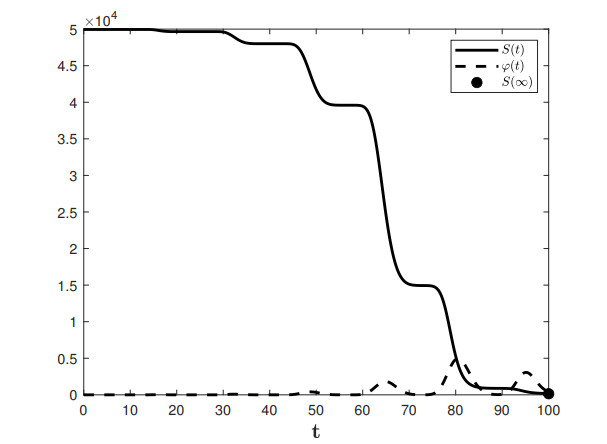
An accurate hysteresis model is fundamental to well capture the non-linearity phenomena occurring in structural and non-structural elements in building structures, that are usually made of reinforced concrete or steel materials. In this sense, this paper aims to numerically estimate through simplified non-linear analyses, the cyclic response of a reinforced concrete frame using different hysteretic models present in the literature. A commercial Finite Element Method package is used to carry out most of the simulations using polygonal hysteretic models and a fiber model, and additionally, a MATLAB script is developed to use a smooth hysteresis model. The experimental data is based on the experiments carried out in the Laboratório Nacional de Engenharia Civil, Portugal. The numerical outcomes are further compared with the experimental result to evaluate the accuracy of the simplified analysis based on the lumped plasticity or plastic hinge method for the reinforced concrete bare frame. Results show that the tetralinear Takeda's model fits closely the experimental hysteresis loops. The fiber model can well capture the hysteresis behavior, though it requires knowledge and expertise on parameter calibration. Sivaselvan and Reinhorn's smooth hysteresis model was able to satisfactorily reproduce the actual non-linear cyclic behavior of the RC frame structure in a global way.
Citation: Pedro Folhento, Manuel Braz-César, Rui Barros. Cyclic response of a reinforced concrete frame: Comparison of experimental results with different hysteretic models[J]. AIMS Materials Science, 2021, 8(6): 917-931. doi: 10.3934/matersci.2021056
[1] | Colin Klaus, Matthew Wascher, Wasiur R. KhudaBukhsh, Grzegorz A. Rempała . Likelihood-Free Dynamical Survival Analysis applied to the COVID-19 epidemic in Ohio. Mathematical Biosciences and Engineering, 2023, 20(2): 4103-4127. doi: 10.3934/mbe.2023192 |
[2] | Marcin Choiński, Mariusz Bodzioch, Urszula Foryś . A non-standard discretized SIS model of epidemics. Mathematical Biosciences and Engineering, 2022, 19(1): 115-133. doi: 10.3934/mbe.2022006 |
[3] | Maghnia Hamou Maamar, Matthias Ehrhardt, Louiza Tabharit . A nonstandard finite difference scheme for a time-fractional model of Zika virus transmission. Mathematical Biosciences and Engineering, 2024, 21(1): 924-962. doi: 10.3934/mbe.2024039 |
[4] | Yong Yang, Zunxian Li, Chengyi Xia . Forced waves and their asymptotic behaviors in a Lotka-Volterra competition model with spatio-temporal nonlocal effect under climate change. Mathematical Biosciences and Engineering, 2023, 20(8): 13638-13659. doi: 10.3934/mbe.2023608 |
[5] | Sai Zhang, Li Tang, Yan-Jun Liu . Formation deployment control of multi-agent systems modeled with PDE. Mathematical Biosciences and Engineering, 2022, 19(12): 13541-13559. doi: 10.3934/mbe.2022632 |
[6] | A Othman Almatroud, Noureddine Djenina, Adel Ouannas, Giuseppe Grassi, M Mossa Al-sawalha . A novel discrete-time COVID-19 epidemic model including the compartment of vaccinated individuals. Mathematical Biosciences and Engineering, 2022, 19(12): 12387-12404. doi: 10.3934/mbe.2022578 |
[7] | Jianquan Li, Zhien Ma, Fred Brauer . Global analysis of discrete-time SI and SIS epidemic models. Mathematical Biosciences and Engineering, 2007, 4(4): 699-710. doi: 10.3934/mbe.2007.4.699 |
[8] | Ziqiang Cheng, Jin Wang . Modeling epidemic flow with fluid dynamics. Mathematical Biosciences and Engineering, 2022, 19(8): 8334-8360. doi: 10.3934/mbe.2022388 |
[9] | Dimitri Breda, Davide Liessi . A practical approach to computing Lyapunov exponents of renewal and delay equations. Mathematical Biosciences and Engineering, 2024, 21(1): 1249-1269. doi: 10.3934/mbe.2024053 |
[10] | Sarah Treibert, Helmut Brunner, Matthias Ehrhardt . A nonstandard finite difference scheme for the SVICDR model to predict COVID-19 dynamics. Mathematical Biosciences and Engineering, 2022, 19(2): 1213-1238. doi: 10.3934/mbe.2022056 |
An accurate hysteresis model is fundamental to well capture the non-linearity phenomena occurring in structural and non-structural elements in building structures, that are usually made of reinforced concrete or steel materials. In this sense, this paper aims to numerically estimate through simplified non-linear analyses, the cyclic response of a reinforced concrete frame using different hysteretic models present in the literature. A commercial Finite Element Method package is used to carry out most of the simulations using polygonal hysteretic models and a fiber model, and additionally, a MATLAB script is developed to use a smooth hysteresis model. The experimental data is based on the experiments carried out in the Laboratório Nacional de Engenharia Civil, Portugal. The numerical outcomes are further compared with the experimental result to evaluate the accuracy of the simplified analysis based on the lumped plasticity or plastic hinge method for the reinforced concrete bare frame. Results show that the tetralinear Takeda's model fits closely the experimental hysteresis loops. The fiber model can well capture the hysteresis behavior, though it requires knowledge and expertise on parameter calibration. Sivaselvan and Reinhorn's smooth hysteresis model was able to satisfactorily reproduce the actual non-linear cyclic behavior of the RC frame structure in a global way.
