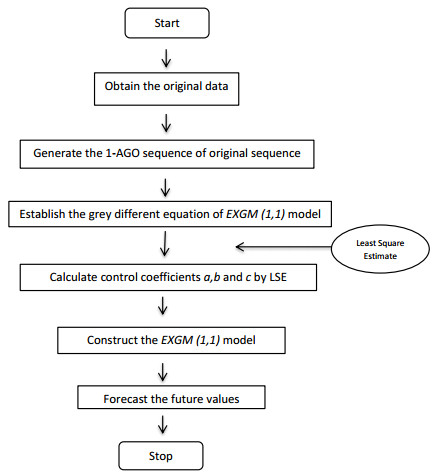
Citation: Halis Bilgil. New grey forecasting model with its application and computer code[J]. AIMS Mathematics, 2021, 6(2): 1497-1514. doi: 10.3934/math.2021091
[1] | Yubin Cai, Xin Ma . A novel ensemble learning-based grey model for electricity supply forecasting in China. AIMS Mathematics, 2021, 6(11): 12339-12358. doi: 10.3934/math.2021714 |
[2] | Maolin Cheng . A new method for parameter estimation of extended grey GM(2, 1) model based on difference equation and its application. AIMS Mathematics, 2023, 8(7): 15993-16012. doi: 10.3934/math.2023816 |
[3] | Caixia Liu, Wanli Xie . An optimized fractional grey model based on weighted least squares and its application. AIMS Mathematics, 2023, 8(2): 3949-3968. doi: 10.3934/math.2023198 |
[4] | Dewang Li, Meilan Qiu, Shuiping Yang, Chao Wang, Zhongliang Luo . An optimal fractional-order accumulative Grey Markov model with variable parameters and its application in total energy consumption. AIMS Mathematics, 2023, 8(11): 26425-26443. doi: 10.3934/math.20231349 |
[5] | Yichen Lv, Xinping Xiao . Grey parameter estimation method for extreme value distribution of short-term wind speed data. AIMS Mathematics, 2024, 9(3): 6238-6265. doi: 10.3934/math.2024304 |
[6] | Hong Yang, Jiangli Liu . A novel unemployment rate forecasting method based on fuzzy information granules and GM(1,1) model. AIMS Mathematics, 2024, 9(4): 8689-8711. doi: 10.3934/math.2024421 |
[7] | Mustafa Ekici . On an axiomatization of the grey Banzhaf value. AIMS Mathematics, 2023, 8(12): 30405-30418. doi: 10.3934/math.20231552 |
[8] | Dewang Li, Daming Xu, Meilan Qiu, Shuiping Yang . Forecasting the public financial budget expenditure in Dongguan with an optimal weighted combination Markov model. AIMS Mathematics, 2023, 8(7): 15600-15617. doi: 10.3934/math.2023796 |
[9] | I. H. K. Premarathna, H. M. Srivastava, Z. A. M. S. Juman, Ali AlArjani, Md Sharif Uddin, Shib Sankar Sana . Mathematical modeling approach to predict COVID-19 infected people in Sri Lanka. AIMS Mathematics, 2022, 7(3): 4672-4699. doi: 10.3934/math.2022260 |
[10] | CW Chukwu, S. Y. Tchoumi, Z. Chazuka, M. L. Juga, G. Obaido . Assessing the impact of human behavior towards preventative measures on COVID-19 dynamics for Gauteng, South Africa: a simulation and forecasting approach. AIMS Mathematics, 2024, 9(5): 10511-10535. doi: 10.3934/math.2024514 |
The grey prediction models play an important role in the grey system theory, which was proposed by Deng in 1982 [1]. These models are often named as grey models (GM) as they are developed based on the grey system theory. The grey models have been useful in solving uncertain problems with small samples and inadequate information.
The grey prediction models have been widely and successfully applied to various fields, such as industry, science and technology, economy, energy consumption and other fields [2,3,4,5,6,7,8,9]. In recent years, some extended and modified the grey prediction models have been developed based on GM (1, 1) because of practicality and the prediction accuracy. Ma and Lui [10] proposed a time-delayed polynomial grey prediction model called TDPGM (1, 1) model, Cui et al. [11] developed a parameter optimization method to improve the ONGM (1, 1, k) model, Ma et al. [12] developed a novel nonlinear multivariate forecasting grey model based on the Bernoulli equation named NGBMC (1, n), Wang et al. [13] introduced a seasonal grey model called SGM (1, 1), Wu et al. [14] proposed a new grey model alled BNGM (1, 1, t2) model, Liu and Wu [15] proposed ANDGM model, Ye et al. [16] proposed a novel accumulative time-delay multivariate grey prediction model called ATGM (1, N), Wu et al. [17] developed a novel grey Riccati model (GRM).
Generally all models in the literature are suitable when the given data sequence satisfies exponential growth. However, the abnormal decreasing in the given data will have negatively effects on the prediction accuracy. The main point of this article is to improve predictive accuracy by adding a decreasing term, (e−t), in the whitenization differential equation. Therefore, the monotone decreasing term (e−t) will suppress the growth of the prediction error. The whitening equation is taken as a linear equation of time in the most of studies on the grey modelling mechanism. However, these models generally neglects the second-order Taylor expansion in the right side of the equation. Thence the errors produced by the model will increase with time, and the structural relations of the variables will not be accurately represented. The standart GM (1, 1) model is improved by Kedong et al. [18] for these reasons and they propose a new EOGM (1, 1) model. In this paper, we propose to further improve the EOGM (1, 1) grey forecasting model and obtain a better prediction of the forecasting results. The right side of EOGM (1, 1) is modified by adding a paramater to balance of abnormal changing in the given data. In addition, the parameters are estimated by using the linear least squares estimation to the a parameter is taken as a=1 in EOGM (1, 1).
The new Coronavirus (COVID-19) is an emerging disease responsible for infecting millions of people since the first notification until nowadays. Today (Jan 9, 2020), there have been reported approximately 280 thousand confirmed COVID-19 cases and 6.2 thousand deaths in TURKEY since Jan 3, 2020. Developing efficient short-term forecasting models allow forecasting the number of future cases. The findings of this research may help government and other agencies to reshape their strategies according to the forecasted situation. As the data generating process is identified in terms of time series models, then it can be updated with the arrival of new data and provide forecasted scenario in future.
The novelty of this paper is essentially shown in two viewpoints. Firstly, this paper introduces an exponential optimization grey model termed EXGM (1, 1) model to improve the prediction accuracy of grey forecasting model. Secondly, it is to predict the future output value of short-term total COVID-19 cases in Turkey.
This paper is outlined as follows: Section 1 includes relevant literature. Section 2 introduces the original GM (1, 1) model. The definition and theorem of EXGM (1, 1) is introduced in Section 3. In Section 4, we present a series of samples to validate EXGM (1, 1). The prediction concerning short-term COVID-19 cases in Turkey will be conducted in Section 4. Section 5 includes computer code. Finally, the conclusions of this study are given in Sections 6.
The grey model GM (1, 1) is one of the most commonly used grey forecasting models and requires at least four observations. Firstly, an accumulating generation operator (AGO) is applied to the data and then the governing differential equation of the model is solved to obtain the predicted value of the system. Finally, the predicted value of the original data is obtained by using the inverse accumulating generation operator (IAGO). The traditional GM (1, 1) modeling process is as follows:
X(0)={x(0)(1),x(0)(2),...,x(0)(n)} | (2.1) |
is a non-negative sequence of raw data and it's the accumulating generation (AGO) sequence X(1) is
X(1)={x(1)(1),x(1)(2),...,x(1)(n)}, | (2.2) |
where x(1)(1)=x(0)(1) and,
x(1)(k)=k∑i=1x(0)(k), k=2,3,..,n | (2.3) |
and the sequence mean generated of consecutive neighbors of X(1) is,
Z(1)={z(1)(1),z(1)(2),...,z(1)(n)} | (2.4) |
where,
z(1)(k)=x(1)(k)+x(1)(k−1)2, k=2,...,n. | (2.5) |
The equation,
x(0)(k)+az(1)(k)=b | (2.6) |
is called the basic form of the GM (1, 1) model and the whitenization equation is established as,
dx(1)dt+ax(1)=b. | (2.7) |
The whitenization equation is solved and the prediction value of X(1) can be calculated as following
ˆx(1)(k)=(x(0)(1)−ba)e−a(k−1)+ba, k=2,3,... | (2.8) |
Therefore the prediction values can be generated by,
ˆx(0)(1)=x(0)(1)ˆx(0)(k)=ˆx(1)(k)−ˆx(1)(k−1), k=2,3,...,n. | (2.9) |
In Eq (2.6), k is a time point, a is a the development and b is called driving coefficients [19]. The parameters a, b in Eq (2.6) can be estimated using the least squares method with the difference Eq (2.6) as,
[a,b]T=[BTB]−1BTY, | (2.10) |
where
B=[−z(1)(2)1−z(1)(3)1....−z(1)(n)1], Y=[x(0)(2)x(0)(3)..x(0)(n)]. | (2.11) |
The readers may consults the reference [11] for the proof of the traditional GM (1, 1) model, so its details were omitted here.
