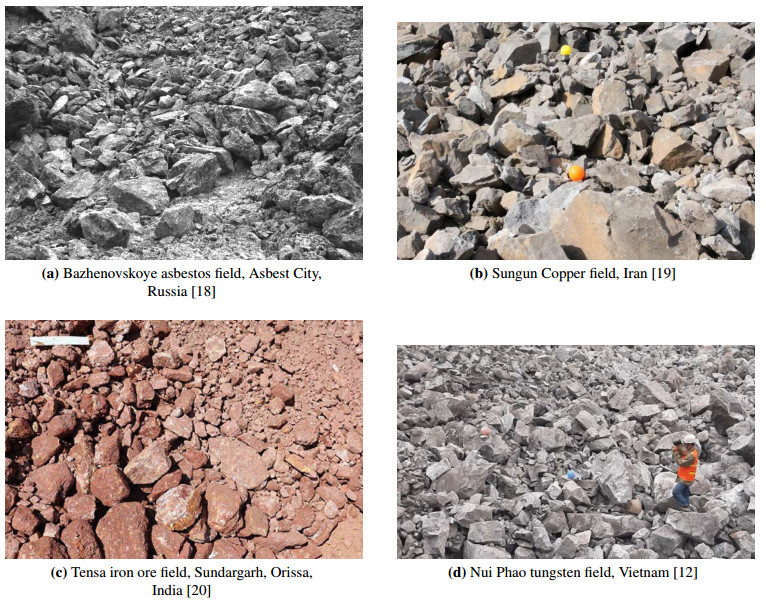
Interval type-2 fuzzy logic systems (IT2 FLSs) already become an emerging technology in recent years. As the most popular type-reduction (TR) algorithms, Karnik-Mendel (KM) algorithms own the advantage of maintaining the uncertainties flow in systems. This paper analyzes the initialization for KM types of algorithms. Furthermore, the weighting approaches of them are also given by means of the Newton-Cotes quadrature formulas. Importantly, the reasonable initialization weighted enhanced Karnik-Mendel (RIWEKM) algorithms are provided to complete the centroid type-reduction of IT2 FLSs. Three computer simulation experiments illustrate that, the proposed RIWEKM algorithms own both smaller absolute errors and faster convergence speeds in contrast to the EKM and RIEKM algorithms.
Citation: Yang Chen, Jiaxiu Yang, Chenxi Li. Design of reasonable initialization weighted enhanced Karnik-Mendel algorithms for centroid type-reduction of interval type-2 fuzzy logic systems[J]. AIMS Mathematics, 2022, 7(6): 9846-9870. doi: 10.3934/math.2022549
[1] | Mashael M Asiri, Abdelwahed Motwakel, Suhanda Drar . Robust sign language detection for hearing disabled persons by Improved Coyote Optimization Algorithm with deep learning. AIMS Mathematics, 2024, 9(6): 15911-15927. doi: 10.3934/math.2024769 |
[2] | Mashael Maashi, Mohammed Abdullah Al-Hagery, Mohammed Rizwanullah, Azza Elneil Osman . Deep convolutional neural network-based Leveraging Lion Swarm Optimizer for gesture recognition and classification. AIMS Mathematics, 2024, 9(4): 9380-9393. doi: 10.3934/math.2024457 |
[3] | Wahida Mansouri, Amal Alshardan, Nazir Ahmad, Nuha Alruwais . Deepfake image detection and classification model using Bayesian deep learning with coronavirus herd immunity optimizer. AIMS Mathematics, 2024, 9(10): 29107-29134. doi: 10.3934/math.20241412 |
[4] | Eman A. Al-Shahari, Marwa Obayya, Faiz Abdullah Alotaibi, Safa Alsafari, Ahmed S. Salama, Mohammed Assiri . Accelerating biomedical image segmentation using equilibrium optimization with a deep learning approach. AIMS Mathematics, 2024, 9(3): 5905-5924. doi: 10.3934/math.2024288 |
[5] | Madhusundar Nelson, Surendran Rajendran, Youseef Alotaibi . Vision graph neural network-based neonatal identification to avoid swapping and abduction. AIMS Mathematics, 2023, 8(9): 21554-21571. doi: 10.3934/math.20231098 |
[6] | Manal Abdullah Alohali, Fuad Al-Mutiri, Kamal M. Othman, Ayman Yafoz, Raed Alsini, Ahmed S. Salama . An enhanced tunicate swarm algorithm with deep-learning based rice seedling classification for sustainable computing based smart agriculture. AIMS Mathematics, 2024, 9(4): 10185-10207. doi: 10.3934/math.2024498 |
[7] | Maha M. Althobaiti, José Escorcia-Gutierrez . Weighted salp swarm algorithm with deep learning-powered cyber-threat detection for robust network security. AIMS Mathematics, 2024, 9(7): 17676-17695. doi: 10.3934/math.2024859 |
[8] | Mesut GUVEN . Leveraging deep learning and image conversion of executable files for effective malware detection: A static malware analysis approach. AIMS Mathematics, 2024, 9(6): 15223-15245. doi: 10.3934/math.2024739 |
[9] | Muhammad Ali Khan, Saleem Abdullah, Alaa O. Almagrabi . Analysis of deep learning technique using a complex spherical fuzzy rough decision support model. AIMS Mathematics, 2023, 8(10): 23372-23402. doi: 10.3934/math.20231188 |
[10] | Álvaro Abucide, Koldo Portal, Unai Fernandez-Gamiz, Ekaitz Zulueta, Iker Azurmendi . Unsteady-state turbulent flow field predictions with a convolutional autoencoder architecture. AIMS Mathematics, 2023, 8(12): 29734-29758. doi: 10.3934/math.20231522 |
Interval type-2 fuzzy logic systems (IT2 FLSs) already become an emerging technology in recent years. As the most popular type-reduction (TR) algorithms, Karnik-Mendel (KM) algorithms own the advantage of maintaining the uncertainties flow in systems. This paper analyzes the initialization for KM types of algorithms. Furthermore, the weighting approaches of them are also given by means of the Newton-Cotes quadrature formulas. Importantly, the reasonable initialization weighted enhanced Karnik-Mendel (RIWEKM) algorithms are provided to complete the centroid type-reduction of IT2 FLSs. Three computer simulation experiments illustrate that, the proposed RIWEKM algorithms own both smaller absolute errors and faster convergence speeds in contrast to the EKM and RIEKM algorithms.
The mining industry has undergone a rapid transformation in recent years, with the digitization of its operations. This transformation has often been referred to as the fourth industrial revolution or Industry 4.0. One of the most notable developments in this area has been the increasing use of deep learning-based approaches for automating a range of mining applications, including drilling, blasting, haulage and factory processing. Despite the widespread adoption of deep learning in the mining industry, its deployment has been relatively infrequent compared to other industries. Today in the mining industry and other fields, many jobs are carried out manually by highly qualified specialists, although some can be performed automatically. This is especially relevant when solving routine tasks, which require extensive specialist experience. Yet specialists often cannot explain (or formally describe) how they solve problems. Nevertheless, experts sometimes make mistakes (so-called human factors), and they require a lot of time and resources to become proficient. However, nowadays modern neural networks can automate such tasks, as shown by the general practice of deep learning (DL) implementation. The problem may be reduced to a few-times dataset collection for which expert markup is needed. The automated DL solution will reduce specialists' workload by handling routine tasks for them or providing guidance. This is done, for example, by suggesting a solution to a problem or giving information required for finding a solution.
In recent years, there has been an increasing trend in utilizing DL Computer Vision (CV) techniques for solving various applied problems in the mining industry. These problems include, but are not limited to, the classification of mineral types and their fraction relation [1,2,3], detection and analysis of the size distribution of mineral grains or particles [4,5], analysis of boring and drilling results [6,7], autonomous haulage vehicles driving [8], exploration and analysis of open-pit mines [9,10,11,12] and recognition of terrain types [13].
The mining industry is faced with numerous challenges, including the analysis and evaluation of the performance and productivity of valuable rock resources mining, particularly in open-pit mines. This task is of the utmost importance, as it is critical to achieving optimal operational efficiency, reducing costs and maximizing profits. To achieve this goal, it is necessary to accurately estimate rock fragmentation, blast quality and other related factors [12,14,15,16,17,18]. Rock fragmentation estimation is an essential aspect of mining operations, as it affects a range of downstream processes, such as crushing and grinding. Accurate estimation of rock fragmentation can help optimize these processes, resulting in improved efficiency and productivity. Furthermore, it can also reduce the environmental impact of mining operations, as it can help minimize the amount of waste material generated. Similarly, blast quality estimation is also crucial for optimizing mining operations. This involves assessing the effectiveness of the blasting process, which is essential for achieving the desired rock fragmentation. Accurate blast quality estimation can help reduce costs by minimizing the amount of explosives required, while also improving safety by ensuring that the blasting process is carried out correctly.
In the context of mining operations, an open-pit mine can be described as the collection and processing of a large number of rock fragments resulting from the blasting of multiple smaller work areas. These rock fragments are then transported to a processing plant for further treatment, and the overall production yield for the entire open-pit mine is determined by the productivity estimates of all individual work areas. As a result, accurate assessment and control of productivity at each open-pit workplace are essential for effective management of the mine and processing plant. The examples of such open-pit workplaces are illustrated in Figures 1 for several cases [12,18,19,20]
In recent years, there has been increasing interest in CV techniques for analyzing rock fragmentation and related tasks in the mining industry. These studies emphasize the need for fast and accurate DL methods in CV to tackle these challenges. This need is particularly crucial due to the use of low-performance mobile devices and the requirement for real-time measurements in mining operations.
DL-based approaches offer promising solutions by enabling efficient and accurate analysis of large amounts of visual data obtained from diverse mining operations. Real-time data processing is essential for making timely decisions and optimizing operations for maximum productivity and efficiency.
Furthermore, the development of DL-based techniques for mining applications is important because the data obtained in such operations is often complex and highly variable. Visual data from different rock fragments and work areas in an open pit, for example, can vary significantly in terms of size, shape and color. To effectively handle such variability and produce accurate results, DL methods must be trained on large and diverse datasets.
Unlike conventional DL CV problems, rock fragmentation tasks involve processing images of numerous relatively small and overlapping objects. The specifics and quality of the images can vary depending on the work conditions (e.g., open-pit mines, tunnels, conveyor belts) and weather conditions, such as lighting and air clarity. These factors can adversely affect CV algorithms, resulting in lower-quality results. Therefore, fine-tuning and modifying DL architectures and applying sophisticated pre- and post-processing techniques are often necessary. In addition, the CV systems for these tasks are often intended to work in real time, processing dozens of images per second.
The objective of our systematic literature review is to gather knowledge about recent advancements in DL-based CV systems for rock fragmentation and related tasks in the mining industry. We aim to identify the main methodologies and techniques used to solve rock fragmentation problems and correlate them with the current state-of-the-art in real-time CV and performance of neural networks. By doing so, we intend to place this topic in the context of DL tasks and neural network implementations. While we cannot suggest specific recipes for all cases, we provide recommendations for researchers in the fields of mining industry on promising fast approaches and architectures for real-time instance segmentation and performance optimization of training and inference on various devices.
The main contribution of our study is to draw attention to current methods for solving fragmentation problems, emphasize that problems can be solved in real time and demonstrate current trends in dealing with fragmentation problems and CV-related problems in general. The current state of solving fragmentation problems is several years behind the general CV, as we demonstrate in the review.
The paper is organized as follows. Section 2 describes the methodology of our survey, statistical results of the considered publications and their summary. Section 3 presents a brief review of the main publications. All papers were divided into DL tasks, namely semantic segmentation, instance segmentation, object detection and a classification/regression approach. Section 4 discusses the main findings from the reviewed papers and compares them with current state-of-the-art for DL CV algorithm architectures and optimization techniques for implementing neural networks. Section 5 concludes our survey and formulates our position on promising techniques and approaches to the discussed topics.
To collect related papers, we formulated search strings by combining a pair of keywords from two groups:
● "rock fragmentation", "blast quality estimation", "open-pit mining", "mining productivity estimation", "mining industry problem";
● "computer vision", "deep learning", "computer vision neural network architectures", "feature extractors for computer vision", "semantic segmentation", "instance segmentation", "real-time instance segmentation".
Then, we used the resulting search strings in the Google Scholar and Scopus databases. We utilized the following criteria to filter out articles with insufficient content and assess paper quality. The paper must explain how CV is specifically used in the mining industry for tasks that include rock fragmentation or such relative tasks as: particle size distribution, blast quality estimation, rock size estimation or their detection. In addition to the DL-based methods, we also consider the traditional CV-based systems. Also during the review we pay attention to the topic of computation specificity and how parallel computing architectures with GPUs are used to implement neural network training and inference. We also discard the papers not directly devoted to the image analysis, for example, the works on mathematical models of size distribution.
