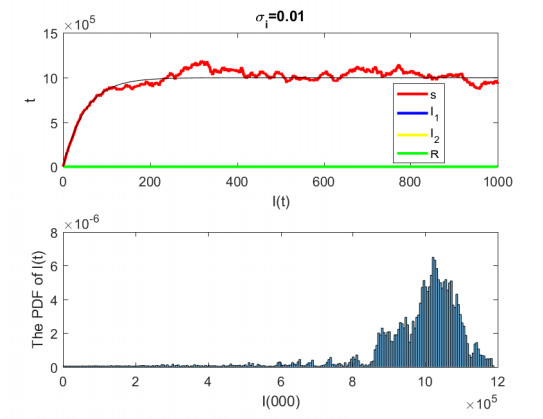
In this paper, a deterministic and stochastic model for hepatitis C with different types of virus genomes is proposed and analyzed. Some sufficient conditions are obtained to ensure the stability of the deterministic equilibrium points. We perform a stochastic extension of the deterministic model to study the fluctuation between environmental factors. Firstly, the existence of a unique global positive solution for the stochastic model is investigated. Secondly, sufficient conditions for the extinction of the hepatitis C virus from the stochastic system are obtained. Theoretical and numerical results show that the smaller white noise can ensure the persistence of susceptible and infected populations while the larger white noise can lead to the extinction of disease. By introducing the basic reproduction number R0 and the stochastic basic reproduction number Rs0, the conditions that cause the disease to die out are indicated. The importance of environmental noise in the propagation of hepatitis C viruses is highlighted by these findings.
Citation: Yousef Alnafisah, Moustafa El-Shahed. Deterministic and stochastic model for the hepatitis C with different types of virus genome[J]. AIMS Mathematics, 2022, 7(7): 11905-11918. doi: 10.3934/math.2022664
[1] | Yousef Alnafisah, Moustafa El-Shahed . Correction: Deterministic and stochastic model for the hepatitis C with different types of virus genome. AIMS Mathematics, 2022, 7(9): 15992-15993. doi: 10.3934/math.2022875 |
[2] | Nauman Ahmed, Ali Raza, Ali Akgül, Zafar Iqbal, Muhammad Rafiq, Muhammad Ozair Ahmad, Fahd Jarad . New applications related to hepatitis C model. AIMS Mathematics, 2022, 7(6): 11362-11381. doi: 10.3934/math.2022634 |
[3] | Tingting Wang, Shulin Sun . Dynamics of a stochastic epidemic model with quarantine and non-monotone incidence. AIMS Mathematics, 2023, 8(6): 13241-13256. doi: 10.3934/math.2023669 |
[4] | Xiaodong Wang, Kai Wang, Zhidong Teng . Global dynamics and density function in a class of stochastic SVI epidemic models with Lévy jumps and nonlinear incidence. AIMS Mathematics, 2023, 8(2): 2829-2855. doi: 10.3934/math.2023148 |
[5] | Abdul Qadeer Khan, Ayesha Yaqoob, Ateq Alsaadi . Neimark-Sacker bifurcation, chaos, and local stability of a discrete Hepatitis C virus model. AIMS Mathematics, 2024, 9(11): 31985-32013. doi: 10.3934/math.20241537 |
[6] | Ruoyun Lang, Yuanshun Tan, Yu Mu . Stationary distribution and extinction of a stochastic Alzheimer's disease model. AIMS Mathematics, 2023, 8(10): 23313-23335. doi: 10.3934/math.20231185 |
[7] | Yan Ren, Yan Cheng, Yuzhen Chai, Ping Guo . Dynamics and density function of a HTLV-1 model with latent infection and Ornstein-Uhlenbeck process. AIMS Mathematics, 2024, 9(12): 36444-36469. doi: 10.3934/math.20241728 |
[8] | Ruyue Hu, Chi Han, Yifan Wu, Xiaohui Ai . Analysis of a stochastic Leslie-Gower three-species food chain system with Holling-II functional response and Ornstein-Uhlenbeck process. AIMS Mathematics, 2024, 9(7): 18910-18928. doi: 10.3934/math.2024920 |
[9] | Yue Dong, Xinzhu Meng . Stochastic dynamic analysis of a chemostat model of intestinal microbes with migratory effect. AIMS Mathematics, 2023, 8(3): 6356-6374. doi: 10.3934/math.2023321 |
[10] | Yue Wu, Shenglong Chen, Ge Zhang, Zhiming Li . Dynamic analysis of a stochastic vector-borne model with direct transmission and media coverage. AIMS Mathematics, 2024, 9(4): 9128-9151. doi: 10.3934/math.2024444 |
In this paper, a deterministic and stochastic model for hepatitis C with different types of virus genomes is proposed and analyzed. Some sufficient conditions are obtained to ensure the stability of the deterministic equilibrium points. We perform a stochastic extension of the deterministic model to study the fluctuation between environmental factors. Firstly, the existence of a unique global positive solution for the stochastic model is investigated. Secondly, sufficient conditions for the extinction of the hepatitis C virus from the stochastic system are obtained. Theoretical and numerical results show that the smaller white noise can ensure the persistence of susceptible and infected populations while the larger white noise can lead to the extinction of disease. By introducing the basic reproduction number R0 and the stochastic basic reproduction number Rs0, the conditions that cause the disease to die out are indicated. The importance of environmental noise in the propagation of hepatitis C viruses is highlighted by these findings.
Hepatitis C is a disease caused by a virus that infects the liver. The virus, called hepatitis C virus or HCV, is just one of the hepatitis viruses. Global statistics estimate that nearly 350 million people worldwide are infected with HCV, which significantly affects human health, especially the liver [16]. Mathematical models are currently a useful way to assess the transmissibility of many infectious diseases, predict future morbidity, and assess the efficacy of prevention and therapy [1,11,14,17,21,34,35,36,42,46]. Many successful mathematical models investigating fundamental hepatitis C viral dynamics have been produced in recent decades, including Feng et al.[12], Lestari et al. [23] and Li et al. [24] to cite only a few. Moneim and Mosa [31,32], constructed a mathematical model to study the spread of HCV-subtype 4a amongst the Egyptian population. They divided the population into three groups, susceptible class, S(t), the hepatitis C subtype 4a infective class, I1 and the HCV from all other subtype classes, I2. They assumed that all types of the class I2 could mutate to I1 at a constant rate μ>0. A few years after the appearance of [31,32], effective treatment for the hepatitis C virus appeared. For this, we will add to the mathematical model another class (R(t)), which represents those who have been cured of this virus. Following [8,18,19,39,47], the saturated incidence rate will replace the bilinear incidence rate. The hepatitis C virus becomes as follows.
dSdt=A−k1SI1a+S−k2SI2a+S−bS,dI1dt=k1SI1a+S+μI1I2−bI1−γI1,dI2dt=k2SI2a+S−μI1I2−bI2−δI2,dRdt=γI1+δI2−bR, | (1.1) |
where A denotes the recruitment rate of susceptible individuals, a expresses the half-saturation constant for susceptible individuals with HCV. γ and δ are the recovery rates, k1 is the transmission rate of virus C when susceptible individuals S(t) contact with corresponding infected I1(t) individuals, and k2 is the transmission rate when S(t) contact with corresponding I2(t) individuals. Following [31,32], we assume that the population is mixing in a homogenous manner, i.e., every person has the same chance of coming in contact with an infected person also we assume that the birth and death rates are equal and positive constant rate b.
