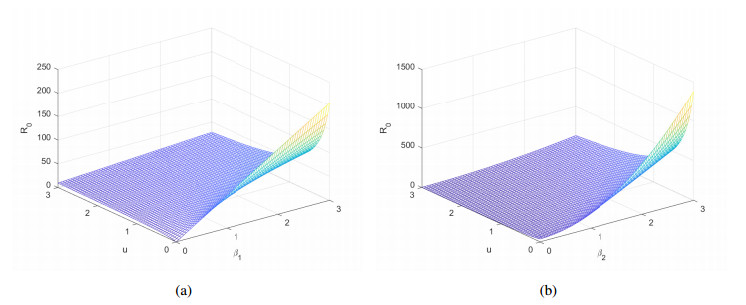
The virus mutation can increase the complexity of the infectious disease. In this paper, the dynamical characteristics of the virus mutation model are discussed. First, we built a stochastic virus mutation model with time delay. Second, the existence and uniqueness of global positive solutions for the proposed model is proved. Third, based on the analysis of the ergodic stationary distribution for the model, we discuss the influence mechanism between the different factors. Finally, the numerical simulation verifies the theoretical results.
Citation: Juan Ma, Shaojuan Ma, Xinyu Bai, Jinhua Ran. Ergodic stationary distribution of stochastic virus mutation model with time delay[J]. AIMS Mathematics, 2023, 8(9): 21371-21392. doi: 10.3934/math.20231089
[1] | Chuangliang Qin, Jinji Du, Yuanxian Hui . Dynamical behavior of a stochastic predator-prey model with Holling-type III functional response and infectious predator. AIMS Mathematics, 2022, 7(5): 7403-7418. doi: 10.3934/math.2022413 |
[2] | Xiaodong Wang, Kai Wang, Zhidong Teng . Global dynamics and density function in a class of stochastic SVI epidemic models with Lévy jumps and nonlinear incidence. AIMS Mathematics, 2023, 8(2): 2829-2855. doi: 10.3934/math.2023148 |
[3] | Ying He, Bo Bi . Conditions for extinction and ergodicity of a stochastic Mycobacterium tuberculosis model with Markov switching. AIMS Mathematics, 2024, 9(11): 30686-30709. doi: 10.3934/math.20241482 |
[4] | Yuhuai Zhang, Xinsheng Ma, Anwarud Din . Stationary distribution and extinction of a stochastic SEIQ epidemic model with a general incidence function and temporary immunity. AIMS Mathematics, 2021, 6(11): 12359-12378. doi: 10.3934/math.2021715 |
[5] | Chaodong Chen, Dapeng Gao, Peng Guo . The stationary distribution of a stochastic rumor spreading model. AIMS Mathematics, 2021, 6(2): 1234-1248. doi: 10.3934/math.2021076 |
[6] | Ying He, Bo Bi . Threshold dynamics and density function of a stochastic cholera transmission model. AIMS Mathematics, 2024, 9(8): 21918-21939. doi: 10.3934/math.20241065 |
[7] | Hanan S. Gafel, Saima Rashid, Sayed K. Elagan . Novel codynamics of the HIV-1/HTLV-Ⅰ model involving humoral immune response and cellular outbreak: A new approach to probability density functions and fractional operators. AIMS Mathematics, 2023, 8(12): 28246-28279. doi: 10.3934/math.20231446 |
[8] | Jinji Du, Chuangliang Qin, Yuanxian Hui . Optimal control and analysis of a stochastic SEIR epidemic model with nonlinear incidence and treatment. AIMS Mathematics, 2024, 9(12): 33532-33550. doi: 10.3934/math.20241600 |
[9] | Yue Dong, Xinzhu Meng . Stochastic dynamic analysis of a chemostat model of intestinal microbes with migratory effect. AIMS Mathematics, 2023, 8(3): 6356-6374. doi: 10.3934/math.2023321 |
[10] | Abdon Atangana, Saima Rashid . Analysis of a deterministic-stochastic oncolytic M1 model involving immune response via crossover behaviour: ergodic stationary distribution and extinction. AIMS Mathematics, 2023, 8(2): 3236-3268. doi: 10.3934/math.2023167 |
The virus mutation can increase the complexity of the infectious disease. In this paper, the dynamical characteristics of the virus mutation model are discussed. First, we built a stochastic virus mutation model with time delay. Second, the existence and uniqueness of global positive solutions for the proposed model is proved. Third, based on the analysis of the ergodic stationary distribution for the model, we discuss the influence mechanism between the different factors. Finally, the numerical simulation verifies the theoretical results.
Different mathematical models have been used to study the dynamical behavior of infectious diseases by many researchers. Stone et al. [1] analyzed classical models of seasonal forced SIR epidemics and to further understand the nonlinear dynamics of recurrent disease. A cutaneous leishmaniasis disease model was proposed by Sinan et al. [2], who investigated the basic properties of the model. Sheergojri et al. [3] built a mathematical model of tumor development using a fuzzy logic method. Sabbar [4] studied the conditions for asymptotic extinction and sustained survival in a stochastic infectious disease model with different intervention measures. The dynamical behavior of the fractional-order models for infectious disease was studied in [5,6,7]. Ahmadet et al. [8] built a fractional-order model about COVID-19 to predict the second wave of COVID-19 transmission in Pakistan in the next 50 days.
In recent years, during the environmental change, the virus will mutate due to the influence of various factors in the transmission process, which is expected to lead to a large-scale outbreak of diseases [9,10,11]. With the normalization of COVID-19 prevention and control, the researchers paid more attention to the virus mutation models [12,13,14]. Cacciapaglia et al. [15] used a modified SIR model to simulate the interplay and competition of COVID-19 virus variants within a given population. Dobie [16] discussed the stability of a model based on horizontal and vertical transmissions, size variation and virus mutation. Avila-Ponce de León et al. [17] applied mathematical models to simulate the potential effects of the Omicron variant on the USA population. A study with multiple vaccinations and virus mutations found that early vaccination is the key to reducing disease mutations [18]. Meanwhile, utilizing the principle of epidemic dynamics, Liu et al. [19] established an SEIR model with a mutated virus in wireless sensor networks, which had guiding significance to control the spread of a mutated virus. Xu et al. [20] proposed a complex network model with virus variation and considered the influence of virus variation factors on network transmission.
