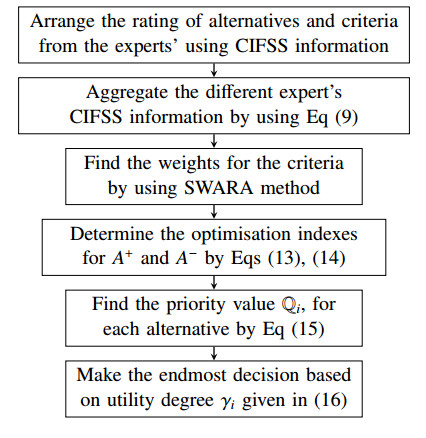
In this manuscript, we propose an integrated framework based on COmplex PRoportional ASsessment and Step-wise Weight Assessment Ratio Analysis approach within the complex intuitionistic fuzzy soft (CIFS) context. This context is an ideal technique with complex fuzzy foundation that means to denote multi-dimensional data in a concise. In this framework, criteria weights are evaluated by the SWARA technique, and the ranking of alternatives is determined by the COPRAS method using CIFSs. Further, to illustrate the applicability of the presented technique, an empirical case study of ERP software selection problem is taken. A comparative study and sensitivity analysis is presented to verify the strength of the presented methodology.
Citation: Harish Garg, J. Vimala, S. Rajareega, D. Preethi, Luis Perez-Dominguez. Complex intuitionistic fuzzy soft SWARA - COPRAS approach: An application of ERP software selection[J]. AIMS Mathematics, 2022, 7(4): 5895-5909. doi: 10.3934/math.2022327
[1] | Adel Fahad Alrasheedi, Jungeun Kim, Rukhsana Kausar . q-Rung orthopair fuzzy information aggregation and their application towards material selection. AIMS Mathematics, 2023, 8(8): 18780-18808. doi: 10.3934/math.2023956 |
[2] | Admi Nazra, Jenizon, Yudiantri Asdi, Zulvera . Generalized hesitant intuitionistic fuzzy N-soft sets-first result. AIMS Mathematics, 2022, 7(7): 12650-12670. doi: 10.3934/math.2022700 |
[3] | Sumbal Ali, Asad Ali, Ahmad Bin Azim, Abdul Samad Khan, Fuad A. Awwad, Emad A. A. Ismail . TOPSIS method based on q-rung orthopair picture fuzzy soft environment and its application in the context of green supply chain management. AIMS Mathematics, 2024, 9(6): 15149-15171. doi: 10.3934/math.2024735 |
[4] | Tareq M. Al-shami, José Carlos R. Alcantud, Abdelwaheb Mhemdi . New generalization of fuzzy soft sets: $ (a, b) $-Fuzzy soft sets. AIMS Mathematics, 2023, 8(2): 2995-3025. doi: 10.3934/math.2023155 |
[5] | Atiqe Ur Rahman, Muhammad Saeed, Hamiden Abd El-Wahed Khalifa, Walaa Abdullah Afifi . Decision making algorithmic techniques based on aggregation operations and similarity measures of possibility intuitionistic fuzzy hypersoft sets. AIMS Mathematics, 2022, 7(3): 3866-3895. doi: 10.3934/math.2022214 |
[6] | Shichao Li, Zeeshan Ali, Peide Liu . Prioritized Hamy mean operators based on Dombi t-norm and t-conorm for the complex interval-valued Atanassov-Intuitionistic fuzzy sets and their applications in strategic decision-making problems. AIMS Mathematics, 2025, 10(3): 6589-6635. doi: 10.3934/math.2025302 |
[7] | Rana Muhammad Zulqarnain, Xiao Long Xin, Muhammad Saeed . Extension of TOPSIS method under intuitionistic fuzzy hypersoft environment based on correlation coefficient and aggregation operators to solve decision making problem. AIMS Mathematics, 2021, 6(3): 2732-2755. doi: 10.3934/math.2021167 |
[8] | Doaa Al-Sharoa . (α1, 2, β1, 2)-complex intuitionistic fuzzy subgroups and its algebraic structure. AIMS Mathematics, 2023, 8(4): 8082-8116. doi: 10.3934/math.2023409 |
[9] | Maliha Rashid, Akbar Azam, Maria Moqaddas, Naeem Saleem, Maggie Aphane . Ciric fixed point theorems of $ \mathtt{£} $-fuzzy soft set-valued maps with applications in fractional integral inclusions. AIMS Mathematics, 2025, 10(3): 4641-4661. doi: 10.3934/math.2025215 |
[10] | Sumaira Yasmin, Muhammad Qiyas, Lazim Abdullah, Muhammad Naeem . Linguistics complex intuitionistic fuzzy aggregation operators and their applications to plastic waste management approach selection. AIMS Mathematics, 2024, 9(11): 30122-30152. doi: 10.3934/math.20241455 |
In this manuscript, we propose an integrated framework based on COmplex PRoportional ASsessment and Step-wise Weight Assessment Ratio Analysis approach within the complex intuitionistic fuzzy soft (CIFS) context. This context is an ideal technique with complex fuzzy foundation that means to denote multi-dimensional data in a concise. In this framework, criteria weights are evaluated by the SWARA technique, and the ranking of alternatives is determined by the COPRAS method using CIFSs. Further, to illustrate the applicability of the presented technique, an empirical case study of ERP software selection problem is taken. A comparative study and sensitivity analysis is presented to verify the strength of the presented methodology.
Uncertainties and vagueness are characteristics that are prevalent in problems which occurring in science, engineering, medical science, economics, decision making, etc. To exceed these, some of the theories were designated like fuzzy set (FS) [38], intuitionistic fuzzy set (IFS) [2], soft set (SS) [23] and intuitionistic fuzzy soft set (IFSS) [18]. MCDM problems often require the decision makers to give evaluation information about the alternative and the criteria with a FS, soft set, IFS and other extended sets [12,15,20,36,37]. Under these existing sets, there is a difficulty to handle the seasonality or periodicity that to be in many real life problems. The complex numbers can be used to model the periodicity of the elements by its phase term and also complex numbers can represent the two dimensional information. The complex valued models have been widely used in various fields of applications [3,34,35].
In this regard, Ramot et al. [28] introduced a novel concept known as complex fuzzy sets (CFSs), which included the amplitude and phase terms. By adding the degree of non-belongingness to CFSs, Alkouri et al. [1] proposed the idea of complex intuitionistic fuzzy sets (CIFSs). Further, Kumar et al. [13] defined the CIFSSs (complex intuitionistic fuzzy soft sets) concept. In recent times, CIFS is a powerful context means to represent the two dimensional data arisen in natural complicated problems [6,29]. Consequently, numerous methodologies and theories have been established under the CFSs extensions [9,16,17,19,24,25,26,27,32]. In this work, a novel MCDM technique is proposed to select the ideal choice in which the data is represented in the form of CIFSS environment.
Zavadskas et al. [39] defined a novel approach known as COPRAS (COmplex PRoportional ASsessment), which is a realistic decision making framework. The advantages of the COPRAS framework are: (ⅰ) it contains the ratios of benefit and cost solutions simultaneously; (ⅱ) it is a valuable and flexible method to solve the problems. Several works have extended the COPRAS method in various uncertain contexts. Recently, Garg [7] proposed the COPRAS method in possibility intuitionistic fuzzy soft sets environment for the decision problem. Also, Mishra et al. [14,22] introduced the COPRAS Method in various fuzzy extensions to solve the decision making. The weights of the criteria are key factors in the decision process. There are two kinds of criteria weights: (ⅰ) subjective; (ⅱ) objective. The objective weights are computed from the data given in decision matrices and the subjective weights are evaluated based on the data given by the experts. To evaluate the subjective criteria weights, Kersuliene et al. [11] introduced a novel approach called SWARA, which has a least computational complexity. The SWARA and fuzzy COPRAS approach was integrated by Ighravwe and Oke [4] to select the maintenance technician. Further, the combined SWARA and COPRAS approach is developed in various domain such as hesitant fuzzy sets [30] and intuitionistic fuzzy sets (IFSs) [21]. These approaches had their own significance in the evaluation of weight and preference ranking of an alternative, respectively.
The existing studies such as IFS, IFSS etc., have been widely applicable in many fields, although it has been restricted in nature. In such theories, the information collected related to the object is deal with only one dimension information at a time, which may result to loss of some information at a particular time. In everyday life, we come across complex phenomena where it becomes necessary to add the other dimension to the membership and non-membership grades. By defining this other dimension, the complete information can be projected in single set, and hence, information loss can be ignored. To illustrate the importance of the phase term, consider an automotive manufacturing company in India, where they decide to select the ERP software for improving their business activities. The company consults an expert team regarding (ⅰ) overall rating about an alternative; (ⅱ) corresponding latest version of ERP software. This is a two-dimensional problem and this is unable to done accurately using IFS and IFSS [2,18] structure. To the best of our knowledge, this work develops a new framework called Complex Intuitionistic Fuzzy Soft-SWARA-COPRAS (CIFS-SWARA-COPRAS) with CIFSS domain for solving the two dimensional problem. In CIFSS atmosphere, the amplitude term represents the expert's decision with respect to the alternative and the phase term represents the expert's decision with respect to the current version of ERP software.