In this section, we will introduce a novel exponential grey prediction model, called as the EXGM (1, 1) model. The exponential change of the raw data is an important property of the grey forecasting model. If the exponential variation of the raw data sequence is separated, it can be seen that the grey action quantity is time dependent and this change is exponential with time. The standard GM (1, 1) treats the grey action quantity as a constant and its effect is inadequate for the prediction accuracy. Hence, the estimated error produced by the model will increase with time. Therefore, this study considers the grey action quantity as an exponential function of time and a constant.
Definition 1. The linear differential equation
dx(1)(t)dt+ax(1)(t)=b+ce−t | (3.1) |
is called the whitening equation of the EXGM (1, 1) model. One of the reasons for choosing a linear equation is that the sequence Eq (2.1) is monotonically increased and the solution of the linear equations include increased exponential functions. Another reason is that the first order derivative in the linear equation can be written as a difference equation form. \end{definition}The grey derivative for the first-order grey differential equation with AGO data as the intermediate information is represented as,
dx(1)(t)dt=limΔt→0x(1)(t+Δt)−x(1)(t)Δt | (3.2) |
where Δt represents the increment of the parameter t which can be time, position or other usable parameter, and considered to be constant [20]. Hence, we can make it as the unit amount, while x(1)(t+Δt)−x(1)(t) is data difference between the consecutive points in the data sequence, therefore
dx(1)(t)dt≈x(1)(k+1)−x(1)(k)=x(0)(k). | (3.3) |
Theorem 1.
x(0)(k)+az(1)(k)=b+c(e−1)e−k | (3.4) |
is called the basic difference equation of the EXGM (1, 1) model, where z(1)(k) is given by Eq (2.5).
Proof. Integrating of both sides of the whitenization differential Eq (3.1) in the interval [k−1,k] following as,
k∫k−1dx(1)(t)dtdt+ak∫k−1x(1)(t)dt=k∫k−1(b+ce−t)dt. | (3.5) |
Then,
x(1)(k)−x(1)(k−1)+ak∫k−1x(1)(t)dt=b+c(e−1)e−k | (3.6) |
By using the condition k∫k−1x(1)(t)dt=0.5(x(1)(k)+x(1)(k−1))=z(1)(k) and the Eq (3.3), the Eq (3.6) can be written as,
x(0)(k)+az(1)(k)=b+c(e−1)e−k. |
The solution of linear Eq (3.1) can be easily obtained as follows,
x(1)(k)=ba+ca−1e−t+de−at | (3.7) |
where d is integral constant. By using the initial condition x(1)(1)=x(0)(1), the constant d can be found as,
d=(x(0)(1)−ba−ca−1e−1)ea. |
Therefore the grey prediction model Eq (3.7) can be obtained as following
ˆx(1)(k)=(x(0)(1)−ba−ca−1e−1)ea(1−t)+ba+ca−1e−t . | (3.8) |
The response Eq (3.8) is used to compute the values of the series ˆx(1)(k), and the predicted values of the original series ˆx(0)(k) can be obtained as
ˆx(0)(k)=ˆx(1)(k)−ˆx(1)(k−1), k=2,3,...,n. | (3.9) |
The linear equations system (3.4) can be written as following
x(0)(2)=−az(1)(2)+b+c(e−1)e−2x(0)(3)=−az(1)(3)+b+c(e−1)e−3...x(0)(n)=−az(1)(n)+b+c(e−1)e−n | (3.10) |
or
Y=B ˆα | (3.11) |
where
B=[−z(1)(2)1(e−1)e−2−z(1)(3)1(e−1)e−3......−z(1)(n)1(e−1)e−n], Y=[x(0)(2)x(0)(3)..x(0)(n)], ˆα=[abc] | (3.12) |
in which n is the number of samples used to construct the model.
The parameters (a,b and c) estimation of the EXGM (1, 1) model can be easily obtained using a similar way of the GM (1, 1) model as follows
[a,b,c]T=(BTB)−1BTY. | (3.13) |
The flow mechanism of the EXGM (1, 1) is given in Figure 1.
Evaluative accuracy of the forecasting model
Relative percentage error (RPE) and mean absolute percentage error (MAPE) are used to evaluate the overall forecast performance of the prediction models. They are defined as follows:
RPE(k)=|ˆx(0)(k)−x(0)(k)x(0)(k)|×100% | (3.14) |
MAPE=1nn∑k=1RPE(k) | (3.15) |
where x(0)(k) is the original series, and ˆx(0)(k) is the predicted series. The accuracy evaluation is given in Table 1 [10].
MAPE (%) | Forecasting power |
≤10 | Excellent |
10−20 | Good |
20−50 | Reasonable |
50≤ | Incorrect |
Turkey's Ministry of Health reports complete, correct and official data about COVID-19 situation report of Turkey. The data includes the numbers of total COVID-19 cases, deaths, recovered patients. This section presents a systematic predicting methodology which includes data collection, parameter estimation, result analysis and prediction of near future COVID-19 situation for Turkey.
The modelling values and predicted values by EXGM (1, 1) are tabulated in Tables 2–7 and as shown in Figures 2–7. The forecast values obtained by EXGM (1, 1), GM (1, 1), ONGM (1, 1), TDPGM (1, 1) and the real value are shown in Tables 2–7, it shows that proposed EXGM (1, 1) forecasting model is better for forecasting of COVID-19 situation of Turkey, the precision of EXGM (1, 1) model is much better than the comparison models. The MAPE for the proposed predictions demonstrate the suitability of the proposed model for prediction.
