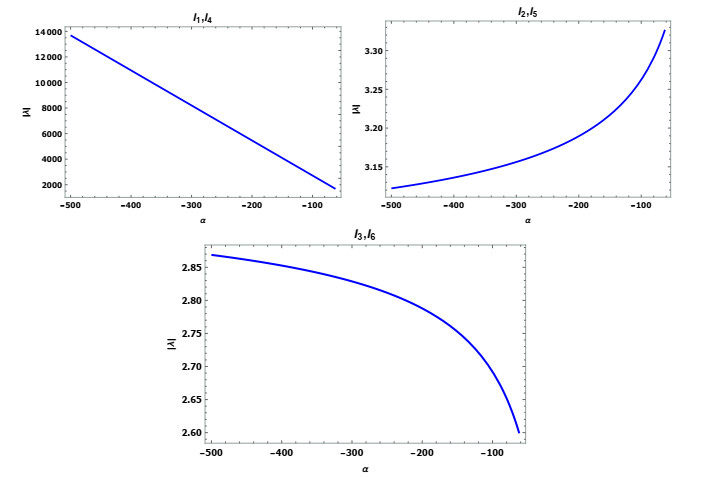
In this study, we consider positivity and other related concepts such as α−convexity and α−monotonicity for discrete fractional operators with exponential kernel. Namely, we consider discrete Δ fractional operators in the Caputo sense and we apply efficient initial conditions to obtain our conclusions. Note positivity results are an important factor for obtaining the composite of double discrete fractional operators having different orders.
Citation: Pshtiwan Othman Mohammed, Donal O'Regan, Aram Bahroz Brzo, Khadijah M. Abualnaja, Dumitru Baleanu. Analysis of positivity results for discrete fractional operators by means of exponential kernels[J]. AIMS Mathematics, 2022, 7(9): 15812-15823. doi: 10.3934/math.2022865
[1] | Ling Zhou, Zuhan Liu . Blow-up in a p-Laplacian mutualistic model based on graphs. AIMS Mathematics, 2023, 8(12): 28210-28218. doi: 10.3934/math.20231444 |
[2] | Meiling Hu, Shuli Li . Cospectral graphs for the normalized Laplacian. AIMS Mathematics, 2022, 7(3): 4061-4067. doi: 10.3934/math.2022224 |
[3] | Jahfar T K, Chithra A V . Central vertex join and central edge join of two graphs. AIMS Mathematics, 2020, 5(6): 7214-7233. doi: 10.3934/math.2020461 |
[4] | Sara Pouyandeh, Amirhossein Morovati Moez, Ali Zeydi Abdian . The spectral determinations of connected multicone graphs Kw ▽ mCP(n). AIMS Mathematics, 2019, 4(5): 1348-1356. doi: 10.3934/math.2019.5.1348 |
[5] | Ali Raza, Mobeen Munir, Tasawar Abbas, Sayed M Eldin, Ilyas Khan . Spectrum of prism graph and relation with network related quantities. AIMS Mathematics, 2023, 8(2): 2634-2647. doi: 10.3934/math.2023137 |
[6] | Ze-Miao Dai, Jia-Bao Liu, Kang Wang . Analyzing the normalized Laplacian spectrum and spanning tree of the cross of the derivative of linear networks. AIMS Mathematics, 2024, 9(6): 14594-14617. doi: 10.3934/math.2024710 |
[7] | Jia-Bao Liu, Kang Wang . The multiplicative degree-Kirchhoff index and complexity of a class of linear networks. AIMS Mathematics, 2024, 9(3): 7111-7130. doi: 10.3934/math.2024347 |
[8] | Zhi-Yu Shi, Jia-Bao Liu . Topological indices of linear crossed phenylenes with respect to their Laplacian and normalized Laplacian spectrum. AIMS Mathematics, 2024, 9(3): 5431-5450. doi: 10.3934/math.2024262 |
[9] | Yunzhe Zhang, Youhui Su, Yongzhen Yun . Existence and stability of solutions for Hadamard type fractional differential systems with p-Laplacian operators on benzoic acid graphs. AIMS Mathematics, 2025, 10(4): 7767-7794. doi: 10.3934/math.2025356 |
[10] | Azhar Iqbal, Abdullah M. Alsharif, Sahar Albosaily . Numerical study of non-linear waves for one-dimensional planar, cylindrical and spherical flow using B-spline finite element method. AIMS Mathematics, 2022, 7(8): 15417-15435. doi: 10.3934/math.2022844 |
In this study, we consider positivity and other related concepts such as α−convexity and α−monotonicity for discrete fractional operators with exponential kernel. Namely, we consider discrete Δ fractional operators in the Caputo sense and we apply efficient initial conditions to obtain our conclusions. Note positivity results are an important factor for obtaining the composite of double discrete fractional operators having different orders.
From the early ages, real-world and engineering problems have required solving nonlinear equations or systems of nonlinear equations. These solutions usually can only be estimated, as their exact values cannot be calculated. This is the area where iterative methods show their power: to obtain approximations of the solutions of these kinds of problems in the most efficient way.
Therefore, our main goal is to solve the equation f(x)=0, where f:D⊂R→R is a scalar function, or vectorial F(x)=0, where F:D⊂Rn→Rn, n>1, defined on a convex set D. From a seed x(0)∈Rn (x0∈R if n=1), the most employed iterative scheme for estimating the zeroes of these kinds of functions is Newton's scheme, with iterative expression
x(k+1)=x(k)−[F′(x(k))]−1F(x(k)), k=0,1,…, |
where F′(x(k)) is the Jacobian matrix of F evaluated at x(k).
Some classical results about the extension of scalar one-point iterative methods to systems can be found in texts from Traub [29], Ortega and Rheimboldt [22] or Ostrowski [23]. Also, a good and more recent review can be found in [15]. Multipoint methods arrived with the need of improving the efficiency of the schemes without using second-order derivatives. Gerlach in [18] and Weerakoon and Fernando in [31] used quadrature formulas for this aim, and this task was generalized by Frontini and Sormani in [17], Cordero and Torregrosa in [12,13], Ozban in [24] and Wang et al. in [30].
Other techniques were developed to design vectorial methods, such as the pseudocomposition [14], still directly related with quadrature formulas. However, Artidiello et al., in [2], developed the matrix weight function technique to get higher order schemes. Other authors have also used these and other procedures to construct multidimensional methods, as in [3,4,20,25,26,27,32,34], but the amount of this kind of scheme in the literature is still low, compared with their scalar partners. Moreover, it is known that the higher the order of convergence is, the smaller the set of converging initial estimations.
To assure the convergence of Newton's procedure, a seed near to the solutions is necessary, but in general, the modeling of most technical and scientific problems does not hold this condition. To enlarge the set of converging initial estimations, a modification of classical Newton's scheme was designed for the scalar case f(x)=0 in [16,33] as
xk+1=xk−γkf(x)f′(xk),k=0,1,…, |
where γk is defined as a sequence of real numbers depending on previous and actual iterations.
Let us remark that, for fixed values of γk along the iterative process, the order of convergence is only linear. Nevertheless, if it is calculated at each iteration from the available information, it holds the quadratic convergence, and the sets of converging initial estimations are wider (see [5]).
One of the possibilities for γk is due to Kalitkin and Ermakov (see [16]),
γk=‖f(xk)‖2‖f(xk)‖2+‖f(xk−f(x)f′(xk))‖2,k=0,1,…, |
where ‖⋅‖ denotes any norm. In [5], Budzko et al. designed a two-step scheme, and also its multidimensional extension was proposed. In it, the predictor step is a damped Newton type (γk=α) and the corrector is Kalitkin-Ermakov type. In the scalar case,
γkeq=f(xk)2bf(xk)2+cf(xk−f(xk)f′(xk))2,k=0,1,… |
For systems, coefficient γkeq becomes
Γ(k)sys(α,b,c)=[bI+cα2(1αI−[F′(x(k))]−1[x(k),y(k);F])2]−1, |
with I the identity matrix and α,b,c free parameters.
In this paper, a novel class (PMKE) for solving nonlinear systems is proposed and studied. This class has frozen Jacobian and sixth-order convergence. With the aim of widening the regions of starting points that assure the convergence, this family has been designed with the classical Newton's procedure at the first step. Moreover, the second step has a damping factor of Kalitkin-Ermakov type, and the third step is based on a weight function procedure. For this class, the damped coefficient in the second step for systems has the form Γ(k)sys(1,1,1)=Γ(k).
As we construct a whole class of iterative procedures, depending on the weight function to be selected and also on any parameter, we apply techniques from discrete dynamical systems in order to select those members with better stability properties. We understand these improvements in terms of the wideness of the sets of initial guesses able to converge to the roots of nonlinear problems. In [11], the authors developed a procedure to arrange this kind of analysis by means of multidimensional real dynamics. From that moment on, different authors have used this technique (or complex dynamics if they return to the scalar case) in order to study the qualitative behavior of the iterative schemes designed. See, for example, [1,6,7,8,9,10,19], among others. These techniques are useful in solving nonlinear partial differential equations, as well as stochastic differential equations. In the numerical section, proposed methods are applied to an equation of heat conduction for a non-homogeneous medium.
