (5.1), it represents a financial investors' subjective judgment. It means that for a real number x∗∈R+ which is called a rational expected portfolio if it attains the supremum of the fuzzy expectation (5.1), i.e.,
E(˜β)=min{˜β(x∗),φ(x∗)}, |
then we have the following definition
α−=sup{α∈[0,1]:ψ−α≤(˜βUt)α},α+=sup{α∈[0,1]:ψ−α≤(˜βLt)α}, | (5.2) |
where α+, α− are the grade of the fuzzy expectation of lookback options hedging portfolio, and we can deduce that
ψ−α−=(˜βLt)−α,ψ+α+=(˜βLt)+α. | (5.3) |
According to Theorem 4.1 in [4], we can know that the rational expected portfolio of the lookback option is given by
x−=ψ−α−,x+=ψ−α+. | (5.4) |
Combine (5.2) and (5.4) we can get the interval [x−,x+], {which} can be written as
[x−,x+]={x∈R:˜βt≥ψ(x)}, | (5.5) |
which is the range of hedging portfolio x such that the reliability degree of the expected hedging portfolio, ˜β(x) is greater than the degree of financial investors' satisfaction, ψ(x). Therefore, this interval means financial investors' permissible range of expected hedging portfolio under their fuzzy goal.
Next, let us consider a fuzzy goal
ψ(x)={1−e−x,x≥00.x<0. |
Then we have ψ−α=−ln(1−α) for α∈(0,1). By (5.3) we can easily calculate the grade of the fuzzy expectation of the fuzzy hedging portfolio, respectively α−≈0.7275, a+≈0.8108. The grades means the degrees of financial investors' satisfaction in hedging. From (5.4), the corresponding permissible range of rational expected hedging portfolio with respect to the lookback option under one's fuzzy goal ψ is [x−,x+]≈[1.3002,1.6647]. In Figure 3, we can more intuitively comprehend this correspondence. When b=c=0, S1=S2=32, the crisp expected hedging portfolio is calculated as 1.4690, which is included in the interval (5.5). (5.5) shows the financial investor a confidence interval of expected hedging portfolio of lookback option under uncertainty.
In this paper, we proposed a hedging technique using fuzzy space Malliavin calculus for fixed strike lookback options. Our pricing model is based on the fuzzy stochastic differential equation in the Skorohod sense. With the help of the properties of nth fuzzy Malliavin derivative and its adjoint (divergence operator), we obtained the fuzzy Clark-Ocone formula, which plays a significant role in hedging. Then, we derived the explicit expression for the fuzzy hedging portfolio of fixed strike lookback options by reflection principle and Esscher transform. Finally, some numerical experiments are carried out. On the one hand, we proceeded the sensitivity analysis of the hedging portfolio βt. On the other hand, we considered the financial investors' subjective judgment, the permissible range of the expected hedging portfolio of fixed strike lookback options such that the reliability degree of the optimal expected hedging portfolio is greater than the degree of one's satisfaction is given.
However, two further extensions of the application of fuzzy malliavin calculus in hedging would be interesting. One possible extension is turning our model into multidimensional so that the hedging results are more consistent with the real market. Another extension would be to extend the research on the exotic options to make our conclusions more general.
This work is supported by the Education Department of Jilin Province (JJKH20200030KJ), the department of Science and Technology of Jilin Province (YDZJ202102ZYTS156), the project of natural science foundation joint fund of Jilin Province (YDZJ202101ZYTS167), and Beihua University Youth Research and Innovation Team. We also thank the referee and associate editors for their comments and suggestions, which led to numerous improvements.
The authors declare that they have no competing interests in this paper.
