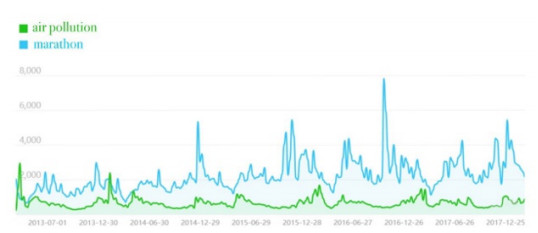
Citation: Nataša Katić, Joakim S. Korshøj, Helle F. Christensen. Bryozoan limestone experience—the case of Stevns Klint[J]. AIMS Geosciences, 2019, 5(2): 163-183. doi: 10.3934/geosci.2019.2.163
[1] | Zuqin Ding, Yaxiao Li, Xiaomeng Wang, Huling Li, Yongli Cai, Bingxian Wang, Kai Wang, Weiming Wang . The impact of air pollution on the transmission of pulmonary tuberculosis. Mathematical Biosciences and Engineering, 2020, 17(4): 4317-4327. doi: 10.3934/mbe.2020238 |
[2] | Li Xu, Ping Guo, Guoqin Pan . Effects of inter-industry agglomeration on environmental pollution: Evidence from China. Mathematical Biosciences and Engineering, 2023, 20(4): 7113-7139. doi: 10.3934/mbe.2023307 |
[3] | Peng-Yeng Yin . Spatiotemporal retrieval and feature analysis of air pollution episodes. Mathematical Biosciences and Engineering, 2023, 20(9): 16824-16845. doi: 10.3934/mbe.2023750 |
[4] | Yanhong Feng, Qingqing Hu . Heterogeneity and spillover effects of carbon emission trading on green innovation. Mathematical Biosciences and Engineering, 2023, 20(4): 6468-6497. doi: 10.3934/mbe.2023279 |
[5] | Xiaomeng Wang, Xue Wang, Xinzhu Guan, Yun Xu, Kangwei Xu, Qiang Gao, Rong Cai, Yongli Cai . The impact of ambient air pollution on an influenza model with partial immunity and vaccination. Mathematical Biosciences and Engineering, 2023, 20(6): 10284-10303. doi: 10.3934/mbe.2023451 |
[6] | Yangyan Zeng, Yidong Zhou, Wenzhi Cao, Dongbin Hu, Yueping Luo, Haiting Pan . Big data analysis of water quality monitoring results from the Xiang River and an impact analysis of pollution management policies. Mathematical Biosciences and Engineering, 2023, 20(5): 9443-9469. doi: 10.3934/mbe.2023415 |
[7] | Qi Zhou, Huaimin Yuan, Qimin Zhang . Dynamics and approximation of positive solution of the stochastic SIS model affected by air pollutants. Mathematical Biosciences and Engineering, 2022, 19(5): 4481-4505. doi: 10.3934/mbe.2022207 |
[8] | Jie Ren, Shiru Qu, Lili Wang, Yu Wang . An en route capacity optimization model based on air traffic control process. Mathematical Biosciences and Engineering, 2022, 19(4): 4277-4299. doi: 10.3934/mbe.2022198 |
[9] | Qiongyang Zhou, Tianyu Zhao, Kaidi Feng, Rui Gong, Yuhui Wang, Huijun Yang . Artificial intelligence in acupuncture: A bibliometric study. Mathematical Biosciences and Engineering, 2023, 20(6): 11367-11378. doi: 10.3934/mbe.2023504 |
[10] | Vincenzo Capasso, Sebastian AniȚa . The interplay between models and public health policies: Regional control for a class of spatially structured epidemics (think globally, act locally). Mathematical Biosciences and Engineering, 2018, 15(1): 1-20. doi: 10.3934/mbe.2018001 |
Reducing air pollution in urban areas is one of the most active debated public policies related to city management, green development and energy saving around the world [1,2,3]. Although different tools have been used to control air pollution, a quite confusing feature is that even similar public policies can leads to different outcomes based on different governmental behaviours [4,5,6]. For example, the policy of banning vehicles from driving one day per week in Beijing can significantly reduce air pollution [7]. However, a similar policy taken by Tianjin, another Chinese super-sized city located 110 km from Beijing, has not been proved to improve air quality. The existing research does not pay much attention to the effects of decentralization on air pollution: it is the changes of incentives of local government caused by decentralization that may cause the heterogeneous effects of air pollution reduction. Also, the impact of authority decentralization on organizing sports events are seldom considered by previous literature. Understanding the effects of local governments' incentives after decentralization on reducing air pollution not only can help to reveal the logic of officials' behaviours, but also is important for making effective environmental policies.
We use Chinese authority decentralization of holding marathon games as a quasi-experiment to identify the causal relationship between local governments' incentives and air pollution reduction. In 2015, the General Administration of Sport of China (GASC) cancelled the approval of marathon games nationwide. From then on, the right to hold marathon games is de facto decentralised from central to local governments. Since then, marathon games thrived in Chinese cities: according to China Marathon Annual Report (2017), 1 the number of national marathon games reaches 1102 in 2017, while from 2012 to 2016, this number is only 33, 39, 51, 134, and 328, respectively. The decentralization of holding sports games from central to local governments since 2015 may strengthen the incentives of local government to perform better, as they do not need to petition the central government. Therefore, because marathon games held for the first time after 2015 is independently initiated by local government rather than organizing under the order or guidance by central governments, local officials with better understanding of local situation and more private information have stronger motivation perform better during marathon games. In this paper, we discuss an important outcome related to local governments' effort: the reduce of local air pollution.
1 http://www.athletics.org.cn/
We investigate the effects of authority decentralization of sports game on reducing air pollution for two reasons: first, severe air pollution during marathon games is an important issue in Chinese society. Figure 1 shows the graph of Baidu trend based on the key words of 'air pollution' and 'marathon' searched online. The results show that when marathon games become a hot topic, the problem of air pollution is also closely followed by the public. This trend has been obvious since 2014, especially when air pollution in China has become a serious issue in recent years. According to a World Bank survey including 188 countries, China is the country suffering the second highest concentrations of PM2.5 in 2013. From 1990 to 2013, the welfare losses due to air pollution have increased by 10.9%, which is the second fastest growth among all countries (in India it is 7.0%) [8]. In reaction to public anger about air pollution and social concerns about health problems, the motivation of city officials to cut air pollution during marathon games is strong. Therefore, after the decentralization of sports games, marathon games that are independently held by local governments may have stronger motivation and willingness to reduce air pollution compared to those cities that are authorized before decentralization.
Second, the booming of marathon game gives us an opportunity to identify the causal effects of authority decentralization on controlling air pollution. After 2015, the right of holding new marathon games is decentralized to local government nationwide, which is unexpected by local governments. Therefore, we can estimate the causal effects of authority decentralization on air quality by comparing the changes of air quality during the days of marathon games before or after the enforcement of decentralization policy. The empirical results find that local air pollution during marathon days decrease significantly. More importantly, air pollution during the days of marathon games that are held after decentralization have a larger reduction. To investigate the mechanisms of these results, we further show that political cycles have a close relationship to reducing air pollution under authority decentralization. Furthermore, we point out that the improvement of air quality under decentralization will become larger with the increase of local leaders' career concerns.
This study deepens our understanding of how local officials are incentivised by two different organisational forms—decentralization and centralisation—which is important to environmental protection [9,10,11,12,13]. Also, this study helps to evaluate the efficiency of environment regulation under strong career concerns by using China as an example [14]. Based on the policy shock of authority decentralization related to holding marathon games, this study provides new evidence about the effects of cadre-based incentives on environmental regulation.
This study also echoes the research related to managerial hierarchy (Kräkel, 2021; Liu and Migrow, 2022) [15,16]. Our findings resonate with the arguments that the decentralization of public organisations can influence both the economic and non-economic performances of local cities (Huang et al., 2017; Yu and Zhang, 2021) [17,18]. In China's administrative hierarchy system, local leaders get promoted based on their performances evaluated by higher level of governments [19], and economic growth has been regarded as the major criteria to evaluate the capacity of officials for a long time [20]. Compared to existing studies, this paper explores whether decentralization can improve government governances from microlevel. Also, this paper shows that local governments with decentralized rights can improve the total efficiency in the non-economic areas such as organizing sports games, especially if central governments are less competent to allocating local resources.
Furthermore, this study adds new empirical evidence about political career incentives related to environmental regulations. In recent decades, reducing pollution has become an important task related to the promotion of local leaders in China [21]. Existing research mainly studies the direct relationship between environmental regulation and political incentives [22]. For example, Foulon et al. [23] show that public disclosure can strengthen environmental performance. Zheng et al. [24] point out that there is a positive relationship between career promotion and pollution reduction. Jia [25] finds that the connection between leaders is positively associated with the pay-off of environmental pollution. Chen et al. [26] show that target-based evaluation system incentivizes local officials to shift their efforts from GDP growth to environmental performance. Gao et al. [27] consider the relationship between political incentives and environmental regulation on industrial land supply. Wu and Cao [28] show that county leaders who can reduce air pollution have higher possibility of promotion. Compared to these studies, we focus on the relationship between environmental regulation and political incentives at city level. We also prove that the improvement of air quality only last for a very short period, which implies the possible existence of opportunism among local leaders when making effort to reduce air pollution.
The contribution of this paper is as follows: Firstly, based on a new perspective compared to previous studies, we discuss the impact of authority decentralization, which shows that air quality during the dates of games will increase when cities no longer need to petition the central government in order to hold a marathon. Secondly, we find the positive effects of political career concerns on reducing air pollution by considering the events of organizing sports games, which is less considered by previous studies. Thirdly, the identification strategy based on high-frequency dataset can attenuate the endogenous problem when considering the causal relationship between authority decentralization and environmental regulation.
The rest of this paper is organised as follows: Section 2 discusses the background of Chinese marathon games and data sources, Section 3 contains the empirical result analysis, Section 4 investigates potential mechanisms, and Section 5 concludes.
Before 2015, a Chinese city that wants to hold new national or international marathon games needs to be authorised by GASC. Because of the exclusive right of GASC, the number of new approved marathon games is rare. The turning point came in October 2014 when Chinese State Council decided to accelerate the approval of mass and commercial sports events by superior authority. In December 2014, GASC announced the cancellation of the approval of organising national and international sports events. In January 2015, Chinese Athletics Association (CAA), a division of GASC, formally decentralized the right of holding new marathon games.