An ERP system is the brain of the company's technology system. Because ERP combines all features of a business involving development of product, manufacturing, retail and sales. ERP software selection is one of the most important decision making issues covering both qualitative and quantitative criterion for organizations. The authors of [10], in their conjoint work of 1008 calculation completed in 126 organizations state that reliability, functionality, ease of use and ease of customization are considered as the most important criteria. Based on these criteria, an automotive manufacturing company in India, where they decides to select the ERP software by using CIFS-SWARA-COPRAS technique.
The main contributions of this paper include:
1) A new framework named as CIFS-SWARA-COPRAS is introduced in the work. In this framework, criteria weights are evaluated by the SWARA technique, and the ranking of alternatives is determined by the COPRAS method using CIFSs information.
2) In the proposed technique, the weights are calculated by using the SWARA method which include the exactness of experts' opinion and the ranking of an alternative is determined by using the COPRAS approach.
3) To illustrate the applicability of the presented framework, an empirical case study of ERP software selection problem is examined under CIFSSs environment. The problem is two dimensional, that is to find the ideal ERP software with its current version.
4) A sensitivity analysis and comparative study are presented to show the validity and stability of the defined methodology.
The rest of this paper is organized as follows: Section 2 describes the notion of complex fuzzy sets and complex intuitionistic fuzzy soft sets. Section 3 proposes the CIFS-SWARA-COPRAS method under the CIFSS-environment. The applicability of the stated method is demonstrated through a case study of ERP software selection in Section 4. Also, a comparative study and sensitivity analysis is conducted to strengthen the results over the existing studies results. At last, Section 5 concludes the work.
In this section, we review the notions of CFS, CIFS and CIFSS.
Definition 2.1. [28] A complex fuzzy set (CFS) C over U is distinguished by a membership function μC(u) that belongs to the unit circle in the complex plane and is denoted by μC(u)=rC(u)eisC(u), where rC(u) and sC(u) are both real-valued, rC(u)∈[0,1] and i=√−1. A CFS C is represented by,
C={(u,μC(u)):u∈U}={(u,rC(u)eisC(u)):u∈U} | (1) |
Definition 2.2. [13] Let CIFS(U) denotes the set of all CIFs over U, E be a set of criteria. Then
(˜F,E)={(e,˜F(e)):e∈E,˜F(e)∈CIFS(U)}, | (2) |
is a complex intuitionistic fuzzy soft set (CIFSS) over U, where ˜F:E⟶CIFS(U).
For all ϵ∈E,
˜F(ϵ)={(u,μ˜F(ϵ)(u),ν˜F(ϵ)(u)):u∈U}={(u,r˜F(ϵ)(u)eiwμ˜F(ϵ)(u),k˜F(ϵ)(u)eiwν˜F(ϵ)(u)):u∈U} | (3) |
where μ˜F(ϵ):U⟶{a|a∈C,|a|≤1} and ν˜F(ϵ):U⟶{a′|a′∈C,|a′|≤1}, |μ˜F(ϵ)(u)+ν˜F(ϵ)(u)|≤1.
For convince, we denote δ=(reis,τeiψ) and called as CIFN ("complex intuitionistic fuzzy number") with the condition that r,τ,s,ψ∈[0,1] and ∣reis+τeiψ∣≤1.
Definition 2.3. [6] Let δ=(reis,τeiψ) be a CIFN. Then the score function of δ is defined as
S(δ)=(r−τ)+12π(s−ψ). | (4) |
and the accuracy function is
H(δ)=(r+τ)+12π(s+ψ). | (5) |
It is clearly seen that S(δ)∈[−2,2] and H(δ)∈[0,2].
Definition 2.4. Let δ be a CIFN. Then the normalized score function of δ is defined as
S∗(δ)=14(S(δ)+2), | (6) |
where S(δ) is the score function of δ and S∗(δ)∈[0,1].
Definition 2.5. [6] Let δj=(rjeisj,ηjeiψj)(j=1,2,...,n) be CIFNs. The aggregated value of these CIFNs is obtained by using the complex intuitionistic fuzzy weighted averaging operator (CIFWA) and given as
CIFWA(δ1,δ2,...,δn)=n⨁j=1wjδj=((1−n∏j=1(1−rj)wj)ei2π(1−n∏j=1(1−sj)wj),(n∏j=1τwjj)ei2π(n∏j=1ψwjj)) | (7) |
where w=(w1,w2,...,wn)T is a weight vector of δj with wj>0 and n∑j=1wj=1.
In this section, we propose the concept of CIFS-SWARA-COPRAS method and the working framework of it in the following steps:
Step 1: Originate the data about the alternatives according to the criteria.
Let A={a1,a2,...,am} be a collection of decision criteria and U={x1,x2,...,xn} denotes the set of alternatives and let DE={E1,E2,...,El} be a set of decision experts for the decision process and W={w1,w2,...,wl} represents the weights for the decision experts. Suppose X be the initial CIFSs decision matrix
X=(x(k)11x(k)12⋯x(k)1mx(k)21x(k)22⋯x(k)2m⋮⋮⋱⋮x(k)n1x(k)n2⋯x(k)nm) | (8) |
where x(k)ij=(r(k)ijeis(k)ij,η(k)ijeiψ(k)ij) denotes the initial value for xi as to the criteria aj given by the kth expert in terms of CIFS set, for j=1,2,...,m, i=1,2,...,n and k=1,2,...,l.
Step 2: Generate the aggregated CIFSS decision matrix.
Compute the aggregated value of the expert x(k)ij=(r(k)ijeis(k)ij,η(k)ijeiψ(k)ij) for k=1,2,...,l into CIFSS decision matrix ˆX=(ˆxij)n×m by utilizing the Definition 2.5. The obtained values of ˆxij=(rijeisij,ηijeiψij) are as follows
ˆxij=CIFWA(x(1)jk,x(2)jk,...,x(l)jk)=((1−l∏k=1(1−rjk)wk)ei2π(1−l∏k=1(1−sjk)wk),(l∏k=1τwkjk)ei2π(l∏k=1ψwkjk)),i=1,2,...,n | (9) |
Step 3: Evaluate the weights for the criteria by using SWARA method.
The weights of the criteria are computed by using SWARA method, whose steps are summarized as follows.
ⅰ) Evaluate the crisp values wj for the criteria according to the experts' preference and find the normalized score values of wj (i.e., S∗(wj)), by using the Definition 2.4.
ⅱ) Order the criteria from the most to the least score value.
ⅲ) Compute the comparative value kj for the ordered score value, which starts from the second place by differencing the jth value and (j−1)th value.
ⅳ) Calculate the comparative coefficient pj by
pj={1ifj=1kj+1ifj>1. | (10) |
ⅴ) Evaluate the recalculated weights qj by
qj={1ifj=1q(j−1)pjifj>1. | (11) |
ⅵ) Estimate the criteria weights wj by using the following equation
wj=qjm∑j=1qj. | (12) |
Step 4: Determine the optimisation indexes α+i and α−i for A+ and A−, respectively.
Let us assume A+={a1,a2,...,ar} where r<m be the set of positive (beneficial) criteria. Then we calculate the optimisation index (α+i) for each alternative xi, as follows
α+i=r⨁j=1wjˆxij,i=1,...,n | (13) |
Let A−={ar+1,ar+2,...,am} be the non-beneficial (negative) criteria for the decision problem. Then we determine the optimization index (α−i) for each alternative xi, as
α−i=m⨁j=r+1wjˆxij,i=1,2,...,n | (14) |
Step 5: Calculate the priority value (Qi), for each xi.
By using COPRAS technique, we compute the priority value Qi, for every xi as:
Qi=S∗(α+i)+n∑i=1S∗(α−i)S∗(α−i)n∑i=1(1S∗(α−i)),i=1,...,nprovidedS∗(α−i)≠0 | (15) |
Step 6: Make the endmost decision based on utility degree γi.
Calculate the utility degree γi, for every xi by
γi=QiQmax×100%, | (16) |
where Qmax≠0 is the largest value of Qi. Then, rank the utility degree in which the highest utility degree is ranked as first, while least utility degree is ranked as last. Finally, make the conclusion based on the utility degree γi.
The implementation framework of the CIFS-SWARA-COPRAS method is shown in Figure 1.
To demonstrate the working of the proposed CIFS-SWARA-COPRAS method, we provide a numerical example related to ERP software selection, which can be read as follows.