Year | Actual Value | EXGM (1, 1) | Error (Rpe %) | GM(1, 1) | Error (Rpe %) | ONGM (1, 1) | Error (Rpe %) | TDPGM(1, 1) | Error (Rpe %) |
22–28 Jun 2020 | 198284 | 198284 | 0.00 | 198284 | 0.00 | 198284 | 0.00 | 198284 | 0.00 |
29 Jun–05 July 2020 | 206847 | 207395 | 0.27 | 206432 | 0.20 | 173403 | 16.17 | 205609 | 0.60 |
06–12 July 2020 | 214029 | 213612 | 0.19 | 213225 | 0.38 | 215708 | 0.78 | 210665 | 1.57 |
13–19 July 2020 | 220658 | 220122 | 0.24 | 220241 | 0.19 | 233470 | 5.81 | 215078 | 2.53 |
20–26 July 2020 | 227107 | 227261 | 0.07 | 227489 | 0.17 | 240927 | 6.09 | 218956 | 3.59 |
27 July–02 Aug 2020 | 233860 | 234791 | 0.40 | 234975 | 0.48 | 244058 | 4.36 | 222411 | 4.90 |
03–09 Aug 2020 | 241808 | 242629 | 0.34 | 242707 | 0.37 | 245373 | 1.47 | 225559 | 6.72 |
10–16 Aug 2020 | 250313 | 250751 | 0.17 | 250694 | 0.15 | 245925 | 1.75 | 228518 | 8.71 |
17–23 Aug 2020 | 259253 | 259152 | 0.04 | 258943 | 0.12 | 246157 | 5.05 | 237444 | 8.41 |
24–30 Aug 2020 | 268546 | 267837 | 0.26 | 267464 | 0.40 | 246254 | 8.30 | 239389 | 10.86 |
MAPE | 0.22 | 0.27 | 5.53 | 5.32 |
Week | Date | Forecasting values | Week | Date | Forecasting values |
W1 | 31 Aug–06 Sept 2020 | 276815 | W11 | 09–15 Nov 2020 | 384930 |
W2 | 07–13 Sept 2020 | 286094 | W12 | 16–22 Nov 2020 | 397833 |
W3 | 14-20 Sept 2020 | 295684 | W13 | 23–29 Nov 2020 | 411169 |
W4 | 21–27 Sept 2020 | 305595 | W14 | 30 Nov-06 Dec 2020 | 424952 |
W5 | 28 Sept–04 Oct 2020 | 315839 | W15 | 07–13 Dec 2020 | 439197 |
W6 | 05–11 Oct 2020 | 326426 | W16 | 14–20 Dec 2020 | 453919 |
W7 | 12–18 Oct 2020 | 337368 | W17 | 21–27 Dec 2020 | 469135 |
W8 | 19–25 Oct 2020 | 348677 | W18 | 28 Dec 2020–03 Jan 2021 | 484861 |
W9 | 26 Oct–01 Nov 2020 | 360365 | W19 | 04–10 Jan 2021 | 501114 |
W10 | 02–08 Nov 2020 | 372445 | W20 | 11–17 Jan 2021 | 517911 |
Date | Actual Value | EXGM (1, 1) | Error (Rpe %) | GM (1, 1) | Error (Rpe %) | ONGM (1, 1) | Error (Rpe %) | TDPGM (1, 1) | Error (Rpe %) |
22–28 Jun 2020 | 5097 | 5097 | 0.00 | 5097 | 0.00 | 5097 | 0.00 | 5097 | 0.00 |
29 Jun–05 July 2020 | 5225 | 5231 | 0.11 | 5230 | 0.10 | 4593 | 12.10 | 4518 | 13.53 |
06–12 July 2020 | 5363 | 5352 | 0.21 | 5351 | 0.22 | 5450 | 1.62 | 5066 | 5.54 |
13–19 July 2020 | 5491 | 5476 | 0.27 | 5475 | 0.29 | 5738 | 4.50 | 5498 | 0.13 |
20–26 July 2020 | 5613 | 5603 | 0.18 | 5602 | 0.20 | 5836 | 3.97 | 5541 | 1.28 |
27 July–02 Aug 2020 | 5728 | 5732 | 0.07 | 5732 | 0.07 | 5868 | 2.44 | 5860 | 2.30 |
03–09 Aug 2020 | 5844 | 5865 | 0.36 | 5865 | 0.36 | 5879 | 0.60 | 5901 | 0.98 |
10–16 Aug 2020 | 5974 | 6001 | 0.45 | 6001 | 0.45 | 5883 | 1.52 | 5913 | 1.02 |
17–23 Aug 2020 | 6121 | 6140 | 0.31 | 6140 | 0.31 | 5889 | 3.79 | 5919 | 3.30 |
24–30 Aug 2020 | 6326 | 6283 | 0.68 | 6282 | 0.70 | 5901 | 6.72 | 6028 | 4.71 |
MAPE | 0.29 | 0.30 | 4.14 | 3.64 |
Week | Date | Forecasting values | Week | Date | Forecasting values |
W1 | 31 Aug–06 Sept 2020 | 6428 | W1 | 09–15 Nov 2020 | 8083 |
W2 | 07–13 Sept 2020 | 6577 | W2 | 16–22 Nov 2020 | 8271 |
W3 | 14–20 Sept 2020 | 6729 | W3 | 23–29 Nov 2020 | 8462 |
W4 | 21–27 Sept 2020 | 6885 | W4 | 30 Nov–06 Dec 2020 | 8658 |
W5 | 28 Sept–04 Oct 2020 | 7045 | W5 | 07–13 Dec 2020 | 8859 |
W6 | 05–11 Oct 2020 | 7208 | W6 | 14–20 Dec 2020 | 9065 |
W7 | 12–18 Oct 2020 | 7375 | W7 | 21–27 Dec 2020 | 9275 |
W8 | 19–25 Oct 2020 | 7546 | W8 | 28 Dec 2020–03 Jan 2021 | 9490 |
W9 | 26 Oct–01 Nov 2020 | 7721 | W9 | 04–10 Jan 2021 | 9710 |
W10 | 02–08 Nov 2020 | 7900 | W10 | 11–17 Jan 2021 | 9935 |
Date | Actual Value | EXGM (1, 1) | Error (Rpe %) | GM (1, 1) | Error (Rpe %) | ONGM (1, 1) | Error (Rpe %) | TDPGM (1, 1) | Error (Rpe %) |
22–28 Jun 2020 | 170595 | 170595 | 0.00 | 170595 | 0.00 | 170595 | 0.00 | 170595 | 0.00 |
29 Jun–05 July 2020 | 180680 | 172009 | 0.11 | 186719 | 3.34 | 173341 | 4.06 | 176719 | 2.19 |
06–12 July 2020 | 194515 | 190440 | 0.21 | 193322 | 0.61 | 193390 | 0.58 | 187622 | 3.54 |
13–19 July 2020 | 202010 | 201084 | 0.27 | 200158 | 0.92 | 211869 | 4.88 | 205567 | 1.76 |
20–26 July 2020 | 209487 | 208979 | 0.18 | 207236 | 1.07 | 220393 | 5.21 | 211873 | 1.14 |
27 July–02 Aug 2020 | 216494 | 215985 | 0.07 | 214564 | 0.89 | 224325 | 3.62 | 219508 | 1.39 |
03–09 Aug 2020 | 223759 | 222788 | 0.36 | 222152 | 0.72 | 226139 | 1.06 | 227665 | 1.75 |
10–16 Aug 2020 | 230969 | 229645 | 0.45 | 230007 | 0.42 | 226975 | 1.73 | 229010 | 0.85 |
17–23 Aug 2020 | 237165 | 236654 | 0.31 | 238141 | 0.41 | 227361 | 4.13 | 233109 | 1.71 |
24–30 Aug 2020 | 243839 | 243855 | 0.68 | 246562 | 1.12 | 227539 | 6.68 | 236903 | 2.84 |
MAPE | 1.01 | 1.06 | 3.55 | 1.91 |
Week | Date | Forecasting values | Week | Date | Forecasting values |
W1 | 31 Aug–06 Sept 2020 | 251268 | W1 | 09–15 Nov 2020 | 338928 |
W2 | 07–13 Sept 2020 | 258902 | W2 | 16–22 Nov 2020 | 349224 |
W3 | 14–20 Sept 2020 | 266768 | W3 | 23–29 Nov 2020 | 359883 |
W4 | 21–27 Sept 2020 | 274872 | W4 | 30 Nov–06 Dec 2020 | 370764 |
W5 | 28 Sept–04 Oct 2020 | 283222 | W5 | 07–13 Dec 2020 | 382027 |
W6 | 05–11 Oct 2020 | 291826 | W6 | 14–20 Dec 2020 | 393632 |
W7 | 12–18 Oct 2020 | 300691 | W7 | 21–27 Dec 2020 | 405590 |
W8 | 19–25 Oct 2020 | 309826 | W8 | 28 Dec 2020–03 Jan 2021 | 417911 |
W9 | 26 Oct–01 Nov 2020 | 319238 | W9 | 04–10 Jan 2021 | 430606 |
W10 | 02–08 Nov 2020 | 328935 | W10 | 11–17 Jan 2021 | 443687 |
The data for COVID-19 cases of Turkey from 22 June 2020 to 31 August 2020 are applied to construct the grey model, while the data up to 17 January 2021 are used for prediction. The values are listed in Tables 3 and 4, indicating that the EXGM (1, 1) model outperforms the other models in this case. Figures 2 and 3 represent time series actual and forecasted data for COVID-19 cases of Turkey using the new proposed EXGM (1, 1) model. It is seen from the Figure 2 that the actual (black line) and forecasted (red line) data are matched to each other. Table 3 shows forecasted results using EXGM (1, 1). It is seen that trends of new COVID-19 cases will continue in the upcoming to 2021.
Tables 4 and 5 gives forecasted results using EXGM (1, 1) for deaths numbers. Actual and forecasted data for COVID-19 deaths of Turkey are shown in Figures 4 and 5.
The real and forecasted results for total number of COVID-19 recovered are given in Tables 6 and 7. Actual and forecasted data for COVID-19 recovered of Turkey are shown in Figures 6 and 7.
