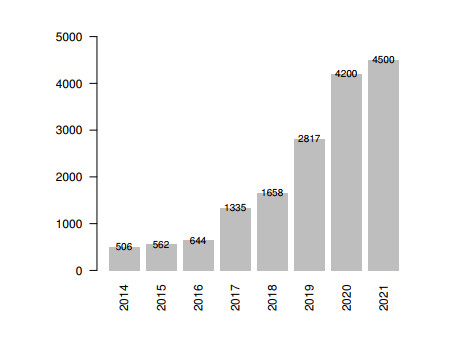
Cryptocurrency is a digital currency and also exists in the form of coins. It has turned out as a leading method for peer-to-peer online cash systems. Due to the importance and increasing influence of Bitcoin on business and other related sectors, it is very crucial to model or predict its behavior. Therefore, in recent, numerous researchers have attempted to understand and model the behaviors of cryptocurrency exchange rates. In the practice of actuarial and financial studies, heavy-tailed distributions play a fruitful role in modeling and describing the log returns of financial phenomena. In this paper, we propose a new family of distributions that possess heavy-tailed characteristics. Based on the proposed approach, a modified version of the logistic distribution, namely, a new modified exponential-logistic distribution is introduced. To illustrate the new modified exponential-logistic model, two financial data sets are analyzed. The first data set represents the log-returns of the Bitcoin exchange rates. Whereas, the second data set represents the log-returns of the Ethereum exchange rates. Furthermore, to forecast the high volatile behavior of the same datasets, we apply dual machine learning algorithms, namely Artificial neural network and support vector regression. The effectiveness of these models is evaluated against self exciting threshold autoregressive model.
Citation: Zubair Ahmad, Zahra Almaspoor, Faridoon Khan, Sharifah E. Alhazmi, M. El-Morshedy, O. Y. Ababneh, Amer Ibrahim Al-Omari. On fitting and forecasting the log-returns of cryptocurrency exchange rates using a new logistic model and machine learning algorithms[J]. AIMS Mathematics, 2022, 7(10): 18031-18049. doi: 10.3934/math.2022993
[1] | Naif Alotaibi, A. S. Al-Moisheer, Ibrahim Elbatal, Salem A. Alyami, Ahmed M. Gemeay, Ehab M. Almetwally . Bivariate step-stress accelerated life test for a new three-parameter model under progressive censored schemes with application in medical. AIMS Mathematics, 2024, 9(2): 3521-3558. doi: 10.3934/math.2024173 |
[2] | Huda M. Alshanbari, Zubair Ahmad, Faridoon Khan, Saima K. Khosa, Muhammad Ilyas, Abd Al-Aziz Hosni El-Bagoury . Univariate and multivariate analyses of the asset returns using new statistical models and penalized regression techniques. AIMS Mathematics, 2023, 8(8): 19477-19503. doi: 10.3934/math.2023994 |
[3] | Sajid Mehboob Zaidi, Mashail M. AL Sobhi, M. El-Morshedy, Ahmed Z. Afify . A new generalized family of distributions: Properties and applications. AIMS Mathematics, 2021, 6(1): 456-476. doi: 10.3934/math.2021028 |
[4] | Fiaz Ahmad Bhatti, G. G. Hamedani, Mashail M. Al Sobhi, Mustafa Ç. Korkmaz . On the Burr XII-Power Cauchy distribution: Properties and applications. AIMS Mathematics, 2021, 6(7): 7070-7092. doi: 10.3934/math.2021415 |
[5] | Gil Cohen . Intraday trading of cryptocurrencies using polynomial auto regression. AIMS Mathematics, 2023, 8(4): 9782-9794. doi: 10.3934/math.2023493 |
[6] | Alanazi Talal Abdulrahman, Khudhayr A. Rashedi, Tariq S. Alshammari, Eslam Hussam, Amirah Saeed Alharthi, Ramlah H Albayyat . A new extension of the Rayleigh distribution: Methodology, classical, and Bayes estimation, with application to industrial data. AIMS Mathematics, 2025, 10(2): 3710-3733. doi: 10.3934/math.2025172 |
[7] | Melina Melina, Sukono, Herlina Napitupulu, Norizan Mohamed . Investment risk forecasting model using extreme value theory approach combined with machine learning. AIMS Mathematics, 2024, 9(11): 33314-33352. doi: 10.3934/math.20241590 |
[8] | Hanan Haj Ahmad, Kariema A. Elnagar . A novel quantile regression for fractiles based on unit logistic exponential distribution. AIMS Mathematics, 2024, 9(12): 34504-34536. doi: 10.3934/math.20241644 |
[9] | Remigijus Leipus, Jonas Šiaulys, Dimitrios Konstantinides . Minimum of heavy-tailed random variables is not heavy tailed. AIMS Mathematics, 2023, 8(6): 13066-13072. doi: 10.3934/math.2023658 |
[10] | Aisha Fayomi, Ehab M. Almetwally, Maha E. Qura . A novel bivariate Lomax-G family of distributions: Properties, inference, and applications to environmental, medical, and computer science data. AIMS Mathematics, 2023, 8(8): 17539-17584. doi: 10.3934/math.2023896 |
Cryptocurrency is a digital currency and also exists in the form of coins. It has turned out as a leading method for peer-to-peer online cash systems. Due to the importance and increasing influence of Bitcoin on business and other related sectors, it is very crucial to model or predict its behavior. Therefore, in recent, numerous researchers have attempted to understand and model the behaviors of cryptocurrency exchange rates. In the practice of actuarial and financial studies, heavy-tailed distributions play a fruitful role in modeling and describing the log returns of financial phenomena. In this paper, we propose a new family of distributions that possess heavy-tailed characteristics. Based on the proposed approach, a modified version of the logistic distribution, namely, a new modified exponential-logistic distribution is introduced. To illustrate the new modified exponential-logistic model, two financial data sets are analyzed. The first data set represents the log-returns of the Bitcoin exchange rates. Whereas, the second data set represents the log-returns of the Ethereum exchange rates. Furthermore, to forecast the high volatile behavior of the same datasets, we apply dual machine learning algorithms, namely Artificial neural network and support vector regression. The effectiveness of these models is evaluated against self exciting threshold autoregressive model.