Due to the pathogenesis and epidemic law of infectious diseases, some scholars considered stochastic and time delays for an infectious disease model. Liu et al. [21] studied the dynamics of a stochastic delayed SIR epidemic model with dual diseases and vaccination driven by Lˊevy jumps. Zhang and Liu [22] analyzed the sufficient conditions for extinction and persistence of a stochastic delayed model with vertical transmission and vaccination. The stochastic delayed SIRS model was investigated by Xu and Li [23] found that the immunity period of vaccination would affect the threshold of extinction or persistence. In [24,25], stochastic delayed models with Markov switching was discussed. A delayed differential model of the tumor and immune system with noise was investigated by Alsakaji et al. [26]. Ali and Khan [27] proposed a stochastic delayed SIRS model with exponential birth and saturated incidence rates, and they found that a large amount of noise would lead to the extinction of the disease. In order to further study the persistent effects of stochastic disturbance on models with time delays, many scholars considered the ergodic stationary distribution of stochastic delayed models [28]. Khan et al. [29] proposed an epidemic model of SEIQ with stochastic disturbance and time delay, they used the Lyapunov function to analyze the ergodic stability. In [30], the ergodic stationary distribution of a hepatitis B virus system with a higher-order stochastic delayed differential model is investigated. Ikram et al. [31] built a stochastic delayed COVID-19 model with cross-immune class and transmission terms to study the effects of white noise on disease extinction. Liu et al. [32] used Markov semigroup theory to obtain the existence and uniqueness of ergodic stationary distribution. Sun et al. [33] studied the ergodic stationary distribution of a stochastic viral model with cytotoxic T lymphocyte responsiveness and time delay. By analyzing the threshold conditions for the persistence of ergodic stationary distribution, the effects of time delay and noise on disease were obtained [34]. Although the scholars have studied a lot on ergodic stability analysis for infectious disease models with noise and time delay, there are few studies on ergodic stability analysis of mutation models. In this paper, by considering the time delay from pre-mutation to post-mutation in the stochastic mutation model, we study the influence of different factors on the persistence and extinction for a model based on analyzing the ergodic stationary distribution.
This paper is presented as follows. In Section 2, we set up a stochastic virus mutation model with time delay. The existence and uniqueness of global positive solutions for the model are given in Section 3. In Section 4, we obtain the ergodic stationary distribution of the stochastic model with time delay. In the last section, the theoretical results are verified by numerical simulations.
After the virus infects the susceptible individuals and goes through an incubation period, some of the latent individuals will transform into infected individuals. During this period, the virus will mutate due to environmental factors and the infected individuals are either pre-mutation individuals or post-mutation individuals. Both groups of infected individuals are infectious during this period of treatment. Considering that the virus will mutate within a certain period of time τ, the SEIR model with virus variation and time delay is established. Besides, the following assumptions were taken into consideration while formulating the model:
1) All initial population sizes are non-negative.
2) Variables and parameters are non-negative.
3) All groups of individuals experienced natural death.
4) τ is the time delay before and after virus mutation.
Considering the above assumptions, the deterministic virus mutation model with time delay can be expressed by the following differential equations in Eq (2.1):
{dS(t)dt=A−dS(t)−β1S(t)I1(t)−β2S(t)I2(t),dE(t)dt=β1S(t)I1(t)+β2S(t)I2(t)−(ε+d)E(t),dI1(t)dt=εE(t)−(d+r1)I1(t)−uI1(t−τ),dI2(t)dt=uI1(t−τ)−(d+r2)I2(t),dR(t)dt=r1I1(t)+r2I2(t)−dR(t), | (2.1) |
where S(t), E(t), I1(t), I2(t), R(t) denote the sizes of susceptible (S), latent (E), pre-mutation patient (I1), post-mutation patient (I2) and recovered individuals (R), respectively, which satisfy N(t)=S(t)+E(t)+I1(t)+I2(t)+R(t). The parameters in Eq (2.1) have the following meanings: A is the birth rate, β1 and β2 represent the coefficients of patient infection rate before and after virus mutation respectively, u is the rate at which the pre-mutation patient becomes the post-mutation patient, ε represents the rate at which the latent person becomes the pre-mutation patient, r1 and r2 are the patient recovery rate before and after virus mutation respectively, τ is the time delay before and after virus mutation and d is the death rate. All parameters are positive. The recovered population R(t) has no effect on the dynamics of S(t), E(t), I1(t) I2(t), so the following simplified model can be obtained:
{dS(t)dt=A−dS(t)−β1S(t)I1(t)−β2S(t)I2(t),dE(t)dt=β1S(t)I1(t)+β2S(t)I2(t)−(ε+d)E(t),dI1(t)dt=εE(t)−(d+r1)I1(t)−uI1(t−τ),dI2(t)dt=uI1(t−τ)−(d+r2)I2(t), | (2.2) |
set Ω={S(t),E(t),I1(t),I2(t)|S(t)≥0,E(t)≥0,I1(t)≥0,I2(t)≥0}, and the basic reproductive number of Eq (2.2) is
R0=Aβ1ε(d+r2)+Aβ2εu(ε+d)(d+r1+u)(d+r2)d. |
The dynamical behavior of Eq (2.2) is obtained as follows:
(ⅰ) If R0≤1, Eq (2.2) has the unique disease-free equilibrium
E0=(S0,0,0,0)=(Ad,0,0,0), |
which is globally asymptotically stable.
(ⅱ) If R0>1, disease-free equilibrium E0 is unstable and Eq (2.2) has a unique positive endemic equilibrium E1=(S∗,E∗,I∗1,I∗2), which is globally asymptotically stable, where
S∗=dd+β1I1∗+β2uI1∗d+r2,E∗=(d+r1+u)I1∗ε,I1∗=d(R0−1)β1+β2ud+r2,I2∗=uI1∗d+r2. |
The sensitivity analysis of R0 in Figure 1 shows that the related parameters β1 and β2 each have a great effect on disease transmission. Considering that biological systems are inevitably disturbed by uncertain environmental factors, we use white noise to represent stochastic interference, which can be represented by external interventions, policy interventions, drug therapy, media reports, vaccination and so on. In this paper, Gaussian white noise represents stochastic disturbance and the stochastic model with time delay is proposed as follows:
{dS(t)=[A−dS(t)−β1S(t)I1(t)−β2S(t)I2(t)]dt+σ1S(t)dB1(t),dE(t)=[β1S(t)I1(t)+β2S(t)I2(t)−(ε+d)E(t)]dt+σ2E(t)dB2(t),dI1(t)=[εE(t)−(d+r1)I1(t)−uI1(t−τ)]dt+σ3I1(t)dB3(t),dI2(t)=[uI1(t−τ)−(d+r2)I2(t)]dt+σ4I2(t)dB4(t), | (2.3) |
where Bi(t)(i=1,2,3,4) denotes independent standard Brownian motions and σi>0(i=1,2,3,4) represents the intensities of Bi(t). The variables in Eq (2.3) are constrained by the following initial conditions:
{S(θ)=ψ1(θ),E(θ)=ψ2(θ),I1(θ)=ψ3(θ),I2(θ)=ψ4(θ),θ∈[−τ,0], | (2.4) |
where ψi(θ)∈C(i=1,2,3,4) such that C is a family of Lebesgue integrable functions from [−τ,0] to R4+.