Table Appendix summarizes the publications that meet the criteria above. Let us note that most of the reviewed papers cover the period of 2018–2023. However, the most significant of the older publications are also considered. The studies in the table are sorted by publication year and grouped by practical field of the rock fragmentation problem.
The main features in Table Appendix, which were extracted during the review, were the following. In the review, we found that most of the papers utilized CV approaches in the traditional form of photo (or, in rare cases, in 3D by laser scanning). Thus, we did not consider other methods of solving the fragmentation problem.
● Year of publication.
● Practical Problem. This problem is the same as specificity of work conditions, we divide them into conveyor belt, open-pit, tunnel and other conditions. The term "other" types includes rare paper conditions or large scale images, which could be considered out of our topic.
● CV Task. Approach to CV problem solving. This is a taxonomy of the types of approaches common to DL in CV applications. The main types of problems are: classification/regression; object detection; semantic segmentation (or pixel/image segmentation) and instance segmentation. Semantic segmentation includes both segmentation and boundary segmentation, or their combination.
● DL application. All papers are categorized based on whether they use only classical CV approaches or approach with DL neural networks. A classical approach refers to using hand-crafted features and a variety of different decision making algorithms (such as the Watershed algorithm), in contrast to a DL approach that pulls features and makes decisions automatically using neural networks. Let us note here, that almost all papers that apply DL use convolutional neural networks (CNN). Thus, we did not divide DL and CNN techniques.
● Specific models or methods/algorithms applied in the paper.
● Primary CV Model. In this section, we highlight only the main method/algorithm considered by publication.
The review results in Table Appendix are shown in Figures 2–6. Figure 2 shows the visualization of the DL vs. classical CV approach for problem solution in the publications. Figure 3 shows the popularity of the fragmentation problem (practical problem) in publications over time. Figure 4 shows CV task vs. the practical problem type distribution. Figure 5 shows the primary CV model distribution by year starting from 2004. Figure 6 shows the visualization of CV task relative to the primary model distribution.
Figures 2–6 show that, starting from 2018, DL approach is applied in rock fragmentation and similar tasks. Moreover, since 2020, DL CNN is favored in rock fragmentation and similar tasks (see Figure 2). Also, the popularity of investigation of the fragmentation task in open pits is growing from 2018, probably with the general DL popularity growing (see Figure 3).
The instance segmentation approach is the most popular approach in open-pit conditions. Among all instance segmentation DL models, Mask R-CNN remains the most popular solution to date. However, since 2022 real-time object detection approaches (including YOLO-based models) have been considered as alternatives to the Mask R-CNN solutions (see Figures 4, 5, 6).
The peak of the investigation of the fragmentation task in the conveyor belt condition was in 2020. In these conditions, the semantic segmentation approach is the most popular one. Among all semantic segmentation DL models U-Net is the most popular solution. Additionally, DeepLab3+-based architectures have been utilized as some alternatives to U-Net-based models from 2022 (see Figures 4, 5, 6).
Among all classical models, the Watershed algorithm is the most popular solution (corresponds to instance segmentation). The peak of the Watershed popularity was from 2005 to 2019. This algorithm is mostly applied to conveyor belt problems, but also was tested for open-pit conditions (see Figure 6).
Let us note that by a classification/regression problem we address the estimation of coarse fragmentation sizes. This is done, for instance, by dividing the images into classes by the size of present fragments. Also, under semantic segmentation we assume either full object segmentation or (and) their boundaries segmentation. Frequently, such results can be divided into objects by any post-processing technique to extract instance parameters. As opposed to this, in instance segmentation problems we assume end-to-end routines to recognize instances. By estimating only the boundary box and central position of an instance, object detection is a part of instance segmentation. Figure 7 shows typical illustrations of the types of problems under consideration.
The next section will focus on the main papers on the considered topic from Table Appendix.
A CV-based approach to rock fragmentation estimation is related to what is referred to as "indirect" methods [21]. Analysis of computer vision in the context of the considered and related tasks has shown that this approach is known as one of the most accurate in the literature. Most of the works here can be divided into two classes. The first one is based on applying classical CV techniques. The second one is built using contemporary DL techniques. The main difference lies in the way features are extracted and processed. In classical methods, features are extracted manually based on a synthetic assumption and using a variety of techniques. Afterwards, another method for making decisions (fragmentation in our case) is applied to the results that were obtained. In the reviewed literature, the Watershed algorithm is the most popular among them. Alternatively, DL assumes an end-to-end approach, with automatic feature extraction as well as the decision making part within one neural network architecture. The classical-based approach suffers from variations in daytime, weather and season conditions [22,23,24].
Moreover, it is shown that the classical approach works well only for high resolution rock chunks in images and does not provide sufficiently accurate results for images of large open-pit places. However, such algorithms could be less resource-consuming than the DL-based approaches currently applied in this field [12,21]. Most classical CV based solutions already exist in commercial software, like the Gold Size, Wipfrag and other [12,21,25,26,27]. In the preceding software, the fragment distributions are frequently estimated as values of the circle area with comparable sizes as rock chunks are expected to have [27]. This equivalent size value could be an incorrect measure and does not directly represent the maximum dimension size of a rock fragment [12,21]. The current achievements of DL for instance segmentation tasks show the ability to achieve comparative performance with higher accuracy and robustness of the results, while allowing to overcome most of the classical CV approach drawbacks [22,23,24].
The basic idea of using convolutional architecture was proposed in 1989 for the recognition of hand-written ZIP Code numbers [28,29]. The main application of this approach is processing data with known regular structure, such as images (two-dimensional) or time series (one-dimensional). Besides image processing, CNN models are also widely used in various applications in civil engineering, such as fault prediction [30,31] and structural health monitoring [32,33,34,35].
The modern way of using CNN in DL CV was launched by the LeNet architecture in 1998 [36]. Automated feature extraction is the main benefit of using DL in CV. It is well known that most CV tasks are difficult to describe formally. However, it is well known that a well-suited set of features is necessary for a large generalization ability. Unlike shallow neural networks and other machine learning approaches, DL networks include two main parts: the feature extractor and the decision-making (so-called head) part. A CNN feature extractor consists of convolution and auxiliary layers. In the case of CV convolution operation, it has several inductive biases: providing scale, location, and rotation invariant feature extraction with a fewer number of parameters in comparison to other approaches [37,38]. Currently, these advantages make CNN the main way of solving CV problems.
Generally, Table Appendix shows that the rock fragmentation estimation task as a CV problem could be solved as a contour segmentation task utilizing a semantic segmentation approach (see, for instance, [15,16,39]) or as an instance segmentation task (see, for instance [12,20,40]). However, some authors also propose object detection-based solutions (see, for instance [41,42,43]) or classification/regression-based methods of strict fractions forecasting. The following sections are organized in accordance with this division.
The specifics of CV could be explained as follows. Typical CV problems require a lot of extracted features which cannot be formally described as usual. DL allows one to automatically extract complex data representations even from unprocessed datasets, providing meaningful information. On the other hand, this area requires rather large databases with all the ensuing difficulties of a typical big data application. Big data presents many challenges, but the most critical are its computational performance and data storage space requirements. Another particularly difficult aspect is the high diversity of valuable feature distributions that we often have during the training of a decision algorithm. Nearly any modern neural network architecture can be subject to these challenges [44].
In addition to the mentioned above DL-based methods, let us note some classical-based solutions to the fragmentation problem. The principle of the watershed algorithm [45] is to represent an image as a 3D structure. In the obtained structure the points of the so-called basins cross-sections are considered as instance boundaries. In the basic form, the Watershed algorithm suffers from noise influence and blurring of boards in the low-contrast cases. Both of these problems can lead to oversegmentation or undersegmentation issues [46]. Thus, an additional pre-processing step is usually required. This image processing can be done either by DL semantic segmentation or as a classical pipeline. The first case leads to the considered DL application. The classical approach seems to be challenging for segmentation in the case of multi-scale and multi-shape objects. It suffers from unstable outer conditions such as light, daytime, etc. which affect the texture, colors and other image parameters [20]. Despite the above problems, paper [47] suggests the use of the modified Watershed alone algorithm for open-pit segmentation.
The semantic segmentation problem assumes the solution of the so-called pixelwise classification (in the sense of DL). The output of the neural network should have the same spatial dimensions as the input, and the number of channels should equal the number of classes. Then, each pixel for all channels is soft-reweighted (with softmax, as a vector) to determine the class it belongs to.
Among all DL semantic segmentation architectures, the most popular choice is the U-Net [48]. Even though it was introduced in 2015, this architecture remains one of the most popular today. Figure 8 illustrates the U-Net architecture and the pixelwise softmax. The U-Net architecture is related to encoder-decoder segmentation networks. Its main characteristic is that it uses feature maps from the encoder in the decoder. Since 2015 many architectural modifications have been proposed. Most of them were proposed for the medical applications [49,50,51,52,53] and some of them for the microscopic images analysis applications [54].
Paper [15] considers the ore size determination problem as the contour segmentation problem (as a part of the semantic segmentation task). The authors propose slightly modified Res-U-Net architectures for ore size determination in both conveyor belt and open pit conditions, with accuracy of about 90% in both cases. In addition, the authors have tested the classical Watershed algorithm, which shows comparable accuracy for high-scale images on the conveyor belt, but much less accurate for the open pit. For open-pit conditions, authors combined the U-Net segmentation with the Watershed algorithm to group results into objects.
A U-Net-based model with an Efficient-net-B3 backbone for conveyor belt conditions was proposed in paper [18]. The system allows one to use the same approach for rock chunks detection as well as for valuable resources inside it. The images are taken in the near-infrared range of light. The model achieved 0.97 of Dice coefficient for stone segmentation and 0.6 for valuable veins material segmentation.
An instance segmentation model is proposed for recognition of rock fragments during tunnel boring in work [55]. It is based on two subnetworks: object detection and semantic segmentation. The object detection network is based on a modified Single-Shot Detector (SSD) with multilevel feature fusion, prior anchors and self-attention. The semantic segmentation is using the U-Net architecture. In the downsampling stage, the data from the object detection network was used to share features of rock fragments. In the upsampling stage, the skip connection and self-attention were utilized. For the test data, 0.87 average recall and 0.76 average IoU were achieved. On a workstation with the Intel i9-9900k and the NVIDIA GeForce RTX 2080 Ti GPU, the inference for a full size image of resolution takes 7.5 seconds, while the resolution requires 0.15 seconds. Post-processing and statistical analysis takes 0.18 seconds. Therefore, the proposed method can be used in real time.
Work [56] is aimed at analyzing the rock segmentation distribution on images of rocks produced by a tunnel boring machine, namely, collect statistical data on size distribution. The core algorithm is based on the semantic segmentation. Thus, the task of detecting the rock fragment is considered as a pixel-wise classification problem. To distinguish between adjacent rocks, additionally, the rock contours are considered. The labeling for contours is obtained from instance segmentation labels by the Canny edge detection algorithm. The dataset contains instance labels, semantic labels and contour labels. For semantic segmentation, five networks were trained: FCN8s, GCN, DFN, DeepLab v3, PSPNet. For contour segmentation, different models (based on the same architectures) were trained on the contour labels. For predicting rock object masks and contour masks, the mask fusion algorithm was applied to split adjacent rocks during the inference stage. Post-processing is used to reduce noise in the final prediction masks. The authors compare their approach with alternative methods, such as instance segmentation and traditional contour detection (Sobel operator) and show that their approach has better accuracy, achieving the best visual results. In terms of segmentation accuracy, the mean IoU is 68.3%. To optimize the inference of two separate networks, the authors used the shared lightweight Y-shaped encoder network. The authors performed the inference speed test for the image resolution of on the NVIDIA Titan XP GPU. By using the shared encoder, the performance went from 6.8 images per second to 33.3 without significant loss of accuracy. When authors replaced the ResNet101 by the lightweight MobileNetv2, the segmentation IoU score went from 75 to 68, but the size statistic accuracy was not lost. The performance was boosted to 57.2 images per second. For the larger resolution of , the achieved inference speed was 19.4 images per second, which satisfies the system design requirement of 10.
