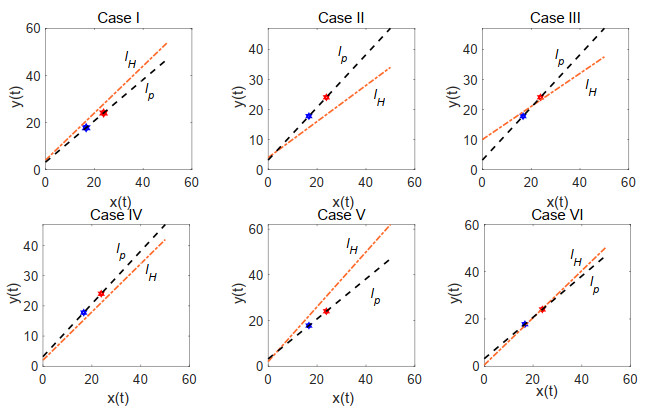
Dynamic modeling, analysis, and control of fish ecosystems are important for promoting the sustainable development of fish stocks. The objective of this study is to analyze the dynamic behavior of prey-predator systems with discontinuous prey refuge effect and different types of harvesting activities in an uncertain environment. Initially, a Filippov-type prey-predator model with fuzzy parameters is formulated and the positivity and bounded-ness of the solutions and the dynamic properties of Filippov prey-predator system are discussed. Next, from the perspective of effective exploitation and utilization of fish resources, a state linearly dependent fishing strategy is adopted into the system and a fishing model based on threshold feedback is established, as well as an analysis on the complex dynamics of the control system. Finally, to illustrate the theoretical results, computer simulations are presented step by step with an explanation on the practical significance. This study provides a reference for in-depth understanding of the development dynamics of fish resources and scientific planning of fishery resources exploitation.
Citation: Yuan Tian, Hua Guo, Wenyu Shen, Xinrui Yan, Jie Zheng, Kaibiao Sun. Dynamic analysis and validation of a prey-predator model based on fish harvesting and discontinuous prey refuge effect in uncertain environments[J]. Electronic Research Archive, 2025, 33(2): 973-994. doi: 10.3934/era.2025044
[1] | Yuan Tian, Yang Liu, Kaibiao Sun . Complex dynamics of a predator-prey fishery model: The impact of the Allee effect and bilateral intervention. Electronic Research Archive, 2024, 32(11): 6379-6404. doi: 10.3934/era.2024297 |
[2] | Yujia Xiang, Yuqi Jiao, Xin Wang, Ruizhi Yang . Dynamics of a delayed diffusive predator-prey model with Allee effect and nonlocal competition in prey and hunting cooperation in predator. Electronic Research Archive, 2023, 31(4): 2120-2138. doi: 10.3934/era.2023109 |
[3] | San-Xing Wu, Xin-You Meng . Hopf bifurcation analysis of a multiple delays stage-structure predator-prey model with refuge and cooperation. Electronic Research Archive, 2025, 33(2): 995-1036. doi: 10.3934/era.2025045 |
[4] | Fengrong Zhang, Ruining Chen . Spatiotemporal patterns of a delayed diffusive prey-predator model with prey-taxis. Electronic Research Archive, 2024, 32(7): 4723-4740. doi: 10.3934/era.2024215 |
[5] | Yichao Shao, Hengguo Yu, Chenglei Jin, Jingzhe Fang, Min Zhao . Dynamics analysis of a predator-prey model with Allee effect and harvesting effort. Electronic Research Archive, 2024, 32(10): 5682-5716. doi: 10.3934/era.2024263 |
[6] | Mengting Sui, Yanfei Du . Bifurcations, stability switches and chaos in a diffusive predator-prey model with fear response delay. Electronic Research Archive, 2023, 31(9): 5124-5150. doi: 10.3934/era.2023262 |
[7] | Ruizhi Yang, Dan Jin . Dynamics in a predator-prey model with memory effect in predator and fear effect in prey. Electronic Research Archive, 2022, 30(4): 1322-1339. doi: 10.3934/era.2022069 |
[8] | Jiani Jin, Haokun Qi, Bing Liu . Hopf bifurcation induced by fear: A Leslie-Gower reaction-diffusion predator-prey model. Electronic Research Archive, 2024, 32(12): 6503-6534. doi: 10.3934/era.2024304 |
[9] | Érika Diz-Pita . Global dynamics of a predator-prey system with immigration in both species. Electronic Research Archive, 2024, 32(2): 762-778. doi: 10.3934/era.2024036 |
[10] | Xiaowen Zhang, Wufei Huang, Jiaxin Ma, Ruizhi Yang . Hopf bifurcation analysis in a delayed diffusive predator-prey system with nonlocal competition and schooling behavior. Electronic Research Archive, 2022, 30(7): 2510-2523. doi: 10.3934/era.2022128 |
Dynamic modeling, analysis, and control of fish ecosystems are important for promoting the sustainable development of fish stocks. The objective of this study is to analyze the dynamic behavior of prey-predator systems with discontinuous prey refuge effect and different types of harvesting activities in an uncertain environment. Initially, a Filippov-type prey-predator model with fuzzy parameters is formulated and the positivity and bounded-ness of the solutions and the dynamic properties of Filippov prey-predator system are discussed. Next, from the perspective of effective exploitation and utilization of fish resources, a state linearly dependent fishing strategy is adopted into the system and a fishing model based on threshold feedback is established, as well as an analysis on the complex dynamics of the control system. Finally, to illustrate the theoretical results, computer simulations are presented step by step with an explanation on the practical significance. This study provides a reference for in-depth understanding of the development dynamics of fish resources and scientific planning of fishery resources exploitation.