To study the dynamical behavior of a stochastic infectious model with time delay, it is necessary to verify that the solution of Eq (2.3) is non-negative and has a unique global positive solution (that is no explosion in finite time), so we have the following theorem.
Theorem 1. For the any given initial value (2.4), the system (2.3) has a unique positive solution (S(t),E(t),I1(t),I2(t)) on t≥−τ, and the solution remains in R4+ with a probability of 1.
Proof: For the given initial approximation {S(0),E(0),I1(0),I2(0)}, the coefficients of Eq (2.3) are locally Lipschitz continuous, so there is a unique local solution {S(t),E(t),I1(t),I2(t)} on t∈[−τ,τe], where τe refers the explosion time. In order to show that this solution is global, we need to show that τe=∞ is true. Assume that ξ0≥1 is so large that S(θ)∈[1ξ0,ξ0], E(θ)∈[1ξ0,ξ0], I1(θ)∈[1ξ0,ξ0], I2(θ)∈[1ξ0,ξ0] and θ∈[−τ,0] are all satisfied. For each integer ξ>ξ0(ξ>0), define the stopping time as follows:
τξ=inf{t∈[−τ,τe):min{S(t),E(t),I1(t),I2(t)}≤1ξ,ormax{S(t),E(t),I1(t),I2(t)}≥ξ}. |
Consider infϕ=∞(ϕisvoidset). τξ is increasing as ξ→∞. Assume that τ∞=limn→∞τn and let τ∞≤τe a.s. Hence, we need to prove that τ∞=∞ a.s., then τe=∞ a.s. and {S(0),E(0),I1(0),I2(0)}∈R4+ a.s. Otherwise, there exist the constants T>0 and δ∈(0,1) which yield that P(τ∞≤T)>δ. Then there exist the integers ξ1>ξ0 which take
P{τξ≤T}≥δ,∀ξ>ξ1. | (3.1) |
Define a C2-function V:R4+→R+ as follows:
V(S,E,I1,I2)=(S−a−alnSa)+(E−1−lnE)+(I1−1−lnI1)+(I2−1−lnI2)+∫t+τtauI1(s−τ)ds, |
where a>0 is a constant. According to Itˆo′s formula
dV(S,E,I1,I2)=LVdt+σ1(S−a)dB1(t)+σ2(E−1)dB2(t)+σ3(I1−1)dB3(t)+σ4(I2−1)dB4(t), |
where
LV=(1−aS)[A−dS−β1SI1−β2SI2]+(1−1E)[β1SI1+β2SI2−(ε+d)E]+(1−1I1)[εE−(d+r1)I1−uI1(t−τ)]+(1−1I2)[uI1(t−τ)−(d+r2)I2]+aσ212+σ222+σ232+σ242+auI1−auI1(t−τ)≤A−dS−β1SI1−β2SI2−AaS+aSdS+aSβ1SI1+aSβ2SI2+β1SI1+β2SI2−(ε+d)E−1Eβ1SI1−1Eβ2SI2+εE−(d+r1)I1−uI1(t−τ)−1I1εE+d+r1+uI1I1(t−τ)+uI1(t−τ)−(d+r2)I2(t)−1I2uI1(t−τ)+d+r2≤A+3d+ε+r1+r2+ad+I1(au+aβ1−d−r1)+aσ21+σ22+σ23+σ242, |
let a=d+r1u+β1, then we can obtain
LV≤A+ε+r1+r2+d(a+3)+aσ21+σ22+σ23+σ242≤K1, | (3.2) |
where K1>0, we can get
dV(S,E,I1,I2)≤K1dt+σ1(S−a)dB1(t)+σ2(E−1)dB2(t)+σ3(I1−1)dB3(t)+σ4(I2−1)dB4(t), | (3.3) |
integrate both sides of Eq (3.3) from 0 to τξ∧T, and then obtain
∫τξ∧T0dV(S,E,I1,I2)≤∫τξ∧T0K1dt+∫τξ∧T0σ1(S−a)dB1(t)+∫τξ∧T0σ2(E−1)dB2(t)+∫τξ∧T0σ3(I1−1)dB3(t)+∫τξ∧T0σ4(I2−1)dB4(t), | (3.4) |
take the expectation of both sides of Eq (3.4)
EV[S(τξ∧T),E(τξ∧T),I1(τξ∧T),I2(τξ∧T)]≤EV(S(0),E(0),I1(0),I2(0))+K1T. |
Let Ωξ={τξ≤T}, for ξ>ξ1 and in view of Eq (3.1), we obtain P(Ωξ)≥δ such that for each w∈Ωξ there is at least one among S(τξ,w),E(τξ,w),I1(τξ,w)andI2(τξ,w) that is equal either ξ or 1ξ. Then
V[S(τξ∧T),E(τξ∧T),I1(τξ∧T),I2(τξ∧T)]≥min{ξ−1−lnξ,1ξ−1−ln1ξ}, |
it follows that
EV(S(0),E(0),I1(0),I2(0))+K1T≥E[1ΩξV(S(τξ∧T),E(τξ∧T),I1(τξ∧T),I2(τξ∧T))]≥δ(ξ−1−lnξ)∧(1ξ−1−ln1ξ), |
where 1Ωξ is the indicative function of Ωξ. Taking ξ→∞ gives
∞>EV(S(0),E(0),I1(0),I2(0))+K1T=∞. |
This contradicts the initial condition, so τ∞=∞ holds almost everywhere. Therefore, Theorem 1 is proved.
In this section, we construct an appropriate random Lyapunov function to study the existence and uniqueness of the ergodic stationary distribution of Eq (2.3). Taking X(t) as a regular time-homogeneous Markov process defined in d dimensional Euclidean space Ed, which can be expressed by the stochastic differential equation
dX(t)=d∑i=1gr(t,X(t))dBr(t)+f(X(t−τ),X(t),t)dt, |
the corresponding diffusion matrix is
A(X)=(aij(x)),aij(x)=k∑r=1hir(x)hjr(x). |
Lemma 1. ([35]) The Markov process of X(t) has one ergodic stationary distribution π(⋅) and a bounded domain U⊂Rd with the regular boundary Γ, then the following is true:
(ⅰ) There is a positive number K such that d∑i,j=1aij(x)ξiξj≥K|ξ|2, x∈U, ξ∈Rd.