The decentralization arouses the enthusiasm of local governments to hold new marathon games. There is much anecdotal evidence of the relationship between marathon games and local leader's increasing efforts. As commented by Wisdom Sports Group, a Chinese listed firm, the decentralization by GASC gives local governments higher motivation to hold marathon games.2 Guangming Daily, one of the biggest official newspaper in China, pointed out that organising new marathon games has become a way of showing officials' political achievements.3 Since marathon game has been regarded as a way to exhibit good image of city, local governments have more incentives to improve air quality during marathon days by putting more resources into organising games. For example, during Nanjing International Marathon Games in 2015, high-emission plants are required to be closed to reduce air pollution.4
2 http://epaper.21jingji.com/html/2016-02/29/content_33078.htm
3 http://theory.people.com.cn/n1/2016/0122/c49157-28075423.html
4 http://www.xinhuanet.com/politics/2015-11/06/c_128400872.htm
Marathon games. Since 2012, the CAA divides the marathon games from low to high into three levels: bronze, silver, and gold based on "The rating standard of Chinese marathon and related sports events" and "CAA marathon and related sporting events grading approach".5 The CAA- graded marathon games are the top-level long-distance races in China. We manually collect these marathon games from China Annual Marathon Reports from 2013 to 2017 and supplement it by other online information. Figure 2 depicts the number of CAA-graded marathon games from 2013 to 2017 in 98 major cities in China, which shows that the number of marathon games before 2015 is less than 30. Since 2015, the number reaches more than 50 and continues to increase to over 90 in 2017.
5 http://www.runchina.org.cn/mlsf/text/2018013115513152.pdf
The results in Figure 3 further present the number of new marathon games from 1956 to 2017. The number of new marathon games is restricted to fewer than 10 before 2014. From 2015 to 2017, as cities have more freedoms to hold new marathon games, the number increases significantly to 24, 27, and 20, respectively.
Air pollution. The data of air pollution are collected from the Ministry of Ecology and Environment (MEE). Since 2013, the MEE began to use hourly air quality index (AQI), a comprehensive index ranging from 0-500, to measure ambient air quality based on the concentrations of six air pollutants: PM10, PM2.5, CO, SO2, NO2, and O3. The data are based on environmental monitoring stations (increasing in number in major cities from 76 in 2013 to 368 in 2016). We calculate the average city-level daily AQI and concentrations of each pollutant from 2013 to 2017. Figure 4 exhibits the average AQI and the dates of marathon games based on the forty-day moving average method, which reflects a strong seasonality related to the values of AQI. The results based on daily data in Figure A1 of the appendix are much the same. The vertical red lines represent the dates of marathon games, which reveals that most games are organised in winters when temperatures are low. In Figure A2 of the appendix, we also divide cities into two groups by considering if marathons were first held before or after 2015, and the daily average of AQI in these two groups also shows similar trends.
Weather. Daily weather data are collected from the National Climatic Data Center under the National Oceanic and Atmospheric Administration (NOAA) of the United States, which contains temperature, wind speed, precipitation, dew point, and station pressure recorded by 499 weather monitoring stations around China on consecutive days. Table 1 displays the descriptive statistics of the key variables related to air pollution and weather. On average, the AQI of marathon cities is 78, and the PM2.5 and PM10 arrive at 51 and 88 μ/m3, respectively.
Variable | Obs. | Mean | Std. Dev. | Min. | Max. |
AQI | 83, 711 | 78.19 | 46.12 | 0 | 497.90 |
PM2.5 (μ/m3) | 83, 711 | 50.74 | 39.13 | 0 | 878.82 |
PM10 (μ/m3) | 83, 711 | 87.85 | 60.06 | 0 | 1586.01 |
Average temperature (°F) | 83, 711 | 62.11 | 16.31 | -14.95 | 97.03 |
Dew Point (°F) | 83, 711 | 50.74 | 19.36 | -24.30 | 83.40 |
Wind speed (km/h) | 83, 711 | 5.12 | 2.45 | 0 | 37.35 |
Air pressure (pa) | 83, 711 | 676.10 | 388.62 | 0 | 1037.03 |
Precipitation (mm) | 83, 711 | 1.66 | 10.60 | 0 | 99.90 |
Holiday | 83, 711 | 0.05 | 0.22 | 0 | 1.00 |
Note: Data are collected and calculated by authors. |
Local government leaders. We manually collect the characteristics of municipal secretaries and mayors such as education, age and tenure from the internet. Table 2 is the summary statistics of local leaders from 2013 to 2017.
Variable | Obs. | Mean | Std. Dev. |
Panel A: Municipal secretary | |||
Age (year) | 78, 199 | 53.67 | 3.50 |
Tenure (year) | 78, 199 | 2.09 | 1.62 |
Second term indicator (tenure > 5) | 78, 199 | 0.05 | 0.22 |
Panel A: Municipal secretary | |||
Education indicator (PhD = 1, lower than PhD = 0) | 78, 199 | 0.35 | 0.48 |
Turnover (Within 6 months before and after the marathon games = 1, others = 0) | 78, 199 | 0.36 | 0.48 |
Promotion (Promoted to higher level next term = 1, others = 0) | 51, 051 | 0.48 | 0.50 |
Panel B: Mayor | |||
Age (year) | 78, 657 | 52.48 | 3.89 |
Tenure (year) | 78, 657 | 2.08 | 1.58 |
Second term indicator (tenure > 5) | 78, 657 | 0.04 | 0.20 |
Education indicator (PhD = 1, lower = 0) | 78, 657 | 0.23 | 0.42 |
Turnover (Within 6 months before and after the marathon dates = 1, others = 0) | 78, 657 | 0.35 | 0.48 |
Promotion (Promoted to higher level next tenure = 1, others = 0) | 54, 851 | 0.67 | 0.47 |
Note: Data are collected and calculated by authors. |
Firstly, we estimate the impact of air pollution within an 11-day window based on Eq (1):
log(Pit)=αit++5∑t=−5βitDti×decentralize+f(ti)+Γt+Wit+εit | (1) |
where decentralize measures the effect of decentralization on air pollution, and it equals 1 if the year of new marathon games that are first held is after 2015, otherwise 0.Dit denotes variables related to air pollution such as AQI, PM2.5 and PM10 in city i at the tth day before or after marathon games. αit represents city-year fixed effects, which can capture time-varying factors within city-level. D−ti and D+ti equal 1 for city i in the tth day before or after marathon games, separately. D0i, which is the variable we are most interested in, equals 1 for city i in the day of marathon games, otherwise 0. βit depicts the dynamic effects before, during or after the games within an 11-day window; f(ti) includes a series of city-specific polynomial time trends from the first to third orders, which controls the non-linear time-varying confounding variables across cities such as nonlinear dynamic factors or unexpected time shocks [29,30,31,32]; Γt contains holiday, year-city, week and day of week fixed effects. To control other un-observed variations across cities during marathon days, we include a marathon date dummy which equals 1 at the date when any one of the cities have marathon games. Wit is a vector of weather control variables as mentioned in the previous section. εit is an error term.
As a preliminary test, in Figure 5, we first visualize the dynamic effects of marathon on PM2.5 and PM10 based on Eq (1). In Figure 5(A), the graph implies that the levels of PM2.5 and PM10 decrease significantly on the day of marathon. In Figure 5(B), we include co-pollutants of CO, NO2, SO2, and O3, and the graph presents similar results. These findings suggest that air pollution decreases during marathon games, which also is consistent with the initiation that air quality during marathon days will improve because of less traffic flow. In Table A1 of the appendix, we also report the effects of marathon games on local AQI within an 11-day window from 2013 to 2017 based on different control variables, and the results are much the same.
In this section, we first estimate the effects of authority decentralization on air pollution by considering subsamples of marathon games that were first held before or after decentralization since 2015, separately. The dynamic results in Figure 6(A) imply that the level of AQI during the days of marathons that are first organised before decentralisation does not change significantly. The results based on PM2.5 and PM10 in Figure 6(B) are much the same. On the contrary, the results in Figure 6(C), (D) illustrate that air quality during the days of marathon games that are first held after decentralisation improves significantly. The preliminary results in Figure 6 show that authority decentralization has significant negative effects on reducing air pollution.
Next, we formally consider the relationship between marathon decentralization and local air quality based on Eq (1). To attenuate the endogenous problem such that the improvement of air quality may be affected by leaders' deliberate choices of marathon days, we construct an instrument variable (IV) based on marathon temperature. Specifically, a city that wants to hold marathon games should choose the date that meets the requirements of suitable temperatures. According to the "Grading approach of Marathon and related sporting events" released by the CAA, the suitable temperature for marathon runners is around 15 ℃. The results in Figure A3 of the appendix show that the average temperatures on the days of marathon games are around 15 ℃. We therefore construct IV based on suitable temperature: if temperature is between 5 and 25 ℃, the value of IV is one, otherwise 0. We also choose the temperature interval of 5–15 ℃ as a robustness check. The results based on 2SLS model are reported in Table 3, which reveal a negative impact of decentralization on air pollution. Specifically, the authority decentralization of marathon games leads to the decrease of air pollution during the days of marathon games (D0 × decentralize). Overall, our basic results in Table 3 prove that the air pollution during marathon games held after decentralization will significantly decrease by around 10–20%.
(1) | (2) | |
Temp5-25 IV | Temp5-15 IV | |
D–5 × decentralize | -0.07 | -0.03 |
(-1.63) | (-0.41) | |
D–4 × decentralize | -0.04 | 0.04 |
(-0.73) | (0.47) | |
D–3 × decentralize | -0.09** | 0.01 |
(-2.02) | (0.16) | |
D–2 × decentralize | -0.09* | -0.06 |
(-1.95) | (-0.57) | |
D–1 × decentralize | -0.05 | -0.10 |
(-1.20) | (-1.19) | |
D0 × decentralize | -0.11*** | -0.20** |
(-2.72) | (-2.33) | |
D+1 × decentralize | -0.10** | -0.15* |
(-2.31) | (-1.71) | |
D+2 × decentralize | -0.05 | 0.09 |
(-1.09) | (1.03) | |
D+3 × decentralize | -0.06 | 0.07 |
(-1.33) | (0.81) | |
D+4 × decentralize | -0.03 | 0.04 |
(-0.61) | (0.39) | |
D+5 × decentralize | -0.04 | 0.02 |
(-0.80) | (0.17) | |
Weather | YES | YES |
City-year FE | YES | YES |
Week FE | YES | YES |
Day of week FE | YES | YES |
Obs. | 83, 578 | 83, 578 |
R2 | 0.48 | 0.48 |
First stage results | ||
Temp × decentralize | 0.57*** | 0.58*** |
(9.45) | (10.37) | |
F-Stat. | 23.79 | 7.64 |
Note: Robust t-statistics are reported in parentheses. Standard errors are clustered at the city level. *** p < 0.01, ** p < 0.05, * p < 0.1. |
One concern about the robustness of the results in Section 3.3 is that the negative effects of decentralization on air pollution during the days of marathon might be found by chance. In this section, we try to attenuate such concerns by replacing the true marathon dates with randomly selected ones for 1000 times within the same cities and years as a placebo test. The distribution of the coefficients of the interactive terms in Eq (1) based on fake marathon dates is depicted in Figure 7(A), which turns out that the true coefficient is at the left end tail. Figure 7(B) further shows that only 50 out of 1000 cases of estimated terms have p-values at less than the 5% significant level, which further confirms the robustness of our results.