A similar approach was used in work [57] for automatic segmentation of rock chips produced by the tunnel boring machine. The authors used a dual U-Net architecture with multi-scale inputs and side-output (MSD-UNet). Dual U-Net was used for multi-task semantic segmentation. Two decoders work to process the extracted features and generate segmentation maps separately for the regions and boundaries of the rocks. The post-processing was performed by smoothing the region masks, subtracting the boundary masks, using multi-radius erosion to separate the regions and using the seed filling algorithm to estimate the size and shape of the individual rocks. The authors compare their approach with U-Net and conventional segmentation methods, and show that it achieves a higher F1 score (0.867 and 0.64 for regions and boundaries respectively). Using the NVIDIA TITAN RTX GPU, their method can process a image in 4 seconds. They note that only 10% of this time is spent on the inference, while the rest is spent on post-processing, namely, fusing the predictions and estimating size and shape. The boring system collects 30 images every 3 minutes. Thus, the method has sufficient performance to analyze the data in real-time.
Another application of the idea of separate region and boundary segmentation is presented in work [58]. It proposes the modification of U-Net for semantic segmentation of ore images. The model decoder includes two subnetworks, one for boundary detection and one for mask segmentation. The results of two networks is fused in boundary mask fusion blocks. As shown in experiments, the proposed architecture outscores the original U-Net as well as the other known architectures, in terms of pixel accuracy, IoU for masks and error in ore particle size statistics. The models were trained using two NVIDIA GeForce GTX 1080 GPUs. The inference speed on a single GPU is 105 ms for the proposed method, in comparison with 44 ms for the original U-Net for the resolution of .
Work [59] describes the segmentation method for processing images of ore fragments on a conveyor belt. The model is based on the RDU-Net model. It consists of DUNet (a modified U-Net segmentation network) and the residual connection structure of ResNet. This approach reduces the loss of information that occurs during information transfer between the deep convolution layers and improves the accuracy of model detection. The authors compare their model with traditional segmentation methods as well as with the DL methods, such as U-Net and DUNet. The RDU-Net model shows better accuracy metrics than alternative methods. The experiments were performed using the Intel i5-7500 CPU and the NVIDIA GeForce GTX 1050 Ti. The model achieves an inference speed of 20 seconds per image for an unspecified resolution which is a multiple of .
A DL method for detecting and characterizing the rock fractures in tunnel face images was proposed in work [60]. The authors propose the FraSegNet model for pixel-wise semantic segmentation. It is based on a modified VGG19 CNN including five convolutional and four pooling layers, atrous spatial pyramid pooling module for sampling the feature maps and a decoder module that up-samples, fuses the resulting maps and provides a final prediction using a guided filter. By qualitative comparison, the proposed model performs better than the traditional edge detection algorithms (namely, Canny and Laplacian) as well as the general-purpose semantic segmentation models (such as FCN and DeepLabV3+) in terms of boundary recognition and noise avoidance at the pixel level. On the NVIDIA GeForce GTX 1080 Ti GPU, the inference takes 0.44 seconds per image of size , while the post-processing and statistical evaluation takes 7.2 seconds per image.
A model for segmenting the coal and gangue in the mining waste is presented in paper [14]. It is based on the U-Net architecture for pixel-level segmentation. The authors compare their model with several other gangue classification methods, namely, based on LeNet, AlexNet and CG-RPN. Their method provides comparable accuracy, and provides more information, not only identifying the samples, but also background pixels. The experiments were performed on an NVIDIA GeForce GTX 1080 Ti GPU. Training took 5.8 hours. The segmentation of a single image takes 48 ms for an image size of which is sufficient for real-time processing.
In work [39], an ore segmentation method was proposed on the basis of U-Net architecture and the Watershed algorithm. To speed up the processing, the authors have modified the structure of U-Net without reducing accuracy. The modification consists of halving the number of convolution kernels in each layer of the network. To compensate for this reduction, the number of convolution operations at each stage is increased, making the network deeper. The loss function is modified to add the influence of uniform distribution and increase the weight of the background. The marked Watershed algorithm is used to post-process and optimize the segmentation results. The experiments were performed on the NVIDIA GeForce GTX 1080 Ti GPU. The inference speed for the resolution of is 46 ms per image for the unmodified U-Net and 34 ms per image for the modified one. The post-processing takes 16 ms per image.
The instance segmentation task in DL is generally thought of as an object detection task with semantic segmentation of instances in each bounding box [61]. Besides there could be several other approaches, for instance, based on the idea of grouping semantic segmentation results into objects [62].
The most common architecture for instance segmentation is Mask R-CNN [63], which has a multistage architecture.. The Mask R-CNN consists of a common feature extractor (such as ResNet [64]), small region proposal network (for extracting a preliminary set of class-agnostic regions of interest called anchors), then the head part consists of classification, bounding box parameter regression and semantic segmentation for each anchor. After obtaining the network results, the Non-Maximum-Suppression (NMS) algorithm is applied to final object proposals. The illustration of the Mask R-CNN architecture is shown in Figure 9 [63]. Mask R-CNN was first proposed in 2017, but it remains one of the most popular solutions for segmentation [65].
The Mask R-CNN based model was proposed in [12] where the authors achieved accuracy of about 90% for an open-pit workplace. Furthermore, it has been shown that DL algorithms perform better than conventional CV approaches for high-scale images of workplaces. Also, this work notes that the accuracy of classical algorithms depends on daytime, sufficient light, dry weather and other external conditions.
Almost similar results were shown in paper [66]. The paper compares conventional and DL approaches to large-scale images of open-pit workplaces. The authors stated the advantages of the Mask R-CNN for large scale images. Many contemporary authors state that traditional approaches suffer from wide dispersion of rock chunk sizes, overlapping instances and image quality for large-scale images, e.g., see [5,12,23,66].
Automatic CV system for estimating asbestos productivity in open pit workplace is constructed in work [67]. Its overall operation consists of 4 stages, namely, detecting rock chunks on the whole open-pit image using Mask R-CNN, fetching the images of individual rock chunk, segmenting the asbestos veins for the chunk and estimating average asbestos content in the workplace. The obtained results show high enough overall accuracy (absolute error is 0.4%), but less than geological service (manual estimation by an expert) do in the same condition.
The paper [68] proposed utilizing a Mask R-CNN based approach to the estimation of rock chunk size distribution on soil-rock mixture images. The authors proposed using simple Mask R-CNN model with ResNet-50 encoder. To correctly estimate the chunk size, the images contain a size standard. For results of the instance segmentation, equivalent size was calculated and distribution is compared to the sieve size distribution. The method of comparison is not pointed in the paper. The training was performed on an NVIDIA Tesla K80 GPU using the Detectron2 implementation of Mask R-CNN.
A DL model for analyzing the fragmentation (size distribution of particles) of rocks for producing rock-filled concrete is presented in [69]. Mask R-CNN based on the FPN and Resnet-101 is used for instance segmentation. The training and inference procedures run on a computer with an Intel Core i7-10700K CPU and NVIDIA GeForce GTX 1660 Ti GPU.
Another instance segmentation model for estimating the size distribution of rock-filled materials is constructed in work [70]. It is based on a modified Mask R-CNN network with ResNeXt101 as a backbone and Squeeze-and-Excitation block attention mechanism for enhanced feature extraction. The core of SE-block is to automatically obtain the importance of each feature channel through loss learning. Thus, the network can use global information to selectively enhance beneficial feature channels, so as to achieve adaptive calibration of feature channels. The results of comparative experiments show that the authors' model achieves better results than other models (based on various Mask R-CNN backbones with or without SE-block), namely, 0.934 average precision and 0.879 average IoU values on the test images. The experiments were performed on an NVIDIA GeForce 3070 GPU and Intel i7-10700k CPU. The segmentation model takes 57 seconds to process the original resolution of and 2.3 seconds for the size of . The authors state that such performance is sufficient for practical use in real time.
A CV approach was proposed in work [71] for estimating the characteristics of riprap (rocks used to armor technical structures from erosion) from visual data. At its core, it has an instance segmentation model based on the Mask R-CNN and FCN architectures. This model provides 80% average completeness (ratio of segmented particles to ground-truth labeled particles) and 87% average IoU for the validation data set.
A DL approach for analyzing the rock sizes in the blast-induced rock fragmentation images was proposed in work [23]. It is based on a Mask R-CNN architecture, namely, ResNet50, which is used for instance segmentation, and global average pooling followed by dense fully connected layers as the top layer to predict fragmentation parameters, such as size distribution. The experiments were performed on an NVIDIA GeForce RTX 2080 Ti GPU. The trained model takes 5 seconds to process 153 image samples (30 images per second).
The object detection approach is based on the idea of coarse bounding box determination for each object. This box could include the center position, width and height of each box. The difference with instance segmentation is that the object detection task does not incorporate segmentation of the objects inside each box. Some baseline of the object detection approach could be Faster-R-CNN which is acting similar to Mask R-CNN (see in Figure 9 but without a segmentation branch in the head part).
The "You Look Only Once" (YOLO) family of architectures was designed as some attempt at the trade-off between inference speed and accuracy. The main idea is to divide an image into uniform cells. Each object is detected as belonging to one of these cells. Each cell corresponds to one vector on the model output. The vector may include one or several anchors with sizes, shift of center coordinates predictions, class and objectness prediction. The term objectness means object or background determination in the cell. The result of objects is determined using a non-maximum suppression algorithm [72]. The YOLOv3 architecture utilizes a convolutional backbone based on the darknet architecture with a feature pyramid-based neck part and three scales of the output combined together. Each scale produces objects of the different sizes with a different trade-off between receptive field and sensitivity to small details [73]. The architecture family after YOLOv3 was designed using a lot of experiments with architecture and training techniques called "Bag of Specials" and "Bag of Fribies" correspondingly [74,75,76]. The illustration of the YOLOv3 architecture is shown in Figure 10.
Paper [41] describes coke particle size distribution on a conveyor belt using the object detection approach. The CV approach in the task is proposed as a rapid and precise alternative to the sieving-based old-fashioned technique. The paper notices that the most common features for distribution estimation are area, circumference and diameter of estimated rock chunks. This set of features is obtained using a segmentation approach to the problem solution. The authors of [41] compare the common parameters of the bounding boxes: length, width, average of length and width, diagonal length and equivalent circle diameter to the real particle size making the conclusion that the width of boxes is the most relevant measure. As an additional benefit of using such measures selection, authors notice the reliability for the problem of over-lapping rock-chunks.
The authors of [41,77] compare several object detection models for the described problem solution. Among the compared models are: Single Shot MultiBox Detector (SSD), YOLOv3, YOLOv4, YOLOv5 and deformable convolution YOLO3 (DCN-YOLOv3), and the results indicated that DCN-YOLOv3 showed the highest accuracy and speed. It is noticed that as an additional benefit of using such measure selection, authors notice the reliability for the problem of overlapping rock chunks [41]. The algorithm of the DCN-YOLOv3 implementation to the coal gangue detection and recognition on the conveyor belt was also proposed in [41]. The authors achieved an inference speed of 2.7 to 2.9 images per second for YOLOv3 using the NVIDIA Tesla V100 16GB GPU for the resolution of . Training took 8 hours. In [77], using the DCN-YOLOv3, authors achieved a speed of 33 images per second on the NVIDIA GeForce RTX 2070 Super GPU for a resolution of .
Also, the paper [78] compares several popular approaches to the instance sizes distribution estimation. The following models were considered: Faster-R-CNN, SVM+HOG, Watershed and Yolo3. The comparison was done in the laboratory conditions. The Yolo3 shows the highest accuracy of 93% by mean average precision measures. The obtained accuracy for Faster-R-CNN is almost the same at 91%, but the inference speed is 5 times higher. As the authors state, the accuracy is enough for industry applications.
A system for detecting and sorting coal and gangue on the conveyor belt is described in paper [79]. The authors proposed their own YOLO3-based tiny architecture with a squeeze-and-excitation module and SPP-block. The achieved accuracy of 94% by mAP is comparable with the Faster-R-CNN based system but allowing it to work on a real-time scale. The authors trained the model on the Intel i7-8550U CPU in mixed precision using the NVIDIA APEX and Tensorflow frameworks. The inference speed is 4.28 seconds per image with full precision, and 1.56 seconds with mixed precision for resolution.
Paper [42] suggests the YOLOv5 [80] for rock chunk determination and their size estimation, proving that this task may be considered as a problem of object detection. Via the use of the YOLOv5, open pit image fragmentation problems can be solved 10 times faster than the "traditional" Mask R-CNN approach without a significant loss of accuracy.
The problem of classifying the rock fragments produced by a tunnel boring machine is considered in paper [81]. The goal is to determine the category of image by the maximal size of rock fragments presented in it. For recognizing the fragments, a modified AlexNet was utilized. The modification consists of changing the output layer to contain 3 nodes (for 3 image classes) and integrating the network structure into a single GPU (because the original AlexNet is intended for multiple GPUs). The results of experiments show the competitive accuracy of the proposed methodology. The experiments were performed on a workstation with the NVIDIA GeForce RTX 2080 Ti GPU. For the input size of the inference time is 28 ms per image (36 images per second).