In recent times, cryptocurrency and blockchain technology has gained the ability to revolutionize economic phenomena worldwide. Over the past couple of years, a new fashion to make payments via cryptocurrencies has arisen. It allows money/asset transfer across the globe without consulting a centralized third party; see Phillip et al. [1], and Alzaatreh and Sulieman [2]. Since 2009, after the introduction of Bitcoin into the financial market, numerous cryptocurrencies have been introduced. Since 2014, around 4500 cryptocurrencies have been launched worldwide. Figure 1 shows the number of cryptocurrencies from 2014 to the present.
Due to the increasing interest of investors in Bitcoin, it has attracted considerable attention in recent years. There is a vast literature on Bitcoin, for example, (i) Ciaian et al. [3] implemented a regression approach for predicting Bitcoin prices, (ii) Núñez et al. [4] performed a statistical analysis of Bitcoin, (iii) Punzo and Bagnato [5] modeled the cryptocurrency return data by implementing the Laplace scale mixtures, (iv) Ibrahim et al. [6] used different time series models for predicting the direction moment of Bitcoin, (v) Hachicha and Hachicha [7] performed a comparative study of stochastic volatility and MCMC (Markov Chain Monte-Carlo) algorithm using the Bitcoin stock market indexes, and (vi) Livieris et al. [8] used a machine learning approach for cryptocurrency forecasting. For more studies related to Bitcoin and other cryptocurrencies, we refer to Chkili et al. [9], Cebrián-Hernández et al. [10], Bazán-Palomino [11], Qin et al. [12], Ghabri et al. [13], Yan [14], Mahdi et al. [15], Liu et al. [16], Naimy et al. [17], and Umar et al. [18].
In this paper, we propose a new method for introducing new HT (heavy-tailed) distributions. The proposed method can be implemented for modeling the HT financial and other related data sets. For case studies, we analyze two data sets related to the log-returns of Bitcoin and Ethereum ERs (exchange rates) based on the USA (United States of America) dollars.
Now, we introduce a new modfied exponential-X (for short "NME-X") family of distributions. The NME-X is introduced by combining the exponential model having PDF (probability density function) k(t)=e−t with the T-X family method.
Consider a random variable, say T, with PDF k(t), where T∈[π1,π2] for −∞≤π1<π2≤∞. Let X be a random variable with CDF (cumulative distribution function) expressed by W(x;ΔΔ) depending on the parameter vector ΔΔ. Let suppose that F[W(x;ΔΔ)] be a function of CDF of X, satisfying the following conditions
(i) F[W(x;ΔΔ)]∈[π1,π2],
(ii) F[W(x;ΔΔ)] is differentiable and monotonically increasing,
(iii) F[W(x;ΔΔ)]→π1 as x→−∞ and F[W(x;ΔΔ)]→π2 as x→∞.
Then, according to Alzaatreh et al. [19], the CDF of the T-X distributions approach is defined by
M(x;ΔΔ)=∫F[W(x;ΔΔ)]π1k(t)dt,x∈R, | (1.1) |
where F[W(x;ΔΔ)] satisfies the above conditions presented in (i)–(iii). Corresponding to Eq (1.1), the PDF of the T-X distributions is given by
m(x;ΔΔ)={ddxF[W(x;ΔΔ)]}k{F[W(x;ΔΔ)]},x∈R. |
Now, using k(t)=e−t and replacing F[W(x;ΔΔ)]=β2[1−W(x;ΔΔ)][β+W(x;ΔΔ)]2 in Eq (1.1), we get the CDF of the NME-X family, given by
M(x;β,ΔΔ)=1−β2ˉW(x;ΔΔ)[β+W(x;ΔΔ)]2,β>0,x∈R, | (1.2) |
where, ˉW(x;ΔΔ)=1−W(x;ΔΔ) is the SF (survival function) of the baseline model.
Based on our study of the literature, the new method defined in Eq (1.2) has not been proposed/used so far. This is one of the key motivations of this work. Henceforth, using the proposed method numerous new distributions can also be introduced for data modeling in different sectors. The PDF m(x;β,ΔΔ) corresponding to Eq (1.2) is given by
m(x;β,ΔΔ)=β2w(x;ΔΔ)[β+W(x;ΔΔ)]3[β+2−W(x;ΔΔ)],β>0,x∈R, | (1.3) |
where ddxW(x;ΔΔ)=w(x;ΔΔ).
Corresponding to Eq (1.2) and (1.3), the SF S(x;β,ΔΔ)=1−M(x;β,ΔΔ) and hazard function (HF) h(x;β,ΔΔ)=m(x;β,ΔΔ)1−M(x;β,ΔΔ), are given, respectively, by
S(x;β,ΔΔ)=β2ˉW(x;ΔΔ)[β+W(x;ΔΔ)]2,β>0,x∈R, |
and
h(x;β,ΔΔ)=w(x;ΔΔ)ˉW(x;ΔΔ)[β+W(x;ΔΔ)][β+2−W(x;ΔΔ)]. |
In this paper, we implement the proposed approach in Eq (1.2) and introduce a new modified/extended version of the logistic distribution, namely, a new modified exponential-logistic (NME-Logistic) model. The next section offers the CDF, PDF, SF, and HF of the NME-Logistic model. Furthermore, different PDF behaviors of the NME-Logisticmodel are also presented in the same section.