The information of this paper is organized as follows: In Section 2, we introduce, after some basic definitions, the new three step iterative scheme, and its convergence study is presented in Section 3. Next, the dynamical analysis of the proposed method is realized in Section 4. Then, some numerical experiments are shown, and some conclusions are stated, in Sections 5 and 6, respectively.
For the sake of completeness, we first introduce some main definitions about iterative processes in numerical analysis. After that, we define some concepts and properties of discrete dynamical systems, which are necessary to follow the results obtained in Section 4.
Let us consider a sequence {x(k)}k≥0 in Rn converging to the root of F(x)=0. Then, convergence has order p≥1, if there exist N>0 (0<N<1 with p=1) and k0 satisfying
‖e(k+1)‖≤N‖e(k)‖p,∀k≥k0, with e(k)=x(k)−ξ, |
or
‖x(k+1)−ξ‖≤N‖x(k)−ξ‖p,∀k≥k0. |
Assuming three consecutive iterates x(k−1),x(k),x(k+1) close to ξ, ρ was introduced in [12] as the Approximated Computational Order of Convergence (ACOC),
p≈ACOC=ln( ‖ x(k − 1) − x(k) ‖ ‖ x(k) − x(k − 1) ‖ )ln( ‖ x(k) − x(k − 1) ‖ ‖ x(k − 1) − x(k − 2) ‖ ). | (2.1) |
On the other hand, as F is a sufficiently Fréchet differentiable function, we can consider ξ+h∈Rn in a neighborhood of ξ. By using Taylor expansions and F′(ξ) being non-singular,
F(ξ+h)=F′(ξ)[h+p−1∑q=2Cqhq]+O(hp). | (2.2) |
In this expression, Cq=1q![F′(ξ)]−1F(q)(ξ), q≥2. Let us remark that Cqhq∈Rn, since [F′(ξ)]−1∈L(Rn), and F(q)(ξ)∈L(Rn×⋯×Rn,Rn). Then, we can express F′ as
F′(ξ+h)=F′(ξ)[I+p−1∑q=2qCqhq−1]+O(hp−1), | (2.3) |
where qCqhq−1∈L(Rn).
Moreover, following the notation defined by Artidello et al. in [2], if X=Rn×n is the Banach space of real n×n matrices, a matrix function H:X→X can be defined such that its Fréchet derivatives satisfy
(a) H′(u)(v)=H1uv,beingH′:X→L(X), H1∈R,
(b) H″(u,v)(v)=H2uvw,beingH″:X×X→L(X) H2∈R.
Let G be a vectorial rational operator related to an iterative scheme applied on a polynomial function p:D⊆Rn→Rn, and the orbit of a point x∈Rn is defined as the successive images G(x0) in the form {x,G(x),…,Gm(x)}.
If x∗ is a such point that G(x∗)=x∗, then it is denominated a fixed point of G. This point is attracting if all the eigenvalues λj of G′(x∗) satisfy the condition |λj|<1, it is unstable if one eigenvalue satisfies the condition |λj0|>1, and it is repelling if all λj satisfy |λj|>1. On the other hand, the basin of attraction B(x∗) of a certain x∗ is defined as the set of pre-images of any order such that
B(x∗)={x∈Rn:Gm(x)→x∗,m→∞}. |
If xc is such a point that G(xc)=0, then it is called a critical point. Those critical points different from the roots of F(x)=0 are called free critical points. In each basin of attraction of a periodic point, there exists one critical point, so the study of those points and their orbits is crucial.
All these notations are widely used in the demonstration of the convergence and dynamical study in the following sections.
Let us consider the three step iterative method PMKE:
y(k)=x(k)−F′(x(k))−1F(x(k)),z(k)=y(k)−Γ(k)F′(x(k))−1F(y(k)),x(k+1)=z(k)−H(τ(k))F′(x(k))−1F(z(k)),k≥0, | (3.1) |
where Γ(k)=[I−(I−[F′(x(k))]−1[y(k),x(k);F])2]−1 and τ(k)=I+[F′(x(k))]−1[z(k),y(k);F]Γ(k). In the following result, we state the conditions to be satisfied to get fifth- and sixth-order convergence of class (3.1).
Theorem 3.1. Let us consider a sufficiently Fréchet differentiable function F:D⊆Rn→Rn defined in an open neighborhood D of its zero ξ∈R, and H:Rn×n→Rn×n is a sufficiently differentiable matrix function. Let us also assume that F′(ξ) is non-singular, and x(0) is a seed near enough to ξ. Then, the sequence {x(k)}k≥0 obtained from (3.1) converges to ξ with order 5 if H0=(2+H2)I,H1=−(1+2H2), and |H2|<∞, where I is the identity matrix, and H0=H(I). In this case, the error equation is
e(k+1)=−8(−1+H2)C42e(k)5+O(e(k)6). |
Moreover, if H2=1, the order of convergence is six, and the error equation is
e(k+1)=(24C52−4C3C32)e(k)6+O(e(k)7), |
where Cq=1q![F′(ξ)]−1F(q)(ξ), q=2,3,...
Proof. By means of Taylor series of F(x) around ξ, we get the expansion of F(x(k)), F′(x(k)), F″(x(k)) and F‴(x(k)) around ξ. Indeed,
[F′(x(k))]−1=[I+X2e(k)+X3e(k)2+X4e(k)3+X5e(k)4+X6e(k)5][F′(ξ)]−1+O(e(k)5), | (3.2) |
where X2=−2C2, X3=−3C3+4C22, X4=−4C4+6C2C3+6C3C2−8C32, X5=−5C5+8C2C4−12C22C3+9C23+8C4C2−12C2C3C2+16C42−12C3C22, and
X6=−32C52+24C32C3−18C2C23−16C22C4+12C3C4+10C2C5−6C6=+24C22C3C2+18C23C2−16C2C4C2+10C5C2+24C2C3C22−16C4C22=+24C3C32+12C4C3−18C3C2C3. |
These values of Xi, for i=2,3,…,6, have been obtained by assuming that [F′(x(k))]−1[F′(x(k))]=I is satisfied and solving the subsequent linear system. Then,
[F′(x(k))]−1F(x(k))=e(k)−C2e(k)2+2(C22−C3)e(k)3+(4C2C3+3C3C2−4C32−3C4)e(k)4+(−4C5+6C2C4−8C22C3+6C23+4C4C2−6C2C3C2+8C42−6C3C22)e(k)5+(−16C52+16C32C3−12C2C23−12C22C4+9C3C4+8C2C5−5C6+12C22C3C2−9C23C2−8C2C4C2+5C5C2+12C2C3C22−8C4C22+12C3C32+8C4C3−12C3C2C3)e(k)6+O(e(k)7), |
and
y(k)−ξ=C2e(k)2−2(C22−C3)e(k)3−(4C2C3+3C3C2−4C32−3C4)e(k)4−(−4C5+6C2C4−8C22C3+6C23+4C4C2−6C2C3C2+8C42−6C3C22)e(k)5−(−16C52+16C32C3−12C2C23−12C22C4+9C3C4+8C2C5−5C6+12C22C3C2−9C23C2−8C2C4C2+5C5C2+12C2C3C22−8C4C22+12C3C32+8C4C3−12C3C2C3)e(k)6+O(e(k)7). | (3.3) |
Therefore,
F(y(k))=F′(ξ)[C2e(k)2+2(C3−C22)e(k)3+(3C4+5C32−3C3C2−4C2C3)e(k)4+(4C5−6C2C4+10C22C3−6C23−4C4C2+8C2C3C2−12C42+6C3C22)e(k)5+(28C52−27C32C3+16C2C23+15C22C4−9C3C4−8C2C5+5C6−16C22C3C2+9C23C2+11C2C4C2−5C5C2−19C2C3C22+8C4C22−11C3C32−8C4C3+12C3C2C3)e(k)6]+O(e(k)7). | (3.4) |
Now, we can express the last product of the second step as
