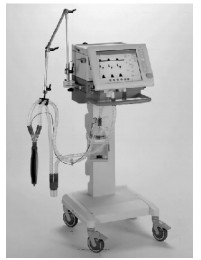
In light of the COVID-19 pandemic, many patients have suffered from Acute Respiratory Distress Syndrome (ARDS) in Intensive Care Units (ICUs) around the world. In the medical field, it is known that the so-called artificial ventilation device, which has become the mainstay of treatment of such syndrome, decreases mortality in critically ill COVID-19 patients. Due to the high reliability of this device, there is an emergency need to follow up the progress made on designing a robust controller for improving its performance. From this perspective, this work introduces different control design schemes for obtaining an optimal Fractional-order PID controller (or simply PIρDμ-controller) of the Artificial Ventilation (AV) system through two optimization algorithms: the Bacteria Foraging Optimization (BFO) and the Particle Swarm Optimization (PSO) algorithms. The realization of the controller is accomplished using four approximations: Oustaloup's approximation, the Continued Fractional Expansion (CFE) approximation and the 1st- and 2nd-order El-Khazali approximations. The validation of the controller design and the AV system behavior are verified via numerical simulation in order to demonstrate the effectiveness and the potency of all proposed schemes.
Citation: Iqbal M. Batiha, Reyad El-Khazali, Osama Y. Ababneh, Adel Ouannas, Radwan M. Batyha, Shaher Momani. Optimal design of PIρDμ-controller for artificial ventilation systems for COVID-19 patients[J]. AIMS Mathematics, 2023, 8(1): 657-675. doi: 10.3934/math.2023031
[1] | Menderes Gashi . On the symmetric block design with parameters (280,63,14) admitting a Frobenius group of order 93. AIMS Mathematics, 2019, 4(4): 1258-1273. doi: 10.3934/math.2019.4.1258 |
[2] | Weerawat Sudsutad, Jehad Alzabut, Chutarat Tearnbucha, Chatthai Thaiprayoon . On the oscillation of differential equations in frame of generalized proportional fractional derivatives. AIMS Mathematics, 2020, 5(2): 856-871. doi: 10.3934/math.2020058 |
[3] | Songran Wang, Zhinmin Wang . Function space properties of the Cauchy transform on the Sierpinski gasket. AIMS Mathematics, 2023, 8(3): 6064-6073. doi: 10.3934/math.2023306 |
[4] | Alexander G. Ramm . When does a double-layer potential equal to a single-layer one?. AIMS Mathematics, 2022, 7(10): 19287-19291. doi: 10.3934/math.20221058 |
[5] | Chin-Lin Shiue, Tzu-Hsien Kwong . Distance 2-restricted optimal pebbling in cycles. AIMS Mathematics, 2025, 10(2): 4355-4373. doi: 10.3934/math.2025201 |
[6] | Xiaoyu Qian, Jiang Zhou . Morrey spaces on weighted homogeneous trees. AIMS Mathematics, 2025, 10(4): 7664-7683. doi: 10.3934/math.2025351 |
[7] | Essam H. Houssein, Nagwan Abdel Samee, Maali Alabdulhafith, Mokhtar Said . Extraction of PEM fuel cell parameters using Walrus Optimizer. AIMS Mathematics, 2024, 9(5): 12726-12750. doi: 10.3934/math.2024622 |
[8] | Naila Mehreen, Rashid Farooq, Shehnaz Akhter . On partition dimension of fullerene graphs. AIMS Mathematics, 2018, 3(3): 343-352. doi: 10.3934/Math.2018.3.343 |
[9] | Ruishen Qian, Xiangling Zhu . Invertible weighted composition operators preserve frames on Dirichlet type spaces. AIMS Mathematics, 2020, 5(5): 4285-4296. doi: 10.3934/math.2020273 |
[10] | Xinfeng Zhang, Zhibin Zhu, Chongqi Zhang . Multi-stage differential evolution algorithm for constrained D-optimal design. AIMS Mathematics, 2021, 6(3): 2956-2969. doi: 10.3934/math.2021179 |
In light of the COVID-19 pandemic, many patients have suffered from Acute Respiratory Distress Syndrome (ARDS) in Intensive Care Units (ICUs) around the world. In the medical field, it is known that the so-called artificial ventilation device, which has become the mainstay of treatment of such syndrome, decreases mortality in critically ill COVID-19 patients. Due to the high reliability of this device, there is an emergency need to follow up the progress made on designing a robust controller for improving its performance. From this perspective, this work introduces different control design schemes for obtaining an optimal Fractional-order PID controller (or simply PIρDμ-controller) of the Artificial Ventilation (AV) system through two optimization algorithms: the Bacteria Foraging Optimization (BFO) and the Particle Swarm Optimization (PSO) algorithms. The realization of the controller is accomplished using four approximations: Oustaloup's approximation, the Continued Fractional Expansion (CFE) approximation and the 1st- and 2nd-order El-Khazali approximations. The validation of the controller design and the AV system behavior are verified via numerical simulation in order to demonstrate the effectiveness and the potency of all proposed schemes.
Nowadays, all hospitals around the world have confronted a huge surge of COVID-19 patients that require emergency health-care and services. This matter, however, has caused an enormous pressure on the available resources in many hospitals, especially with limited resources [1]. Meanwhile, worldwide knowledge and expertise have rapidly developed new and innovative ideas to reduce the spread of COVID-19 and treat infected patients. Acute Respiratory Distress Syndrome (ARDS) is one of the likely symptoms of coronavirus patients that can possibly cause death [2]. This intractable symptom can be overcome using one of the significant medical devices called an Artificial Ventilator (AV). In general, such a device is put forward to help individuals to regain healthy breathing when normal breathing is not possible. The principle of its operation is based on introducing oxygen into the lungs and withdrawing extra carbon dioxide from the body. This would enhance the gas exchange rate and hence provide comfortable breathing of sufferers. In view of technological advancement and continual modernization in introducing some technical procedures associated with control theory for medical devices, an AV system is one of these typical devices (see, e.g., [3,4,5,6,7,8]). Hence, for complementing this work, this paper proposes several optimal proportional-integral-derivative fractional-order controllers (or simply PIρDμ-controllers), where 0<ρ<1 and 0<μ<1 represent the orders of the integral and the differential parts of the controller, respectively. This is for the purpose of developing dynamic stabilization capabilities of the Volume-Controlled Artificial Ventilation (VCAV) system, see Figure 1.
It is known that the optimized PIρDμ-controller has better performance than the traditional PID controller. As is known to all, it can offer more degrees of freedom by adding two parameters to the construction of the traditional one, as was reported in numerous references. For instance, it was demonstrated in [9] that one can obtain better simulation results with the use of the PIρDμ-controller than with the integer-order PID controllers when we deal with some time-delay systems. In addition, the same result was presented in [10], when the authors designed a multivariable decoupling PIρDμ-controller for variable air volume systems.
In this work, all proposed controllers have been optimally designed by carrying out two optimization techniques, namely, Particle Swarm Optimization (PSO) [11] and Bacteria Foraging Optimization (BFO) [12], for the purpose of fulfilling the high performance of the VCAV system. In particular, the key role of these two techniques is minimizing the objective function's value in light of specific constraints related to time or frequency domain, such as the system's rise time, overshoot and settling time. These constraints have a major task in quantifying the robustness of the controlled system. It is entirely normal that, once the two algorithms generate successfully the five optimal parameters (κp, κi, κd, ρ and μ) of the PIρDμ-controller, the focus would be oriented towards the needs of approximating the so-called fractional-order Laplacian operator sρ and/or sμ (or simply s±γ, where γ={ρ, μ} and 0<γ<1). Such approximation would be in the form of a finite integer-order rational transfer function due to the arbitrariness of sγ in its original form [13,14,15,16,17,18]. Actually, this new analytical function of sγ, which permits one to design and analyze the system without a need to address some hard time-domain compositions, can be typically derived by applying multiple approximations, like Oustaloup's approximation [19,20], the Continued Fraction Expansion (CFE) [19,21] approximation and more recently the 1st- and 2nd-order El-Khazali approximations [22,23]. In this work, only these four approximations will be used in order to provide the operators s±γ with their corresponding transfer functions. Thus, we intend to tune eight PIρDμ-BFO/PSO controllers by generating them through implementing the two aforesaid optimization algorithms. Numerical simulations of these eight controllers provide sufficient information to adopt the best performance of the controlled AV system, such as settling time, minimal overshoot and least rise time.