The deterministic HCV system (1.1) ignores the possible importance of environmental noise. In reality, stochastic effects can be important during the transmission of hepatitis C viruses, because various cells and infective virus particles react differently in the same environment. Consequently, the deterministic models do not provide an adequate understanding of viral dynamics due to the stochastic behavior of viruses and the complexity of the immune system. The primary purpose of this paper is to propose and analyze a deterministic and stochastic model of HCV. The paper is arranged as follows: In Section 2, the dynamics of the deterministic system are verified. The stochastic extension of the deterministic model is performed in Section 3, and the existence of a unique global positive solution for the stochastic model is investigated, and the sufficient conditions for the extinction of the hepatitis C virus from the stochastic system are obtained. In Section 4, some numerical simulations are presented to verify the obtained theoretical results. Finally, Section 5 contains the conclusion.
In the following, two critical parameters R01, and R02, can be used to classify the dynamics of the hepatitis C virus model (1.1). The threshold parameter R01 defined by R01=Ak1ρ(A+ab), ρ=b+γ. While the threshold parameter R02 defined by R02=Ak2θ(A+ab), θ=b+δ. Using the next generation method, one can obtain the basic reproduction number R0 defined by R0=max{R01,R02}.
The hepatitis C virus model (1.1) has the following four equilibrium points:
(1) The disease-free equilibrium point E0=(Ab,0,0,0), which always exists. At this point, all individuals are susceptible, and there is no infection present in the population.
(2) The equilibrium point E1=(S1,I11,0,R1), where
S1=aρk1−ρ,I11=(a+S1)(A+ab)ak1(R01−1),R1=γ(a+S1)(A+ab)abk1(R01−1). |
E1 exists if k1>ρ and R01>1.
(3) The equilibrium point E2=(S2,0,I22,R2), where
S2=aθk2−θ,I22=(a+S2)(A+ab)ak2(R02−1),R2=δ(a+S2)(A+ab)abk2(R02−1). |
E2 exists if k2>θ and R02>1.
(4) The coexistence equilibrium point E3=(S3,I13,I23,R3), where
S3=−β+√β2+4abA2b,I13=1μ(k2S3a+S3−θ),I23=1μ(ρ−k1S3a+S3),R3=1μ(γI13+δI23), |
β=ab−A+ρk2μ−θk1μ. The coexistence equilibrium point E3 exists if k2S3θ(a+S3)>1 and k1S3ρ(a+S3)<1.
The boundedness of the solutions of model (1.1) is given as follows. Let (S(t),I1(t),I2(t),R(t)) be any solution of system (1.1) with non-negative initial conditions and assume that the total population size N(t)=S(t)+I1(t)+I2(t)+R(t), then dNdt+bN=A, consequentially N(t)=Ab+N(0)e−bt, thus it follows that 0≤N(t)≤Ab,ast→∞.
The locally asymptotically stable equilibrium points of system (1.1) are now investigated. The Jacobian matrix is given as follows:
J=(−k1aI1(a+S)2−k2aI2(a+S)2−b−k1Sa+S−k2Sa+S0k1aI1(a+S)2k1Sa+S+μI2−ρμI10k2aI2(a+S)2−μI2k2Sa+S+μI1−θ00γδ−b). |
The eigenvalues of J(E0) are −b,−b,ρ(R01−1), and θ(R02−1). Thus, E0 is locally asymptotically stable if R0<1. The eigenvalues of J(E1) are λ1=−b and λ2=k2S1a+S1−μI11−θ. The other roots are determined by
λ2+(k1aI11(a+S1)2+b)λ+k21aS1I11(a+S1)3=0. |
The eigenvalues λ3 and λ4 have negative real parts. Thus, if k2S1(μI11+θ)(a+S1)<1 then the equilibrium point E1 is locally asymptotically stable. The eigenvalues of J(E2) are λ1=−b and λ2=k1S2(a+S2)+μI22−ρ. The other roots are determined by
λ2+(k2aI22(a+S2)2+b)λ+k22aS2I22(a+S2)3=0. |
The eigenvalues λ3 and λ4 have negative real parts. Thus, if k1S2(a+S2)+μI22<ρ then the equilibrium point E2 is locally asymptotically stable. The stability of the coexistence equilibrium point E3=(S3,I13,I23,R3) is investigated as follows
The first eigenvalues of J(E3) is λ1=−b. The other roots are determined by
λ3+c1λ2+c2λ+c3=0, | (2.1) |
where
c1=a(ab+k1I13+k2I23)+2abS3+bS23(a+S3)2,c2=I23(ak22S3+μ2I13(a+S3)3)+ak21S3I13(a+S3)3,c3=μ2I13I23(a(ab+k1I13+k2I23)+2abS3+bS23)(a+S3)2. |
c1c2−c3=aS3(k21I13+k22I23)(a(ab+k1I13+k2I23)+2abS3+bS23)(a+S3)5. |
It is clear that c1,c2,c3>0 and c1c2−c3>0. It follows from the Routh-Hurwitz criterion that all roots of (2.1) have negative real parts. Thus, the equilibrium point E3 is locally asymptotically stable.
In this section, we will perform a stochastic extension of model (1.1). The hepatitis virus C deterministic model (1.1) will be extended to include the environmental noise as follows.
dS=[A−k1SI1a+S−k2SI2a+S−bS]dt+σ1SdW1,dI1=[k1SI1a+S+μI1I2−bI1−γI1]dt+σ2I1dW2,dI2=[k2SI2a+S−μI1I2−bI2−δI2]dt+σ3I2dW3,dR=[γI1+δI2−bR]dt+σ4RdW4, | (3.1) |
where W={W1,W2,W3,W4,t≥0} represents the four-dimensional standard Brownian motions with Wi(0)=0 and σ2i(i=1,2,3,4) denote the intensities of the white noise. The white noise is defined in a complete probability space (Ω,Ft≥0,P) with a filtration Ft≥0 satisfying the usual conditions. In many applications, the solution of the Itô stochastic differential equation must preserve the positivity of the solutions [9,10,37]. According to Theorem 2.2 and Corollary 1 in [9], the solutions of (3.1) emanating from nonnegative initial data (almost surely) remain nonnegative as long as they exist. The next theorem gives another approach according to [30] to prove the existence and uniqueness of a positive global solution of the system (3.1). This approach has recently been used in many papers, and one can highlight [4,5,6,7,13,17,20,22,27,28,33,38,40,41,42,43,44,45,48].