As an important branch of ecosystem research, the study on population dynamic model has always been one of the important topics in bioscience and mathematics. Especially in the fishery industry, understanding the relations between predator fish and prey fish is helpful for the utilization and development of fish resources in a sustainable and reasonable way. On the theoretical side, mathematical models play a key role in understanding changes in biological systems and the effects of related control measures [1,2]. The classical model in literature describing the relationship between predator species and prey species is the well-known Lotka-Volterra model [3,4]. Subsequently, for different application scenarios, scholars considered different factors in the modeling process [5,6]. In natural ecosystems, most prey species perceive the danger of predators well and hide in cover to avoid being eaten when predators are present. Thus, the predator does not use the entire prey population as its food resource, and the prey refuge concept was introduced into the predator-prey system [7]. In the literature, various types of prey refuge effect were studied, which can be roughly divided into three types: constant quantity [7], constant proportion [8], and variable proportion [9]. In this paper, inspired by the piecewise form of prey refuge, a Filippov type prey-predator model with piecewise form of prey refuge is put forward, where prey refuge makes sense once the number of predator exceeds a certain threshold related to the number of prey. The Filippov theory, a primary tool for analyzing Filippov models, is widely applied and studied. For example, Tang and Liang [10] investigated a Filippov predator-prey system with non-smooth refuge. Chen and Huang [11] analyzed a Filippov ratio-dependent prey-predator model with behavioral refuges caused by prey instinct anti-predator behavior. Li et al. [12] analyzed a Filippov-type plant disease models with an interaction ratio threshold. Subsequently, Li et al. [13] studied a Filippov predator-prey model with two thresholds for integrated pest management. In this study, considering the piecewise form of prey refuge, a fishery model involving discontinuous prey refuge effect is presented and investigated.
In the actual world, the natural environment is always in change and fluctuation, which will naturally influence the survival and reproduction of the species living there. To describe the influence caused by environment fluctuation, scholars introduced different models with interval-valued imprecise parameters and fuzzy parameters, for example, Pal et al. [14] replaced the deterministic parameters in the predator-prey models with interval-valued imprecise parameters and fuzzy parameters. Zhang and Zhao [15] discussed the bifurcation and optimal harvesting of a diffusive predator-prey system with delays and interval biological parameters. Pal et al. [16,17] discussed the stability and bionomic analysis of a prey-predator with fuzzy parameters. Xiao et al. [18] analyzed a competition fishery model with interval-valued parameters and discussed the extinction, coexistence, bionomic equilibria, and optimal harvesting policy. Wang et al. [19] incorporated prey refuge into a predator-prey system with imprecise parameter estimates. Yu et al. [20] analyzed a predator-prey fishery model with interval imprecise parameters, taking into account the interaction between predators in the system and the refuge effect of prey. Meng and Wu [21] analyzed the dynamics of a fuzzy phytoplankton-zooplankton model with refuge, fishery protection, and harvesting. Wang et al. [22] discussed the stability and optimal harvesting of a predator-prey system combining prey refuge with fuzzy biological parameters. Chen and Zheng [23] discussed the diffusion-driven instability of a predator-prey model with interval biological coefficients. Xu et al. [24] discussed the optimal harvesting of a fuzzy water hyacinth-fish model with Kuznets curve effect. Guo et al. [25] analyzed the dynamics of two fishery capture models with a variable search rate and fuzzy biological parameters. Cao et al. [26] discussed the Hopf bifurcation in a predator-prey model under fuzzy parameters involving prey refuge and fear effects. Studies on freshwater fish have shown that fish species are more vulnerable to environmental changes. In addition, environmental changes affect different fish species in different ways [27]. In view of this feature, species related imprecise parameters were introduced into the prey-predator model [28] and the impact of imprecise parameters on the dynamics of the systems were discussed. In the current work, a Filippov type prey-predator model with triangle fuzzy parameters is studied and the impact of fuzzy parameters on the dynamic behaviour is analyzed.