The rest of the paper is arranged as follows: An overview of the fractional-order linear time-invariant system is presented in the next section, followed by presenting some basic concepts of designing PIρDμ-controllers in Section 2. Four approximations of finite-order rational transfer functions of the fractional-order integro-differential Laplacian operators are outlined in Section 3, while the final section includes the main results and all numerical outcomes of the proposed design methods.
Fundamentally, the elementary principles of fractional calculus are typically utilized as a tool to transmit a class of control systems called the integer-order linear-time invariant (LTI) system into its fractional-order version, which is called the fractional-order LTI system (or simply FoLTI system) [24,25]. In light of some manifestations presented in [24,26], it has been confirmed that the FoLTI systems exceed the other integer-order counterparts by virtue of their flexibility in taking into account more additional parameters. In general, a FoLTI system can be expressed by the following fractional-order differential equation [24,27]:
pnDδny(t)+pn−1Dδn−1y(t)+...+p1Dδ1y(t)+p0Dδ0y(t)=qmDνmu(t)+qm−1Dνm−1u(t)+...+q1Dν1u(t)+q0Dν0u(t), | (2.1) |
where y(t) and u(t) are two variables over the time t that indicate the control output and input of the system, respectively, and where D{δi,νk} represents the Caputo fractional differential operator of orders δi,i=1,2,3,...,n, and νk,k=1,2,3,...,m, such that n,m∈N.
Modeling system (2.1) can be accomplished by replacing s±γ by finite-order rational transfer functions, where 0<γ<1. This would permit one to design and analyze the controlled system with finite-order controllers. The numerical method that generates such an approximate transfer function determines the bounds on the order of the controller. In a similar context, the frequency response of the LTI system can be represented by a transfer function which is the ratio of the Laplace transform of the system's output to the Laplace transform of its input for zero initial conditions [27], i.e.,
T(s)=Y(s)U(s)=qmsνm+qm−1sνm−1+...+q1sν1+q0sν0pnsδn+pn−1sδn−1+...+p1sδ1+p0sδ0, | (2.2) |
where Y(s)=L{y(t)}, U(s)=L{u(t)} are the Laplace transforms of y(t) and u(t), respectively.
The principal structure of the PIρDμ-controller was proposed by Podlubny et al. [28]. It was shown that the PIρDμ-controller outperforms the traditional PID-controller. From this vantage point, many real-life technical applications have been improved by applying this controller. The structure of this controller is based upon appending two further parameters (ρ and μ) to the primary parameters (κp,κi,κd) of the traditional PID-controller. Those two additional parameters would offer additional degrees of freedom in the controller algorithms. Nevertheless, the PIρDμ-controller is definitely inferred from the following fractional-order integro-differential equation [24,28]:
u(t)=κpe(t)+κiIρe(t)+κdDμe(t), | (3.1) |
where e(t) is the error signal, Dμ is the Caputo operator of order μ, and Iρ is the Riemann-Liouville operator of order ρ. The transfer function of the PIρDμ-controller is given by
C(s)=U(s)E(s)=κp+κis−ρ+κdsμ, | (3.2) |
where E(s)=L{e(t)}.
The subsequent mission concentrates on using the PIρDμ-controller with one of the most significant industrial systems during this coronavirus time: the AV system. The key purpose of implementing such a controller is to further improve the process control of the VCAV system by enhancing its dynamic performance. This demands implementing a robust optimization algorithm to enhance the system's step response by means of optimally designing the five parameters of the PIρDμ-controller. For this purpose, the BFO and PSO algorithms will be applied to determine the optimum values of these parameters through different approximations of s±γ that are encountered in (3.2), where γ={ρ, μ}, 0<γ<1. It is necessary to establish the main objective function of the optimization algorithms. Notice that there are multiple standard objective functions that could be engaged for tuning the best parameters for the PIρDμ-controller, like the Integral Absolute Error (IAE), Integral Time Square Error (ITSE), Integral Time-Absolute Error (ITAE) and Integral Square Error (ISE). For instance, minimizing any objective function value is the key goal of the chosen optimization algorithm with the aim of accomplishing the best values of the PIρDμ-controller. The motivation of using the PSO and BFO algorithms comes back to their simple concepts, easy implementations, powerful abilities to control parameters and computational efficiencies when compared with other mathematical algorithms and other heuristic optimization techniques [29]. Nevertheless, to get a full overview about the BFO and PSO algorithms, one may consult [27,30,31,32,33,34]. The design procedure of the PIρDμ-controller through the BFO and the PSO algorithms is described by the block diagram shown in Figure 2.
To obtain the best parameters of the PIρDμ-controller, an objective function is established based on four terms to be minimized: the rise time, steady-state error, peak overshoot and the settling time of the controlled system. It takes the following form [32,35]:
J=(1−e−β)(Mp+ess)+e−β(Ts−Tr), | (3.3) |
where ess is the steady state error, Ts is the settling time, Tr is the rise time, Mp is the peak overshoot and β is a scaling factor. It is relevant to note that although the scaling factor β is typically chosen by a designer, it can definitely identify the roles of the four aforementioned items in the basic objective function [32,35]. In this work, this factor has been selected to be 0.5 for the same reasons as reported in [32].
This part briefly introduces four approximations formulated as finite-order rational transfer functions of the fractional-order integro-differential Laplacian operators, s±γ, where 0<γ<1. The need to employ such approximations promptly emerges after determining the best values of the PIρDμ-controller by applying some optimization algorithms. Those four approximations permit one to design the target system without the need to treat some hard time-domain compositions [13,14].
El-Khazali proposed two practical approximations of 1st- and 2nd-orders, respectively. The 1st-order approximation is represented by a rational transfer function to replace sγ, where 0<γ<1, and given by [22]:
sγ=N(s,γ)D(s,γ)≅sτωcn+1sωcn+τ, | (4.1) |
where ωcn is a corner frequency, and
τ=tanγπ2+secγπ2. | (4.2) |
It is worth mentioning that the fractional-order integral Laplacian operator s−γ could be simply found from the reciprocal of (4.1) [22]. MATLAB Code C1, to calculate (4.1), is given in Appendix A for completeness.
In [23], a 2nd-order approximation was proposed for setting up a finite-order rational transfer function of the operators sγ, where 0<γ<1. The reciprocal of such approximation yields s−γ. It provides an exact phase response at its center frequency and can be represented by the following modular structure [23]:
(sωg)γ=n∏i=1Fi(s/ωi)=n∏i=1Ni(s(ωi/ωg))Di(s(ωi/ωg)), | (4.3) |
where ωi,i=1,2,...,n, is the center frequency of each biquadratic module and ωg=n√∏ni=1ωi is their geometric mean. If the leading center frequency ω1 of the beginning section is determined, then to detect the constant phase element, the next center frequencies of every section could be evaluated from the following recursive equation [19]:
ωi=ω2(i−1)xω1, i=2,3,...,n, | (4.4) |
where ωx is the maximum real solution of the following equation:
a0a2ηλ4+a1(a2−a0)λ3+(a21−a22−a20)ηλ2+a1(a2−a0)λ+a0a2η=0, | (4.5) |
where η=tanγπ4. Every biquadratic component in (4.3) can be determined by
(sωg)γ=Fi(sωi)=Ni(sωi)Di(sωi)≅a0(sωi)2+a1(sωi)+a2a2(sωi)2+a1(sωi)+a0, | (4.6) |
where i=1,2,3,..., and
{a0=γγ+2γ+1a2=γγ−2γ+1a1=(a2−a0)tan((2+γ)π4)=−6γ tan((2+γ)π4). | (4.7) |
Note that (4.6) is the exclusive approximation that leads to Fi(sωi)=(sωi) as γ→1. In other words,
Fi(sωi)=a0(sωi)2+a0(sωi)a0(sωi)+a0=(sωi). | (4.8) |
Similarly, Code C2 in Appendix A simulates the approximation given by (4.6).
This approximation is the most widespread one among several approximations that are employed to generate approximate finite-order rational transfer functions for the operators s±γ, where 0<γ<1. The bandwidth could be defined to offer a proper adaptation to such operators by predetermining the frequency band. Typically, in order to obtain a finite-order approximation of sγ over the frequency range (ωb,ωh), the following rational transfer function is implemented [36]:
sγ≅KN∏k=−Ns+ω′ks+ωk, | (4.9) |
where the gain, zeros and poles can be calculated from the following states:
ωk=ωb(ωhωb)k+N+0.5(1+γ)2N+1 | (4.10a) |
ω′k=ωb(ωhωb)k+N+0.5(1−γ)2N+1 | (4.10b) |
K=(ωhωb)−γ2N∏k=−Nωkω′k. | (4.10c) |
It should be pointed out that the following equation allows one to compute the unity-gain geometric frequency ωu [36]:
ωu=√ωb⋅ωh. | (4.11) |
Having the form of the transfer function given in (4.9) in mind, one could observe that such a function will be always of odd order (n=2N+1). Anyhow, Code C3, given in Appendix A, illustrates suitable MATLAB code for calculating a finite-order rational transfer function corresponding to s±γ using Oustaloup's approximation, where 0<γ<1.