Theorem 1. For any given initial value (S(0),I1(0),I2(0),R(0))∈R4+, there exists a unique solution (S(t),I1(t),I2(t),R(t)) of system (3.1) for t≥0 and the global positive solution remains in R4+ with probability one.
Proof. Firstly, one can consider the local solution (S(t),I1(t),I2(t),R(t)) of system (3.1) for t∈[0,τe), where τe is the explosion time [30]. By making the transformation of variables
x(t)=lnS(t),y(t)=lnI1(t),z(t)=lnI2(t),w=lnR(t). |
Using Itô formula, one can change system (3.1) as follows
dx(t)=[Aex−k1eya+ex−k2eza+ex−b−σ212]dt+σ1dW1,dy(t)=[k1exa+ex+μez−ρ−σ222]dt+σ2dW2,dz(t)=[k2exa+ex−μey−θ−σ232]dt+σ3dW3,dw(t)=[γey−w−δez−w−b−σ242]dt+σ4dW4. | (3.2) |
For X0=(x0,y0,z0,w0)∈R4+, the coefficients of system (3.2) satisfy the local Lipschitz conditions, consequently, there exists a unique local solution (S(t),I1(t),I2(t),R(t))=(ex(t),ey(t),ez(t),ew(t)) on [0,τe). To ensure that this solution is global, one needs to prove that τe=∞ a.s. Let s0>0 be sufficiently large for every coordinate (S(0),I1(0),I2(0),R(0)) in the interval [1s0,s0]. For each integer s>s0, we define the stopping time
τs=inf{t∈[0,τe):min{S(t),I1(t),I2(t),R(t)}∉(1s,s)ormax{S(t),I1(t),I2(t),R(t)}∉(1s,s)}. | (3.3) |
From (3.3), one can note that τs is increasing as s→∞. Assume τ∞=lim, then \tau_\infty\leq \tau_e almost sure. In the next, one needs to verify that \tau_\infty = \infty . If this is not true, then there exists a constant T > 0 and \epsilon \in (0, 1) such that \mathbb P(\tau_\infty \leq T)\geq \epsilon . As a result, there exists an integer s_1\geq s_0 such that \mathbb P(\tau_s \leq T)\geq \epsilon, \, \, \, s\geq s_1 . Define the following C^2 positive definite function V_1 (S, I_1, I_2, R) as
V_1(S,I_1,I_2,R) = (S+1-{\rm{ln}} S)+(I_1+1-{\rm{ln}} I_1)+(I_2+1-{\rm{ln}} I_2)+(R+1-{\rm{ln}} R). |
Using \rm{Itô} 's formula, one obtains
\begin{align*} \begin{split} d V_1 = &\biggl[(S-1)\left( \frac{A}{S} -\frac{k_1 I_1}{a+S}-\frac{k_2 I_2}{a+S}-b \right) + (I_1-1)\left(\frac{k_1 S }{a+S}+\mu I_2 -\rho\right)\\ +&(I_2-1)\left(\frac{k_2 S }{a+S}-\mu I_1 -\theta\right)+ (1-\frac{1}{R})(\gamma I_1+\delta I_2-b R)+\frac{1}{2}\sum\limits_{i = 1}^{4} \sigma_i^2\biggr] dt\\ +& \sigma_1 (S-1) dW_1+\sigma_2 (I_1-1) dW_2+\sigma_3 (I_2-1) dW_3+\sigma_4 (R-1) dW_4\\ \leq&\left[A+2b+\rho+\theta+ \frac{1}{2}\sum\limits_{i = 1}^{4} \sigma_i^2+\left(\frac{k_1}{a}+\mu+\gamma \right)I_1+(\frac{k_2}{a}+\delta) I_2 \right]dt\\ +& \sigma_1 (S-1) dW_1+\sigma_2 (I_1-1) dW_2+\sigma_3 (I_2-1) dW_3+\sigma_4 (R-1) dW_4. \end{split} \end{align*} |
Using the inequality x\leq 2(x+1-{\rm{ln}} x) , for any x > 0 , one obtains
\begin{align*} \begin{split} d V_1 \leq&\biggl[A+2b+\rho+\theta+ \frac{1}{2}\sum\limits_{i = 1}^{4} \sigma_i^2+ 2\left( S+1-{\rm{ln}} S\right) +2\left(\frac{k_1}{a}+\mu+\gamma \right)\left(I_1+1- {\rm{ln}} I_1\right)\\ +& 2(\frac{k_2}{a}+\delta) \left(I_2+1- {\rm{ln}} I_2\right)+ 2\left( R+1-{\rm{ln}} R\right)\biggr] dt+\sigma_1 (S-1) dW_1+\sigma_2 (I_1-1) dW_2\\+&\sigma_3 (I_2-1) dW_3+\sigma_4 (R-1) dW_4, \end{split} \end{align*} |
which means that
\begin{equation} d V_1 \leq K(1+V_1) dt+\sigma_1 (S-1) dW_1+\sigma_2 (I_1-1) dW_2+\sigma_3 (I_2-1) dW_3+\sigma_4 (R-1) dW_4, \end{equation} | (3.4) |
where K = {\rm{max}} \left\lbrace K_1, K_2\right\rbrace , K_1 = A+2b+\rho+\theta+ \frac{1}{2}\sum_{i = 1}^{4} \sigma_i^2 and K_2 = {\rm{max}} \left\lbrace 2, 2(\frac{k_1}{a}+\mu+\gamma), 2(\frac{k_2}{a}+\delta)\right\rbrace . For t_1 \leq T , integrating both sides of (3.4) from 0 to t_1 \wedge \tau_s and then taking the expectation leads to
\begin{align*} \begin{split} E V_1 & \left( S(t_1 \wedge \tau_s), I_1(t_1 \wedge \tau_s),I_2(t_1 \wedge \tau_s), R(t_1 \wedge \tau_s)\right)\\ \leq &V_1\left( S(0),I_1(0),I_2(0),R(0)\right) +K E \int_0^{t_1 \wedge \tau_s} (1+V_1) \,\, dt\\ \leq &V_1\left( S(0),I_1(0),I_2(0),R(0)\right) +K T \\ &+ K \int_0^{t_1 \wedge \tau_s} E V_1 \left( S(t_1 \wedge \tau_s), I_1(t_1 \wedge \tau_s),I_2(t_1 \wedge \tau_s), R(t_1 \wedge \tau_s)\right)\,\, dt. \end{split} \end{align*} |
Following [25,26,29,44], applying Grownwall's inequality, one gets
E V_1 \left( S(t_1 \wedge \tau_s), I_1(t_1 \wedge \tau_s),I_2(t_1 \wedge \tau_s), R(t_1 \wedge \tau_s)\right)\leq \left[ V_1\left( S(0),I_1(0),I_2(0),R(0)\right) + K \,T \right] e^{K T} = K_3. |
The rest of the proof is similar to [25,26,29] and hence is omited here. This complete the proof.