Consider the CDF W(x;ΔΔ) and PDF w(x;ΔΔ) of the two parameters (λ>0,η>0) logistic distribution given by
W(x;ΔΔ)=11+e−(x−ηλ),x∈R,η∈R,λ∈R+, | (2.1) |
and
w(x;ΔΔ)=e−(x−ηλ)(1+e−(x−ηλ ) )2,x∈R,η∈R,λ∈R+, |
respectively, where ΔΔ=(ϕ,φ). Using Eq (2.1) in Eq (1.2), we get the CDF of the NMExp-Weibull model given by
M(x;β,ΔΔ)=1−β2(1−11+e−(x−ηλ ) )[β+11+e−(x−ηλ ) ]2,x∈R,η∈R,λ,β∈R+, | (2.2) |
with PDF
m(x;β,ΔΔ)=β2e−(x−ηλ)(1+e−(x−ηλ ) )2[β+11+e−(x−ηλ ) ]3[β+2−11+e−(x−ηλ ) ],x∈R. | (2.3) |
Different plots for PDF of the NME-Logistic model are illustrated in Figure 2. The PDF plots of the NME-Logistic model are obtained for η=4,λ=1.5, and different values of β.
Furthermore, the SF and HF of the NME-logistic model are given by
S(x;β,ΔΔ)=β2(1−11+e−(x−ηλ ) )[β+11+e−(x−ηλ ) ]2,x∈R, |
and
h(x;β,ΔΔ)=1(1+e−(x−ηλ ) )[β+11+e−(x−ηλ ) ][β+2−11+e−(x−ηλ)],x∈R, |
respectively.
Here, we mathematically prove that the NMExp-X distributions possess the HT characteristics.
The RVTB (regularly varying tail behavior) plays a curial role to identify HT distributions. In this subsection, we derive the RVTB of the NMExp-X family. As per the results of Karamata's theorem Seneta [20], in terms of SF ˉW(x;Δ), we have
Theorem 1. If S(x;Δ)=1−W(x;Δ) is a RVF (regularly varying function), then S(x;β,Δ)=1−M(x;β,Δ) is also a RVF.
Proof. Assume limx→∞S(kx;Δ)S(x;Δ)=g(k) is finite but nonzero for every a>0. By incorporating Eq (1.2), we have
S(kx;β,ΔΔ)S(x;β,ΔΔ)=β2[1−W(kx;ΔΔ)][β+W(kx;ΔΔ)]2×[β+W(x;ΔΔ)]2β2[1−W(x;ΔΔ)], |
S(kx;β,ΔΔ)S(x;β,ΔΔ)=[1−W(kx;ΔΔ)][1−W(x;ΔΔ)]×[β+W(x;ΔΔ)]2[β+W(kx;ΔΔ)]2. |
Applying limx→∞ on both sides, we get
limx→∞S(kx;β,ΔΔ)S(x;β,ΔΔ)=limx→∞[1−W(kx;ΔΔ)][1−W(x;ΔΔ)]×[β+W(x;ΔΔ)]2[β+W(kx;ΔΔ)]2. | (3.1) |
Since limx→∞W(x;ΔΔ)=1. So, from Eq (3.1), we have
limx→∞S(kx;β,ΔΔ)S(x;β,ΔΔ)=limx→∞[1−W(kx;ΔΔ)][1−W(x;ΔΔ)]×[β+W(∞;ΔΔ)]2[β+W(k.∞;ΔΔ)]2, |
limx→∞S(kx;β,ΔΔ)S(x;β,ΔΔ)=limx→∞[1−W(kx;ΔΔ)][1−W(x;ΔΔ)]×[β+W(∞;ΔΔ)]2[β+W(∞;ΔΔ)]2, |
limx→∞S(kx;β,ΔΔ)S(x;β,ΔΔ)=limx→∞[1−W(kx;ΔΔ)][1−W(x;ΔΔ)]×[β+1]2[β+1]2, |
limx→∞S(kx;β,ΔΔ)S(x;β,ΔΔ)=limx→∞S(kx;ΔΔ)S(x;ΔΔ), |
limx→∞S(kx;β,ΔΔ)S(x;β,ΔΔ)=g(k). | (3.2) |
The expression provided in Eq (3.2) is finite but nonzero for every k>0. Therefore, S(x;β,ΔΔ) is a RVF.
Let's assume that the distribution of X has a power-law behavior, then we have
S(x;ΔΔ)=1−W(x;ΔΔ)=P(X>x)∼x−λ. |
Using the results of Karamata's characterization theorem, we can write S(x;β,ΔΔ) as
S(x;β,ΔΔ)=x−λL(x), |
where L(x) is a SVF. Note that
S(x;β,ΔΔ)=β2[1−W(x;ΔΔ)][β+W(x;ΔΔ)]2. | (3.3) |
Since 1−W(x;ΔΔ)∼x−λ. Therefore, from Eq (3.3), we get
S(x;β,ΔΔ)=β2x−λ(β+x−λ)2. |
S(x;β,ΔΔ)=x−λL(x), |
where L(x)=β2(β+x−λ)2. So, if we can show that L(x) is a SVF, then the RVTB obtained is true. For all λ>0, we must show that
limx→∞L(kx)L(x)=1. | (3.4) |
By carrying out the computation, we have
L(kx)L(x)=β2(β+(kx)−λ)2×(β+x−λ)2β2, |
L(kx)L(x)=(β+x−λ)2(β+(kx)−λ)2. |
Applying limx→∞ on both sides, we get
limx→∞L(kx)L(x)=limx→∞(β+x−λ)2(β+(kx)−λ)2. | (3.5) |
Since, x→∞, then limx→∞1xλ=0, and limx→∞1xλkλ=0. Therefore, from Eq (3.5), we obtain
limx→∞L(kx)L(x)=β2β2, |
which leads to the proof of Eq (3.4), given by
limx→∞L(kx)L(x)=1. |
In this section, we implement the NME-logistic distribution for analyzing two real-life data taken from the finance sector. The first data set represents the log-returns of the daily Bitcoin ERs. Whereas, the second data set represents the log-returns of the daily Ethereum ERs.
We fit the NME-logistic distribution to the daily Bitcoin and Ethereum ERs and compare its fitting results with the logistic and Gumble distributions. The SFs corresponding to the competing models are given by
● Logistic distribution
S(x;λ,η)=e−(x−ηλ)1+e−(x−ηλ),x∈R,η∈R,λ∈R+, |
and
● Gumble distribution
S(x;λ,η)=1−exp{−e−(x−ηλ)},x∈R,η∈R,λ∈R+, |
respectively.