In this section a computer code for EXGM(1, 1) model is prepared by using Fortran. Certainly, this code can be modified to C++, Python or another compiler languages. Further predictions can be made with this solution mechanism for COVID-19. In addition, the solution mechanism in this code can be used the other grey models. The working mechanism of the program is as follows:
Steps Destination Named as
1 enter the raw data x0
2 build AGO sequence x1
3 build the mean generated sequence, z1
4 create B matrix BB
5 create Y matrix YY
6 generate the transpose of B matrix BT
7 multiply B and BT BC=BTB
8 build inverse of BC BCT
9 multiply BT and Y BTY
10 multiply BCT and BTY SON=(BTB)−1BTY
11 find the coefficients a,b,c,d SON(k,1)
12 write solution of whitezation diff. Eq. ˆx(1)(k) X1S
13 calculate the prediction values ˆx(0)(k) X0S
The computer code is given in appendix.
Since the traditional GM (1, 1) is one of the basic and most important grey prediction model, there are many scholars proposing new methods to improve the precision of the traditional GM (1, 1). Hence, an optimization for the traditional GM (1, 1) model has been developed in this study. The result of the numerical example indicated that the proposed improved grey prediction model aims to achieve effective performance for medium and short term predictions. The structural parameters (a, b and c) of the model can be dynamically adjusted according to the actual system. In addition, the traditional GM (1, 1) model can be considered as the special case of EXGM (1, 1) model (for c=0). The proposed EXGM (1, 1) model is suitable for predicting the data sequence with the characteristics of non-homogeneous exponential law. The EXGM (1, 1) model has achieved higher accuracy than the comparison models such as GM (1, 1), ONGM (1, 1), TDPGM (1, 1). However, they can all be employed for estimations.
Scientific simulation and accurate forecasting of future COVID-19 data is a crucial issue for environmental sciences, health sciences and government policy of a country. In this paper, a new exponential grey prediction model, namely EXGM (1, 1) model, has been proposed and applied in order to forecast future values of Turkey's COVID-19 situation by applying the grey modelling technique. Furthermore, Turkey's short-term COVID-19 situation has been predicted by the EXGM (1, 1). It is clear that further predictions (long-term) can be made with this solution mechanism for COVID-19 or another applications.These results provide the growth trend of the future COVID-19 cases of Turkey (if no vaccine for the virus is found or mutation is not seen), and also offer a guideline for policymaking and project planning. It is clear that EXGM (1, 1) can be practically used, with much better accuracy for COVID-19 data forecasting with the smallest MAPE than other models in this comparison.
Short-term daily or weekly estimates are important for making strategic decisions for the future days. Short-term forecasting can provide information to decision makers to schedule to prevent the spreading of COVID-19. Figure 2 and Table 3 show that if adequate precautions are not taken, the confirmed cases of COVID-19 in Turkey will continue to grow. The public health officials and government should take hard decisions to control the rapid increase of the COVID-19. Out sides of officials, the general public should keep social distancing, mask, hygiene and the other precautions to ensure their safety and prevent the spread of disease.
Finally, a new grey forecasting model is introduced and the proposed model was applied to forecast the number of confirmed COVID-19 cases, deaths and recovers. The results indicate that the introduced approach acts well in forecasting the future confirmed COVID-19 indicators. It is clear that, these forecastings can be further expanded for the future months and it can also be applied for estimating COVID-19 data of other countries or the other applications.
The proposed EXGM (1, 1) model play an important role in enriching the theoretical system of grey forecasting theory. However, the proposed EXGM (1, 1) model is only one variable grey prediction model. It is suitable for medium and short term prediction especially. For multi-variable and long term prediction, further research is needed in future. Some modifications such as optimization of the grey derivative, optimization of the background value will be focused mainly onto improve predictive accuracy of EXGM (1, 1) model in future work.
parameter (n = 12, m = 3)
doubleprecision x0, x1, z1, t, a, b, c, a1, a2, a3, a4, a5, c1, c2, c3, xxx, BB, yy, bt, bc, bct, bty, son, X1S, top, ff1, ff2, fonk, X0S
dimension x0(n), x1(n), z1(n), BB(n-1, m), YY(n-1, 1), BT(m, n-1), X1S(2*n), Bc(m, m), bct(m, m), bty(m, 1), son(m, 1), ff1(n), ff2(n), top(n), fonk(n), X0S(2*n)
open(2, file = 'exgm.txt')
! The raw data is entered
do 44 I = 1, n
44 read*, x0(I)
! The AGO is arranged
do 1 k = 1, n
do 2 I = 1, k
T = T+x0(I)
2 continue
x1(k) = T
T = 0
1 continue
! The sequence Z1 is generated
do 4 k = 2, n
4 z1(k) = (0.5d0)*(x1(k)+x1(k-1))
! The B matrix is created.
do 5 k = 1, n-1
BB(k, 1) = -z1(k+1)
5 continue
do 55 k = 1, n-1
BB(k, 2) = 1.d0
55 continue
do 56 k = 1, n-1
BB(k, 3) = BB(k, 3)+dexp(-0.5*k-0.5d0)
56 continue
! The Y matrix is created.
do 6 k = 1, n-1
YY(k, 1) = x0(k+1)
6 continue
! The transpose of the B matrix is created (BT).
do 8 I = 1, m
do 9 J = 1, n-1
BT(I, J) = BB(J, I)
9 continue
8 continue
! The matrix multiply of B and it's transpose is generated (BC).
do 10 i = 1, m
do 11 j = 1, m
BC(i, j) = 0
do 12 k = 1, n-1
BC(i, j) = BC(i, j)+BT(i, k)*BB(k, j)
12 continue
11 continue
10 continue
! The inverse of the BC matrix is calculated.
call inverse(bc, bct)
! The multiply of BT and Y matrix is calculated (BTY).
do 13 i = 1, m
do 14 j = 1, 1
BTY(i, j) = 0
do 15 k = 1, n-1
BTY(i, j) = BTY(i, j)+BT(i, k)*YY(k, j)
15 continue
14 continue
13 continue
! The matrix multiply of BCT and BTY is calculated (named SON)
to find of the coefficients a, b, c, d.
do 16 i = 1, m
do 17 j = 1, 1
son(i, j) = 0
do 18 k = 1, m
SON(i, j) = SON(i, j)+BCT(i, k)*BTY(k, j)
18 continue
17 continue
16 continue
! The coefficients a, b, c, d are writing.
do 19 k = 1, m
19 write(2, *) SON(k, 1)
! The solution of the whitenization
differential equations is, $ x^1(k) $, calculated (X1S).
do 24 k = 1, 14
X1S(k) = (x0(1)-SON(2, 1)/SON(1, 1)-
SON(m, 1)*dexp(-0.5d0)/(SON(1, 1)-0.5d0))*dexp(-SON(1, 1)*(k-1))+
(SON(m, 1)/(SON(1, 1)-0.5d0))*dexp(-k*0.5d0)+SON(2, 1)/SON(1, 1)
24 continue
! X1S(1) = x0(1)
! The prediction values is genereted and printed.
do 26 k = 2, 13
x0S(k) = X1S(k)-X1S(k-1)
write(2, *), k, X0S(k)
26 continue
end
! print the inverse matrix $ C = A^{-1} $
subroutine inverse(BC, BCT)
parameter (m = 3)
double precision BC(m, m), BCT(m, m)
double precision L7(m, m), U7(m, m), b7(m), d7(m), x7(m)
double precision coeff
integer i, j, k
! step 0: initialization for matrices L and U and b
L7 = 0.0
U7 = 0.0
b7 = 0.0
! step 1: forward elimination
do 1 k = 1, m-1
do 2 i = k+1, m
coeff = BC(i, k)/BC(k, k)
L7(i, k) = coeff
do 3 j = k+1, m
BC(i, j) = BC(i, j)-coeff*BC(k, j)
3 continue
2 continue
1 continue
! Step 2: prepare L and U matrices
! L matrix is a matrix of the elimination coefficient
! + the diagonal elements are 1.0
do 5 i = 1, m
L7(i, i) = 1.0
5 continue
! U matrix is the upper triangular part of A
do 7 j = 1, m
do 6 i = 1, j
U7(i, j) = BC(i, j)
6 continue
7 continue
! Step 3: compute columns of the inverse matrix C
do 8 k = 1, m
b7(k) = 1.0
d7(1) = b7(1)
! Step 3a: Solve Ld = b using the forward substitution
do 9 i = 2, m
d7(i) = b7(i)
do 10 j = 1, i-1
d7(i) = d7(i) - L7(i, j)*d7(j)
10 continue
9 continue
! Step 3b: Solve Ux = d using the back substitution
x7(m) = d7(m)/U7(m, m)
do 11 i = m-1, 1, -1
x7(i) = d7(i)
do 12 j = m, i+1, -1
x7(i) = x7(i)-U7(i, j)*x7(j)
12 continue
x7(i) = x7(i)/u7(i, i)
11 continue
! Step 3c: fill the solutions x(n) into column k of C
do 13 i = 1, m
BCT(i, k) = x7(i)
13 continue
b7(k) = 0.0
8 continue
return
end
The author declares no conflict of interest in this paper.