The above theorem shows that the stochastic HCV system (3.1) have positive global solution remain in \mathbb{R}_+^4 with probability one. The non-explosion characteristic in an epidemic model is essential but often insufficient. Hence, one needs to indicate that the solution will be ultimately bounded with a large probability. In the following, we will establish the stochastically ultimate boundedness property of the HCV system (3.1).
Lemma 2. Let N(t) = S(t)+I_1(t)+I_2(t)+R(t) , then for any given initial value (S(0), I_1 (0), I_2(0), R(0)) \in \mathbb{R}_+^4 , the following inequality holds:
\lim\limits_{t \to \infty} N(t) < \infty \,\,\,a.s. |
Proof. It follows from HCV model (3.1) that
\begin{equation} dN(t) = (A-b N(t))dt +\sigma_1 S(t) dW_1(t)+\sigma_2 I_1 (t) dW_2(t)+\sigma_3 I_2 (t) dW_3(t)+\sigma_4 R(t) dW_4(t) \end{equation} | (3.5) |
Then, the solution of Eq (3.5) has the following form
N(t) = \frac{A}{b}+\left( N(0)-\frac{A}{b}\right) e^{-b t}+M(t), |
where
\begin{align*} \begin{split} M(t) = & \int_0^t e^{-b (t-s)}\sigma_1 S(s) dW_1(s)+\int_0^t e^{-b (t-s)}\sigma_2 I_1(s) dW_2(s)+\int_0^t e^{-b (t-s)}\sigma_3 I_2(s) dW_3(s)\\ +& \int_0^t e^{-b (t-s)}\sigma_4 R(s) dW_4(s), \end{split} \end{align*} |
which is a contiuous local martingal with M(0) = 0 , almost surely. Define \Lambda(t) = \frac{A}{b}[1-e^{-b t}] and U(t) = N(0)[1-e^{-b t}], then we have N(t) = N(0)+\Lambda(t)-U(t)+M(t). Clearly, \Lambda(t) and U(t) are continuous adapted increasing process on t\geq 0 with \Lambda(0) = U(0) = 0 . Then by Theorem 3.9 in [30], we have \lim_{t \to \infty} N(t) < \infty a.s.
Remark 3. It follows from the first equation of HCV system (3.1) that
S(t)-S(0) = A t-b \int_0^t S(s) ds-\int_0^t \frac{k_1 S(s) I_1 (s)}{a+S(s)}-\int_0^t \frac{k_2 S(s) I_2 (s)}{a+S(s)}+\sigma_1\int_0^t S(s) dW_1(s), |
and it follows that
\langle S(t)\rangle\leq \lim\limits_{t \to \infty} \left[\frac{A}{b}- \frac{S(t)-S(0)}{t}+\frac{\sigma_1}{t}\int_0^t S(s) dW_1(s) \right] \leq \frac{A}{b}. |
Theorem 4. If \frac{\sigma_1^2}{2} +\frac{1}{2} < b, \, \, \, k_1+\frac{\sigma_2^2}{2} +\frac{1}{2} < \rho, \, \, \, k_2+\frac{\sigma_3^2}{2} +\frac{1}{2} < \theta, \, \, \, \sigma_4^2 +\frac{1}{2} < b , then the solutions of (3.1) are stochastically ultimate bounded.
Proof. For (S(t), I_1 (t), I_2(t), R(t))\in \mathbb{R}_+^4 , define the following function
V_2(S(t),I_1 (t),I_2(t),R(t)) = S(t)^2+I_1 (t)^2+I_2(t)^2+R(t)^2. |
By \rm{Itô} formula, one has
dV_2(S,I_1,I_2,R) = LV_2(S,I_1,I_2,R)dt+2\sigma_1 S^2 dW_1+2\sigma_2 I_1^2 dW_2+2\sigma_3 I_2^2 dW_3+2\sigma_4 R^2 dW_4, |
where
\begin{align*} \begin{split} LV_2(S,I_1,I_2,R) = & 2\left( A S -\frac{k_1 S^2 I_1}{a+S}-\frac{k_2 S^2 I_2}{a+S}-b S^2 \right) +2 I_1^2\left(\frac{k_1 S }{a+S}+\mu I_2 -\rho\right)\\ &+2I_2^2\left(\frac{k_2 S }{a+S}-\mu I_1 -\theta\right)+2(\gamma I_1 R+\delta I_2 R-b R^2)\\ &+\sigma_1^2 S^2+\sigma_2^2 I_1^2+\sigma_3^2 I_2^2+\sigma_4^2 R^2 \\ \leq&(\sigma_1^2 -2b+1)S^2+\left(2 k_1+\sigma_2^2 -2\rho+1\right) I_1^2\\ &+\left(2 k_2+\sigma_3^2 -2\theta+1\right) I_2^2+\left( \sigma_4^2 -2 b+1\right) R^2\\ & -\left[S(t)^2+I_1 (t)^2+I_2(t)^2+R(t)^2\right] +2 \left( A S+\mu I_1^2 I_2+\gamma I_1 R+\delta I_2 R\right) . \end{split} \end{align*} |
Assume f(S(t), I_1 (t), I_2(t), R(t)) = (\sigma_1^2 -2b+1)S^2+\left(2 k_1+\sigma_2^2 -2\rho+1\right) I_1^2+\left(2k_2+\sigma_3^2 -2\theta+1\right) I_2^2+\left(\sigma_4^2 -2b+1\right) R^2+2\left(A S+\mu I_1^2 I_2+\gamma I_1 R+\delta I_2 R\right). According to Lemma 2 and Remark 3, one can find that the function f(S(t), I_1 (t), I_2(t), R(t)) has an upper bound.