[F′(x(k))]−1F(y(k))=C2e(k)2+(2C3−4C22)e(k)3+(13C32−8C2C3+3C4−6C3C2)e(k)4+(−38C42+26C22C3−12C23−12C2C4+4C5+20C2C3C2−+18C3C220−8C4C20)e(k)5+(104C52−79C32C3+40C2C23+39C22C4−18C3C4−16C2C5+5C6−56C22C3C2+27C23C2+27C2C4C2−10C5C2−55C2C3C22+24C4C22−50C3C32−16C4C3+36C3C2C3)e(k)6+O(e(k)7). | (3.5) |
Using the Hermite-Genocchi formula (see [22]) for the operator of first divided difference [x+h,x;F]=∫10F′(x+th)dt=F′(x)+12F″(x)h+16F‴(x)h2+O(h3), ∀(x,h)∈Rn×Rn, we can obtain the operator [y,x;F] related to step two of the iterative scheme (3.1):
[y(k),x(k);F]=F′(ξ)[1+C2e(k)+(C22+C3)e(k)2+(−2C32+2C2C3+2C4+C3C2)e(k)3+(4C42−4C22C3+2C23+3C2C4+5C5−3C2C3C2−2C4C2−C3C22)e(k)4]+O(e(k)5), | (3.6) |
and
[F′(x(k))]−1[y(k),x(k);F]=I−C2e(k)+(3C22−2C3)e(k)2+(−8C32+6C2C3−2C4+4C3C2)e(k)3+(20C42−16C22C3+8C23+7C2C4−11C2C3C2+2C4C2−10C3C22)e(k)4+(−48C52+40C32C3−22C2C23−20C22C4+9C3C4+4C2C5+5C6+28C22C3C2−13C23C2−8C2C4C2−5C5C2+26C2C3C22−4C4C22+24C3C32+4C4C3−20C3C2C3)e(k)5+(112C62−96C42C3+56C22C23−26C33+52C32C4−27C2C3C4+2C24−16C22C5+4C3C5−5C2C6+14C7−56C32C3C2+35C2C23C2+24C22C4C2−7C3C4C2+5C2C5C2−19C6C2−64C22C3C22+29C23C22+16C2C4C22+15C5C22+8C4C32−60C2C3C32−56C3C42−16C2C4C3−10C5C3+52C2C3C2C3−8C4C2C3+48C3C22C3−24C3C2C4−12C32C3C2−2C4C3C2+32C3C2C3C2)e(k)6+O(e(k)7). |
The Taylor expansion around the solution of the term B=I+(I−[F′(x(k))]−1[x(k),y(k);F])2 in the second step of (3.1) can be expressed as
B=I+C22e(k)2+(−6C32+2C2C3+2C3C2)e(k)3+(25C42−12C22C3+4C23+2C2C4+2C4C2−10C2C3C2)e(k)4+O(e(k)5). | (3.7) |
Let us now find the inverse of B that can be defined as B−1=Γ(k)=I+K1e(k)+K2e(k)2+K3e(k)3+K4e(k)4+O(e(k)5). Fixing the condition BB−1=B−1B=I, we can find the coefficients:
K1=0,K2=−C22,K3=2(3C32−C2C3−C3C2),K4=C32−25C42+12C22C3−4C23−2C2C4+10C2C3C2+10C3C22−2C4C2. |
Therefore,
Γ(k)=I−C22e(k)2+2(3C32−C2C3−C3C2)e(k)3+(C32−25C42+12C22C3−4C23−2C2C4+10C2C3C2−10C3C22+2C4C2)e(k)4+O(e(k)5), | (3.8) |
and with the help of expressions (3.3), (3.5) and (3.8), we obtain the error in the second step:
z(k)−ξ=(y(k)−ξ)−[I+(I−[F′(x(k))]−1[x(k),y(k);F])2]−1F′(x(k))−1F(y(k))=2C22e(k)3+(−8C32+4C2C3+3C3C2)e(k)4+(20C42−14C22C3+6C23+6C2C4−12C2C3C2+4C4C2−12C3C22)e(k)5+(C42+42C52−39C32C3+32C2C23+34C22C4−18C3C4+5C6−16C2C5−32C22C3C2+23C23C2+25C2C4C2−10C5C2−45C2C3C22+24C4C22−50C3C32−16C4C3+36C3C2C3)e(k)6+O(e(k)7). | (3.9) |
To get the error equation, we obtain first the first order divided difference [z(k),y(k);F], knowing that
h(k)=z(k)−y(k)=−C2e(k)2+(4C22−2C3)e(k)3+O(e(k)4), | (3.10) |
and
F(z(k))=F′(ξ)[(z(k)−ξ)+C2(z(k)−ξ)2]+O((z(k)−ξ)3)=F′(ξ)[2C22e(k)3+(−8C32+4C2C3+3C3C2)e(k)4+(20C42−14C22C3+6C23+6C2C4+4C4C2−12C2C3C2−12C3C22)e(k)5+(−22C52−C42+23C32C3−20C2C23+9C3C4−22C22C4+20C22C3C2+8C2C5−14C23C2−17C2C4C2+33C2C3C22+5C5C2+38C3C32−16C4C22+8C4C3−24C3C2C3)e(k)6]+O(e(k)7). | (3.11) |
Using (3.10) and the Hermite-Genocchi formula, we get
[z(k),y(k);F]=F′(ξ)[I+C22e(k)2+(3C2C4−4C42−3C2C3C20+4C3C220)e(k)4+(12C52−8C32C3−6C2C23+2C2C23+4C2C5+8C2C3C22−20C3C32+8C3C2C3)e(k)5+(−10C62−C52+27C42C3+4C33−12C32C4+5C2C6−9C2C3C4+2C32C3C2+25C2C23C2+3C3C4C2−9C22C4C2−2C23C22−11C22C3C22−28C2C3C32−2C2C4C22+76C3C42−2C4C32+16C2C3C2C3−49C3C22C3+12C3C2C4−26C3C2C3C2)e(k)6]+O(e(k)7). | (3.12) |
By using (3.2), (3.8) and (3.12), the variable τ(k) can be expanded as
τ(k)=I+[F′(x(k))]−1[z(k),y(k);F][I+(I−[F′(x(k))]−1[y(k),x(k);F])2]−1=2C2e(k)+(−4C22+3C3)e(k)2+(2C32−6C2C3+4C4−4C3C2)e(k)3+(−C32+26C43−9C2C4+5C5+C2C3C2−6C4C2−2C3C22)e(k)4+(8C42−152C52−2C22C3+58C32C3−4C2C23+5C22C4−8C3C4+6C6−14C2C5+37C22C3C2−2C2C3C2+3C2C4C2−6C23C2+37C2C3C22−10C5C2+8C4C22)+52C3C32−8C4C3−10C3C2C3)e(k)5+(−40C52+554C62+16C32C3−295C42C3−4C2C23+82C22C23−21C33+59C32C4−2C22C4+4C2C3C4−12C24+24C22C5−15C3C5+7C7−22C2C6+14C22C3C2−185C32C3C2+42C2C23C2−2C2C4C2+37C4C2C22+2C3C4C2+21C2C5C2−17C6C2+10C2C3C22−195C22C3C22+61C23C22+22C2C4C22+3C3C32+25C5C22+20C4C32−222C2C3C32−276C3C42−2C2C4C3−15C5C3+86C2C3C2C3+8C4C2C3+125C3C22C3−7C3C2C4+4C4C3C2+81C3C2C3C2)e(k)6+O(e(k)7). | (3.13) |
Then, employing (3.9), (3.11)–(3.13) and the Taylor expansion of the weight function H(τ(k))=H0+H1(τ(k)−I)+H2(τ(k)−I)2+O((τ(k)−I)3), the error equation takes the form
e(k+1)=(2C22−2(H0+H1+H2)C22)e(k)3+(−8C32+4C3C2+3C3C2−2(−2C2H1−4C2H2)C22−(H0+H1+H2)(−12C32+4C3C2+3C3C2))e(k)4+(20C42−16C22C3+6C23+6C2C4−12C2C3C2+4C4C2−10C3C22−2(H1(4C22−3C3)+H2(12C22−6C3))C22−(−2H1C2−4H2C2)(−12C32+4C2C3+3C3C2)−(H0+H1+H2)(44C42−24C22C3+6C23+6C2C4−18C2C3C2+4C4C2−16C3C22))e(k)5+(−C42−26C52+43C32C3−24C2C23−24C22C4+9C3C4+8C2C5+26C22C3C2−14C23C2−17C2C4C2+5C5C2+25C2C3C22−14C4C22+20C3C32+8C4C3−20C3C2C3−2(H1(−2C32+6C2C3−4C4+4C3C2)+H2(−20C32+18C2C3−8C4+14C3C2))C22−(H2(12C22−6C3)+H1(4C22−3C3))(−12C32+4C2C3+3C3C2) |
−(H0+H1+H2)(−C42−110C52+91C32C3−36C2C23−36C22C4+9C3C4+8C2C5+62C22C3C2−23C23C2−25C2C4C2+5C5C2+57C2C3C22−22C4C22+56C3C32+8C4C3−32C3C2C3))e(k)6+O(e(k)7). | (3.14) |
Fixing H0=(2+H2)I and H1=−(1+2H2), the error equation becomes
e(k+1)=−8(−1+H2)C42e(k)5+O(e(k)6). |
Finally, making H2=1, we obtain
e(k+1)=(24C52−4C3C32)e(k)6+O(e(k)7), |
and the proof is complete.
Once the conditions that the weight function must meet have been obtained, there are several ways to define the matrix weight function H, and each of them generates a different iterative family.
Family 1. The weight function defined by the polynomial
H(τ)=β(τ−2I)2−τ+3I, |
where β∈R satisfies the hypothesis of Theorem 3.1 and τ(k)=I+[F′(x(k))]−1[z(k),y(k);F][I+(I−[F′(x(k))]−1[y(k), x(k);F])2]−1, generates a new parametric class of fifth order for β≠1 and a simple scheme of sixth order for β=1,
y(k)=x(k)−F′(x(k))−1F(x(k)),z(k)=y(k)−[I+(I−[F′(x(k))]−1[x(k),y(k);F])2]−1F′(x(k))−1F(y(k)),x(k+1)=z(k)−[β(τ−2I)2−τ+3I]F′(x(k))−1F(z(k)),k≥0. | (3.15) |
This family is denoted by PM(β)KEp depending on the β parameter.