To figure out analytically which competing distribution provides the close fit to the Bitcoin ERs data, three goodness of fit tests such as (i) Anderson–Darling (AD) test, (ii) Cramér–von Mises (CVM) test, and (iii) Kolmogorov–Smirnov (KS) test, were considered. Besides these tests, the p-value of the competing models has also been calculated.
In this subsection, we analyze the log-returns of the Bitcoin ERs data using the NME-logistic distribution and the other competing distributions. The data set is available at https://coinmarketcap.com/currencies/bitcoin/. The considered data set represents the daily Bitcoin ERs based on the USA dollars from June 30, 2014, to June 30, 2022.
The log-returns of the daily Bitcoin ERs, rt, is obtained as rt=log(Pt)−log(Pt−1), where Pt represents the exchange rate at time t. The summary statistics (SS) of log returns of the Bitcoin ERs are ˉx=−0.0014, mininum =−0.1740,Q1=−0.0222,Q2=0.0003,Q3=0.0188, maximum =0.1358, variance =0.0013, range =0.3098, skewness =−0.3939, and kurtosis =5.2472. Some basic plots of the daily Bitcoin ERs are presented in Figure 3.
Corresponding to the Bitcoin ERs data, the MLE (ˆλMLE,ˆηMLE,ˆβMLE) results of the fitted models are reported in Table 1. Whereas, the values of AD, CVM, KS, and p-value are obtained in Table 2. Based on the reported results in Table 2, it is obvious that the NME-Logistic model provides a close fit to the Bitcoin ERs data.
Model | ˆηMLE | ˆλMLE | ˆβMLE |
NME-Logistic | 0.0269 | 0.0197 | 0.8200 |
Logistic | -0.0021 | 0.0214 | - |
Gumbel | 0.1932 | 0.1076 | - |
Model | CVM | AD | KS | p-value |
NME-Logistic | 0.0690 | 0.3808 | 0.0364 | 0.7445 |
Logistic | 0.1321 | 0.7310 | 0.0654 | 0.1011 |
Gumbel | 0.3876 | 0.9765 | 0.1075 | 0.0953 |
Besides the numerical illustration of the fitted models, a visual comparison of the fitted models is also provided. For the visual illustration, the plots of fitted the PDFs (Figure 4), CDFs, and Kaplan-Meier (KM) survival plots (Figure 5) are considered. Based on the visual illustration provided in Figures 4 and 5, it is clear that the NME-Logistic model provides a close fit to the Bitcoin ERs data.
Here, we implement the NME-logistic distribution to analyze the log-returns of the Ethereum ERs data set. The Ethereum ERs data is available at https://www.google.com/finance/quote/ETH-USD. The second data set represents the daily Ethereum ERs based on the USA dollars from June 30, 2017, to June 30, 2022. The SS of the log-returns of the Ethereum ERs are ˉx=−0.0019, mininum =−0.1777,Q1=−0.0274,Q2=0.0006,Q3=0.0243, maximum =0.1258, variance =0.0020, range =0.3036, skewness =−0.3096, and kurtosis =4.0979. Some key plots of the daily Ethereum ERs are provided in Figure 6.
Using the Ethereum ERs data set, the values ˆλMLE,ˆηMLE, and ˆβMLE are presented in Table 3. Whereas, the values of AD, CVM, KS and p-value of the fitted distributions are obtained in Table 4. From the numerical results in Table 4, it is clear that the NME-Logistic distribution provides the best fit for the Ethereum ERs data.
Model | ˆηMLE | ˆλMLE | ˆβMLE |
NME-Logistic | 0.0577 | 0.0272 | 0.3193 |
Logistic | -0.0008 | 0.0246 | - |
Gumbel | 0.1264 | 0.0907 | - |
Model | CVM | AD | KS | p-value |
NME-Logistic | 0.0243 | 0.2030 | 0.0239 | 0.9850 |
Logistic | 0.0737 | 0.4548 | 0.0289 | 0.9195 |
Gumbel | 0.1280 | 0.8543 | 0.1015 | 0.8362 |
In addition to the numerical illustration of the competing distributions, a visual comparison of the competing models is also provided. For this purpose, the plots of fitted PDFs (Figure 7), CDFs, and KM plots (Figure 8) are obtained. Based on the visual comparison of the competing distributions in Figures 7 and 8, it is obvious that the NME-Logistic model provides the best fit for the Ethereum ERs data.
In the previous section, the modified version of the logistic distribution is examined in contrast to some existing probability distributions through real phenomena related to the log-returns of the daily Bitcoin and Ethereum ERs data sets. This section aims to forecast the log-returns of the daily Bitcoin and Ethereum ERs data by applying two popular machine learning techniques, such as artificial neural networks (ANNs) and support vector regression (SVR) including SETAR model. The effectiveness of ML techniques is assessed against the traditional model, namely, the self exciting threshold autoregressive (SETAR) model.
The ANN is a flexible computing algorithm for analyzing a wide range of non-linear problems. A significant superiority of the ANN algorithms over competitive nonlinear tools is, that they can approximate the huge class of functions with a higher degree of accuracy. In addition to this advantage, the construction of ANNs does not require any prior assumption. Alternatively, the network is ascertained by the characteristics of the data; see Zhang [21]. Among a list of numerous networks, the single hidden layer feed-forward network is the most popular algorithm type for predictive modeling. The algorithm is basically characterized by connecting three layers, namely, input layers, hidden layers, and output layers; see Peng et al. [22].
Mathematically, the connection between input layers (∅t−1,∅t−2,...,∅t−a,) and output layer (∅t) can be expressed as
∅t=β0+k∑i=1βiD(zi+a∑m=1zim∅t−m)+ϵt, |
where βi(i=1,2,3,...,k),zim(i=1,2,3,...,k,m=1,2,3,...,a) are the unknown parameters, often refers to connection weight, k and a are indicating the number of hidden and input layers, respectively. Using the first data set, we estimate the model with 3 hidden layers and 5 input layers (lagged variables), and for the second data set, 3 hidden layers and 3 input layers are used. Both hidden layers and input variables are chosen through the error and trial approach followed by Khashei and Hajirahimi [23].