Let N_1 = \sup f(S(t), I_1 (t), I_2(t), R(t))+1 . As a result
dV_2(S,I_1,I_2,R) = (N_1-V_2) dt+2\sigma_1 S^2 dW_1+2\sigma_2 I_1^2 dW_2+2\sigma_3 I_2^2 dW_3+2\sigma_4 R^2 dW_4. |
By \rm{Itô} formula, one obtains
d(e^t V_2)\le e^t N_1 dt+e^t \left[ 2\sigma_1 S^2 dW_1+2\sigma_2 I_1^2 dW_2+2\sigma_3 I_2^2 dW_3+2\sigma_4 R^2 dW_4\right]. |
Integrating both sides of the above equation from 0 to t and then taking expectations, one gets
e^t V_2(S(t),I_1 (t),I_2(t),R(t))\le V_2(S(0),I_1 (0),I_2(0),R(0))+N_1 e^t-N_1, |
hence
\lim\limits_{t \to \infty} \rm{sup}\,\,\, \mathbb{E}[|X(t)|^2]\leq N_1. |
Using Chebyshev's inequality, one can obtains
\mathbb{P}[|X(t)|\ge H]\leq \frac{\mathbb{E}[|X(t)|^2]}{H^2}, |
where H = \frac{\sqrt{N_1}}{\sqrt{\epsilon}}, \, \, \epsilon > 0. Then
\lim\limits_{t \to \infty} \rm{sup}\,\,\, \mathbb{P}[|X(t)|\geq H]\leq \frac{N_1}{H^2} = \epsilon. |
This completes the proof.
In the following, we will establish the conditions for extinction of hepatitis C virus from the stochastic system (3.1). The threshold parameter R_{01}^s defined by R_{01}^s = \frac{ k_1}{\rho+\frac{\sigma_2^2}{2}}, while the threshold parameter R_{02}^s defined by R_{02}^s = \frac{ k_2}{\theta+\frac{\sigma_3^2}{2}} and the threshold parameter R_{03}^s defined by R_{03}^s = \frac{k_1+k_2}{\rho+\theta} . The stochastic basic reproduction number R_{0}^s defined by R_{0}^s = {\rm{max}} \left\lbrace R_{01}^s, R_{02}^s, R_{03}^s\right\rbrace .
Theorem 5. The disease die out exponentially with probability one if R_{0}^s < 1.
Proof. Let V_3(I_1, I_2) = {\rm{ln}} (I_1+I_2) . Using \rm{Itô} formula, one obtains
\begin{align*} \begin{split} d(V_3(I_1,I_2)) = &\biggl[\frac{1}{(I_1+I_2)}\left(\frac{k_1 S I_1}{a+S}+\frac{k_2 S I_2}{a+S}-\rho I_1-\theta I_2\right)- \frac{\sigma_2^2 I_1^2}{2(I_1+I_2)^2} -\frac{\sigma_3^2 I_2^2}{2(I_1+I_2)^2}\biggr] dt\\& +\frac{\sigma_2 I_1}{(I_1+I_2)} dW_2 +\frac{\sigma_3 I_2}{(I_1+I_2)} dW_3\\\leq&\frac{1}{(I_1+I_2)^2}\biggl[ \left(\frac{k_1 S }{(a +S)}-\rho-\frac{\sigma_2^2}{2}\right) \\& I_1^2+\left(\frac{k_2 S }{(a +S)}-\theta-\frac{\sigma_3^2}{2}\right) I_2^2+\left(\frac{k_1 S }{(a +S)}-\rho\right) I_1 I_2 \\&+\left(\frac{k_2 S }{(a +S)}-\theta\right) I_1 I_2\biggr] dt +\frac{\sigma_2 I_1}{(I_1+I_2)} dW_2 +\frac{\sigma_3 I_2}{(I_1+I_2)} dW_3\\\leq&\frac{1}{(I_1+I_2)^2}\biggl[ \left(k_1-\rho-\frac{\sigma_2^2}{2}\right) I_1^2+\left(k_2-\theta-\frac{\sigma_3^2}{2}\right) I_2^2+\left(k_1-\rho\right) I_1 I_2 \\&+\left(k_2-\theta\right) I_1 I_2\biggr] dt +\frac{\sigma_2 I_1}{(I_1+I_2)} dW_2 +\frac{\sigma_3 I_2}{(I_1+I_2)} dW_3 \\ \leq&\biggl[ (\rho+\frac{\sigma_2^2}{2})\left( R_{01}^s-1 \right)+(\theta+\frac{\sigma_3^2}{2})\left( R_{02}^s-1 \right) + (\rho+ \theta) \left( R_{03}^s-1\right) \biggr]dt \\& +\frac{\sigma_2 I_1}{(I_1+I_2)} dW_2 +\frac{\sigma_3 I_2}{(I_1+I_2)} dW_3\\ \leq&\biggl[ 2(\rho+ \theta)+\frac{\sigma_2^2}{2}+\frac{\sigma_3^2}{2} \biggr]\left(R_{0}^s-1 \right)dt +\frac{\sigma_2 I_1}{(I_1+I_2)} dW_2 +\frac{\sigma_3 I_2}{(I_1+I_2)} dW_3, \end{split} \end{align*} |
using integration from 0 to t , one obtains
\begin{align*} \begin{split} {\rm{ln}} \,\,(I_1(t)+I_2(t))\leq&\,\, {\rm{ln}}\, (I_1(0)+I_2(0))+\biggl[ 2(\rho+ \theta)+\frac{\sigma_2^2}{2}+\frac{\sigma_3^2}{2} \biggr]\left( R_{0}^s-1 \right) t \\&+\int_0^t \frac{\sigma_2 I_1}{2(I_1+I_2)} dW_2 +\int_0^t \frac{\sigma_3 I_2}{2(I_1+I_2)} dW_3. \end{split} \end{align*} |
Applying strong law of large numbers for local martingales one gets
\begin{align*} \begin{split} \lim\limits_{t \to \infty} \rm{sup} \frac{{\rm{ln}} \,\,(I_1(t)+I_2(t))}{t}\leq& \biggl[ 2(\rho+ \theta)+\frac{\sigma_2^2}{2}+\frac{\sigma_3^2}{2} \biggr]\left( R_{0}^s-1 \right) < 0, \end{split} \end{align*} |
as a result, diseases I_1(t) and I_2(t) die out and tend to zero exponentially a.s., if R_{0}^s < 1.
Remark 6. Theorem 3 indicates that the disease die out exponentially a.s., if R_{0}^s < 1 with the consequence that the recover class R(t) also goes to extinction a.s.