Family 2. The weight function defined by
H(τ)=(2+β)τ−1, |
where β∈R also satisfies the hypothesis of Theorem 3.1, generates a new class of fifth and sixth order with β≠1 and β=1, respectively.
y(k)=x(k)−F′(x(k))−1F(x(k)),z(k)=y(k)−[I+(I−[F′(x(k))]−1[x(k),y(k);F])2]−1F′(x(k))−1F(y(k)),x(k+1)=z(k)−(2+β)τ−1F′(x(k))−1F(z(k)),k≥0, | (3.16) |
with τ(k)=I+[F′(x(k))]−1[z(k),y(k);F][I+(I−[F′(x(k))]−1[y(k), x(k);F])2]−1. In the following we will use the family 1 (PM(β)KEp) for all the numerical studies. The algorithm of this class is presented.
Algorithm:
● Step 1: Knowning the nonlinear system to be solved, defined by function F, and its related Jacobian matrix F′, an initial estimation x(0), a small constant tol as the accuracy, let k=0, and start the iteration process.
● Step 2: Apply iterative expression 3.17 to get the next iterate, x(1).
● Step 3: If ‖F(x(1))‖<tol, we stop and output x(1) as the solution. Otherwise, set k=k+1, go to Step 2 and continue the iteration process.
In this section, we study the performance of the vectorial rational function obtained by applying iterative class PM(β)KEp on p(x)={x21−1,x22−1} for x=(x1,x2)∈R2. Because of the dependence of this family on the beta parameter, it is important to study the existence of fixed points different from the roots of the system p(x)=0, mainly those with attractive character and other attractive structures that can be interesting from the dynamical point of view. Through this analysis, those members whose only behavior is the convergence to the roots will be derived.
So, by applying PM(β)KEp on p(x), we get its rational vectorial operator G(x,β)={g1(x,β),g2(x,β)}, whose j-th coordinate is
gj(x,β)=18xj3(17xj4−2xj2+1)6(−β(xj2−1)3(169xj6−51xj4+11xj2−1)(409xj8+84xj6+14xj4+4xj2+1)2+2(17xj4−2xj2+1)2(−1+16xj2−135xj4+944xj6−4474xj8+21008xj10−67422xj12+204304xj14−335157xj16+374304xj18+68757xj20)). | (4.1) |
Taking into account the last result, it is possible to formulate the following theorem about the stability of fixed points of G(x,β).
Theorem 4.1. The duples (1,1),(1,−1),(−1,1),(−1,−1) are superattractive fixed points of the rational function G(x,beta) which are zeroes of p(x). In addition, denoting by B the set of strange fixed points of G(x,β), it is defined by (li,lj), (±1,lj) and (li,±1) for i,j≤26, whose elements different from ±1 are real zeroes of
l(t,β)=(153359006+28270489β)xj26+(−189944026−53459981β)xj24+(157699748+23572126β)xj22+(−80245084+748834β)xj20+(34849386+406419β)xj18+(−11271950+333817β)xj16+(3284120+129684β)xj14+(−758824−6964β)xj12+(159762+5039β)xj10+(−26838+565β)xj8+(3972−18β)xj6+(−444−14β)xj4+(38+5β)xj2−2−β. |
Hence, the amount of fixed points in B depends on β:
i) If β∈(−∞,−61.956], B is composed by thirty-six repulsive and twenty-four saddle strange fixed points.
ii) If β∈(−61.956,−5.4247), B has the same composition as the previous item.
iii) If β∈(−5.4247,−4.8788), B is composed by sixteen repulsive and sixteen saddle strange fixed points.
iv) If β∈[−4.8788,−2), the composition of B is the same as the previous item.
v) For β∈[−2,−0.9328), there exist sixty strange fixed points, whose characters depend on β. Because of their stability, two different situations can be meet:
a) If β∈[−2,−0.9578), B has the same composition as the first item.
b) When β∈(−0.9578,−0.9329), B has twelve attractor, sixteen repulsive and thirty-two saddle strange fixed points.
vii) If β∈(−0.9329,+∞), B has four repulsive and eight saddle strange fixed points.
Proof. G(x,β) is symmetric with respect to its component functions. Considering that the fixed points are solutions of the equation gj(x,β)=xj, j=1,2, we get
(x2j−1)l(xj,β)=0,j=1,2, | (4.2) |
where
l(xj,β)=−β−2+(+38+38+5β)xj2+(−444−14β)xj4+(3972−18β)xj6+(−26838+565β)xj8+(159762+5039β)xj10+(−758824−6964β)xj12+(+3284120+129684β)xj14+(−11271950+333817β)xj16+(34849386+406419β)xj18+(−80245084+748834β)xj20+(157699748+23572126β)xj22+(−189944026−53459981β)xj24+(153359006+28270489β)xj26. |
Considering (4.2), it is observed that xj=±1 are solutions of this equation, and therefore, (1,1), (1,−1), (−1,1), (−1,−1) are fixed points of the rational operator G(x,β) and at the same time roots of the polynomial p(x). In order to study the stability of the rational operator, the diagonal matrix form of the associated Jacobian G′(x,beta) is computed:
G′(x,β)=(J1(x1,β)00J2(x2,β)), |
where
Jj(xj,β)=−(xj−1)2(xj+1)28xj4(17xj4−2xj2+1)7r(xj), j=1,2, | (4.3) |
and
r(xj)=(480598313β−675606282)xj28+(1615581902β+3201012552)xj26+(1326760599β−1571407002)xj24+(869157440−288459620β)xj22+(74267881β−295949922)xj20+(17885090β+109500040)xj18+(−5710273β−34047410)xj16+(212808β+10036672)xj14+(148099β−2525438)xj12+(522200−54734β)xj10+(−5971β−90670)xj8+(1884β+11776)xj6+(−533β−1302)xj4+(30β+88)xj2−3β−6. |
It is straightforward that Jj(±1,β)=0, j=1,2, for any β, and therefore the zeroes of p(x) are superattracting fixed points, given by the fact that the eigenvalues of G′((±1,±1),β) are null.
Fixed points can be calculated through l(t,β) by means of s=t2. A 13-th degree polynomial is then obtained,
L(s,β)=(153359006+28270489β)s13+(−189944026−53459981β)s12+(157699748+23572126β)s11+(−80245084+748834β)s10+(34849386+406419β)s9+(−11271950+333817β)s8+(3284120+129684β)s7+(−758824−6964β)s6+(159762+5039β)s5+(−26838+565β)s4+(3972−18β)s3+(−444−14β)s2+(38+5β)s−2−β. |
The operator G(x,beta) has real fixed points that are symbolized as Li, with Li any real and positive root of L(s). Therefore, the amount of components of B is related to the number of real and positive roots of L(s), as well as their combinations with ±1. Then,
i) Three real and positive roots L1, L2, L3 are found if β∈(−∞,−61.956], and the roots {+√L1,+√L2,+√L3,−√L1,−√L2,−√L3} are denoted by li for i∈{1,2,3,4,5,6}. The set of all strange fixed points is obtained combining in pairs li, i=1,2,…,6 with themselves and with 1 and −1. In the first case, we have that all combined pairs (li,lk) are 36, in the second the kind of (li,±1),(±1,li) pair are 12+12=24. The information about stability of these fixed points of G(x,β) can be inferred from the analysis of the absolute value of the eigenvalues of matrix G′((li,lk),(β)), i,k∈{1,2,3,4,5,6}; these functions of β are called stability functions of the respective fixed points. Due to the nature of the polynomial system, the eigenvalues λ((li,lk),(β))=J1((li,lk),(β))=J2((li,lk),(β)) for i,k∈{1,2,3,4,5,6}; however, if any of the components of the fixed point is ±1, the corresponding eigenvalue is always null.
Figure 1 shows the values of |λ| associated to the Jacobian matrix and evaluated at li for i∈{1,2,3,4,5,6}. For all of them the corresponding absolute values of λ are greater than one, so the behavior of the strange fixed points (li,lk) is repulsive for the current interval. Indeed, fixed points (li,±1),(±1,li), for i∈{1,2,3,4,5,6}, are saddle points, as one of the eigenvalues is zero, and the another one is greater than one.
ii) The amount of fixed points for β∈(−61.956,−5.4247) is the same as in the previous case, due to the existence of three real and positive roots. The information about qualitative behavior of fixed points can be extracted from the stability functions represented in Figure 2. For all of them, the corresponding absolute values of λ are greater than one, and therefore the pair (li,±1),(±1,li) are saddle points for i∈{1,2,3,4,5,6}.
iii) If β∈(−5.4247,−4.8788) the polynomial L(s) has two real positive roots L1, L2, and the elements {+√L1,+√L2,−√L1,−√L2} are denoted by li for i∈{1,2,3,4}. The analysis of stability functions associated to the fixed points in Figure 3, shows that the pairs (li,lk) for i,k∈{1,2,3,4} are repulsive, because their corresponding |λ| are greater than one, so (li,±1) and (±1,li) are saddle fixed points.
iv) For β∈[−4.8788,−2), the same structure and the same reasoning as in the previous case take place; see Figure 4.
v) As the first item, in this case L(s) has three real positive roots, and the elements li are denoted in the same way for i∈{1,2,3,4,5,6}. For some values of β, there are attractive fixed points, and such values can be calculated by solving the equation |lambdaj((li,lk),(β))|=1, for i,k∈{1,2,3,4,5,6} and j=1,2. As a result we have found that the values of β1≈−0.9578 and β2≈−0.9328 satisfy the above equation, related to the strange fixed points (li,lk) with i,k, Figure 5c, d.