This scheme is regarded as the principal mathematical method for offering approximate rational transfer functions for s±γ, where 0<γ<1. This approximation was set as follows [21]:
(1+z)γ=11−γz1+(1+γ)z2+(1−γ)z3−(2+γ)z2+(2−γ)z5+…+(n+γ)z2+(n−γ)z2n+1+..., | (4.12) |
where 0<γ<1, and n∈N. For the sake of simplification, (4.12) can be rewritten in the following form [21]:
(1+z)γ=11−γz1+(1+γ)z2+(1−γ)z3+(2+γ)z2+(2−γ)z5+(n+γ)z2+(n−γ)z2n+1+. | (4.13) |
In order to find a proper finite-order approximation for sγ using (4.12), one may replace z=(sγ−1) in (4.12) or (4.13). This replacement step permits the nth-order approximation of such operator to be found, about the center frequency ω0=1 rad/sec, in the following form [26]:
sγ≅γ0sn+γ1sn−1+…+γn−1s+γnγnsn+γn−1sn−1+…+γ1s+γ0, | (4.14) |
where γi∈(0, 1), which can be found in [37] for i=0,1,...,5. Two more observations should be made at the end of this part. The first one is associated with the operator s−γ, which could be easily achieved by inverting upside down the formula given in (4.14). The second one is related to computing the finite-order rational transfer function corresponding to s±γ using the CFE approximation, which can be illustrated by tracking the MATLAB Code C4 given in Appendix A.
It is common knowledge in the biomedical engineering field that the 1st-order lumped parameter model is the most streamlined model for the mechanics of the breathing system. In this model, the pressure in the upper airways is typically assumed to equal zero throughout spontaneous ventilation. The diaphragm, if it is active, may add subatmospheric pressure [38,39]. In general, there are commonly two actuators within many breathing systems of ventilators employed in ICU or anesthesia. These actuators are responsible for exhaling and inhaling processes. Since the AV system is just one of those breathing systems, its major components consist of these two actuators. In many circumstances, the exhaling actuator is actuated by a Positive End Expiratory Pressure (PEEP) valve, whereas the inhalator actuator, on the other hand, can be actuated by a piston drive mechanism. Also, the inhalator actuator system is linked with piston driven and lung mechanisms [38,39]. The overall system could be modeled by a 3rd-order differential equation by taking into account the linear friction and the electrical time constant of the motor (see [38,39]). Through such system, the two input variables Tl and Va, which respectively indicate the load torque and the applied motor voltage, are fed back to the subsystem of the lung mechanics. In particular, in view of possible estimations for all required parameters of the VCAV system, an open-loop transfer function of this system has been recently reported in [38] as follows:
G(s)=Y(s)Vi(s)=14.471s3+76.43s2+109.76s+0.129, | (5.1) |
where Y(s)=L{y(t)} and Vi(s)=L{vi(t)} are respectively the Laplace transforms of the output variable, y(t), which indicates the piston position, and the input variable of the system, vi(t), which indicates the voltage source of the motor.
The key target of this work is to enhance the dynamic behavior of the VCAV system by considering the transfer function given in (5.1). However, to show the efficiency of all feedback tuning schemes of PIρDμ-controllers, the objective function given in (3.3) is minimized by running the BFO and PSO algorithms, which take into account the four approximations of s±γ in Section 3. The maximum value of iterations and the population size in both algorithms have been taken as 100 and 20, respectively. The optimum parameters of the PIρDμ-controllers for β=0.5 and β=0.9, which are used to generate the corresponding approximation schemes, are given in Tables 1 and 2, respectively.
Algorithm | Type of approximation | κp | κi | κd | ρ | μ |
PSO | 1st-order El-Khazali | 31.4119 | 0.54000 | 25.4162 | 0.31300 | 0.90600 |
2nd-order El-Khazali | 41.0000 | 15.4404 | 45.0000 | 0.09000 | 0.91100 | |
Oustaloup | 59.0000 | 0.76000 | 61.0000 | 0.82100 | 0.92503 | |
CFE | 0.16000 | 45.5900 | 51.0000 | 0.17700 | 0.86700 | |
BFO | 1st-order El-Khazali | 2.71690 | 2.50680 | 7.71930 | 0.91510 | 0.57810 |
2nd-order El-Khazali | 20.8839 | 12.9392 | 21.7497 | 0.86760 | 0.90610 | |
Oustaloup | 25.4065 | 18.5733 | 32.2031 | 0.54090 | 0.15850 | |
CFE | 2.56650 | 10.0268 | 15.2471 | 0.18520 | 0.33260 |
Algorithm | Type of approximation | κp | κi | κd | ρ | μ |
PSO | 1st-order El-Khazali | 31.6262 | 0.5400 | 25.5702 | 0.9030 | 0.9060 |
2nd-order El-Khazali | 41.000 | 0.3200 | 45.000 | 0.7990 | 0.8557 | |
Oustaloup | 59.000 | 24.5393 | 61.0000 | 0.1650 | 0.9760 | |
CFE | 41.5691 | 0.3100 | 51.000 | 0.1770 | 0.8670 | |
BFO | 1st-order El-Khazali | 4.5230 | 4.3728 | 6.7855 | 0.8750 | 0.2870 |
2nd-order El-Khazali | 13.7804 | 2.0452 | 16.5894 | 0.2662 | 0.9468 | |
Oustaloup | 17.5025 | 11.2505 | 21.6484 | 0.8129 | 0.2091 | |
CFE | 2.2440 | 2.1768 | 8.1292 | 0.9151 | 0.5781 |
Remark 1. The scaling factor β is chosen to define a trade-off between the overshoot and the steady-state error (Mp+ess) and the time difference between the settling and the rise times (Ts−Tr). For example, for β>0.7 more emphasis will be given to (Mp+ess) than (Ts−Tr). The impact of β is highlighted by several numerical results of the proposed system model for β=0.5 and β=0.9, reported below for completeness.
Clearly, Figures 3 and 4 show the performances of the closed-loop controlled system using both the PSO and BFO optimization algorithms by utilizing the four different approximations of the fractional-order operator repeated many times, coupled with assuming β=0.5. In addition, we also plot in Figures 5 and 6 the performances of the closed-loop controlled system using both algorithms by assuming β=0.9.
In both optimization techniques, the size of the controllers when using El-Khazali approximation is much less than that of the other two ones, as depicted in Tables 3 and 4. Moreover, there is a significant improvement in the step responses when using the PSO algorithm over that of the BFO algorithm. More precisely, one could notice that the minimum overshoot of the closed-loop VCAV system has been achieved when the PIρDμ-controller was applying the PSO algorithm.