Fishing is the main way for human to obtain fish resources, and its pattern presents two types: continuous and discontinuous. For continuous fishing activities, the modeling is relatively simple, which can be directly described by adding fishing items in the model. For intermittent fishing patterns, fishing activities are usually taken at discrete moments. Among many intermittent fishing activities, state-dependent feedback fishing is a typical one, which takes into account the current state of prey or predator and is able to avoid destroying the sustainability of fish resources. There are many studies on state-dependent feedback control in the literature, which can be roughly divided into several types, such as prey-dependent [29], predator-dependent [30], nonlinear-dependent [31], weighted-dependent [32], and ratio-dependent [33]. Among these kinds of state-dependent feedback control, ratio-dependent feedback control considers the relation between prey population and predator population and implements control when the ratio of predator population to prey population reaches a certain threshold. In this study, a state's linearly dependent feedback fishing strategy is considered and the fishery model with such control is analyzed.
Motivated by above discussions, a Filippov type prey-predator model with discontinuous refuge effect, triangle fuzzy biological parameters and continuous harvesting is put forward and analyzed. The paper is organized as follows: In Section 2, two types of prey-predator models with different fishing model and fuzzy parameters are presented and followed by a presentation of some preliminaries. In Section 3, the dynamics of Filippov type prey-predator with a continuous harvesting strategy is analyzed. Then, the complex dynamics of the fishery model with state linearly dependent feedback harvesting is investigated. In Section 4, numerical simulations are presented to illustrate the main results. In the last section, a conclusion is summarized with a presentation of the future work.
Prey refuge is a common phenomenon among fish species. Let xr denote the volume of prey in refuge. In this work, a discontinuous refuge effect is considered, that is,
xr={mx,y>nx+yT,0,y<nx+yT, |
where m∈]0,1[ is the proportion of refuge prey; yT>0 is the minimum level of predators, when the density of predators is lower than yT, prey will come out of the refuge; n>0 is the threshold for the ratio of predator to prey in the system, and if the ratio exceeds the threshold of n in the case of sufficient prey density, the prey will choose to hide in the shelter.
Then, the Filippov type prey-predator model with continuous harvesting is expressed as
{dx(t)dt=rx(t)(1−x(t)K)−a(x(t)−xr)y−q1E1(x(t)−xr),dy(t)dt=−dy(t)+ca(x(t)−xr)y−q2E2y(t),x(0)=x0>0,y(0)=y0>0, | (2.1) |
where t∈[0,+∞[, x(t) and y(t) represent the biomass of prey and predator species at time t, respectively. r characterizes the prey's growth rate; K characterizes the prey's environmental capacity; a characterizes the predator's predation rate, x(t)−xr is the density of prey outside the shelter; d characterizes the predator's death rate; c characterizes the conversion efficiency from prey biomass into predator biomass; E1 and E2 represent fishing effort for prey and predator, respectively; q1 and q2 represent the capture rate for prey and predator species.