a) For β∈[−2,β1) all thirty-six pairs (li,lj) are repulsive fixed points, as absolute values of |λj((li,lk),(β))|>1 for i,k∈{1,2,3,4,5,6} and j=1,2. On the other hand, pairs like (li,±1), (±1,li) are saddle fixed points, and their quantity is twenty-four for i∈{1,2,3,4,5,6}. The stability of any of these fixed points can be inferred from Figure 5.
b) There are not hyperbolic points for the values β=β1,β2. When β∈(β1,β2) the stability of fixed points (li,±1), (±1,lk) and (li,lk) for i,k∈{2,5} correspond to a Jacobian matrix whose eigenvalues take values |λj((li,±1),(β))|<1, |λj((li,±1),(β))|=0 and |λj((li,lk),(β))|<1, |λj((li,lk),(β))|=0 for j={1,2}, so they are attracting, and their quantity is twelve. On the other hand, all forty-eight pairs (l2,li), (li,l2), (l5,li), (li,l5), (±1,li) and (li,±1) are saddle fixed points due to the fact that |λj((li,lk),(β))|>1, for i∈{1,3,4,6}, Figure 5.
vi) Finally, when β∈(−0.9328,+∞) the polynomial L(s) has one real positive root L1, and the elements {+√L1,−√L1} are denoted by li for i∈{1,4}. The four fixed points (li,lk) for i,k∈{1,4} and j=1,2 are repulsive, as absolute values of |λj((li,lk),(β))|>1. On the other hand, pairs such as (l1,±1), (±1,l1), (l4,±1) (eight in total) are saddle fixed points due to the fact that λj((±1,±1),β)=0 and |λj((li,lk),β)|>1 (see Figure 6).
The stability of strange fixed points is already known, and it has been shown that attractive fixed points only appear for β at(−0.9578,−0.9329). However, in addition to the latter, there are other attractive structures whose values of the β parameter should also be avoided to achieve stable performance of the iterative methods. These structures must be sought in basins of attraction in which the free critical points, those different from the roots of p(x)=0, are also found. Related to the latter, we can make the following statement about the location of the critical points.
Theorem 4.2. Let K denote the set of free real critical points of G(x,β) related to iterative family 2 and let the values {α1=−0.46806,α2=6.28814,α3=66.1728} for the real roots collection of a 23th-degree polynomial.
Then, K is the collection of the duples (qi,qj), (qi,±1) and (±1,qj) for i,j≤10 whose entries different from ±1 are the real zeroes of polynomial
r(t)=(480598313β−675606282)t28+(1615581902β+3201012552)t26+(1326760599β−1571407002)t24+(869157440−288459620β)t22+(74267881β−295949922)t20+(17885090β+109500040)t18+(−5710273β−34047410)t16+(212808β+10036672)t14+(148099β−2525438)t12+(522200−54734β)t10+(−5971β−90670)t8+(1884β+11776)t6+(−533β−1302)t4+(30β+88)t2−3β−6. |
Then, K is made up as follows:
i) If β∈(−∞,−2], K contains 12 free critical points.
ii) If β∈(−2,−0.5], K contains 32 free critical points.
iii) If β∈(−0.5,α1], K contains 100 free critical points.
iv) If β∈(α1,1.40576), K contains 32 free critical points.
v) If β∈(1.40576,α2), β∈[α2,α3) or β∈(α3,+∞), K contains 12 free critical points.
Proof. Is known from Theorem 4.1 that the eigenvalues of Jacobian matrix G′(x,β) are λj(x,β)=Jj(x,β), for j∈{1,2}, that is,
λj(x,β)=−(xj−1)2(xj+1)28x14(17x14−2xj2+1)7r(xj), j=1,2, | (4.4) |
where
r(t)=(480598313β−675606282)t28+(1615581902β+3201012552)t26+(1326760599β−1571407002)t24+(869157440−288459620β)t22+(74267881β−295949922)t20+(17885090β+109500040)t18+(−5710273β−34047410)t16+(212808β+10036672)t14+(148099β−2525438)t12+(522200−54734β)t10+(−5971β−90670)t8+(1884β+11776)t6+(−533β−1302)t4+(30β+88)t2−3β−6. | (4.5) |
On the other hand, the critical points are the solutions of equation λj(x,α)=0 for j∈{1,2} that are found by using t2=s on r(t). Its roots (real and positive, symbolized as Qj) will be the entries of the free critical points, as qk=±√Qj. The quantity of positive roots depends on the value of parameter β, forcing Qj to be real:
(i) When β∈(−∞,−2], r(s) has one real positive root, denoted by Q1. Then, K is composed by (q1,q1),(q1,q5),(q5,q1) and (q5,q5), where q1,5=±√Q1, and also by eight critical points of the kinds (qj,±1) and (±1,qj), j∈{1,5}.
(ii) If β∈(−2,−0.5], r(s) has only two positive real roots Q1 and Q2. Therefore, K={(qi,qj),(qi,±1),(±1,qj) : i,j∈{1,2,5,6}}, holding 32 different free critical points.
(iii) For β∈(−0.5,α1], there exist four real positive roots of polynomial r(s): Q1, Q2, Q3 and Q4. So, K has the elements of kind (qi,qj), with i,j∈{1,2,…,8}, where q1,5=±√Q1, q2,6=±√Q2, q3,7=±√Q3 and q4,8=±√Q4. In addition, mixed points (±1,qj) and (qi,±1) where i,j∈{1,2,…,8} also belong to K. The total quantity of critical points is 100.
iv) When β∈(α1,1.40576), r(s) has two real positive roots, and the K composition is the same as in (ii).
v) If β∈(1.40576,α2), β∈[α2,α3) or β∈(α3,+∞), r(s) has one real positive root (different in each case), and the K composition is the same as in (i).
Theorems 4.1 and 4.2 give us information about stability of the iterative class acting on a certain test polynomial. In general, elements of the strange fixed and critical point collections should be avoided as initial estimates.
However, from the dynamic point of view, the analysis of the long-term performance of free critical points can give us the key about other strange attractive structures, periodic orbits or even chaotic behavior.
To take a first glance to the study of the critical points we are going to use the tool named parameter line, first used in [21].
This tool consists of a mesh of 500×500 points in a specific interval of the β parameter. Each parameter-dependent free critical point used as initial estimate is colored red, if after 200 iterations it converges to some root of the polynomial p(x), and black in any other case. Each of these points is thickened by a multiplication by [0,1]. The error tolerance is 10−3. The red color is more clear when the number of iterations needed to converge is lower.
Figure 7 shows the limit performance of free critical points for β∈(−2,−0.5]. In Figure 7a, all the free critical points (qi,qj) with i,j∈{1,5} converge to any of the roots of p(x); moreover, in Figure 7b, unstable behavior is discovered for critical points (qi,qj) with i,j∈{2,6}, (q1,qm) for m∈{2,6} and (q2,qn) for n∈{1,5} around the values β≈−1.75 and β≈−0.95.
We continue the study of the unstable performance in the two dark zones using bifurcation diagrams. They are calculated using each free critical point of the rational operator as a seed, for β∈(−2,0.5], in a mesh of 3000 subintervals. In this interval they are real, and we observe their performance in the last 100 of 1000 iterations. In Figure 8, detailed bifurcation diagrams of critical point (q2,q6) are shown. They are related with the dark zones of no convergence in the parameter lines.
Because of polynomial p(x) has separate variables, the coordinate functions of the rational operator are symmetrical. Taking it into account, in the bifurcation diagrams the x1 and x2 dimensions are plotted in blue and red color, respectively. For the details, only positive coordinates were chosen.
Observing the details of bifurcation diagrams given by Figure 8b, e, we can find patterns of bifurcations, such as period doubling cascade, periodic orbits and chaotic behaviors for β≈−1.76,−1.762 and −1.765 in the first case and for β≈−0.96,−0.97 and −0.986 in the second case, respectively. In the following we will find and show some of these pathological attractive structures previously observed in the bifurcations diagrams.
To take a full view of dynamic behavior of the rational operator G(x,β) we plotted a dynamical plane, and these graphics are constructed by iterating each point of a mesh with a 0.01 step and painting according to the root to which they converge, with an error tolerance lower than 10−3 or with a maximum of 50 iterations as a stopping criterion. The brighter the color is the smaller the amount of iteration needed to converge. In case of non-convergence after the number of iterations is completed, the point is colored black. The roots of the polynomial are represented with circles.
Related with the first dark zone on parameter line (see Figure 7b), in Figure 9a, we can see that the amount of connected components in the basins of attraction of the roots for β=−1.7665 is infinite. Green and white diamonds represent the repulsive and saddle strange fixed points, respectively. All of them lie in the Julia set. On the other hand, free critical points are represented with yellow squares. Some of them lie in the basin of attraction of the roots of p(x), and the rest are located in the black zone. Observing the bifurcation diagram in Figure 8b and knowing the position of critical point (q2,q6), we can explore the regions around points (∓0.48,±0.48) to find attractive elements. In Figure 9a, we can observe an attractive structure (see small white region around the points (∓0.48,±0.48)). Any initial estimation in those zones tends to densely fill these same symmetrical regions. The details of these small regions can be observed in Figure 9b.