Algorithm | Type of approximation | Controller transfer function, C(s) |
PSO | 1st-order El-Khazali approximation | C{1st−Kh}(s)=626.9s2+1135s+462.31.67s2+23.58s+13.52 |
2nd-order El-Khazali approximation | C{2nd−Kh}(s)=344.4s4+1705s3+2852s2+1810s+3710.1917s4+8.814s3+28.9s2+25.83s+6.079 | |
Oustaloup approximation | COus(s)=1.92e05s10+8.374e06s9+1.191e08s8+6.29e08s7+1.468e09s6+1.569e09s5 +7.846e08s4+1.793e08s3+1.675e07s2+6.184e05s+659943.85s10+5837s9+1.847e05s8+2.14e06s7+9.029e06s6+1.54e07s5 +9.936e06s4+2.592e06s3+2.463e05s2+8567s+70.81 | |
The CFE approximation | CCFE(s)=1.637e04s10+5.268e05s9+5.684e06s8+2.671e07s7+6.385e07s6+8.343e07s5 +6.143e07s4+2.489e07s3+5.182e06s2+4.744e05s+1.467e042.254s10+543.4s9+1.462e04s8+1.312e05s7+5.014e05s6+9.102e05s5 +8.165e05s4+3.595e05s3+7.334e04s2+6038s+142 | |
BFO | 1st-order El-Khazali approximation | C{1st−Kh}(s)=379.1s2+303.8s+124.714.97s2+44.52s+2.907 |
2nd-order El-Khazali approximation | C{2nd−Kh}(s)=301.3s4+1018s3+1314s2+772.4s+186.40.3702s4+15.65s3+31.49s2+16.57s+0.555 | |
Oustaloup approximation | COus(s)=1132s10+8.422e04s9+1.968e06s8+1.638e07s7+5.564e07s6+7.554e07s5 +4.426e07s4+1.068e07s3+1.106e06s2+4.292e04s+550.112.07s10+1008s9+2.53e04s8+2.234e05s7+7.698e05s6+1.02e06s5 +5.413e05s4+1.104e05s3+8797s2+246.4s+2.075 | |
The CFE approximation | CCFE(s)=184.2s10+7443s9+1.039e05s8+5.885e05s7+1.588e06s6+2.15e06s5 +1.499e06s4+5.243e05s3+8.724e04s2+5874s+137.92.342s10+141.1s9+2552s8+1.726e04s7+5.29e04s6+7.875e04s5 +5.864e04s4+2.135e04s3+3575s2+232.4s+4.71 |
Algorithm | Type of approximation | Controller transfer function, C(s) |
PSO | 1st-order El-Khazali approximation | C{1st−Kh}(s)=4944s2+6329s+548.813.1s2+178.1s+13.52 |
2nd-order El-Khazali approximation | C{2nd−Kh}(s)=577.3s4+2012s3+2207s2+778.2s+40.670.5624s4+15.49s3+31.31s2+16.83s+0.8531 | |
Oustaloup approximation | COus(s)=1.529e06s10+7.401e08s9+9.569e10s8+1.819e12s7+1.058e13s6+1.81e13s5 +1.14e13s4+1.881e12s3+8.537e10s2+5.842e08s+1.088e063.126s10+3.135e04s9+1.393e07s8+1.702e09s7+2.953e10s6+1.378e11s5 +1.149e11s4+1.94e10s3+7.952e08s2+4.859e06s+8017 | |
The CFE approximation | CCFE(s)=9.315e04s10+2.947e06s9+3.076e07s8+1.401e08s7+3.212e08s6+3.971e08s5 +2.709e08s4+9.988e07s3+1.848e07s2+1.468e06s+3.426e042.254s10+2084s9+6.151e04s8+5.866e05s7+2.343e06s6+4.418e06s5 +4.103e06s4+1.87e06s3+3.946e05s2+3.376e04s+809.4 | |
BFO | 1st-order El-Khazali approximation | C{1st−Kh}(s)=134.8s2+187.8s+66.7810.15s2+13.45s+1.226 |
2nd-order El-Khazali approximation | C{2nd−Kh}(s)=144.4s4+613.8s3+875.3s2+475.1s+80.650.1251s4+9.486s3+29.32s2+24.11s+4.499 | |
Oustaloup approximation | COus(s)=4.56e04s10+6.061e07s9+6.876e09s8+1.95e11s7+1.091e12s6+1.394e12s5 +6.238e11s4+7.936e10s3+2.854e09s2+2.559e07s+2.134e04274.6s10+6.801e05s9+9.577e07s8+3.703e09s7+2.237e10s6+3.132e10s5 +9.348e09s4+4.765e08s3+6.737e06s2+1.324e04s+6.861 | |
The CFE approximation | CCFE(s)=3.669e04s10+9.368e05s9+8.288e06s8+3.18e07s7+6.075e07s6+6.215e07s5 +3.635e07s4+1.26e07s3+2.545e06s2+2.556e05s+9712262.6s10+2.016e04s9+3.028e05s8+1.752e06s7+4.556e06s6+5.762e06s5 +3.585e06s4+1.068e06s3+1.365e05s2+6128s+16.91 |
Tables 5 and 6, however, list the performance results of the unit-step responses of the controlled system using the four approximations for β=0.5 and β=0.9, respectively. It can be seen from Table 5 that the three approximations, i.e., Oustaloup, CFE, and the 2nd-order El-Khazali approximations, gave the same performance. However, the 2nd-order El-Khazali approximation yields a 4th-order PIρDμ, while the other two yield 10th-order controllers. This is a significant reduction in the size of controller. A closer look at the rise time, settling time and the maximum overshoot justifies the use of the El-Khazali 2nd-order approximation. Similarly, when using the BFO optimization algorithm, the 2nd-order El-Khazali approximation was superior to the other three approximations. Therefore, both remarks satisfy the use of the biquadratic approximation described by Eqs (4.3) to (4.8).
Step Response | HPSO{1st−Kh} | HPSO{2nd−Kh} | HPSOCFE | HPSOOus | HBFO{1st−Kh} | HBFO{2nd−Kh} | HBFOCFE | HBFOOus |
Rise Time | 0.2829 | 0.1858 | 0.2333 | 0.1516 | 1.3512 | 0.4828 | 0.5938 | 0.3225 |
Settling Time | 0.4526 | 0.3260 | 0.3783 | 0.2514 | 14.6911 | 5.2044 | 3.3122 | 4.7305 |
Settling Min. | 0.9001 | 0.9010 | 0.9012 | 0.9008 | 0.9017 | 0.9000 | 0.9079 | 0.7189 |
Settling Max. | 0.9997 | 0.9998 | 0.9999 | 0.9994 | 1.1660 | 1.0741 | 1.2092 | 1.5552 |
Overshoot | 5.2705e-04 | 0.0000 | 0.0000 | 0.0000 | 16.6236 | 7.4173 | 20.9616 | 55.5270 |
Peak | 0.9997 | 0.9998 | 0.9999 | 0.9994 | 1.1660 | 1.0741 | 1.2092 | 1.5552 |
Peak Time | 0.5622 | 0.5414 | 0.5430 | 0.3460 | 4.9523 | 2.2783 | 1.3415 | 0.8683 |
Step Response | HPSO{1st−Kh} | HPSO{2nd−Kh} | HPSOCFE | HPSOOus | HBFO{1st−Kh} | HBFO{2nd−Kh} | HBFOCFE | HBFOOus |
Rise Time | 0.2817 | 0.1854 | 0.2200 | 0.1387 | 0.9100 | 0.9252 | 1.6685 | 0.4199 |
Settling Time | 0.4506 | 0.3016 | 0.3499 | 0.2433 | 5.4000 | 2.5069 | 18.9348 | 3.9376 |
Settling Min. | 0.9018 | 0.9005 | 0.9022 | 0.9014 | 0.9075 | 0.8997 | 0.9006 | 0.8633 |
Settling Max. | 0.9998 | 0.9998 | 0.9996 | 0.9997 | 1.2930 | 0.9982 | 1.1533 | 1.4392 |
Overshoot | 2.4138e-004 | 0.0000 | 0.0000 | 0.0000 | 29.3215 | 0.0000 | 15.3356 | 43.9248 |
Peak | 0.9998 | 0.9998 | 0.9996 | 0.9997 | 1.2930 | 0.9982 | 1.1533 | 1.4392 |
Peak Time | 0.5620 | 0.3872 | 0.4728 | 0.3791 | 2.3246 | 4.3336 | 6.2231 | 1.1085 |
Figures 7 and 8 shows the control signals of the controlled system using the PSO and the BFO optimization algorithms for β=0.5, while Figures 9 and 10 show the control signals for β=0.9. In addition, Table 7 shows the values of the objective function (3.3) using the two optimization algorithms for β=0.5 and β=0.9. One concludes that the PSO algorithm yields a better result than that of the BFO algorithm. Even though the initial magnitude of the control signal of the PSO algorithm is larger than that of the BFO algorithm, the value of the objective function J described by (3.3) is smaller when using the PSO algorithm. This is also verified by the step responses of the controlled systems, as shown by Figures 5 and 6. It is clear that the 2nd-order El-Khazali approximation yields a smaller controller signal than the other approximations and provides a competitive steady-state error compared to its other counterparts in both algorithms and for both β=0.5 and β=0.9.
Algorithm | Type of approximation | When β=0.5 | When β=0.9 |
PSO | 1st-order El-Khazali approximation | 0.2190 | 0.2199 |
2nd-order El-Khazali approximation | 0.1247 | 0.1662 | |
Oustaloup approximation | 0.1131 | 0.0949 | |
The CFE approximation | 0.1336 | 0.1732 | |
BFO | 1st-order El-Khazali approximation | 0.8197 | 1.403 |
2nd-order El-Khazali approximation | 0.3074 | 0.4393 | |
Oustaloup approximation | 0.7388 | 0.8126 | |
The CFE approximation | 0.6341 | 0.9395 |
A new PIρDμ-controller is developed to control and improve the behavior of the Volume-Controlled Artificial Ventilation (VCAV) system. Two optimization algorithms, Bacteria Foraging Optimization (BFO) and Particle Swarm Optimization (PSO), are successfully used in conjunction with the use of four different approximations of the fractional-order integro-differential Laplacian operators, s±γ, where 0<γ<1. These approximations are the 1st- and the 2nd-order El-Khazali approximations, Oustaloup's approximation and the Continued Fractional Expansion (CFE) approximation. The PSO algorithm yields a better performance compared to that of the BFO algorithm, especially when using the 2nd-order El-Khazali approximations. It provided a significant improvement in terms of a smaller controller size, i.e., a 4th-order one, and in terms of the overall performance of the controlled system step response.