[1] |
J. L. Deng, Control problems of grey systems, Syst. Control Lett., 1 (1982), 288-294. doi: 10.1016/S0167-6911(82)80025-X
![]() |
[2] |
L. F. Wu, S. F. Liu, D. Chen, L. Yao, W. Cui, Using gray model with fractional order accumulation to predict gas emission, Nat. Hazards, 71 (2014), 2231-2236. doi: 10.1007/s11069-013-0960-z
![]() |
[3] | Y. G. Zhang, Y. Xu, Z. P. Wang, GM (1, 1) grey prediction of lorenz chaotic system, Chaos Solitons Fract., 42 (2009), 1003-1009. |
[4] | W. Zhou, J. M. He, Generalized GM (1, 1) model and its application in forecasting of fuel production, Appl. Math. Modell., 37 (2013), 6234-6243. |
[5] | S. A. Javed, S. Liu, Predicting the research output/growth of selected countries: application of even GM (1, 1) and ndgm models, Scientometrics, 115 (2018), 395-413. |
[6] |
S. L. Ou, Forecasting agricultural output with an improved grey forecasting model based on the genetic algorithml, Comput. Electron. Agric., 85 (2012), 33-39. doi: 10.1016/j.compag.2012.03.007
![]() |
[7] | B. Zeng, Y. Tan, H. Xu, J. Quan, L. Wang, X. Zhou, Forecasting the electricity consumption of commercial sector in hong kong using a novel grey dynamic prediction model, J. Grey Syst., 30 (2018), 157-172. |
[8] | S. Ene, N. Ozturk, Grey modelling based forecasting system for return flow of end-of-life vehicles, Technol. Forecast. Soc. Change, 117 (2018), 155-166. |
[9] | U. Şahin, T. Şahin, Forecasting the cumulative number of confirmed cases of COVID-19 in italy, uk and usa using fractional nonlinear grey bernoulli model, Chaos Solitons Fract., 138 (2020), 109948. |
[10] |
X. Ma, Z. B. Liu, Application of a novel time-delayed polynomial grey model to predict the natural gas consumption in china, J. Comput. Appl. Math., 324 (2017), 17-24. doi: 10.1016/j.cam.2017.04.020
![]() |
[11] |
J. Cui, S. Liu, B. Zeng, N. Xie, A novel grey forecasting model and its optimization, Appl. Math. Model., 37 (2013), 4399-4406. doi: 10.1016/j.apm.2012.09.052
![]() |
[12] |
X. Ma, Z. Liu, Y. Wang, Application of a novel nonlinear multivariate grey bernoulli model to predict the tourist income of china, J. Comput. Appl. Math., 347 (2019), 84-94. doi: 10.1016/j.cam.2018.07.044
![]() |
[13] | Z. X. Wang, Q. Li, L. L. Pei, A seasonal GM (1, 1) model for forecasting the electricity consumption of the primary economic sectors, Energy, 154 (2018), 522-534. |
[14] |
L. Z. Wu, S. H. Li, R. Q. Huang, Q. Xi, A new grey prediction model and its application to predicting landslide displacement, Appl. Soft Comput., 95 (2020), 106543. doi: 10.1016/j.asoc.2020.106543
![]() |
[15] |
L. Liu, L. Wu, Forecasting the renewable energy consumption of the european countries by an adjacent non-homogeneous grey model, Appl. Math. Modell., 89 (2021), 1932-1948. doi: 10.1016/j.apm.2020.08.080
![]() |
[16] |
L. Ye, N. Xie, A. Hu, A novel time-delay multivariate grey model for impact analysis of CO2 emissions from chinas transportation sectors, Appl. Math. Modell., 91 (2021), 493-507. doi: 10.1016/j.apm.2020.09.045
![]() |
[17] |
W. Wu, X. Ma, Y. Wang, W. Cai, B. Zeng, Predicting chinas energy consumption using a novel grey riccati model, Appl. Soft Comput., 95 (2020), 106555. doi: 10.1016/j.asoc.2020.106555
![]() |
[18] |
K. D. Yin, Y. Geng, X. M. Li, Improved grey prediction model based on exponential grey action quantity, J. Syst. Eng. Electron., 29 (2018), 560-570. doi: 10.21629/JSEE.2018.03.13
![]() |
[19] |
D. Akay, M. Atak, Grey prediction with rolling mechanism for electricity demand forecasting of Turkey, Energy, 32 (2007), 1670-1675. doi: 10.1016/j.energy.2006.11.014
![]() |
[20] |
J. Y. Chiang, C. K. Chen, Application of grey prediction to inverse nonlinear heat conduction problem, Int. J. Heat Mass Transfer, 51 (2008), 576-585. doi: 10.1016/j.ijheatmasstransfer.2007.05.015
![]() |
1. | Shalini Shekhawat, Akash Saxena, Ramadan A. Zeineldin, Ali Wagdy Mohamed, Prediction of Infectious Disease to Reduce the Computation Stress on Medical and Health Care Facilitators, 2023, 11, 2227-7390, 490, 10.3390/math11020490 | |
2. | Minfeng Wu, Wen Chen, Forecast of Electric Vehicle Sales in the World and China Based on PCA-GRNN, 2022, 14, 2071-1050, 2206, 10.3390/su14042206 | |
3. | Herojeet Rajkumar, Pradeep K. Naik, Gagandeep Singh, Madhuri Rishi, Hydrogeochemical characterization, multi-exposure deterministic and probabilistic health hazard evaluation in groundwater in parts of Northern India, 2023, 42, 1556-9543, 204, 10.1080/15569543.2022.2080222 | |
4. | Jiwu Li, Hongwei Yang, Xin Feng, Jing Zhang, 2021, ABGM(1,1): A Joint Optimization GM(1,1) Model Based on Background Values and Initial Conditions, 978-1-6654-1674-0, 378, 10.1109/EIECS53707.2021.9588158 | |
5. | Huiming Duan, Qiqi Zhou, A novel partial grey prediction model based on traffic flow wave equation and its application, 2024, 133, 09521976, 108142, 10.1016/j.engappai.2024.108142 | |
6. | Havisha Jahajeeah, Aslam A. E. F. Saib, Greymodels: A Shiny Package for Grey Forecasting Models in R, 2024, 0927-7099, 10.1007/s10614-024-10610-8 | |
7. | Atif Maqbool Khan, Artur Wyrwa, A Survey of Quantitative Techniques in Electricity Consumption—A Global Perspective, 2024, 17, 1996-1073, 4910, 10.3390/en17194910 | |
8. | Ümmügülsüm Erdinç, Halis Bilgil, ANALYZING COAL CONSUMPTION IN CHINA: FORECASTING WITH THE ECFGM(1, 1) MODEL AND A PERSPECTIVE ON THE FUTURE, 2024, 8, 2618-6594, 45, 10.34110/forecasting.1458131 | |