[1] | L. Zadeh, Fuzzy sets, Inform. Control, 8 (1965), 338–353. https://doi.org/10.1016/S0019-9958(65)90241-X |
[2] | P. Nowak, M. Romaniuk, Computing option price for levy process with fuzzy parameters, Eur. J. Oper. Res., 201 (2010), 206–210. https://doi.org/10.1016/j.ejor.2009.02.009 |
[3] | H. C. Wu, Using fuzzy sets theory and black-scholes formula to generate pricing boundaries of european options, Appl. Math. Comput., 185 (2007), 136–146. https://doi.org/10.1016/j.amc.2006.07.015 |
[4] | Y. Yoshida, The valuation of european options in uncertain environment, Eur. J. Oper. Res., 145 (2003), 221–229. https://doi.org/10.1016/S0377-2217(02)00209-6 |
[5] | X. Wang, J. He, A geometric levy model for n-fold compound option pricing in a fuzzy framework, J. Comput. Appl. Math., 306 (2016), 248–264. https://doi.org/10.1016/j.cam.2016.04.021 |
[6] | H. C. Wu, Pricing european options based on the fuzzy pattern of black-scholes formula, Comput. Oper. Res., 31 (2004), 1069–1081. https://doi.org/10.1016/S0305-0548(03)00065-0 |
[7] | V. Lakshmikantham, R. Mohapatra, Theory of fuzzy differential equations and inclusions, Nonlinear Anal.-Theor., 1 (2003). https://doi.org/10.1201/9780203011386 |
[8] |
Y. Feng, Fuzzy stochastic differential systems, Fuzzy Set. Syst., 115 (2000), 351–363. https://doi.org/10.1016/S0165-0114(98)00389-3 doi: 10.1016/S0165-0114(98)00389-3
![]() |
[9] | O. Kaleva, Fuzzy differential equations, Fuzzy Set. Syst., 24 (1987), 301–317. https://doi.org/10.1016/0165-0114(87)90029-7 |
[10] |
H. Kwakernaak, Fuzzy random variables—I. definitions and theorems, Inform. Sciences, 15 (1978), 1–29. https://doi.org/10.1016/0020-0255(78)90019-1 doi: 10.1016/0020-0255(78)90019-1
![]() |
[11] | M. L. Puri, D. A. Ralescu Fuzzy random variables, Read. Fuzzy Set. Intell. Syst., 1993,265–271. https://doi.org/10.1016/B978-1-4832-1450-4.50029-8. |
[12] |
M. Michta, K. Ł. Świkatek, Two-parameter fuzzy-valued stochastic integrals and equations, Stoch. Anal. Appl., 33 (2015), 1115–1148. https://doi.org/10.1080/07362994.2015.1089516 doi: 10.1080/07362994.2015.1089516
![]() |
[13] |
M. T. Malinowski, M. Michta, J. Sobolewska, Set-valued and fuzzy stochastic differential equations driven by semimartingales, Nonlinear Anal.-Theor., 79 (2013), 204–220. https://doi.org/10.1016/j.na.2012.11.015 doi: 10.1016/j.na.2012.11.015
![]() |
[14] | M. T. Malinowski, M. Michta, Stochastic fuzzy differential equations with an application, Kybernetika, 47 (2011), 123–143. |
[15] |
W. Fei, Existence and uniqueness of solution for fuzzy random differential equations with non-lipschitz coefficients, Inform. Sciences, 177 (2007), 4329–4337. https://doi.org/10.1016/j.ins.2007.03.004 doi: 10.1016/j.ins.2007.03.004
![]() |
[16] |
M. T. Malinowski, Itô type stochastic fuzzy differential equations with delay, Syst. Control Lett., 61 (2012), 692–701. https://doi.org/10.1016/j.sysconle.2012.02.012 doi: 10.1016/j.sysconle.2012.02.012
![]() |
[17] |
M. T. Malinowski, Strong solutions to stochastic fuzzy differential equations of Itô type, Math. Comput. Model., 55 (2012), 918–928. https://doi.org/10.1016/j.mcm.2011.09.018 doi: 10.1016/j.mcm.2011.09.018
![]() |
[18] |
M. T. Malinowski, Some properties of strong solutions to stochastic fuzzy differential equations, Inform. Sciences, 252 (2013), 62–80. https://doi.org/10.1016/j.ins.2013.02.053 doi: 10.1016/j.ins.2013.02.053
![]() |
[19] |
W. Fei, H. Liu, W. Zhang, On solutions to fuzzy stochastic differential equations with local martingales, Syst. Control Lett., 65 (2014), 96–105. https://doi.org/10.1016/j.sysconle.2013.12.009 doi: 10.1016/j.sysconle.2013.12.009
![]() |
[20] |
H. Jafari, Sensitivity of option prices via fuzzy malliavin calculus, Fuzzy Set. Syst., 434 (2022), 98–116. https://doi.org/10.1016/j.fss.2021.11.005 doi: 10.1016/j.fss.2021.11.005
![]() |
[21] |
H. Li, A. Ware, L. Di, G. Yuan, A. Swishchuk, S. Yuan, The application of nonlinear fuzzy parameters pde method in pricing and hedging european options, Fuzzy Set. Syst., 331 (2018), 14–25. https://doi.org/10.1016/j.fss.2016.12.005 doi: 10.1016/j.fss.2016.12.005
![]() |
[22] |
X. Yu, W. Huang, Y. Tan, L. Liu, The optimal fuzzy portfolio strategy with option hedging, Theor. Math. Appl., 2 (2012), 1–12. https://doi.org/10.1166/asl.2012.2662 doi: 10.1166/asl.2012.2662