This work is supported by Ajman University grant 2020-COVID-19-08.
The authors declare no conflict of interest.
Code C1: MATLAB code for calculating the 1st-order El-Khazali approximation for s±γ, where 0<γ<1.
![]() |
Code C2: MATLAB code for calculating the 2nd-order El-Khazali approximation for s±γ, where 0<γ<1.
![]() |
Code C3: MATLAB code for calculating the Oustaloup approximation for s±γ, where 0<γ<1.
![]() |
Code C4: MATLAB code for calculating the CFE approximation for s±γ, where 0<γ<1.
![]() |
[1] |
E. Fan, J. R. Beitler, L. Brochard, C. S. Calfee, N. D. Ferguson, A. S. Slutsky, et al., COVID-19-associated acute respiratory distress syndrome: is a different approach to management warranted? Lancet Resp. Med., 8 (2020), 816–821. https://doi.org/10.1016/S2213-2600(20)30304-0 doi: 10.1016/S2213-2600(20)30304-0
![]() |
[2] |
H. D. Poor, C. E. Ventetuolo, T. Tolbert, G. Chun, G. Serrao, A. Zeidman, et al., COVID-19 critical illness pathophysiology driven by diffuse pulmonary thrombi and pulmonary endothelial dysfunction responsive to thrombolysis, Lancet Resp. Med., 10 (2020), e44. https://doi.org/10.1002/ctm2.44 doi: 10.1002/ctm2.44
![]() |
[3] |
J. Guay, E. A. Ochroch, S. Kopp, Intraoperative use of low volume ventilation to decrease postoperative mortality, mechanical ventilation, lengths of stay and lung injury in adults without acute lung injury, Cochrane Database Syst. Rev., 2018 (2018), CD011151. https://doi.org/10.1002/14651858.CD011151.pub3 doi: 10.1002/14651858.CD011151.pub3
![]() |
[4] |
T. Yoshida, S. Nakahashi, M. A. M. Nakamura, Y. Koyama, R. Roldan, V. Torsani, et al., Volume-controlled ventilation does not prevent injurious inflation during spontaneous effort, Am. J. Resp. Cri. Care, 196 (2017), 590–601. https://doi.org/10.1164/rccm.201610-1972OC doi: 10.1164/rccm.201610-1972OC
![]() |
[5] |
Y. Shi, B. Zhang, M. Cai, X. D. Zhang, Numerical simulation of volume-controlled mechanical ventilated respiratory system with 2 different lungs, Int. J. Numer. Meth. Bio., 33 (2017), e2852. https://doi.org/10.1002/cnm.2852 doi: 10.1002/cnm.2852
![]() |
[6] | S. S. Vidhya, K. Keerthana, M. Janaki, J. Kanimozhi, A survey of control algorithms used in physiological closed loop control for oxygen therapy, Int. J. Appl. Eng. Res., 14 (2019), 694–702. |
[7] |
C. Kirakli, I. Naz, O. Ediboglu, D. Tatar, A. Budak, E. Tellioglu, A randomized controlled trial comparing the ventilation duration between adaptive support ventilation and pressure assist/control ventilation in medical patients in the ICU, Chest, 147 (2015), 1503–1509. https://doi.org/10.1378/chest.14-2599 doi: 10.1378/chest.14-2599
![]() |
[8] |
S. Mesic, R. Babuska, H. C. Hoogsteden, A. F. Verbraak, Computer-controlled mechanical simulation of the artificially ventilated human respiratory system, IEEE T. Biomed. Eng., 50 (2003), 731–743. https://doi.org/10.1109/TBME.2003.812166 doi: 10.1109/TBME.2003.812166
![]() |
[9] |
M. E. Shahri, S. Balochian, H. Balochian, Y. Zhang, Design of fractional–order PID controllers for time delay systems using differential evolution, Indian J. Sci. Technol., 7 (2014), 1307–1315. https://doi.org/10.17485/ijst/2014/v7i9.28 doi: 10.17485/ijst/2014/v7i9.28
![]() |
[10] |
N. Nasirpour, S. Balochian, Optimal design of fractional-order PID controllers for multi-input multi-output (variable air volume) air-conditioning system using particle swarm optimization, Intell. Build. Int., 9 (2017), 107–119. https://doi.org/10.1080/17508975.2016.1170659 doi: 10.1080/17508975.2016.1170659
![]() |
[11] | J. Kennedy, R. Eberhart, Particle swarm optimization, In: Proceedings of ICNN'95-international conference on neural networks, 1995, 1942–1948. https://doi.org/10.1109/ICNN.1995.488968 |
[12] | K. M. Passino, Distributed optimization and control using only a germ of intelligence, In: The 2000 IEEE international symposium on intelligent control. Held jointly with the 8th IEEE Mediterranean conference on control and automation (Cat. No.00CH37147), 2000, 5–13. https://doi.org/10.1109/ISIC.2000.882888 |
[13] | S. Momani, I. M. Batiha, R. El-Khazali, Design of PIλDδ-heart rate controllers for cardiac pacemaker, In: 2019 IEEE international symposium on signal processing and information technology (ISSPIT), 2019. https://doi.org/10.1109/ISSPIT47144.2019.9001785 |
[14] | I. M. Batiha, R. El-Khazali, S. Momani, Dynamic responses of PIλ, PDδ, and PIλDδ controllers for prosthetic hand model using PSO algorithm, In: 2019 IEEE international symposium on signal processing and information technology (ISSPIT), 2019. https://doi.org/10.1109/ISSPIT47144.2019.9001835 |
[15] |
I. M. Batiha, S. A. Njadat, R. M. Batyha, A. Zraiqat, A. Dababneh, S. Momani, Design fractional-order PID controllers for single-joint robot arm model, Int. J. Advance Soft Compu. Appl., 14 (2022), 96–114. https://doi.org/10.15849/IJASCA.220720.07 doi: 10.15849/IJASCA.220720.07
![]() |
[16] |
S. Momani, I. M. Batiha, Tuning of the fractional-order PID controller for some real-life industrial processes using particle swarm optimization, Prog. Fract. Differ. Appl., 8 (2022), 377–391. https://doi.org/10.18576/pfda/080303 doi: 10.18576/pfda/080303
![]() |
[17] |
R. El-Khazali, I. M. Batiha, S. Momani, The drug administration via fractional-order PIλDδ-controller, Prog. Fract. Differ. Appl., 8 (2022), 53–62. https://doi.org/10.18576/pfda/080103 doi: 10.18576/pfda/080103
![]() |
[18] | I. M. Batiha, J. Oudetallah, A. Ouannas, A. A. Al-Nana, I. H. Jebril, Tuning the fractional-order PID-controller for blood glucose level of diabetic patients, Int. J. Advance Soft Compu. Appl., 13 (2021), 1–10. |
[19] | A. K. Gil'mutdinov, P. A. Ushakov, R. El-Khazali, Fractal elements and their applications, Switzerland: Springer International Publishing, 2017. https://doi.org/10.1007/978-3-319-45249-4 |