A system for coal and empty rocks (gangue) classification on the conveyor belt using a custom CNN was proposed in paper [16]. The authors collected a 300-image dataset of images with only coal, mix and rocks. This was enough to achieve about 90% accuracy with both predefined and random augmentations.
Authors of [82] proposed to reduce the task of distribution estimation to the regression task. As a baseline, the VGG-16 network was proposed. The distribution was proposed to divide into 10 fractions parts. The novel loss function was also proposed to take into account the original regression problem as well as the relation of true fraction class predictions. The accuracy by R2-score of 80% was achieved in the on-belt system conditions. Training was conducted using Google Colab on an NVIDIA Tesla K80 GPU.
Paper [83] is devoted to classifying the rock structures on the images of a tunnel face. The authors use the CNN architecture Inception-ResNet-V2 combining GoogLeNet and ResNet backbone. The authors compare this architecture and other backbones, such as ResNet-50, ResNet-101 and Inception-v4. Their model achieves best performance in terms of precision, recall and F1 score. It also achieves the best inference time, namely, 0.325 seconds per image on the NVIDIA GeForce GTX 1080Ti GPU and 13 seconds per image on the Intel i7-8700K CPU. This means that the GPU is 40 times faster than the CPU.
A method for classifying the rock samples on the basis of CNN is proposed in paper [1]. Five types of CNN architectures were used for testing, namely, AlexNet, VGG16, ResNet50, InceptionV3 and MobileNet. The proposed models additionaly use attention-based Squeeze-and-Excitation module (SENet) to improve the sensitivity of the model to channel features. The comparative experiments demonstrated the use of transfer learning to be effective against improving classification performance, such as reaching 94% with the MobileNet model.
Let us briefly recite the main findings from the literature. The trends are divided in accordance with the methodology of the survey.
● The most results were obtained after 2000. Before this, only early attempts at fragmentation systems based on the classical CV approach were done.
● The main methods utilized in the range 2001-2017 are the Watershed and other classical algorithms, such as Otsu's thresholding and morphological reconstruction. Performance of these methods decreases under various outer conditions (light, fog, etc) and for very large-scale images.
● The classification/regression-based DL systems were popular in 2015–2019. The models are based on the prediction of each fraction's content. The results of such methods are very uninterpretable.
● The semantic segmentation systems was the main way in 2017–2021. Such systems require either an additional post-processing for boundaries detection or a separate semantic segmentation model for boundaries. The systems are mainly applied in conveyor conditions. The most popular solutions here are the U-Net-based architectures.
● The Mask R-CNN based instance segmentation based systems are applied starting from 2020. Such models allow one to work on large-scale images of the open-pit in different daytime and other conditions. But the Mask R-CNN models family require too much computing resources and are not capable of working in real time on poor-performance terminal devices.
● The YOLO-based object detection models are applied from 2021. These models provide quite accurate results at high speed of work.
According to the reviewed studies, the main solutions for the CV problems in the mining industry are U-Net [48] as an auxiliary semantic segmentation problem and Mask R-CNN [63] for prime instance segmentation tasks. Both networks contain feature extractors based primarily on the basic ResNet block and specific to head parts. However, in the original form these architectures do not provide high performance and accuracy compared to modern models (see for instance [84,85,86].
Training and inference of neural network models for mining tasks is predominantly conducted on GPU-equipped workstations. However, there is significant variability in the reported performance data due to variations in GPU models and input image resolutions employed across different studies. Some authors provide specifications of devices without specific data on training or inference speeds. A number of papers correlate performance data with the throughput of associated equipment (e.g., imaging cameras). Authors can draw conclusions about achieving real-time performance or the performance required to solve a practical problem. Notably, certain studies (e.g., [60], [57]) demonstrate inference times that are considerably lower than post-processing times, diminishing the significance of neural network implementation performance. Certain authors [39,56] propose structural optimizations for neural network models, resulting in significant speed improvements in both training and inference. However, the performance and accuracy of these optimizations are typically evaluated only for specific tasks. In study [79], the NVIDIA APEX AMP framework is utilized to convert the model to mixed precision, leading to up to three-fold inference speedup.
The majority of the reviewed studies use different architectures and datasets, making direct comparisons difficult. Regardless of the obtained results, the task requires fast computation while maintaining high accuracy. These requirements can be satisfied by combining modern lightweight neural network architectures and computation optimization techniques for DL from the other side.
The survey shows that researchers and organizations in the field of rock fragmentation continue to use the basic (and rather outdated) methods of CV both with and without DL neural networks. The majority of the related architectures refer to original papers published before 2017 (such architectures as U-Net and Mask R-CNN). These architectures are the most popular in the corresponding areas and can be considered as baseline models. In general, authors often provide limited discussions on the performance aspects of their developed models. Nevertheless, the intended industrial applications of the research results often involve lightweight, low-performance, energy-efficient computational devices, suggesting that the use of neural network optimization techniques would be beneficial.
In our opinion, the most promising approaches to rock fragmentation problems in open-pit mining appear to be so-called real-time semantic segmentation, instance segmentation and object detection tasks. The efficient models for these problems can be constructed using modifications in both the feature extractor part for more valid feature set extraction and head part for more time-efficient solution of the respective CV task. To achieve real-time performance, we recommend utilizing the modern high-performance implementation and optimization techniques for neural networks.
Sections 4.1 and 4.2 summarize the state-of-the-art achievements and trends both in models for real-time CV tasks and optimizing the implementations of neural networks on parallel architectures.
Different feature extractor (or its block) architectures could be tested as a way of reducing computational complexity while keeping the trade-off with accuracy. Such feature extractors can be based on the EfficientNet V1/V2 [87,88]; MobileNet [89,90,91] or Mobile ViT [92] principles of work rather than base-line ResNet [64,93]. Also some other modifications of ResNet block could be tested [64,94]. Also, a more advanced network training and regularization strategy could include such techniques as cross-data augmentation [95], batch normalization analogs [96] and meta-learning [97] to reduce computational costs while keeping high accuracy.
A survey of real-time semantic segmentation up to 2021 is presented in study [98]. The current (2023) best architectures in are PIDNet [99] and PP-LiteSeg [100]. PIDNet is based on the combination of CNN and Proportional-Integration Derivative (PID) controller to parse the information on details, context and boundary. The boundary information allows it to balance between the context and details and provide the precise annotation around boundary. PP-LiteSeg is a real-time semantic segmentation model based on the lightweight decoder, attention fusion module that utilizes spatial and channel attention to enhance the input feature, and the pyramid pooling module that aggregates global context at low computation cost.
Among approaches for fast object detection that can be tested, we want to note YOLOv5 – YOLOv8 [80,86,101,102] and other models of the YOLO family. All of these models are based on the ideas cited above (see Section 3.4). However, today most of these architectures can be modified in the feature extractor part for different goals [75,76]. Additionally, some of the YOLO family architectures provide real-time instance segmentation mode.
Among the other state-of-the-art results in real-time instance segmentation we can name YOLACT method [84] and their modifications, such as YOLACT++ [103] and SOLOv2 [104]. The discussed methods employ center-based segmentation as opposed to region-based segmentation in Mask R-CNN. Here, the model is trained to distinguish pixels belonging to the same or different objects. For instance, YOLACT [84] generates prototype masks over the entire image, predicting a set of coefficients per instance by two parallel heads. The final masks are constructed after Non-Maximum suppression of predicted instances. The YOLACT++ architecture [103] improves previous results [84] by improving the feature extractor and adding deformable convolutions to the head part. The SOLO architecture [105] assumed that instances can be divided by their center position and sizes. The center positions are calculated by dividing the image into cells and calculating the center position inside each of them. The sizes of instances are determined by a pyramidal feature extractor. The SOLOv2 [104] improves the previous results by generating kernels for each mask. This concept is called a dynamic kernel.
Another model for real-time instance segmentation is proposed in work [106]. It uses the instance activation-guided queries to dynamically pick the pixel embeddings with rich semantic information for the initial queries for the Transformer decoder. A dual-path architecture is used for updating query and pixel features alternately. The ground truth mask-guided learning is used to replace the standard masked attention mechanism. As a result, masked attention can be directed to more appropriate regions.
An efficient fully convolutional framework for real-time instance segmentation is proposed in work [85]. Instead of using object detection and generating mask predictions on the basis of bounding boxes or dense centers, this model uses a new object representation. A sparse set of instance activation maps is used to highlight informative regions for objects. By aggregating features for the highlighted regions, the instance features for recognition and segmentation are obtained. This approach also allows one to avoid using non-maximum suppression in the post-processing phase.
To date (2023), the best performance in real-time segmentation is achieved by RTMDet model [107]. It is based on large-kernel depth-wise convolutions in a basic building block of the backbone and neck in the model. This allows the model to better capture the global context. To reduce the model depth and computational complexity, the number of building block is reduced, and model width is increased.
We should also note that the YOLOv5 and RTMDet models provide the oriented bounding box (or rotated object detection) mode, which extends the object detection task by predicting the slope of a bounding box at a little cost increase. Using this approach instead of traditional object detection may significantly increase the accuracy of some rock fragmentation problems such as particle size distribution estimation.
Table 1 presents the comparison of state-of-the-art real-time instance segmentation models. It contains the values of mask average precision obtained for the COCO dataset, as well as data on inference speed. It also contains the total number of parameters of the model, allowing one to estimate their complexity. Note that modern architectures, such as YOLO and RTMDet, provide models of varying sizes to allow users to achieve the best size-accuracy trade-off for specific application scenarios. The table contains data on the smallest and largest variants of these models. We also show the number of tunable parameters (such as score thresholds and loss functions) estimated from the article text or demonstration code for each model. We do not account for the standard hyper-parameters such as learning rate, batch size, etc. Note that more quantitative comparisons between the state-of-the-art architectures for various CV (and other machine learning) tasks are presented on the "Papers with Code" website*. Of course, achievements in benchmarks and synthetic tests do not guarantee performance in real practical applications.
Model | Year | Tunable parameters | Total parameters (M) | AP (%) | Inference Speed (FPS) |
Mask R-CNN [63] | 2017 | 10 | 63.3 | 35.7 | 5 (Titan XP) |
YOLACT-550 [84] | 2019 | 6 | 50 | 29.8 | 33.3 (Titan XP) |
YOLACT-550++ [103] | 2020 | 6 | No data | 34.6 | 27.3 (Titan XP) |
SOLOv2-512 [105] | 2020 | 7 | 46.4 | 37.1 | 31.3 (V100) |
SparseInst [85] | 2022 | 4 | 33.3 | 37.9 | 40 (RTX 2080 Ti) |
FastInst [106] | 2023 | 20 | 53 | 39.9 | 28 FPS (V100) |
YOLOv5n-seg [80] | 2020 | 20+ | 2 | 23.4 | 833 (A100) |
YOLOv5x-seg | 2020 | 20+ | 88.8 | 41.4 | 222 (A100) |
YOLOv8n-seg | 2022 | 20+ | 3.4 | 36.7 | 826 (A100) |
YOLOv8x-seg | 2022 | 20+ | 71.8 | 43.4 | 248 (A100) |
RTMDet-Ins-s [107] | 2022 | 6 | 5.6 | 38.7 | 518 (RTX 3090) |
RTMDet-Ins-x | 2022 | 6 | 102.7 | 44.6 | 188 (RTX 3090) |
*https://paperswithcode.com/sota
Understanding of how tunable parameters influence model performance is a separate research. For example, the work [108] describes the collection of empirical techniques and refinements used to improve the accuracy of CNN models, in particular, the ResNet-50 feature extractor. Similarly, study [86] presents the model re-parameterization and model scaling methods for improving the YOLOv7 object detection model. Work [102] describes the model enhancement and training techniques used to boost the performance of YOLOv6 architecture so that in real-time object detection the new YOLOv6 v3.0 can compete with the latest YOLO models of similar size. A survey on hyper-parameter optimization methods is presented in study [109].
We would also like to acknowledge the latest achievements in the field of instance and semantic segmentation based on the Segment Anything [110] and Diffusion [111,112,113] approaches, although these methods have yet to prove their effectiveness in real-time applications in general and in rock fragmentation problems in particular.
Key trends in optimizing DL neural network implementations include quantization, pruning, hardware and architecture co-design, knowledge distillation and parallelization techniques for high-performance systems.
Hardware manufacturers and software developers introduce capabilities for mixed- and reduced-precision calculations, as well as sparse computations, to support quantization and pruning techniques [114,115]. These techniques enable model size reduction and computation time reduction, making trained models applicable to lightweight devices. The comprehensive survey of quantization techniques and specialized frameworks for mixed-precision neural networks is presented in work [116]. Automatic tools for adaptive application of mixed-precision techniques are available in modern neural network frameworks [117,118,119,120].