The SVR approach was initially proposed by Cortes and Vapnik [24], and till today, it is widely used for classification and regression problems. The SVR is based on the structured risk minimization principle and statistical learning theory, which in turn evades the overfitting problem, and thus yields an accurate forecast. Awan et al. [25] argued that SVR approximates linear and nonlinear real-world problems precisely.
In practice, kernel function plays a key role in the forecasting performance of SVR. The utilization of kernel functions performs operations in the input space in place of higher-dimensional space. Numerous kernel functions are utilized in the literature including linear, polynomial, and sigmoid Radial Basis Functions (RBF) and splines; see Yu et al. [26]. Among these, RBF has gained a substantial focus by dint of its exceptional performance in capturing the non-linear nexus Ghosh [27] and Raje and Mujumdar [28].
The SVR is helpful while determining the margin of error which is acceptable in the model; see Bibi et al. [29] and Ribeiro et al. [30]. The mathematical expression for SVR with kernel function is illustrated as
∅t=k∑i=1(βi−β∗i)Z(Di−D)+ϵt, |
where D illustrates the support-vector, k indicates the size of support vector, Z(Di−D) indicates the kernel function, and ϵt illustrates the threshold value. Herein, the RBF with a parameter ℜ2 can be expressed as
Z(Di−Dk)=e−||Di−Dk||22ℜ2, |
where ||Di−Dk||2 denotes the Euclidean distance amidst the two covariates in squared form, ℜ2 denotes the width of RBF. In our study, we use the RBF as a kernel function for SVR.
Unlike the ML tools delineated in the previous subsections, the SETAR model belongs to the traditional statistical procedures of time series. The SETAR model was initially proposed by Tong and Lim [31], which is basically the modified form of the autoregressive model. The SETAR model is parametric, non-linear in nature, and a special case of markov switiching models; see D'Amato et al. [32]. It assumes that the series behavior varies post entering into a different regime. In Eq (12), suppose m denotes the number of regimes, often equal to two or three (in our case, the high regime and the low regime are represented by mH and mL respectively), and τ is the threshold indicator, where a series changes the regime, a represent the lagged values, the model SETAR(m = 2, a) can be expressed as
∅t=1(τt−1≤th)(zmL0+zmL1∅t−1+...+zmLa∅t−a)+1(τt−1>th)(zmH0+zmH1∅t−1+...+zmHa∅t−a). | (5.1) |
If the value of the threshold variable is fixed, then the model is linear and can be estimated by conditional least squares.
This work uses log-return of daily Bitcoin ERs data in order to quantify the predictability of ANN and SVM algorithms against SETAR model. Therefore, we split the data into two parts, intending to facilitate the out-of-sample prediction accuracy. For estimation, we use the data from June 30, 2014, to June 30, 2022 (log-returns of daily Bitcoin ERs), and June 30, 2017, to June 30, 2022 (log-returns of daily Ethereum ERs), for checking the models' multistep ahead out-of-sample forecasting accuracy. We use 80 percent data for estimating the models, and the remaining 20 percent data, for checking the models' multistep ahead out-of-sample forecasting accuracy, as followed by Ahmad et al. [33].
The predictability is evaluated through popular statistical measures, namely, root-mean-square error (RMSE) and mean absolute error (MAE). The model with lower RMSE and MAE is considered the best model comparatively. The mathematical expressions for MAE and RMSE are, respectively, given by
MAE=mean(|∅t−ˆ∅t|), |
and
RMSE=√mean(∅t−ˆ∅t)2, |
where the observed and forecasted values of log-returns of daily Bitcoin and Ethereum ERs data sets are given by ∅t and ˆ∅t, respectively.
Figure 9 depicts the log-returns of daily Bitcoin ERs data, which shows a high volatility nature, and thus it is a very difficult task to forecast it through traditional models. The alternative approaches to capture its high volatile behavior with satisfactory prediction accuracy, are machine learning tools. In this section, we apply ANN, SVR, and SETAR models to analyze the high volatile data.
In Figure 9, the log-returns of daily Bitcoin ERs data are divided by a vertical blue dotted line, where the training part for model estimation and the second part (testing data) is used for out-of-sample prediction.
The standard accuracy measures, namely, the RMSE and MAE for ML algorithms and the SETAR model are visually displayed in Figure 10. The height of the bar represents the models' forecasting performance in terms of RMSE and MAE. The smaller the height of a bar, the more accurate the forecast is comparatively.
From Figure 10, it can be seen that the corresponding bars for ANN are smaller than the SVR and SETAR models. In addition, SETAR outperforms the SVR. Therefore, we can infer that ANN yields a more satisfactory forecast than the other competitive approaches (SVR and SETAR).
The log-returns of daily Ethereum ERs series are very noisy in nature, as shown by Figure 11, thereby linear models have no capability to fit such data precisely, and provide accurate forecasts. Therefore, our study adopts alternate procedures, including the ML algorithms and SETAR model to fit the high volatile time series along with satisfactory forecasting accuracy. The vertical dotted line splits the log-returns of daily Ethereum ERs time series into two parts, as shown in Figure 11, where the first part (training set) is used for estimation and the second part (testing set) is used for post-sample prediction.
The forecast comparison between ML algorithms and the SETAR model is built through dual accuracy measures such as RMSE and MAE, which are presented in Figure 12. The bar height illustrates the predictability of the selected models in terms of RMSE and MAE. In general, the lesser height of a bar ensures a more reliable forecast. We can observe that the RMSE and MAE computed for SETAR are smaller than the ML algorithms, which ensures the robustness of the SETAR model. Moreover, across the ML techniques, the ANN produces a more accurate forecast than the SVR.