The proposed CIFS-SWARA-COPRAS algorithm is applied to a Enterprise Resource Planning (ERP) software selection process for the automotive manufacturing company in India. In the manufacturing company, the operation managers need to buy an ERP software system for increasing the day-to-day business activities such as production management, accounting, manufacturing execution, procurement, supply chain operations and compliance. Initially, a decision expert team of 3 DE={E1,E2,E3} consisting of a senior representative and a functional expert and a senior manager was constituted. Then, the expert team decides seven criteria (Functionality, Reliability, Vendor Viability, Cost, Support and Training, Ease of Use and Difficulty in Customization and Implementation) based on their experiences and studies in the literature. Accordingly, the criteria and its direction for the ERP selection process is given in Table 1. In addition, the selection of ERP system is not only based on overall average rating about an alternative and it is also based on the recent reviews about an alternative. Because, the average value represents the collected reviews over time do not always give the current customer review - recent reviews about the latest version are more likely to give the current sentiment. Therefore the selection should be based on both overall rating and latest version of an alternative. This can be presented by the amplitude and phase expression of CIFSS. The given each expert needs to select the appropriate ERP software system among the four alternatives x1, x2, x3, and x4. For this, the steps of the proposed method are implemented to find the desired alternative(s) as follows:
Criteria | Description | Direction |
a1 | Functionality | + |
a2 | Reliability | + |
a3 | Vendor Viability | + |
a4 | Cost | - |
a5 | Support and Training | + |
a6 | Ease of Use | + |
a7 | Difficulty in Customization and Implementation | - |
Step 1: The ratings of each expert towards the evaluation of alternatives under the different criteria are provided in terms of linguistic expressions as given in Table 2. In this table, each pair of linguistic intimation represents the amplitude and phase term. The amplitude term means to give an expert's decision with respect to alternative and the phase term can be used to denote an expert's decision with respect to the current version.
Alternatives | |||||
Criteria | Experts | x1 | x2 | x3 | x4 |
E1 | L, SL | H, SH | M, M | H, SH | |
a1 | E2 | H, SH | M, M | H, SH | VH, EH |
E3 | H, SH | L, SL | L, SL | M, M | |
E1 | H, SH | M, M | H, SH | L, SL | |
a2 | E2 | VH, EH | L, SL | M, M | VL, EL |
E3 | H, SH | VH, EH | H, SH | L, SL | |
E1 | M, M | H, SH | VH, EH | M, M | |
a3 | E2 | H, SH | VH, EH | H, SH | VH, EH |
E3 | VH, EH | L, SL | VL, EL | H, SH | |
E1 | H, SH | M, M | L, SL | M, M | |
a4 | E2 | H, SH | L, SL | L, SL | H, SH |
E3 | L, SL | VL, EL | H, SH | L, SL | |
E1 | VL, EL | L, SL | H, SH | VH, EH | |
a5 | E2 | H, SH | VL, EL | M, M | H, SH |
E3 | M, M | VH, EH | L, SL | VH, EH | |
E1 | VH, EH | L, SL | H, SH | M, M | |
a6 | E2 | M, M | M, M | VH, EH | H, SH |
E3 | H, SH | VH, EH | M, M | VL, EL | |
E1 | VL, EL | M, M | H, SH | L, SL | |
a7 | E2 | L, SL | VL, EL | M, M | H, SH |
E3 | M, M | L, SL | H, SH | M, M |
For instance, suppose the expert E1 thinks that the over all rating about x1 is "Low" and the rating about the current version of x1 is "Slightly Low" for the first attribute. So the value of x1 for the first attribute can be represented as "L, SL". However, the respective ratings of each linguistic term in the form of CIFSS features are summarized in Table 3.
r | s | τ | ψ | |
EH- Extremely high | 0.95 | (0.9)2π | 0.02 | (0.1)2π |
VH- Very High | 0.90 | (0.8)2π | 0.05 | (0.15)2π |
H- High | 0.80 | (0.75)2π | 0.10 | (0.20)2π |
SH- Slightly High | 0.70 | (0.7)2π | 0.25 | (0.1)2π |
M- Medium | 0.65 | (0.5)2π | 0.30 | (0.4)2π |
SL- Slightly Low | 0.50 | (0.3)2π | 0.45 | (0.6)2π |
L- Low | 0.40 | (0.25)2π | 0.50 | (0.6)2π |
VL- Very Low | 0.25 | (0.2)2π | 0.60 | (0.65)2π |
EL- Extremely Low | 0.15 | (0.1)2π | 0.65 | (0.7)2π |
Step 2: By taking the experts' E1,E2,E3 weight as 0.253, 0.621, 0.126, which is provided by the company senior members, we aggregate the different expert preferences into the collective one using Eq. (9). The results corresponding to them are summarized in Table 4.
x1 | x2 | x3 | x4 | |
a1 | (0.736ei2π(0.628),0.150ei2π(0.157)) | (0.675ei2π(0.542),0.243ei2π(0.296)) | (0.735ei2π(0.620),0.162ei2π(0.178)) | (0.861ei2π(0.838),0.075ei2π(0.119)) |
a2 | (0.870ei2π(0.848),0.065ei2π(0.100)) | (0.582ei2π(0.497),0.329ei2π(0.432)) | (0.717ei2π(0.588),0.198ei2π(0.237)) | (0.291ei2π(0.155),0.573ei2π(0.673)) |
a3 | (0.789ei2π(0.703),0.121ei2π(0.142)) | (0.851ei2π(0.831),0.080ei2π(0.125)) | (0.802ei2π(0.739),0.105ei2π(0.128)) | (0.850ei2π(0.827),0.086ei2π(0.142)) |
a4 | (0.770ei2π(0.666),0.123ei2π(0.125)) | (0.462ei2π(0.336),0.450ei2π(0.552)) | (0.478ei2π(0.371),0.408ei2π(0.479)) | (0.735ei2π(0.620),0.162ei2π(0.178)) |
a5 | (0.700ei2π(0.578),0.181ei2π(0.195)) | (0.450ei2π(0.360),0.419ei2π(0.527)) | (0.675ei2π(0.542),0.242ei2π(0.296)) | (0.846ei2π(0.802),0.077ei2π(0.100)) |
a6 | (0.762ei2π(0.688),0.166ei2π(0.237)) | (0.657ei2π(0.556),0.272ei2π(0.372)) | (0.861ei2π(0.838),0.075ei2π(0.119)) | (0.728ei2π(0.608),0.166ei2π(0.182)) |
a7 | (0.407ei2π(0.285),0.491ei2π(0.593)) | (0.399ei2π(0.280),0.492ei2π(0.596)) | (0.717ei2π(0.588),0.198ei2π(0.237)) | (0.688ei2π(0.573),0.188ei2π(0.201)) |
Step 3: Considering the different experts' preferences weight as given in Table 5 with respect to each criteria, we aggregate their preferences and their result is summarized in the last column of the Table 5.
E1 | E2 | E3 | Aggregated Value | |
a1 | (0.65ei2π(0.5),0.30ei2π(0.4)) | (0.80ei2π(0.7),0.10ei2π(0.1)) | (0.40ei2π(0.3),0.50ei2π(0.6)) | (0.735ei2π(0.620),0.162ei2π(0.178)) |
a2 | (0.80ei2π(0.7),0.1ei2π(0.1)) | (0.90ei2π(0.9),0.05ei2π(0.1)) | (0.90ei2π(0.9),0.05ei2π(0.1)) | (0.881ei2π(0.868),0.060ei2π(0.100)) |
a3 | (0.40ei2π(0.3),0.50ei2π(0.6)) | (0.65ei2π(0.5),0.30ei2π(0.4)) | (0.80ei2π(0.7),0.10ei2π(0.1)) | (0.626ei2π(0.490),0.297ei2π(0.372)) |
a4 | (0.25ei2π(0.1),0.60ei2π(0.7)) | (0.40ei2π(0.3),0.50ei2π(0.6)) | (0.25ei2π(0.3),0.50ei2π(0.6)) | (0.347ei2π(0.254),0.524ei2π(0.624)) |
a5 | (0.65ei2π(0.5),0.30ei2π(0.4)) | (0.80ei2π(0.7),0.10ei2π(0.1)) | (0.80ei2π(0.7),0.10ei2π(0.1)) | (0.770ei2π(0.659),0.132ei2π(0.142)) |
a6 | (0.80ei2π(0.7),0.10ei2π(0.1)) | (0.80ei2π(0.7),0.10ei2π(0.1)) | (0.90ei2π(0.9),0.05ei2π(0.1)) | (0.817ei2π(0.739),0.092ei2π(0.100)) |
a7 | (0.40ei2π(0.3),0.50ei2π(0.6)) | (0.25ei2π(0.3),0.50ei2π(0.6)) | (0.25ei2π(0.3),0.50ei2π(0.6)) | (0.291ei2π(0.155),0.573ei2π(0.673)) |
By using the steps of the SWARA techniques, as mentioned in Step 3 of the proposed method, the values of kj, pj and qj are summarized in Table 6. In this Table, the most significant criteria is ranked as first and the least significant criteria is ranked as last.