(ⅱ) There is a non negative C2 operator V such that LV is negative for any Rd∖U.
Then Markov process X(t) has a unique ergodic stationary distribution π(⋅).
In order to get the conditions of the existence of ergodic stationary distribution, we set Theorem 2.
Theorem 2. Define
R∗=Auε(d+σ212)(d+ε+σ222)(d+r1+σ232)(d+r2+σ242), |
assuming R∗>1 and d−σ21∨σ22∨σ23∨σ242>0, then, for any given initial value (S(0),E(0),I1(0),I2(0))∈R4+, the solution (S(t),E(t),I1(t),I2(t)) of Eq (2.3) is normal and has a unique ergodic stationary distribution π(⋅).
Proof: To prove Theorem 2, we need to verify that conditions (ⅰ) and (ⅱ) in Lemma 1 are satisfied. First, we verify (ⅱ).
Define a C2-function V:R4+→R+ as follows:
V(S,E,I1,I2)=M(−lnS−a1lnE−a2lnI1−a3lnI2+u∫t+τtI1(S−τ)ds)−lnS+u∫t+τtI1(S−τ)ds−lnI1−lnI2+1m+1(S+E+I1+I2)m+1=MV1+V2+V3+V4+V5, | (4.1) |
where ai(i=1,2,3) is a positive constant. Notice that V(S,E,I1,I2) is not only continuous, but also tends to ∞ as (S,E,I1,I2) approaches the boundary of R4+. So it must be lower bounded and achieve this lower bound at a point (S(0),E(0),I1(0),I2(0)) in the interior of R4+. Thus the C2-function ˜V can be defined as follows:
˜V(S,E,I1,I2)=M(−lnS−a1lnE−a2lnI1−a3lnI2+u∫t+τtI1(S−τ)ds)−lnS+u∫t+τtI1(S−τ)ds−lnI1−lnI2+1m+1(S+E+I1+I2)m+1−V(S(0),E(0),I1(0),I2(0))=MV1+V2+V3+V4+V5−V(S(0),E(0),I1(0),I2(0)), | (4.2) |
where (S,E,I1,I2)∈(1n,n)×(1n,n)×(1n,n)×(1n,n), n>1 is a sufficiently large integer. According to Eq (4.2), V1, V2, V3, V4, V5 are respectively defined as follows:
V1=−lnS−a1lnE−a2lnI1−a3lnI2+u∫t+τtI1(S−τ)ds, |
V2=−lnS+u∫t+τtI1(S−τ)ds, |
V3=−lnI1,V4=−lnI2, |
V5=1m+1(S+E+I1+I2)m+1. |
Itˆo′s formula is applied to V1 as follows:
LV1=−AS+d+β1I1+β2I2+σ212−a1Eβ1SI1−a1Eβ2SI2+a1(ε+d)+a1σ222−a2I1εE+a2(d+r1)+a2I1uI1(t−τ)+a2σ232−a3I2uI1(t−τ)+a3(d+r2)+a3σ242+uI1−uI1(t−τ)≤−33√Auεa1a2a3+d+σ212+a1(ε+d+σ222)+a2(d+r1+σ232)+a3(d+r2+σ242)+β1I1+β2I2+uI1=−φ+I1(β1+u), |
where
φ=Auε(ε+d+σ222)(d+r2+σ242)(d+r1+σ232)−(d+σ212)>0, |
a1=Auε(d+ε+σ222)2(d+r1+σ232)(d+r2+σ242),a2=Auε(d+ε+σ222)(d+r1+σ232)2(d+r2+σ242),a3=Auε(d+ε+σ222)(d+r1+σ232)(d+r2+σ242)2. |
Applying Itˆo′s formula to V2,V3,V4,V5, we can get
LV2=−AS+d+β1I1+β2I2+uI1−uI1(t−τ)+σ212, |
LV3=−1I1εE−(d+r1)+uI1(t−τ)I1+σ232, |
LV4=−uI1(t−τ)I2+d+r2+σ242, |
LV5=(S+E+I1+I2)m[A−d(S+E+I1+I2)]+m2(S+E+I1+I2)m−1×[σ21S2+σ22E2+σ23I12+σ24I22]≤(S+E+I1+I2)m[A−d(S+E+I1+I2)]+m2(S+E+I1+I2)m+1×[σ21∨σ22∨σ23∨σ24]≤A(S+E+I1+I2)m−(S+E+I1+I2)m+1[d−m2(σ21∨σ22∨σ23∨σ24)]≤C−12[d−m2(σ21∨σ22∨σ23∨σ24)](Sm+1+Em+1+I1m+1+I2m+1), |
where
C=sup(S,E,I1,I2)∈R4+{A(S+E+I1+I2)m−12[d−m2(σ21∨σ22∨σ23∨σ24)](S+E+I1+I2)m+1}<∞. |
So, applying Itˆo′s formula to ˜V, we can get
L˜V=M[−φ+I1(β1+u)]+d−AS+β1I1+β2I2+uI1−uI1(t−τ)+σ212−1I1εE+d+r1+uI1(t−τ)I1+σ232−uI1(t−τ)I2+d+r2+σ242+C−12[d−m2(σ21∨σ22∨σ23∨σ24)](Sm+1+Em+1+I1m+1+I2m+1)≤−Mφ+I1M(β1+u)−14[d−m2(σ21∨σ22∨σ23∨σ24)](Sm+1+Em+1+Im+11+Im+12)−AS−14[d−m2(σ21∨σ22∨σ23∨σ24)]Im+11+3d+r1+r2+σ212+σ232+σ242+β2I2−uI1(t−τ)−1I1εE+uI1(t−τ)I1−uI1(t−τ)I2+C, |
m>1 is a constant that satisfies
d>m2(σ21∨σ22∨σ23∨σ24), |
M is a sufficiently large value satisfying the following condition
−Mφ+ξ≤−2, |
where
ξ=sup(S,E,I1,I2)∈R4+(−14[d−m2(σ21∨σ22∨σ23∨σ24)]Im+11+3d+r1+r2+C+σ212+σ232+σ242). |
Now we construct compact subsets U such that condition (ⅱ) in Lemma 1 is satisfied. We define bounded closed sets as follows:
U={(S,E,I1,I2)∈R4+:δ≤S≤1δ,δ≤E≤1δ,δ2≤I1≤1δ2,δ3≤I2≤1δ3}. |
For δ>0, in the set R4+∖U, we choose δ to satisfy the following conditions:
{−Aδ+D≤−1,−Mφ+Mδ2(β1+u)+ξ≤−1,−εδ+D≤−1,−uδ+D≤−1,−14[d−m2(σ21∨σ22∨σ23∨σ24)]1δm+1+D≤−1,−14[d−m2(σ21∨σ22∨σ23∨σ24)]1δ2(m+1)+D≤−1,−14[d−m2(σ21∨σ22∨σ23∨σ24)]1δ3(m+1)+D≤−1, | (4.3) |
where
D=sup(S,E,I1,I2){MI1(β1+u)−14[d−m2(σ21∨σ22∨σ23∨σ24)]Im+11+3d+r1+r2+C+σ212+σ232+σ242}. |
Then, we prove that L˜V≤−1 holds for any (S,E,I1,I2)∈R4+∖U by dividing R4+∖U into eight regions R4+∖U=U8i=1Ui as follows:
U1={(S,E,I1,I2)∈R4+;0<S<δ},U2={(S,E,I1,I2)∈R4+;0<E<δ},U3={(S,E,I1,I2)∈R4+;0<I1<δ2,E≥δ},U4={(S,E,I1,I2)∈R4+;0<I2<δ3,I1≥δ2},U5={(S,E,I1,I2)∈R4+;S≥1δ},U6={(S,E,I1,I2)∈R4+;E≥1δ},U7={(S,E,I1,I2)∈R4+;I1≥1δ2},U8={(S,E,I1,I2)∈R4+;I2≥1δ3}. |
We prove that L˜V(S,E,I1,I2)≤−1 holds for any (S,E,I1,I2)∈R4+∖U. This is equivalent to proving that it holds in each of the above right regions.
Case 4.1. If (S,E,I1,I2)∈U1, we get
L˜V≤−AS+MI1(u+β1)−14[d−m2(σ21∨σ22∨σ23∨σ24)]Im+11+3d+r1+r2+C+σ212+σ232+σ242≤−Aδ+D. | (4.4) |
Case 4.2. If (S,E,I1,I2)∈U2, we get
L˜V≤−Mφ+MI1(β1+u)−14[d−m2(σ21∨σ22∨σ23∨σ24)]Im+11+3d+r1+r2+C+σ212+σ232+σ242≤−Mφ+Mδ2(β1+u)+ξ. | (4.5) |
Case 4.3. If (S,E,I1,I2)∈U3, we get
L˜V≤−εEI1+MI1(β1+u)−14[d−m2(σ21∨σ22∨σ23∨σ24)]Im+11+C+3d+r1+r2≤−εδ+D. | (4.6) |
Case 4.4. If (S,E,I1,I2)∈U4, we get
L˜V≤−uI1I2+MI1(β1+u)−14[d−m2(σ21∨σ22∨σ23∨σ24)]Im+11+C+3d+r1+r2≤−uδ+D. | (4.7) |
Case 4.5. If (S,E,I1,I2)∈U5, we get
L˜V≤−14[d−m2(σ21∨σ22∨σ23∨σ24)]Sm+1+MI1(β1+u)−14[d−m2(σ21∨σ22∨σ23∨σ24)]Im+11+C+3d+r1+r2+σ212+σ232+σ242≤−14[d−m2(σ21∨σ22∨σ23∨σ24)]1δm+1+D. | (4.8) |
Case 4.6. If (S,E,I1,I2)∈U6, we get
L˜V≤−14[d−m2(σ21∨σ22∨σ23∨σ24)]Em+1+MI1(β1+u)−14[d−m2(σ21∨σ22∨σ23∨σ24)]Im+11+C+3d+r1+r2+σ212+σ232+σ242≤−14[d−m2(σ21∨σ22∨σ23∨σ24)]1δm+1+D. | (4.9) |
Case 4.7. If (S,E,I1,I2)∈U7, we get
L˜V≤−14[d−m2(σ21∨σ22∨σ23∨σ24)]Im+11+MI1(β1+u)−14[d−m2(σ21∨σ22∨σ23∨σ24)]Im+11+C+3d+r1+r2+σ212+σ232+σ242≤−14[d−m2(σ21∨σ22∨σ23∨σ24)]1δ2(m+1)+D. | (4.10) |
Case 4.8. If (S,E,I1,I2)∈U8, we get
L˜V≤−14[d−m2(σ21∨σ22∨σ23∨σ24)]Im+12+MI1(β1+u)−14[d−m2(σ21∨σ22∨σ23∨σ24)]Im+11+C+3d+r1+r2+σ212+σ232+σ242≤−14[d−m2(σ21∨σ22∨σ23∨σ24)]1δ3(m+1)+D. | (4.11) |
Obviously, from Eq (4.3) to Eq (4.11), we can obtain that L˜V(S,E,I1,I2)≤−1 is true for any (S,E,I1,I2)∈R4+∖U. Therefore, condition (ⅱ) in Lemma 1 is satisfied.
On the other hand, to verify condition (ⅰ) in Lemma 1, the diffusion matrix of Eq (2.3) can be given as follows:
A=(σ21S20000σ22E20000σ23I210000σ24I22). |
Obviously, the matrix A is positive definite for any compact subset of R4+, so condition (ⅰ) in Theorem 2 is satisfied. From Theorem 2, we can know that Eq (2.3) has a unique ergodic stationary distribution π(⋅).
In this section, we present numerical simulations to illustrate the influence of stochastic disturbance on disease persistence and extinction. We used the Euler-Maruyama method [36,37] to solve Eq (2.3) numerically. In order to apply the numerical method to Eq (2.3), we discretized the interval. Then, we computed the discrete Brownian path and used it to generate the desired increment in Eq (2.3). The discretization transformation takes the form as follows:
{Sn+1=Sn+[A−dSn−β1SnI1,n−β2SnI2,n]Δt+σ1Sn√Δtε1,n,En+1=En+[β1SnI1,n+β2SnI2,n−(ε+d)En]Δt+σ2En√Δtε2,n,I1,n+1=I1,n+[εEn−(d+r1)I1,n−uI1,n−h]Δt+σ3I1,n√Δtε3,n,I2,n+1=I2,n+[uI1,n−h−(d+r2)I2,n]Δt+σ4I1,n√Δtε4,n, | (5.1) |
where h is an integer and the time delay can be expressed by the step size as τ=hΔt. εi,n(i=1,2,3,4) denotes independent random variables and subject to Gaussian distribution N(0,1). σi>0(i=1,2,3,4) represents the intensity of white noise.