The analysis of the dynamical behaviour of numerical methods for differential and integral equation epidemic models has been a topic of interest [1,2,3,4,5,6,7,8], because it satisfies the need to have robust numerical methods that share the same properties of the analytical solution. While most of the existing papers are devoted to the simulation of specific models, here we consider the following general problem, which consists of a Volterra integro-differential system of 2M+1 equations of the type
S′i(t)=−βiSi(t)Vi(t),φi(t)=φi0(t)+βi∫t0Ai(t−τ)Si(τ)Vi(τ) dτ,P(t)=P0(t)+∫t0B(t−τ)M∑r=1crφr(τ) dτ, | (1.1) |
where t≥0, Vi(t)=∑Mr=1βirφr(t)+αiP(t), and i=1,…,M. Here φi0(t), Ai(t), i=1,…,M, P0(t) and B(t) are given continuous functions and αi,βi,ci,βir≥0, i,r=1,…,M, are given constants. At time t=0 the value of Si(t), i=1,…,M, is S0i, given. The motivation for considering system (1.1) is that it represents a general framework that includes a variety of epidemic mathematical models that, taking into account the age of infection, involve memory terms. We report some examples where we give the biological definitions of the variables and parameters.
I. An age-of-infection epidemic model is described in [9,p.139] by the following system
S′(t)=−βS(t)φ(t),φ(t)=φ0(t)+β∫t0A(t−τ)S(τ)φ(τ) dτ, | (1.2) |
S(t) is the number of susceptibles and φ(t) is the total infectivity at time t, β>0 is the rate of effective contacts and A(τ) is the mean infectivity of members of population with infection age τ. Moreover, φ0(t) represents the infectivity at time t of people who were infected before the initial outbreak. The general model (1.1) reduces to (1.2), with M=1, α1=0, β11=1, β1=β, c1=0, and B(t)=P0(t)=0, for t≥0.
II. A model with both symptomatic and asymptomatic infections is described in [10], by the following system
S′(t)=−aNS(t)(φs(t)+φa(t)),φs(t)=φs0(t)+aN∫t0f(t−τ)As(t−τ)S(τ)(φs(τ)+φa(τ)) dτ,φa(t)=φa0(t)+aN∫t0(1−f(t−τ))Aa(t−τ)S(τ)(φs(τ)+φa(τ)) dτ. | (1.3) |
Model (1.3) traces the evolution of the epidemics by distinguishing the infectivity functions of symptomatic and asymptomatic people, being φs(t) the former and φa(t) the latter. Thus, an analogous distinction is made for the known functions φs0(t) and φa0(t), which describe the contribution to the total infectivity of people who got infected before the initial time. Here, N>0 is the total size of the population, a>0 is the average number of contacts made by a member per unit of time and f(t)∈(0,1) is the probability for an individual to become symptomatic after the infection. Furthermore, As(τ) and Aa(τ) represent the mean infectivity of symptomatic and asymptomatic individuals with infection age τ, respectively. System (1.3) corresponds to the general model (1.1) with M=2, αi=0, βij=1, βi=aN, ci=0, for i,j=1,2 and S1(t)=S2(t)=S(t), φ1(t)=φs(t), φ2(t)=φa(t), A1(t)=f(t)As(t), A2(t)=(1−f(t))Aa(t) and B(t)=P0(t)=0, for t≥0.
III. An age of infection epidemic model in a multi-group heterogeneous populations is proposed in [11]. The system, when the state space is discrete and the population is divided into M subgroups of sizes N1,…,NM, reads
S′i(t)=−aiSi(t)M∑j=1pijφj(t)Nj,φi(t)=φi0(t)+ai∫t0Ai(t−τ)Si(τ)M∑j=1pijφj(τ)Nj dτ, | (1.4) |
i=1,…,M. Here, in group i, Si(t) represents the number of susceptible members and φi(t) is the infectivity at time t, φi0(t) is the infectivity at time t of members who were infected before time 0, and Ai(τ) is the mean infectivity of individuals of group i with infection age τ. Furthermore, ai≥0 is the contact rate for members of group i, and pij is the fraction of contacts made by a member of group i with a member of group j. Referring to the notations in [11,12], the general model (1.1) reduces to (1.4), with αi=0, βij=pijNj, βi=ai, ci=0, for i,j=1,…,M and B(t)=P0(t)=0, for t≥0.
IV. In [13] the virus shedding epidemic model is studied
S′i(t)=−βiSi(t)P(t),φi(t)=φi0(t)+βi∫t0Ai(t−τ)Si(τ)P(τ) dτ,P(t)=P0(t)+∫t0Γ(t−τ)(r1φ1(τ)+r2φ2(τ)) dτ, | (1.5) |
i=1,2, where P(t) is the pathogen shed by infected individuals of each group at a rate r1 and r2, respectively. Here, βi, i=1,2, are the contact rates, A1(τ) and A2(τ) are the mean infectivity of individuals in group 1 and 2 at age of infection τ and Γ(τ) is the fraction of pathogen remaining τ time units after having been shed by an infectious individual (see also [14,p. 168] in case of homogeneous mixing). The general model (1.1) reduces to (1.5), with M=2, αi=1, βij=0, ci=ri, i,j=1,2 and B(t)=Γ(t), for t≥0.
Since numerical simulations are of fundamental importance in the process of understanding the dynamic and the asymptotic behaviour of the system, we focus on the construction of a numerical model that preserves the global properties of the continuous problem. In the literature, there have been several investigations looking at numerical approximations of integral and integro-differential equations whose solutions remain bounded or converge to zero, including [15,16,17,18,19,20,21]. Here we concentrate on the general system (1.1) where, under suitable assumptions on the known parameters, Si(t) tends, as t→+∞, to a limit Si(∞), which is the solution of a known nonlinear limiting equation. In case of the epidemic models listed above and in many other cases (see for example [22,23] and references therein), Si(∞) is the final size, one of the most relevant parameters in the description of the epidemic. We focus on a numerical method for (1.1) and we are interested to prove that, for any positive value of the size h of the discretization, the limit of the numerical approximation, S∞i(h), exists and turns out to be the solution of an analogous nonlinear limiting equation. Furthermore, we show that S∞i(h) tends to Si(∞), as h vanishes. The convergence as h→0 is an obvious property that a numerical method must satisfy while integrating over a limited range, but it is not at all guaranteed and, in general, difficult to prove for the asymptotic solution.
Then, an important feature of our investigation is that it directly shows, under suitable conditions on the integro-differential system, that the discrete model has properties which ensure its solutions to behave asymptotically as the continuous ones. The numerical investigation is performed on a general discrete system obtained approximating (1.1) by non-standard finite differences. Then, the stability analysis, consisting in a comparative study of the behaviour of the analytical and the numerical solutions, is carried out in significant cases (that is (1.2)–(1.5)) and can be extended to other models, that fall into the form (1.1).