9. | Chuang Sheng, Jun Fu, HongChuan Qin, YanMin Zu, YeZhe Liang, ZhongHua Deng, Zhuo Wang, Xi Li, Short-term hybrid prognostics of fuel cells: A comparative and improvement study, 2024, 237, 09601481, 121742, 10.1016/j.renene.2024.121742 | |
10. | Changchun Li, Youjun Chen, Yanhui Xiang, An unbiased non-homogeneous grey forecasting model and its applications, 2025, 137, 0307904X, 115677, 10.1016/j.apm.2024.115677 | |
11. | Deniz YERİNDE, Merve ER, Compartmental Models of the COVID-19 Pandemic: A Systematic Literature Review, 2023, 6, 2667-579X, 254, 10.55117/bufbd.1395736 | |
12. | Zafer ÖZTÜRK, Halis BİLGİL, Ümmügülsüm ERDİNÇ, An optimized continuous fractional grey model for forecasting of the time dependent real world cases, 2022, 51, 2651-477X, 308, 10.15672/hujms.939543 | |
13. | Dipak W. Wajgi, Jitendra V. Tembhurne, Rakhi D. Wajgi, Distance-Based Localization in Wireless Sensor Network Using Exponential Grey Prediction Model, 2024, 19, 1548-0631, 1, 10.4018/IJBDCN.353393 | |
14. | ||
15. | Wanli Xie, Wen-Ze Wu, Chong Liu, Caixia Liu, Mingyong Pang, The general conformable fractional grey system model and its applications, 2024, 136, 09521976, 108817, 10.1016/j.engappai.2024.108817 | |
16. | Wanli Xie, Wen-Ze Wu, Zhenguo Xu, Caixia Liu, Keyun Zhao, The fractional neural grey system model and its application, 2023, 121, 0307904X, 43, 10.1016/j.apm.2023.04.028 | |
17. | Nenyasha Madyavanhu, Vaneet Kumar, Utilizing multi-population ant colony system and exponential grey prediction model for multi-objective virtual machine consolidation in Cloud Data Centers, 2024, 2511-2104, 10.1007/s41870-024-01949-0 | |
18. | Chukiat Chaiboonsri, The Potential Analytical Impact of Significant Sectoral Creative Economy on Thailand’s Economy: A Case Study of the IRS-CGE Model vs. the CRS-CGE Model for Both the National and Provincial Economies, 2024, 12, 2227-7099, 44, 10.3390/economies12020044 | |
19. | Pingping Xiong, Xiaojie Wu, Xiaosu Zeng, Lingshan Hu, Xue Yan, Covid-19 epidemic and regional carbon emissions: A study based on metabolic multivariate grey model with new information priority, 2023, 126, 09521976, 106820, 10.1016/j.engappai.2023.106820 | |
20. | Lalla Aicha Kone, Anna Ouskova Leonteva, Mamadou Tourad Diallo, Ahmedou Haouba, Pierre Collet, 2023, Chapter 6, 978-3-031-44354-1, 77, 10.1007/978-3-031-44355-8_6 | |
21. | Khisal Wijesinghe, Performance optimization through Hybrid Modelling: An application to Dengue Disease, 2024, 0, 2450-0054, 23, 10.5604/01.3001.0054.6286 | |
22. | Mehdi Abroun, Alireza Jahangiri, Ahmad Ghaderi Shamim, Hanif Heidari, Predicting long-term electricity prices using modified support vector regression method, 2024, 106, 0948-7921, 4103, 10.1007/s00202-023-02174-x | |
23. | Dewang Li, Daming Xu, Meilan Qiu, Shuiping Yang, Forecasting the public financial budget expenditure in Dongguan with an optimal weighted combination Markov model, 2023, 8, 2473-6988, 15600, 10.3934/math.2023796 | |
24. | En-Chih Chang, Rong-Ching Wu, Heidi H. Chang, Chun-An Cheng, A State-Feedback Control Strategy Based on Grey Fast Finite-Time Sliding Mode Control for an H-Bridge Inverter with LC Filter Output, 2024, 13, 2079-9292, 2118, 10.3390/electronics13112118 | |
25. | Qiqi Zhou, Huiming Duan, Derong Xie, A multivariate partial grey prediction model based on second-order traffic flow kinematics equation and its application, 2025, 463, 03770427, 116505, 10.1016/j.cam.2025.116505 | |
26. | ||
27. | Halis Bilgil, Ümmügülsüm Erdinç, China Total Energy Consumption Forecast with Optimized Continuous Conformable Fractional Grey Model, 2024, 12, 2148-2225, 157, 10.17093/alphanumeric.1447211 | |
28. | Muhammet Mesut Ertilav, Muhammet Burak Kılıç, Forecasting the Population of Türkiye Using Grey Models, 2024, 12, 2148-2225, 227, 10.17093/alphanumeric.1507101 | |
29. | ||
30. | ||
31. | ||
32. | ||
33. | ||
34. | ||
35. | ||
36. | Halis Bilgil, Simge Yüksel, Comparision of Conformable and Caputo fractional grey models, 2025, 03770427, 116500, 10.1016/j.cam.2025.116500 | |
37. | ||
38. | ||
39. | ||
40. | ||
41. | ||
42. | ||
43. | ||
44. | ||
45. | ||
46. | ||
47. | ||
48. | ||
49. | ||
50. | ||
51. | ||
52. | ||
53. | ||
54. | ||
55. | ||
56. | ||
57. | O. Ozan Evkaya, F. Sevinç Kurnaz, Ozancan Ozdemir, Pakize Yigit, Leveraging Ensemble and Hybrid Forecasting Tools to Increase Accuracy: Turkey COVID-19 Case Study, 2025, 6, 2661-8907, 10.1007/s42979-025-03658-2 | |
58. | ||
59. | ||
60. | ||
61. | ||
62. | ||
63. | ||
64. | ||
65. | Yu Chen, Huahan Zhang, Lingling Pei, The development trend of China’s marine economy: a predictive analysis based on industry level, 2025, 12, 2296-7745, 10.3389/fmars.2025.1544612 | |
66. | ||
67. | ||
68. | ||
69. | ||
70. | ||
71. | ||
72. | ||
73. | ||
74. | ||
75. | ||
76. | ||
77. | ||
78. | ||
79. | ||
80. | ||
81. | ||
82. | ||
83. | ||
84. | ||
85. | ||
86. | ||
87. | ||
88. | ||
89. | ||
90. | ||
91. | ||
92. | ||
93. | ||
94. | ||
95. | ||
96. | ||
97. | ||
98. | ||
99. | ||
100. | ||
101. | ||
102. | ||
103. | ||
104. | ||
105. | ||
106. | ||
107. | ||
108. | ||
109. | ||
110. | ||
111. | ||
112. | ||
113. | ||
114. | ||
115. | ||
116. | Hasnain Iftikhar, Faridoon Khan, Paulo Canas Rodrigues, Abdulmajeed Atiah Alharbi, Jeza Allohibi, Forecasting of Inflation Based on Univariate and Multivariate Time Series Models: An Empirical Application, 2025, 13, 2227-7390, 1121, 10.3390/math13071121 | |
117. | ||
118. | ||
119. | ||
120. | ||
121. | ||