We argue that the negative relationship between authority decentralization and air pollution might be caused by cadre incentives and political cycles of local leaders. In this section, we empirically explore some potential mechanisms based on these ideas.
Local leaders with higher possibility of promotion might be more willing to take actions to improve air quality during the days of marathon games after decentralization. Specifically, local leaders with stronger career concerns will make greater effort to reduce air pollution to show their capabilities. We consider if it is the promotion of local leaders associated with authority decentralization that leads to the improvement of air quality by introducing Eq (2):
log(Pit)=αit++5∑t=−5βitDti×decentralizeit×promoteit+age+edu+tenure+f(ti)+Γt+Wit+εit | (2) |
where promote is 1 if leader of city i is promoted in the next term, otherwise 0. 6, 7We control several characteristics of leaders such as age (in years), temire (working years) and education (1 if PhD, 0 otherwise).
6 In China, a dual political system of communist party and government organs exists among each level of the political hierarchy. At a city-level, the top position is a municipal party secretary, followed immediately by a mayor.
7 Follow the definition of Li and Zhou (2005), for a municipal secretary, we consider the following position changes as promotions: (1) Member of Standing Committee of Provincial Committee (MSCPC); (2) Provincial (vice-) Governor or Provincial (vice-) Secretary; (3) Any other positions that are equal to, or above, the MSCPC. As for a mayor, besides these three positions, we also consider becoming municipal secretary as a promotion in his political rank. As Chinese political officials have few options available outside the political system, we define other situations of local leaders as non-promotional, which includes moving to a same-level position, retirement, or demotion.
The results in Columns (1) and (2) of Table 4 proves that when the promotion probability of city leader is high, the air pollution during marathon date will significantly decrease after marathon decentralization. Such results imply that promotion incentive is an important factor related to the reduction of air pollution after authority decentralization. In Table A2 of the appendix, the estimations based on 2SLS model are also similar.
Municipal secretary | Mayor | |
(1) | (2) | |
Log (AQI) | ||
D0 × promote × decentralize | -0.18*** | -0.14*** |
(-3.12) | (-2.74) | |
Obs. | 50, 883 | 54, 673 |
R2 | 0.47 | 0.48 |
Log (PM2.5) | ||
D0 × promote × decentralize | -0.26*** | -0.18** |
(-3.36) | (-2.58) | |
Obs. | 50, 883 | 54, 673 |
R2 | 0.46 | 0.47 |
Log (PM10) | ||
D0 × promote × decentralize | -0.20*** | -0.15*** |
(-2.76) | (-2.61) | |
Obs. | 50, 883 | 54, 673 |
R2 | 0.50 | 0.52 |
Weather | YES | YES |
City-year FE | YES | YES |
Week FE | YES | YES |
Day of week FE | YES | YES |
Note: For simplicity, the table only shows the coefficients of interactions during marathon games. Robust t-statistics are reported in parentheses. Standard errors are clustered at the city level. *** p < 0.01, ** p < 0.05, * p < 0.1. |
Political cycles are essential for local economic development. The motivations of the incumbent and newcomers are not the same because of the huge variation in promotion incentives, thus might also affect the changes of air quality during marathon games after authority decentralization. To test such argument, we set a dummy as turnover: if the transition of local leaders happened within six months before or after the dates of a marathon game, its value is 1, otherwise 0. The coefficients in Columns (1) and (2) reveal that if cities are undergoing local leader turnovers, the effects of authority decentralization will become larger. Specifically, the level of air pollution during marathon significantly decreases by about 18% and 14%, respectively, when secretaries or mayors are about to change. In Table A3 of the appendix, the results based on 2SLS model are much the same. Such results confirm that the reduction of air pollution during marathon days has a close relationship with political cycles.
Municipal secretary | Mayor | |
(1) | (2) | |
Log (AQI) | ||
D0 × turnover × decentralize | -0.18*** | -0.14*** |
(-3.68) | (-2.88) | |
Obs. | 78, 010 | 78, 472 |
R2 | 0.48 | 0.48 |
Log (PM2.5) | ||
D0 × turnover × decentralize | -0.23*** | -0.19*** |
(-3.33) | (-2.76) | |
Obs. | 78, 010 | 78, 472 |
R2 | 0.47 | 0.46 |
Log (PM10) | ||
D0 × turnover × decentralize | -0.21*** | -0.14*** |
(-3.37) | (-2.64) | |
Obs. | 78, 010 | 78, 472 |
R2 | 0.51 | 0.51 |
Weather | YES | YES |
City-year FE | YES | YES |
Week FE | YES | YES |
Day of week FE | YES | YES |
Note: For simplicity, the table only shows the coefficients of interactions during marathon games. Robust t-statistics are reported in parentheses. Standard errors are clustered at the city level. *** p < 0.01, ** p < 0.05, * p < 0.1. |
To further disentangle the heterogenous effects of political cycles on reducing air pollution after decentralization, we separately estimate the effects of new or old leaders based on the idea of Liu et al. [33] and Li et al. [34]. We construct a dummy BEF, which is 1 if the dates of marathon are less than 12 months before the leave of the incumbent leaders, and otherwise 0. The dummy variable AFT is 1 if the dates of marathon are less than 12 months after new leaders take office, and otherwise 0. The results in Table 6 show that after marathon decentralization, leaders that will leave soon have stronger motivation to take actions to improve air quality during the days of marathon games. These findings imply that there is a stronger promotion incentive of local leaders to perform well when the probability of political turnover is higher. The results in Table 6 also prove the asymmetric effects of political turnovers on the improvement of air quality.
Municipal secretary | Mayor | |||
(1) OLS |
(2) IV |
(3) OLS |
(4) IV |
|
Log (AQI) | ||||
D0 × BEF × year2015 | -0.20*** | -0.29*** | -0.21*** | -0.23*** |
(-3.96) | (-3.86) | (-4.27) | (-2.69) | |
D0 × AFT × year2015 | -0.01 | -0.05 | 0.08 | -0.08 |
(-0.33) | (-0.73) | (1.52) | (-0.91) | |
Obs. | 78, 010 | 78, 010 | 78, 472 | 78, 472 |
R2 | 0.48 | 0.48 | 0.48 | 0.48 |
Log (PM2.5) | ||||
D0 × BEF × decentralize | -0.27*** | -0.43*** | -0.31*** | -0.37*** |
(-3.88) | (-4.09) | (-4.37) | (-3.26) | |
D0 × AFT × decentralize | -0.01 | -0.13 | 0.14* | -0.11 |
(-0.13) | (-1.28) | (1.96) | (-0.87) | |
Obs. | 78, 010 | 78, 010 | 78, 472 | 78, 472 |
R2 | 0.47 | 0.47 | 0.46 | 0.46 |
Log (PM10) | ||||
D0 × BEF × decentralize | -0.22*** | -0.39*** | -0.22*** | -0.28*** |
(-3.42) | (-4.03) | (-3.67) | (-2.74) | |
D0 × AFT × decentralize | -0.01 | -0.02 | 0.10 | -0.06 |
(-0.27) | (-0.20) | (1.60) | (-0.56) | |
Obs. | 78, 010 | 78, 010 | 78, 472 | 78, 472 |
R2 | 0.51 | 0.51 | 0.51 | 0.51 |
Weather | YES | YES | YES | YES |
City-year FE | YES | YES | YES | YES |
Week FE | YES | YES | YES | YES |
Day of week FE | YES | YES | YES | YES |
Note: The instrumental variable is temperature dummy with 5–25–degree interval. For simplicity, the table only shows the coefficients of interactions at the marathon date. Robust t-statistics are reported in parentheses. Standard errors are clustered at the city level. F statistic is for the Kleibergen-Paap rk Wald test. *** p < 0.01, ** p < 0.05, * p < 0.1. |
As have been proved in previous sections, the effects of authority decentralization on air pollution might be affected by political returns, which reflects the career concerns of city leaders. In this section, we use leaders' age as a proxy to measure career concern and its effects on reducing air pollution during marathon games after decentralization. In Chinese political system, prefectural leaders who are not promoted to higher levels usually will retire at the age of 65. Therefore, local leaders will have stronger motivation to reduce air pollution after authority decentralization with the increase of age before retirement. The results in Columns (1) and (2) of Table 7 indicate that air quality during the dates of marathon game will significantly improve in cities with the increase of leaders' age after marathon decentralization. Specifically, local AQI will decrease by 0.2% with the increase of leaders' age after decentralization. The results based on IV model in Table A4 of the appendix are similar.
Municipal secretary | Mayor | |
(1) | (2) | |
Log (AQI) | ||
D0 × age × decentralize | -0.002*** | -0.002*** |
(-3.43) | (-3.32) | |
Obs. | 78, 010 | 78, 472 |
R2 | 0.48 | 0.48 |
Log (PM2.5) | ||
D0 × age × decentralize | -0.003*** | -0.002*** |
(-3.09) | (-3.16) | |
Obs. | 78, 010 | 78, 472 |
R2 | 0.47 | 0.47 |
Log (PM10) | ||
D0 × age × decentralize | -0.002*** | -0.002*** |
(-3.36) | (-2.66) | |
Obs. | 78, 010 | 78, 472 |
R2 | 0.51 | 0.51 |
Weather Controls | YES | YES |
City-year FE | YES | YES |
Week FE | YES | YES |
Day of week FE | YES | YES |
Note: For simplicity, the table only shows the coefficients of interactions at the marathon date. Robust t-statistics are reported in parentheses. Standard errors are clustered at the city level. *** p < 0.01, ** p < 0.05, * p < 0.1. |
Overall, the empirical results suggest that the impact of decentralization on reducing air pollution during the dates of marathon has a close relationship with the political incentives of local leaders. Some explanations are in order. While air quality improves significantly after decentralization, the duration of the effects is short. Almost at the end of marathon games, the coefficients of the interaction terms are not significant anymore. The U-shaped dynamic effects imply the necessity to adjust the decentralization policy to overcome the possible opportunistic behaviours of local governments.
In this study, we investigate the impacts of authority decentralization on reducing air pollution based on the policy of decentralizing the right to hold marathon games by local governments. The empirical results show that during marathon games, local air quality improves significantly. Moreover, the reduction of local air pollution during the days of marathon is mainly caused by the authority decentralization since 2015. We also find that the effect of authority decentralization on reducing air pollution is closely linked to the incentives of local leaders.
In the future, more detailed data need to be used to consider to what extent authority decentralization can have influence on the behaviours of local governments. Firstly, the long-term impact of authority decentralization on air pollution can be identified based on updated data related to local air pollution. Secondly, the incentives of local governments need to be further studied by considering spill-over effects and competing effects across different cities. Finally, the incentives of air pollution reduction induced by decentralization may exist in other field related to city management such as driving restriction, which can also be studied to better understand the effects of authority decentralization on air pollution.
This study was financially supported by the Postgraduate Scientific Research Innovation Project of Hunan Province (CX20220384).