Criteria | Ordered crisp value kj | Comparative coefficient pj | Comparative weights qj | Recalculated wj | Criteria weights |
a2 | 0.897 | - | 1 | 1 | 0.177 |
a6 | 0.841 | 0.056 | 1.056 | 0.947 | 0.167 |
a5 | 0.789 | 0.052 | 1.052 | 0.900 | 0.159 |
a1 | 0.754 | 0.035 | 1.035 | 0.870 | 0.154 |
a3 | 0.612 | 0.142 | 1.142 | 0.762 | 0.135 |
a4 | 0.363 | 0.249 | 1.249 | 0.610 | 0.108 |
a7 | 0.300 | 0.063 | 1.063 | 0.574 | 0.101 |
Hence, the criteria weights are computed as
wj={0.154,0.177,0.135,0.108,0.159,0.167,0.101}. |
Step 4: Use Eqs (13), (14), we compute the values of α−i, α+i for each alternative xi,i=1,2,3,4 as
α+1=(0.701ei2π(0.625),0.196ei2π(0.233));α−1=(0.200ei2π(0.141),0.742ei2π(0.758))α+2=(0.576ei2π(0.498),0.329ei2π(0.413));α−2=(0.112ei2π(0.075),0.854ei2π(0.890))α+3=(0.685ei2π(0.601),0.217ei2π(0.260));α−3=(0.179ei2π(0.130),0.771ei2π(0.799))α+4=(0.679ei2π(0.617),0.251ei2π(0.296));α−4=(0.230ei2π(0.173),0.694ei2π(0.706)) |
Step 5: Using the values of α+i,α−i, we compute the priority values Qi's for each xi with Eq (15) and get
Q1=0.8796;Q2=0.8775;Q3=0.8787;Q4=0.8172 |
Step 6: The utility degree γi's for each alternative is computed by using Eq (16) and get
γ1=100%;γ2=99.76%;γ3=99.89%;γ4=92.91% |
From these values, the order preference of the given ERP software system is found as x1≻x3≻x2≻x4 and, thus x1 is the best alternative.
In this section, the advantages of the proposed CIFS-SWARA-COPRAS method are underlined, which are listed as follows.
The criteria weights which are determined by SWARA approach include the exactness of experts' opinion in ERP software selection process. Also, COPRAS procedure contains the ratios of the benefit and the cost solutions, simultaneously. The automotive manufacturing company needs to select the suitable alternative of ERP system with its current version simultaneously. This two-dimensional problem can be solved by CIFSS atmosphere. In CIFSS, the amplitude term denoted an experts' decision regarding option of ERP system and the phase term denoted an experts' decision regarding current version of ERP system. This cannot be done by using traditional IFSs. To verify the strength of the proposed technique a comparative study is obtained in Table 7. From Table 7, we determined that x3 is the best alternative by using [20,21]. This result is based on overall rating about an alternative. Suppose the rating about current version of an ERP software is taken into account, the result may be affected. This findings certify that the proposed technique is more powerful than previously developed techniques [6,20,21].
x1 | x2 | x3 | x4 | Rank Order | |
IF-WASPAS [20] | |||||
(ⅰ) Weight Sum Model (WSM) | 0.9155 | 0.8175 | 0.9300 | 0.9025 | x3≻x1≻x4≻x2 |
(ⅱ) Weight Product Model (WPM) | 0.6965 | 0.5305 | 0.7270 | 0.6250 | x3≻x1≻x4≻x2 |
(ⅲ) Weighted Aggregated Sum Product | |||||
Assessment (WASPAS) with ϑ=0.5 | 0.8065 | 0.6745 | 0.8285 | 0.7640 | x3≻x1≻x4≻x2 |
IF-SWARA-COPRAS [21] | 0.8985 | 0.8660 | 0.9270 | 0.8580 | x3≻x1≻x2≻x4 |
CIFWA operator with criteria weights wj [6] | 0.7595 | 0.6275 | 0.7545 | 0.6145 | x1≻x3≻x2≻x4 |
Proposed CIFS - SWARA - COPRAS | 0.8796 | 0.8775 | 0.8787 | 0.8172 | x1≻x3≻x2≻x4 |
In this section, we investigate a sensitivity according to the strategy value of the decision expert (DE) in [0, 1] by using Eq (17), which can be demonstrated by Eq (15).
Qi=ϑS∗(α+i)+(1−ϑ)n∑i=1S∗(α−i)S∗(α−i)n∑i=1(1S∗(α−i)), | (17) |
where ϑ∈[0,1]. If ϑ<0.5, the DE indicates pessimistic type, (i.e., ) the least value is related as the weight to the benefit criteria. Consequently, if ϑ>0.5, the DE gives optimistic type and so, the least value is related as the weight to the cost criteria. Also, if ϑ=0.5, the DE indicates neutral and the same preference is associated with both benefit and cost criteria. From this, the various values of ϑ can able to evaluate the sensitivity of the proposed technique. The ranking values as to the parameters are given in Table 8 and Figure 2. From this Table, we can see that an alternative x1 has the highest value, when ϑ= 0.5 to 1 whereas x2 has the highest value when ϑ = 0 to 0.4. On the other side, x4 has the lowest value when ϑ = 0 to 0.6 and x2 has the lowest value when ϑ = 0.7 to 1. It is cleared that the proposed technique has good stability.
ϑ | 0 | 0.1 | 0.2 | 0.3 | 0.4 | 0.5 | 0.6 | 0.7 | 0.8 | 0.9 | 1 |
x1 | 0.1556 | 0.2125 | 0.2693 | 0.3262 | 0.3830 | 0.4398 | 0.4967 | 0.5534 | 0.6103 | 0.6672 | 0.7240 |
x2 | 0.2945 | 0.3233 | 0.3522 | 0.3810 | 0.4099 | 0.4387 | 0.4676 | 0.4964 | 0.5253 | 0.5541 | 0.5830 |
x3 | 0.1767 | 0.2292 | 0.2817 | 0.3343 | 0.3868 | 0.4393 | 0.4919 | 0.5444 | 0.5969 | 0.6495 | 0.7020 |
x4 | 0.1172 | 0.1859 | 0.2416 | 0.2973 | 0.3529 | 0.4086 | 0.4643 | 0.5200 | 0.5756 | 0.6313 | 0.6870 |
The main contribution of the work is listed in the following points.
1) The present study proposed an integrated CIFS-SWARA-COPRAS method by utilizing the features of the CIFS information. CIFS is a poweful way to handle the imprecise information using the two-dimensional information including the phase term. In this stated framework, criteria weights are evaluated by the SWARA technique, and the ranking of alternatives is determined by the COPRAS method using CIFSs. The presented approach has been applied to handle the ERP software selection problem.
2) A multiple experts has been taken during the process to rate the information in terms of the CIFS features. A weighted average CIFWA operator has been used to aggregate such preferences. The criteria weight are computed by following the SWARA method which include the exactness of experts' opinion during the process.
3) A utility degree has been used to rank the different alternatives.
4) To verify the strength of the proposed technique a comparative study is conducted with the existing studies [6,20,21] and found that the proposed method has its superiority over these existing methods. Furthermore, the sensitivity analysis by varying the degree ϑ∈[0,1]. A decision maker may chose the the parameter according to their choices as pessimistic ϑ<0.5 or optimistic ϑ>0.5 or neutral ϑ=0.5 towards the benefit and cost criteria. The ranking order corresponding to each parameter is listed in Table 8 and Figure 2.
In future, the work will be developed, by considering a maximum number of alternatives and DEs as well as to solve real data. Moreover, we expand our work using different multi-criteria decision making platforms under the different environment [5,8,31,33].
There is no conflict of interest.
The authors would like to acknowledge to Consejo Nacional de Ciencia y Tecnologia for providing the funding with grant number CONACYT_SNI-CVU: 405262.