122. | ||
123. | ||
124. | ||
125. | ||
126. | ||
127. | ||
128. | ||
129. | ||
130. |
MAPE (%) | Forecasting power |
≤10 | Excellent |
10−20 | Good |
20−50 | Reasonable |
50≤ | Incorrect |
Year | Actual Value | EXGM (1, 1) | Error (Rpe %) | GM(1, 1) | Error (Rpe %) | ONGM (1, 1) | Error (Rpe %) | TDPGM(1, 1) | Error (Rpe %) |
22–28 Jun 2020 | 198284 | 198284 | 0.00 | 198284 | 0.00 | 198284 | 0.00 | 198284 | 0.00 |
29 Jun–05 July 2020 | 206847 | 207395 | 0.27 | 206432 | 0.20 | 173403 | 16.17 | 205609 | 0.60 |
06–12 July 2020 | 214029 | 213612 | 0.19 | 213225 | 0.38 | 215708 | 0.78 | 210665 | 1.57 |
13–19 July 2020 | 220658 | 220122 | 0.24 | 220241 | 0.19 | 233470 | 5.81 | 215078 | 2.53 |
20–26 July 2020 | 227107 | 227261 | 0.07 | 227489 | 0.17 | 240927 | 6.09 | 218956 | 3.59 |
27 July–02 Aug 2020 | 233860 | 234791 | 0.40 | 234975 | 0.48 | 244058 | 4.36 | 222411 | 4.90 |
03–09 Aug 2020 | 241808 | 242629 | 0.34 | 242707 | 0.37 | 245373 | 1.47 | 225559 | 6.72 |
10–16 Aug 2020 | 250313 | 250751 | 0.17 | 250694 | 0.15 | 245925 | 1.75 | 228518 | 8.71 |
17–23 Aug 2020 | 259253 | 259152 | 0.04 | 258943 | 0.12 | 246157 | 5.05 | 237444 | 8.41 |
24–30 Aug 2020 | 268546 | 267837 | 0.26 | 267464 | 0.40 | 246254 | 8.30 | 239389 | 10.86 |
MAPE | 0.22 | 0.27 | 5.53 | 5.32 |
Week | Date | Forecasting values | Week | Date | Forecasting values |
W1 | 31 Aug–06 Sept 2020 | 276815 | W11 | 09–15 Nov 2020 | 384930 |
W2 | 07–13 Sept 2020 | 286094 | W12 | 16–22 Nov 2020 | 397833 |
W3 | 14-20 Sept 2020 | 295684 | W13 | 23–29 Nov 2020 | 411169 |
W4 | 21–27 Sept 2020 | 305595 | W14 | 30 Nov-06 Dec 2020 | 424952 |
W5 | 28 Sept–04 Oct 2020 | 315839 | W15 | 07–13 Dec 2020 | 439197 |
W6 | 05–11 Oct 2020 | 326426 | W16 | 14–20 Dec 2020 | 453919 |
W7 | 12–18 Oct 2020 | 337368 | W17 | 21–27 Dec 2020 | 469135 |
W8 | 19–25 Oct 2020 | 348677 | W18 | 28 Dec 2020–03 Jan 2021 | 484861 |
W9 | 26 Oct–01 Nov 2020 | 360365 | W19 | 04–10 Jan 2021 | 501114 |
W10 | 02–08 Nov 2020 | 372445 | W20 | 11–17 Jan 2021 | 517911 |
Date | Actual Value | EXGM (1, 1) | Error (Rpe %) | GM (1, 1) | Error (Rpe %) | ONGM (1, 1) | Error (Rpe %) | TDPGM (1, 1) | Error (Rpe %) |
22–28 Jun 2020 | 5097 | 5097 | 0.00 | 5097 | 0.00 | 5097 | 0.00 | 5097 | 0.00 |
29 Jun–05 July 2020 | 5225 | 5231 | 0.11 | 5230 | 0.10 | 4593 | 12.10 | 4518 | 13.53 |
06–12 July 2020 | 5363 | 5352 | 0.21 | 5351 | 0.22 | 5450 | 1.62 | 5066 | 5.54 |
13–19 July 2020 | 5491 | 5476 | 0.27 | 5475 | 0.29 | 5738 | 4.50 | 5498 | 0.13 |
20–26 July 2020 | 5613 | 5603 | 0.18 | 5602 | 0.20 | 5836 | 3.97 | 5541 | 1.28 |
27 July–02 Aug 2020 | 5728 | 5732 | 0.07 | 5732 | 0.07 | 5868 | 2.44 | 5860 | 2.30 |
03–09 Aug 2020 | 5844 | 5865 | 0.36 | 5865 | 0.36 | 5879 | 0.60 | 5901 | 0.98 |
10–16 Aug 2020 | 5974 | 6001 | 0.45 | 6001 | 0.45 | 5883 | 1.52 | 5913 | 1.02 |
17–23 Aug 2020 | 6121 | 6140 | 0.31 | 6140 | 0.31 | 5889 | 3.79 | 5919 | 3.30 |
24–30 Aug 2020 | 6326 | 6283 | 0.68 | 6282 | 0.70 | 5901 | 6.72 | 6028 | 4.71 |
MAPE | 0.29 | 0.30 | 4.14 | 3.64 |
Week | Date | Forecasting values | Week | Date | Forecasting values |
W1 | 31 Aug–06 Sept 2020 | 6428 | W1 | 09–15 Nov 2020 | 8083 |
W2 | 07–13 Sept 2020 | 6577 | W2 | 16–22 Nov 2020 | 8271 |
W3 | 14–20 Sept 2020 | 6729 | W3 | 23–29 Nov 2020 | 8462 |
W4 | 21–27 Sept 2020 | 6885 | W4 | 30 Nov–06 Dec 2020 | 8658 |
W5 | 28 Sept–04 Oct 2020 | 7045 | W5 | 07–13 Dec 2020 | 8859 |
W6 | 05–11 Oct 2020 | 7208 | W6 | 14–20 Dec 2020 | 9065 |
W7 | 12–18 Oct 2020 | 7375 | W7 | 21–27 Dec 2020 | 9275 |
W8 | 19–25 Oct 2020 | 7546 | W8 | 28 Dec 2020–03 Jan 2021 | 9490 |
W9 | 26 Oct–01 Nov 2020 | 7721 | W9 | 04–10 Jan 2021 | 9710 |
W10 | 02–08 Nov 2020 | 7900 | W10 | 11–17 Jan 2021 | 9935 |
Date | Actual Value | EXGM (1, 1) | Error (Rpe %) | GM (1, 1) | Error (Rpe %) | ONGM (1, 1) | Error (Rpe %) | TDPGM (1, 1) | Error (Rpe %) |
22–28 Jun 2020 | 170595 | 170595 | 0.00 | 170595 | 0.00 | 170595 | 0.00 | 170595 | 0.00 |
29 Jun–05 July 2020 | 180680 | 172009 | 0.11 | 186719 | 3.34 | 173341 | 4.06 | 176719 | 2.19 |
06–12 July 2020 | 194515 | 190440 | 0.21 | 193322 | 0.61 | 193390 | 0.58 | 187622 | 3.54 |
13–19 July 2020 | 202010 | 201084 | 0.27 | 200158 | 0.92 | 211869 | 4.88 | 205567 | 1.76 |
20–26 July 2020 | 209487 | 208979 | 0.18 | 207236 | 1.07 | 220393 | 5.21 | 211873 | 1.14 |
27 July–02 Aug 2020 | 216494 | 215985 | 0.07 | 214564 | 0.89 | 224325 | 3.62 | 219508 | 1.39 |
03–09 Aug 2020 | 223759 | 222788 | 0.36 | 222152 | 0.72 | 226139 | 1.06 | 227665 | 1.75 |
10–16 Aug 2020 | 230969 | 229645 | 0.45 | 230007 | 0.42 | 226975 | 1.73 | 229010 | 0.85 |
17–23 Aug 2020 | 237165 | 236654 | 0.31 | 238141 | 0.41 | 227361 | 4.13 | 233109 | 1.71 |
24–30 Aug 2020 | 243839 | 243855 | 0.68 | 246562 | 1.12 | 227539 | 6.68 | 236903 | 2.84 |
MAPE | 1.01 | 1.06 | 3.55 | 1.91 |
Week | Date | Forecasting values | Week | Date | Forecasting values |
W1 | 31 Aug–06 Sept 2020 | 251268 | W1 | 09–15 Nov 2020 | 338928 |
W2 | 07–13 Sept 2020 | 258902 | W2 | 16–22 Nov 2020 | 349224 |
W3 | 14–20 Sept 2020 | 266768 | W3 | 23–29 Nov 2020 | 359883 |
W4 | 21–27 Sept 2020 | 274872 | W4 | 30 Nov–06 Dec 2020 | 370764 |
W5 | 28 Sept–04 Oct 2020 | 283222 | W5 | 07–13 Dec 2020 | 382027 |
W6 | 05–11 Oct 2020 | 291826 | W6 | 14–20 Dec 2020 | 393632 |
W7 | 12–18 Oct 2020 | 300691 | W7 | 21–27 Dec 2020 | 405590 |
W8 | 19–25 Oct 2020 | 309826 | W8 | 28 Dec 2020–03 Jan 2021 | 417911 |
W9 | 26 Oct–01 Nov 2020 | 319238 | W9 | 04–10 Jan 2021 | 430606 |