On the other hand, for the value of β=−1.7621 (see the bifurcation diagram, Figure 8b), we find periodic orbits around critical point (q2,q6). One of them, with period 8, is shown in Figure 9c, and its elements are
{(−0.4968,0.4968),(0.4756,−0.4756),(−0.4938,0.4938),(0.4829,−0.4829),(−0.4966,0.4966),(0.4763,−0.4763),(−0.4945,0.4945),(0.4814,−0.4814)}. |
Its details can be seen in Figure 9d.
Finally, by studying the second dark zone of the parameter line in Figure 7b, it is important to note in Figure 8d, e the same color of the bifurcation patterns. This means that the x1 and x2 coordinates maintain the same sign in any attractive structure, that is, each of those attractive elements must be confined in small areas where the coordinates sign of its points do not change. In Figure 10a, for β=−0.986, this behavior can be seen in a small area around critical point (q2,q6) and the coordinates (∓0.48,∓0.48) where every iteration of initial estimates tend densely to fill this area. Details are shown in Figure 10b. Meanwhile, observing the bifurcation diagram in Figure 8e for β=−0.97 we find periodic orbits of period four, and one example can be seen in Figure 10c, d whose components are (0.4589,−0.4589),(0.4891,−0.4891),(0.4543,−0.4543),(0.5010,−0.5010).
In what follows, we show the behavior of PM(β)KEp (described by (3.15)) on some real-life examples and in comparison with the Newton method. The β values used (β=1,2) provides convergence of order 6 and 5, respectively, in accordance with the obtained results of Theorem 3.1. To develop the numerical test, we use MATLAB software with variable precision arithmetic and 2000 digits of mantissa. The experiments have been performed on a computer with Intel(R) Core(TM) i7-1065G7 CPU and 16 GB of RAM. The stopping criterion used is ‖F(x(k+1))‖<10−200 or ‖x(k+1)−x(k)‖<10−200. The first order divided difference operator used has the elements given by the expression (see [22])
[d,c;F]ij = fi( d1, ..., dj−1, dj, cj+1, ..., am )−fi( d1, ..., dj−1, cj, cj+1, ..., cm )dj−xj,1≤i,j≤n, |
being fi, i=1,2,…,n, the coordinate functions of F. For each system, one table of results is displayed, where x(0) is the initial approximation, k is the number of iterations needed to converge ("nc" appears in the table if the method does not converge), the value of residuals ‖F(x(k+1))‖ or ‖x(k+1)−x(k)‖ at the last iterate ("-" if there is no convergence), and ACOC [12] is
ACOC=ln( ‖ x(k+1) − x(k) ‖ ‖ x(k) − x(k − 1) ‖ )ln( ‖ x(k) − x(k − 1) ‖ ‖ x(k − 1) − x(k − 2) ‖ ). |
In relation to the latter, it is necessary to determine when the root(‖F(x(k+1))‖<10−200 is achieved) is reached or if it is only a very slow convergence with a no significant difference between the two last iterates (‖x(k+1)−x(k)‖<10−200 but ‖F(x(k+1))‖>10−200), or both criteria are satisfied. The nonlinear test systems used are the following:
Example1. In this example, with the use of PM(1)KEp, we will study the equation of heat conduction [28] for a non-homogeneous medium :∂∂x(k(u)∂u∂x)+F(x,t)=cρ∂u∂t, with F(x,t) the thermal source density and k, c and ρ the material quantitative properties of the thermal conductivity coefficient, specific heat capacity and medium density, respectively.
For the sake of simplicity we are taking c, ρ as constants and the thermal conductivity coefficient as a linear function of temperature k(u)=k0+k1u with k0=1. The thermal sources have been distributed equispaced throughout the space-time grid, and their signs have been chosen randomly. After a few transformations and adding some boundary and initial conditions, we state the problem to be studied:
{αu2x+(1+αu)uxx+f=ut,α=k1cρ,f=Fcρ,u(x0,t)=0,u(xf,t)=sin2(t),u(x,0)=sech2(7x), |
where f=f(x,t) is obtained from F(x, t) (the thermal source density) after the transformations leading to the definition of the resulting boundary problem. By applying an implicit finite differences scheme, we transform the equation of heat conduction in a family of nonlinear systems to be solved. The estimated solution for each instant tk is obtained from the approximation calculated at tk−1. The space-time mesh is constructed taking the spatial step h=8nx and the timing step l=Tmaxnt, with nx, nt and Tmax the amount of subintervals for variables x, t and final instant of the numerical study, respectively.
Making x0=−4 and xf=4, we get the mesh domain as [x0,xf]×[0,Tmax] with (nx+1)×(nt+1) equispaced nodes (xi,tk). Denoting by ui,k the estimation of the solution at the (xi,tk) and using the estimations of the derivatives
ut≈u(x,t)−u(x,t−h)h,ux≈u(x+h,t)−u(x,t)land uxx≈u(x+h,t)−2u(x,t)+u(x−h,t)l, |
we construct the nonlinear system with α=1,
kui+1,k−(h2+k(2+α(ui+1,k−ui−1,k)))ui,k−kαu2i,k+k(ui+1,k+αu2i+1,k)+f=−h2ui,k−1. |
The results for different discretization and thermal sources are shown in Table 1. The mean of the number of iterations employed for solving the nonlinear systems for each instant is denominated as "iter" and ‖F(x(k+1))‖ is the norm of the solution of the last system. As the number of iterations employed for solving each system is very low, the ACOC cannot be calculated.
Tmax | nx | nt | iter | ‖F(x(k+1))‖ | f | Tmax | nx | nt | iter | ‖F(x(k+1))‖ | f | |
0.5 | 20 | 10 | 1.9091 | 4.6694×10−31 | 0 | 0.5 | 20 | 10 | 1.9091 | 1.4974×10−26 | 2 | |
0.5 | 20 | 20 | 1.4762 | 6.9684×10−8 | 0 | 0.5 | 20 | 20 | 1.9048 | 4.9125×10−28 | 2 | |
1.0 | 20 | 10 | 1.9091 | 3.1137×10−25 | 0 | 1.0 | 20 | 10 | 1.9091 | 3.0552×10−20 | 2 | |
1.0 | 40 | 20 | 1.9524 | 7.2903×10−32 | 0 | 1.0 | 40 | 20 | 1.9524 | 2.9880×10−26 | 2 | |
5.0 | 20 | 10 | 2.1818 | 3.3624×10−10 | 0 | 5.0 | 20 | 10 | 2.1818 | 2.3446×10−11 | 2 | |
5.0 | 40 | 20 | 2.1905 | 1.3758×10−12 | 0 | 5.0 | 40 | 20 | 2.1905 | 1.3758×10−12 | 2 | |
0.5 | 20 | 10 | 1.9091 | 1.5948×10−18 | 5 | 0.5 | 20 | 10 | 1.9091 | 5.4491×10−13 | 10 | |
0.5 | 20 | 20 | 1.9048 | 3.4320×10−22 | 5 | 0.5 | 20 | 20 | 1.9048 | 5.6519×10−17 | 10 | |
1.0 | 20 | 10 | 1.9091 | 9.9130×10−17 | 5 | 1.0 | 20 | 10 | 1.9091 | 6.5624×10−13 | 10 | |
1.0 | 40 | 20 | 1.9524 | 2.8640×10−21 | 5 | 1.0 | 40 | 20 | 1.9524 | 8.2734×10−18 | 10 | |
5.0 | 20 | 10 | 2.1818 | 1.1617×10−11 | 5 | 5.0 | 20 | 10 | nc | nc | 10 | |
5.0 | 40 | 20 | 2.2381 | 1.0439×10−19 | 5 | 5.0 | 40 | 20 | nc | nc | 10 |
In Figure 11 are shown the approximated solutions of the head equation for different thermal sources. See the influence of f(x,t) in Figure 11b–d, compared with Figure 11a.
Table 1 and Figure 11 show that the scheme is robust enough to reproduce with effectiveness the behavior of the model for different values of thermal sources density.
Example2. This system has variable size and has the solution ξ≈(0.5149,...,0.5149)T. It is defined by
xi−cos(2xi−4∑j=1xj)=0,i=1,2,…,n, |
with n=5, and the initial estimation is x(0)=(0.75,...,0.75)T. The results appear in Table 2.
x(0) | Method(β) | k | ‖x(k+1)−x(k)‖ | ‖F(x(k+1))‖ | ACOC |
PM(1)KEp | 3 | 53.9112×10−36 | 334.579×10−210 | 5.7320 | |
(0.75,...,0.75) | PM(2)KEp | 4 | 6.2324×10−141 | 0.0 | 5 |
N | 11 | 157.9286×10−162 | 11.4870×10−321 | 2 |
In there, it can be observed that the PM(β)KEp converges to the solution in fewer iterations than Newton's method, as we might expect. In the case of PM(2)KEp the convergence occurs with null (for the fixed precision of the machine at 2000 digits) or almost null residual ‖F(x(k+1))‖ for PM(2)KEp and the parameter values involved. In addition, the ACOC is in good accordance with the theoretical one, as we can expect due to Theorem 3.1.
