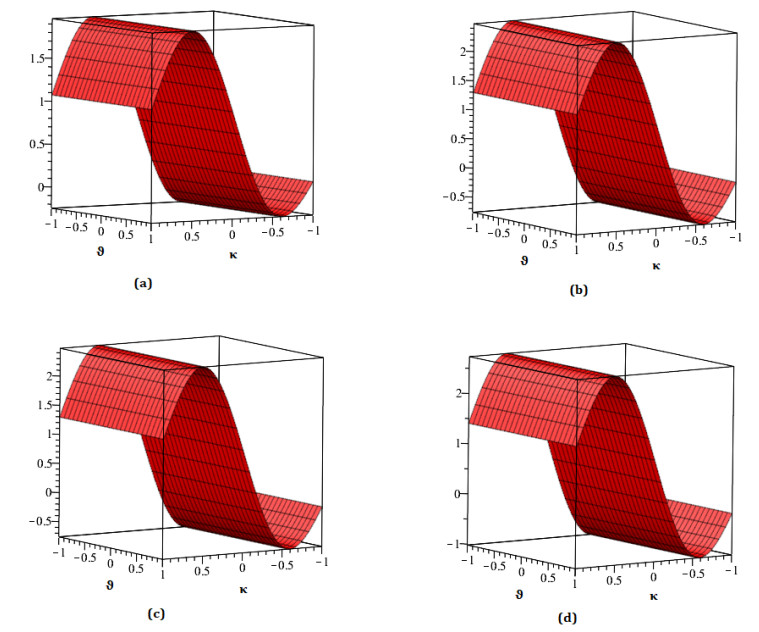
This article defines the concepts of picture fuzzy filter, picture fuzzy grill, picture fuzzy section, picture fuzzy base, picture fuzzy subbase, picture fuzzy ultrafilter, as well as their fundamental features. Characteristics of the aforementioned concepts are addressed, and equivalence between the picture fuzzy filter and picture fuzzy grills is established. Real-world examples are offered to demonstrate the advantages of picture fuzzy filters in the classification of sets using a clustering technique. Illustration is provided to show the advantages of picture fuzzy sets and the results are compared with intuitionistic fuzzy sets. Clustering technique is applied to the picture fuzzy filter collection reduces the computational process which helps the decision makers to classify the sets with fewer iterations.
Citation: K. Tamilselvan, V. Visalakshi, Prasanalakshmi Balaji. Applications of picture fuzzy filters: performance evaluation of an employee using clustering algorithm[J]. AIMS Mathematics, 2023, 8(9): 21069-21088. doi: 10.3934/math.20231073
[1] | Xiujiao Chi, Pengtong Li . Characterization of frame vectors for A-group-like unitary systems on Hilbert C∗-modules. AIMS Mathematics, 2023, 8(9): 22112-22126. doi: 10.3934/math.20231127 |
[2] | Adel Alahmadi, Tamador Alihia, Patrick Solé . The build up construction for codes over a non-commutative non-unitary ring of order 9. AIMS Mathematics, 2024, 9(7): 18278-18307. doi: 10.3934/math.2024892 |
[3] | Yan Ling Fu, Wei Zhang . Some results on frames by pre-frame operators in Q-Hilbert spaces. AIMS Mathematics, 2023, 8(12): 28878-28896. doi: 10.3934/math.20231480 |
[4] | Yunpeng Xue . On enhanced general linear groups: nilpotent orbits and support variety for Weyl module. AIMS Mathematics, 2023, 8(7): 14997-15007. doi: 10.3934/math.2023765 |
[5] | Bhabesh Das, Helen K. Saikia . On the Sum of Unitary Divisors Maximum Function. AIMS Mathematics, 2017, 2(1): 96-101. doi: 10.3934/Math.2017.1.96 |
[6] | Xiaogang Liu, Muhammad Ahsan, Zohaib Zahid, Shuili Ren . Fault-tolerant edge metric dimension of certain families of graphs. AIMS Mathematics, 2021, 6(2): 1140-1152. doi: 10.3934/math.2021069 |
[7] | Lyimo Sygbert Mhagama, Muhammad Faisal Nadeem, Mohamad Nazri Husin . On the edge metric dimension of some classes of cacti. AIMS Mathematics, 2024, 9(6): 16422-16435. doi: 10.3934/math.2024795 |
[8] | Ali N. A. Koam, Adnan Khalil, Ali Ahmad, Muhammad Azeem . Cardinality bounds on subsets in the partition resolving set for complex convex polytope-like graph. AIMS Mathematics, 2024, 9(4): 10078-10094. doi: 10.3934/math.2024493 |
[9] | Hui Chen . Characterizations of normal cancellative monoids. AIMS Mathematics, 2024, 9(1): 302-318. doi: 10.3934/math.2024018 |
[10] | Chenggang Huo, Humera Bashir, Zohaib Zahid, Yu Ming Chu . On the 2-metric resolvability of graphs. AIMS Mathematics, 2020, 5(6): 6609-6619. doi: 10.3934/math.2020425 |
This article defines the concepts of picture fuzzy filter, picture fuzzy grill, picture fuzzy section, picture fuzzy base, picture fuzzy subbase, picture fuzzy ultrafilter, as well as their fundamental features. Characteristics of the aforementioned concepts are addressed, and equivalence between the picture fuzzy filter and picture fuzzy grills is established. Real-world examples are offered to demonstrate the advantages of picture fuzzy filters in the classification of sets using a clustering technique. Illustration is provided to show the advantages of picture fuzzy sets and the results are compared with intuitionistic fuzzy sets. Clustering technique is applied to the picture fuzzy filter collection reduces the computational process which helps the decision makers to classify the sets with fewer iterations.
Fractional differential equations have gotten attention and prominence due to their numerous applications in engineering and science. These equations, for instance, are increasingly being utilized to explain phenomena in many different physical processes, including fluid mechanics, biology, electromagnetic, signal processing, acoustics and many more. In these and other applications, the non-locality of fractional differential equations is their main benefit. The integer order differential operator is typically considered a local operator, whereas the fractional order differential operator is non-local. This shows that the next state of a system depends on both its present state and all of its previous states. This is more practical, which is one of the reasons fractional calculus is growing in popularity [1,2,3,4,5,6,7,8,9].