![]() |
[23] | Y. Yoshida, M. Yasuda, J. I. Nakagami, M. Kurano, A new evaluation of mean value for fuzzy numbers and its application to american put option under uncertainty, Fuzzy Set. Syst., 157 (2006), 2614–2626. https://doi.org/10.1016/j.fss.2003.11.022 |
[24] |
F. Escher, On the probability function in the collective theory of risk, Skand. Aktuar. J., 15 (1932), 175–195. https://doi.org/10.1080/03461238.1932.10405883 doi: 10.1080/03461238.1932.10405883
![]() |
[25] | H. U. Gerber, E. S. W. Shiu, Option pricing by esscher transforms, HEC Ecole des hautes études commerciales, Paris, 1994. https://doi.org/10.1016/0167-6687(95)97170-Y |
[26] |
L. Stefanini, L. Sorini, M. L. Guerra, W. Pedrycz, A. Skowron, V. Kreinovich, Fuzzy numbers and fuzzy arithmetic, Handb. Granul. Comput., 12 (2008), 249–284. https://doi.org/10.1002/9780470724163.ch12 doi: 10.1002/9780470724163.ch12
![]() |
[27] |
B. K. Kim, J. H. Kim, Stochastic integrals of set-valued processes and fuzzy processes, J. Math. Anal. Appl., 236 (1999), 480–502. https://doi.org/10.1006/jmaa.1999.6461 doi: 10.1006/jmaa.1999.6461
![]() |
[28] |
G. Wang, Y. Zhang, The theory of fuzzy stochastic processes, Fuzzy Set. Syst., 51 (1992), 161–178. https://doi.org/10.1016/0165-0114(92)90189-B doi: 10.1016/0165-0114(92)90189-B
![]() |
[29] |
Y. K. Kim, Measurability for fuzzy valued functions, Fuzzy Set. Syst., 129 (1993), 105–109. https://doi.org/10.1016/S0165-0114(01)00121-X doi: 10.1016/S0165-0114(01)00121-X
![]() |
[30] |
J. Li, J. Wang, Fuzzy set-valued stochastic lebesgue integral, Fuzzy Set. Syst., 200 (2012), 48–64. https://doi.org/10.1016/j.fss.2012.01.021 doi: 10.1016/j.fss.2012.01.021
![]() |
[31] |
H. Jafari, H. Farahani, M. Paripour, Fuzzy malliavin derivative and linear skorohod fuzzy stochastic differential equation, J. Intell. Fuzzy Syst., 35 (2018), 2447–2458. https://doi.org/10.3233/JIFS-18043 doi: 10.3233/JIFS-18043
![]() |
[32] | M. Kisielewicz, Differential inclusions and optimal control, Springer, Netherlands, 1991. |
[33] |
M. T. Malinowski, M. Michta, Fuzzy stochastic integral equations, Dyn. Syst. Appl., 19 (2010), 473. https://doi.org/10.1016/j.amc.2013.05.040 doi: 10.1016/j.amc.2013.05.040
![]() |
[34] | B. Bede, L. Stefanini, Generalized differentiability of fuzzy-valued functions, Fuzzy Set. Syst., 230 (2013), 119–141. https://doi.org/10.1016/j.fss.2012.10.003 |
[35] | L. T. Gomes, L. C. Barros, A note on the generalized difference and the generalized differentiability, Fuzzy Set. Syst., 280 (2015), 142–145. https://doi.org/10.1016/j.fss.2015.02.015 |
[36] | I. Nourdin, G. Peccati, Normal approximations with Malliavin calculus: From Stein's method to universality, Cambridge University Press, USA, 2012. http://dx.doi.org/10.1017/CBO9781139084659.003 |
[37] | G. D. Nunno, B. Øksendal, F. Proske, Malliavin calculus for Lévy processes with applications to finance, Springer, Berlin, 2009. https://doi.org/10.1007/978-3-540-78572-9 |
[38] |
D. Nualart, É. Pardoux, Stochastic calculus with anticipating integrands, Prob. Theory Rel., 78 (1988), 535–581. https://doi.org/10.1007/BF00353876 doi: 10.1007/BF00353876
![]() |
[39] |
H. P. Bermin, Hedging lookback and partial lookback options using malliavin calculus, Appl. Math. Financ., 7 (2000), 75–100. https://doi.org/10.1080/13504860010014052 doi: 10.1080/13504860010014052
![]() |
[40] |
H. Lee, E. Kim, B. Ko, Valuing lookback options with barrier, N. Am. J. Econ. Financ., 60 (2022), 101660. https://doi.org/10.1016/j.najef.2022.101660 doi: 10.1016/j.najef.2022.101660
![]() |
[41] |
W. Zhang, Z. Li, Y. Liu, Y. Zhang, Pricing European option under fuzzy mixed fractional brownian motion model with jumps, Comput. Econ., 58 (2021), 483–515. https://doi.org/10.1007/s10614-020-10043-z doi: 10.1007/s10614-020-10043-z
![]() |
1. | Jorge de Andrés-Sánchez, Fuzzy Random Option Pricing in Continuous Time: A Systematic Review and an Extension of Vasicek’s Equilibrium Model of the Term Structure, 2023, 11, 2227-7390, 2455, 10.3390/math11112455 |
α | [˜βLα,˜βUα] |
0.90 | [1.3833, 1.6318] |
0.91 | [1.3880, 1.6281] |
0.92 | [1.3926, 1.6243] |
0.93 | [1.3972, 1.6206] |
0.94 | [1.4018, 1.6168] |
0.95 | [1.4063, 1.6130] |
0.96 | [1.4109, 1.6093] |
0.97 | [1.4154, 1.6055] |
0.98 | [1.4199, 1.6016] |
0.99 | [1.4244, 1.5978] |
1.00 | [1.4289, 1.5940] |