In this part, the numerical results will be compared with the theorems formulated in the previous sections. The interactions between populations classes will be simulated by the following parameters [32]: N = 1000000, \mu = 0.02, a = 1, b = 0.02, k_1 = 0.00001, k_2 = 0.00002, \gamma = 0.001, \delta = 0.001 . To give some numerical finding to the HCV system (3.1), we use the Milstein method mentioned in [2,3,15]. The stochastic hepatitis virus C system (3.1) reduces to the following discrete system
\begin{align} \begin{split} S_{(j+1)}& = x_j+h \left( A -\frac{k_1 S_j I_{1j}}{a+S_j}-\frac{k_2 S_j I_{2j}}{a+S}-b S_j\right) +\sigma_1 S_j\sqrt{h}\epsilon_{1j}+\frac{\sigma_1^2}{2}S_j\left[\epsilon_{1j}^2-1 \right] h,\\ I_{1(j+1)}& = I_{1j}+h \, \left( \frac{k_1 S_j I_{1j}}{a+S_j}+\mu I_{1j} I_{2j} -b I_{1j}-\gamma I_{1j} \right) +\sigma_2 I_{1j}\sqrt{h}\epsilon_{2j}+\frac{\sigma_2^2}{2} I_{1j}\left[\epsilon_{2j}^2-1 \right] h,\\ I_{2(j+1)}& = I_{2j}+h \left( \frac{k_2 S_j I_{2j}}{a+S_j}-\mu I_{1j} I_{2j} -b I_{2j}-\delta I_{2j} \right)+\sigma_3 I_{2j}\sqrt{h}\epsilon_{3j}+\frac{\sigma_3^2}{2} I_{2j}\left[\epsilon_{3j}^2-1 \right] h,\\ R_{(j+1)}& = R_j+h \left( \gamma I_{1j}+\delta I_{2j}-b R_j\right)+\sigma_4 R_j\sqrt{h}\epsilon_{4j}+\frac{\sigma_4^2}{2} R_j\left[\epsilon_{4j}^2-1 \right] h , \end{split} \end{align} | (4.1) |
where h is a positive time increment and \epsilon_{ij}, (i = 1, 2, 3, 4) are independent random Gaussian variables N(0, 1) . One can note that for the given parameters, the value of the stochastic basic reproduction number R_0^s = 0.00095 . As a result, the conditions of Theorem 5 are verified and the disease die out exponentially with probability one if R_{0}^s < 1 . Figure 1 represents the dynamical behavior of model (3.1) when the noise strength law ( \sigma_i = 0.01 ). For k_1 = k_2 = 0.0308 , the conditions of Theorem 5 are verified as R_{0}^s = 1.130769 > 1 as shown in Figure 2. It is shown that the trajectories of the stochastic system (3.1) oscillates around the coexistence equilibrium of the deterministic system (1.1).
The novelty of this study is that we introduced a new compartment and saturated incidence rate into the classical hepatitis C virus genome model. A deterministic and stochastic model for hepatitis C with different types of virus genomes is proposed and analyzed. We perform a stochastic extension of the deterministic model to study the fluctuation between environmental factors. Firstly, the existence of a unique global positive solution for the stochastic model is investigated. Secondly, sufficient conditions for the extinction of hepatitis C virus from the stochastic system are obtained. Theoretical results are illustrated using numerical simulations. Theoretical and numerical results show that the smaller white noise can ensure the persistence of susceptible and infected populations while the larger white noise can lead to the extinction of populations. For the deterministic model, some sufficient conditions are obtained to ensure the stability of the equilibrium points. The results show that when the basic reproduction number R_0 < 1 , the deterministic model is asymptotic stable around the free disease equilibrium point E_0 . By introducing the basic reproduction number R_0 and the stochastic basic reproduction number R_0^s , the conditions that cause the disease to die out are indicated. Biologically, it can be noted that by social distancing with hepatitis C patients, this can lead to a fluctuation in the value of k_1 and k_2 , and thus R_0^s becomes less than one, which leads to the disappearance of the epidemic.
The authors gratefully acknowledge Qassim University, represented by the Deanship of Scientific Research. The authors thank Dr. Sanling Yuan of University of Shanhai for Science and Technology for his kind cooperation.
The researcher would like to thank the Deanship of Scientific Research, Qassim University, for funding the publication of this project.
The authors declare that there is no conflict of interests.
[1] |
S. Allegretti, I. M. Bulai, R. Marino, M. A. Menandro, K. Parisi, Vaccination effect conjoint to fraction of avoided contacts for a SARS-CoV-2 mathematical model, Math. Model. Numer. Simul. Appl., 1 (2021), 56–66. https://doi.org/10.53391/mmnsa.2021.01.006 doi: 10.53391/mmnsa.2021.01.006
![]() |
[2] |
Y. Alnafisah, The implementation of milstein scheme in two-dimensional SDEs using the fourier method, Abstr. Appl. Anal., 2018 (2018). https://doi.org/10.1155/2018/3805042 doi: 10.1155/2018/3805042
![]() |
[3] | Y. A. Alnafisah, Comparison between milstein and exact coupling methods using MATLAB for a particular two-dimensional stochastic differential equation, J. Inform. Sci. Eng., 36 (2020), 1223–1232. |
[4] |
I. Bashkirtseva, L. Ryashko, T. Ryazanova, Analysis of regular and chaotic dynamics in a stochastic eco-epidemiological model, Chaos Soliton. Fract., 131 (2020), 109549. https://doi.org/10.1016/j.chaos.2019.109549 doi: 10.1016/j.chaos.2019.109549
![]() |
[5] |
B. Berrhazi, M. El Fatini, A. Lahrouz, A. Settati, R. Taki, A stochastic SIRS epidemic model with a general awareness-induced incidence, Physica A, 512 (2018), 968–980. https://doi.org/10.1016/j.physa.2018.08.150 doi: 10.1016/j.physa.2018.08.150
![]() |
[6] |
Y. Cai, Y. Kang, W. Wang, A stochastic SIRS epidemic model with nonlinear incidence rate, Appl. Math. Comput., 305 (2017), 221–240. https://doi.org/10.1016/j.amc.2017.02.003 doi: 10.1016/j.amc.2017.02.003
![]() |