Finding solutions to fractional differential equations has therefore received a lot of interest. A fractional differential equation is typically challenging to solve exactly. Researchers were interested in numerical approaches, the perturbation method among them. However, there are significant drawbacks to perturbation methods. For instance, the approximate solution necessitates a number of small parameters, which is problematic because most nonlinear problems do not have any small parameters. Even if an adequate selection of minor parameters occasionally results in the optimal solution, inappropriate selections frequently have negative consequences for the solutions. J. H. He, a Chinese researcher, invented the homotopy perturbation method (HPM) and presented it in 1998 [10,11].
The Adomian decomposition method [12,13] is a powerful approach for solving linear and nonlinear, homogeneous and nonhomogeneous partial and differential equations, and integro-differential equations of integer and non-integer order, which gives us convergent series from exact solutions. Several mathematicians used the homotopy perturbation method for handling nonlinear equations arising in engineering and science [14,15,16,17]. Many authors have focused their attention recently on examining the solutions to both linear and nonlinear partial differential equations using a variety of techniques such as Elzaki transform decomposition method [18,19], homotopy perturbation transform technique [20,21], homotopy analysis transform method [22,23], q-homotopy analysis transform method [24], iterative Laplace transform method [25], variational iteration method [26,27] and many others among them.
The mathematical theory of shock waves describes the first-order quasi-linear hyperbolic system of partial differential equations (PDEs) surface of discontinuity's characteristics. In the latter half of the 19th century, concerns with the compressible fluids and motion of gases gave rise to the mathematical theory of shock waves. The research of Earnshaw [28], Riemann [29], Rankine [30] and Hugoniot [31] laid the groundwork for it. One imagines a medium devoid of dissipation, viscosity and thermal conductivity as an idealization of actual gases and fluids. There may be discontinuities in the distribution of all flow parameters during motion in such idealized media (the temperature, the pressure, the velocity, the density, etc.). The collection of flow parameter discontinuity spots may be highly intricate when this set has piecewise-smooth surfaces of discontinuity made up of discontinuity points of the first kind; only the most elementary basic cases have been carefully taken into account. In the general scenario, discontinuous two-dimensional surfaces move in the three-dimensional space R3 as time passes. Conservation laws are among the characteristics shared by all continuum physics theories. By connecting the values of the primary vector field J to the flux f, constitutive relations that characterize the specific medium in question are added to the laws. By connecting the principal vector field J values to the flux f, constitutive relations that characterize the specific medium in question are added to the laws.
Since we are assuming that these relations are represented by smooth forms in this scenario, the conservation laws lead to nonlinear hyperbolic PDEs, which are expressed in their most basic form as
Jϑ(κ,ϑ)+f(J(κ,ϑ))κ=0,κ∈R,ϑ>0, | (1.1) |
subjected to the initial condition
J(κ,0)=J0(κ),κ∈R. |
A model for a variety of physical phenomena, including shock waves and three-phase flow in porous media, uses (1.1). Traffic flow, explosions, aeroplanes breaking the sound barrier, glacier waves, and other phenomena all use shock waves. Nonlinear hyperbolic PDEs are used to formulate them [32,33,34]. The Adomian decomposition method (ADM) [35,36,37] and the homotopy perturbation method (HPM) [38,39,40] have both been used to study the shock wave and wave equations. Since solutions are simple to find, the classical shock wave and wave equations provide excellent predictive equations. We introduce a fractional-order generalization of shock wave (1.1) in this article, which has the following form:
DρϑJ(κ,ϑ)+f(J(κ,ϑ))κ=0,κ∈R,ϑ>0, | (1.2) |
where a parameter named ρ designates the order of the time-derivative. In the Caputo sense, the derivative is regarded. A parameter specifying the order of the fractional-order shock wave equation is included in the general response expression and can be changed to generate different responses. The fractional-order shock wave equation is reduced to the standard shock wave equation when ρ=1.
This study solves the fractional-order shock wave and wave equations analytically and numerically using the new homotopy perturbation transform technique and the Yang transform decomposition method. This method's ability to combine two effective approaches for generating precise and approximative analytical solutions for nonlinear equations is an advantage. It is important to note that the suggested methodology can perform better overall since it can reduce the amount of computational work required compared to the other techniques while still keeping the high accuracy of the numerical result.
The rest of the paper is organized as follows: In Section 2, we introduce some fundamental preliminaries. In Section 3, we presented the general methodology of HPYTM to obtain the solution of general arbitrary order PDE, while in Section 4, we presented the general method of YDTM to get the solution of general arbitrary order PDE. In Section 5, the suggested techniques are implemented for solving nonlinear fractional-order shock wave equations, and in Section 6, results and discussion are given. Similarly, in the end, a brief conclusion is given in Section 7.
In this section, we discuss a number of important concepts, terminology and ideas relevant to the fractional derivative operators used in the current study.
Definition 2.1. The fractional Caputo derivative is stated as
DρJJ(κ,ϑ)=1Γ(k−ρ)∫ϑ0(ϑ−ρ)k−ρ−1J(k)(κ,ρ)dρ,k−1<ρ≤k,k∈N. | (2.1) |
Definition 2.2. The Yang transform (YT) of a function J(ϑ) is stated as
Y(J(ϑ))=M(u)=∫∞0e−ϑuJ(ϑ)dϑ,ϑ>0,u∈(−ϑ1,ϑ2), | (2.2) |
having Yang inverse transform as
Y−1{M(u)}=J(ϑ). | (2.3) |
Definition 2.3. The Yang transform of nth derivatives is stated as
Y(Jn(ϑ))=M(u)un−n−1∑k=0Jk(0)un−k−1, | (2.4) |
for all n=1,2,3,….
Definition 2.4. The Yang transform of fractional-order derivative is stated as
Y(Jρ(ϑ))=M(u)uρ−n−1∑k=0Jk(0)uρ−(k+1),0<ρ≤n. | (2.5) |
This section is concerned with fundamental solution process of HPYTM to get the solution of general arbitrary order partial differential equation
DρϑJ(κ,ϑ)=P1[κ]J(κ,ϑ)+Q1[κ]J(κ,ϑ),0<ρ≤2, | (3.1) |
subjected to the initial conditions
J(κ,0)=ξ(κ),∂∂ϑJ(κ,0)=ζ(κ), |
where Dρϑ=∂ρ∂ϑρ is the fractional Caputo derivative, P1[κ] and Q1[κ] are linear and nonlinear operators.