[1] | A. U. M. Alkouri, A. R. Salleh, Complex Intuitionistic Fuzzy Sets, AIP Conf. Proc., 1482 (2012), 464–470. |
[2] |
K. T. Atanassov, Intuitionistic fuzzy sets, Fuzzy Sets Syst., 20 (1986), 87–96. https://doi.org/10.1016/S0165-0114(86)80034-3 doi: 10.1016/S0165-0114(86)80034-3
![]() |
[3] |
J. J. Buckley, Fuzzy complex numbers, Fuzzy Sets Syst., 33 (1989), 333–345. https://doi.org/10.1016/0165-0114(89)90122-X doi: 10.1016/0165-0114(89)90122-X
![]() |
[4] |
D. E. Ighravwe, S. A. Oke, An integrated approach of SWARA and fuzzy COPRAS for maintenance technicians' selection factors ranking, Int. J. Syst. Assur. Eng. Manage., 10 (2019), 1615–1626. https://doi.org/10.1007/s13198-019-00912-8 doi: 10.1007/s13198-019-00912-8
![]() |
[5] |
F. Feng, Z. Wan, J. C. R. Alcantud, H. Garg, Three-way decision based on canonical soft sets of hesitant fuzzy sets, AIMS Math., 7 (2022), 2061–2083. https://doi.org/10.3934/math.2022118 doi: 10.3934/math.2022118
![]() |
[6] |
H. Garg, D. Rani, Some Generalized Complex Intuitionistic Fuzzy Aggregation Operators and Their Application to Multicriteria Decision-Making Process, Arabian J. Sci. Eng., 44 (2019), 2679–2698. https://doi.org/10.1007/s13369-018-3413-x doi: 10.1007/s13369-018-3413-x
![]() |
[7] |
H. Garg, R. Arora, Algorithms Based on COPRAS and Aggregation Operators with New Information Measures for Possibility Intuitionistic Fuzzy Soft Decision-Making, Math. Probl. Eng., 2020 (2020), 1563768. https://doi.org/10.1155/2020/1563768 doi: 10.1155/2020/1563768
![]() |
[8] |
H. Garg, Nancy, Algorithms for possibility linguistic single-valued neutrosophic decision-making based on COPRAS and aggregation operators with new information measures, Measurement, 138 (2019), 278–290. https://doi.org/10.1016/j.measurement.2019.02.031 doi: 10.1016/j.measurement.2019.02.031
![]() |
[9] |
H. Garg, D. Rani, New prioritized aggregation operators with priority degrees among priority orders for complex intuitionistic fuzzy information, J. Ambient Intell. Humanized Comput., 2021 (2021), 1–27. https://doi.org/10.1007/s12652-021-03164-2 doi: 10.1007/s12652-021-03164-2
![]() |
[10] |
M. Keil, A. Tiwana, Relative Importance of Evaluation Criteria for Enterprise Systems: A Conjoint Study, Inf. Syst. J., 16 (2006), 237–262. https://doi.org/10.1111/j.1365-2575.2006.00218.x doi: 10.1111/j.1365-2575.2006.00218.x
![]() |
[11] |
V. Kersuliene, E. Zavadskas, Z. Turskis, Selection of rational dispute resolution method by applying new step-wise weight assessment ratio analysis (SWARA), J. Bus. Econ. Manage., 11 (2010), 243–258. https://doi.org/10.3846/jbem.2010.12 doi: 10.3846/jbem.2010.12
![]() |
[12] |
Z. Kong, J. Zhao, L. Wang, J. Zhang, A new data filling approach based on probability analysis in incomplete soft sets, Expert Syst. Appl., 184 (2021), 115358. https://doi.org/10.1016/j.eswa.2021.115358 doi: 10.1016/j.eswa.2021.115358
![]() |
[13] |
T. Kumar, R. K. Bajaj, On complex intuitionistic fuzzy soft sets with distance measures and entropies, J. Math., 2014 (2014), 972198. https://doi.org/10.1155/2014/972198 doi: 10.1155/2014/972198
![]() |
[14] |
R. Kumari, A. R. Mishra, Multi-criteria COPRAS Method Based on Parametric Measures for Intuitionistic Fuzzy Sets: Application of Green Supplier Selection, Iran. J. Sci. Technol., Trans. Electr. Eng., 44 (2020), 1645–1662. https://doi.org/10.1007/s40998-020-00312-w doi: 10.1007/s40998-020-00312-w
![]() |
[15] |
J. Liu, L. Huaxiong, H. Bing, L. Yu, L. Dun, Convex combination-based consensus analysis for intuitionistic fuzzy three-way group decision, Inf. Sci., 574 (2021), 542–566. https://doi.org/10.1016/j.ins.2021.06.018 doi: 10.1016/j.ins.2021.06.018
![]() |
[16] | J. Ma, L. Feng, J. Yang, Using complex fuzzy sets for strategic cost evaluation in supply chain downstream, 2017 IEEE International Conference on Fuzzy Systems (FUZZ-IEEE), 2017, 1–6. https://doi.org/10.1109/FUZZ-IEEE.2017.8015452 |
[17] |
X. Ma, J. Zhan, M. Khan, Complex fuzzy sets with applications in signals, Comput. Appl. Math., 38 (2019), 150. https://doi.org/10.1007/s40314-019-0925-2 doi: 10.1007/s40314-019-0925-2
![]() |
[18] | P. K. Maji, R. Biswas, A. R. Roy, Intuitionistic fuzzy soft sets, J. Fuzzy Math., 9 (2001), 677–692. |
[19] |
T. Mahmood, Z. Ali, Prioritized Muirhead mean aggregation operators under the complex single-valued neutrosophic settings and their application in multi-attribute decision making, J. Comput. Cognit. Eng., (2021). https://doi.org/10.47852/bonviewJCCE2022010104 doi: 10.47852/bonviewJCCE2022010104
![]() |
[20] |
A. R. Mishra, R. K. Singh, D. Motwani, Multi-criteria assessment of cellular mobile telephone service providers using intuitionistic fuzzy WASPAS method with similarity measures, Granular Comput., 4 (2019), 511–529. https://doi.org/10.1007/s41066-018-0114-5 doi: 10.1007/s41066-018-0114-5
![]() |
[21] |
A. R. Mishra, P. Rani, K. Pandey, A. Mardani, J. Streimikis, D. Streimikiene, et al., Novel Multi-Criteria Intuitionistic Fuzzy SWARA-COPRAS Approach for Sustainability Evaluation of the Bioenergy Production Process, Sustainability, 12 (2020), 4155. https://doi.org/10.3390/su12104155 doi: 10.3390/su12104155
![]() |
[22] |
A. R. Mishra, P. Rani, A. Mardani, K. R. Pardasani, K. Govindan, M. Alrasheedi, Healthcare evaluation in hazardous waste recycling using novel interval-valued intuitionistic fuzzy information based on complex proportional assessment method, Comput. Ind. Eng., 139 (2020), 106140. https://doi.org/10.1016/j.cie.2019.106140 doi: 10.1016/j.cie.2019.106140
![]() |
[23] |
D. Molodtsov, Soft set theory - First result, Comput. Math. Appl., 37 (1999), 19–31. https://doi.org/10.1016/S0898-1221(99)00056-5 doi: 10.1016/S0898-1221(99)00056-5
![]() |
[24] |
R. T. Ngan, L. H. Son, M. Ali, D. E. Tamir, N. D. Rishe, A. Kandel, Representing complex intuitionistic fuzzy set by quaternion numbers and applications to decision making, Appl. Soft Comput., 87 (2020). https://doi.org/10.1016/j.asoc.2019.105961 doi: 10.1016/j.asoc.2019.105961
![]() |
[25] |
S. Rajareega, J. Vimala, Operations on complex intuitionistic fuzzy soft lattice ordered group and CIFS-COPRAS method for equipment selection process, J. Intell. Fuzzy Syst., 41 (2021), 5709–5718. https://doi.org/10.3233/JIFS-189890 doi: 10.3233/JIFS-189890
![]() |
[26] |
S. Rajareega, J. Vimala, D. Preethi, Complex Intuitionistic Fuzzy Soft Lattice Ordered Group and Its Weighted Distance Measures, Mathematics, 8 (2020), 705. https://doi.org/10.3390/math8050705 doi: 10.3390/math8050705
![]() |