[20] | R. El-Khazali, I. M. Batiha, S. Momani, Approximation of fractional-order operators, In: Fractional calculus, Singapore: Springer, 2019,121–151. https://doi.org/10.1007/978-981-15-0430-3_8 |
[21] |
B. T. Krishna, Studies on fractional order differentiators and integrators: A survey, Signal Process., 91 (2011), 386–426. https://doi.org/10.1016/j.sigpro.2010.06.022 doi: 10.1016/j.sigpro.2010.06.022
![]() |
[22] | R. El-Khazali, Fractional-order LCαL filter-based grid connected PV systems, In: 2019 IEEE 62nd international midwest symposium on circuits and systems (MWSCAS), 2019,533–536. https://doi.org/10.1109/MWSCAS.2019.8885133 |
[23] |
R. El-Khazali, On the biquadratic approximation of fractional-order Laplacian operators, Analog Integr. Circ. Sig. Procss., 82 (2015), 503–517. https://doi.org/10.1007/s10470-014-0432-8 doi: 10.1007/s10470-014-0432-8
![]() |
[24] | I. Podlubny, Fractional-order systems and PIλDμ-controllers, In: IEEE transactions on automatic control, 1999,208–214. https://doi.org/10.1109/9.739144 |
[25] |
I. M. Batiha, R. El-Khazali, A. AlSaedi, S. Momani, The general solution of singular fractional-order linear time-invariant continuous systems with regular pencils, Entropy, 20 (2018), 400. https://doi.org/10.3390/e20060400 doi: 10.3390/e20060400
![]() |
[26] |
R. El-Khazali, Fractional-order PIλDμ controller design, Comput. Math. Appl., 66 (2013), 639–646. https://doi.org/10.1016/j.camwa.2013.02.015 doi: 10.1016/j.camwa.2013.02.015
![]() |
[27] |
S. Momani, R. El-Khazali, I. M. Batiha, Tuning PID and PIλDδ controllers using particle swarm optimization algorithm via El-Khazali's approach, AIP Conf. Proc., 2172 (2019), 050003. https://doi.org/10.1063/1.5133522 doi: 10.1063/1.5133522
![]() |
[28] | I. Podlubny, L. Dorcak, I. Kostial, On fractional derivatives, fractional-order dynamic systems and PIλDμ -controllers, In: Proceedings of the 36th IEEE conference on decision and control, 1997, 4985–4990. https://doi.org/10.1109/CDC.1997.649841 |
[29] |
T. Hamadneh, I. Abu-Falahah, M. AlQudah, Numerical optimization and positivity certificates for polynomials and rationals over simplices, J. Comput. Appl. Math., 414 (2022), 114430. https://doi.org/10.1016/j.cam.2022.114430 doi: 10.1016/j.cam.2022.114430
![]() |
[30] | W. Lin, Z. Chongquan, Design of optimal fractional-order PID controllers using particle swarm optimization algorithm for DC motor system, In: 2015 IEEE advanced information technology, electronic and automation control conference (IAEAC), 2015,175–179. https://doi.org/10.1109/IAEAC.2015.7428542 |
[31] | S. Das, A. Biswas, S. Dasgupta, A. Abraham, Bacterial foraging optimization algorithm: Theoretical foundations, analysis, and applications, In: Foundations of computational intelligence, Heidelberg: Springer, 2009, 23–55. https://doi.org/10.1007/978-3-642-01085-9_2 |
[32] | M. Nasri, H. Nezamabadi-pour, M. Maghfoori, A PSO-based optimum design of PID controller for a linear brushless DC motor, Proceedings of World Academy of Science, Engineering and Technology, 20 (2007), 211–215. |
[33] | B. D. Halilu, E. C. Anene, E. E. Omigzegba, L. Maijama'a, S. A. Baraza, Optimization of PID controller gains for identified magnetic levitation plant using bacteria foraging algorithm, Int. J. Eng. Modern Technol., 5 (2019), 12–18. |
[34] | M. A. Muñoz, S. K. Halgamuge, W. Alfonso, E. F. Caicedo, Simplifying the bacteria foraging optimization algorithm, In: IEEE congress on evolutionary computation, 2010, 1–7. https://doi.org/10.1109/CEC.2010.5586025 |
[35] | K. E. Dagher, Design of an auto-tuning PID controller for systems based on slice genetic algorithm, IJCCCE, 13 (2013), 1–9. |
[36] |
A. Oustaloup, F. Levron, B. Mathieu, F. M. Nanot, Frequency band complex noninteger differentiator: characterization and synthesis, IEEE T. Circuits Syst. I, 47 (2000), 25–39. https://doi.org/10.1109/81.817385 doi: 10.1109/81.817385
![]() |
[37] | I. Dimeas, Design of an integrated fractional-order controller, Patras: University of Patras, 2017. |
[38] | A. T. Yimchunger, D. Acharya, D. K. Das, Particle swarm optimization based PID-controller design for volume control of artificial ventilation system, In: 2020 IEEE calcutta conference (CALCON), 2020,278–282. https://doi.org/10.1109/CALCON49167.2020.9106480 |
[39] | M. Walter, S. Leonhardt, Control applications in artificial ventilation, In: 2007 Mediterranean conference on control & automation, 2007, 1–6. https://doi.org/10.1109/MED.2007.4433762 |
1. | Iqbal M. Batiha, Osama Y. Ababneh, Abeer A. Al-Nana, Waseem G. Alshanti, Shameseddin Alshorm, Shaher Momani, A Numerical Implementation of Fractional-Order PID Controllers for Autonomous Vehicles, 2023, 12, 2075-1680, 306, 10.3390/axioms12030306 | |
2. | Manal Almuzini, Iqbal M. Batiha, Shaher Momani, 2023, A study of fractional-order monkeypox mathematical model with its stability analysis, 979-8-3503-2168-5, 1, 10.1109/ICFDA58234.2023.10153214 | |
3. | Iqbal M. Batiha, Shameseddin Alshorm, Abdallah Al-Husban, Rania Saadeh, Gharib Gharib, Shaher Momani, The n-Point Composite Fractional Formula for Approximating Riemann–Liouville Integrator, 2023, 15, 2073-8994, 938, 10.3390/sym15040938 | |
4. | Amir Veisi, Hamoun Maleki, Hadi Delavari, 2023, Adaptive Fractional Sliding Mode Controller for Controlling Airway Pressure in an Artificial Ventilation System, 979-8-3503-6601-3, 1, 10.1109/ICCIA61416.2023.10506394 |
Algorithm | Type of approximation | κp | κi | κd | ρ | μ |
PSO | 1st-order El-Khazali | 31.4119 | 0.54000 | 25.4162 | 0.31300 | 0.90600 |
2nd-order El-Khazali | 41.0000 | 15.4404 | 45.0000 | 0.09000 | 0.91100 | |
Oustaloup | 59.0000 | 0.76000 | 61.0000 | 0.82100 | 0.92503 | |
CFE | 0.16000 | 45.5900 | 51.0000 | 0.17700 | 0.86700 | |
BFO | 1st-order El-Khazali | 2.71690 | 2.50680 | 7.71930 | 0.91510 | 0.57810 |
2nd-order El-Khazali | 20.8839 | 12.9392 | 21.7497 | 0.86760 | 0.90610 | |
Oustaloup | 25.4065 | 18.5733 | 32.2031 | 0.54090 | 0.15850 | |
CFE | 2.56650 | 10.0268 | 15.2471 | 0.18520 | 0.33260 |