By employing YT, we have
Y[DρϑJ(κ,ϑ)]=Y[P1[κ]J(κ,ϑ)+Q1[κ]J(κ,ϑ)], | (3.2) |
1uρ{M(u)−uJ(0)−u2J′(0)}=Y[P1[κ]J(κ,ϑ)+Q1[κ]J(κ,ϑ)]. | (3.3) |
On simplification, we get
M(J)=uJ(0)+u2J′(0)+uρY[P1[κ]J(κ,ϑ)+Q1[κ]J(κ,ϑ)]. | (3.4) |
Operating inverse YT both sides, we have
J(κ,ϑ)=J(0)+J′(0)+Y−1[uρY[P1[κ]J(κ,ϑ)+Q1[κ]J(κ,ϑ)]]. | (3.5) |
Now, by using the HPM
J(κ,ϑ)=∞∑k=0ϵkJk(κ,ϑ), | (3.6) |
with perturbation parameter ϵ∈[0,1].
The nonlinear terms are decomposed as
Q1[κ]J(κ,ϑ)=∞∑k=0ϵkHn(J), | (3.7) |
and He's polynomials Hk(J) are given as
Hn(J0,J1,…,Jn)=1Γ(n+1)Dkϵ[Q1(∞∑k=0ϵiJi)]ϵ=0, | (3.8) |
where Dkϵ=∂k∂ϵk.
Using (3.7) and (3.8) in (3.5), we have
∞∑k=0ϵkJk(κ,ϑ)=J(0)+J′(0)+ϵ(Y−1{uρY(P1∞∑k=0ϵkJk(κ,ϑ)+∞∑k=0ϵkHk(J))}). | (3.9) |
Thus, by equating the coefficient of ϵ, we get
ϵ0:J0(κ,ϑ)=J(0)+J′(0),ϵ1:J1(κ,ϑ)=Y−1[uρY(P1[κ]J0(κ,ϑ)+H0(J))],ϵ2:J2(κ,ϑ)=Y−1[uρY(P1[κ]J1(κ,ϑ)+H1(J))],⋮ϵk:Jk(κ,ϑ)=Y−1[uρY(P1[κ]Jk−1(κ,ϑ)+Hk−1(J))], | (3.10) |
for k>0, k∈N.
Finally, we use truncated series to approximate the analytical solution Jk(κ,ϑ),
J(κ,ϑ)=limM→∞M∑k=1Jk(κ,ϑ). | (3.11) |
The solutions to the aforementioned series usually converge quickly.
This section is concerned with the fundamental solution process of YTDM to get the solution of general arbitrary order partial differential equation
DρϑJ(κ,ϑ)=P1(κ,ϑ)+Q1(κ,ϑ),0<ρ≤1, | (4.1) |
subjected to the initial conditions
J(κ,0)=ξ(κ),∂∂ϑJ(κ,0)=ζ(κ), |
where Dρϑ=∂ρ∂ϑρ is the fractional Caputo derivative, P1 and Q1 are linear and non-linear operators, respectively.
By employing YT, we have
Y[DρϑJ(κ,ϑ)]=Y[P1(κ,ϑ)+Q1(κ,ϑ)], | (4.2) |
using the differentiation property of the YT, we have
1uρ{M(u)−uJ(0)−u2J′(0)}=Y[P1(κ,ϑ)+Q1(κ,ϑ)]. | (4.3) |
On simplification, we get
M(J)=uJ(0)+u2J′(0)+uρY[P1(κ,ϑ)+Q1(κ,ϑ)]. | (4.4) |
Operating inverse YT both sides, we have
J(κ,ϑ)=J(0)+J′(0)+Y−1[uρY[P1(κ,ϑ)+Q1(κ,ϑ)]. | (4.5) |
Thus, by means of YTDM the infinite series solution of J(κ,ϑ),
J(κ,ϑ)=∞∑m=0Jm(κ,ϑ). | (4.6) |
The decomposition of of nonlinear terms Q1 in terms of the Adomian polynomials are given as
Q1(κ,ϑ)=∞∑m=0Am, | (4.7) |
where
Am=1m![∂m∂ℓm{Q1(∞∑k=0ℓkκk,∞∑k=0ℓkϑk)}]ℓ=0. | (4.8) |
By putting (4.6) and (4.8) into (4.5), we obtain
∞∑m=0Jm(κ,ϑ)=J(0)+J′(0)+Y−1uρ[Y(P1(∞∑m=0κm,∞∑m=0ϑm)+∞∑m=0Am)]. | (4.9) |
We illustrate the below terms:
J0(κ,ϑ)=J(0)+ϑJ′(0), | (4.10) |
J1(κ,ϑ)=Y−1[uρY+{P1(κ0,ϑ0)+A0}]. | (4.11) |
Hence, in general for m≥1, we have
Jm+1(κ,ϑ)=Y−1[uρY+{P1(κm,ϑm)+Am}]. |
This section is concerned with the implementation of YTDM and HPYTM to solve fractional-order nonlinear shock wave equations.
Example 5.1. Consider the nonlinear fractional-order shock wave equation
∂ρJ∂ϑρ+(1c0−Υ+12Jc20)∂J∂κ=0,0<ρ≤1, | (5.1) |
with the initial condition
J(κ,0)=exp(−κ22), |
where c0 and Υ are constants with Υ representing the specific heat. In the present study, we choose c0=2 and Υ=1.5, which represent the airflow.