[7] | Y. Cai, X. Mao, Stochastic prey-predator system with foraging arena scheme, Appl. Math. Model., 64 (2018), 357–371. https://doi.org/10.1016/j.apm.2018.07.034 https://doi.org/10.1080/17442508.2019.1612897 |
[8] |
Z. Chang, X. Meng, X. Lu, Analysis of a novel stochastic SIRS epidemic model with two different saturated incidence rates, Physica A, 472 (2017), 103–116. https://doi.org/10.1016/j.physa.2017.01.015 doi: 10.1016/j.physa.2017.01.015
![]() |
[9] |
J. Cresson, B. Puig, S. Sonner, Stochastic models in biology and the invariance problem, Discrete Cont. Dyn.-B, 21 (2016), 2145. https://doi.org/10.3934/dcdsb.2016041 doi: 10.3934/dcdsb.2016041
![]() |
[10] |
J. Cresson, S. Sonner, A note on a derivation method for sde models: Applications in biology and viability criteria, Stoch. Anal. Appl., 36 (2018), 224–239. https://doi.org/10.1080/07362994.2017.1386571 doi: 10.1080/07362994.2017.1386571
![]() |
[11] |
B. Daşbaşı, Stability analysis of an incommensurate fractional-order SIR model, Math. Model. Numer. Simul. Appl., 1 (2021), 44–55. http://doi.org/10.53391/mmnsa.2021.01.005 doi: 10.53391/mmnsa.2021.01.005
![]() |
[12] |
T. Feng, Z. Qiu, X. Meng, Dynamics of a stochastic hepatitis C virus system with host immunity, Discrete Cont. Dyn.-B, 24 (2019), 6367. https://doi.org/10.3934/dcdsb.2019143 doi: 10.3934/dcdsb.2019143
![]() |
[13] |
C. Gokila, M. Sambath, K. Balachandran, Y. K. Ma, Analysis of stochastic predator-prey model with disease in the prey and Holling type Ⅱ functional response, Adv. Math. Phys., 2020 (2020). https://doi.org/10.1155/2020/3632091 doi: 10.1155/2020/3632091
![]() |
[14] |
Z. Hammouch, M. Yavuz, N. Özdemir, Numerical solutions and synchronization of a variable-order fractional chaotic system, Math. Model. Numer. Simul. Appl., 1 (2021), 11–23. https://doi.org/10.53391/mmnsa.2021.01.002 doi: 10.53391/mmnsa.2021.01.002
![]() |
[15] |
D. J. Higham, An algorithmic introduction to numerical simulation of stochastic differential equations, SIAM Review, 43 (2001), 525–546. https://doi.org/10.1137/S0036144500378302 doi: 10.1137/S0036144500378302
![]() |
[16] | J. L. Horsley-Silva, H. E. Vargas, New therapies for hepatitis C virus infection, Gastroenterol. Hepat., 13 (2017), 22. |
[17] |
R. Ikram, A. Khan, M. Zahri, A. Saeed, M. Yavuz, P. Kumam, Extinction and stationary distribution of a stochastic COVID-19 epidemic model with time-delay, Comput. Biol. Med., 141 (2022), 105115. https://doi.org/10.1016/j.compbiomed.2021.105115 doi: 10.1016/j.compbiomed.2021.105115
![]() |
[18] |
J. Jiang, S. Gong, B. He, Dynamical behavior of a rumor transmission model with Holling-type Ⅱ functional response in emergency event, Physica A, 450 (2019), 228–240. https://doi.org/10.1016/j.physa.2015.12.143 doi: 10.1016/j.physa.2015.12.143
![]() |
[19] |
T. Kar, P. K. Mondal, Global dynamics and bifurcation in delayed SIR epidemic model, Nonlinear Anal.-Real, 12 (2011), 2058–2068. https://doi.org/10.1016/j.nonrwa.2010.12.021 doi: 10.1016/j.nonrwa.2010.12.021
![]() |
[20] |
A. Khan, G. Hussain, A. Yusuf, A. H. Usman, A hepatitis stochastic epidemic model with acute and chronic stages, Adv. Differ. Equ., 2021 (2021), 1–10. https://doi.org/10.1186/s13662-021-03335-7 doi: 10.1186/s13662-021-03335-7
![]() |
[21] |
P. Kumar, V. S. Erturk, Dynamics of cholera disease by using two recent fractional numerical methods, Math. Model. Numer. Simul. Appl., 1 (2021), 102–111. https://doi.org/10.53391/mmnsa.2021.01.010 doi: 10.53391/mmnsa.2021.01.010
![]() |
[22] |
G. Lan, S. Yuan, B. Song, The impact of hospital resources and environmental perturbations to the dynamics of SIRS model, J. Franklin I., 358 (2021), 2405–2433. https://doi.org/10.1016/j.jfranklin.2021.01.015 doi: 10.1016/j.jfranklin.2021.01.015
![]() |
[23] |
D. Lestari, N. Y. Megawati, N. Susyanto, F. Adi-Kusumo, Qualitative behaviour of a stochastic hepatitis C epidemic model in cellular level, Math. Biosci. Eng., 19 (2022), 1515–1535. https://doi.org/10.3934/mbe.2022070 doi: 10.3934/mbe.2022070
![]() |
[24] |
J. Li, K. Men, Y. Yang, D. Li, Dynamical analysis on a chronic hepatitis C virus infection model with immune response, J. Theor. Biol., 365 (2015), 337–346. https://doi.org/10.1016/j.jtbi.2014.10.039 doi: 10.1016/j.jtbi.2014.10.039
![]() |
[25] |
J. Li, M. Shan, M. Banerjee, W. Wang, Stochastic dynamics of feline immunodeficiency virus within cat populations, J. Franklin I., 353 (2016), 4191–4212. https://doi.org/10.1016/j.jfranklin.2016.08.004 doi: 10.1016/j.jfranklin.2016.08.004
![]() |
[26] | L. Li, W. Zhao, Deterministic and stochastic dynamics of a modified Leslie-Gower prey-predator system with simplified Holling-type Ⅳ scheme, Math. Biosci. Eng., 18 (2021), 2813–2831. |
[27] |
Q. Li, F. Cong, T. Liu, Y. Zhou, Stationary distribution of a stochastic HIV model with two infective stages, Physica A, 554 (2020), 124686. https://doi.org/10.1016/j.physa.2020.124686 doi: 10.1016/j.physa.2020.124686
![]() |
[28] |
Q. Liu, D. Jiang, T. Hayat, A. Alsaedi, B. Ahmad, A stochastic SIRS epidemic model with logistic growth and general nonlinear incidence rate, Physica A, 551 (2020), 124152. https://doi.org/10.1016/j.physa.2020.124152 doi: 10.1016/j.physa.2020.124152
![]() |
[29] |
P. S. Mandal, M. Banerjee, Stochastic persistence and stationary distribution in a Holling-Tanner type prey-predator model, Physica A, 391 (2012), 1216–1233. https://doi.org/10.1016/j.physa.2011.10.019 doi: 10.1016/j.physa.2011.10.019
![]() |
[30] | X. Mao, Stochastic differential equations and applications, Elsevier, 2007. |