Various hardware architectures, including CPUs, GPUs and specialized accelerators based on FPGA and ASIC technologies are employed for neural network implementations. While GPUs have significant potential for training and inference, their price and energy efficiency are often suboptimal. Lightweight specialized hardware accelerators offer better for energy efficiency, but their optimal performance requires full utilization of the hardware architecture. Hardware/software co-design strategies for implementing convolutional neural networks are extensively surveyed in work [121].
Knowledge distillation involves training a smaller student model from a pre-trained larger teacher model. A comprehensive study covering training schemes, architectures, distillation algorithms, performance comparison and applications of knowledge distillation is available in the survey [122].
To efficiently utilize the power of massive parallel systems, such as multi-GPU ones, for training DL networks, specialized parallelism strategies like data parallelism, model parallelism, pipelining or hybrid approaches are employed. Challenges and recent achievements in adopting these strategies are explored in survey [123].
This study presents a comprehensive survey of the application of DL-based CV systems for rock fragmentation estimation and related tasks in the mining industry, focusing on recent advancements and current research trends. Additionally, a brief summary is provided of state-of-the-art achievements in real-time semantic segmentation, instance segmentation, object detection and performance optimization of DL neural networks.
The problem of rock fragmentation consists of identification and differentiation of multiple rock chunks of various sizes from visual data. This review reveals a lack of consensus on both the choice of general CV approaches and individual machine learning models for solving these problems. Most studies in the field concentrate on size distribution estimation of rock fragments using either semantic segmentation or instance segmentation, while object detection has gained more recent attention.
Through the review, it was observed that contemporary methods in rock fragmentation lag behind the overall progress in CV over the past 2-3 years. Many studies primarily focus on baseline DL architectures such as U-Net, Mask R-CNN and YOLO for semantic segmentation, instance segmentation and object detection, respectively. Promising approaches for the task involve real-time DL instance segmentation or object detection (including oriented object detection) architectures utilizing various feature encoders and head parts. This could include considering new versions of the YOLO family (YOLO v7, v8) and RTMDet models, as well as optimizing models with feature extractors such as EfficientNet, modern ResNet-based or mobile ViT-based blocks. Improving model accuracy can also be achieved through meta-learning or other regularization techniques. However, the specific architecture choice, pros and cons of different approaches, and recommendations for usage require separate research.
The majority of studies in this field employ parallel computing architectures for training and inference, typically utilizing workstations with graphics processors, without performance optimization. Modern techniques for optimizing the performance and size of DL models include mixed-precision arithmetic and sparse matrix computations. Lightweight DL models can be trained from larger pretrained models using knowledge distillation methods. Several strategies for parallelizing deep neural networks on massive parallel systems can be identified, including data parallelism, model parallelism, pipelining and hybridization. Implementing these techniques would enable researchers in the field of rock fragmentation to achieve higher performance, both in training their models on high-performance systems in data centers and in inference on specialized low-performance computing devices in industrial installations.
In conclusion, progress in the field of rock fragmentation can be achieved by striking a balance between state-of-the-art deep learning computer vision architectures and optimizing work performance using modern techniques.
The authors declare they have not used Artificial Intelligence (AI) tools in the creation of this article.
This research was supported by the Russian Science Foundation and Government of Sverdlovsk region, Joint Grant No 22-21-20051, https://rscf.ru/en/project/22-21-20051/.
The authors declare that they have no known competing financial interests or personal relationships that could have appeared to influence the work reported in this paper.
The following table presents the works on the survey topic. Important works summarized in Section 3 are marked in bold. The studies in the table are sorted by publication year and grouped by practical field of rock fragmentation problem.
Reference | Year | Practical Problem | CV Task | Approach | Specifics | Primary CV Model |
[124] | 2023 | Conveyor | Semantic | DL | SLIC + U-Net | U-Net |
[41] | 2022 | Conveyor | Object | DL | SSD, YOLOv3, YOLOv4, YOLOv5, DCN-YOLOv3 | YOLO |
[79] | 2022 | Conveyor | Object | DL | Yolo3, SPPNet, Yolo1, 2 | YOLO |
[1] | 2022 | Conveyor | Classification | DL | AlexNet, VGG16, ResNet50, InceptionV3, MobileNet, SENet | MobileNet |
[125] | 2021 | Conveyor | Semantic | DL | DexiNed Edge Detection + Morphologic + Watershed | Other |
[18] | 2021 | Conveyor | Semantic | DL | U-Net | U-Net |
[14] | 2020 | Conveyor | Semantic | DL | U-Net | U-Net |
[15] | 2020 | Conveyor | Semantic | DL | U-Net, Res-U-Net + Watershed | U-Net |
[59] | 2020 | Conveyor | Semantic | DL | U-Net, RDUNET, DUNET | U-Net |
[126] | 2020 | Conveyor | Semantic | DL | U-Net + Watershed | U-Net |
[127] | 2020 | Conveyor | Semantic | DL | CNN + Watershed | Watershed |
[128] | 2019 | Conveyor | Semantic | Classical | Otsu + Histogram accumulation moment | Other |
[129] | 2019 | Conveyor | Other | Classical | Super Voxel 3D | Other |
[20] | 2023 | Open Pit | Instance | DL | Mask R-CNN | Mask R-CNN |
[47] | 2022 | Open Pit | Instance | Classical | Modified Watershed | Watershed |
[70] | 2022 | Open Pit | Instance | DL | ResNeXt101 + SE | Mask R-CNN |
[68] | 2022 | Open Pit | Instance | DL | ResNet-50 | Mask R-CNN |
[42] | 2022 | Open Pit | Object | DL | YOLO | YOLO |
[58] | 2021 | Open Pit | Semantic | DL | Dual U-Net, boundary and regions | U-Net |
[46] | 2021 | Open Pit | Semantic | Classical | Wavelet + Superpixel segmentation | Other |
[12] | 2021 | Open Pit | Instance | DL | Mask R-CNN | Mask R-CNN |
[130] | 2021 | Open Pit | Instance | Classical | Super-Voxel Segmentation | Other |
[67] | 2021 | Open Pit | Instance | DL | Mask R-CNN | Mask R-CNN |
[131] | 2021 | Open Pit | Instance | Classical | Watershed | Watershed |
[40] | 2021 | Open Pit | Instance | DL | Mask R-CNN | Mask R-CNN |
[7] | 2021 | Open Pit | Classification | Classical | ResNeXt-50 | ResNet |
[132] | 2021 | Open Pit | Classification | Classical | SM, GMB, SVM + Firefly evolutionary algorithm | Other |
[133] | 2021 | Open Pit | Classification | Classical | ANN, Monte Carlo dropout | Other |
[134] | 2021 | Open Pit | Other | Classical | Power Sieve3 software | Other |
[82] | 2020 | Open Pit | Classification | DL | VGG-16 | VGG |
[21] | 2019 | Open Pit | Instance | Classical | Split-Desktop Software | Other |
[6] | 2019 | Open Pit | Other | Classical | Custom | Other |
[135] | 2018 | Open Pit | Classification | Classical | SVM | Other |
[136] | 2013 | Open Pit | Classification | Classical | ANN | Other |
[137] | 2009 | Open Pit | Instance | Classical | Watershed | Watershed |
[22] | 2005 | Open Pit | Instance | Classical | Watershed | Watershed |
[138] | 2003 | Open Pit | Other | Classical | WipFrag software | Other |
[56] | 2021 | Tunnel | Semantic | DL | FCN8s, GCN, DFN, DeepLabV3, PSPNet | DFN |
[57] | 2021 | Tunnel | Semantic | DL | Dual U-Net, boundary and regions | U-Net |
[55] | 2021 | Tunnel | Instance | DL | SSD + U-Net + post-processing | U-Net |
[81] | 2021 | Tunnel | Classification | DL | AlexNet | Other |
[83] | 2021 | Tunnel | Classification | DL | ResNet + GoogLeNet | ResNet |
[139] | 2013 | Tunnel | Instance | Classical | Watershed | Watershed |
[140] | 2011 | Tunnel | Instance | Classical | Watershed | Watershed |
[141] | 2023 | Other | Semantic | DL | MSBA-Unet + CDD post-processing | U-Net |
[43] | 2023 | Other | Object | DL | YOLO | YOLO |
[78] | 2023 | Other | Object | DL | Watershed, FASTER-R-CNN, YOLO3 | YOLO |
[142] | 2022 | Other | Semantic | DL | Swin-Unet | U-Net |
[143] | 2022 | Other | Semantic | DL | DeepLab3+ | DeepLab |
[77] | 2022 | Other | Object | DL | DCN-YOLOv3 | YOLO |
[144] | 2022 | Other | Classification | Classical | ANN + SVR | Other |
[145] | 2021 | Other | Semantic | DL | GAN + U-Net | U-Net |
[60] | 2021 | Other | Semantic | DL | FraSegNet: VGG19 + ASPP | Other |
[23] | 2021 | Other | Instance | Other | ResNet50 | Mask R-CNN |
[69] | 2021 | Other | Instance | DL | Mask R-CNN | Mask R-CNN |
[146] | 2021 | Other | Classification | DL | AlexNet, VGG | VGG |
[2] | 2021 | Other | Other | Classical | Custom | Other |
[147] | 2020 | Other | Semantic | DL | Lightweight U-Net + Morphologic + Watershed | U-Net |
[71] | 2020 | Other | Instance | DL | Mask R-CNN + FCN | Mask R-CNN |
[3] | 2020 | Other | Object | DL | Faster R-CNN | Faster R-CNN |
[148] | 2020 | Other | Classification | Classical | Edge Detection | Other |
[16] | 2020 | Other | Other | DL | Custom CNN | Other |
[17] | 2020 | Other | Other | Classical | Custom | Other |
[4] | 2019 | Other | Semantic | DL | SegNet | Other |
[5] | 2019 | Other | Instance | Classical | SLIC Segmentation + Features + Classification | Other |
[149] | 2019 | Other | Instance | Classical | Wavelet + manual features + ANN | Other |
[150] | 2019 | Other | Instance | Classical | Morphological Reconstruction | Other |
[151] | 2019 | Other | Instance | Classical | Otsu + Watershed | Watershed |
[152] | 2018 | Other | Semantic | DL | Holistically-nested convolutional network | Other |
[153] | 2018 | Other | Instance | Classical | Morphologic + Watershed | Watershed |
[154] | 2018 | Other | Instance | Classical | Thresholding + Watershed | Watershed |