By employing YT, we have
Y(∂ρJ∂ϑρ)=−Y[(1c0−Υ+12Jc20)∂J∂κ]. | (5.2) |
Now, by YT differentiation property, we get
1uρ{M(u)−uJ(0)}=−Y[(1c0−Υ+12Jc20)∂J∂κ] | (5.3) |
and
M(u)=uJ(0)−uρY[(1c0−Υ+12Jc20)∂J∂κ]. | (5.4) |
Operating inverse YT both sides, we have
J(κ,ϑ)=J(0)−Y−1{uρ[Y((1c0−Υ+12Jc20)∂J∂κ)]},J(κ,ϑ)=exp(−κ22)−Y−1{uρ[Y((1c0−Υ+12Jc20)∂J∂κ)]}. | (5.5) |
Thus, by using the HPM, we obtain
∞∑k=0ϵkJk(κ,ϑ)=γ+ϵ(Y−1{uρY[1c0∂J∂κ(∞∑k=0ϵkJk(κ,ϑ))−Υ+121c20(∞∑k=0ϵkHk(J))]}), | (5.6) |
where non-linear terms are represented by He's polynomial Hk(J) and is stated as
∞∑k=0ϵkHk(J)=J∂J∂κ. | (5.7) |
Some components of He's polynomials are calculated as
H0(J)=J0∂J0∂κ,H1(J)=J0∂J1∂κ+J1∂J0∂κ. |
By comparing the coefficient of ϵ, we obtain
ϵ0:J0(κ,ϑ)=exp(−κ22),ϵ1:J1(κ,ϑ)=Y−1{uρY(1c0∂J0∂κ−Υ+121c20H0(J))}=−[1c0−Υ+12c20exp(−κ22)]κexp(−κ22)ϑρΓ(ρ+1),ϵ2:J2(κ,ϑ)=Y−1{uρY[(1c0∂J1∂κ−Υ+121c20H1(J))}=exp(−κ22)[−1c20+κ2c20−Υ+1c30exp(−κ22)−2(Υ+1)c30κ2exp(−κ22)−(Υ+1)24c40exp(−κ2)+3(Υ+1)24c40κ2exp(−κ2)]ϑ2ρΓ(2ρ+1),⋮ |
The remaining elements of the HPYTM solution can be achieved by continuing in the same way. Thus,
J(κ,ϑ)=exp(−κ22)−[1c0−Υ+12c20exp(−κ22)]κexp(−κ22)ϑρΓ(ρ+1)+exp(−κ22)×[−1c20+κ2c20−Υ+1c30exp(−κ22)−2(Υ+1)c30κ2exp(−κ22)−(Υ+1)24c40exp(−κ2)+3(Υ+1)24c40κ2exp(−κ2)]ϑ2ρΓ(2ρ+1)+⋯. |
By employing YT, we have
Y(∂ρJ∂ϑρ)=−Y((1c0−Υ+12Jc20)∂J∂κ). | (5.8) |
Now, by YT differentiation property, we get
1uρ{M(u)−uJ(0)}=−Y((1c0−Υ+12Jc20)∂J∂κ), | (5.9) |
M(u)=uJ(0)+uρY((1c0−Υ+12Jc20)∂J∂κ). | (5.10) |
Operating inverse YT both sides, we have
J(κ,ϑ)=J(0)−Y−1{uρ[Y((1c0−Υ+12Jc20)∂J∂κ)]},J(κ,ϑ)=exp(−κ22)−Y−1{uρ[Y((1c0−Υ+12Jc20)∂J∂κ)]}. | (5.11) |
Thus, by means of YTDM the infinite series solution of J(κ,ϑ),
J(κ,ϑ)=∞∑m=0Jm(κ,ϑ), | (5.12) |
and by means of Adomian polynomial, the nonlinear terms are derived as J∂J∂κ=∑∞m=0Am, we have
∞∑m=0Jm(κ,ϑ)=J(κ,0)−Y−1{uρ[Y((1c0∂J∂κ−Υ+12c20∞∑m=0Am))]},∞∑m=0Jm(κ,ϑ)=exp(−κ22)−Y−1{uρ[Y((1c0∂J∂κ−Υ+12c20∞∑m=0Am))]}. | (5.13) |
In this way, the nonlinear terms are decomposed as
A0=J0∂J0∂κ,A1=J0∂J1∂κ+J1∂J0∂κ. |
On comparing both sides, we get
J0(κ,ϑ)=exp(−κ22). |
On m=0, we have
J1(κ,ϑ)=−[1c0−Υ+12c20exp(−κ22)]κexp(−κ22)ϑρΓ(ρ+1). |
On m=1, we have
J2(κ,ϑ)=exp(−κ22)[−1c20+κ2c20−Υ+1c30exp(−κ22)−2(Υ+1)c30κ2exp(−κ22)−(Υ+1)24c40exp(−κ2)+3(Υ+1)24c40κ2exp(−κ2)]ϑ2ρΓ(2ρ+1). |
Thus, the remaining elements ρm of YTDM for (m≥2) are easy to get. Hence, we write the series form solution as
J(κ,ϑ)=∞∑m=0Jm(κ,ϑ)=J0(κ,ϑ)+J1(κ,ϑ)+J2(κ,ϑ)+⋯,J(κ,ϑ)=exp(−κ22)−[1c0−Υ+12c20exp(−κ22)]κexp(−κ22)ϑρΓ(ρ+1)+exp(−κ22)[−1c20+κ2c20−Υ+1c30exp(−κ22)−2(Υ+1)c30κ2exp(−κ22)−(Υ+1)24c40exp(−κ2)+3(Υ+1)24c40κ2exp(−κ2)]ϑ2ρΓ(2ρ+1)+⋯. |
Example 5.2. Consider the nonlinear fractional-order shock wave equation
∂ρJ∂ϑρ+J∂J∂κ−∂∂ϑ∂J2∂κ2=0,0<ρ≤1, | (5.14) |
with initial source
J(κ,0)=3 sech2(κ−152). |
By employing YT, we have
Y(∂ρJ∂ϑρ)=Y(∂∂ϑ∂J2∂κ2−J∂J∂κ). | (5.15) |
Now, by YT differentiation property, we get
1uρ{M(u)−uJ(0)}=Y(∂∂ϑ∂J2∂κ2−J∂J∂κ) | (5.16) |
and
M(u)=uJ(0)+uρY(∂∂ϑ∂J2∂κ2−J∂J∂κ). | (5.17) |
Operating inverse YT both sides, we have
J(κ,ϑ)=J(0)+Y−1{uρ[Y(∂∂ϑ∂J2∂κ2−J∂J∂κ)]},J(κ,ϑ)=3 sech2(κ−152)+Y−1{uρ[Y(∂∂ϑ∂J2∂κ2−J∂J∂κ)]}. | (5.18) |
Now, by using the HPM, we obtain
∞∑k=0ϵkJk(κ,ϑ)=3 sech2(κ−152)+ϵ(Y−1{uρY[(∞∑k=0ϵkJk(κ,ϑ))κκϑ−(∞∑k=0ϵkHk(J))]}), | (5.19) |