The parameter values in Table 1 were derived.
A | ε | d | u | r1 | r2 | β1 | β2 |
0.1 | 0.4 | 0.005 | 0.005 | 0.0666 | 0.1428 | 0.7 | 0.6 |
0.0119 | 0.07 | 0.0005 | 0.04 | 0.31 | 0.51 | 0.51 | 0.25 |
We analyzed the ergodic stationary distribution of Eq (2.3) and selected the parameter values in Figure 2 as follows:
A=0.1,ε=0.4,d=0.005,u=0.005,r1=0.0666,r2=0.1428,τ=1.5,β1=0.7,β2=0.6,σi=0.08(i=1,2,3,4). |
By direct calculation we have
R∗=Auε(d+σ212)(ε+d+σ222)(r1+d+σ232)(r2+d+σ242)>1, |
as well as
d−σ21∨σ22∨σ23∨σ242=0.0018>0. |
The conditions in Theorem 2 are satisfied. According to the conclusion of Theorem 2, we can get the trend of the Eq (2.3) with unique ergodic stationary distribution which is shown in Figure 2. From the curves of the S,E,I1,I2, it can be found that susceptible, latent, pre-mutation and post-mutation individuals are asymptotically stable and the disease is persistent. In a biological sense, it means that the disease will not disappear under current conditions. If there is no intervention, the disease will spread in local areas.
We selected the parameters in Figure 3 as follows:
A=0.0119,ε=0.07,d=0.0005,u=0.04,r1=0.31,r2=0.51,τ=1.6,β1=0.51,β2=0.25,σ1=0.08,σ2=0.05,σ3=0.09,σ4=0.08. |
By direct calculation we have
R∗=Auε(d+σ212)(ε+d+σ222)(r1+d+σ232)(r2+d+σ242)<1, |
as well as
d−σ21∨σ22∨σ23∨σ242=−0.0035<0. |
The conditions in Theorem 2 are not satisfied. That is, the condition for ergodic stationary distribution is not satisfied. From Figure 3 we can see that the pre-mutation patient and post-mutation patient gradually tended to zero with the increase of time and that the stochastic model Eq (2.3) is extinct with a probability of 1. That is, the external conditions required for disease occurrence have changed and there is a high possibility that the disease will disappear in local areas.
We applied τ=2.6, τ=3.6 and τ=4.6 while the other parameters in Figure 3 remained unchanged. By direct calculation we have
R∗=Auε(d+σ212)(ε+d+σ222)(r1+d+σ232)(r2+d+σ242)<1, |
as well as
d−σ21∨σ22∨σ23∨σ242=−0.0035<0. |
It can be seen that the conditions for ergodic stationary distribution are not satisfied. When the time delay τ is increased in Figure 4, the curves of I1 and I2 fluctuate and no longer tend to zero whereas the curves of S and E continue to fluctuate. The disease will manifest as periodic outbreaks as shown in Figure 4 and lead to the reinfection of susceptible, latent and infected individuals. Through the analysis of Figure 4, it can be seen that with the increase of time delay, the disease will break out periodically at higher frequencies.
In a biological sense, after a fairly regular interval, the disease will outbreak again locally and have the necessary conditions for exogenous pathogens to cause disease. Therefore, during the spread of an infectious disease, we can realize the periodic law of virus mutation through systematic scientific research and take relevant protective measures. We can develop drugs and improve the effectiveness of vaccines, so that they can adapt to different mutations of the virus antibodies.
As shown in Figure 5, we increased the intensities of the noise and applied σ1=0.09,σ2=0.08,σ3=0.1,σ4=0.09. As shown in Figure 6, we continued to increase the intensities of the noise and applied σ1=0.2,σ2=0.1,σ3=0.2,σ4=0.1. For the results shown in Figures 5 and 6, the other parameters were consistent with those in Figure 4, where the ergodic stationary distribution of Theorem 2 is also not satisfied. We respectively studied the effect of increasing noise on disease extinction when τ=2.6, τ=3.6 and τ=4.6. It can be seen from Figures 5 and 6 that the curves of I1 and I2 gradually approach zero as time goes on, and that the periodic outbreak of disease caused by time delay τ can be reduced by increasing the intensity of the noise. It means that diseases with periodic outbreaks will become extinction again. Through the analysis of Figures 5 and 6, it can be found that noise has a great influence on the persistence and extinction of a virus mutation model with time delay.
On the basis of the above research, we can enhance noise interference in different measures to promote the extinction of the disease, such as the government strengthening the early warning of the disease to avoid wide spread, strengthening personal protection through the media for the public and hospitals strengthening prevention and control measures against large-scale diseases.
In infectious disease models, the combination of white noise and time delay has great influence on the spread and disappearance of an infectious disease, which makes the dynamic behavior of an the system complicated. We considered the effects of stochastic disturbance on the persistence of virus mutations in a model with time delay. First, the existence and uniqueness of the positive solution of the proposed model was proved using stochastic inequalities. Second, by constructing an appropriate stochastic Lyapunov function, if R∗>1 and d−σ21∨σ22∨σ23∨σ242>0 are satisfied, the proposed model has an ergodic stationary distribution which means that the disease is persistent; otherwise, the disease will die out. Finally, numerical simulations were carried out to verify the theoretical results. The results show that stochastic disturbance has great influence on the spread and persistence of infectious diseases with time delay.
Through the above theoretical and numerical analyses, we found that with the increase of the time delay from pre-mutation to post-mutation, the virus will undergo periodic outbreaks and susceptible, latent and infected people will be reinfected. When the noise intensity is large enough under certain constraints, the periodic diseases caused by the time delay can become extinct again. Therefore, the following measures can be taken to prevent and control the spread of mutated infectious diseases. On the one hand, we can reduce the periodic outbreaks of disease by reducing the time delay before and after the virus mutates. It is necessary to realize the periodicity of virus mutations and carry out drug research according to the conditions of periodic outbreaks so as to strengthen the resistance of vaccines to the mutated environment. On the other hand, we can promote disease extinction by increasing stochastic perturbations. The government should strengthen early warnings of the disease, increase personal protection and strengthen prevention and control measures against large-scale diseases.
The authors declare they have not used Artificial Intelligence (AI) tools in the creation of this article.