The authors declare that they have no known competing financial interests or personal relationships that could have appeared to influence the work reported in this paper.
(1) | (2) | (3) | (4) | (5) | (6) | (7) | |
D-5 | -0.024 | -0.024 | -0.023 | -0.023 | -0.011 | -0.028 | -0.031 |
(-1.11) | (-1.11) | (-1.08) | (-1.08) | (-0.53) | (-1.35) | (-1.44) | |
D-4 | -0.003 | -0.004 | -0.002 | -0.002 | 0.003 | -0.012 | -0.013 |
(-0.12) | (-0.18) | (-0.09) | (-0.10) | (0.11) | (-0.54) | (-0.54) | |
D-3 | -0.045** | -0.047** | -0.044* | -0.044* | -0.044* | -0.052** | -0.055** |
(-1.98) | (-2.08) | (-1.94) | (-1.94) | (-1.96) | (-2.48) | (-2.41) | |
D-2 | -0.050** | -0.052** | -0.049** | -0.049** | -0.050** | -0.057*** | -0.061** |
(-2.05) | (-2.14) | (-2.02) | (-2.02) | (-2.04) | (-2.69) | (-2.53) | |
D-1 | -0.027 | -0.029 | -0.027 | -0.027 | -0.023 | -0.028 | -0.041* |
(-1.25) | (-1.35) | (-1.22) | (-1.22) | (-1.03) | (-1.45) | (-1.88) | |
D0 | -0.056** | -0.056** | -0.055** | -0.055** | -0.043* | -0.042** | -0.057** |
(-2.47) | (-2.46) | (-2.44) | (-2.44) | (-1.89) | (-2.01) | (-2.49) | |
D+1 | -0.052** | -0.051** | -0.051** | -0.051** | -0.035 | -0.024 | -0.044** |
(-2.37) | (-2.33) | (-2.34) | (-2.34) | (-1.61) | (-1.22) | (-1.99) | |
D+2 | -0.024 | -0.025 | -0.024 | -0.024 | -0.012 | -0.016 | -0.022 |
(-1.16) | (-1.17) | (-1.13) | (-1.13) | (-0.58) | (-0.85) | (-1.05) | |
D+3 | -0.027 | -0.029 | -0.026 | -0.026 | -0.019 | -0.013 | -0.020 |
(-1.21) | (-1.30) | (-1.18) | (-1.19) | (-0.86) | (-0.62) | (-0.90) | |
D+4 | -0.006 | -0.009 | -0.006 | -0.006 | -0.005 | 0.007 | 0.003 |
(-0.28) | (-0.40) | (-0.24) | (-0.25) | (-0.21) | (0.31) | (0.13) | |
D+5 | -0.010 | -0.012 | -0.009 | -0.009 | -0.012 | -0.004 | -0.011 |
(-0.39) | (-0.49) | (-0.37) | (-0.37) | (-0.49) | (-0.18) | (-0.43) | |
Weather | YES | YES | YES | YES | YES | YES | YES |
Holiday | YES | YES | YES | YES | YES | YES | |
Year-City FE | YES | YES | YES | YES | YES | YES | |
Week FE | YES | YES | YES | YES | YES | ||
Day of week FE | YES | YES | YES | YES | YES | YES | |
Month FE | YES | ||||||
Date FE | YES | ||||||
Marathon date dummy | YES | ||||||
City time trends | No | 1st | 2nd | 3rd | 1st | 1st | 1st |
Obs. | 83, 578 | 83, 578 | 83, 578 | 83, 578 | 83, 578 | 83, 589 | 83, 578 |
Adj. R2 | 0.481 | 0.481 | 0.481 | 0.481 | 0.467 | 0.548 | 0.483 |
Note: Robust t-statistics are reported in parentheses. Standard errors are clustered at the city level. *** p < 0.01, ** p < 0.05, * p < 0.1. |
Municipal secretary | Mayor | |||
(1) | (2) | (3 | (5) | |
OLS | IV | OLS | IV | |
Log (AQI) | ||||
D0 × promote × decentralize | -0.171*** | -0.217* | -0.132*** | -0.153* |
(-3.05) | (-1.92) | (-2.68) | (-1.82) | |
Obs. | 50, 883 | 50, 883 | 54, 673 | 54, 673 |
R2 | 0.474 | 0.474 | 0.484 | 0.484 |
Log (PM2.5) | ||||
D0 × promote × decentralize | -0.256*** | -0.372*** | -0.174** | -0.252** |
(-3.30) | (-2.66) | (-2.53) | (-2.25) | |
Obs. | 50, 883 | 50, 883 | 54, 673 | 54, 673 |
R2 | 0.460 | 0.460 | 0.470 | 0.470 |
Log (PM10) | ||||
D0 × promote × decentralize | -0.191*** | -0.278** | -0.142** | -0.242** |
(-2.69) | (-2.23) | (-2.53) | (-2.38) | |
Obs. | 50, 883 | 50, 883 | 54, 673 | 54, 673 |
R2 | 0.502 | 0.502 | 0.515 | 0.515 |
First stage results | ||||
Temp × promote × decentralize | 0.508*** | 0.448*** | ||
(6.97) | (8.96) | |||
F-Stat. | 48.60 | 80.23 | ||
Weather Controls | YES | YES | YES | YES |
City-year FE | YES | YES | YES | YES |
Week FE | YES | YES | YES | YES |
Day of week FE | YES | YES | YES | YES |
Note: The results are based on the equation as follows: log(Pit)=αit+βiD0idecentralize×incentivei+age+edu+tenure+f(ti)+Γt+Wit+εit The instrumental variable is 5–25–degree temperature dummy. For simplicity, the table only shows the coefficients of interactions during marathon games. Robust t-statistics are reported in parentheses. Standard errors are clustered at the city level. *** p < 0.01, ** p < 0.05, * p < 0.1. |
Municipal secretary | Mayor | |||
(1) | (2) | (3) | (4) | |
OLS | IV | OLS | IV | |
Log (AQI) | ||||
D0 × turnover × decentralize | -0.178*** | -0.216*** | -0.134*** | -0.221*** |
(-3.63) | (-2.99) | (-2.90) | (-2.79) | |
Obs. | 78, 010 | 78, 010 | 78, 472 | 78, 472 |
R2 | 0.480 | 0.480 | 0.479 | 0.479 |
Log (PM2.5) | ||||
D0 × turnover × decentralize | -0.220*** | -0.360*** | -0.183*** | -0.324*** |
(-3.28) | (-3.67) | (-2.79) | (-2.96) | |
Obs. | 78, 010 | 78, 010 | 78, 472 | 78, 472 |
R2 | 0.465 | 0.465 | 0.464 | 0.464 |
Log (PM10) | ||||
D0 × turnover × decentralize | -0.205*** | -0.289*** | -0.141*** | -0.230** |
(-3.31) | (-3.06) | (-2.66) | (-2.51) | |
Obs. | 78, 010 | 78, 010 | 78, 472 | 78, 472 |
R2 | 0.508 | 0.507 | 0.506 | 0.506 |
First stage results | ||||
Temp × turnover × decentralize | 0.568*** | 0.570*** | ||
(9.43) | (10.27) | |||
F-Stat. | 88.89 | 105.50 | ||
Weather Controls | YES | YES | YES | YES |
City-year FE | YES | YES | YES | YES |
Week FE | YES | YES | YES | YES |
Day of week FE | YES | YES | YES | YES |
Note: The results are based on the equation as follows: log(Pit)=αit+βiD0idecentralizei×incentivei+age+edu+tenure+f(ti)+Γt+Wit+εit The instrumental variable is temperature dummy with 5-25-degree interval. For simplicity, the table only shows the coefficients of interactions at the marathon date. Robust t-statistics are reported in parentheses. Standard errors are clustered at the city level. *** p < 0.01, ** p < 0.05, * p < 0.1. |
Municipal secretary | Mayor | |||
(1) | (2) | (3) | (4) | |
OLS | IV | OLS | IV | |
Log (AQI) | ||||
D0 × age × decentralize | -0.002*** | -0.004*** | -0.002*** | -0.004*** |
(-3.39) | (-3.45) | (-3.29) | (-3.14) | |
Obs. | 78, 010 | 78, 010 | 78, 472 | 78, 472 |
R2 | 0.480 | 0.480 | 0.479 | 0.479 |
Log (PM2.5) | ||||
D0 × age × decentralize | -0.003*** | -0.006*** | -0.003*** | -0.006*** |
(-3.06) | (-3.95) | (-2.97) | (-3.70) | |
Obs. | 78, 010 | 78, 010 | 78, 472 | 78, 472 |
R2 | 0.465 | 0.465 | 0.464 | 0.464 |
Log (PM10) | ||||
D0 × age × decentralize | -0.002*** | -0.004*** | -0.002*** | -0.004*** |
(-3.33) | (-3.56) | (-3.11) | (-3.12) | |
Obs. | 78, 010 | 78, 010 | 78, 472 | 78, 472 |
R2 | 0.508 | 0.507 | 0.506 | 0.506 |
First stage results | ||||
Temp × age × decentralize | 0.464*** | 0.439*** | ||
(13.24) | (12.89) | |||
F-Stat. | 175.28 | 166.08 | ||
Weather Controls | YES | YES | YES | YES |
City-year FE | YES | YES | YES | YES |
Week FE | YES | YES | YES | YES |
Day of week FE | YES | YES | YES | YES |
Note: The results are based on the equation as follows: log(Pit)=αit+βiD0idecentralizei×incentivei+age+ed+tenure+f(ti)+Γt+Wit+εit The instrumental variable is 5-25-degrees temperature dummy. For simplicity, table only shows the coefficients of interactions during marathon games. Robust t-statistics are reported in parentheses. Standard errors are clustered at the city level. *** p < 0.01, ** p < 0.05, * p < 0.1. |
[1] | Jackson PG, Steenfelt JS, Foged NN, et al. (2004) Evaluation of Bryozoan limestone properties based on in-situ and laboratory element tests. Geotech Geophys Site Charact 1813–1820. |
[2] | Foged NN (2008) Rock Mass Characterisation in Limestone. Lecture presented at Dansk Geoteknisk Forening Mode nr. 2 Generalforsamling. (Danish Geotechnical Society Meeting nr. 2 General assembly.) |
[3] | Foged NN, Hansen SL, Stabell S (2010) Developments in Rock Mass Evaluation of Limestone in Denmark. In: Rock mechanics in the Nordic countries, Norway: Kongberg. |
[4] | Galsgaard J. (2014) Flint in the Danian København Limestone Formation. (Flint in the Danian Copenhagen Limestone Formation.) Available from: https://www.geo.dk/media/1951/flint-in-the-danian-koebenhavn-limestone-formation_jgalsgaard_2014.pdf |