[27] | S. Rajareega, J. Vimala, D. Preethi, The Role of (α,β) - Level set on Complex Intuitionistic Fuzzy Soft Lattice Ordered Groups, Int. J. Adv. Sci. Technol., 28 (2019), 116–123. |
[28] |
D. Ramot, R. Milo, M. Friedman, A. Kandel, Complex fuzzy sets, IEEE Trans. Fuzzy Syst., 10 (2002), 171–186. https://doi.org/10.1109/91.995119 doi: 10.1109/91.995119
![]() |
[29] |
D. Rani, H. Garg, Complex intuitionistic fuzzy power aggregation operators and their applications in multi-criteria decision-making, Expert Syst., 35 (2018), e12325. https://doi.org/10.1111/exsy.12325 doi: 10.1111/exsy.12325
![]() |
[30] |
P. Rani, A. R. Mishra, R. Krishankumar, A. Mardani, F. Cavallaro, K. S. Ravichandran, et al., Hesitant Fuzzy SWARA-Complex Proportional Assessment Approach for Sustainable Supplier Selection (HF-SWARA-COPRAS), Symmetry, 12 (2020), 1152. https://doi.org/10.3390/sym12071152 doi: 10.3390/sym12071152
![]() |
[31] |
M. Unver, M. Olgun, E. Turkarslan, Cosine and cotangent similarity measures based on Choquet integral for Spherical fuzzy sets and applications to pattern recognition, J. Comput. Cognit. Eng., (2021). https://doi.org/10.47852/bonviewJCCE2022010105 doi: 10.47852/bonviewJCCE2022010105
![]() |
[32] |
F. Xiao, CEQD: A complex mass function to predict interference effects, IEEE Trans. Cybern., 2021 (2021), 1–13. https://doi.org/10.1109/TCYB.2020.3040770 doi: 10.1109/TCYB.2020.3040770
![]() |
[33] |
F. Xiao, GIQ: A generalized intelligent quality-based approach for fusing multi-source information, IEEE Trans. Fuzzy Syst., 29 (2021), 2018–2031. https://doi.org/10.1109/TFUZZ.2020.2991296 doi: 10.1109/TFUZZ.2020.2991296
![]() |
[34] |
F. Xiao, Generalization of Dempster - Shafer theory: A complex mass function, Appl. Intell., 50 (2020), 3266–3275. https://doi.org/10.1007/s10489-019-01617-y doi: 10.1007/s10489-019-01617-y
![]() |
[35] |
F. Xiao, Generalized belief function in complex evidence theory, J. Intell. Fuzzy Syst., 38 (2020), 3665–3673. https://doi.org/10.3233/JIFS-179589 doi: 10.3233/JIFS-179589
![]() |
[36] |
J. Yang, Y. Yiyu, A three-way decision based construction of shadowed sets from Atanassov intuitionistic fuzzy sets, Inf. Sci., 577 (2021), 1–21. https://doi.org/10.1016/j.ins.2021.06.065 doi: 10.1016/j.ins.2021.06.065
![]() |
[37] |
J. Yang, Y. Yiyu, Z. Xianyong, A model of three-way approximation of intuitionistic fuzzy sets, Int. J. Mach. Learn. Cybern., 2021 (2021), 1–12. https://doi.org/10.1007/s13042-021-01380-y doi: 10.1007/s13042-021-01380-y
![]() |
[38] |
L. A. Zadeh, Fuzzy sets, Inf. Control, 8 (1965), 338–353. https://doi.org/10.1016/S0019-9958(65)90241-X doi: 10.1016/S0019-9958(65)90241-X
![]() |
[39] | E. Zavadskas, A. Kaklauskas, V. Sarka, The new method of multi criteria complex proportional assessment of projects, Technol. Econ. Dev. Econ., 1 (1994), 131–139. |
1. | Ivana Đaković Radojičić, Jelena Raut, Slavica Mitrović Veljković, Branislav Dudić, Silvia Treľová, Vijoleta Vrhovac, Factors That Limit the Development of the Digital Entrepreneurial System in the Scale-Up Phase of the Enterprise Life Cycle, 2022, 10, 2227-7390, 1606, 10.3390/math10091606 | |
2. | Muhammad Ihsan, Muhammad Saeed, Atiqe Ur Rahman, Hamiden Abd El-Wahed Khalifa, Salwa El-Morsy, An intelligent fuzzy parameterized multi-criteria decision-support system based on intuitionistic fuzzy hypersoft expert set for automobile evaluation, 2022, 14, 1687-8132, 168781322211100, 10.1177/16878132221110005 | |
3. | Huilin Li, Jiaqi Yang, Ziquan Xiang, A Fuzzy Linguistic Multi-Criteria Decision-Making Approach to Assess Emergency Suppliers, 2022, 14, 2071-1050, 13114, 10.3390/su142013114 | |
4. | Yu Deng, Sustainable competitiveness evaluation of regional financial centers with fuzzy number intuitionistic fuzzy TODIM algorithms, 2023, 10641246, 1, 10.3233/JIFS-221247 | |
5. | Hui Liu, Performance evaluation of family business strategic transition based on the 2-tuple linguistic neutrosophic number multiple attribute group decision making, 2023, 44, 10641246, 3271, 10.3233/JIFS-221514 | |
6. | Mouhamed Bayane Bouraima, Yanjun Qiu, Željko Stević, Vladimir Simić, Assessment of alternative railway systems for sustainable transportation using an integrated IRN SWARA and IRN CoCoSo model, 2023, 86, 00380121, 101475, 10.1016/j.seps.2022.101475 | |
7. | Abeer Ali Alharbi, Dilshad Alghazzawi, Some Characterizations of Certain Complex Fuzzy Subgroups, 2022, 14, 2073-8994, 1812, 10.3390/sym14091812 | |
8. | Nazlı GOKER, COVID-19 Pandemisi Döneminde Çevik Dış Kaynak Sağlayıcısı Seçimi Problemi İçin Sezgisel Bulanık Çok Ölçütlü Karar Verme Yöntemi: Türk Beyaz Eşya Sektöründe Bir Uygulama, 2022, 2146-0574, 2097, 10.21597/jist.1102015 | |
9. | Saeid Jafarzadeh Ghoushchi, Mohd Nizam Ab Ab Rahman, Moein Soltanzadeh, Muhammad Zeeshan Rafique, Hernadewita Hernadewita, Fatemeh Yadegar Marangalo, Ahmad Rasdan Ismail, Assessing Sustainable Passenger Transportation Systems to Address Climate Change Based on MCDM Methods in an Uncertain Environment, 2023, 15, 2071-1050, 3558, 10.3390/su15043558 | |
10. | Songsong Dai, Linguistic Complex Fuzzy Sets, 2023, 12, 2075-1680, 328, 10.3390/axioms12040328 | |
11. | Karahan Kara, Galip Cihan Yalçın, Vladimir Simic, İsmail Önden, Sercan Edinsel, Nebojsa Bacanin, A single-valued neutrosophic-based methodology for selecting warehouse management software in sustainable logistics systems, 2024, 129, 09521976, 107626, 10.1016/j.engappai.2023.107626 | |
12. | S. Anita Shanthi, K.G. Basavaraju, D.A. Joshi, N.B. Ibrahim, D.M. Sangeetha, Estimating the overall efficiency of storage batteries in Fermatean picture fuzzy environment, 2023, 405, 2267-1242, 02028, 10.1051/e3sconf/202340502028 | |
13. | Kannan Jeevitha, Harish Garg, Jayakumar Vimala, Hanan Aljuaid, Abdel-Haleem Abdel-Aty, Linear diophantine multi-fuzzy aggregation operators and its application in digital transformation, 2023, 45, 10641246, 3097, 10.3233/JIFS-223844 | |
14. | A. S. Wungreiphi, Fokrul Alom Mazarbhuiya, Mohamed Shenify, On Extended Lr-Norm-Based Derivatives to Intuitionistic Fuzzy Sets, 2023, 12, 2227-7390, 139, 10.3390/math12010139 | |
15. | Jayakumar Vimala, Harish Garg, Kannan Jeevitha, Prognostication of Myocardial Infarction Using Lattice Ordered Linear Diophantine Multi-fuzzy Soft Set, 2024, 26, 1562-2479, 44, 10.1007/s40815-023-01574-2 | |
16. | Mouhamed Bayane Bouraima, Janet Oyaro, Ertugrul Ayyildiz, Melike Erdogan, Ndiema Kevin Maraka, An integrated decision support model for effective institutional coordination framework in planning for public transportation, 2023, 1432-7643, 10.1007/s00500-023-09425-w | |