This work was supported by grants from the Natural Science Foundation (No. 11772002 and No. 12202005), Ningxia Higher Education First-Class Discipline Construction Funding Project (NXYLXK2017B09), Major Special Project of North Minzu University (No. ZDZX201902) and Postgraduate Innovation Project of North Minzu University (YCX23069).
The authors declare that there is no conflict of interest.
[1] |
L. Stone, R. Olinky, A. Huppert, Seasonal dynamics of recurrent epidemics, Nature, 446 (2007), 533–536. https://doi.org/10.1038/nature05638 doi: 10.1038/nature05638
![]() |
[2] |
M. Sinan, K. J. Ansari, A. Kanwal, K. Shah, T. Abdeljawad, B. Abdalla, et al., Analysis of the mathematical model of cutaneous leishmaniasis disease, Alex. Eng. J., 72 (2023), 117–134. https://doi.org/10.1016/j.aej.2023.03.065 doi: 10.1016/j.aej.2023.03.065
![]() |
[3] |
A. R. Sheergojri, P. Iqbal, P. Agarwal, N. Ozdemir, Uncertainty-based Gompertz growth model for tumor population and its numerical analysis, International Journal of Optimization and Control: Theories and Applications, 12 (2022), 137–150. https://doi.org/10.11121/ijocta.2022.1208 doi: 10.11121/ijocta.2022.1208
![]() |
[4] |
Y. Sabbar, Asymptotic extinction and persistence of a perturbed epidemic model with different intervention measures and standard Lˊevy jumps, Bulletin of Biomathematics, 1 (2023), 58–77. https://doi.org/10.59292/bulletinbiomath.2023004 doi: 10.59292/bulletinbiomath.2023004
![]() |
[5] |
F. Evirgen, U. Esmehan, U. Sümeyra, N. Ozdemir, Modelling influenza a disease dynamics under Caputo-Fabrizio fractional derivative with distinct contact rates, Mathematical Modelling and Numerical Simulation with Applications, 3 (2023), 58–73. https://doi.org/10.53391/mmnsa.1274004 doi: 10.53391/mmnsa.1274004
![]() |
[6] |
K. Shah, T. Abdeljawad, H. Alrabaish, On coupled system of drug therapy via piecewise equations, Fractals, 30 (2022), 2240206. https://doi.org/10.1142/S0218348X2240206X doi: 10.1142/S0218348X2240206X
![]() |
[7] |
K. Shah, T. Abdeljawad, F. Jarad, F. Jarad, Q. Al-Mdallal, On nonlinear conformable fractional order dynamical system via differential transform method, CMES Comp. Model. Eng., 163 (2023), 1457–1472. http://doi.org/10.32604/cmes.2023.021523 doi: 10.32604/cmes.2023.021523
![]() |
[8] |
S. W. Ahmad, M. Sarwar, K. Shah, A. Ahmadian, S. Salahshour, Fractional order mathematical modeling of novel corona virus (COVID‐19), Math. Methods Appl. Sci., 46 (2023), 7847–7860. https://doi.org/10.1002/mma.7241 doi: 10.1002/mma.7241
![]() |
[9] |
L. Liu, X. Ren, X. Liu, Dynamical behaviors of an influenza epidemic model with virus mutation, J. Biol. Syst., 26 (2018), 455–472. https://doi.org/10.1142/S0218339018500201 doi: 10.1142/S0218339018500201
![]() |
[10] |
B. Li, A. Deng, K. Li, Y. Hu, Z. C. Li, Y. L. Shi, et al., Viral infection and transmission in a large, well-traced outbreak caused by the SARS-CoV-2 Delta variant, Nat. Commun., 13 (2022), 460. https://doi.org/10.1038/s41467-022-28089-y doi: 10.1038/s41467-022-28089-y
![]() |
[11] |
Y. Liu, A. Feng, S. Zhao, W. Wang, D. He, Large-scale synchronized replacement of Alpha (B.1.1.7) variant by the Delta (B.1.617.2) variant of SARS-COV-2 in the COVID-19 pandemic, Math. Biosci. Eng., 19 (2022), 3591–3596. https://doi.org/10.3934/mbe.2022165 doi: 10.3934/mbe.2022165
![]() |
[12] |
R. M. Chen, Track the dynamical features for mutant variants of COVID-19 in the UK, Math. Biosci. Eng., 18 (2021), 4572–4585. https://doi.org/10.3934/mbe.2021232 doi: 10.3934/mbe.2021232
![]() |
[13] |
Y. Yu, Y. Liu, S. Zhao, D. He, A simple model to estimate the transmissibility of the Beta, Delta, and Omicron variants of SARS-COV-2 in South Africa, Math. Biosci. Eng., 19 (2022), 10361–10373. https://doi.org/10.3934/mbe.2022485 doi: 10.3934/mbe.2022485
![]() |
[14] |
S. P. Otto, T. Day, J. Arino, C. Colijn, J. Dushoff, M. Li, et al., The origins and potential future of SARS-CoV-2 variants of concern in the evolving COVID-19 pandemic, Curr. Biol., 31 (2021), R918–R929. https://doi.org/10.1016/j.cub.2021.06.049 doi: 10.1016/j.cub.2021.06.049
![]() |
[15] |
G. Cacciapaglia, C. Cot, A. D. Hoffer, S. Hohenegger, F. Sannino, S. Vatani, Epidemiological theory of virus variants, Phys. A, 596 (2022), 127071. https://doi.org/10.1016/j.physa.2022.127071 doi: 10.1016/j.physa.2022.127071
![]() |
[16] |
A. P. Dobie, Susceptible-infectious-susceptible (SIS) model with virus mutation in a variable population size, Ecol. Complex., 50 (2022), 101004. https://doi.org/10.1016/j.ecocom.2022.101004 doi: 10.1016/j.ecocom.2022.101004
![]() |
[17] |
U. A. de León, A. G. C. Pérez, E. Avila-Vales, Modeling the SARS-CoV-2 Omicron variant dynamics in the United States with booster dose vaccination and waning immunity, Math. Biosci. Eng., 20 (2023), 10909–10953. https://doi.org/10.3934/mbe.2023484 doi: 10.3934/mbe.2023484
![]() |
[18] |
Y. R. Kim, Y. J. Choi, Y. Min, A model of COVID-19 pandemic with vaccines and mutant viruses, Plos One, 17 (2022), e0275851. https://doi.org/10.1371/journal.pone.0275851 doi: 10.1371/journal.pone.0275851
![]() |
[19] |