[26] | 2018 | Other | Other | Classical | WipFrag software | Other |
[155] | 2016 | Other | Classification | Classical | Morphologic + Watershed + SVM for classification | Watershed |
[156] | 2016 | Other | Other | Classical | WipFrag software | Other |
[157] | 2015 | Other | Classification | Classical | ANN + post-processing | Other |
[158] | 2013 | Other | Classification | Classical | ANN + Regression | Other |
[159] | 2013 | Other | Other | Classical | GoldSize software | Other |
[160] | 2012 | Other | Classification | Classical | SVM | Other |
[161] | 2011 | Other | Instance | Classical | Watershed | Watershed |
[163] | 2010 | Other | Instance | Classical | Watershed | Watershed |
[164] | 2009 | Other | Semantic | Classical | Thresholding + morphologic | Other |
[165] | 2009 | Other | Classification | Classical | Fuzzy Logic | Other |
[166] | 2008 | Other | Semantic | Classical | Edge Detection | Other |
[167] | 2005 | Other | Other | Classical | Laser 3d + Watershed | Other |
[168] | 1999 | Other | Classification | Classical | Morphologic + ANN for classification | Other |
[169] | 1996 | Other | Other | Classical | Image Preparation | Other |
[1] | J. M. Mendel, Uncertain rule-based fuzzy systems, Springer International Publishing, 2017. https://doi.org/10.1007/978-3-319-51370-6 |
[2] |
H. Hagras, C. Wagner, Towards the wide spread use of type-2 fuzzy logic systems in real world applications, IEEE Comput. Intell. M., 7 (2012), 14–24. https://doi.org/10.1109/MCI.2012.2200621 doi: 10.1109/MCI.2012.2200621
![]() |
[3] |
J. M. Mendel, Type-2 fuzzy sets and systems: An overview, IEEE Comput. Intell. M., 2 (2007), 20–29. https://doi.org/10.1109/MCI.2007.380672 doi: 10.1109/MCI.2007.380672
![]() |
[4] |
M. H. F. Zarandi, B. Rezaee, I. B. Turksen, E. Neshat, A type-2 fuzzy rule-based expert system model for stock price analysis, Expert Syst. Appl., 36 (2009), 139–154. https://doi.org/10.1016/j.eswa.2007.09.034 doi: 10.1016/j.eswa.2007.09.034
![]() |
[5] |
Y. Chen, D. Z. Wang, S. C. Tong, Forecasting studies by designing Mamdani interval type-2 fuzzy logic systems: with combination of BP algorithms and KM algorithms, Neurocomputing, 174 (2016), 1133–1146. https://doi.org/10.1016/j.neucom.2015.10.032 doi: 10.1016/j.neucom.2015.10.032
![]() |
[6] |
A. Khosravi, S. Nahavandi, Load forecasting using interval type-2 fuzzy logic systems: Optimal type reduction, IEEE T. Ind. Electron., 10 (2014), 1055–1063. https://doi.org/10.1109/TII.2013.2285650 doi: 10.1109/TII.2013.2285650
![]() |
[7] |
M. Biglarbegian, W. W. Melek, J. M. Mendel, Design of novel interval type-2 fuzzy controllers for modular and reconfigurable robots: theory and experiments, IEEE T. Ind. Electron., 58 (2011), 1371–1384. https://doi.org/10.1109/TIE.2010.2049718 doi: 10.1109/TIE.2010.2049718
![]() |
[8] |
D. Z. Wang, Y. Chen, Study on permanent magnetic drive forecasting by designing Takagi Sugeno Kang type interval type-2 fuzzy logic systems, T. I. Meas. Control, 40 (2018), 2011–2023. https://doi.org/10.1177/0142331217694682 doi: 10.1177/0142331217694682
![]() |
[9] |
R. S. Rama, P. Latha, An effective torque ripple reduction for permanent magnet synchronous motor using ant colony optimization, Int. J. Fuzzy Syst., 17 (2015), 577–584. https://doi.org/10.1007/s40815-015-0077-5 doi: 10.1007/s40815-015-0077-5
![]() |
[10] |
O. Linda, M. Manic, Interval type-2 voter design for fault tolerant systems, Inform. Sci., 181 (2011), 2933–2950. https://doi.org/10.1016/j.ins.2011.03.008 doi: 10.1016/j.ins.2011.03.008
![]() |
[11] |
A. Niewiadomski, On finity, countability, cardinalities, and cylindric extensions of type-2 fuzzy sets in linguistic summarization of databases, IEEE T. Fuzzy Syst., 18 (2010), 532–545. https://doi.org/10.1109/TFUZZ.2010.2042719 doi: 10.1109/TFUZZ.2010.2042719
![]() |
[12] |
D. R. Wu, J. M. Mendel, Uncertainty measures for interval type-2 fuzzy sets, Inform. Sci., 177 (2007), 5378–2393. https://doi.org/10.1016/j.ins.2007.07.012 doi: 10.1016/j.ins.2007.07.012
![]() |
[13] |
A. Khosravi, S. Nahavandi, D. Creighton, D. Srinivasan, Interval type-2 fuzzy logic systems for load forecasting: a comparative study, IEEE T. Power Syst., 27 (2012), 1274–1282. https://doi.org/10.1109/TPWRS.2011.2181981 doi: 10.1109/TPWRS.2011.2181981
![]() |
[14] |
Y. Chen, Study on weighted Nagar-Bardini algorithms for centroid type-reduction of interval type-2 fuzzy logic systems, J. Intell. Fuzzy Syst., 34 (2018), 2417–2428. https://doi.org/10.3233/JIFS-171669 doi: 10.3233/JIFS-171669
![]() |
[15] |
J. M. Mendel, On KM algorithms for solving type-2 fuzzy sets problems, IEEE T. Fuzzy Syst., 21 (2013), 426–446. https://doi.org/10.1109/TFUZZ.2012.2227488 doi: 10.1109/TFUZZ.2012.2227488
![]() |
[16] |
T. Kumbasar, Revisiting Karnik-Mendel algorithms in the framework of linear fractional programming, Int. J. Approx. Reason., 82 (2017), 1–21. https://doi.org/10.1016/j.ijar.2016.11.019 doi: 10.1016/j.ijar.2016.11.019
![]() |
[17] |
J. M. Mendel, F. L. Liu, Super-exponential convergence of the Karnik-Mendel algorithms for computing the centroid of an interval type-2 fuzzy set, IEEE T. Fuzzy Syst., 15 (2007), 309–320. https://doi.org/10.1109/TFUZZ.2006.882463 doi: 10.1109/TFUZZ.2006.882463
![]() |
[18] |
D. R. Wu, J. M. Mendel, Perceptual reasoning for perceptual computing: a similarity based approach, IEEE T. Fuzzy Syst., 17 (2009), 1397–1411. https://doi.org/10.1109/TFUZZ.2009.2032652 doi: 10.1109/TFUZZ.2009.2032652
![]() |
[19] |
D. R. Wu, J. M. Mendel, Enhanced Karnik-Mendel algorithms, IEEE T. Fuzzy Syst., 17 (2009), 923–934. https://doi.org/10.1109/TFUZZ.2008.924329 doi: 10.1109/TFUZZ.2008.924329
![]() |
[20] |
X. W. Liu, J. M. Mendel, D. R. Wu, Study on enhanced Karnik-Mendel algorithms: initialization explanations and computation improvements, Inform. Sci., 184 (2012), 75–91. https://doi.org/10.1016/j.ins.2011.07.042 doi: 10.1016/j.ins.2011.07.042
![]() |
[21] |
Y. Chen, Study on sampling-based discrete noniterative algorithms for centroid type-reduction of interval type-2 fuzzy logic systems, Soft Comput., 24 (2020), 11819–11828. https://doi.org/10.1007/s00500-020-04998-2 doi: 10.1007/s00500-020-04998-2
![]() |
[22] |
O. Castillo, P. Melin, E. Ontiveros, C. Peraza, P. Ochoa, F. Valdez, et al., A high-speed interval type 2 fuzzy system approach for dynamic parameter adaptation in metaheuristics, Eng. Appl. Artif. Intell., 85 (2019), 666–680. https://doi.org/10.1016/j.engappai.2019.07.020 doi: 10.1016/j.engappai.2019.07.020
![]() |
[23] |
G. M. Méndez, M. D. L. A. Hernandez, Hybrid learning mechanism for interval A2-C1 type-2 non-singleton type-2 Takagi-Sugeno-Kang fuzzy logic systems, Inform. Sci., 220 (2013), 149–169. https://doi.org/10.1016/j.ins.2012.01.024 doi: 10.1016/j.ins.2012.01.024
![]() |
[24] |
J. M. Mendel, Type-2 fuzzy sets and systems: an overview, IEEE Comput. Intell. Mag., 2 (2007), 20–29. https://doi.org/10.1109/MCI.2007.380672 doi: 10.1109/MCI.2007.380672
![]() |
[25] |
Y. Chen, D. Z. Wang, Study on centroid type-reduction of general type-2 fuzzy logic systems with weighted enhanced Karnik-Mendel algorithms, Soft Comput., 22 (2018), 1361–1380. https://doi.org/10.1007/s00500-017-2938-3 doi: 10.1007/s00500-017-2938-3
![]() |
[26] |
Y. Chen, D. Z. Wang, Study on centroid type-reduction of general type-2 fuzzy logic systems with weighted Nie-Tan algorithms, Soft Comput., 22 (2018), 7659–7678. https://doi.org/10.1007/s00500-018-3551-9 doi: 10.1007/s00500-018-3551-9
![]() |
[27] |
D. R. Wu, Approaches for reducing the computational cost of interval type-2 fuzzy logic systems: overview and comparisons, IEEE T. Fuzzy Syst., 21 (2013), 80–99. https://doi.org/10.1109/TFUZZ.2012.2201728 doi: 10.1109/TFUZZ.2012.2201728
![]() |
[28] | T. Wang, Y. Chen, S. C. Tong, Fuzzy reasoning models and algorithms on type-2 fuzzy sets, Int. J. Innov. Comput. Inform. Control, 24 (2008), 2451–2460. |
[29] |
J. W. Li, R. John, S. Coupland, G. Kendall, On Nie-Tan operator and type-reduction of interval type-2 fuzzy sets, IEEE T. Fuzzy Syst., 26 (2018), 1036–1039. https://doi.org/10.1109/TFUZZ.2017.2666842 doi: 10.1109/TFUZZ.2017.2666842
![]() |
[30] |
S. Greenfield, F. Chiclana, Accuracy and complexity evaluation of defuzzification strategies for the discretised interval type-2 fuzzy set, Int. J. Approx. Reason., 54 (2013), 1013–1033. https://doi.org/10.1016/j.ijar.2013.04.013 doi: 10.1016/j.ijar.2013.04.013
![]() |
[31] |
E. Ontiveros-Robles, P. Melin, O. Castillo, New methodology to approximate type-reduction based on a continuous root-finding karnik mendel algorithm, Algorithms, 10 (2017), 77–96. https://doi.org/10.3390/a10030077 doi: 10.3390/a10030077
![]() |
[32] |
Y. Chen, J. X. Wu, J. Lan, Study on reasonable initialization enhanced Karnik-Mendel algorithms for centroid type-reduction of interval type-2 fuzzy logic systems, AIMS Math., 5 (2020), 6149–6168. https://doi.org/10.3934/math.2020395 doi: 10.3934/math.2020395
![]() |
[33] |
J. M. Mendel, X. W. Liu, Simplified interval type-2 fuzzy logic systems, IEEE T. Fuzzy Syst., 21 (2013), 1056–1069. https://doi.org/10.1109/TFUZZ.2013.2241771 doi: 10.1109/TFUZZ.2013.2241771
![]() |
[34] |
M. A. Khanesar, A. Jalalian, O. Kaynak, H. Gao, Improving the speed of center of sets type reduction in interval type-2 fuzzy systems by eliminating the need for sorting, IEEE T. Fuzzy Syst., 25 (2017), 1193–1206. https://doi.org/10.1109/TFUZZ.2016.2602392 doi: 10.1109/TFUZZ.2016.2602392
![]() |
[35] |
J. M. Mendel, General type-2 fuzzy logic systems made simple: A tutorial, IEEE T. Fuzzy Syst., 22 (2014), 1162–1182. https://doi.org/10.1109/TFUZZ.2013.2286414 doi: 10.1109/TFUZZ.2013.2286414
![]() |
[36] |
C. H. Hsu, C. F. Juang, Evolutionary robot wall-following control using type-2 fuzzy controller with species-de-activated continuous ACO, IEEE T. Fuzzy Syst., 21 (2013), 100–112. https://doi.org/10.1109/TFUZZ.2012.2202665 doi: 10.1109/TFUZZ.2012.2202665
![]() |
[37] |
Y. Chen, D. Z. Wang, W. Ning, Forecasting by TSK general type-2 fuzzy logic systems optimized with genetic algorithms, Optim. Control Appl. Method., 39 (2018), 393–409. https://doi.org/10.1002/oca.2353 doi: 10.1002/oca.2353
![]() |
[38] |
F. Gaxiola, P. Melin, F. Valdez, J. R. Castro, O. Castillo, Optimization of type-2 fuzzy weights in backpropagation learning for neural networks using GAs and PSO, Appl. Soft Comput., 38 (2016), 860–871. https://doi.org/10.1016/j.asoc.2015.10.027 doi: 10.1016/j.asoc.2015.10.027