[5] | Jakobsen L, Foged NN, Erichsen L, et al. (2015) Face logging in Copenhagen Limestone, Denmark. Proceedings of the XVI ECSMGE Geotechnical Engineering for Infrastructure and Development, 2939–2944. |
[6] | Hansen SL, Galsgaard J, Foged NN (2015) Rock mass characterization for Copenhagen Metro using face logs. SEE TUNNEL-Promoting tunnelling in SE European region, Presented at: 41st General Assembly and Congress of International Tunneling and Underground Space. |
[7] | Katić N, Christensen HF (2014). Upscaling elastic moduli in Copenhagen Limestone. In Rock Engineering and Rock Mechanics: Structures in and on Rock Masses: Proceedings of EUROCK 2014, ISRM European Regional Symposium, London: Taylor & Francis Group, 235–240. |
[8] | Katić N, Christensen HF (2015) Composite Elasticity of Copenhagen Limestone. In Proceedings of the ISRM Regional Symposium EUROCK 2015 & 64th Geomechanics Colloquium-Future Development of Rock Mechanics, Salzburg: Austrian Society for Geomechanics, 451–456. |
[9] | Vangkilde-Pedersen T, Mielby S, Jakobsen PR, et al. (2011) Kortlægning af kalkmagasiner. GEUS. Geo-vejledning 8. De nationale geologiske undersøgelser for Danmark og Grønland. Ministeriet for klima og energy. (Mapping of limestone reservoirs. GEUS. Geo-guidance 8. National geological investigations for Denmark and Greenland. Ministry of climate and energy.) Available from: gk.geus.info/xpdf/geovejledning_8_kalk_final_net.pdf. |
[10] |
Bjerager M, Surlyk F (2007) Danian Cool-Water Bryozoan Mounds at Stevns Klint, Denmark–A New Class of Non-Cemented Skeletal Mounds. J Sediment Res 77:634–660. doi: 10.2110/jsr.2007.064
![]() |
[11] |
Japsen P, Bidstrup T, Lidmar-Berström K (2002) Neogene uplift and erosion of southern Scandinavia induced by the rise of the South Swedish Dome. Geol Soc 196: 183–207. doi: 10.1144/GSL.SP.2002.196.01.12
![]() |
[12] | Andersen TB, Møgaard MR (2018) Copenhagen Area Overview of the geological conditions in the Copenhagen area and surrounding areas. GeoAtlas Live Documentation. Report 1. Available from: http://wgn.geo.dk/geodata/modeldokumentation/Copenhagen%20_25m_2018-07-12.pdf |
[13] | Olsen H, Nielsen UT (2002) Logstratigrafisk inddeling af kalken I Københavns-området. In Frederiksen JK, Eriksen FS, Hansen HK, et al., editors. Ingeniørgeologiske forhold i København. Dgf-Bulletin 19, Danish Geotechnical Society. (Logstratigraphical division of limestone in Copenhagen Area. In Frederiksen JK, Eriksen FS, Hansen HK, et al., editors. Engineering-geological conditions in Copenhagen. Dgf-Bulletin 19, Danish Geotechnical Society.) |
[14] | Damholt T, Surlyk F (2012) Nomination of Stevns Klint for inclusion in the World Heritage List. Østsjællands Museum. Pedersen AS (2011) Rockfalls at Stevns Klint. Landslide hazard assessment based on photogrametrical supported geological analysis of the limestone cliff Stevns Klint in eastern Denmark. Danmarks og Grønlands Geologiske Undersøgelse Rapport 2011/93 (Danmark's and Greenland's Geological Investigaton Report 2011/93). Available from: https://whc.unesco.org/uploads/nominations/1416.pdf. |
[15] | Mortensen N, Hansen HK, Hansen PB (1999) Malmö Citytunneln.Rock Mechanical Description. Limestone. Malmö (SE). 32p. Report 1, 22 March 1999, Revision 1. DGI job No. 155 16092. Geo archive. |
[16] | Jørgensen NO (1975) Mg/Sr distribution and diagenesis of Maastrichtian white chalk and Danian bryozoan limestone from Jylland, Denmark. Bull Geol Soc Den 24: 299–325. |
[17] | Rosenbom A, Jakobsen PR (2000) Kalk, Sprækker og Termografi. Geol Nyt fra GEUS 3: 1–8. (Chalk, Fractures and Thermography. Geol News From GEUS 3: 1–8.) |
[18] | Surlyk F, Damholt T, Bjerager M (2006) Stevns Klint, Denmark: uppermost Maastrichtian chalk, Cretaceous-Tertiary boundary, and lower Danian bryozoan mound complex, Geological Society of Denmark, 54: 1–48. |
[19] | Hoek E, Carter TG, Diedrichs MS (2013) Quantification of the Geological Strength Index Chart. 47th US Rock Mechanics/Geomechanics Symposium, 1757–1764. |
[20] |
Eberli GP, Beachle TG, Anselmetti FS, et al. (2003) Factors controlling elastic properties in carbonate sediments and rocks. Lead Edge 22: 654–660. doi: 10.1190/1.1599691
![]() |
[21] |
Brigaud B, Vincent B, Durlet C, et al. (2010) Acoustic properties of ancient shallow-marine carbonates: effects of depositional environments and diagenetic processes (Middle Jurassic, Paris basin, France). J Sediment Res 80:791–807. doi: 10.2110/jsr.2010.071
![]() |
[22] | Bergdahl U, Steenfelt JS (1994) Digest report on strength and deformation properties of Copenhagen limestone, Swedish Geotechnical Institute/Danish Geotechnical Institute, 67. |
Variable | Obs. | Mean | Std. Dev. | Min. | Max. |
AQI | 83, 711 | 78.19 | 46.12 | 0 | 497.90 |
PM2.5 (μ/m3) | 83, 711 | 50.74 | 39.13 | 0 | 878.82 |
PM10 (μ/m3) | 83, 711 | 87.85 | 60.06 | 0 | 1586.01 |
Average temperature (°F) | 83, 711 | 62.11 | 16.31 | -14.95 | 97.03 |
Dew Point (°F) | 83, 711 | 50.74 | 19.36 | -24.30 | 83.40 |
Wind speed (km/h) | 83, 711 | 5.12 | 2.45 | 0 | 37.35 |
Air pressure (pa) | 83, 711 | 676.10 | 388.62 | 0 | 1037.03 |
Precipitation (mm) | 83, 711 | 1.66 | 10.60 | 0 | 99.90 |
Holiday | 83, 711 | 0.05 | 0.22 | 0 | 1.00 |
Note: Data are collected and calculated by authors. |
Variable | Obs. | Mean | Std. Dev. |
Panel A: Municipal secretary | |||
Age (year) | 78, 199 | 53.67 | 3.50 |
Tenure (year) | 78, 199 | 2.09 | 1.62 |
Second term indicator (tenure > 5) | 78, 199 | 0.05 | 0.22 |
Panel A: Municipal secretary | |||
Education indicator (PhD = 1, lower than PhD = 0) | 78, 199 | 0.35 | 0.48 |
Turnover (Within 6 months before and after the marathon games = 1, others = 0) | 78, 199 | 0.36 | 0.48 |
Promotion (Promoted to higher level next term = 1, others = 0) | 51, 051 | 0.48 | 0.50 |
Panel B: Mayor | |||
Age (year) | 78, 657 | 52.48 | 3.89 |
Tenure (year) | 78, 657 | 2.08 | 1.58 |
Second term indicator (tenure > 5) | 78, 657 | 0.04 | 0.20 |
Education indicator (PhD = 1, lower = 0) | 78, 657 | 0.23 | 0.42 |
Turnover (Within 6 months before and after the marathon dates = 1, others = 0) | 78, 657 | 0.35 | 0.48 |
Promotion (Promoted to higher level next tenure = 1, others = 0) | 54, 851 | 0.67 | 0.47 |
Note: Data are collected and calculated by authors. |
(1) | (2) | |
Temp5-25 IV | Temp5-15 IV | |
D–5 × decentralize | -0.07 | -0.03 |
(-1.63) | (-0.41) | |
D–4 × decentralize | -0.04 | 0.04 |
(-0.73) | (0.47) | |
D–3 × decentralize | -0.09** | 0.01 |
(-2.02) | (0.16) | |
D–2 × decentralize | -0.09* | -0.06 |
(-1.95) | (-0.57) | |
D–1 × decentralize | -0.05 | -0.10 |
(-1.20) | (-1.19) | |
D0 × decentralize | -0.11*** | -0.20** |
(-2.72) | (-2.33) | |
D+1 × decentralize | -0.10** | -0.15* |
(-2.31) | (-1.71) | |
D+2 × decentralize | -0.05 | 0.09 |
(-1.09) | (1.03) | |
D+3 × decentralize | -0.06 | 0.07 |
(-1.33) | (0.81) | |
D+4 × decentralize | -0.03 | 0.04 |
(-0.61) | (0.39) | |
D+5 × decentralize | -0.04 | 0.02 |
(-0.80) | (0.17) | |
Weather | YES | YES |
City-year FE | YES | YES |
Week FE | YES | YES |
Day of week FE | YES | YES |
Obs. | 83, 578 | 83, 578 |
R2 | 0.48 | 0.48 |
First stage results | ||
Temp × decentralize | 0.57*** | 0.58*** |
(9.45) | (10.37) | |
F-Stat. | 23.79 | 7.64 |
Note: Robust t-statistics are reported in parentheses. Standard errors are clustered at the city level. *** p < 0.01, ** p < 0.05, * p < 0.1. |
Municipal secretary | Mayor | |
(1) | (2) | |
Log (AQI) | ||
D0 × promote × decentralize | -0.18*** | -0.14*** |
(-3.12) | (-2.74) | |
Obs. | 50, 883 | 54, 673 |
R2 | 0.47 | 0.48 |
Log (PM2.5) | ||
D0 × promote × decentralize | -0.26*** | -0.18** |
(-3.36) | (-2.58) | |
Obs. | 50, 883 | 54, 673 |
R2 | 0.46 | 0.47 |
Log (PM10) | ||
D0 × promote × decentralize | -0.20*** | -0.15*** |
(-2.76) | (-2.61) | |
Obs. | 50, 883 | 54, 673 |
R2 | 0.50 | 0.52 |
Weather | YES | YES |
City-year FE | YES | YES |
Week FE | YES | YES |