G. Liu, J. Chen, Z. Liang, Z. Peng, J. Li, Dynamical analysis and optimal control for a SEIR model based on virus mutation in WSNs, Mathematics, 9 (2021), 929. https://doi.org/10.3390/math9090929 doi: 10.3390/math9090929
![]() |
[20] |
D. Xu, X. Xu, Y. Xie, C. Yang, Optimal control of an SIVRS epidemic spreading model with virus variation based on complex networks, Commun. Nonlinear Sci., 48 (2017), 200–210. https://doi.org/10.1016/j.cnsns.2016.12.025 doi: 10.1016/j.cnsns.2016.12.025
![]() |
[21] |
Q. Liu, D. Jiang, N. Shi, T. Hayat, Dynamics of a stochastic delayed SIR epidemic model with vaccination and double diseases driven by Lˊevy jumps, Phys. A, 492 (2018), 2010–2018. https://doi.org/10.1016/j.physa.2017.11.116 doi: 10.1016/j.physa.2017.11.116
![]() |
[22] |
X. Zhang, M. Liu, Dynamical analysis of a stochastic delayed SIR epidemic model with vertical transmission and vaccination, Adv. Cont. Discr. Mod., 2022 (2022), 35. https://doi.org/10.1186/s13662-022-03707-7 doi: 10.1186/s13662-022-03707-7
![]() |
[23] |
C. Xu, X. Li, The threshold of a stochastic delayed SIRS epidemic model with temporary immunity and vaccination, Chaos Soliton. Fract., 111 (2018), 227–234. https://doi.org/10.1016/j.chaos.2021.110772 doi: 10.1016/j.chaos.2021.110772
![]() |
[24] |
A. E. Koufi, The power of delay on a stochastic epidemic model in a switching environment, Complexity, 2022 (2022), 5121636. https://doi.org/10.1155/2022/5121636 doi: 10.1155/2022/5121636
![]() |
[25] |
B. Boukanjime, M. El-Fatini, A. Laaribi, R. Taki, K. Wang, A Markovian regime-switching stochastic hybrid time-delayed epidemic model with vaccination, Automatica, 133 (2021), 109881. https://doi.org/10.1016/j.automatica.2021.109881 doi: 10.1016/j.automatica.2021.109881
![]() |
[26] |
H. J. Alsakaji, F. A. Rihan, S. Kundu, O. Mohamed, Dynamics of a time-delay differential model for tumour-immune interactions with random noise, Alex. Eng. J., 61 (2022), 11913–11923. https://doi.org/10.1016/j.aej.2022.05.027 doi: 10.1016/j.aej.2022.05.027
![]() |
[27] |
I. Ali, S. U. Khan, Analysis of stochastic delayed SIRS model with exponential birth and saturated incidence rate, Chaos Soliton. Fract., 138 (2022), 110008. https://doi.org/10.1016/j.chaos.2020.110008 doi: 10.1016/j.chaos.2020.110008
![]() |
[28] |
H. J. Alsakaji, F. A. Rihan, A. Hashish, Dynamics of a stochastic epidemic model with vaccination and multiple time-delays for COVID-19 in the UAE, Complexity, 2022 (2022), 4247800. https://doi.org/10.1155/2022/4247800 doi: 10.1155/2022/4247800
![]() |
[29] |
A. Khan, R. Ikram, A. Din, U. W. Humphries, A. Akgul, Stochastic COVID-19 SEIQ epidemic model with time-delay, Results Phys., 30 (2021), 104775. https://doi.org/10.1016/j.rinp.2021.104775 doi: 10.1016/j.rinp.2021.104775
![]() |
[30] |
F. A. Rihan, H. J. Alsakaji, Analysis of a stochastic HBV infection model with delayed immune response, Math. Biosci. Eng., 18 (2021), 5194–5220. https://doi.org/10.3934/mbe.2021264 doi: 10.3934/mbe.2021264
![]() |
[31] |
R. Ikram, A. Khan, M. Zahri, A. Saeed, M. Yavuz, P. Kumam, Extinction and stationary distribution of a stochastic COVID-19 epidemic model with time-delay, Comput. Biol. Med., 141 (2022), 105115. https://doi.org/10.1016/j.compbiomed.2021.105115 doi: 10.1016/j.compbiomed.2021.105115
![]() |
[32] |
Q. Liu, D. Jiang, T. Hayat, A. Alsaedi, Dynamics of a stochastic SIR epidemic model with distributed delay and degenerate diffusion, J. Franklin I., 356 (2019), 7347–7370. https://doi.org/10.1016/j.jfranklin.2019.06.030 doi: 10.1016/j.jfranklin.2019.06.030
![]() |
[33] |
J. Sun, M. Gao, D. Jiang, Threshold dynamics of a non-linear stochastic viral model with time delay and CTL responsiveness, Life, 11 (2021), 766. https://doi.org/10.3390/life11080766 doi: 10.3390/life11080766
![]() |
[34] |
F. A. Rihan, H. J. Alsakaji, Dynamics of a stochastic delay differential model for COVID-19 infection with asymptomatic infected and interacting people: case study in the UAE, Results Phys., 28 (2021), 104658. https://doi.org/10.1016/j.rinp.2021.104658 doi: 10.1016/j.rinp.2021.104658
![]() |
[35] | R. Khasminskii, Stochastic stability of differential equations, Berlin: Springer, 2011. https://doi.org/10.1007/978-3-642-23280-0 |
[36] | E. Buckwar, Euler-Maruyama and Milstein approximations for stochastic functional differential equations with distributed memory term, Berlin: Humboldt-Universität, 2005. http://doi.org/10.18452/3583 |
[37] |
D. J. Higham, An algorithmic introduction to numerical simulation of stochastic differential equations, SIAM Rev., 43 (2001), 525–546. https://doi.org/10.1137/S0036144500378302 doi: 10.1137/S0036144500378302
![]() |
[38] |
J. Gao, T. Zhang, Analysis on an SEIR epidemic model with logistic death rate of virus mutation, Journal of Mathematical Research with Applications, 39 (2019), 259–268. https://doi.org/10.3770/j.issn:2095-2651.2019.03.005 doi: 10.3770/j.issn:2095-2651.2019.03.005
![]() |
A | ε | d | u | r1 | r2 | β1 | β2 |
0.1 | 0.4 | 0.005 | 0.005 | 0.0666 | 0.1428 | 0.7 | 0.6 |
0.0119 | 0.07 | 0.0005 | 0.04 | 0.31 | 0.51 | 0.51 | 0.25 |