Cryptocurrencies are taking place in most aspects of daily life dealing with payments. Among the cryptocurrencies, Bitcoin and Ethereum hold key places and experience heavy-tailed behaviors. This paper contributed to the literature on distribution theory by introducing a new HT version of the logistic distribution, namely, a new modified exponential-logistic distribution. The HT characteristics of the NME-logistic distribution were proved empirically and visually. To establish the applicability of the NME-logistic distribution, two financial data sets related to the log-returns of Bitcoin and Ethereum exchange rates are analyzed. Furthermore, in order to forecast the high volatile behavior of the same datasets, we implement the dual ML algorithms, namely ANN and SVR in comparison to the SETAR model. To assess the effectiveness of these models, we split the data into two parts intending to facilitate the out-of-sample prediction accuracy. For models' estimation, we utilize 80 percent data, and the remaining part of the data is used, for checking the models' multistep ahead post sample predictive capability. Using the first dataset, the ANN produces promising results in contrast to SVR and SETAR. As we move towards the second dataset for analysis, the SETAR showed an outstanding performance than the ML algorithms. The study concludes that models' performance varies from data to data, because of its varying nature. Future studies can compare the aforementioned tools with other ML techniques like bagging, boosting, and random forest. In addition, to achieve more improvement in the prediction accuracy, the ensemble procedure can be developed using ANN, SVR, and SETAR.
The author Sharifah E. Alhazmi (sehazmi@uqu.edu.sa) would like to thank the Deanship of Scientific Research at Umm Al-Qura University for supporting this work by Grant Code: (22UQU4282396DSR14).
The authors declare no conflict of interest.
[1] |
A. Phillip, J. S. K. Chan, C. Peiris, A new look at cryptocurrencies, Econ. Lett., 163 (2018), 6–9. http://doi.org/10.1016/j.econlet.2017.11.020 doi: 10.1016/j.econlet.2017.11.020
![]() |
[2] |
A. Alzaatreh, H. Sulieman, On fitting cryptocurrency log-return exchange rates, Empir. Econ., 60 (2019), 1157–1174. http://doi.org/10.1007/s00181-019-01782-6 doi: 10.1007/s00181-019-01782-6
![]() |
[3] |
P. Ciaian, M. Rajcaniova, D. A. Kancs, The economics of BitCoin price formation, Appl. Econ., 48 (2016), 1799–1815. http://doi.org/10.1080/00036846.2015.1109038 doi: 10.1080/00036846.2015.1109038
![]() |
[4] |
J. A. Núñez, M. I. Contreras-Valdez, C. A. Franco-Ruiz, Statistical analysis of bitcoin during explosive behavior periods, PLoS ONE, 14 (2019), e0213919. http://doi.org/10.1371/journal.pone.0213919 doi: 10.1371/journal.pone.0213919
![]() |
[5] |
A. Punzo, L. Bagnato, Modeling the cryptocurrency return distribution via Laplace scale mixtures, Physica A, 563 (2021), 125354. http://doi.org/10.1016/j.physa.2020.125354 doi: 10.1016/j.physa.2020.125354
![]() |
[6] |
A. Ibrahim, R. Kashef, L. Corrigan, Predicting market movement direction for bitcoin: A comparison of time series modeling methods, Comput. Electr. Eng., 89 (2021), 106905. http://doi.org/10.1016/j.compeleceng.2020.106905 doi: 10.1016/j.compeleceng.2020.106905
![]() |
[7] |
A. Hachicha, F. Hachicha, Analysis of the bitcoin stock market indexes using comparative study of two models SV with MCMC algorithm, Rev. Quant. Finan. Acc., 56 (2021), 647–673. http://doi.org/10.1007/s11156-020-00905-w doi: 10.1007/s11156-020-00905-w
![]() |
[8] |
I. E. Livieris, N. Kiriakidou, S. Stavroyiannis, P. Pintelas, An advanced CNN-LSTM model for cryptocurrency forecasting, Electronics, 10 (2021), 287. http://doi.org/10.3390/electronics10030287 doi: 10.3390/electronics10030287
![]() |
[9] |
W. Chkili, A. B. Rejeb, M. Arfaoui, Does bitcoin provide hedge to Islamic stock markets for pre-and during COVID-19 outbreak? A comparative analysis with gold, Resour. Policy, 74 (2021), 102407. http://doi.org/10.1016/j.resourpol.2021.102407 doi: 10.1016/j.resourpol.2021.102407
![]() |
[10] |
Á. Cebrián-Hernández, E. Jiménez-Rodríguez, Modeling of the Bitcoin volatility through key financial environment variables: An application of conditional correlation MGARCH models, Mathematics, 9 (2021), 267. http://doi.org/10.3390/math9030267 doi: 10.3390/math9030267
![]() |
[11] |
W. Bazán-Palomino, How are Bitcoin forks related to Bitcoin?, Financ. Res. Lett., 40 (2021), 101723. http://doi.org/10.1016/j.frl.2020.101723 doi: 10.1016/j.frl.2020.101723
![]() |
[12] |
M. Qin, C. W. Su, R. Tao, BitCoin: A new basket for eggs?, Econ. Model., 94 (2021), 896–907, http://doi.org/10.1016/j.econmod.2020.02.031 doi: 10.1016/j.econmod.2020.02.031
![]() |
[13] |