Day of week FE | YES | YES |
Note: For simplicity, the table only shows the coefficients of interactions during marathon games. Robust t-statistics are reported in parentheses. Standard errors are clustered at the city level. *** p < 0.01, ** p < 0.05, * p < 0.1. |
Municipal secretary | Mayor | |
(1) | (2) | |
Log (AQI) | ||
D0 × turnover × decentralize | -0.18*** | -0.14*** |
(-3.68) | (-2.88) | |
Obs. | 78, 010 | 78, 472 |
R2 | 0.48 | 0.48 |
Log (PM2.5) | ||
D0 × turnover × decentralize | -0.23*** | -0.19*** |
(-3.33) | (-2.76) | |
Obs. | 78, 010 | 78, 472 |
R2 | 0.47 | 0.46 |
Log (PM10) | ||
D0 × turnover × decentralize | -0.21*** | -0.14*** |
(-3.37) | (-2.64) | |
Obs. | 78, 010 | 78, 472 |
R2 | 0.51 | 0.51 |
Weather | YES | YES |
City-year FE | YES | YES |
Week FE | YES | YES |
Day of week FE | YES | YES |
Note: For simplicity, the table only shows the coefficients of interactions during marathon games. Robust t-statistics are reported in parentheses. Standard errors are clustered at the city level. *** p < 0.01, ** p < 0.05, * p < 0.1. |
Municipal secretary | Mayor | |||
(1) OLS |
(2) IV |
(3) OLS |
(4) IV |
|
Log (AQI) | ||||
D0 × BEF × year2015 | -0.20*** | -0.29*** | -0.21*** | -0.23*** |
(-3.96) | (-3.86) | (-4.27) | (-2.69) | |
D0 × AFT × year2015 | -0.01 | -0.05 | 0.08 | -0.08 |
(-0.33) | (-0.73) | (1.52) | (-0.91) | |
Obs. | 78, 010 | 78, 010 | 78, 472 | 78, 472 |
R2 | 0.48 | 0.48 | 0.48 | 0.48 |
Log (PM2.5) | ||||
D0 × BEF × decentralize | -0.27*** | -0.43*** | -0.31*** | -0.37*** |
(-3.88) | (-4.09) | (-4.37) | (-3.26) | |
D0 × AFT × decentralize | -0.01 | -0.13 | 0.14* | -0.11 |
(-0.13) | (-1.28) | (1.96) | (-0.87) | |
Obs. | 78, 010 | 78, 010 | 78, 472 | 78, 472 |
R2 | 0.47 | 0.47 | 0.46 | 0.46 |
Log (PM10) | ||||
D0 × BEF × decentralize | -0.22*** | -0.39*** | -0.22*** | -0.28*** |
(-3.42) | (-4.03) | (-3.67) | (-2.74) | |
D0 × AFT × decentralize | -0.01 | -0.02 | 0.10 | -0.06 |
(-0.27) | (-0.20) | (1.60) | (-0.56) | |
Obs. | 78, 010 | 78, 010 | 78, 472 | 78, 472 |
R2 | 0.51 | 0.51 | 0.51 | 0.51 |
Weather | YES | YES | YES | YES |
City-year FE | YES | YES | YES | YES |
Week FE | YES | YES | YES | YES |
Day of week FE | YES | YES | YES | YES |
Note: The instrumental variable is temperature dummy with 5–25–degree interval. For simplicity, the table only shows the coefficients of interactions at the marathon date. Robust t-statistics are reported in parentheses. Standard errors are clustered at the city level. F statistic is for the Kleibergen-Paap rk Wald test. *** p < 0.01, ** p < 0.05, * p < 0.1. |
Municipal secretary | Mayor | |
(1) | (2) | |
Log (AQI) | ||
D0 × age × decentralize | -0.002*** | -0.002*** |
(-3.43) | (-3.32) | |
Obs. | 78, 010 | 78, 472 |
R2 | 0.48 | 0.48 |
Log (PM2.5) | ||
D0 × age × decentralize | -0.003*** | -0.002*** |
(-3.09) | (-3.16) | |
Obs. | 78, 010 | 78, 472 |
R2 | 0.47 | 0.47 |
Log (PM10) | ||
D0 × age × decentralize | -0.002*** | -0.002*** |
(-3.36) | (-2.66) | |
Obs. | 78, 010 | 78, 472 |
R2 | 0.51 | 0.51 |
Weather Controls | YES | YES |
City-year FE | YES | YES |
Week FE | YES | YES |
Day of week FE | YES | YES |
Note: For simplicity, the table only shows the coefficients of interactions at the marathon date. Robust t-statistics are reported in parentheses. Standard errors are clustered at the city level. *** p < 0.01, ** p < 0.05, * p < 0.1. |
(1) | (2) | (3) | (4) | (5) | (6) | (7) | |
D-5 | -0.024 | -0.024 | -0.023 | -0.023 | -0.011 | -0.028 | -0.031 |
(-1.11) | (-1.11) | (-1.08) | (-1.08) | (-0.53) | (-1.35) | (-1.44) | |
D-4 | -0.003 | -0.004 | -0.002 | -0.002 | 0.003 | -0.012 | -0.013 |
(-0.12) | (-0.18) | (-0.09) | (-0.10) | (0.11) | (-0.54) | (-0.54) | |
D-3 | -0.045** | -0.047** | -0.044* | -0.044* | -0.044* | -0.052** | -0.055** |
(-1.98) | (-2.08) | (-1.94) | (-1.94) | (-1.96) | (-2.48) | (-2.41) | |
D-2 | -0.050** | -0.052** | -0.049** | -0.049** | -0.050** | -0.057*** | -0.061** |
(-2.05) | (-2.14) | (-2.02) | (-2.02) | (-2.04) | (-2.69) | (-2.53) | |
D-1 | -0.027 | -0.029 | -0.027 | -0.027 | -0.023 | -0.028 | -0.041* |
(-1.25) | (-1.35) | (-1.22) | (-1.22) | (-1.03) | (-1.45) | (-1.88) | |
D0 | -0.056** | -0.056** | -0.055** | -0.055** | -0.043* | -0.042** | -0.057** |
(-2.47) | (-2.46) | (-2.44) | (-2.44) | (-1.89) | (-2.01) | (-2.49) | |
D+1 | -0.052** | -0.051** | -0.051** | -0.051** | -0.035 | -0.024 | -0.044** |
(-2.37) | (-2.33) | (-2.34) | (-2.34) | (-1.61) | (-1.22) | (-1.99) | |
D+2 | -0.024 | -0.025 | -0.024 | -0.024 | -0.012 | -0.016 | -0.022 |
(-1.16) | (-1.17) | (-1.13) | (-1.13) | (-0.58) | (-0.85) | (-1.05) | |
D+3 | -0.027 | -0.029 | -0.026 | -0.026 | -0.019 | -0.013 | -0.020 |
(-1.21) | (-1.30) | (-1.18) | (-1.19) | (-0.86) | (-0.62) | (-0.90) | |
D+4 | -0.006 | -0.009 | -0.006 | -0.006 | -0.005 | 0.007 | 0.003 |
(-0.28) | (-0.40) | (-0.24) | (-0.25) | (-0.21) | (0.31) | (0.13) | |
D+5 | -0.010 | -0.012 | -0.009 | -0.009 | -0.012 | -0.004 | -0.011 |
(-0.39) | (-0.49) | (-0.37) | (-0.37) | (-0.49) | (-0.18) | (-0.43) | |
Weather | YES | YES | YES | YES | YES | YES | YES |
Holiday | YES | YES | YES | YES | YES | YES | |
Year-City FE | YES | YES | YES | YES | YES | YES | |
Week FE | YES | YES | YES | YES | YES | ||
Day of week FE | YES | YES | YES | YES | YES | YES | |
Month FE | YES | ||||||
Date FE | YES | ||||||
Marathon date dummy | YES | ||||||
City time trends | No | 1st | 2nd | 3rd | 1st | 1st | 1st |
Obs. | 83, 578 | 83, 578 | 83, 578 | 83, 578 | 83, 578 | 83, 589 | 83, 578 |
Adj. R2 | 0.481 | 0.481 | 0.481 | 0.481 | 0.467 | 0.548 | 0.483 |
Note: Robust t-statistics are reported in parentheses. Standard errors are clustered at the city level. *** p < 0.01, ** p < 0.05, * p < 0.1. |
Municipal secretary | Mayor | |||
(1) | (2) | (3 | (5) | |
OLS | IV | OLS | IV | |
Log (AQI) | ||||
D0 × promote × decentralize | -0.171*** | -0.217* | -0.132*** | -0.153* |
(-3.05) | (-1.92) | (-2.68) | (-1.82) | |
Obs. | 50, 883 | 50, 883 | 54, 673 | 54, 673 |
R2 | 0.474 | 0.474 | 0.484 | 0.484 |
Log (PM2.5) | ||||
D0 × promote × decentralize | -0.256*** | -0.372*** | -0.174** | -0.252** |
(-3.30) | (-2.66) | (-2.53) | (-2.25) | |
Obs. | 50, 883 | 50, 883 | 54, 673 | 54, 673 |
R2 | 0.460 | 0.460 | 0.470 | 0.470 |
Log (PM10) | ||||
D0 × promote × decentralize | -0.191*** | -0.278** | -0.142** | -0.242** |
(-2.69) | (-2.23) | (-2.53) | (-2.38) | |
Obs. | 50, 883 | 50, 883 | 54, 673 | 54, 673 |
R2 | 0.502 | 0.502 | 0.515 | 0.515 |
First stage results | ||||
Temp × promote × decentralize | 0.508*** | 0.448*** | ||
(6.97) | (8.96) | |||
F-Stat. | 48.60 | 80.23 | ||
Weather Controls | YES | YES | YES | YES |
City-year FE | YES | YES | YES | YES |
Week FE | YES | YES | YES | YES |
Day of week FE | YES | YES | YES | YES |
Note: The results are based on the equation as follows: log(Pit)=αit+βiD0idecentralize×incentivei+age+edu+tenure+f(ti)+Γt+Wit+εit The instrumental variable is 5–25–degree temperature dummy. For simplicity, the table only shows the coefficients of interactions during marathon games. Robust t-statistics are reported in parentheses. Standard errors are clustered at the city level. *** p < 0.01, ** p < 0.05, * p < 0.1. |
Municipal secretary | Mayor | |||
(1) | (2) | (3) | (4) | |
OLS | IV | OLS | IV | |
Log (AQI) | ||||
D0 × turnover × decentralize | -0.178*** | -0.216*** | -0.134*** | -0.221*** |
(-3.63) | (-2.99) | (-2.90) | (-2.79) | |
Obs. | 78, 010 | 78, 010 | 78, 472 | 78, 472 |
R2 | 0.480 | 0.480 | 0.479 | 0.479 |
Log (PM2.5) | ||||
D0 × turnover × decentralize | -0.220*** | -0.360*** | -0.183*** | -0.324*** |
(-3.28) | (-3.67) | (-2.79) | (-2.96) | |
Obs. | 78, 010 | 78, 010 | 78, 472 | 78, 472 |
R2 | 0.465 | 0.465 | 0.464 | 0.464 |
Log (PM10) | ||||
D0 × turnover × decentralize | -0.205*** | -0.289*** | -0.141*** | -0.230** |
(-3.31) | (-3.06) | (-2.66) | (-2.51) | |