In this viewpoint, we say that the numerical scheme is coherent with the continuous problem and system (1.1) can be considered as a test problem for proving such coherence.
Here we assume that the given functions in (1.1) belong to C[0,+∞)∩L1[0,+∞), and that:
Assumptions A for i=1,…,M:
● αi≥0,βir≥0,r=1,…,M,
● S0i=Si(0)>0,φi0(t)≥0,Ai(t)≥0,t≥0,
and
● P0(t)≥0,B(t)≥0,t≥0.
Assumptions B there exist positive constants φ0,max, P0,max, Amax, and ˉB such that:
● φ0(t)≤φ0,max,P0(t)≤P0,max, t≥0,
● Ai(t)≤Amax, i=1,…,M, t≥0,
● h∑∞n=0B(nh)≤ˉB, h>0.
Assumptions C there exist positive constants ˉA, ˉφ0, ˉP0 such that for h>0:
● h∑+∞n=0Ai(nh)≤ˉA,i=1,…,M,
● h∑+∞n=0φ0i(nh)≤ˉφ0,i=1,…,M,
● h∑+∞n=0P0(nh)≤ˉP0.
Observe that the third of Assumptions B and the Assumptions C have the form h∑+∞n=0Q(nh)≤ˉQ, h>0, with Q∈L1[0,+∞) and ˉQ>0, which is certainly accomplished when, for example, the function Q′(t)∈L1[0,+∞) (see [19]) or when (usually true in realistic situations) Q(t) is definitely non-increasing.
A generalization to the results in [9,13,14] implies that:
● under the Assumptions A, Si(t) is positive and non-increasing, φi(t)≥0, for i=1,…,M, and P(t)≥0;
● if in addition Assumptions B hold, then Si(t), φi(t), i=1,…,M and P(t) are bounded;
● limt→+∞φi(t)=0 and limt→+∞Si(t)=Si(∞), where Si(∞) satisfies the limiting algebraic system Ri(x)=0, i=1,…,M, where
Ri(x)=log(S0ixi)−βiαi∫+∞0P0(t) dt−βiM∑r=1(βir+αicr∫+∞0B(t) dt)⋅(S0r(1−xrS0r)∫+∞0Ar(t) dt+∫+∞0φ0r(t) dt)=0, | (1.6) |
with x=(x1,…,xM), if a solution to (1.6) exists.
The paper is organized as follows. In the next section we present a numerical method based on a nonlocal discretization to solve the general system (1.1) and we prove the basic non-negativity and boundedness properties of the numerical solution under the Assumptions A and B on the known functions. These results hold for any value of the size h>0 of the discretization. In Section 3 we give some preliminary results that are used in Section 4, where the numerical asymptotic solution is shown to be the root of a nonlinear system of algebraic equations. Here we prove that this solution is unique and that it converges to its analytical counterpart as the stepsize h→0. In Section 5 we present some cases of interest in epidemic models and apply the theory developed in the previous sections in order to study the coherence of the numerical method with the epidemic models. Furthermore, we show the results of some simulations performed. Finally, in Section 6 we conclude the paper with some remarks.
Define a stepsize h>0, and an uniform mesh {tn=nh,n=0,1,…}. Our numerical approach to solve the nonlinear system (1.1) is the following finite difference scheme
Sn+1i=Sni−hβiSn+1iVni,φn+1i=φi0(tn+1)+hβin∑j=0Ai(tn+1−j)Sj+1iVji,Pn+1=P0(tn+1)+hn∑j=0B(tn+1−j)M∑r=1crφjr, | (2.1) |
n=0,1,…, where Vni=∑Mr=1βirφnr+αiPn, and i=1,…,M. Here S0i=Si(0), φ0i=φi0(0), and P0=P0(0) are given. Furthermore, Sni≈Si(tn), φni≈φi(tn), for i=1,…,M, and Pn≈P(tn), for n=0,1,…. We say that the method (2.1) is non-standard since the nonlinear terms in (1.1) are approximated in a nonlocal way. A pseudocode implementation of the numerical method (2.1) is reported with the Algorithm 1.
Algorithm 1: Nonlocal finite difference scheme for (1.1) |
Inputs:h,T,M,{S0i}Mi=1,{φi0(t)}Mi=1,P0(t),{Ai(t)}Mi=1,B(t),{βi}Mi=1,{βir}Mi,r=1,{αi}Mi=1,{ci}Mi=1 |
Outputs: (t0,…,tˉn),{(S0i,…,Sˉni)}Mi=1,{(φ0i,…,φˉni)}Mi=1,(P0,…,Pˉn) |
1 ˉn←⌈T/h⌉ |
2 t0←0, P0←P0(0) |
3 for 1≤i≤M do |
![]() |
The following results, concerning non-negativity and boundedness, hold.
Theorem 2.1. Consider equation (2.1) with Assumptions A, then the solution sequences {Sni}n∈N0, {φni}n∈N0, i=1,…,M and {Pn}n∈N0, are non-negative, ∀h>0. Furthermore, the sequence {Sni}n∈N0, i=1,…,M, is positive and non-increasing.
Proof. We proceed by induction to prove that the statement Sni>0, φni≥0, Pn≥0, holds for all n∈N0, h>0 and 1≤i≤M. The case n=0 is true because the initial values are non-negative and V0i=∑Mr=1βirφ0r+αiP0≥0, i=1,…,M. Consider n≥1 and assume that the properties are true for each 0≤j≤n−1. It follows that Vji≥0, for 0≤j≤n−1, therefore it is
Sni=Sn−1i1+hβiVn−1i>0,i=1,…,M, | (2.2) |
and then, from (2.1), also φni≥0, i=1,…,M and Pn≥0. Furthermore, from (2.2), Sni≤Sn−1i, for all n≥1 and i=1,…,M, which completes the proof.
From the first equation of (2.1) it is
Sn+1i=S0i∏nj=0(1+hβiVji), |
and this leads to the following equation for the asymptotic numerical solution S∞i(h),
logS0iS∞i(h)=+∞∑n=0log(1+hβiVni), | (2.3) |
where S∞i(h)=limn→∞Sni, for any fixed stepsize h>0, which exists since the sequence {Sni}+∞n=0 is non-increasing, for each 1≤i≤M.
Theorem 2.2. Consider equation (2.1) with Assumptions A and B, then the solution sequences {Sni}n∈N0, {φni}n∈N0, i=1,…,M and {Pn}n∈N0, are bounded, ∀h>0.