Algorithm | Type of approximation | κp | κi | κd | ρ | μ |
PSO | 1st-order El-Khazali | 31.6262 | 0.5400 | 25.5702 | 0.9030 | 0.9060 |
2nd-order El-Khazali | 41.000 | 0.3200 | 45.000 | 0.7990 | 0.8557 | |
Oustaloup | 59.000 | 24.5393 | 61.0000 | 0.1650 | 0.9760 | |
CFE | 41.5691 | 0.3100 | 51.000 | 0.1770 | 0.8670 | |
BFO | 1st-order El-Khazali | 4.5230 | 4.3728 | 6.7855 | 0.8750 | 0.2870 |
2nd-order El-Khazali | 13.7804 | 2.0452 | 16.5894 | 0.2662 | 0.9468 | |
Oustaloup | 17.5025 | 11.2505 | 21.6484 | 0.8129 | 0.2091 | |
CFE | 2.2440 | 2.1768 | 8.1292 | 0.9151 | 0.5781 |
Algorithm | Type of approximation | Controller transfer function, C(s) |
PSO | 1st-order El-Khazali approximation | C{1st−Kh}(s)=626.9s2+1135s+462.31.67s2+23.58s+13.52 |
2nd-order El-Khazali approximation | C{2nd−Kh}(s)=344.4s4+1705s3+2852s2+1810s+3710.1917s4+8.814s3+28.9s2+25.83s+6.079 | |
Oustaloup approximation | COus(s)=1.92e05s10+8.374e06s9+1.191e08s8+6.29e08s7+1.468e09s6+1.569e09s5 +7.846e08s4+1.793e08s3+1.675e07s2+6.184e05s+659943.85s10+5837s9+1.847e05s8+2.14e06s7+9.029e06s6+1.54e07s5 +9.936e06s4+2.592e06s3+2.463e05s2+8567s+70.81 | |
The CFE approximation | CCFE(s)=1.637e04s10+5.268e05s9+5.684e06s8+2.671e07s7+6.385e07s6+8.343e07s5 +6.143e07s4+2.489e07s3+5.182e06s2+4.744e05s+1.467e042.254s10+543.4s9+1.462e04s8+1.312e05s7+5.014e05s6+9.102e05s5 +8.165e05s4+3.595e05s3+7.334e04s2+6038s+142 | |
BFO | 1st-order El-Khazali approximation | C{1st−Kh}(s)=379.1s2+303.8s+124.714.97s2+44.52s+2.907 |
2nd-order El-Khazali approximation | C{2nd−Kh}(s)=301.3s4+1018s3+1314s2+772.4s+186.40.3702s4+15.65s3+31.49s2+16.57s+0.555 | |
Oustaloup approximation | COus(s)=1132s10+8.422e04s9+1.968e06s8+1.638e07s7+5.564e07s6+7.554e07s5 +4.426e07s4+1.068e07s3+1.106e06s2+4.292e04s+550.112.07s10+1008s9+2.53e04s8+2.234e05s7+7.698e05s6+1.02e06s5 +5.413e05s4+1.104e05s3+8797s2+246.4s+2.075 | |
The CFE approximation | CCFE(s)=184.2s10+7443s9+1.039e05s8+5.885e05s7+1.588e06s6+2.15e06s5 +1.499e06s4+5.243e05s3+8.724e04s2+5874s+137.92.342s10+141.1s9+2552s8+1.726e04s7+5.29e04s6+7.875e04s5 +5.864e04s4+2.135e04s3+3575s2+232.4s+4.71 |
Algorithm | Type of approximation | Controller transfer function, C(s) |
PSO | 1st-order El-Khazali approximation | C{1st−Kh}(s)=4944s2+6329s+548.813.1s2+178.1s+13.52 |
2nd-order El-Khazali approximation | C{2nd−Kh}(s)=577.3s4+2012s3+2207s2+778.2s+40.670.5624s4+15.49s3+31.31s2+16.83s+0.8531 | |
Oustaloup approximation | COus(s)=1.529e06s10+7.401e08s9+9.569e10s8+1.819e12s7+1.058e13s6+1.81e13s5 +1.14e13s4+1.881e12s3+8.537e10s2+5.842e08s+1.088e063.126s10+3.135e04s9+1.393e07s8+1.702e09s7+2.953e10s6+1.378e11s5 +1.149e11s4+1.94e10s3+7.952e08s2+4.859e06s+8017 | |
The CFE approximation | CCFE(s)=9.315e04s10+2.947e06s9+3.076e07s8+1.401e08s7+3.212e08s6+3.971e08s5 +2.709e08s4+9.988e07s3+1.848e07s2+1.468e06s+3.426e042.254s10+2084s9+6.151e04s8+5.866e05s7+2.343e06s6+4.418e06s5 +4.103e06s4+1.87e06s3+3.946e05s2+3.376e04s+809.4 | |
BFO | 1st-order El-Khazali approximation | C{1st−Kh}(s)=134.8s2+187.8s+66.7810.15s2+13.45s+1.226 |
2nd-order El-Khazali approximation | C{2nd−Kh}(s)=144.4s4+613.8s3+875.3s2+475.1s+80.650.1251s4+9.486s3+29.32s2+24.11s+4.499 | |
Oustaloup approximation | COus(s)=4.56e04s10+6.061e07s9+6.876e09s8+1.95e11s7+1.091e12s6+1.394e12s5 +6.238e11s4+7.936e10s3+2.854e09s2+2.559e07s+2.134e04274.6s10+6.801e05s9+9.577e07s8+3.703e09s7+2.237e10s6+3.132e10s5 +9.348e09s4+4.765e08s3+6.737e06s2+1.324e04s+6.861 | |
The CFE approximation | CCFE(s)=3.669e04s10+9.368e05s9+8.288e06s8+3.18e07s7+6.075e07s6+6.215e07s5 +3.635e07s4+1.26e07s3+2.545e06s2+2.556e05s+9712262.6s10+2.016e04s9+3.028e05s8+1.752e06s7+4.556e06s6+5.762e06s5 +3.585e06s4+1.068e06s3+1.365e05s2+6128s+16.91 |
Step Response | HPSO{1st−Kh} | HPSO{2nd−Kh} | HPSOCFE | HPSOOus | HBFO{1st−Kh} | HBFO{2nd−Kh} | HBFOCFE | HBFOOus |
Rise Time | 0.2829 | 0.1858 | 0.2333 | 0.1516 | 1.3512 | 0.4828 | 0.5938 | 0.3225 |
Settling Time | 0.4526 | 0.3260 | 0.3783 | 0.2514 | 14.6911 | 5.2044 | 3.3122 | 4.7305 |
Settling Min. | 0.9001 | 0.9010 | 0.9012 | 0.9008 | 0.9017 | 0.9000 | 0.9079 | 0.7189 |
Settling Max. | 0.9997 | 0.9998 | 0.9999 | 0.9994 | 1.1660 | 1.0741 | 1.2092 | 1.5552 |
Overshoot | 5.2705e-04 | 0.0000 | 0.0000 | 0.0000 | 16.6236 | 7.4173 | 20.9616 | 55.5270 |
Peak | 0.9997 | 0.9998 | 0.9999 | 0.9994 | 1.1660 | 1.0741 | 1.2092 | 1.5552 |
Peak Time | 0.5622 | 0.5414 | 0.5430 | 0.3460 | 4.9523 | 2.2783 | 1.3415 | 0.8683 |
Step Response | HPSO{1st−Kh} | HPSO{2nd−Kh} | HPSOCFE | HPSOOus | HBFO{1st−Kh} | HBFO{2nd−Kh} | HBFOCFE | HBFOOus |
Rise Time | 0.2817 | 0.1854 | 0.2200 | 0.1387 | 0.9100 | 0.9252 | 1.6685 | 0.4199 |
Settling Time | 0.4506 | 0.3016 | 0.3499 | 0.2433 | 5.4000 | 2.5069 | 18.9348 | 3.9376 |
Settling Min. | 0.9018 | 0.9005 | 0.9022 | 0.9014 | 0.9075 | 0.8997 | 0.9006 | 0.8633 |
Settling Max. | 0.9998 | 0.9998 | 0.9996 | 0.9997 | 1.2930 | 0.9982 | 1.1533 | 1.4392 |
Overshoot | 2.4138e-004 | 0.0000 | 0.0000 | 0.0000 | 29.3215 | 0.0000 | 15.3356 | 43.9248 |
Peak | 0.9998 | 0.9998 | 0.9996 | 0.9997 | 1.2930 | 0.9982 | 1.1533 | 1.4392 |
Peak Time | 0.5620 | 0.3872 | 0.4728 | 0.3791 | 2.3246 | 4.3336 | 6.2231 | 1.1085 |
Algorithm | Type of approximation | When β=0.5 | When β=0.9 |
PSO | 1st-order El-Khazali approximation | 0.2190 | 0.2199 |
2nd-order El-Khazali approximation | 0.1247 | 0.1662 | |
Oustaloup approximation | 0.1131 | 0.0949 | |
The CFE approximation | 0.1336 | 0.1732 | |
BFO | 1st-order El-Khazali approximation | 0.8197 | 1.403 |
2nd-order El-Khazali approximation | 0.3074 | 0.4393 | |
Oustaloup approximation | 0.7388 | 0.8126 | |
The CFE approximation | 0.6341 | 0.9395 |
Algorithm | Type of approximation | κp | κi | κd | ρ | μ |
PSO | 1st-order El-Khazali | 31.4119 | 0.54000 | 25.4162 | 0.31300 | 0.90600 |
2nd-order El-Khazali | 41.0000 | 15.4404 | 45.0000 | 0.09000 | 0.91100 | |
Oustaloup | 59.0000 | 0.76000 | 61.0000 | 0.82100 | 0.92503 | |