Y. Ghabri, K. Guesmi, A. Zantour, Bitcoin and liquidity risk diversification, Financ. Res. Lett., 40 (2021), 101679. http://doi.org/10.1016/j.frl.2020.101679 doi: 10.1016/j.frl.2020.101679
![]() |
[14] |
M. Liu, H. Chen, J. Yan, Detecting roles of money laundering in Bitcoin mixing transactions: A goal modeling and mining framework, Front. Phys., 9 (2021), 665399. http://doi.org/10.3389/fphy.2021.665399 doi: 10.3389/fphy.2021.665399
![]() |
[15] |
E. Mahdi, V. Leiva, S. Mara'Beh, C. Martin-Barreiro, A new approach to predicting cryptocurrency returns based on the gold prices with support vector machines during the COVID-19 pandemic using sensor-related data, Sensors, 21 (2021), 6319. http://doi.org/10.3390/s21186319 doi: 10.3390/s21186319
![]() |
[16] |
X. F. Liu, X. J. Jiang, S. H. Liu, C. K. Tse, Knowledge discovery in cryptocurrency transactions: a survey, IEEE Access, 9 (2021), 37229–37254. http://doi.org/10.1109/ACCESS.2021.3062652 doi: 10.1109/ACCESS.2021.3062652
![]() |
[17] |
V. Naimy, O. Haddad, G. Fernández-Avilés, R. El Khoury, The predictive capacity of GARCH-type models in measuring the volatility of crypto and world currencies, PLoS ONE, 16 (2021), e0245904. http://doi.org/10.1371/journal.pone.0245904 doi: 10.1371/journal.pone.0245904
![]() |
[18] |
M. Umar, C. W. Su, S. K. A. Rizvi, X. F. Shao, Bitcoin: A safe haven asset and a winner amid political and economic uncertainties in the US?, Technol. Forecast. Soc., 167 (2021), 120680. http://doi.org/10.1016/j.techfore.2021.120680 doi: 10.1016/j.techfore.2021.120680
![]() |
[19] |
A. Alzaatreh, C. Lee, F. Famoye, A new method for generating families of continuous distributions, METRON, 71 (2013), 63–79. http://doi.org/10.1007/s40300-013-0007-y doi: 10.1007/s40300-013-0007-y
![]() |
[20] |
E. Seneta, Karamata's characterization theorem, feller and regular variation in probability theory, Publications de l'Institut Mathématique, 71 (2002), 79–89. http://doi.org/10.2298/PIM0271079S doi: 10.2298/PIM0271079S
![]() |
[21] |
G. P. Zhang, Time series forecasting using a hybrid ARIMA and neural network model, Neurocomputing, 50 (2003), 159–175, http://doi.org/10.1016/S0925-2312(01)00702-0 doi: 10.1016/S0925-2312(01)00702-0
![]() |
[22] |
Z. Peng, F. U. Khan, F. Khan, P. A. Shaikh, Y. Dai, I. Ullah, et al., An application of hybrid models for weekly stock market index prediction: Empirical evidence from SAARC countries, Complexity, 2021 (2021), 5663302. http://doi.org/10.1155/2021/5663302 doi: 10.1155/2021/5663302
![]() |
[23] |
M. Khashei, Z. Hajirahimi, A comparative study of series arima/mlp hybrid models for stock price forecasting, Commun. Stat.-Simulat. Comput., 48 (2019), 2625–2640. http://doi.org/10.1080/03610918.2018.1458138 doi: 10.1080/03610918.2018.1458138
![]() |
[24] | C. Cortes, V. Vapnik, Support-vector networks, Mach. Learn., 20 (1995), 273–297. http://doi.org/10.1007/BF00994018 |
[25] | S. M. Awan, Z. A. Khan, M. Aslam, W. Mahmood, A. Ahsan, Application of NARX based FFNN, SVR and ANN Fitting models for long term industrial load forecasting and their comparison, 2012 IEEE International Symposium on Industrial Electronics, IEEE, 2012,803–807. http://doi.org/10.1109/ISIE.2012.6237191 |
[26] |
P. S. Yu, T. C. Yang, S. Y. Chen, C. M. Kuo, H. W. Tseng, Comparison of random forests and support vector machine for real-time radar-derived rainfall forecasting, J. Hydrol., 552 (2017), 92–104. http://doi.org/10.1016/j.jhydrol.2017.06.020 doi: 10.1016/j.jhydrol.2017.06.020
![]() |
[27] |
S. Ghosh, SVM‐PGSL coupled approach for statistical downscaling to predict rainfall from GCM output, J. Geophys. Res.: Atmos., 115 (2010), D22102. http://doi.org/10.1029/2009JD013548 doi: 10.1029/2009JD013548
![]() |
[28] |
D. Raje, P. P. Mujumdar, A comparison of three methods for downscaling daily precipitation in the Punjab region, Hydrol. Process., 25 (2011), 3575–3589. http://doi.org/10.1002/hyp.8083 doi: 10.1002/hyp.8083
![]() |
[29] |
N. Bibi, I. Shah, A. Alsubie, S. Ali, S. A. Lone, Electricity spot prices forecasting based on ensemble learning, IEEE Access, 9 (2021), 150984–150992. http://doi.org/10.1109/ACCESS.2021.3126545 doi: 10.1109/ACCESS.2021.3126545
![]() |
[30] |
M. H. D. M. Ribeiro, R. G. da Silva, V. C. Mariani, L. dos Santos Coelho, Short-term forecasting COVID-19 cumulative confirmed cases: Perspectives for Brazil, Chaos Soliton. Fract., 135 (2020), 109853. http://doi.org/10.1016/j.chaos.2020.109853 doi: 10.1016/j.chaos.2020.109853
![]() |
[31] | H. Tong, K. S. Lim, Threshold autoregression, limit cycles and cyclical data, In: Exploration of a nonlinear world: An appreciation of Howell Tong's contributions to statistics, World Scientific Publishing, 2009, 9–56. https://doi.org/10.1142/9789812836281_0002 |
[32] |
V. D'Amato, S. Levantesi, G. Piscopo, Deep learning in predicting cryptocurrency volatility, Physica A, 596 (2022), 127158. http://doi.org/10.1016/j.physa.2022.127158 doi: 10.1016/j.physa.2022.127158