Proof. From Theorem 2.1, {Sni}n∈N0 is a non-increasing sequence, thus it is bounded from above by S0i, i=1,…,M. Consider φni, from the first equation and the second equation of (2.1), it is
φni≤φ0,max+Amax(S0i−Sn+1i)≤φ0,max+AmaxS0i. |
Finally, the inequality
Pn≤P0,max+ˉBM∑r=1cr(φ0,max+AmaxS0r), |
directly follows from the assumptions and the third equation in (2.1).
As already pointed out in the previous section, the time-continuous solution to (1.1) is non-negative and bounded and the proposed numerical solution algorithm preserves boundedness and nonnegativity unconditionally with respect to time step size.
In order to study the convergence of the numerical method, we assume that the known functions are continuously differentiable on [0,T], with T<+∞, and we investigate the behaviour of the local truncation error (see [24] for the definition). Here, we consider the case of M=1, since the generalization to M>1 is straightforward. In this case, the local truncation error of the discretization in (2.1) reads, for T=ˉnh and n=0,…,ˉn,
δn(h)=∫tn0[−β1S1(τ)V1(τ)−β1S1(τ)V1(τ)A1(tn−τ)c1B(tn−τ)φ1(τ)]dτ−hn−1∑j=0[−β1S1(tj+1)V1(tj)−β1S1(tj+1)V1(tj)A1(tn−j)c1B(tn−j)φ1(tj)]. | (2.4) |
By the mean value theorem
∫tn0[−β1S1(τ)V1(τ)−β1S1(τ)V1(τ)A1(tn−τ)c1B(tn−τ)φ1(τ)]dτ=n−1∑j=0∫tj+1tj([−β1S1(τ+h)V1(τ)−β1S1(τ+h)V1(τ)A1(tn−τ)c1B(tn−τ)φ1(τ)]−h[−β1S′(τ+θjh)V1(τ)−β1S′(τ+θjh)V1(τ)A1(tn−τ)c1B(tn−τ)φ1(τ)])dτ, |
with θj∈(0,1), j=0,…,n−1. Moreover, due to the convergence properties of the rectangular quadrature rule (see, for instance, [25]), the bound
‖∫tj+1tj[−β1S1(τ+h)V1(τ)−β1S1(τ+h)V1(τ)A1(tn−τ)c1B(tn−τ)φ1(τ)]dτ−h[−β1S1(tj+1)V1(tj)−β1S1(tj+1)V1(tj)A1(tn−j)c1B(tn−j)φ1(tj)] ‖≤Ch2, |
holds for each 0≤j≤n−1, with C>0, independent of h. It then follows from (2.4) that
‖δn(h)‖≤ˉnCh2+hˉn−1∑j=0∫tj+1tj‖[−β1S′(τ+θjh)V1(τ)−β1S′(τ+θjh)V1(τ)A1(tn−τ)c1B(tn−τ)φ1(τ)]‖dτ, |
for each n=0,…,ˉn. Hence
max0≤n≤ˉn‖δn(h)‖≤˜Ch, | (2.5) |
being ˜C a positive constant not depending on h. Denote by
En(h)=[…,Si(tn)−Sni,…,φi(tn)−φni,…,P(tn)−Pn]T∈R2M+1, |
the global error of the discretization (2.1). The following theorem, that we prove by standard techniques, provides sufficient conditions for the convergence of the numerical method.
Theorem 2.3. Assume that the given functions Ai(t), i=1,…,M and B(t), describing problem (1.1), are continuously differentiable on an interval [0,T] and that {Sni}n∈N0, {φni}n∈N0, {Pn}n∈N0 are the approximations to the solution of (1.1), defined by (2.1). Let h=T/ˉn, with ˉn positive integer, then
limh→0max0≤n≤ˉn‖En(h)‖=0. |
Furthermore, the order of convergence is 1.
Proof. We prove the result for M=1, since the generalization to M>1 is straightforward. In this case, the time-continuous system (1.1) reads
[S1(tn)φ1(tn)P(tn)]=[S1(0)φ10(tn)P0(tn)]+∫tn0[−β1S1(τ)V1(τ)−β1S1(τ)V1(τ)A1(tn−τ)c1B(tn−τ)φ1(τ)]dτ=[S1(0)φ10(tn)P0(tn)]+hn−1∑j=0[−β1S1(tj+1)V1(tj)−β1S1(tj+1)V1(tj)A1(tn−j)c1B(tn−j)φ1(tj)]+δn(h), |
and subtracting (2.1) from it leads to the global error
En(h)=δn(h)+hn−1∑j=0[−β1000β1A1(tn−j)000c1B(tn−j)][S1(tj+1)V1(tj)−Sj+11Vj1S1(tj+1)V1(tj)−Sj+11Vj1φ1(tj)−φj1], | (2.6) |
for n=0,…,ˉn. Furthermore, for each j=0,…,n−1,
|S1(tj+1)V1(tj)−Sj+11Vj1|=|S1(tj+1)(V1(tj)−Vj1)+Vj1(S1(tj+1)−Sj+11)|≤K(‖Ej(h)‖+‖Ej+1(h)‖), |
hence, from (2.6),
‖En(h)‖≤‖δn(h)‖+h˜Kn−1∑j=0(‖Ej(h)‖+‖Ej+1(h)‖), |
with K and ˜K positive constants depending on the parameters of the problem but not on h. Therefore, for a sufficiently small h,
‖En(h)‖≤‖δn(h)‖1−h˜K+h 2˜K1−h˜Kn−1∑j=0‖Ej(h)‖,n=0,…,ˉn. |
Finally, the Gronwall discrete inequality yields (see, for instance [24,p.101]),
‖En(h)‖≤(max0≤n≤ˉn‖δn(h)‖1−h˜K+h 2˜K(S01+φ01+P0)1−h˜K)exp(2˜KT1−h˜K), |
for n=0,…,ˉn. Then, from (2.5), the result follows.
In this section we prove some preliminary results needed to describe the asymptotic behaviour of the solution to system (2.1).
Lemma 3.1. Consider system (2.1), for i=1,…,M, it is
+∞∑n=0Vni=αi+∞∑n=0P0(tn)+M∑r=1(βir+αicrh+∞∑n=1B(tn))(S0r(1−S∞r(h)S0r)+∞∑n=1Ar(tn)++∞∑n=0φ0r(tn)). |
Proof. Summing from 0 to ∞ in the last equation of (2.1), interchanging the order of summation and adding to both members P(0)=P0, it is
+∞∑n=0Pn=+∞∑n=0P0(tn)+M∑r=1crh+∞∑n=1B(tn)+∞∑n=0φnr. |
The same can be done for the second equation of (2.1), where taking into account that, from the first of (2.1) it is hβr∑+∞j=0Sj+1rVjr=S0r−S∞r(h), we have
+∞∑n=0φnr=(S0r−S∞r(h))+∞∑n=1Ar(tn)++∞∑n=0φ0r(tn), | (3.1) |
for any r=1,…,M. Combining the previous expressions with
+∞∑n=0Vni=αi+∞∑n=0Pn+M∑r=1βir+∞∑n=0φnr,i=1,…,M, |
we get the result.