17. | Wentao Deng, Guoqing Ma, Methodology for evaluating the ideological and political education quality of chinese universities based on fuzzy number intuitionistic fuzzy multiple-attribute decision-making, 2023, 45, 10641246, 1103, 10.3233/JIFS-224145 | |
18. | Bing Cao, Yongsheng Jin, Alptekin Ulutaş, Ayse Topal, Željko Stević, Darjan Karabasevic, Cipriana Sava, A new integrated rough multi-criteria decision-making model for enterprise resource planning software selection, 2024, 10, 2376-5992, e2096, 10.7717/peerj-cs.2096 | |
19. | Yushuo Cao, Xiao Han, Xuzhong Wu, Muhammet Deveci, Seifedine Kadry, Dursun Delen, Evaluation of food waste treatment techniques using the complex q-rung orthopair fuzzy generalized TODIM method with weighted power geometric operator, 2024, 353, 03014797, 120105, 10.1016/j.jenvman.2024.120105 | |
20. | Fu Chen, A decision-making framework for classroom teaching quality evaluation of college badminton training with 2-tuple linguistic neutrosophic numbers, 2023, 45, 10641246, 5939, 10.3233/JIFS-231731 | |
21. | Mouhamed Bayane Bouraima, Yanjun Qiu, Ertugrul Ayyildiz, Aslihan Yildiz, Prioritization of strategies for a sustainable regional transportation infrastructure by hybrid spherical fuzzy group decision-making approach, 2023, 35, 0941-0643, 17967, 10.1007/s00521-023-08660-4 | |
22. | Jayakumar Vimala, P. Mahalakshmi, Atiqe Ur Rahman, Muhammad Saeed, A customized TOPSIS method to rank the best airlines to fly during COVID-19 pandemic with q-rung orthopair multi-fuzzy soft information, 2023, 27, 1432-7643, 14571, 10.1007/s00500-023-08976-2 | |
23. | Şura Toptancı, Hakan Gökhan Gündoğdu, Selçuk Korucuk, Ahmet Aytekin, Željko Stević, Corporate sustainability strategy selection for a metropolitan municipality using a trapezoidal interval type-2 fuzzy SWARA–COPRAS framework, 2023, 1432-7643, 10.1007/s00500-023-08800-x | |
24. | Dana Marsetiya Utama, Muhammad Faisal Ibrahim, Ahmed Nedal Abid Al Kareem Jabari, A Fuzzy Multi-Criteria Approach for Selecting Open-Source ERP Systems in SMEs Using Fuzzy AHP and TOPSIS, 2025, 23, 2442-8795, 167, 10.25077/josi.v23.n2.p167-187.2024 | |
25. | Nadeem Salamat, Muhammad Kamran, Shahzaib Ashraf, Manal Elzain Mohammed Abdulla, Rashad Ismail, Mohammed M. Al-Shamiri, Complex Decision Modeling Framework with Fairly Operators and Quaternion Numbers under Intuitionistic Fuzzy Rough Context, 2024, 139, 1526-1506, 1893, 10.32604/cmes.2023.044697 |
Criteria | Description | Direction |
a1 | Functionality | + |
a2 | Reliability | + |
a3 | Vendor Viability | + |
a4 | Cost | - |
a5 | Support and Training | + |
a6 | Ease of Use | + |
a7 | Difficulty in Customization and Implementation | - |
Alternatives | |||||
Criteria | Experts | x1 | x2 | x3 | x4 |
E1 | L, SL | H, SH | M, M | H, SH | |
a1 | E2 | H, SH | M, M | H, SH | VH, EH |
E3 | H, SH | L, SL | L, SL | M, M | |
E1 | H, SH | M, M | H, SH | L, SL | |
a2 | E2 | VH, EH | L, SL | M, M | VL, EL |
E3 | H, SH | VH, EH | H, SH | L, SL | |
E1 | M, M | H, SH | VH, EH | M, M | |
a3 | E2 | H, SH | VH, EH | H, SH | VH, EH |
E3 | VH, EH | L, SL | VL, EL | H, SH | |
E1 | H, SH | M, M | L, SL | M, M | |
a4 | E2 | H, SH | L, SL | L, SL | H, SH |
E3 | L, SL | VL, EL | H, SH | L, SL | |
E1 | VL, EL | L, SL | H, SH | VH, EH | |
a5 | E2 | H, SH | VL, EL | M, M | H, SH |
E3 | M, M | VH, EH | L, SL | VH, EH | |
E1 | VH, EH | L, SL | H, SH | M, M | |
a6 | E2 | M, M | M, M | VH, EH | H, SH |
E3 | H, SH | VH, EH | M, M | VL, EL | |
E1 | VL, EL | M, M | H, SH | L, SL | |
a7 | E2 | L, SL | VL, EL | M, M | H, SH |
E3 | M, M | L, SL | H, SH | M, M |
r | s | τ | ψ | |
EH- Extremely high | 0.95 | (0.9)2π | 0.02 | (0.1)2π |
VH- Very High | 0.90 | (0.8)2π | 0.05 | (0.15)2π |
H- High | 0.80 | (0.75)2π | 0.10 | (0.20)2π |
SH- Slightly High | 0.70 | (0.7)2π | 0.25 | (0.1)2π |
M- Medium | 0.65 | (0.5)2π | 0.30 | (0.4)2π |
SL- Slightly Low | 0.50 | (0.3)2π | 0.45 | (0.6)2π |
L- Low | 0.40 | (0.25)2π | 0.50 | (0.6)2π |
VL- Very Low | 0.25 | (0.2)2π | 0.60 | (0.65)2π |
EL- Extremely Low | 0.15 | (0.1)2π | 0.65 | (0.7)2π |
x1 | x2 | x3 | x4 | |
a1 | (0.736ei2π(0.628),0.150ei2π(0.157)) | (0.675ei2π(0.542),0.243ei2π(0.296)) | (0.735ei2π(0.620),0.162ei2π(0.178)) | (0.861ei2π(0.838),0.075ei2π(0.119)) |
a2 | (0.870ei2π(0.848),0.065ei2π(0.100)) | (0.582ei2π(0.497),0.329ei2π(0.432)) | (0.717ei2π(0.588),0.198ei2π(0.237)) | (0.291ei2π(0.155),0.573ei2π(0.673)) |
a3 | (0.789ei2π(0.703),0.121ei2π(0.142)) | (0.851ei2π(0.831),0.080ei2π(0.125)) | (0.802ei2π(0.739),0.105ei2π(0.128)) | (0.850ei2π(0.827),0.086ei2π(0.142)) |
a4 | (0.770ei2π(0.666),0.123ei2π(0.125)) | (0.462ei2π(0.336),0.450ei2π(0.552)) | (0.478ei2π(0.371),0.408ei2π(0.479)) | (0.735ei2π(0.620),0.162ei2π(0.178)) |
a5 | (0.700ei2π(0.578),0.181ei2π(0.195)) | (0.450ei2π(0.360),0.419ei2π(0.527)) | (0.675ei2π(0.542),0.242ei2π(0.296)) | (0.846ei2π(0.802),0.077ei2π(0.100)) |
a6 | (0.762ei2π(0.688),0.166ei2π(0.237)) | (0.657ei2π(0.556),0.272ei2π(0.372)) | (0.861ei2π(0.838),0.075ei2π(0.119)) | (0.728ei2π(0.608),0.166ei2π(0.182)) |
a7 | (0.407ei2π(0.285),0.491ei2π(0.593)) | (0.399ei2π(0.280),0.492ei2π(0.596)) | (0.717ei2π(0.588),0.198ei2π(0.237)) | (0.688ei2π(0.573),0.188ei2π(0.201)) |
E1 | E2 | E3 | Aggregated Value | |
a1 | (0.65ei2π(0.5),0.30ei2π(0.4)) | (0.80ei2π(0.7),0.10ei2π(0.1)) | (0.40ei2π(0.3),0.50ei2π(0.6)) | (0.735ei2π(0.620),0.162ei2π(0.178)) |
a2 | (0.80ei2π(0.7),0.1ei2π(0.1)) | (0.90ei2π(0.9),0.05ei2π(0.1)) | (0.90ei2π(0.9),0.05ei2π(0.1)) | (0.881ei2π(0.868),0.060ei2π(0.100)) |
a3 | (0.40ei2π(0.3),0.50ei2π(0.6)) | (0.65ei2π(0.5),0.30ei2π(0.4)) | (0.80ei2π(0.7),0.10ei2π(0.1)) | (0.626ei2π(0.490),0.297ei2π(0.372)) |
a4 | (0.25ei2π(0.1),0.60ei2π(0.7)) | (0.40ei2π(0.3),0.50ei2π(0.6)) | (0.25ei2π(0.3),0.50ei2π(0.6)) | (0.347ei2π(0.254),0.524ei2π(0.624)) |
a5 | (0.65ei2π(0.5),0.30ei2π(0.4)) | (0.80ei2π(0.7),0.10ei2π(0.1)) | (0.80ei2π(0.7),0.10ei2π(0.1)) | (0.770ei2π(0.659),0.132ei2π(0.142)) |
a6 | (0.80ei2π(0.7),0.10ei2π(0.1)) | (0.80ei2π(0.7),0.10ei2π(0.1)) | (0.90ei2π(0.9),0.05ei2π(0.1)) | (0.817ei2π(0.739),0.092ei2π(0.100)) |
a7 | (0.40ei2π(0.3),0.50ei2π(0.6)) | (0.25ei2π(0.3),0.50ei2π(0.6)) | (0.25ei2π(0.3),0.50ei2π(0.6)) | (0.291ei2π(0.155),0.573ei2π(0.673)) |
Criteria | Ordered crisp value kj | Comparative coefficient pj | Comparative weights qj | Recalculated wj | Criteria weights |
a2 | 0.897 | - | 1 | 1 | 0.177 |
a6 | 0.841 | 0.056 | 1.056 | 0.947 | 0.167 |
a5 | 0.789 | 0.052 | 1.052 | 0.900 | 0.159 |