[31] | I. Moneim, M. Al-Ahmed, G. Mosa, Stochastic and monte carlo simulation for the spread of thehepatitis B, Aust. J. Basic Appl. Sci., 3 (2009), 1607–1615. |
[32] |
I. Moneim, G. Mosa, Modelling the hepatitis C with different types of virus genome, Comput. Math. Method. M., 7 (2006), 3–13. https://doi.org/10.1080/10273660600914121 doi: 10.1080/10273660600914121
![]() |
[33] |
Y. Mu, W. C. Lo, Stochastic dynamics of populations with refuge in polluted turbidostat, Chaos Soliton. Fract., 147 (2021), 110963. https://doi.org/10.1016/j.chaos.2021.110963 doi: 10.1016/j.chaos.2021.110963
![]() |
[34] |
P. A. Naik, Z. Eskandari, H. E. Shahraki, Flip and generalized flip bifurcations of a two-dimensional discrete-time chemical model, Math. Model. Numer. Simul. Appl., 1 (2021), 95–101. https://doi.org/10.53391/mmnsa.2021.01.009 doi: 10.53391/mmnsa.2021.01.009
![]() |
[35] |
F. Özköse, M. Yavuz, Investigation of interactions between COVID-19 and diabetes with hereditary traits using real data: A case study in Turkey, Comput. Biol. Med., 141 (2022), 105044. https://doi.org/10.1016/j.compbiomed.2021.105044 doi: 10.1016/j.compbiomed.2021.105044
![]() |
[36] |
F. Özköse, M. Yavuz, M. T. Şenel, R. Habbireeh, Fractional order modelling of omicron SARS-CoV-2 variant containing heart attack effect using real data from the United Kingdom, Chaos Soliton. Fract., 157 (2022), 111954. https://doi.org/10.1016/j.chaos.2022.111954 doi: 10.1016/j.chaos.2022.111954
![]() |
[37] |
T. A. Phan, J. P. Tian, B. Wang, Dynamics of cholera epidemic models in fluctuating environments, Stoch. Dynam., 21 (2021), 2150011. https://doi.org/10.1142/S0219493721500118 doi: 10.1142/S0219493721500118
![]() |
[38] |
S. Rajasekar, M. Pitchaimani, Qualitative analysis of stochastically perturbed SIRS epidemic model with two viruses, Chaos Soliton. Fract., 118 (2019), 207–221. https://doi.org/10.1016/j.chaos.2018.11.023 doi: 10.1016/j.chaos.2018.11.023
![]() |
[39] |
R. Shi, T. Lu, C. Wang, Dynamic analysis of a fractional-order model for hepatitis B virus with Holling Ⅱ functional response, Complexity, 2019 (2019). https://doi.org/10.1155/2019/1097201 doi: 10.1155/2019/1097201
![]() |
[40] |
G. Song, Dynamics of a stochastic population model with predation effects in polluted environments, Adv. Differ. Equ., 2021 (2021), 1–19. https://doi.org/10.1186/s13662-021-03297-w doi: 10.1186/s13662-021-03297-w
![]() |
[41] |
X. Wang, C. Wang, K. Wang, Extinction and persistence of a stochastic SICA epidemic model with standard incidence rate for HIV transmission, Adv. Differ. Equ., 2021 (2021), 1–17. https://doi.org/10.1186/s13662-021-03392-y doi: 10.1186/s13662-021-03392-y
![]() |
[42] |
X. Wang, Y. Tan, Y. Cai, K. Wang, W. Wang, Dynamics of a stochastic HBV infection model with cell-to-cell transmission and immune response, Math. Biosci. Eng., 18 (2021), 616–642. https://doi.org/10.3934/mbe.2021034 doi: 10.3934/mbe.2021034
![]() |
[43] |
Z. Wang, M. Deng, M. Liu, Stationary distribution of a stochastic ratio-dependent predator-prey system with regime-switching, Chaos Soliton. Fract., 142 (2021), 110462. https://doi.org/10.1016/j.chaos.2020.110462 doi: 10.1016/j.chaos.2020.110462
![]() |
[44] |
C. Wei, J. Liu, S. Zhang, Analysis of a stochastic eco-epidemiological model with modified Leslie-Gower functional response, Adv. Differ. Equ., 2018 (2018), 1–17. https://doi.org/10.1186/s13662-018-1540-z doi: 10.1186/s13662-018-1540-z
![]() |
[45] |
C. Xu, G. Ren, Y. Yu, Extinction analysis of stochastic predator-prey system with stage structure and crowley-martin functional response, Entropy, 21 (2019), 252. https://doi.org/10.3390/e21030252 doi: 10.3390/e21030252
![]() |
[46] |
M. Yavuz, N. Sene, Stability analysis and numerical computation of the fractional predator-prey model with the harvesting rate, Fractal Fract., 4 (2020), 35. https://doi.org/10.3390/fractalfract4030035 doi: 10.3390/fractalfract4030035
![]() |
[47] |
J. Z. Zhang, Z. Jin, Q. X. Liu, Z. Y. Zhang, Analysis of a delayed SIR model with nonlinear incidence rate, Discrete Dyn. Nat. Soc., 2008 (2008). https://doi.org/10.1155/2008/636153 doi: 10.1155/2008/636153
![]() |
[48] |
Q. Zhang, X. Wen, D. Jiang, Z. Liu, The stability of a predator-prey system with linear mass-action functional response perturbed by white noise, Adv. Differ. Equ., 2016 (2016), 1–24. https://doi.org/10.1186/s13662-016-0776-8 doi: 10.1186/s13662-016-0776-8
![]() |
1. | Yousef Alnafisah, Moustafa El-Shahed, Correction: Deterministic and stochastic model for the hepatitis C with different types of virus genome, 2022, 7, 2473-6988, 15992, 10.3934/math.2022875 | |
2. | Xiaodong Wang, Kai Wang, Zhidong Teng, Global dynamics and density function in a class of stochastic SVI epidemic models with Lévy jumps and nonlinear incidence, 2023, 8, 2473-6988, 2829, 10.3934/math.2023148 | |
3. | Liangwei Wang, Fengying Wei, Zhen Jin, Xuerong Mao, Stationary distribution and extinction of an HCV transmission model with protection awareness and environmental fluctuations, 2025, 160, 08939659, 109356, 10.1016/j.aml.2024.109356 | |
4. | A. Q. Khan, S. Younis, Chaos and bifurcations of a two-dimensional hepatitis C virus model with hepatocyte homeostasis, 2024, 34, 1054-1500, 10.1063/5.0203886 | |
5. | Nabeela Anwar, Iftikhar Ahmad, Hijab Javaid, Adiqa Kausar Kiani, Muhammad Shoaib, Muhammad Asif Zahoor Raja, Dynamical analysis of hepatitis B virus through the stochastic and the deterministic model, 2025, 1025-5842, 1, 10.1080/10255842.2025.2470798 |