where non-linear terms are presented by He's polynomial Hk(J) and is stated as
∞∑k=0ϵkHk(J)=J∂J∂κ. | (5.20) |
Some terms of He's polynomials are calculated as
H0(J)=J0∂J0∂κ,H1(J)=J0∂J1∂κ+J1∂J0∂κ. |
By comparing the coefficient of ϵ, we obtain
ϵ0:J0(κ,ϑ)=3 sech2(κ−152),ϵ1:J1(κ,ϑ)=Y−1{uρY(∂∂ϑ∂J20∂κ2−H0(J))}=9 sech4(κ−152)tanh(κ−152)ϑρΓ(ρ+1),ϵ2:J2(κ,ϑ)=Y−1{uρY(∂∂ϑ∂J21∂κ2−H1(J))}=[1892 sech6(κ−152)tanh2(κ−152)−272 sech6(κ−152)]ϑ2ρΓ(2ρ+1)+[1352 sech4(κ−152)tanh3(κ−152)−632 sech4(κ−152tanh(κ−152))]Γ(ρ+1)ϑ2ρ−1Γ(2ρ),⋮ |
The remaining elements of the HPYTM solution can be achieved by continuing in the same way. Thus,
J(κ,ϑ)=3 sech2(κ−152)+9 sech4(κ−152)tanh(κ−152)ϑρΓ(ρ+1)+[1892 sech6(κ−152)tanh2(κ−152)−272 sech6(κ−152)]ϑ2ρΓ(2ρ+1)+[1352 sech4(κ−152)tanh3(κ−152)−632 sech4(κ−152tanh(κ−152))]Γ(ρ+1)ϑ2ρ−1Γ(2ρ)+⋯. |
By employing YT, we have
Y(∂ρJ∂ϑρ)=Y(∂∂ϑ∂J2∂κ2−J∂J∂κ). | (5.21) |
Now, by YT differentiation property, we have
1uρ{M(u)−uJ(0)}=Y(∂∂ϑ∂J2∂κ2−J∂J∂κ) | (5.22) |
and
M(u)=uJ(0)+uρY(∂∂ϑ∂J2∂κ2−J∂J∂κ). | (5.23) |
Operating inverse YT both sides, we have
J(κ,ϑ)=J(0)+Y−1{uρ[Y(∂∂ϑ∂J2∂κ2−J∂J∂κ)]},J(κ,ϑ)=1(1+exp(κ))2+Y−1{uρ[Y(∂∂ϑ∂J2∂κ2−J∂J∂κ)]}. | (5.24) |
Thus, by means of YTDM the infinite series solution of J(κ,ϑ),
J(κ,ϑ)=∞∑m=0Jm(κ,ϑ), | (5.25) |
and by means of Adomian polynomial, the nonlinear terms are derived as J∂J∂κ=∑∞m=0Am, we have
∞∑m=0Jm(κ,ϑ)=J(κ,0)+Y−1{uρ[Y(∂∂ϑ∂J2∂κ2−∞∑m=0Am)]},∞∑m=0Jm(κ,ϑ)=1(1+exp(κ))2+Y−1{uρ[Y(∂∂ϑ∂J2∂κ2−∞∑m=0Am)]}. | (5.26) |
In this way, the nonlinear terms are decomposed as
A0=J0∂J0∂κ,A1=J0∂J1∂κ+J1∂J0∂κ. |
On comparing both sides, we get
J0(κ,ϑ)=3 sech2(κ−152). |
On m=0, we have
J1(κ,ϑ)=9 sech4(κ−152)tanh(κ−152)ϑρΓ(ρ+1). |
On m=1, we have
J2(κ,ϑ)=[1892 sech6(κ−152)tanh2(κ−152)−272 sech6(κ−152)]ϑ2ρΓ(2ρ+1)+[1352 sech4(κ−152)tanh3(κ−152)−632 sech4(κ−152tanh(κ−152))]Γ(ρ+1)ϑ2ρ−1Γ(2ρ). |
Thus, the remaining elements ρm of YTDM for (m≥3) are easy to get. Hence, we write the series form solution as
J(κ,ϑ)=∞∑m=0Jm(κ,ϑ)=J0(κ,ϑ)+J1(κ,ϑ)+⋯,J(κ,ϑ)=3 sech2(κ−152)+9 sech4(κ−152)tanh(κ−152)ϑρΓ(ρ+1)+[1892 sech6(κ−152)tanh2(κ−152)−272 sech6(κ−152)]ϑ2ρΓ(2ρ+1)+[1352 sech4(κ−152)tanh3(κ−152)−632 sech4(κ−152tanh(κ−152))]Γ(ρ+1)ϑ2ρ−1Γ(2ρ)+⋯. |
At ρ=1, we get in closed form the exact solution as
J(κ,ϑ)=3 sech2(κ−15−ϑ2). | (5.27) |
In this section, we present the physical explanations for the presented problems. We note that our approaches can handle time fractional-order shock wave equations perfectly. It can be shown that J(κ,ϑ) reduces with the growth of κ and ϑ for ρ=0.25,0.50,0.75 and 1 in Figure 1, which shows the surface solutions of J(κ,ϑ) for various time fractional equations in Brownian. The analytical solution derived using the suggested approaches is shown in Figure 2, together with the exact solution for various values of κ and ϑ. Figure 2 also shows the surface solutions of J(κ,ϑ). Figure 3 shows the absolute error behavior of Example 5.2. Similarly, in Table 1, we show the behavior of the accurate and proposed method solutions at various fractional orders of ρ having different values of κ and ϑ. The figures and table show that the solution of the proposed method is in good contact with the exact solution.