To consider the impact of environmental changes and fluctuations, triangular fuzzy numbers (TFNs) [20] are adopted to describe the uncertainty of parameters. For a TFN ˜U≡(u1,u2,u3) and α∈(0,1], the α-cut set is denoted by [Ul(α),Ur(α)], where Ul(α)={x:μ˜U(x)≥α}=u1+α(u2−u1), Ur(α)={x:μ˜U(x)≥α}=u3+α(u3−u2). Given that the birth rate of prey, the death rate and conversion rate of predator most susceptible to environmental changes, these three parameters are assumed to present some imprecision, represented by TFNs, that is, ˜r=(rL,rM,rR), ˜d=(dL,dM,dR) and ˜c=(cL,cM,cR). Using theory of α-cut fuzzy number, we introduce fuzzy triangle parameters into above model:
{(dx(t)dt)l(α)=rl(α)x(t)(1−x(t)K)−a(x(t)−xr)y−q1E1(x(t)−xr),(dx(t)dt)u(α)=ru(α)x(t)(1−x(t)K)−a(x(t)−xr)y−q1E1(x(t)−xr),(dy(t)dt)l(α)=−du(α)y(t)+cl(α)a(x(t)−xr)y(t)−q2E2y(t),(dy(t)dt)u(α)=−dl(α)y(t)+cu(α)a(x(t)−xr)y(t)−q2E2y(t). | (2.2) |
Using the utility function method [16], there is
{dx(t)dt=w1(dx(t)dt)l(α)+(1−w1)(dx(t)dt)u(α),dy(t)dt=w2(dy(t)dt)l(α)+(1−w2)(dy(t)dt)u(α), | (2.3) |
where 0≤w1,w2≤1. For convenience, define
ˆr=w1rl(α)+(1−w1)ru(α),ˆd=w2du(α)+(1−w2)dl(α),ˆc=w2cl(α)+(1−w2)cu(α). | (2.4) |
Combining Eqs (2.4) and (2.1) gives
{dx(t)dt=ˆrx(t)(1−x(t)K)−a(x(t)−xr)y(t)−q1E1(x(t)−xr),dy(t)dt=−ˆdy(t)+ˆca(x(t)−xr)y(t)−q2E2y(t),x(0)=x0>0,y(0)=y0>0. | (2.5) |
For y(t)<nx(t)+yT, system complies with the model
{dx(t)dt=ˆrx(t)(1−x(t)K)−ax(t)y(t)−q1E1x(t):=x(t)f11(x(t),y(t)),dydt=−ˆdy(t)+ˆcax(t)y(t)−q2E2y:=y(t)f12(x(t)),x(0)=x0>0,y(0)=y0>0. | (2.6) |
and for y>nx+yT, system complies with the model
{dx(t)dt=ˆrx(t)(1−x(t)K)−a(x(t)−mx(t))y(t)−q1E1(x(t)−mx(t)):=x(t)f21(x(t),y(t)),dy(t)dt=−ˆdy(t)+ˆca[x(t)−mx(t)]y(t)−q2E2y(t):=y(t)f22(x(t)),x(0)=x0>0,y(0)=y0>0. | (2.7) |
In this subsection, we consider a scenario where fishing is allowed only when prey and predator populations exceed a certain limit. Let yH be the minimum fishing level of the predator, below which fishing activity may cause the extinction of the predator fish. Moreover, predator fish feed on prey populations, and when the ratio of predator to prey exceeds a certain threshold, denoted by l, fishing activity is conducive to the sustainable development of fish resources. Based on the above consideration, we establish the following harvesting model with uncertain parameters:
{dx(t)dt=ˆrx(t)(1−x(t)K)−a(x(t)−xr)ydy(t)dt=−ˆdy(t)+ˆca(x(t)−xr)yx(0)=x0>0,y(0)=y0>0.}y(t)<lx(t)+yH,Δx(t):=x(t+)−x(t)=−q1E1(x(t)−xr)Δy(t):=y(t+)−y(t)=−q2E2y(t)}y(t)=lx(t)+yH. | (2.8) |
where l>0, yH>0 are predetermined constants.
Let ˜U be a fuzzy set on the real set R, i.e., ˜U∈F(R), μ˜U(⋅) be the membership function of ˜U.
Definition 1 (α-cut set [16,25]). For α∈]0,1], the α-cut set for ˜U is defined as ˜Uα={x:μ˜U(x)≥α}.
Definition 2 (TFN[16,25]). If ˜U is normal (i.e., there is x∈R and μ˜U(x)=1), and for any α∈]0,1[, ˜Uα is a closed interval, then ˜U is said to be a fuzzy number (FN). A TFN ˜U≡(uL,uM,uR) is a FN with membership defined by
μ˜U(u)={u−uLuM−uL,ifuL≤u≤uM,uR−uuR−uM,ifuM≤u≤uR,0,otherwise. |
Clearly, α-cut set of TFN ˜U≡(aL,aM,aR) is [Ul(α),Ur(α)], where Ul(α)=inf{x:μ˜U(x)≥α}=uL+α(uM−uL) and Ur(α)=sup{x:μ˜U(u)≥α}=uR+α(uR−uM).