Theorem 3.1. Consider equation (2.1) with Assumptions A, B and C, then there exists 0<ˉV<+∞, such that h∑+∞n=0Vnr<ˉV.
Proof. From Lemma 3.1, for i=1,…,M, it is
h+∞∑n=0Vni≤αiˉP0+(maxr=1,…,Mβir+ˉBαimaxr=1,…,Mcr)(ˉAM∑r=1S0r+Mˉφ0). |
The results of Section 2 ensure that the approximation of the solution to (1.1), obtained by (2.1), unconditionally retains the basic properties of the model. Here, we investigate the asymptotic properties and prove that the limit of the numerical solution behaves exactly as its continuous counterpart.
First of all, we observe that from Assumptions C and (3.1), for each positive h,
h+∞∑n=0φni≤(S0i−S∞i(h))ˉA+¯φ0<+∞,thuslimn→+∞φni=0,i=1,…,M. | (4.1) |
Define, for x=(x1,…,xM), h>0 and i=1,…,M,
Ri(x,h)=log(S0ixi)−βiαiUi(h)h+∞∑n=0P0(tn)−βiUi(h)M∑r=1(βir+αicrh+∞∑n=1B(tn))⋅(S0r(1−xrS0r)h+∞∑n=1Ar(tn)+h+∞∑n=0φ0r(tn)). | (4.2) |
Here, Ui(h) is given by
Ui(h)=+∞∑n=0log(1+hβiVni)hβi+∞∑n=0Vni,i=1,…,M. | (4.3) |
We observe that, due to (4.3), the relation (2.3) is equivalent to
logS0iS∞i(h)−Ui(h)(hβi+∞∑n=0Vni)=0,i=1,…,M. |
Hence, by substituting in it the expression of ∑+∞n=0Vni from Lemma 3.1, it is clear that for any fixed h>0, the asymptotic numerical solution S∞i(h), i=1,…,M, is a root of the nonlinear system of equations
Ri(x(h),h)=0,i=1,…,M, | (4.4) |
if a solution to (4.4) exists.
In order to show that S∞i(h) tends to Si(∞), as h→0, we need the following result.
Lemma 4.1. Consider equation (2.1) with Assumptions A, B and C, then, for Ui(h) defined in (4.3), it is
limh→0Ui(h)=1,i=1,…,M. | (4.5) |
Proof. From its definition and Lemma 3.1, Vni, i=1,…,M, n≥0, is bounded by a constant independent of h. This implies that limh→0hVni=0, uniformly with respect to h. In this situation, we are allowed to proceed as in the proof of [19,Theorem 4.3] to get (4.5).
Now we want to prove that system (4.4) has a unique solution for h>0, so we consider a general nonlinear algebraic system,
Γi(x1,…,xM)=0,i=1,…,M, | (4.6) |
for which the following result holds.
Theorem 4.1. Denote by D=∏Mi=1Di, with Di=(ai,bi], i=1,…,M. Let Γi:D→R, i=1,…,M, be a twice continuously differentiable function and assume that, for each i=1,…,M and any fixed (x1,…,xi−1,xi+1,…,xM)∈M∏j=1,j≠iDj,
a) Γi(x1,…,xi−1,ζ,xi+1,…,xM) admits at least one zero in Di;
b) limζ→a+iΓi(…,xi−1,ζ,xi+1,…)>0 and Γi(…,xi−1,bi,xi+1,…)<0;
c) ∂xjΓi(x)>0, for all x∈D, i,j=1,…,M and i≠j;
d) ∂2xjxkΓi(x)≥0, for all x∈D and j,k=1,…,M.
Then, system (4.6) has a unique solution in D.
Proof. We proceed by induction on M. Choose M=2. Let x1∈D1, then from a), b) and d), Γ2(x1,ζ) has a unique zero ξ2=ξ2(x1)∈D2, with ∂x2Γ2(x1,ξ2)<0. Therefore, taking in account the arbitrariness of x1 in D1, from the implicit function theorem, ∀x∈D1, there exists a unique z(x)∈D2 such that u2(x):=Γ2(x,z(x))≡0. Since u′2(x)≡0 and u′′2(x)≡0, using assumption c) and the fact that a), b) and d) imply ∂x2Γ2(x,z(x))<0, we conclude that z′(x)>0 and z′′(x)≥0. Now, we exploit the function z(x) to build a solution to system (4.6) with M=2.\\ For an arbitrary α0∈D1, let α1∈D1 be the unique root of the function Γ1(ζ,z(α0)). If α1=α0, then (α0,z(α0)) is a solution to (4.6) with M=2. Otherwise we can suppose, with no loss of generality, that α0<α1. It follows that z(α0)<z(α1) and, from c), 0=Γ1(α1,z(α0))<Γ1(α1,z(α1)). Thus Γ1(ζ,z(α1)) has a unique zero α2∈D1, for which α0<α1<α2. Similar arguments lead to an increasing sequence {αn}n∈N⊂D1, such that
∀n>0,Γ1(αn+1,z(αn))=0,α=limn→+∞αn,andΓ1(α,z(α))=Γ2(α,z(α))=0. |
Hence, (α,z(α))∈D is a solution to system (4.6), with M=2.
To prove its uniqueness, we consider another solution (β,γ)∈D and define the function u1:x∈D1→Γ1(x,z(x)). Since Γ2(β,γ)=0, it follows that γ=z(β) and u1(β)=0=u1(α). Since u1 is convex and, from b), it admits a unique zero β=α, then the solution to (4.6) for M=2 is unique.