ϑ | κ | ρ=0.6 | ρ=0.8 | ρ=1(approx) | ρ=1(approx) | ρ=1(exact) |
0.2 | 0.000004490 | 0.000004487 | 0.000004483 | 0.000004483 | 0.000004479 | |
0.4 | 0.000005481 | 0.000005479 | 0.000005476 | 0.000005476 | 0.000005470 | |
0.01 | 0.6 | 0.000006693 | 0.000006691 | 0.000006688 | 0.000006688 | 0.000006681 |
0.8 | 0.000008171 | 0.000008171 | 0.000008169 | 0.000008169 | 0.000008161 | |
1 | 0.000009982 | 0.000009980 | 0.000009978 | 0.000009978 | 0.000009968 | |
0.2 | 0.000004490 | 0.000004487 | 0.000004483 | 0.000004483 | 0.000004474 | |
0.4 | 0.000005482 | 0.000005478 | 0.000005476 | 0.000005476 | 0.000005465 | |
0.02 | 0.6 | 0.000006693 | 0.000006690 | 0.000006688 | 0.000006688 | 0.000006675 |
0.8 | 0.000008177 | 0.000008172 | 0.000008169 | 0.000008169 | 0.000008153 | |
1 | 0.000009987 | 0.000009982 | 0.000009978 | 0.000009978 | 0.000009958 | |
0.2 | 0.000004489 | 0.000004485 | 0.000004483 | 0.000004483 | 0.000004470 | |
0.4 | 0.000005489 | 0.00000583 | 0.000005476 | 0.000005476 | 0.000005459 | |
0.03 | 0.6 | 0.000006693 | 0.000006690 | 0.000006688 | 0.000006688 | 0.000006668 |
0.8 | 0.000008179 | 0.000008174 | 0.000008169 | 0.000008169 | 0.000008145 | |
1 | 0.000009989 | 0.000009982 | 0.000009978 | 0.000009978 | 0.000009948 | |
0.2 | 0.000004492 | 0.000004487 | 0.000004483 | 0.000004483 | 0.000004465 | |
0.4 | 0.000005487 | 0.000005481 | 0.000005476 | 0.000005476 | 0.000005454 | |
0.04 | 0.6 | 0.000006699 | 0.000006691 | 0.000006688 | 0.000006688 | 0.000006661 |
0.8 | 0.000008183 | 0.000008177 | 0.000008169 | 0.000008169 | 0.000008136 | |
1 | 0.000009999 | 0.000009983 | 0.000009978 | 0.000009978 | 0.000009938 | |
0.2 | 0.000004497 | 0.000004489 | 0.000004483 | 0.000004483 | 0.000004461 | |
0.4 | 0.000005490 | 0.000005479 | 0.000005476 | 0.000005476 | 0.000005448 | |
0.05 | 0.6 | 0.000006697 | 0.000006691 | 0.000006688 | 0.000006688 | 0.000006655 |
0.8 | 0.000008191 | 0.000008185 | 0.000008169 | 0.000008169 | 0.000008128 | |
1 | 0.000009997 | 0.000009988 | 0.000009978 | 0.000009978 | 0.000009928 |
The approximate and analytical solutions of the fractional-order nonlinear shock wave and wave equations are successfully obtained using the HPYTM and YTDM. The proposed methods are completed in two steps. First, we implement the Yang transform to simplify the proposed problems. After simplification, Adomian decomposition and homotopy perturbation methods are used to get the close form solution to the issues presented. The significance of fractional derivatives and the method for handling the recurrence relation are both shown in this study. The HPYTM and YTDM are used directly to generate the series solutions. Compared to previous methods examined in the literature, the current schemes exhibit more efficiency and require less computation. With the aid of MAPLE Software, all iterations were calculated. According to the solution graphs, this method can be applied to a wide range of nonlinear fractional differential equations in science and engineering. In subsequent research, these strategies could be expanded to address diverse nonlinear obstacle issues.
This work was supported by Princess Nourah bint Abdulrahman University Researchers Supporting Project (No. PNURSP2022R183).
This work was supported by Princess Nourah bint Abdulrahman University Researchers Supporting Project (No. PNURSP2022R183).
The authors declare that they have no competing interests.
[1] |
T. Al-shami, H. Ibrahim, A. Azzam, A. El-Maghrabi, SR-fuzzy sets and their weighted aggregated operators in application to decision-making, J. Funct. Space., 2022 (2022), 3653225. http://dx.doi.org/10.1155/2022/3653225 doi: 10.1155/2022/3653225
![]() |
[2] |
T. Al-shami, A. Mhemdi, Generalized frame for orthopair fuzzy sets: (m, n)-fuzzy sets and their applications to multi-criteria decision-making methods, Information, 14 (2023), 56. http://dx.doi.org/10.3390/info14010056 doi: 10.3390/info14010056
![]() |
[3] |
T. Al-shami, (2, 1)-Fuzzy sets: properties, weighted aggregated operators and their applications to multi-criteria decision-making methods, Complex Intell. Syst., 9 (2023), 1687–1705. http://dx.doi.org/10.1007/s40747-022-00878-4 doi: 10.1007/s40747-022-00878-4
![]() |
[4] |
T. Al-shami, J. Alcantud, A. Mhemdi, New generalization of fuzzy soft sets: (a, b)-fuzzy soft sets, AIMS Mathematics, 8 (2023), 2995–3025. http://dx.doi.org/10.3934/math.2023155 doi: 10.3934/math.2023155
![]() |
[5] |
Z. Ameen, T. Al-shami, A. Azzam, A. Mhemdi, A novel fuzzy structure: infra-fuzzy topological spaces, J. Funct. Space., 2022 (2022), 9778069. http://dx.doi.org/10.1155/2022/9778069 doi: 10.1155/2022/9778069
![]() |
[6] | K. Atanassov, Intuitionistic fuzzy sets, Fuzzy Set. Syst., 20 (1986), 87–96. http://dx.doi.org/10.1016/S0165-0114(86)80034-3 |