![]() |
[39] |
Y. Chen, D. Z. Wang, Forecasting by general type-2 fuzzy logic systems optimized with QPSO algorithms, Int. J. Control Autom. Syst., 15 (2017), 2950–2958. https://doi.org/10.1007/s12555-017-0793-0 doi: 10.1007/s12555-017-0793-0
![]() |
[40] |
Y. Maldonado, O. Castillo, P. Melin, Particle swarm optimization of interval type-2 fuzzy systems for FPGA applications, Appl. Soft Comput., 13 (2013), 496–508. https://doi.org/10.1016/j.asoc.2012.08.032 doi: 10.1016/j.asoc.2012.08.032
![]() |
[41] |
E. Ontiveros-Robles, P. Melin, O. Castillo, Comparative analysis of noise robustness of type 2 fuzzy logic controllers, Kybernetika, 54 (2018), 175–201. https://doi.org/10.14736/kyb-2018-1-0175 doi: 10.14736/kyb-2018-1-0175
![]() |
[42] |
L. Cervantes, O. Castillo, Type-2 fuzzy logic aggregation of multiple fuzzy controllers for airplane flight control, Inform. Sci., 324 (2015), 247–256. https://doi.org/10.1016/j.ins.2015.06.047 doi: 10.1016/j.ins.2015.06.047
![]() |
[43] |
O. Castillo, L. Amador-Angulo, J. R. Castro, M. Garcia-Valdez, A comparative study of type-1 fuzzy logic systems, interval type-2 fuzzy logic systems and generalized type-2 fuzzy logic systems in control problems, Inform. Sci., 354 (2016), 257–274. https://doi.org/10.1016/j.ins.2016.03.026 doi: 10.1016/j.ins.2016.03.026
![]() |
[44] |
C. W. Tao, J. S. Taur, C. W. Chang, Y. H. Chang, Simplified type-2 fuzzy sliding controller for wing rocket system, Fuzzy Set. Syst., 207 (2012), 111–129. https://doi.org/10.1016/j.fss.2012.02.015 doi: 10.1016/j.fss.2012.02.015
![]() |
[45] |
D. R. Wu, J. M. Mendel, Recommendations on designing practical interval type-2 fuzzy systems, Eng. Appl. Artif. Intell., 85 (2019), 182–193. https://doi.org/10.1016/j.engappai.2019.06.012 doi: 10.1016/j.engappai.2019.06.012
![]() |
[46] |
S. C. Tong, Y. M. Li, Observer-based adaptive fuzzy backstepping control of uncertain pure-feedback systems, Sci. China Inform. Sci., 57 (2014), 1–14. https://doi.org/10.1007/s11432-013-5043-y doi: 10.1007/s11432-013-5043-y
![]() |
[47] |
M. Deveci, I. Z. Akyurt, S. Yavuz, GIS-based interval type-2 fuzzy set for public bread factory site selection, J. Enterp. Inform. Manag., 31 (2018), 820–847. https://doi.org/10.1108/JEIM-02-2018-0029 doi: 10.1108/JEIM-02-2018-0029
![]() |
[48] |
S. C. Tong, Y. M. Li, Robust adaptive fuzzy backstepping output feedback tracking control for nonlinear system with dynamic uncertainties, Sci. China Inform. Sci., 53 (2010), 307–324. https://doi.org/10.1007/s11432-010-0031-y doi: 10.1007/s11432-010-0031-y
![]() |
[49] | F. Y. Wang, H. Mo, Some fundamental issues on type-2 fuzzy sets, Acta Autom. Sin., 43 (2017), 1114–1141. |
[50] |
H. Mo, F. Y. Wang, M. Zhou, R. Li, Z. Xiao, Footprint of uncertainty for type-2 fuzzy sets, Inform. Sci., 272 (2014), 96–110. https://doi.org/10.1016/j.ins.2014.02.092 doi: 10.1016/j.ins.2014.02.092
![]() |
[51] |
Y. Chen, J. X. Yang, Study on center-of-sets type-reduction of interval type-2 fuzzy logic systems with noniterative algorithms, J. Intell. Fuzzy Syst., 40 (2021), 11099–11106. https://doi.org/10.3233/JIFS-202264 doi: 10.3233/JIFS-202264
![]() |
[52] |
X. Tao, J. Yi, Z. Pu, T. Xiong, Robust adaptive tracking control for hypersonic vehicle based on interval type-2 fuzzy logic system and small-gain approach, IEEE T. Cybernetics, 51 (2021), 2504–2517. https://doi.org/10.1109/TCYB.2019.2927309 doi: 10.1109/TCYB.2019.2927309
![]() |
[53] |
Y. Chen, J. X. Yang, Design of back propagation optimized Nagar-Bardini structure-based interval type-2 fuzzy logic systems for fuzzy identification, T. I. Meas. Control, 43 (2021), 2780–2787. https://doi.org/10.1177/01423312211006635 doi: 10.1177/01423312211006635
![]() |
[54] | L. Wu, F. Qian, L. Wang, X. Ma, An improved type-reduction algorithm for general type-2 fuzzy sets, Inform. Sci., 2022. |
[55] |
Y. Chen, Study on weighted-based noniterative algorithms for computing the centroids of general type-2 fuzzy sets, Int. J. Fuzzy Syst., 24 (2022), 587–606. https://doi.org/10.1007/s40815-021-01166-y doi: 10.1007/s40815-021-01166-y
![]() |
[56] |
C. Chen, D. Wu, J. M. Garibaldi, R. I. John, J. Twycross, J. M. Mendel, A comprehensive study of the efficiency of type-reduction algorithms, IEEE T. Fuzzy Syst., 29 (2020), 1556–1566. https://doi.org/10.1109/TFUZZ.2020.2981002 doi: 10.1109/TFUZZ.2020.2981002
![]() |
1. | Meennapa Rukhiran, Songwut Boonsong, Paniti Netinant, Sustainable Optimizing Performance and Energy Efficiency in Proof of Work Blockchain: A Multilinear Regression Approach, 2024, 16, 2071-1050, 1519, 10.3390/su16041519 | |
2. | Bo Lu, Junwu Zhou, Yifei Zhang, Yang Liu, Qingkai Wang, An alternative rotating object detection method for rock particle size distribution analysis, 2024, 444, 00325910, 120059, 10.1016/j.powtec.2024.120059 | |
3. | Zhenhua Wang, Guangshi Zhang, Kuifeng Luan, Congqin Yi, Mingjie Li, Image-Fused-Guided Underwater Object Detection Model Based on Improved YOLOv7, 2023, 12, 2079-9292, 4064, 10.3390/electronics12194064 | |
4. | Jian Lei, Yufei Fan, Rock CT Image Fracture Segmentation Based on Convolutional Neural Networks, 2024, 57, 0723-2632, 5883, 10.1007/s00603-024-03824-7 | |
5. | Masoud S. Bahraini, Iman Atighi, A novel intelligent stereo vision approach for blast-induced fragmentation size distribution: Case study at Golgohar open-pit mine, Iran, 2024, 215, 08926875, 108822, 10.1016/j.mineng.2024.108822 | |
6. | Yudi Tang, Yulin Wang, Xin Wang, Joung Oh, Guangyao Si, Automated Scene-Adaptive Rock Fragment Recognition Based on the Enhanced Segment Anything Model and Fine-Tuning RTMDet, 2025, 0723-2632, 10.1007/s00603-024-04360-0 | |
7. | Guoqiang Huang, Chengjin Qin, Tao Zhong, Chengliang Liu, A novel multi-scale hybrid connected neural network for anti-noise rock fragmentation classification of tunnel boring machine, 2025, 161, 08867798, 106555, 10.1016/j.tust.2025.106555 |
Model | Year | Tunable parameters | Total parameters (M) | AP (%) | Inference Speed (FPS) |
Mask R-CNN [63] | 2017 | 10 | 63.3 | 35.7 | 5 (Titan XP) |
YOLACT-550 [84] | 2019 | 6 | 50 | 29.8 | 33.3 (Titan XP) |
YOLACT-550++ [103] | 2020 | 6 | No data | 34.6 | 27.3 (Titan XP) |
SOLOv2-512 [105] | 2020 | 7 | 46.4 | 37.1 | 31.3 (V100) |
SparseInst [85] | 2022 | 4 | 33.3 | 37.9 | 40 (RTX 2080 Ti) |
FastInst [106] | 2023 | 20 | 53 | 39.9 | 28 FPS (V100) |
YOLOv5n-seg [80] | 2020 | 20+ | 2 | 23.4 | 833 (A100) |
YOLOv5x-seg | 2020 | 20+ | 88.8 | 41.4 | 222 (A100) |
YOLOv8n-seg | 2022 | 20+ | 3.4 | 36.7 | 826 (A100) |
YOLOv8x-seg | 2022 | 20+ | 71.8 | 43.4 | 248 (A100) |
RTMDet-Ins-s [107] | 2022 | 6 | 5.6 | 38.7 | 518 (RTX 3090) |
RTMDet-Ins-x | 2022 | 6 | 102.7 | 44.6 | 188 (RTX 3090) |
Reference | Year | Practical Problem | CV Task | Approach | Specifics | Primary CV Model |
[124] | 2023 | Conveyor | Semantic | DL | SLIC + U-Net | U-Net |
[41] | 2022 | Conveyor | Object | DL | SSD, YOLOv3, YOLOv4, YOLOv5, DCN-YOLOv3 | YOLO |
[79] | 2022 | Conveyor | Object | DL | Yolo3, SPPNet, Yolo1, 2 | YOLO |
[1] | 2022 | Conveyor | Classification | DL | AlexNet, VGG16, ResNet50, InceptionV3, MobileNet, SENet | MobileNet |
[125] | 2021 | Conveyor | Semantic | DL | DexiNed Edge Detection + Morphologic + Watershed | Other |
[18] | 2021 | Conveyor | Semantic | DL | U-Net | U-Net |
[14] | 2020 | Conveyor | Semantic | DL | U-Net | U-Net |
[15] | 2020 | Conveyor | Semantic | DL | U-Net, Res-U-Net + Watershed | U-Net |
[59] | 2020 | Conveyor | Semantic | DL | U-Net, RDUNET, DUNET | U-Net |
[126] | 2020 | Conveyor | Semantic | DL | U-Net + Watershed | U-Net |
[127] | 2020 | Conveyor | Semantic | DL | CNN + Watershed | Watershed |
[128] | 2019 | Conveyor | Semantic | Classical | Otsu + Histogram accumulation moment | Other |
[129] | 2019 | Conveyor | Other | Classical | Super Voxel 3D | Other |
[20] | 2023 | Open Pit | Instance | DL | Mask R-CNN | Mask R-CNN |
[47] | 2022 | Open Pit | Instance | Classical | Modified Watershed | Watershed |
[70] | 2022 | Open Pit | Instance | DL | ResNeXt101 + SE | Mask R-CNN |
[68] | 2022 | Open Pit | Instance | DL | ResNet-50 | Mask R-CNN |
[42] | 2022 | Open Pit | Object | DL | YOLO | YOLO |
[58] | 2021 | Open Pit | Semantic | DL | Dual U-Net, boundary and regions | U-Net |
[46] | 2021 | Open Pit | Semantic | Classical | Wavelet + Superpixel segmentation | Other |
[12] | 2021 | Open Pit | Instance | DL | Mask R-CNN | Mask R-CNN |
[130] | 2021 | Open Pit | Instance | Classical | Super-Voxel Segmentation | Other |
[67] | 2021 | Open Pit | Instance | DL | Mask R-CNN | Mask R-CNN |
[131] | 2021 | Open Pit | Instance | Classical | Watershed | Watershed |
[40] | 2021 | Open Pit | Instance | DL | Mask R-CNN | Mask R-CNN |
[7] | 2021 | Open Pit | Classification | Classical | ResNeXt-50 | ResNet |
[132] | 2021 | Open Pit | Classification | Classical | SM, GMB, SVM + Firefly evolutionary algorithm | Other |
[133] | 2021 | Open Pit | Classification | Classical | ANN, Monte Carlo dropout | Other |
[134] | 2021 | Open Pit | Other | Classical | Power Sieve3 software | Other |
[82] | 2020 | Open Pit | Classification | DL | VGG-16 | VGG |
[21] | 2019 | Open Pit | Instance | Classical | Split-Desktop Software | Other |
[6] | 2019 | Open Pit | Other | Classical | Custom | Other |
[135] | 2018 | Open Pit | Classification | Classical | SVM | Other |
[136] | 2013 | Open Pit | Classification | Classical | ANN | Other |
[137] | 2009 | Open Pit | Instance | Classical | Watershed | Watershed |
[22] | 2005 | Open Pit | Instance | Classical | Watershed | Watershed |
[138] | 2003 | Open Pit | Other | Classical | WipFrag software | Other |
[56] | 2021 | Tunnel | Semantic | DL | FCN8s, GCN, DFN, DeepLabV3, PSPNet | DFN |
[57] | 2021 | Tunnel | Semantic | DL | Dual U-Net, boundary and regions | U-Net |
[55] | 2021 | Tunnel | Instance | DL | SSD + U-Net + post-processing | U-Net |
[81] | 2021 | Tunnel | Classification | DL | AlexNet | Other |
[83] | 2021 | Tunnel | Classification | DL | ResNet + GoogLeNet | ResNet |
[139] | 2013 | Tunnel | Instance | Classical | Watershed | Watershed |
[140] | 2011 | Tunnel | Instance | Classical | Watershed | Watershed |
[141] | 2023 | Other | Semantic | DL | MSBA-Unet + CDD post-processing | U-Net |