Obs. | 78, 010 | 78, 010 | 78, 472 | 78, 472 |
R2 | 0.508 | 0.507 | 0.506 | 0.506 |
First stage results | ||||
Temp × turnover × decentralize | 0.568*** | 0.570*** | ||
(9.43) | (10.27) | |||
F-Stat. | 88.89 | 105.50 | ||
Weather Controls | YES | YES | YES | YES |
City-year FE | YES | YES | YES | YES |
Week FE | YES | YES | YES | YES |
Day of week FE | YES | YES | YES | YES |
Note: The results are based on the equation as follows: log(Pit)=αit+βiD0idecentralizei×incentivei+age+edu+tenure+f(ti)+Γt+Wit+εit The instrumental variable is temperature dummy with 5-25-degree interval. For simplicity, the table only shows the coefficients of interactions at the marathon date. Robust t-statistics are reported in parentheses. Standard errors are clustered at the city level. *** p < 0.01, ** p < 0.05, * p < 0.1. |
Municipal secretary | Mayor | |||
(1) | (2) | (3) | (4) | |
OLS | IV | OLS | IV | |
Log (AQI) | ||||
D0 × age × decentralize | -0.002*** | -0.004*** | -0.002*** | -0.004*** |
(-3.39) | (-3.45) | (-3.29) | (-3.14) | |
Obs. | 78, 010 | 78, 010 | 78, 472 | 78, 472 |
R2 | 0.480 | 0.480 | 0.479 | 0.479 |
Log (PM2.5) | ||||
D0 × age × decentralize | -0.003*** | -0.006*** | -0.003*** | -0.006*** |
(-3.06) | (-3.95) | (-2.97) | (-3.70) | |
Obs. | 78, 010 | 78, 010 | 78, 472 | 78, 472 |
R2 | 0.465 | 0.465 | 0.464 | 0.464 |
Log (PM10) | ||||
D0 × age × decentralize | -0.002*** | -0.004*** | -0.002*** | -0.004*** |
(-3.33) | (-3.56) | (-3.11) | (-3.12) | |
Obs. | 78, 010 | 78, 010 | 78, 472 | 78, 472 |
R2 | 0.508 | 0.507 | 0.506 | 0.506 |
First stage results | ||||
Temp × age × decentralize | 0.464*** | 0.439*** | ||
(13.24) | (12.89) | |||
F-Stat. | 175.28 | 166.08 | ||
Weather Controls | YES | YES | YES | YES |
City-year FE | YES | YES | YES | YES |
Week FE | YES | YES | YES | YES |
Day of week FE | YES | YES | YES | YES |
Note: The results are based on the equation as follows: log(Pit)=αit+βiD0idecentralizei×incentivei+age+ed+tenure+f(ti)+Γt+Wit+εit The instrumental variable is 5-25-degrees temperature dummy. For simplicity, table only shows the coefficients of interactions during marathon games. Robust t-statistics are reported in parentheses. Standard errors are clustered at the city level. *** p < 0.01, ** p < 0.05, * p < 0.1. |
Variable | Obs. | Mean | Std. Dev. | Min. | Max. |
AQI | 83, 711 | 78.19 | 46.12 | 0 | 497.90 |
PM2.5 (μ/m3) | 83, 711 | 50.74 | 39.13 | 0 | 878.82 |
PM10 (μ/m3) | 83, 711 | 87.85 | 60.06 | 0 | 1586.01 |
Average temperature (°F) | 83, 711 | 62.11 | 16.31 | -14.95 | 97.03 |
Dew Point (°F) | 83, 711 | 50.74 | 19.36 | -24.30 | 83.40 |
Wind speed (km/h) | 83, 711 | 5.12 | 2.45 | 0 | 37.35 |
Air pressure (pa) | 83, 711 | 676.10 | 388.62 | 0 | 1037.03 |
Precipitation (mm) | 83, 711 | 1.66 | 10.60 | 0 | 99.90 |
Holiday | 83, 711 | 0.05 | 0.22 | 0 | 1.00 |
Note: Data are collected and calculated by authors. |
Variable | Obs. | Mean | Std. Dev. |
Panel A: Municipal secretary | |||
Age (year) | 78, 199 | 53.67 | 3.50 |
Tenure (year) | 78, 199 | 2.09 | 1.62 |
Second term indicator (tenure > 5) | 78, 199 | 0.05 | 0.22 |
Panel A: Municipal secretary | |||
Education indicator (PhD = 1, lower than PhD = 0) | 78, 199 | 0.35 | 0.48 |
Turnover (Within 6 months before and after the marathon games = 1, others = 0) | 78, 199 | 0.36 | 0.48 |
Promotion (Promoted to higher level next term = 1, others = 0) | 51, 051 | 0.48 | 0.50 |
Panel B: Mayor | |||
Age (year) | 78, 657 | 52.48 | 3.89 |
Tenure (year) | 78, 657 | 2.08 | 1.58 |
Second term indicator (tenure > 5) | 78, 657 | 0.04 | 0.20 |
Education indicator (PhD = 1, lower = 0) | 78, 657 | 0.23 | 0.42 |
Turnover (Within 6 months before and after the marathon dates = 1, others = 0) | 78, 657 | 0.35 | 0.48 |
Promotion (Promoted to higher level next tenure = 1, others = 0) | 54, 851 | 0.67 | 0.47 |
Note: Data are collected and calculated by authors. |
(1) | (2) | |
Temp5-25 IV | Temp5-15 IV | |
D–5 × decentralize | -0.07 | -0.03 |
(-1.63) | (-0.41) | |
D–4 × decentralize | -0.04 | 0.04 |
(-0.73) | (0.47) | |
D–3 × decentralize | -0.09** | 0.01 |
(-2.02) | (0.16) | |
D–2 × decentralize | -0.09* | -0.06 |
(-1.95) | (-0.57) | |
D–1 × decentralize | -0.05 | -0.10 |
(-1.20) | (-1.19) | |
D0 × decentralize | -0.11*** | -0.20** |
(-2.72) | (-2.33) | |
D+1 × decentralize | -0.10** | -0.15* |
(-2.31) | (-1.71) | |
D+2 × decentralize | -0.05 | 0.09 |
(-1.09) | (1.03) | |
D+3 × decentralize | -0.06 | 0.07 |
(-1.33) | (0.81) | |
D+4 × decentralize | -0.03 | 0.04 |
(-0.61) | (0.39) | |
D+5 × decentralize | -0.04 | 0.02 |
(-0.80) | (0.17) | |
Weather | YES | YES |
City-year FE | YES | YES |
Week FE | YES | YES |
Day of week FE | YES | YES |
Obs. | 83, 578 | 83, 578 |
R2 | 0.48 | 0.48 |
First stage results | ||
Temp × decentralize | 0.57*** | 0.58*** |
(9.45) | (10.37) | |
F-Stat. | 23.79 | 7.64 |
Note: Robust t-statistics are reported in parentheses. Standard errors are clustered at the city level. *** p < 0.01, ** p < 0.05, * p < 0.1. |
Municipal secretary | Mayor | |
(1) | (2) | |
Log (AQI) | ||
D0 × promote × decentralize | -0.18*** | -0.14*** |
(-3.12) | (-2.74) | |
Obs. | 50, 883 | 54, 673 |
R2 | 0.47 | 0.48 |
Log (PM2.5) | ||
D0 × promote × decentralize | -0.26*** | -0.18** |
(-3.36) | (-2.58) | |
Obs. | 50, 883 | 54, 673 |
R2 | 0.46 | 0.47 |
Log (PM10) | ||
D0 × promote × decentralize | -0.20*** | -0.15*** |
(-2.76) | (-2.61) | |
Obs. | 50, 883 | 54, 673 |
R2 | 0.50 | 0.52 |
Weather | YES | YES |
City-year FE | YES | YES |
Week FE | YES | YES |
Day of week FE | YES | YES |
Note: For simplicity, the table only shows the coefficients of interactions during marathon games. Robust t-statistics are reported in parentheses. Standard errors are clustered at the city level. *** p < 0.01, ** p < 0.05, * p < 0.1. |
Municipal secretary | Mayor | |
(1) | (2) | |
Log (AQI) | ||
D0 × turnover × decentralize | -0.18*** | -0.14*** |
(-3.68) | (-2.88) | |
Obs. | 78, 010 | 78, 472 |
R2 | 0.48 | 0.48 |
Log (PM2.5) | ||
D0 × turnover × decentralize | -0.23*** | -0.19*** |
(-3.33) | (-2.76) | |
Obs. | 78, 010 | 78, 472 |
R2 | 0.47 | 0.46 |
Log (PM10) | ||
D0 × turnover × decentralize | -0.21*** | -0.14*** |
(-3.37) | (-2.64) | |
Obs. | 78, 010 | 78, 472 |
R2 | 0.51 | 0.51 |
Weather | YES | YES |
City-year FE | YES | YES |
Week FE | YES | YES |
Day of week FE | YES | YES |
Note: For simplicity, the table only shows the coefficients of interactions during marathon games. Robust t-statistics are reported in parentheses. Standard errors are clustered at the city level. *** p < 0.01, ** p < 0.05, * p < 0.1. |
Municipal secretary | Mayor | |||
(1) OLS |
(2) IV |
(3) OLS |
(4) IV |
|
Log (AQI) | ||||
D0 × BEF × year2015 | -0.20*** | -0.29*** | -0.21*** | -0.23*** |
(-3.96) | (-3.86) | (-4.27) | (-2.69) | |
D0 × AFT × year2015 | -0.01 | -0.05 | 0.08 | -0.08 |
(-0.33) | (-0.73) | (1.52) | (-0.91) | |
Obs. | 78, 010 | 78, 010 | 78, 472 | 78, 472 |
R2 | 0.48 | 0.48 | 0.48 | 0.48 |
Log (PM2.5) | ||||
D0 × BEF × decentralize | -0.27*** | -0.43*** | -0.31*** | -0.37*** |
(-3.88) | (-4.09) | (-4.37) | (-3.26) | |
D0 × AFT × decentralize | -0.01 | -0.13 | 0.14* | -0.11 |
(-0.13) | (-1.28) | (1.96) | (-0.87) | |
Obs. | 78, 010 | 78, 010 | 78, 472 | 78, 472 |
R2 | 0.47 | 0.47 | 0.46 | 0.46 |
Log (PM10) | ||||
D0 × BEF × decentralize | -0.22*** | -0.39*** | -0.22*** | -0.28*** |
(-3.42) | (-4.03) | (-3.67) | (-2.74) | |
D0 × AFT × decentralize | -0.01 | -0.02 | 0.10 | -0.06 |
(-0.27) | (-0.20) | (1.60) | (-0.56) | |
Obs. | 78, 010 | 78, 010 | 78, 472 | 78, 472 |
R2 | 0.51 | 0.51 | 0.51 | 0.51 |
Weather | YES | YES | YES | YES |
City-year FE | YES | YES | YES | YES |
Week FE | YES | YES | YES | YES |
Day of week FE | YES | YES | YES | YES |