Now assume that the result holds for any M−1 dimensional system satisfying a)–d). Consider M>2, proceeding as in the previous case, for each (x1,…,xM−1)∈∏M−1j=1Dj, there exists a unique function z(x1,…,xM−1) such that
ΓM(x1,…,xM−1,z(x1,…,xM−1))=0, |
∂xiz(x1,…,xM−1)>0and∂2x2iz(x1,…,xM−1)≥0,i=1,…,M−1. | (4.7) |
Define, for i=1,…,M−1, the functions
ui(x1,…,xM−1)=Γi(x1,…,xM−1,z(x1,…,xM−1)). | (4.8) |
Now we want to prove that assumptions a)-d) are true for the M−1 dimensional system
ui(x1,…,xM−1)=0,i=1,…,M−1, | (4.9) |
which is equivalent to (4.6). Regarding the assumption a), we need to prove that, for each fixed (x1,…,xi−1,xi+1,…,xM−1)∈M−1∏j=1,j≠iDj, the function
ui(x1,…,xi−1,ζ,xi+1,…,xM−1) |
has a zero in Di, i=1,…,M−1. For the theorem assumptions, given α0∈Di, there exists a unique α1∈Di, such that
Γi(x1,…,xi−1,α1,xi+1,…,xM−1,z(x1,…,xi−1,α0,xi+1,…,xM−1))=0. |
If α0=α1, we get the result. Otherwise, proceeding as in the M=2 case, we construct a monotone sequence {αn}n∈N such that α=limn→+∞αn∈Di and ui(x1,…,xi−1,α,xi−1,…,xM−1)=0 holds. Furthermore, b), c) and d) immediately follow from the hypotheses of the theorem, from (4.7) and from (4.8). Therefore, induction hypotheses assure that there exists a unique λ∈∏M−1i=1Di, root of the system (4.9). It follows that (λ,z(λ)) is a solution to the original system (4.6).
Finally, if (η,θ) is another solution of (4.6), with η∈∏M−1i=1Di and θ∈DM, then θ=z(η). Thus η solves (4.9) and the uniqueness of its solution, that we have just proved, yields η=λ, which completes the proof.
Consider the nonlinear system (4.4) for h>0, with Ri(x,h) defined in (4.2). The assumptions of Theorem 4.1 apply to this system with Γi(x)=Ri(x,h) and Di=(0,S0i], i=1,…,M. As a matter of fact we analyze, for h>0, i,j=1,…,M and i≠j, the twice continuously differentiable function Ri(x1,…,xi−1,ζ,xi+1,…,xM,h), with 0<ζ≤S0i and 0<xj≤S0j fixed. Since
limζ→0+Ri(x1,…,xi−1,ζ,xi+1,…,xM,h)=+∞,Ri(x1,…,xi−1,S0i,xi+1,…,xM,h)≤0, |
there exists 0<S∞i(h)≤S0i, such that Ri(x1,…,xi−1,S∞i(h),xi+1,…,xM,h)=0. Furthermore, for each i,j=1,…,M, i≠j, it is
∂xjRi(x,h)>0,∂2x2jRi(x,h)=0,and∂2x2iRi(x,h)=1/x2i. |
Thus, according to Theorem 4.1, system (4.4) has, for each h>0, a unique solution (S∞1(h),…,S∞M(h)), with 0<S∞i(h)≤S0i, i=1,…,M, which is the asymptotic numerical solution.
Regarding the time-continuous model, we consider the nonlinear system
Ri(x)=0,i=1,…,M, | (4.10) |
with Ri(x) defined in (1.6). It can be easily seen that the assumptions of Theorem 4.1 are accomplished and then the asymptotic analytical solution Si(∞), i=1,…,M, to (1.1) is the unique solution of (4.10), with 0<Si(∞)≤S0i, i=1,…,M.
In order to investigate the relation between the asymptotic properties of the numerical solution as h→0 and of the time-continuous solution, we assume that
limh→0h+∞∑n=1Ai(tn)=∫+∞0Ai(t) dt,limh→0h+∞∑n=1B(tn)=∫+∞0B(t) dt,limh→0h+∞∑n=1φ0i(tn)=∫+∞0φ0i(t) dt, | (4.11) |
for each h>0 and i=1,…,M. The conditions in (4.11) are true, for instance, if the involved functions are ultimately non-increasing, or if their derivatives belong to L1[0,+∞) (see [19]).
As the stepsize h vanishes, we expect that the asymptotic numerical solution converges to the continuous one. In fact, we apply the following result.
Theorem 4.2. Consider a bounded subset D of RM, and a function
Φ:D×[0,+∞)→RM, |
satisfying:
a) Φ(w,h), continuous for each (w,h)∈D×[0,+∞);
b) equation Φ(w,h)=0, has at least one solution ˉw(h)∈D, ∀h∈[0,+∞);
c) equation Φ(w,h)=0 has a unique solution for h=0, namely ˉw=ˉw(0)∈D.
Then limh→0ˉw(h)=ˉw.
Proof. Straightforward extension of [26,Theorem 3.5] to the case of functions defined on open sets.
The vector function R(x,h)=(R1(x,h),…,RM(x,h)), with Ri defined in (4.2), satisfies all the assumptions of Theorem 4.2, in D=(0,S01]×…×(0,S0M]. Now, if (4.11) holds, the system corresponding to h=0 is given by (4.10). Therefore, this result establishes a connection between the asymptotic numerical solution S∞i(h), i=1,…,M and the solution Si(∞), i=1,…,M of the nonlinear system (4.10), thus emphasizing that the limit of S∞i(h), i=1,…,M, for h→0 exists and
limh→0limn→+∞Sni(h)=limh→0S∞i(h)=Si(∞),i=1,…,M. | (4.12) |
As we have emphasized in Section 1, system (1.1) represents a general theoretical setting which includes a variety of epidemic models in the literature. In this context, the nonlinear system (4.10) is the final size relation for the epidemic and system (4.4) represents the discrete final size relation corresponding to the numerical solution. From this point of view, Sections 3 and 4 analyze how the qualitative properties of the model are preserved when the system is integrated by the numerical scheme (2.1). We focus here on four cases of interest, which act as test cases in our analysis. Due to Theorems 2.1 and 2.2, the numerical solution remains non-negative and bounded for any value of the stepsize h>0, this guarantees that the epidemic is correctly simulated by method (2.1). The asymptotic analysis of the previous section applies to show that also the long time behaviour is preserved since the numerical final size converges, as h→0, to the final size of the epidemic. This is also clear in the figures, which represent the results of the numerical experiments for each test model considered. For our experiments we choose illustrative test equations and we use the non-standard method (2.1).