[43] | 2023 | Other | Object | DL | YOLO | YOLO |
[78] | 2023 | Other | Object | DL | Watershed, FASTER-R-CNN, YOLO3 | YOLO |
[142] | 2022 | Other | Semantic | DL | Swin-Unet | U-Net |
[143] | 2022 | Other | Semantic | DL | DeepLab3+ | DeepLab |
[77] | 2022 | Other | Object | DL | DCN-YOLOv3 | YOLO |
[144] | 2022 | Other | Classification | Classical | ANN + SVR | Other |
[145] | 2021 | Other | Semantic | DL | GAN + U-Net | U-Net |
[60] | 2021 | Other | Semantic | DL | FraSegNet: VGG19 + ASPP | Other |
[23] | 2021 | Other | Instance | Other | ResNet50 | Mask R-CNN |
[69] | 2021 | Other | Instance | DL | Mask R-CNN | Mask R-CNN |
[146] | 2021 | Other | Classification | DL | AlexNet, VGG | VGG |
[2] | 2021 | Other | Other | Classical | Custom | Other |
[147] | 2020 | Other | Semantic | DL | Lightweight U-Net + Morphologic + Watershed | U-Net |
[71] | 2020 | Other | Instance | DL | Mask R-CNN + FCN | Mask R-CNN |
[3] | 2020 | Other | Object | DL | Faster R-CNN | Faster R-CNN |
[148] | 2020 | Other | Classification | Classical | Edge Detection | Other |
[16] | 2020 | Other | Other | DL | Custom CNN | Other |
[17] | 2020 | Other | Other | Classical | Custom | Other |
[4] | 2019 | Other | Semantic | DL | SegNet | Other |
[5] | 2019 | Other | Instance | Classical | SLIC Segmentation + Features + Classification | Other |
[149] | 2019 | Other | Instance | Classical | Wavelet + manual features + ANN | Other |
[150] | 2019 | Other | Instance | Classical | Morphological Reconstruction | Other |
[151] | 2019 | Other | Instance | Classical | Otsu + Watershed | Watershed |
[152] | 2018 | Other | Semantic | DL | Holistically-nested convolutional network | Other |
[153] | 2018 | Other | Instance | Classical | Morphologic + Watershed | Watershed |
[154] | 2018 | Other | Instance | Classical | Thresholding + Watershed | Watershed |
[26] | 2018 | Other | Other | Classical | WipFrag software | Other |
[155] | 2016 | Other | Classification | Classical | Morphologic + Watershed + SVM for classification | Watershed |
[156] | 2016 | Other | Other | Classical | WipFrag software | Other |
[157] | 2015 | Other | Classification | Classical | ANN + post-processing | Other |
[158] | 2013 | Other | Classification | Classical | ANN + Regression | Other |
[159] | 2013 | Other | Other | Classical | GoldSize software | Other |
[160] | 2012 | Other | Classification | Classical | SVM | Other |
[161] | 2011 | Other | Instance | Classical | Watershed | Watershed |
[163] | 2010 | Other | Instance | Classical | Watershed | Watershed |
[164] | 2009 | Other | Semantic | Classical | Thresholding + morphologic | Other |
[165] | 2009 | Other | Classification | Classical | Fuzzy Logic | Other |
[166] | 2008 | Other | Semantic | Classical | Edge Detection | Other |
[167] | 2005 | Other | Other | Classical | Laser 3d + Watershed | Other |
[168] | 1999 | Other | Classification | Classical | Morphologic + ANN for classification | Other |
[169] | 1996 | Other | Other | Classical | Image Preparation | Other |
Model | Year | Tunable parameters | Total parameters (M) | AP (%) | Inference Speed (FPS) |
Mask R-CNN [63] | 2017 | 10 | 63.3 | 35.7 | 5 (Titan XP) |
YOLACT-550 [84] | 2019 | 6 | 50 | 29.8 | 33.3 (Titan XP) |
YOLACT-550++ [103] | 2020 | 6 | No data | 34.6 | 27.3 (Titan XP) |
SOLOv2-512 [105] | 2020 | 7 | 46.4 | 37.1 | 31.3 (V100) |
SparseInst [85] | 2022 | 4 | 33.3 | 37.9 | 40 (RTX 2080 Ti) |
FastInst [106] | 2023 | 20 | 53 | 39.9 | 28 FPS (V100) |
YOLOv5n-seg [80] | 2020 | 20+ | 2 | 23.4 | 833 (A100) |
YOLOv5x-seg | 2020 | 20+ | 88.8 | 41.4 | 222 (A100) |
YOLOv8n-seg | 2022 | 20+ | 3.4 | 36.7 | 826 (A100) |
YOLOv8x-seg | 2022 | 20+ | 71.8 | 43.4 | 248 (A100) |
RTMDet-Ins-s [107] | 2022 | 6 | 5.6 | 38.7 | 518 (RTX 3090) |
RTMDet-Ins-x | 2022 | 6 | 102.7 | 44.6 | 188 (RTX 3090) |
Reference | Year | Practical Problem | CV Task | Approach | Specifics | Primary CV Model |
[124] | 2023 | Conveyor | Semantic | DL | SLIC + U-Net | U-Net |
[41] | 2022 | Conveyor | Object | DL | SSD, YOLOv3, YOLOv4, YOLOv5, DCN-YOLOv3 | YOLO |
[79] | 2022 | Conveyor | Object | DL | Yolo3, SPPNet, Yolo1, 2 | YOLO |
[1] | 2022 | Conveyor | Classification | DL | AlexNet, VGG16, ResNet50, InceptionV3, MobileNet, SENet | MobileNet |
[125] | 2021 | Conveyor | Semantic | DL | DexiNed Edge Detection + Morphologic + Watershed | Other |
[18] | 2021 | Conveyor | Semantic | DL | U-Net | U-Net |
[14] | 2020 | Conveyor | Semantic | DL | U-Net | U-Net |
[15] | 2020 | Conveyor | Semantic | DL | U-Net, Res-U-Net + Watershed | U-Net |
[59] | 2020 | Conveyor | Semantic | DL | U-Net, RDUNET, DUNET | U-Net |
[126] | 2020 | Conveyor | Semantic | DL | U-Net + Watershed | U-Net |
[127] | 2020 | Conveyor | Semantic | DL | CNN + Watershed | Watershed |
[128] | 2019 | Conveyor | Semantic | Classical | Otsu + Histogram accumulation moment | Other |
[129] | 2019 | Conveyor | Other | Classical | Super Voxel 3D | Other |
[20] | 2023 | Open Pit | Instance | DL | Mask R-CNN | Mask R-CNN |
[47] | 2022 | Open Pit | Instance | Classical | Modified Watershed | Watershed |
[70] | 2022 | Open Pit | Instance | DL | ResNeXt101 + SE | Mask R-CNN |
[68] | 2022 | Open Pit | Instance | DL | ResNet-50 | Mask R-CNN |
[42] | 2022 | Open Pit | Object | DL | YOLO | YOLO |
[58] | 2021 | Open Pit | Semantic | DL | Dual U-Net, boundary and regions | U-Net |
[46] | 2021 | Open Pit | Semantic | Classical | Wavelet + Superpixel segmentation | Other |
[12] | 2021 | Open Pit | Instance | DL | Mask R-CNN | Mask R-CNN |
[130] | 2021 | Open Pit | Instance | Classical | Super-Voxel Segmentation | Other |
[67] | 2021 | Open Pit | Instance | DL | Mask R-CNN | Mask R-CNN |
[131] | 2021 | Open Pit | Instance | Classical | Watershed | Watershed |
[40] | 2021 | Open Pit | Instance | DL | Mask R-CNN | Mask R-CNN |
[7] | 2021 | Open Pit | Classification | Classical | ResNeXt-50 | ResNet |
[132] | 2021 | Open Pit | Classification | Classical | SM, GMB, SVM + Firefly evolutionary algorithm | Other |
[133] | 2021 | Open Pit | Classification | Classical | ANN, Monte Carlo dropout | Other |
[134] | 2021 | Open Pit | Other | Classical | Power Sieve3 software | Other |
[82] | 2020 | Open Pit | Classification | DL | VGG-16 | VGG |
[21] | 2019 | Open Pit | Instance | Classical | Split-Desktop Software | Other |
[6] | 2019 | Open Pit | Other | Classical | Custom | Other |
[135] | 2018 | Open Pit | Classification | Classical | SVM | Other |
[136] | 2013 | Open Pit | Classification | Classical | ANN | Other |
[137] | 2009 | Open Pit | Instance | Classical | Watershed | Watershed |
[22] | 2005 | Open Pit | Instance | Classical | Watershed | Watershed |
[138] | 2003 | Open Pit | Other | Classical | WipFrag software | Other |
[56] | 2021 | Tunnel | Semantic | DL | FCN8s, GCN, DFN, DeepLabV3, PSPNet | DFN |
[57] | 2021 | Tunnel | Semantic | DL | Dual U-Net, boundary and regions | U-Net |
[55] | 2021 | Tunnel | Instance | DL | SSD + U-Net + post-processing | U-Net |
[81] | 2021 | Tunnel | Classification | DL | AlexNet | Other |
[83] | 2021 | Tunnel | Classification | DL | ResNet + GoogLeNet | ResNet |
[139] | 2013 | Tunnel | Instance | Classical | Watershed | Watershed |
[140] | 2011 | Tunnel | Instance | Classical | Watershed | Watershed |
[141] | 2023 | Other | Semantic | DL | MSBA-Unet + CDD post-processing | U-Net |
[43] | 2023 | Other | Object | DL | YOLO | YOLO |
[78] | 2023 | Other | Object | DL | Watershed, FASTER-R-CNN, YOLO3 | YOLO |
[142] | 2022 | Other | Semantic | DL | Swin-Unet | U-Net |
[143] | 2022 | Other | Semantic | DL | DeepLab3+ | DeepLab |
[77] | 2022 | Other | Object | DL | DCN-YOLOv3 | YOLO |
[144] | 2022 | Other | Classification | Classical | ANN + SVR | Other |
[145] | 2021 | Other | Semantic | DL | GAN + U-Net | U-Net |
[60] | 2021 | Other | Semantic | DL | FraSegNet: VGG19 + ASPP | Other |
[23] | 2021 | Other | Instance | Other | ResNet50 | Mask R-CNN |
[69] | 2021 | Other | Instance | DL | Mask R-CNN | Mask R-CNN |
[146] | 2021 | Other | Classification | DL | AlexNet, VGG | VGG |
[2] | 2021 | Other | Other | Classical | Custom | Other |
[147] | 2020 | Other | Semantic | DL | Lightweight U-Net + Morphologic + Watershed | U-Net |
[71] | 2020 | Other | Instance | DL | Mask R-CNN + FCN | Mask R-CNN |
[3] | 2020 | Other | Object | DL | Faster R-CNN | Faster R-CNN |
[148] | 2020 | Other | Classification | Classical | Edge Detection | Other |
[16] | 2020 | Other | Other | DL | Custom CNN | Other |
[17] | 2020 | Other | Other | Classical | Custom | Other |
[4] | 2019 | Other | Semantic | DL | SegNet | Other |
[5] | 2019 | Other | Instance | Classical | SLIC Segmentation + Features + Classification | Other |
[149] | 2019 | Other | Instance | Classical | Wavelet + manual features + ANN | Other |
[150] | 2019 | Other | Instance | Classical | Morphological Reconstruction | Other |
[151] | 2019 | Other | Instance | Classical | Otsu + Watershed | Watershed |
[152] | 2018 | Other | Semantic | DL | Holistically-nested convolutional network | Other |
[153] | 2018 | Other | Instance | Classical | Morphologic + Watershed | Watershed |
[154] | 2018 | Other | Instance | Classical | Thresholding + Watershed | Watershed |
[26] | 2018 | Other | Other | Classical | WipFrag software | Other |
[155] | 2016 | Other | Classification | Classical | Morphologic + Watershed + SVM for classification | Watershed |
[156] | 2016 | Other | Other | Classical | WipFrag software | Other |
[157] | 2015 | Other | Classification | Classical | ANN + post-processing | Other |
[158] | 2013 | Other | Classification | Classical | ANN + Regression | Other |
[159] | 2013 | Other | Other | Classical | GoldSize software | Other |
[160] | 2012 | Other | Classification | Classical | SVM | Other |
[161] | 2011 | Other | Instance | Classical | Watershed | Watershed |
[163] | 2010 | Other | Instance | Classical | Watershed | Watershed |
[164] | 2009 | Other | Semantic | Classical | Thresholding + morphologic | Other |
[165] | 2009 | Other | Classification | Classical | Fuzzy Logic | Other |
[166] | 2008 | Other | Semantic | Classical | Edge Detection | Other |
[167] | 2005 | Other | Other | Classical | Laser 3d + Watershed | Other |
[168] | 1999 | Other | Classification | Classical | Morphologic + ANN for classification | Other |
[169] | 1996 | Other | Other | Classical | Image Preparation | Other |