[7] |
K. Atanassov, G. Gargov, Elements of intuitionistic fuzzy logic-Part I, Fuzzy Set. Syst., 95 (1998), 39–52. http://dx.doi.org/10.1016/S0165-0114(96)00326-0 doi: 10.1016/S0165-0114(96)00326-0
![]() |
[8] | C. Chang, Fuzzy topological spaces, J. Math. Anal. Appl., 24 (1968), 182–190. http://dx.doi.org/10.1016/0022-247X(68)90057-7 |
[9] |
D. Coker, An introduction to intuitionistic fuzzy topological spaces, Fuzzy Set. Syst., 88 (1997), 81–89. http://dx.doi.org/10.1016/S0165-0114(96)00076-0 doi: 10.1016/S0165-0114(96)00076-0
![]() |
[10] | B. Cuong, Picture fuzzy sets, Journal of Computer Science and Cybernetics, 30 (2014), 409–420. http://dx.doi.org/10.15625/1813-9663/30/4/5032 |
[11] | B. Cuong, V. Kerinovich, Picture fuzzy sets-a new concept for computational intelligence problems, Proceedings of Third World Congress on Information and Communication Technologies (WICT 2013), 2013, 1–6. http://dx.doi.org/10.1109/WICT.2013.7113099 |
[12] |
P. Dutta, Medical diagnosis based on distance measures between picture fuzzy sets, International Journal of Fuzzy System Applications, 7 (2018), 15–36. http://dx.doi.org/10.4018/IJFSA.2018100102 doi: 10.4018/IJFSA.2018100102
![]() |
[13] |
T. Gerstenkorn, J. Mańko, Correlation of intuitionistic fuzzy sets, Fuzzy Set. Syst., 44 (1991), 39–43. http://dx.doi.org/10.1016/0165-0114(91)90031-K doi: 10.1016/0165-0114(91)90031-K
![]() |
[14] | G. Klir, B. Yuan, Fuzzy sets and fuzzy logic: theory and applications, New Jersey: Prentice-Hall Inc, 1995. |
[15] | O. Montiel, J. Camacho, R. Sepulveda, O. Castillo, Fuzzy system to control the movement of a wheeled mobile robot, In: Soft computing for intelligent control and mobile robotics, Berlin: Springer, 2010,445–463. http://dx.doi.org/10.1007/978-3-642-15534-5_27 |
[16] |
O. Montiel, R. Sepulveda, O. Castillo, A. Basturk, High performance fuzzy systems for real world problems, Adv. Fuzzy Syst., 2012 (2012), 316187. http://dx.doi.org/10.1155/2012/316187 doi: 10.1155/2012/316187
![]() |
[17] |
J. Peng, J. Wang, H. Zhang, T. Sun, X. Chen, OWA aggregation over a continuous fuzzy argument with applications in fuzzy multi-criteria decision-making, J. Intell. Fuzzy Syst., 27 (2014), 1407–1417. http://dx.doi.org/10.3233/IFS-131107 doi: 10.3233/IFS-131107
![]() |
[18] | J. Peters, Associated near sets of distance functions in pattern analysis, In: Multi-disciplinary trends in artificial intelligence, Berlin: Springer, 2011, 1–13. http://dx.doi.org/10.1007/978-3-642-25725-4_1 |
[19] |
A. Razaq, I. Masmali, H. Garg, U. Shuaib, Picture fuzzy topological spaces and associated continuous functions, AIMS Mathematics, 7 (2022), 14840–14861. http://dx.doi.org/10.3934/math.2022814 doi: 10.3934/math.2022814
![]() |
[20] |
M. Sanchez, O. Castillo, J. Castro, P. Melin, Fuzzy granular gravitational clustering algorithm for multivariate data, Inform. Sci., 279 (2014), 498–511. http://dx.doi.org/10.1016/j.ins.2014.04.005 doi: 10.1016/j.ins.2014.04.005
![]() |
[21] |
P. Singh, Correlation coefficients for picture fuzzy sets, J. Intell. Fuzzy Syst., 28 (2015), 591–604. http://dx.doi.org/10.3233/IFS-141338 doi: 10.3233/IFS-141338
![]() |
[22] |
P. Smets, The degree of belief in a fuzzy event, Inform. Sci., 25 (1981), 1–19. http://dx.doi.org/10.1016/0020-0255(81)90008-6 doi: 10.1016/0020-0255(81)90008-6
![]() |
[23] |
M. Sugeno, An introductory survey of fuzzy control, Inform. Sci., 36 (1985), 59–83. http://dx.doi.org/10.1016/0020-0255(85)90026-X doi: 10.1016/0020-0255(85)90026-X
![]() |
[24] |
N. Thao, N. Dinh, Rough picture fuzzy set and picture fuzzy topologies, Journal of Computer Science and Cybernetics, 31 (2015), 245–253. http://dx.doi.org/10.15625/1813-9663/31/3/5046 doi: 10.15625/1813-9663/31/3/5046
![]() |
[25] |
P. Thong, L. Son, Picture fuzzy clustering: a new computational intelligence method, Soft Comput., 20 (2016), 3549–3562. http://dx.doi.org/10.1007/s00500-015-1712-7 doi: 10.1007/s00500-015-1712-7
![]() |
[26] |
C. Tian, J. Peng, S. Zhang, W. Zhang, J. Wang, Weighted picture fuzzy aggregation operators and their applications to multi-criteria decision-making problems, Comput. Ind. Eng., 137 (2019), 106037. http://dx.doi.org/10.1016/j.cie.2019.106037 doi: 10.1016/j.cie.2019.106037
![]() |
[27] |
C. Tian, J. Peng, S. Zhang, J. Wang, M. Goh, A sustainability evaluation framework for WET-PPP projects based on a picture fuzzy similarity-based VIKOR method, J. Clean. Prod., 289 (2021), 125130. http://dx.doi.org/10.1016/j.jclepro.2020.125130 doi: 10.1016/j.jclepro.2020.125130
![]() |
[28] | V. Visalakshi, M. Uma, E. Roja, On soft fuzzy C-structure compactification, Kochi Journal of Mathematics, 8 (2013), 119–133. |
[29] |