CFE | 0.16000 | 45.5900 | 51.0000 | 0.17700 | 0.86700 | |
BFO | 1st-order El-Khazali | 2.71690 | 2.50680 | 7.71930 | 0.91510 | 0.57810 |
2nd-order El-Khazali | 20.8839 | 12.9392 | 21.7497 | 0.86760 | 0.90610 | |
Oustaloup | 25.4065 | 18.5733 | 32.2031 | 0.54090 | 0.15850 | |
CFE | 2.56650 | 10.0268 | 15.2471 | 0.18520 | 0.33260 |
Algorithm | Type of approximation | κp | κi | κd | ρ | μ |
PSO | 1st-order El-Khazali | 31.6262 | 0.5400 | 25.5702 | 0.9030 | 0.9060 |
2nd-order El-Khazali | 41.000 | 0.3200 | 45.000 | 0.7990 | 0.8557 | |
Oustaloup | 59.000 | 24.5393 | 61.0000 | 0.1650 | 0.9760 | |
CFE | 41.5691 | 0.3100 | 51.000 | 0.1770 | 0.8670 | |
BFO | 1st-order El-Khazali | 4.5230 | 4.3728 | 6.7855 | 0.8750 | 0.2870 |
2nd-order El-Khazali | 13.7804 | 2.0452 | 16.5894 | 0.2662 | 0.9468 | |
Oustaloup | 17.5025 | 11.2505 | 21.6484 | 0.8129 | 0.2091 | |
CFE | 2.2440 | 2.1768 | 8.1292 | 0.9151 | 0.5781 |
Algorithm | Type of approximation | Controller transfer function, C(s) |
PSO | 1st-order El-Khazali approximation | C{1st−Kh}(s)=626.9s2+1135s+462.31.67s2+23.58s+13.52 |
2nd-order El-Khazali approximation | C{2nd−Kh}(s)=344.4s4+1705s3+2852s2+1810s+3710.1917s4+8.814s3+28.9s2+25.83s+6.079 | |
Oustaloup approximation | COus(s)=1.92e05s10+8.374e06s9+1.191e08s8+6.29e08s7+1.468e09s6+1.569e09s5 +7.846e08s4+1.793e08s3+1.675e07s2+6.184e05s+659943.85s10+5837s9+1.847e05s8+2.14e06s7+9.029e06s6+1.54e07s5 +9.936e06s4+2.592e06s3+2.463e05s2+8567s+70.81 | |
The CFE approximation | CCFE(s)=1.637e04s10+5.268e05s9+5.684e06s8+2.671e07s7+6.385e07s6+8.343e07s5 +6.143e07s4+2.489e07s3+5.182e06s2+4.744e05s+1.467e042.254s10+543.4s9+1.462e04s8+1.312e05s7+5.014e05s6+9.102e05s5 +8.165e05s4+3.595e05s3+7.334e04s2+6038s+142 | |
BFO | 1st-order El-Khazali approximation | C{1st−Kh}(s)=379.1s2+303.8s+124.714.97s2+44.52s+2.907 |
2nd-order El-Khazali approximation | C{2nd−Kh}(s)=301.3s4+1018s3+1314s2+772.4s+186.40.3702s4+15.65s3+31.49s2+16.57s+0.555 | |
Oustaloup approximation | COus(s)=1132s10+8.422e04s9+1.968e06s8+1.638e07s7+5.564e07s6+7.554e07s5 +4.426e07s4+1.068e07s3+1.106e06s2+4.292e04s+550.112.07s10+1008s9+2.53e04s8+2.234e05s7+7.698e05s6+1.02e06s5 +5.413e05s4+1.104e05s3+8797s2+246.4s+2.075 | |
The CFE approximation | CCFE(s)=184.2s10+7443s9+1.039e05s8+5.885e05s7+1.588e06s6+2.15e06s5 +1.499e06s4+5.243e05s3+8.724e04s2+5874s+137.92.342s10+141.1s9+2552s8+1.726e04s7+5.29e04s6+7.875e04s5 +5.864e04s4+2.135e04s3+3575s2+232.4s+4.71 |
Algorithm | Type of approximation | Controller transfer function, C(s) |
PSO | 1st-order El-Khazali approximation | C{1st−Kh}(s)=4944s2+6329s+548.813.1s2+178.1s+13.52 |
2nd-order El-Khazali approximation | C{2nd−Kh}(s)=577.3s4+2012s3+2207s2+778.2s+40.670.5624s4+15.49s3+31.31s2+16.83s+0.8531 | |
Oustaloup approximation | COus(s)=1.529e06s10+7.401e08s9+9.569e10s8+1.819e12s7+1.058e13s6+1.81e13s5 +1.14e13s4+1.881e12s3+8.537e10s2+5.842e08s+1.088e063.126s10+3.135e04s9+1.393e07s8+1.702e09s7+2.953e10s6+1.378e11s5 +1.149e11s4+1.94e10s3+7.952e08s2+4.859e06s+8017 | |
The CFE approximation | CCFE(s)=9.315e04s10+2.947e06s9+3.076e07s8+1.401e08s7+3.212e08s6+3.971e08s5 +2.709e08s4+9.988e07s3+1.848e07s2+1.468e06s+3.426e042.254s10+2084s9+6.151e04s8+5.866e05s7+2.343e06s6+4.418e06s5 +4.103e06s4+1.87e06s3+3.946e05s2+3.376e04s+809.4 | |
BFO | 1st-order El-Khazali approximation | C{1st−Kh}(s)=134.8s2+187.8s+66.7810.15s2+13.45s+1.226 |
2nd-order El-Khazali approximation | C{2nd−Kh}(s)=144.4s4+613.8s3+875.3s2+475.1s+80.650.1251s4+9.486s3+29.32s2+24.11s+4.499 | |
Oustaloup approximation | COus(s)=4.56e04s10+6.061e07s9+6.876e09s8+1.95e11s7+1.091e12s6+1.394e12s5 +6.238e11s4+7.936e10s3+2.854e09s2+2.559e07s+2.134e04274.6s10+6.801e05s9+9.577e07s8+3.703e09s7+2.237e10s6+3.132e10s5 +9.348e09s4+4.765e08s3+6.737e06s2+1.324e04s+6.861 | |
The CFE approximation | CCFE(s)=3.669e04s10+9.368e05s9+8.288e06s8+3.18e07s7+6.075e07s6+6.215e07s5 +3.635e07s4+1.26e07s3+2.545e06s2+2.556e05s+9712262.6s10+2.016e04s9+3.028e05s8+1.752e06s7+4.556e06s6+5.762e06s5 +3.585e06s4+1.068e06s3+1.365e05s2+6128s+16.91 |
Step Response | HPSO{1st−Kh} | HPSO{2nd−Kh} | HPSOCFE | HPSOOus | HBFO{1st−Kh} | HBFO{2nd−Kh} | HBFOCFE | HBFOOus |
Rise Time | 0.2829 | 0.1858 | 0.2333 | 0.1516 | 1.3512 | 0.4828 | 0.5938 | 0.3225 |
Settling Time | 0.4526 | 0.3260 | 0.3783 | 0.2514 | 14.6911 | 5.2044 | 3.3122 | 4.7305 |
Settling Min. | 0.9001 | 0.9010 | 0.9012 | 0.9008 | 0.9017 | 0.9000 | 0.9079 | 0.7189 |
Settling Max. | 0.9997 | 0.9998 | 0.9999 | 0.9994 | 1.1660 | 1.0741 | 1.2092 | 1.5552 |
Overshoot | 5.2705e-04 | 0.0000 | 0.0000 | 0.0000 | 16.6236 | 7.4173 | 20.9616 | 55.5270 |
Peak | 0.9997 | 0.9998 | 0.9999 | 0.9994 | 1.1660 | 1.0741 | 1.2092 | 1.5552 |
Peak Time | 0.5622 | 0.5414 | 0.5430 | 0.3460 | 4.9523 | 2.2783 | 1.3415 | 0.8683 |
Step Response | HPSO{1st−Kh} | HPSO{2nd−Kh} | HPSOCFE | HPSOOus | HBFO{1st−Kh} | HBFO{2nd−Kh} | HBFOCFE | HBFOOus |
Rise Time | 0.2817 | 0.1854 | 0.2200 | 0.1387 | 0.9100 | 0.9252 | 1.6685 | 0.4199 |
Settling Time | 0.4506 | 0.3016 | 0.3499 | 0.2433 | 5.4000 | 2.5069 | 18.9348 | 3.9376 |
Settling Min. | 0.9018 | 0.9005 | 0.9022 | 0.9014 | 0.9075 | 0.8997 | 0.9006 | 0.8633 |
Settling Max. | 0.9998 | 0.9998 | 0.9996 | 0.9997 | 1.2930 | 0.9982 | 1.1533 | 1.4392 |
Overshoot | 2.4138e-004 | 0.0000 | 0.0000 | 0.0000 | 29.3215 | 0.0000 | 15.3356 | 43.9248 |
Peak | 0.9998 | 0.9998 | 0.9996 | 0.9997 | 1.2930 | 0.9982 | 1.1533 | 1.4392 |
Peak Time | 0.5620 | 0.3872 | 0.4728 | 0.3791 | 2.3246 | 4.3336 | 6.2231 | 1.1085 |
Algorithm | Type of approximation | When β=0.5 | When β=0.9 |
PSO | 1st-order El-Khazali approximation | 0.2190 | 0.2199 |
2nd-order El-Khazali approximation | 0.1247 | 0.1662 | |
Oustaloup approximation | 0.1131 | 0.0949 | |
The CFE approximation | 0.1336 | 0.1732 | |
BFO | 1st-order El-Khazali approximation | 0.8197 | 1.403 |
2nd-order El-Khazali approximation | 0.3074 | 0.4393 | |
Oustaloup approximation | 0.7388 | 0.8126 | |
The CFE approximation | 0.6341 | 0.9395 |