● The age-of-infection epidemic model described in [9,p. 139] by the system (1.2). Here, in case φ0(t)=(N−S0)A(t), being N the constant size of the population, the final size of the epidemic S(∞) is the unique root of the nonlinear equation (see for example [9])
logS0x−R0(1−xN)=0, | (5.1) |
where R0=βN∫+∞0A(t)dt is the basic reproduction number. Given the identifications of the parameters in I, the nonlinear system (4.10) corresponds to system (5.1) (see [27,28] for a general discussion on the meaning and on the analytical and numerical computation of R0). For this model the numerical method (2.1) reduces to the non-standard numerical scheme proposed in [19] and the nonlinear equation (4.4) represents the relation for the numerical final size of the epidemic S∞(h) as obtained in that paper. In [19] it is proved that limh→0S∞(h)=S(∞), provided that this limit exists. In Section 4, Theorem 4.2, we have proved that, in fact, this limit exists since, under the above mentioned assumptions on A(t), (4.11) holds. So, Theorem 4.2 completes the analysis made in [19] and we can assert that the numerical method is asymptotically coherent with (1.2). Figure 1 shows the result when integrating problem (1.2) for t∈[0,100], when the infectivity function has a low regularity (see [29] for reference on this problem)
A(t)={00≤t≤τa,(t−τa)(τh−t)(τb−τa)(τh−τe)τa<t<τb,τh−tτh−τeτb≤t<τh,0t≥τh. | (5.2) |
We have set the parameters β=10−4, τe=12, τa=14, τb=16, τh=19, and we have used S0=49950, N=50000, and stepsize h=0.1. We see that the numerical solution behaves coherently with the theoretical findings. The dot at the left end point of the integration interval is the value for S(∞) obtained by solving the nonlinear equation (5.1) through the Matlab routine fzero. This value is S(∞)=148.83. The value S∞(h) obtained by running the method (2.1) in the interval [0,1000], with h=10−3, is 149.97 and the accuracy in the approximation improves linearly as the stepsize h→0. Furthermore, in compliance with (4.1), the endpoint approximation of φ is numerically zero. Other numerical tests are reported in [19].
● The model (1.3) with both symptomatic and asymptomatic infections in [10]. In this case the final size of the epidemic S(∞) is the root of the nonlinear equation
logS0x−F⋅(S0−xN)−aN(∫+∞0φs0(t) dt+∫+∞0φa0(t) dt)=0, | (5.3) |
where F=a∫+∞0f(t)As(t) dt+a∫+∞0(1−f(t))Aa(t) dt, is considered to be finite. System (5.3) is equivalent to (4.10) with the parameters specified in II. Hence, the findings of Section 4 provide a uniqueness result for S(∞) as the only root in (0,S0] of (5.3). Furthermore, the convergence relation (4.12) confirms the numerical method (2.1) as a reliable tool to predict the asymptotic behaviour of the epidemic and the total number of symptomatic and asymptomatic patients. These considerations extend the investigation of [10]. As an example, we integrate problem (1.3) with
As(t)=πs(t)Bs(t),Bs(t)=exp(−√t/2),πs(t)=5γ(t;1,2),Aa(t)=πa(t)Ba(t),Ba(t)=(1+0.6t)−1, πa(t)=γ(t;3,2),φs0(t)=(N−S0)As(t),φa0(t)=(N−S0)Aa(t),f(t)=0.783,t≥0, | (5.4) |
where
γ(t;k,θ)=tk−1θ−ke−t/θ∫+∞0xk−1e−x dx, |
is the gamma probability density function. The number of symptomatic and asymptomatic individuals at time t, Is(t) and Ia(t), respectively, satisfy the system
Is(t)=Is0(t)+aNf∫t0Bs(t−τ)S(τ)(φs(τ)+φa(τ)) dτ,Ia(t)=Is0(t)+aN(1−f)∫t0Ba(t−τ)S(τ)(φs(τ)+φa(τ)) dτ. | (5.5) |
Because of the absence of demographic turnover, the number of recovered people, at time t, is R(t)=N−(S(t)+Ia(t)+Is(t)). Figure 2 shows the approximation of S(t) by (1.1), as well as the approximations of Is(t), Ia(t) and R(t) computed, for n≥0, by the same nonlocal technique employed in (2.1).
● The multi-group heterogeneous populations model (1.4) proposed in [11]. The nonlinear system for the final sizes Si(∞), of the epidemic in group i, i=1,…,M, is
logS0ixi−aiM∑j=1pijNj((S0j−xj)∫+∞0Aj(t) dt+∫+∞0φj0(t) dt)=0. | (5.6) |
System (4.10), with the parameter specifications in III, corresponds to the final size system (5.6). In Figure 3 we report the numerical solution to equation (1.4), when the population of size N=1000 is divided in 2 subgroups of sizes N1=0.1N and N2=0.9N, respectively. We consider φi0(t)=(Ni−S0i)Ai(t), i=1,2 and we choose S01=99, S02=899, a1=5, a2=10, p11=0.4, p12=0.6, p21=0.5, p22=0.5, and
A1(t)=A2(t)=1σ√2πe−(t−μ)22σ2,μ=0.2,σ=3μ. | (5.7) |
In order to check the asymptotic properties of the numerical solution, we have solved problem (1.4) by (2.1) on a large interval many times, each time halving the value of the stepsize h. Then we have used the numerical solution at the end point of the integration interval to evaluate the expression (5.6). This procedure gives a measure for the errors in approximating Si(∞), which are listed in Table 1, where it is clear that the numerical final size converges linearly to the corresponding continuous one.
h | Error on final size |
0.05 | 0.56 |
0.025 | 0.30 |
0.0125 | 0.15 |
0.00625 | 0.08 |
● The virus shedding epidemic model (1.5) studied in [13]. Here we assume that φi0=∫t−∞Ai(t−s)S(s)P(s)ds, i=1,2 and P0(t)=∫t−∞Γ(t−s)(r1φ1(s)+r2φ2(s))ds, are known functions respectively of the form φi0(t)=(Ni−S0i)Ai(t), and P0(t)=P0Γ(t). In this case the final size relation for Si(∞), i=1,2, is the following
logS0ixi−βi(R1(1−x1N1)+R2(1−x2N2)+P0∫+∞0Γ(t)dt)=0, | (5.8) |
i=1,2. For each group, Ni (i=1,2) are the sizes and
Ri=riNi∫+∞0Ai(t) dt∫+∞0Γ(t) dt, |
are the basic reproduction numbers. Moreover, under the conditions in IV, system (4.10) corresponds to the nonlinear system (5.8), which has a unique solution because of Theorem 4.1. The numerical results are shown in Figure 4 for
A1(t)=A2(t)=1σ√2πe−(t−μ)22σ2,μ=0.2,σ=3μ,Γ(t)=1(1+t)2, | (5.9) |
N1=200, N2=300, S01=199, S02=298, P0=2, β1=0.015, β2=0.03, r1=0.1, r2=1. Again a convergence of order one of the numerical final size S∞i(h), i=1,2, to the one given by the model (1.5), can be experimentally observed.