Z. Wang, Z. Xu, S. Liu, J. Tang, A netting clustering analysis method under intuitionistic fuzzy environment, Appl. Soft Comput., 11 (2011), 5558–5564. http://dx.doi.org/10.1016/j.asoc.2011.05.004 doi: 10.1016/j.asoc.2011.05.004
![]() |
[30] |
Z. Xu, J. Chen, J. Wu, Clustering algorithm for intuitionistic fuzzy sets, Inform. Sci., 178 (2008), 3775–3790. http://dx.doi.org/10.1016/j.ins.2008.06.008 doi: 10.1016/j.ins.2008.06.008
![]() |
[31] | T. Yogalakshmi, O. Castillo, On intuitionistic fuzzy C-ends, In: Advances in algebra and analysis, Cham: Birkhäuser, 2018,177–184. https://dx.doi.org/10.1007/978-3-030-01120-8_21 |
[32] | L. Zadeh, Fuzzy sets, Information and Control, 8 (1965), 338–353. http://dx.doi.org/10.1016/S0019-9958(65)90241-X |
ϑ | κ | ρ=0.6 | ρ=0.8 | ρ=1(approx) | ρ=1(approx) | ρ=1(exact) |
0.2 | 0.000004490 | 0.000004487 | 0.000004483 | 0.000004483 | 0.000004479 | |
0.4 | 0.000005481 | 0.000005479 | 0.000005476 | 0.000005476 | 0.000005470 | |
0.01 | 0.6 | 0.000006693 | 0.000006691 | 0.000006688 | 0.000006688 | 0.000006681 |
0.8 | 0.000008171 | 0.000008171 | 0.000008169 | 0.000008169 | 0.000008161 | |
1 | 0.000009982 | 0.000009980 | 0.000009978 | 0.000009978 | 0.000009968 | |
0.2 | 0.000004490 | 0.000004487 | 0.000004483 | 0.000004483 | 0.000004474 | |
0.4 | 0.000005482 | 0.000005478 | 0.000005476 | 0.000005476 | 0.000005465 | |
0.02 | 0.6 | 0.000006693 | 0.000006690 | 0.000006688 | 0.000006688 | 0.000006675 |
0.8 | 0.000008177 | 0.000008172 | 0.000008169 | 0.000008169 | 0.000008153 | |
1 | 0.000009987 | 0.000009982 | 0.000009978 | 0.000009978 | 0.000009958 | |
0.2 | 0.000004489 | 0.000004485 | 0.000004483 | 0.000004483 | 0.000004470 | |
0.4 | 0.000005489 | 0.00000583 | 0.000005476 | 0.000005476 | 0.000005459 | |
0.03 | 0.6 | 0.000006693 | 0.000006690 | 0.000006688 | 0.000006688 | 0.000006668 |
0.8 | 0.000008179 | 0.000008174 | 0.000008169 | 0.000008169 | 0.000008145 | |
1 | 0.000009989 | 0.000009982 | 0.000009978 | 0.000009978 | 0.000009948 | |
0.2 | 0.000004492 | 0.000004487 | 0.000004483 | 0.000004483 | 0.000004465 | |
0.4 | 0.000005487 | 0.000005481 | 0.000005476 | 0.000005476 | 0.000005454 | |
0.04 | 0.6 | 0.000006699 | 0.000006691 | 0.000006688 | 0.000006688 | 0.000006661 |
0.8 | 0.000008183 | 0.000008177 | 0.000008169 | 0.000008169 | 0.000008136 | |
1 | 0.000009999 | 0.000009983 | 0.000009978 | 0.000009978 | 0.000009938 | |
0.2 | 0.000004497 | 0.000004489 | 0.000004483 | 0.000004483 | 0.000004461 | |
0.4 | 0.000005490 | 0.000005479 | 0.000005476 | 0.000005476 | 0.000005448 | |
0.05 | 0.6 | 0.000006697 | 0.000006691 | 0.000006688 | 0.000006688 | 0.000006655 |
0.8 | 0.000008191 | 0.000008185 | 0.000008169 | 0.000008169 | 0.000008128 | |
1 | 0.000009997 | 0.000009988 | 0.000009978 | 0.000009978 | 0.000009928 |
ϑ | κ | ρ=0.6 | ρ=0.8 | ρ=1(approx) | ρ=1(approx) | ρ=1(exact) |
0.2 | 0.000004490 | 0.000004487 | 0.000004483 | 0.000004483 | 0.000004479 | |
0.4 | 0.000005481 | 0.000005479 | 0.000005476 | 0.000005476 | 0.000005470 | |
0.01 | 0.6 | 0.000006693 | 0.000006691 | 0.000006688 | 0.000006688 | 0.000006681 |
0.8 | 0.000008171 | 0.000008171 | 0.000008169 | 0.000008169 | 0.000008161 | |
1 | 0.000009982 | 0.000009980 | 0.000009978 | 0.000009978 | 0.000009968 | |
0.2 | 0.000004490 | 0.000004487 | 0.000004483 | 0.000004483 | 0.000004474 | |
0.4 | 0.000005482 | 0.000005478 | 0.000005476 | 0.000005476 | 0.000005465 | |
0.02 | 0.6 | 0.000006693 | 0.000006690 | 0.000006688 | 0.000006688 | 0.000006675 |
0.8 | 0.000008177 | 0.000008172 | 0.000008169 | 0.000008169 | 0.000008153 | |
1 | 0.000009987 | 0.000009982 | 0.000009978 | 0.000009978 | 0.000009958 | |
0.2 | 0.000004489 | 0.000004485 | 0.000004483 | 0.000004483 | 0.000004470 | |
0.4 | 0.000005489 | 0.00000583 | 0.000005476 | 0.000005476 | 0.000005459 | |
0.03 | 0.6 | 0.000006693 | 0.000006690 | 0.000006688 | 0.000006688 | 0.000006668 |
0.8 | 0.000008179 | 0.000008174 | 0.000008169 | 0.000008169 | 0.000008145 | |
1 | 0.000009989 | 0.000009982 | 0.000009978 | 0.000009978 | 0.000009948 | |
0.2 | 0.000004492 | 0.000004487 | 0.000004483 | 0.000004483 | 0.000004465 | |
0.4 | 0.000005487 | 0.000005481 | 0.000005476 | 0.000005476 | 0.000005454 | |
0.04 | 0.6 | 0.000006699 | 0.000006691 | 0.000006688 | 0.000006688 | 0.000006661 |
0.8 | 0.000008183 | 0.000008177 | 0.000008169 | 0.000008169 | 0.000008136 | |
1 | 0.000009999 | 0.000009983 | 0.000009978 | 0.000009978 | 0.000009938 | |
0.2 | 0.000004497 | 0.000004489 | 0.000004483 | 0.000004483 | 0.000004461 | |
0.4 | 0.000005490 | 0.000005479 | 0.000005476 | 0.000005476 | 0.000005448 | |
0.05 | 0.6 | 0.000006697 | 0.000006691 | 0.000006688 | 0.000006688 | 0.000006655 |
0.8 | 0.000008191 | 0.000008185 | 0.000008169 | 0.000008169 | 0.000008128 | |
1 | 0.000009997 | 0.000009988 | 0.000009978 | 0.000009978 | 0.000009928 |