Example3. Next system of size n=10 is defined as
arctan(xi)+1−2(n∑j=1x2j−x2i)=0,i=1,2,…,n, |
whose solution is ξ≈(0.1758,...,0.1758)T, and the seed used is x(0)=(1.0,...,1.0)T. Results are given in Table 3. The PM(β)KEp family converges with null residuals ‖F(x(k+1))‖ in fewer iterations than Newton's method, and the ACOC is in total agreement with the theoretical one.
x(0) | Method(β) | k | ‖x(k+1)−x(k)‖ | ‖F(x(k+1))‖ | ACOC |
PM(1)KEp | 5 | 20.4411×10−144 | 0.0 | 6 | |
(1.0, ..., 1.0) | PM(2)KEp | 5 | 250.020×10−111 | 0.0 | 5 |
N | 12 | 168.3834×10−108 | 163.4591×10−213 | 2 |
Example4. Finally, we test the PM(β)KEp family with a nonlinear system of variable size described as
−xi+n∑j=1xj−xiexi=0,i=1,2,…,n, |
with n=20. The initial estimation x(0)=(0.25,...,0.25)T, and the solution is ξ=(0.0,...,0.0)T. The results are given in Table 4. The results are similar to those obtained in Example 2, with fewer iterations than Newton's method, null (for the fixed precision of the machine at 2000 digits) or almost null residual ‖F(x(k+1))‖ and the computational approximation order of convergence in good accordance with the theoretical one.
x(0) | Method(β) | k | ‖x(k+1)−x(k)‖ | ‖F(x(k+1))‖ | ρ |
PM(1)KEp | 3 | 16.2213×10−51 | 5.8210×10−306 | 6.0643 | |
(0.25, ..., 0.25) | PM(2)KEp | 4 | 36.0237×10−192 | 0.0 | 5.0781 |
N | 11 | 174.2412×10−201 | 0.0 | 2 |
In this manuscript, a class of iterative schemes with sixth-order convergence has been designed. Its construction has been made using the Ermakov-Kalitkin approach, which improves the wideness of the set of converging initial guesses. The analysis of the stability of the resulting class has proven the inclusion of very stable members, which have been used in the numerical section to solve real-life and academical problems.
This research was partially supported by Grant PGC2018-095896-B-C22 funded by MCIN/AEI/31000.13039/ "ERDF A way to making Europe", European Union.
There is no conflict of interest regarding publication of this manuscript.
[1] | C. Goodrich, A. C. Peterson, Discrete fractional calculus, Springer, New York, 2015. https://doi.org/10.1007/978-3-319-25562-0 |
[2] | F. M. Atici, P. W. Eloe, A transform method in discrete fractional calculus, Int. J. Differ. Equ., 2 (2007), 165–176. |
[3] |
H. M. Srivastava, P. O. Mohammed, C. S. Ryoo, Y. S. Hamed, Existence and uniqueness of a class of uncertain Liouville-Caputo fractional difference equations, J. King Saud Univ. Sci., 33 (2021), 101497. https://doi.org/10.1016/j.jksus.2021.101497 doi: 10.1016/j.jksus.2021.101497
![]() |
[4] |
C. Lizama, The Poisson distribution, abstract fractional difference equations, and stability, Proc. Amer. Math. Soc., 145 (2017), 3809–3827. https://doi.org/10.1090/proc/12895 doi: 10.1090/proc/12895
![]() |
[5] |
C. Lizama, M. Murillo-Arcila, Well posedness for semidiscrete fractional Cauchy problems with finite delay, J. Comput. Appl. Math., 339 (2018), 356–366. https://doi.org/10.1016/j.cam.2017.07.027 doi: 10.1016/j.cam.2017.07.027
![]() |
[6] |
H. M. Srivastava, P. O. Mohammed, J. L. G. Guirao, Y. S. Hamed, Link theorem and distributions of solutions to uncertain Liouville-Caputo difference equations, Discrete Contin. Dyn. Syst., 15 (2021), 427–440. http://dx.doi.org/10.3934/dcdss.2021083 doi: 10.3934/dcdss.2021083
![]() |
[7] | İlhane, E. Analysis of the spread of Hookworm infection with Caputo-Fabrizio fractional derivative, Turk. J. Sci., 7 (2022), 43–52. |
[8] |
M. A. Ragusa, Parabolic Herz spaces and their applications, Appl. Math. Lett., 25 (2022), 1270–1273. https://doi.org/10.1016/j.aml.2011.11.022 doi: 10.1016/j.aml.2011.11.022
![]() |
[9] |
S. Rezapour, A. Boulfoul, B. Tellab, M. E. Samei, S. Etemad, R. George, Fixed point theory and the Liouville-Caputo integro-differential FBVP with multiple nonlinear terms, J. Funct. Spaces, 2022 (2022), 6713533. https://doi.org/10.1155/2022/6713533 doi: 10.1155/2022/6713533
![]() |
[10] |
T. Abdeljawad, On Riemann and Caputo fractional differences, Comput. Math. Appl., 62 (2011), 1602–1611. https://doi.org/10.1016/j.camwa.2011.03.036 doi: 10.1016/j.camwa.2011.03.036
![]() |
[11] |
T. Abdeljawad, Q. M. Al-Mdallal, M. A. Hajji, Arbitrary order fractional difference operators with discrete exponential kernels and applications, Discrete Dyn. Nat. Soc., 2017 (2017), 4149320. https://doi.org/10.1155/2017/4149320 doi: 10.1155/2017/4149320
![]() |
[12] | T. Abdeljawad, F. Jarad, A. Atangana, P. O. Mohammed, On a new type of fractional difference operators on h-step isolated time scales, J. Fract. Calc. Nonlinear Syst., 1 (2020), 46–74. |
[13] |
T. Abdeljawad, Different type kernel h-fractional differences and their fractional h-sums, Chaos Soliton. Fract., 116 (2018), 146–156. https://doi.org/10.1016/j.chaos.2018.09.022 doi: 10.1016/j.chaos.2018.09.022
![]() |
[14] | R. A. C. Ferreira, D. F. M. Torres, Fractional h-difference equations arising from the calculus of variations, Appl. Anal. Discrete Math., 5 (2011), 110–121. |
[15] |
C. R. Chen, M. Bohner, B. G. Jia, Ulam-Hyers stability of Caputo fractional difference equations, Math. Meth. Appl. Sci., 42 (2019), 7461–7470. https://doi.org/10.1002/mma.5869 doi: 10.1002/mma.5869
![]() |
[16] |
G. Wu, D. Baleanu, Discrete chaos in fractional delayed logistic maps, Nonlinear Dyn., 80 (2015), 1697–1703. https://doi.org/10.1007/s11071-014-1250-3 doi: 10.1007/s11071-014-1250-3
![]() |
[17] | F. Atici, S. Sengul, Modeling with discrete fractional equations, J. Math. Anal. Appl., 369 (2010), 1–9. |
[18] |
B. Ghanbari, On the modeling of the interaction between tumor growth and the immune system using some new fractional and fractional-fractal operators, Adv. Differ. Equ., 2020 (2020), 585. https://doi.org/10.1186/s13662-020-03040-x doi: 10.1186/s13662-020-03040-x
![]() |
[19] |
R. Dahal, C. S. Goodrich, A monotonicity result for discrete fractional difference operators, Arch. Math., 102 (2014), 293–299. https://doi.org/10.1007/s00013-014-0620-x doi: 10.1007/s00013-014-0620-x
![]() |
[20] |
P. O. Mohammed, T. Abdeljawad, F. K. Hamasalh, On Riemann-Liouville and Caputo fractional forward difference monotonicity analysis, Mathematics, 9 (2021), 1303. https://doi.org/10.3390/math9111303 doi: 10.3390/math9111303
![]() |
[21] |
C. S. Goodrich, M. Muellner, An analysis of the sharpness of monotonicity results via homotopy for sequential fractional operators, Appl. Math. Lett., 98 (2019), 446–452. https://doi.org/10.1016/j.aml.2019.07.003 doi: 10.1016/j.aml.2019.07.003
![]() |
[22] |
B. Jia, L. Erbe, A. Peterson, Two monotonicity results for nabla and delta fractional differences, Arch. Math., 104 (2015), 589–597. https://doi.org/10.1007/s00013-015-0765-2 doi: 10.1007/s00013-015-0765-2