W10 | 02–08 Nov 2020 | 328935 | W10 | 11–17 Jan 2021 | 443687 |
MAPE (%) | Forecasting power |
≤10 | Excellent |
10−20 | Good |
20−50 | Reasonable |
50≤ | Incorrect |
Year | Actual Value | EXGM (1, 1) | Error (Rpe %) | GM(1, 1) | Error (Rpe %) | ONGM (1, 1) | Error (Rpe %) | TDPGM(1, 1) | Error (Rpe %) |
22–28 Jun 2020 | 198284 | 198284 | 0.00 | 198284 | 0.00 | 198284 | 0.00 | 198284 | 0.00 |
29 Jun–05 July 2020 | 206847 | 207395 | 0.27 | 206432 | 0.20 | 173403 | 16.17 | 205609 | 0.60 |
06–12 July 2020 | 214029 | 213612 | 0.19 | 213225 | 0.38 | 215708 | 0.78 | 210665 | 1.57 |
13–19 July 2020 | 220658 | 220122 | 0.24 | 220241 | 0.19 | 233470 | 5.81 | 215078 | 2.53 |
20–26 July 2020 | 227107 | 227261 | 0.07 | 227489 | 0.17 | 240927 | 6.09 | 218956 | 3.59 |
27 July–02 Aug 2020 | 233860 | 234791 | 0.40 | 234975 | 0.48 | 244058 | 4.36 | 222411 | 4.90 |
03–09 Aug 2020 | 241808 | 242629 | 0.34 | 242707 | 0.37 | 245373 | 1.47 | 225559 | 6.72 |
10–16 Aug 2020 | 250313 | 250751 | 0.17 | 250694 | 0.15 | 245925 | 1.75 | 228518 | 8.71 |
17–23 Aug 2020 | 259253 | 259152 | 0.04 | 258943 | 0.12 | 246157 | 5.05 | 237444 | 8.41 |
24–30 Aug 2020 | 268546 | 267837 | 0.26 | 267464 | 0.40 | 246254 | 8.30 | 239389 | 10.86 |
MAPE | 0.22 | 0.27 | 5.53 | 5.32 |
Week | Date | Forecasting values | Week | Date | Forecasting values |
W1 | 31 Aug–06 Sept 2020 | 276815 | W11 | 09–15 Nov 2020 | 384930 |
W2 | 07–13 Sept 2020 | 286094 | W12 | 16–22 Nov 2020 | 397833 |
W3 | 14-20 Sept 2020 | 295684 | W13 | 23–29 Nov 2020 | 411169 |
W4 | 21–27 Sept 2020 | 305595 | W14 | 30 Nov-06 Dec 2020 | 424952 |
W5 | 28 Sept–04 Oct 2020 | 315839 | W15 | 07–13 Dec 2020 | 439197 |
W6 | 05–11 Oct 2020 | 326426 | W16 | 14–20 Dec 2020 | 453919 |
W7 | 12–18 Oct 2020 | 337368 | W17 | 21–27 Dec 2020 | 469135 |
W8 | 19–25 Oct 2020 | 348677 | W18 | 28 Dec 2020–03 Jan 2021 | 484861 |
W9 | 26 Oct–01 Nov 2020 | 360365 | W19 | 04–10 Jan 2021 | 501114 |
W10 | 02–08 Nov 2020 | 372445 | W20 | 11–17 Jan 2021 | 517911 |
Date | Actual Value | EXGM (1, 1) | Error (Rpe %) | GM (1, 1) | Error (Rpe %) | ONGM (1, 1) | Error (Rpe %) | TDPGM (1, 1) | Error (Rpe %) |
22–28 Jun 2020 | 5097 | 5097 | 0.00 | 5097 | 0.00 | 5097 | 0.00 | 5097 | 0.00 |
29 Jun–05 July 2020 | 5225 | 5231 | 0.11 | 5230 | 0.10 | 4593 | 12.10 | 4518 | 13.53 |
06–12 July 2020 | 5363 | 5352 | 0.21 | 5351 | 0.22 | 5450 | 1.62 | 5066 | 5.54 |
13–19 July 2020 | 5491 | 5476 | 0.27 | 5475 | 0.29 | 5738 | 4.50 | 5498 | 0.13 |
20–26 July 2020 | 5613 | 5603 | 0.18 | 5602 | 0.20 | 5836 | 3.97 | 5541 | 1.28 |
27 July–02 Aug 2020 | 5728 | 5732 | 0.07 | 5732 | 0.07 | 5868 | 2.44 | 5860 | 2.30 |
03–09 Aug 2020 | 5844 | 5865 | 0.36 | 5865 | 0.36 | 5879 | 0.60 | 5901 | 0.98 |
10–16 Aug 2020 | 5974 | 6001 | 0.45 | 6001 | 0.45 | 5883 | 1.52 | 5913 | 1.02 |
17–23 Aug 2020 | 6121 | 6140 | 0.31 | 6140 | 0.31 | 5889 | 3.79 | 5919 | 3.30 |
24–30 Aug 2020 | 6326 | 6283 | 0.68 | 6282 | 0.70 | 5901 | 6.72 | 6028 | 4.71 |
MAPE | 0.29 | 0.30 | 4.14 | 3.64 |
Week | Date | Forecasting values | Week | Date | Forecasting values |
W1 | 31 Aug–06 Sept 2020 | 6428 | W1 | 09–15 Nov 2020 | 8083 |
W2 | 07–13 Sept 2020 | 6577 | W2 | 16–22 Nov 2020 | 8271 |
W3 | 14–20 Sept 2020 | 6729 | W3 | 23–29 Nov 2020 | 8462 |
W4 | 21–27 Sept 2020 | 6885 | W4 | 30 Nov–06 Dec 2020 | 8658 |
W5 | 28 Sept–04 Oct 2020 | 7045 | W5 | 07–13 Dec 2020 | 8859 |
W6 | 05–11 Oct 2020 | 7208 | W6 | 14–20 Dec 2020 | 9065 |
W7 | 12–18 Oct 2020 | 7375 | W7 | 21–27 Dec 2020 | 9275 |
W8 | 19–25 Oct 2020 | 7546 | W8 | 28 Dec 2020–03 Jan 2021 | 9490 |
W9 | 26 Oct–01 Nov 2020 | 7721 | W9 | 04–10 Jan 2021 | 9710 |
W10 | 02–08 Nov 2020 | 7900 | W10 | 11–17 Jan 2021 | 9935 |
Date | Actual Value | EXGM (1, 1) | Error (Rpe %) | GM (1, 1) | Error (Rpe %) | ONGM (1, 1) | Error (Rpe %) | TDPGM (1, 1) | Error (Rpe %) |
22–28 Jun 2020 | 170595 | 170595 | 0.00 | 170595 | 0.00 | 170595 | 0.00 | 170595 | 0.00 |
29 Jun–05 July 2020 | 180680 | 172009 | 0.11 | 186719 | 3.34 | 173341 | 4.06 | 176719 | 2.19 |
06–12 July 2020 | 194515 | 190440 | 0.21 | 193322 | 0.61 | 193390 | 0.58 | 187622 | 3.54 |
13–19 July 2020 | 202010 | 201084 | 0.27 | 200158 | 0.92 | 211869 | 4.88 | 205567 | 1.76 |
20–26 July 2020 | 209487 | 208979 | 0.18 | 207236 | 1.07 | 220393 | 5.21 | 211873 | 1.14 |
27 July–02 Aug 2020 | 216494 | 215985 | 0.07 | 214564 | 0.89 | 224325 | 3.62 | 219508 | 1.39 |
03–09 Aug 2020 | 223759 | 222788 | 0.36 | 222152 | 0.72 | 226139 | 1.06 | 227665 | 1.75 |
10–16 Aug 2020 | 230969 | 229645 | 0.45 | 230007 | 0.42 | 226975 | 1.73 | 229010 | 0.85 |
17–23 Aug 2020 | 237165 | 236654 | 0.31 | 238141 | 0.41 | 227361 | 4.13 | 233109 | 1.71 |
24–30 Aug 2020 | 243839 | 243855 | 0.68 | 246562 | 1.12 | 227539 | 6.68 | 236903 | 2.84 |
MAPE | 1.01 | 1.06 | 3.55 | 1.91 |
Week | Date | Forecasting values | Week | Date | Forecasting values |
W1 | 31 Aug–06 Sept 2020 | 251268 | W1 | 09–15 Nov 2020 | 338928 |
W2 | 07–13 Sept 2020 | 258902 | W2 | 16–22 Nov 2020 | 349224 |
W3 | 14–20 Sept 2020 | 266768 | W3 | 23–29 Nov 2020 | 359883 |
W4 | 21–27 Sept 2020 | 274872 | W4 | 30 Nov–06 Dec 2020 | 370764 |
W5 | 28 Sept–04 Oct 2020 | 283222 | W5 | 07–13 Dec 2020 | 382027 |
W6 | 05–11 Oct 2020 | 291826 | W6 | 14–20 Dec 2020 | 393632 |
W7 | 12–18 Oct 2020 | 300691 | W7 | 21–27 Dec 2020 | 405590 |
W8 | 19–25 Oct 2020 | 309826 | W8 | 28 Dec 2020–03 Jan 2021 | 417911 |
W9 | 26 Oct–01 Nov 2020 | 319238 | W9 | 04–10 Jan 2021 | 430606 |
W10 | 02–08 Nov 2020 | 328935 | W10 | 11–17 Jan 2021 | 443687 |