![]() |
[33] |
Z. Ahmad, Z. Almaspoor, F. Khan, M. El-Morshedy, On predictive modeling using a new flexible Weibull distribution and machine learning approach: Analyzing the COVID-19 data, Mathematics, 10 (2022), 1792. http://doi.org/10.3390/math10111792 doi: 10.3390/math10111792
![]() |
1. | Guang Lu, Osama Abdulaziz Alamri, Badr Alnssyan, Mohammed A. Alshahrani, A new probabilistic model: Its implementations to time duration and injury rates in physical training, sports, and reliability sector, 2024, 108, 11100168, 839, 10.1016/j.aej.2024.09.049 | |
2. | Huda M. Alshanbari, Gadde Srinivasa Rao, Jin-Taek Seong, Saima K. Khosa, A New Sine-Based Distributional Method with Symmetrical and Asymmetrical Natures: Control Chart with Industrial Implication, 2023, 15, 2073-8994, 1892, 10.3390/sym15101892 | |
3. | Mohammed Ahmed Alomair, Zubair Ahmad, Gadde Srinivasa Rao, Hazem Al-Mofleh, Saima Khan Khosa, Abdulaziz Saud Al Naim, Qichun Zhang, A new trigonometric modification of the Weibull distribution: Control chart and applications in quality control, 2023, 18, 1932-6203, e0286593, 10.1371/journal.pone.0286593 | |
4. | Yiming Zhao, Sultan Salem, Areej M. AL-Zaydi, Jin-Taek Seong, Fatimah M. Alghamdi, M. Yusuf, On fitting and forecasting the log-returns of Bitcoin and Ethereum exchange rates via a new sine-based logistic model and robust regression methods, 2024, 96, 11100168, 225, 10.1016/j.aej.2024.03.080 | |
5. | Yousef F. Alharbi, Ahmed M. T. Abd El-Bar, Mahmoud A. E. Abdelrahman, Ahmed M. Gemeay, Arne Johannssen, A new statistical distribution via the Phi-4 equation with its wide-ranging applications, 2024, 19, 1932-6203, e0312458, 10.1371/journal.pone.0312458 | |
6. | Ahmad Abubakar Suleiman, Hanita Daud, Narinderjit Singh Sawaran Singh, Mahmod Othman, Aliyu Ismail Ishaq, Rajalingam Sokkalingam, A Novel Odd Beta Prime-Logistic Distribution: Desirable Mathematical Properties and Applications to Engineering and Environmental Data, 2023, 15, 2071-1050, 10239, 10.3390/su151310239 | |
7. | Osama Abdulaziz Alamri, Olayan Albalawi, A new probabilistic approach for modeling the confirmation time of transactions on blockchain technology, 2024, 87, 11100168, 591, 10.1016/j.aej.2023.12.060 | |
8. | Sanaa Al-Marzouki, Afaf Alrashidi, Christophe Chesneau, Mohammed Elgarhy, Rana H. Khashab, Suleman Nasiru, On improved fitting using a new probability distribution and artificial neural network: Application, 2023, 13, 2158-3226, 10.1063/5.0176715 | |
9. | Mustafa Kamal, Sabir Ali Siddiqui, Afaf Alrashidi, Maha M. Helmi, Hassan M. Aljohani, Aned Al Mutairi, Ibrahim AlKhairy, Eslam Hussam, M. Yusuf, Samhi Abdelaty Difalla, On modeling the log-returns of Bitcoin and Ethereum prices against the USA Dollar, 2024, 87, 11100168, 340, 10.1016/j.aej.2023.11.080 | |
10. | Li Jiang, Jin-Taek Seong, Marwan H. Alhelali, Basim S.O. Alsaedi, Fatimah M. Alghamdi, Ramy Aldallal, A new cosine-based approach for modelling the time-to-event phenomena in sports and engineering sectors, 2024, 98, 11100168, 19, 10.1016/j.aej.2024.04.037 | |
11. | Zhiyong Qian, Wangsen Xiao, Shulan Hu, The generalization ability of logistic regression with Markov sampling, 2023, 31, 2688-1594, 5250, 10.3934/era.2023267 | |
12. | Huda M. Alshanbari, Omalsad Hamood Odhah, Zubair Ahmad, Faridoon Khan, Abd Al-Aziz Hosni El-Bagoury, A New Probability Distribution: Model, Theory and Analyzing the Recovery Time Data, 2023, 12, 2075-1680, 477, 10.3390/axioms12050477 |
Model | ˆηMLE | ˆλMLE | ˆβMLE |
NME-Logistic | 0.0269 | 0.0197 | 0.8200 |
Logistic | -0.0021 | 0.0214 | - |
Gumbel | 0.1932 | 0.1076 | - |
Model | CVM | AD | KS | p-value |
NME-Logistic | 0.0690 | 0.3808 | 0.0364 | 0.7445 |
Logistic | 0.1321 | 0.7310 | 0.0654 | 0.1011 |
Gumbel | 0.3876 | 0.9765 | 0.1075 | 0.0953 |
Model | ˆηMLE | ˆλMLE | ˆβMLE |
NME-Logistic | 0.0577 | 0.0272 | 0.3193 |
Logistic | -0.0008 | 0.0246 | - |
Gumbel | 0.1264 | 0.0907 | - |
Model | CVM | AD | KS | p-value |
NME-Logistic | 0.0243 | 0.2030 | 0.0239 | 0.9850 |
Logistic | 0.0737 | 0.4548 | 0.0289 | 0.9195 |
Gumbel | 0.1280 | 0.8543 | 0.1015 | 0.8362 |
Model | ˆηMLE | ˆλMLE | ˆβMLE |
NME-Logistic | 0.0269 | 0.0197 | 0.8200 |
Logistic | -0.0021 | 0.0214 | - |
Gumbel | 0.1932 | 0.1076 | - |
Model | CVM | AD | KS | p-value |
NME-Logistic | 0.0690 | 0.3808 | 0.0364 | 0.7445 |
Logistic | 0.1321 | 0.7310 | 0.0654 | 0.1011 |
Gumbel | 0.3876 | 0.9765 | 0.1075 | 0.0953 |
Model | ˆηMLE | ˆλMLE | ˆβMLE |
NME-Logistic | 0.0577 | 0.0272 | 0.3193 |
Logistic | -0.0008 | 0.0246 | - |
Gumbel | 0.1264 | 0.0907 | - |
Model | CVM | AD | KS | p-value |
NME-Logistic | 0.0243 | 0.2030 | 0.0239 | 0.9850 |
Logistic | 0.0737 | 0.4548 | 0.0289 | 0.9195 |
Gumbel | 0.1280 | 0.8543 | 0.1015 | 0.8362 |