Note: The instrumental variable is temperature dummy with 5–25–degree interval. For simplicity, the table only shows the coefficients of interactions at the marathon date. Robust t-statistics are reported in parentheses. Standard errors are clustered at the city level. F statistic is for the Kleibergen-Paap rk Wald test. *** p < 0.01, ** p < 0.05, * p < 0.1. |
Municipal secretary | Mayor | |
(1) | (2) | |
Log (AQI) | ||
D0 × age × decentralize | -0.002*** | -0.002*** |
(-3.43) | (-3.32) | |
Obs. | 78, 010 | 78, 472 |
R2 | 0.48 | 0.48 |
Log (PM2.5) | ||
D0 × age × decentralize | -0.003*** | -0.002*** |
(-3.09) | (-3.16) | |
Obs. | 78, 010 | 78, 472 |
R2 | 0.47 | 0.47 |
Log (PM10) | ||
D0 × age × decentralize | -0.002*** | -0.002*** |
(-3.36) | (-2.66) | |
Obs. | 78, 010 | 78, 472 |
R2 | 0.51 | 0.51 |
Weather Controls | YES | YES |
City-year FE | YES | YES |
Week FE | YES | YES |
Day of week FE | YES | YES |
Note: For simplicity, the table only shows the coefficients of interactions at the marathon date. Robust t-statistics are reported in parentheses. Standard errors are clustered at the city level. *** p < 0.01, ** p < 0.05, * p < 0.1. |
(1) | (2) | (3) | (4) | (5) | (6) | (7) | |
D-5 | -0.024 | -0.024 | -0.023 | -0.023 | -0.011 | -0.028 | -0.031 |
(-1.11) | (-1.11) | (-1.08) | (-1.08) | (-0.53) | (-1.35) | (-1.44) | |
D-4 | -0.003 | -0.004 | -0.002 | -0.002 | 0.003 | -0.012 | -0.013 |
(-0.12) | (-0.18) | (-0.09) | (-0.10) | (0.11) | (-0.54) | (-0.54) | |
D-3 | -0.045** | -0.047** | -0.044* | -0.044* | -0.044* | -0.052** | -0.055** |
(-1.98) | (-2.08) | (-1.94) | (-1.94) | (-1.96) | (-2.48) | (-2.41) | |
D-2 | -0.050** | -0.052** | -0.049** | -0.049** | -0.050** | -0.057*** | -0.061** |
(-2.05) | (-2.14) | (-2.02) | (-2.02) | (-2.04) | (-2.69) | (-2.53) | |
D-1 | -0.027 | -0.029 | -0.027 | -0.027 | -0.023 | -0.028 | -0.041* |
(-1.25) | (-1.35) | (-1.22) | (-1.22) | (-1.03) | (-1.45) | (-1.88) | |
D0 | -0.056** | -0.056** | -0.055** | -0.055** | -0.043* | -0.042** | -0.057** |
(-2.47) | (-2.46) | (-2.44) | (-2.44) | (-1.89) | (-2.01) | (-2.49) | |
D+1 | -0.052** | -0.051** | -0.051** | -0.051** | -0.035 | -0.024 | -0.044** |
(-2.37) | (-2.33) | (-2.34) | (-2.34) | (-1.61) | (-1.22) | (-1.99) | |
D+2 | -0.024 | -0.025 | -0.024 | -0.024 | -0.012 | -0.016 | -0.022 |
(-1.16) | (-1.17) | (-1.13) | (-1.13) | (-0.58) | (-0.85) | (-1.05) | |
D+3 | -0.027 | -0.029 | -0.026 | -0.026 | -0.019 | -0.013 | -0.020 |
(-1.21) | (-1.30) | (-1.18) | (-1.19) | (-0.86) | (-0.62) | (-0.90) | |
D+4 | -0.006 | -0.009 | -0.006 | -0.006 | -0.005 | 0.007 | 0.003 |
(-0.28) | (-0.40) | (-0.24) | (-0.25) | (-0.21) | (0.31) | (0.13) | |
D+5 | -0.010 | -0.012 | -0.009 | -0.009 | -0.012 | -0.004 | -0.011 |
(-0.39) | (-0.49) | (-0.37) | (-0.37) | (-0.49) | (-0.18) | (-0.43) | |
Weather | YES | YES | YES | YES | YES | YES | YES |
Holiday | YES | YES | YES | YES | YES | YES | |
Year-City FE | YES | YES | YES | YES | YES | YES | |
Week FE | YES | YES | YES | YES | YES | ||
Day of week FE | YES | YES | YES | YES | YES | YES | |
Month FE | YES | ||||||
Date FE | YES | ||||||
Marathon date dummy | YES | ||||||
City time trends | No | 1st | 2nd | 3rd | 1st | 1st | 1st |
Obs. | 83, 578 | 83, 578 | 83, 578 | 83, 578 | 83, 578 | 83, 589 | 83, 578 |
Adj. R2 | 0.481 | 0.481 | 0.481 | 0.481 | 0.467 | 0.548 | 0.483 |
Note: Robust t-statistics are reported in parentheses. Standard errors are clustered at the city level. *** p < 0.01, ** p < 0.05, * p < 0.1. |
Municipal secretary | Mayor | |||
(1) | (2) | (3 | (5) | |
OLS | IV | OLS | IV | |
Log (AQI) | ||||
D0 × promote × decentralize | -0.171*** | -0.217* | -0.132*** | -0.153* |
(-3.05) | (-1.92) | (-2.68) | (-1.82) | |
Obs. | 50, 883 | 50, 883 | 54, 673 | 54, 673 |
R2 | 0.474 | 0.474 | 0.484 | 0.484 |
Log (PM2.5) | ||||
D0 × promote × decentralize | -0.256*** | -0.372*** | -0.174** | -0.252** |
(-3.30) | (-2.66) | (-2.53) | (-2.25) | |
Obs. | 50, 883 | 50, 883 | 54, 673 | 54, 673 |
R2 | 0.460 | 0.460 | 0.470 | 0.470 |
Log (PM10) | ||||
D0 × promote × decentralize | -0.191*** | -0.278** | -0.142** | -0.242** |
(-2.69) | (-2.23) | (-2.53) | (-2.38) | |
Obs. | 50, 883 | 50, 883 | 54, 673 | 54, 673 |
R2 | 0.502 | 0.502 | 0.515 | 0.515 |
First stage results | ||||
Temp × promote × decentralize | 0.508*** | 0.448*** | ||
(6.97) | (8.96) | |||
F-Stat. | 48.60 | 80.23 | ||
Weather Controls | YES | YES | YES | YES |
City-year FE | YES | YES | YES | YES |
Week FE | YES | YES | YES | YES |
Day of week FE | YES | YES | YES | YES |
Note: The results are based on the equation as follows: log(Pit)=αit+βiD0idecentralize×incentivei+age+edu+tenure+f(ti)+Γt+Wit+εit The instrumental variable is 5–25–degree temperature dummy. For simplicity, the table only shows the coefficients of interactions during marathon games. Robust t-statistics are reported in parentheses. Standard errors are clustered at the city level. *** p < 0.01, ** p < 0.05, * p < 0.1. |
Municipal secretary | Mayor | |||
(1) | (2) | (3) | (4) | |
OLS | IV | OLS | IV | |
Log (AQI) | ||||
D0 × turnover × decentralize | -0.178*** | -0.216*** | -0.134*** | -0.221*** |
(-3.63) | (-2.99) | (-2.90) | (-2.79) | |
Obs. | 78, 010 | 78, 010 | 78, 472 | 78, 472 |
R2 | 0.480 | 0.480 | 0.479 | 0.479 |
Log (PM2.5) | ||||
D0 × turnover × decentralize | -0.220*** | -0.360*** | -0.183*** | -0.324*** |
(-3.28) | (-3.67) | (-2.79) | (-2.96) | |
Obs. | 78, 010 | 78, 010 | 78, 472 | 78, 472 |
R2 | 0.465 | 0.465 | 0.464 | 0.464 |
Log (PM10) | ||||
D0 × turnover × decentralize | -0.205*** | -0.289*** | -0.141*** | -0.230** |
(-3.31) | (-3.06) | (-2.66) | (-2.51) | |
Obs. | 78, 010 | 78, 010 | 78, 472 | 78, 472 |
R2 | 0.508 | 0.507 | 0.506 | 0.506 |
First stage results | ||||
Temp × turnover × decentralize | 0.568*** | 0.570*** | ||
(9.43) | (10.27) | |||
F-Stat. | 88.89 | 105.50 | ||
Weather Controls | YES | YES | YES | YES |
City-year FE | YES | YES | YES | YES |
Week FE | YES | YES | YES | YES |
Day of week FE | YES | YES | YES | YES |
Note: The results are based on the equation as follows: log(Pit)=αit+βiD0idecentralizei×incentivei+age+edu+tenure+f(ti)+Γt+Wit+εit The instrumental variable is temperature dummy with 5-25-degree interval. For simplicity, the table only shows the coefficients of interactions at the marathon date. Robust t-statistics are reported in parentheses. Standard errors are clustered at the city level. *** p < 0.01, ** p < 0.05, * p < 0.1. |
Municipal secretary | Mayor | |||
(1) | (2) | (3) | (4) | |
OLS | IV | OLS | IV | |
Log (AQI) | ||||
D0 × age × decentralize | -0.002*** | -0.004*** | -0.002*** | -0.004*** |
(-3.39) | (-3.45) | (-3.29) | (-3.14) | |
Obs. | 78, 010 | 78, 010 | 78, 472 | 78, 472 |
R2 | 0.480 | 0.480 | 0.479 | 0.479 |
Log (PM2.5) | ||||
D0 × age × decentralize | -0.003*** | -0.006*** | -0.003*** | -0.006*** |
(-3.06) | (-3.95) | (-2.97) | (-3.70) | |
Obs. | 78, 010 | 78, 010 | 78, 472 | 78, 472 |
R2 | 0.465 | 0.465 | 0.464 | 0.464 |
Log (PM10) | ||||
D0 × age × decentralize | -0.002*** | -0.004*** | -0.002*** | -0.004*** |
(-3.33) | (-3.56) | (-3.11) | (-3.12) | |
Obs. | 78, 010 | 78, 010 | 78, 472 | 78, 472 |
R2 | 0.508 | 0.507 | 0.506 | 0.506 |
First stage results | ||||
Temp × age × decentralize | 0.464*** | 0.439*** | ||
(13.24) | (12.89) | |||
F-Stat. | 175.28 | 166.08 | ||
Weather Controls | YES | YES | YES | YES |
City-year FE | YES | YES | YES | YES |
Week FE | YES | YES | YES | YES |
Day of week FE | YES | YES | YES | YES |
Note: The results are based on the equation as follows: log(Pit)=αit+βiD0idecentralizei×incentivei+age+ed+tenure+f(ti)+Γt+Wit+εit The instrumental variable is 5-25-degrees temperature dummy. For simplicity, table only shows the coefficients of interactions during marathon games. Robust t-statistics are reported in parentheses. Standard errors are clustered at the city level. *** p < 0.01, ** p < 0.05, * p < 0.1. |