a1 | 0.754 | 0.035 | 1.035 | 0.870 | 0.154 |
a3 | 0.612 | 0.142 | 1.142 | 0.762 | 0.135 |
a4 | 0.363 | 0.249 | 1.249 | 0.610 | 0.108 |
a7 | 0.300 | 0.063 | 1.063 | 0.574 | 0.101 |
x1 | x2 | x3 | x4 | Rank Order | |
IF-WASPAS [20] | |||||
(ⅰ) Weight Sum Model (WSM) | 0.9155 | 0.8175 | 0.9300 | 0.9025 | x3≻x1≻x4≻x2 |
(ⅱ) Weight Product Model (WPM) | 0.6965 | 0.5305 | 0.7270 | 0.6250 | x3≻x1≻x4≻x2 |
(ⅲ) Weighted Aggregated Sum Product | |||||
Assessment (WASPAS) with ϑ=0.5 | 0.8065 | 0.6745 | 0.8285 | 0.7640 | x3≻x1≻x4≻x2 |
IF-SWARA-COPRAS [21] | 0.8985 | 0.8660 | 0.9270 | 0.8580 | x3≻x1≻x2≻x4 |
CIFWA operator with criteria weights wj [6] | 0.7595 | 0.6275 | 0.7545 | 0.6145 | x1≻x3≻x2≻x4 |
Proposed CIFS - SWARA - COPRAS | 0.8796 | 0.8775 | 0.8787 | 0.8172 | x1≻x3≻x2≻x4 |
ϑ | 0 | 0.1 | 0.2 | 0.3 | 0.4 | 0.5 | 0.6 | 0.7 | 0.8 | 0.9 | 1 |
x1 | 0.1556 | 0.2125 | 0.2693 | 0.3262 | 0.3830 | 0.4398 | 0.4967 | 0.5534 | 0.6103 | 0.6672 | 0.7240 |
x2 | 0.2945 | 0.3233 | 0.3522 | 0.3810 | 0.4099 | 0.4387 | 0.4676 | 0.4964 | 0.5253 | 0.5541 | 0.5830 |
x3 | 0.1767 | 0.2292 | 0.2817 | 0.3343 | 0.3868 | 0.4393 | 0.4919 | 0.5444 | 0.5969 | 0.6495 | 0.7020 |
x4 | 0.1172 | 0.1859 | 0.2416 | 0.2973 | 0.3529 | 0.4086 | 0.4643 | 0.5200 | 0.5756 | 0.6313 | 0.6870 |
Criteria | Description | Direction |
a1 | Functionality | + |
a2 | Reliability | + |
a3 | Vendor Viability | + |
a4 | Cost | - |
a5 | Support and Training | + |
a6 | Ease of Use | + |
a7 | Difficulty in Customization and Implementation | - |
Alternatives | |||||
Criteria | Experts | x1 | x2 | x3 | x4 |
E1 | L, SL | H, SH | M, M | H, SH | |
a1 | E2 | H, SH | M, M | H, SH | VH, EH |
E3 | H, SH | L, SL | L, SL | M, M | |
E1 | H, SH | M, M | H, SH | L, SL | |
a2 | E2 | VH, EH | L, SL | M, M | VL, EL |
E3 | H, SH | VH, EH | H, SH | L, SL | |
E1 | M, M | H, SH | VH, EH | M, M | |
a3 | E2 | H, SH | VH, EH | H, SH | VH, EH |
E3 | VH, EH | L, SL | VL, EL | H, SH | |
E1 | H, SH | M, M | L, SL | M, M | |
a4 | E2 | H, SH | L, SL | L, SL | H, SH |
E3 | L, SL | VL, EL | H, SH | L, SL | |
E1 | VL, EL | L, SL | H, SH | VH, EH | |
a5 | E2 | H, SH | VL, EL | M, M | H, SH |
E3 | M, M | VH, EH | L, SL | VH, EH | |
E1 | VH, EH | L, SL | H, SH | M, M | |
a6 | E2 | M, M | M, M | VH, EH | H, SH |
E3 | H, SH | VH, EH | M, M | VL, EL | |
E1 | VL, EL | M, M | H, SH | L, SL | |
a7 | E2 | L, SL | VL, EL | M, M | H, SH |
E3 | M, M | L, SL | H, SH | M, M |
r | s | τ | ψ | |
EH- Extremely high | 0.95 | (0.9)2π | 0.02 | (0.1)2π |
VH- Very High | 0.90 | (0.8)2π | 0.05 | (0.15)2π |
H- High | 0.80 | (0.75)2π | 0.10 | (0.20)2π |
SH- Slightly High | 0.70 | (0.7)2π | 0.25 | (0.1)2π |
M- Medium | 0.65 | (0.5)2π | 0.30 | (0.4)2π |
SL- Slightly Low | 0.50 | (0.3)2π | 0.45 | (0.6)2π |
L- Low | 0.40 | (0.25)2π | 0.50 | (0.6)2π |
VL- Very Low | 0.25 | (0.2)2π | 0.60 | (0.65)2π |
EL- Extremely Low | 0.15 | (0.1)2π | 0.65 | (0.7)2π |
x1 | x2 | x3 | x4 | |
a1 | (0.736ei2π(0.628),0.150ei2π(0.157)) | (0.675ei2π(0.542),0.243ei2π(0.296)) | (0.735ei2π(0.620),0.162ei2π(0.178)) | (0.861ei2π(0.838),0.075ei2π(0.119)) |
a2 | (0.870ei2π(0.848),0.065ei2π(0.100)) | (0.582ei2π(0.497),0.329ei2π(0.432)) | (0.717ei2π(0.588),0.198ei2π(0.237)) | (0.291ei2π(0.155),0.573ei2π(0.673)) |
a3 | (0.789ei2π(0.703),0.121ei2π(0.142)) | (0.851ei2π(0.831),0.080ei2π(0.125)) | (0.802ei2π(0.739),0.105ei2π(0.128)) | (0.850ei2π(0.827),0.086ei2π(0.142)) |
a4 | (0.770ei2π(0.666),0.123ei2π(0.125)) | (0.462ei2π(0.336),0.450ei2π(0.552)) | (0.478ei2π(0.371),0.408ei2π(0.479)) | (0.735ei2π(0.620),0.162ei2π(0.178)) |
a5 | (0.700ei2π(0.578),0.181ei2π(0.195)) | (0.450ei2π(0.360),0.419ei2π(0.527)) | (0.675ei2π(0.542),0.242ei2π(0.296)) | (0.846ei2π(0.802),0.077ei2π(0.100)) |
a6 | (0.762ei2π(0.688),0.166ei2π(0.237)) | (0.657ei2π(0.556),0.272ei2π(0.372)) | (0.861ei2π(0.838),0.075ei2π(0.119)) | (0.728ei2π(0.608),0.166ei2π(0.182)) |
a7 | (0.407ei2π(0.285),0.491ei2π(0.593)) | (0.399ei2π(0.280),0.492ei2π(0.596)) | (0.717ei2π(0.588),0.198ei2π(0.237)) | (0.688ei2π(0.573),0.188ei2π(0.201)) |
E1 | E2 | E3 | Aggregated Value | |
a1 | (0.65ei2π(0.5),0.30ei2π(0.4)) | (0.80ei2π(0.7),0.10ei2π(0.1)) | (0.40ei2π(0.3),0.50ei2π(0.6)) | (0.735ei2π(0.620),0.162ei2π(0.178)) |
a2 | (0.80ei2π(0.7),0.1ei2π(0.1)) | (0.90ei2π(0.9),0.05ei2π(0.1)) | (0.90ei2π(0.9),0.05ei2π(0.1)) | (0.881ei2π(0.868),0.060ei2π(0.100)) |
a3 | (0.40ei2π(0.3),0.50ei2π(0.6)) | (0.65ei2π(0.5),0.30ei2π(0.4)) | (0.80ei2π(0.7),0.10ei2π(0.1)) | (0.626ei2π(0.490),0.297ei2π(0.372)) |
a4 | (0.25ei2π(0.1),0.60ei2π(0.7)) | (0.40ei2π(0.3),0.50ei2π(0.6)) | (0.25ei2π(0.3),0.50ei2π(0.6)) | (0.347ei2π(0.254),0.524ei2π(0.624)) |
a5 | (0.65ei2π(0.5),0.30ei2π(0.4)) | (0.80ei2π(0.7),0.10ei2π(0.1)) | (0.80ei2π(0.7),0.10ei2π(0.1)) | (0.770ei2π(0.659),0.132ei2π(0.142)) |
a6 | (0.80ei2π(0.7),0.10ei2π(0.1)) | (0.80ei2π(0.7),0.10ei2π(0.1)) | (0.90ei2π(0.9),0.05ei2π(0.1)) | (0.817ei2π(0.739),0.092ei2π(0.100)) |
a7 | (0.40ei2π(0.3),0.50ei2π(0.6)) | (0.25ei2π(0.3),0.50ei2π(0.6)) | (0.25ei2π(0.3),0.50ei2π(0.6)) | (0.291ei2π(0.155),0.573ei2π(0.673)) |
Criteria | Ordered crisp value kj | Comparative coefficient pj | Comparative weights qj | Recalculated wj | Criteria weights |
a2 | 0.897 | - | 1 | 1 | 0.177 |
a6 | 0.841 | 0.056 | 1.056 | 0.947 | 0.167 |
a5 | 0.789 | 0.052 | 1.052 | 0.900 | 0.159 |
a1 | 0.754 | 0.035 | 1.035 | 0.870 | 0.154 |
a3 | 0.612 | 0.142 | 1.142 | 0.762 | 0.135 |
a4 | 0.363 | 0.249 | 1.249 | 0.610 | 0.108 |
a7 | 0.300 | 0.063 | 1.063 | 0.574 | 0.101 |
x1 | x2 | x3 | x4 | Rank Order | |
IF-WASPAS [20] | |||||
(ⅰ) Weight Sum Model (WSM) | 0.9155 | 0.8175 | 0.9300 | 0.9025 | x3≻x1≻x4≻x2 |
(ⅱ) Weight Product Model (WPM) | 0.6965 | 0.5305 | 0.7270 | 0.6250 | x3≻x1≻x4≻x2 |
(ⅲ) Weighted Aggregated Sum Product | |||||
Assessment (WASPAS) with ϑ=0.5 | 0.8065 | 0.6745 | 0.8285 | 0.7640 | x3≻x1≻x4≻x2 |
IF-SWARA-COPRAS [21] | 0.8985 | 0.8660 | 0.9270 | 0.8580 | x3≻x1≻x2≻x4 |
CIFWA operator with criteria weights wj [6] | 0.7595 | 0.6275 | 0.7545 | 0.6145 | x1≻x3≻x2≻x4 |
Proposed CIFS - SWARA - COPRAS | 0.8796 | 0.8775 | 0.8787 | 0.8172 | x1≻x3≻x2≻x4 |
ϑ | 0 | 0.1 | 0.2 | 0.3 | 0.4 | 0.5 | 0.6 | 0.7 | 0.8 | 0.9 | 1 |
x1 | 0.1556 | 0.2125 | 0.2693 | 0.3262 | 0.3830 | 0.4398 | 0.4967 | 0.5534 | 0.6103 | 0.6672 | 0.7240 |
x2 | 0.2945 | 0.3233 | 0.3522 | 0.3810 | 0.4099 | 0.4387 | 0.4676 | 0.4964 | 0.5253 | 0.5541 | 0.5830 |
x3 | 0.1767 | 0.2292 | 0.2817 | 0.3343 | 0.3868 | 0.4393 | 0.4919 | 0.5444 | 0.5969 | 0.6495 | 0.7020 |
x4 | 0.1172 | 0.1859 | 0.2416 | 0.2973 | 0.3529 | 0.4086 | 0.4643 | 0.5200 | 0.5756 | 0.6313 | 0.6870 |