Problem (1.1) and the corresponding discrete system (2.1) represent a general framework to study and compare the qualitative behaviour of the analytical solution of some epidemic models, currently of interest in the scientific community, and its numerical approximation. The numerical solution is obtained by a non-standard discretization of the nonlinear terms in the system, and agrees with the analytical solution in many important qualitative aspects. Both the behaviour at finite time and the asymptotic properties of the solution are preserved for any value of the stepsize h>0. Furthermore, it is proved that the asymptotic numerical solution is the unique root of a nonlinear system, and that it converges to the analytical one as h→0. The system of equations (1.1) includes, in its general form, some of the well known age-of-infection epidemic models in the literature. In general, the study carried out in this paper can be regarded as a stability numerical investigation on a class of epidemic problems that act as test equations. The role of the test equations here can be considered from two different points of view. First of all, since the proposed method preserves the qualitative peculiarities of the continuous solution, it can be considered reliable also for its quantitative evaluation and so the numerical solution can answer crucial questions such as the peak of the epidemic represented by (1.1). From another point of view, the aforementioned characteristics of the method make it reasonable to expect that it will behave well even on more complex problems, not included in (1.1), such as models with death for disease or with time-varying coefficients [30], where the theory needs simulation to describe the dynamics of the epidemic. The issue of time-varying coefficients represents an interesting point to investigate in a future work within the framework of the general model (1.1).
This research was supported by GNCS-INDAM.
The authors declare there is no conflict of interest.
[1] |
Belleri A, Brunesi E, Nascimbene R, et al. (2015) Seismic performance of precast industrial facilities following major earthquakes in the Italian territory. J Perform Constru Fac 29: 04014135. doi: 10.1061/(ASCE)CF.1943-5509.0000617
![]() |
[2] |
Perrone D, Calvi P, Nascimbene R, et al. (2019) Seismic performance of non-structural elements during the 2016 central Italy earthquake. B Earthq Eng 17: 5655–5677. doi: 10.1007/s10518-018-0361-5
![]() |
[3] |
Brunesi E, Peloso S, Pinho R, et al. (2018) Cyclic testing of a full-scale two-storey reinforced precast concrete wall-slab-wall structure. B Earthq Eng 16: 5309–5339. doi: 10.1007/s10518-018-0359-z
![]() |
[4] | Bianchi F, Nascimbene R, Pavese A (2017) Experimental vs. numerical aimulations: Seismic response of a half scale three-storey infilled RC building strengthened using FRP retrofit. TOCEJ 11: 1158–1169. |
[5] |
Nascimbene R (2015) Numerical model of a reinforced concrete building: Earthquake analysis and experimental validation. Period Polytech-Civ 59: 521–530. doi: 10.3311/PPci.8247
![]() |
[6] |
Rahnavard R, Rebelo C, Hélder C, et al. (2020) Numerical investigation of the cyclic performance of reinforced concrete frames equipped with a combination of a rubber core and a U-shaped metallic damper. Eng Struct 225: 111307. doi: 10.1016/j.engstruct.2020.111307
![]() |
[7] | Visintin A (1994) Differential Models of Hysteresis, Germany: Springer-Verlag. |
[8] |
Sivaselvan M, Reinhorn A (2000) Hysteretic models for deteriorating inelastic structures. J Eng Mech 126: 633–640. doi: 10.1061/(ASCE)0733-9399(2000)126:6(633)
![]() |
[9] | Pires F (1990) Influência das paredes de alvenaria no comportamento de estruturas reticuladas de betão armado sujeitas a acções horizontais [PhD Thesis]. LNEC, Lisboa. |
[10] | Braz-César M, Oliveira D, Barros R (2008) Comparison of cyclic response of reinforced concrete infilled frames with experimental results. The 14th World Conference on Earthquake Engineering, Beijing, China. |
[11] | Fardis M, Panagiotakos T (1997) Seismic design and response of bare and masonry-infilled reinforced concrete buildings. Part Ⅰ: Bare structures. J Earthq Eng 1: 219–256. |
[12] |
Paulay T, Priestley MJN (1992) Seismic Design of Reinforced Concrete and Masonry Buildings, New York: John Wiley & Sons. doi: 10.1002/9780470172841
![]() |
[13] | Clough RW (1966) Effects of stiffness degradation on earthquake ductility requirement. UCB/SESM 1966/16, University of California, Berkeley, USA. |
[14] | Takeda T, Sozen MA, Nielsen NN (1971) Reinforced concrete response to simulated earthquakes. OHBAYASHI-GUMI Technical Research Report 5, Tokyo, Japan. |
[15] | Midas Inc. (2004) Analysis manual: Inelastic time history analysis, Korea. |
[16] | Natick, Massachusetts, MATLAB R2019a 9.6.0.1072779, USA: The MathWorks, Inc. |
[17] | Deng H, Chang Y, Lau D, et al. (2003) A simplified approach for nonlinear response analysis of composite structural members. International Workshop on Steel and Concrete Composite Constructions NCREE, Taiwan, 207–216. |
[18] |
Kent D, Park T (1971) Flexural members with confined concrete. J Struct Div 97: 1969–1990. doi: 10.1061/JSDEAG.0002957
![]() |
[19] | Bouc R (1968) Forced vibration of mechanical systems with hysteresis, In: Rupakhety R, Olafsson S, Bessaon B, Proceedings of the Fourth Conference on Non-linear Oscillation, Prague: Academia. |
[20] | Wen Y (1976) Method for random vibration of hysteretic systems. J Eng Mech-ASCE 102: 249–263. |
[21] |
Wen Y (1980) Equivalent linearization for hysteretic system under random excitation. J Appl Mech 47: 150–154. doi: 10.1115/1.3153594
![]() |
[22] | D'Errico J (2021) fminsearchbnd, fminsearchcon. Available from: https://www.mathworks.com/matlabcentral/fileexchange/8277-fminsearchbnd-fminsearchcon. |
1. | Bálint Máté Takács, Gabriella Svantnerné Sebestyén, István Faragó, High-order reliable numerical methods for epidemic models with non-constant recruitment rate, 2024, 206, 01689274, 75, 10.1016/j.apnum.2024.08.008 | |
2. | Bruno Buonomo, Eleonora Messina, Claudia Panico, Antonia Vecchio, An integral renewal equation approach to behavioural epidemic models with information index, 2025, 90, 0303-6812, 10.1007/s00285-024-02172-y |
h | Error on final size |
0.05 | 0.56 |
0.025 | 0.30 |
0.0125 | 0.15 |
0.00625 | 0.08 |