![]() |
[23] |
C. S. Goodrich, Sharp monotonicity results for fractional nabla sequential differences, J. Differ. Equ. Appl., 25 (2019), 801–814. https://doi.org/10.1080/10236198.2018.1542431 doi: 10.1080/10236198.2018.1542431
![]() |
[24] |
P. O. Mohammed, O. Almutairi, R. P. Agarwal, Y. S. Hamed, On convexity, monotonicity and positivity analysis for discrete fractional operators defined using exponential kernels, Fractal Fract., 6 (2022), 55. https://doi.org/10.3390/fractalfract6020055 doi: 10.3390/fractalfract6020055
![]() |
[25] |
P. O. Mohammed, F. K. Hamasalh, T. Abdeljawad, Difference monotonicity analysis on discrete fractional operators with discrete generalized Mittag-Leffler kernels, Adv. Differ. Equ., 2021 (2021), 213. https://doi.org/10.1186/s13662-021-03372-2 doi: 10.1186/s13662-021-03372-2
![]() |
[26] |
L. Erbe, C. S. Goodrich, B. Jia, A. Peterson, Survey of the qualitative properties of fractional difference operators: Monotonicity, convexity, and asymptotic behavior of solutions, Adv. Differ. Equ., 2016 (2016), 43. https://doi.org/10.1186/s13662-016-0760-3 doi: 10.1186/s13662-016-0760-3
![]() |
[27] |
C. S. Goodrich, A note on convexity, concavity, and growth conditions in discrete fractional calculus with delta difference, Math. Inequal. Appl., 19 (2016), 769–779. https://doi.org/10.7153/MIA-19-57 doi: 10.7153/MIA-19-57
![]() |
[28] |
C. S. Goodrich, C. Lizama, Positivity, monotonicity, and convexity for convolution operators, Discrete Contin. Dyn. Syst., 40 (2020), 4961–4983. http://dx.doi.org/10.3934/dcds.2020207 doi: 10.3934/dcds.2020207
![]() |
[29] |
C. S. Goodrich, C. Lizama, A transference principle for nonlocal operators using a convolutional approach: Fractional monotonicity and convexity, Isr. J. Math., 236 (2020), 533–589. https://doi.org/10.1007/s11856-020-1991-2 doi: 10.1007/s11856-020-1991-2
![]() |
[30] |
D. Mozyrska, D. F. M. Torres, M. Wyrwas, Solutions of systems with the Caputo-Fabrizio fractional delta derivative on time scales, Nonlinear Anal. Hybrid Syst., 32 (2019), 168–176. https://doi.org/10.1016/j.nahs.2018.12.001 doi: 10.1016/j.nahs.2018.12.001
![]() |
[31] |
P. O. Mohammed, T. Abdeljawad, F. K. Hamasalh, On discrete delta Caputo-Fabrizio fractional operators and monotonicity analysis, Fractal Fract., 5 (2021), 116. https://doi.org/10.3390/fractalfract5030116 doi: 10.3390/fractalfract5030116
![]() |
[32] |
C. S. Goodrich, J. M. Jonnalagadda, B. Lyons, Convexity, monotonicity and positivity results for sequential fractional nabla difference operators with discrete exponential kernels, Math. Meth. Appl. Sci., 44 (2021), 7099–7120. https://doi.org/10.1002/mma.7247 doi: 10.1002/mma.7247
![]() |
[33] |
P. O. Mohammed, T. Abdeljawad, Discrete generalized fractional operators defined using h-discrete Mittag-Leffler kernels and applications to AB fractional difference systems, Math. Meth. Appl. Sci., 2020. https://doi.org/10.1002/mma.7083 doi: 10.1002/mma.7083
![]() |
Tmax | nx | nt | iter | ‖F(x(k+1))‖ | f | Tmax | nx | nt | iter | ‖F(x(k+1))‖ | f | |
0.5 | 20 | 10 | 1.9091 | 4.6694×10−31 | 0 | 0.5 | 20 | 10 | 1.9091 | 1.4974×10−26 | 2 | |
0.5 | 20 | 20 | 1.4762 | 6.9684×10−8 | 0 | 0.5 | 20 | 20 | 1.9048 | 4.9125×10−28 | 2 | |
1.0 | 20 | 10 | 1.9091 | 3.1137×10−25 | 0 | 1.0 | 20 | 10 | 1.9091 | 3.0552×10−20 | 2 | |
1.0 | 40 | 20 | 1.9524 | 7.2903×10−32 | 0 | 1.0 | 40 | 20 | 1.9524 | 2.9880×10−26 | 2 | |
5.0 | 20 | 10 | 2.1818 | 3.3624×10−10 | 0 | 5.0 | 20 | 10 | 2.1818 | 2.3446×10−11 | 2 | |
5.0 | 40 | 20 | 2.1905 | 1.3758×10−12 | 0 | 5.0 | 40 | 20 | 2.1905 | 1.3758×10−12 | 2 | |
0.5 | 20 | 10 | 1.9091 | 1.5948×10−18 | 5 | 0.5 | 20 | 10 | 1.9091 | 5.4491×10−13 | 10 | |
0.5 | 20 | 20 | 1.9048 | 3.4320×10−22 | 5 | 0.5 | 20 | 20 | 1.9048 | 5.6519×10−17 | 10 | |
1.0 | 20 | 10 | 1.9091 | 9.9130×10−17 | 5 | 1.0 | 20 | 10 | 1.9091 | 6.5624×10−13 | 10 | |
1.0 | 40 | 20 | 1.9524 | 2.8640×10−21 | 5 | 1.0 | 40 | 20 | 1.9524 | 8.2734×10−18 | 10 | |
5.0 | 20 | 10 | 2.1818 | 1.1617×10−11 | 5 | 5.0 | 20 | 10 | nc | nc | 10 | |
5.0 | 40 | 20 | 2.2381 | 1.0439×10−19 | 5 | 5.0 | 40 | 20 | nc | nc | 10 |
x(0) | Method(β) | k | ‖x(k+1)−x(k)‖ | ‖F(x(k+1))‖ | ACOC |
PM(1)KEp | 3 | 53.9112×10−36 | 334.579×10−210 | 5.7320 | |
(0.75,...,0.75) | PM(2)KEp | 4 | 6.2324×10−141 | 0.0 | 5 |
N | 11 | 157.9286×10−162 | 11.4870×10−321 | 2 |
x(0) | Method(β) | k | ‖x(k+1)−x(k)‖ | ‖F(x(k+1))‖ | ACOC |
PM(1)KEp | 5 | 20.4411×10−144 | 0.0 | 6 | |
(1.0, ..., 1.0) | PM(2)KEp | 5 | 250.020×10−111 | 0.0 | 5 |
N | 12 | 168.3834×10−108 | 163.4591×10−213 | 2 |
x(0) | Method(β) | k | ‖x(k+1)−x(k)‖ | ‖F(x(k+1))‖ | ρ |
PM(1)KEp | 3 | 16.2213×10−51 | 5.8210×10−306 | 6.0643 | |
(0.25, ..., 0.25) | PM(2)KEp | 4 | 36.0237×10−192 | 0.0 | 5.0781 |
N | 11 | 174.2412×10−201 | 0.0 | 2 |
Tmax | nx | nt | iter | ‖F(x(k+1))‖ | f | Tmax | nx | nt | iter | ‖F(x(k+1))‖ | f | |
0.5 | 20 | 10 | 1.9091 | 4.6694×10−31 | 0 | 0.5 | 20 | 10 | 1.9091 | 1.4974×10−26 | 2 | |
0.5 | 20 | 20 | 1.4762 | 6.9684×10−8 | 0 | 0.5 | 20 | 20 | 1.9048 | 4.9125×10−28 | 2 | |
1.0 | 20 | 10 | 1.9091 | 3.1137×10−25 | 0 | 1.0 | 20 | 10 | 1.9091 | 3.0552×10−20 | 2 | |
1.0 | 40 | 20 | 1.9524 | 7.2903×10−32 | 0 | 1.0 | 40 | 20 | 1.9524 | 2.9880×10−26 | 2 | |
5.0 | 20 | 10 | 2.1818 | 3.3624×10−10 | 0 | 5.0 | 20 | 10 | 2.1818 | 2.3446×10−11 | 2 | |
5.0 | 40 | 20 | 2.1905 | 1.3758×10−12 | 0 | 5.0 | 40 | 20 | 2.1905 | 1.3758×10−12 | 2 | |
0.5 | 20 | 10 | 1.9091 | 1.5948×10−18 | 5 | 0.5 | 20 | 10 | 1.9091 | 5.4491×10−13 | 10 | |
0.5 | 20 | 20 | 1.9048 | 3.4320×10−22 | 5 | 0.5 | 20 | 20 | 1.9048 | 5.6519×10−17 | 10 | |
1.0 | 20 | 10 | 1.9091 | 9.9130×10−17 | 5 | 1.0 | 20 | 10 | 1.9091 | 6.5624×10−13 | 10 | |
1.0 | 40 | 20 | 1.9524 | 2.8640×10−21 | 5 | 1.0 | 40 | 20 | 1.9524 | 8.2734×10−18 | 10 | |
5.0 | 20 | 10 | 2.1818 | 1.1617×10−11 | 5 | 5.0 | 20 | 10 | nc | nc | 10 | |
5.0 | 40 | 20 | 2.2381 | 1.0439×10−19 | 5 | 5.0 | 40 | 20 | nc | nc | 10 |
x(0) | Method(β) | k | ‖x(k+1)−x(k)‖ | ‖F(x(k+1))‖ | ACOC |
PM(1)KEp | 3 | 53.9112×10−36 | 334.579×10−210 | 5.7320 | |
(0.75,...,0.75) | PM(2)KEp | 4 | 6.2324×10−141 | 0.0 | 5 |
N | 11 | 157.9286×10−162 | 11.4870×10−321 | 2 |
x(0) | Method(β) | k | ‖x(k+1)−x(k)‖ | ‖F(x(k+1))‖ | ACOC |
PM(1)KEp | 5 | 20.4411×10−144 | 0.0 | 6 | |
(1.0, ..., 1.0) | PM(2)KEp | 5 | 250.020×10−111 | 0.0 | 5 |
N | 12 | 168.3834×10−108 | 163.4591×10−213 | 2 |
x(0) | Method(β) | k | ‖x(k+1)−x(k)‖ | ‖F(x(k+1))‖ | ρ |
PM(1)KEp | 3 | 16.2213×10−51 | 5.8210×10−306 | 6.0643 | |
(0.25, ..., 0.25) | PM(2)KEp | 4 | 36.0237×10−192 | 0.0 | 5.0781 |
N | 11 | 174.2412×10−201 | 0.0 | 2 |