Definition 3 (Utility function [16,25]). Given Ui, i=1,2,⋯,N, and denoted wi as the weight of items Ui, ΣNi=1wi=1. Then, a utility function U is defined by U=ΣNi=1wiUi.
Consider a piecewise-continuous system
(dudtdvdt)={F1(u,v), if (u,v)∈S1={(u,v)∈R2+:H(u,v)>0},F2(u,v), if (u,v)∈S2={(u,v)∈R2+:H(u,v)<0}, | (2.9) |
where H:R2+→R, and the discontinuous demarcation is Σ={(u,v)∈R2+:H(u,v)=0}.
Let
Then, for , with . The discontinuous demarcation can be distinguished into three regions:
1) sliding region: ;
2) crossing region: ;
3) escaping region: .
The dynamics of system (2.9) along is determined by
where with .
Definition 4 (Real, virtual and pseudo-equilibrium [11]). For system (2.9), is a real equilibrium if so that , ; is a virtual equilibrium if so that , ; is a pseudo-equilibrium if , .
For a given planar model
(2.10) |
Definition 5 (Order- periodic solution [32]). The solution of system (2.10) is called periodic if there exists satisfying . Furthermore, is an order- T-periodic solution with .
Lemma 1 (Analogue of Poincaré criterion [32]). The order- -periodic solution of system (2.10) is orbitally asymptotically stable if , where
with
, and , , , , , , , are calculated at , where and .
Define
Obviously, when , there is , then when .
To avoid the extinction of prey and predator fish populations by fishing activity}}, it is required that and .
Theorem 1. For given , , and , the solution of the Filippov system (2.5) with and always keeps positive, that is, , for .
Proof. Suppose that . If intersects with region and then stay in region all the time, it can be easily obtained that and for . Otherwise, define , as the time when trajectory intersects with and subsequently enters into another region. Note that due to the definition of function , we can write
Since in there is
then we have
thus it has for . Similarly, there is for .
Theorem 2. For given , , and , the solution of the Filippov system (2.5) with and is uniformly bounded.
Proof. Define . Then,
Choosing , we have
which implies that
For , there is . Moreover, if , then for all .
Denote
Note that is a real equilibrium for subsystem (2.6) and a virtual equilibrium for subsystem (2.6). If , then exists, which is a real boundary equilibrium for subsystem (2.6); exists, which is a virtual equilibrium for subsystem (2.7).
Theorem 3. For subsystems (2.6) and (2.7) with given , , and , , , and are unstable if , . Moreover, subsystem (2.6) has a unique globally asymptotically stable interior equilibrium , subsystem (2.7) has a unique globally asymptotically stable interior equilibrium , where
Proof. In case of , , the equation set has a positive solution , that is, subsystems (2.6) has a unique interior equilibrium . Similarly, the subsystem (2.7) has a unique interior equilibrium .
The Jacobian matrix of the subsystems (2.6) at is
At , there is
then and , which implies that is saddle and unstable.
At , there is
then and , which implies that is saddle and unstable.
At , there is
then , , which implies that is locally asymptotically stable.
Define . Then, we have
then by the Bendixson-Dulac theorem [34], there does not exist a closed orbit in , so is globally asymptotically stable.
The stability of the equilibria , , and for the subsystems (2.7) can be proved similarly.
It is obvious that and . Define
Then, the slope and the intercept are, respectively,
Theorem 4. For Filippov system (2.5) with given , and , for case-I): , , is real, is virtual; for case-II): , , is virtual, is real; for case-III): , , is real, is real; for case-IV): , , is virtual, is real; for case-V): , , is real, is virtual; for case-VI): , , is virtual, is virtual.
Proof. For case-Ⅰ, that is, , , both of and are under the line , as presented in Figure 1. and , so is a real equilibrium; and , so is a virtual equilibrium. Similarly, the results for case Ⅱ-Ⅵ can be proved.
Next, it discusses the existence of pseudo- equilibrium. Since and
Since
then there exists a unique such that , where
with
For , there is }}, and for , there is .
Similarly, there is
Since
then there exists a unique such that , where
with
For , there is , and for , there is .
Since and for , then it has . Thus, and , where and .
Utilizing the Filippov convex method, the dynamics on is determined by
where
Since on , there is , then we have
Define
Then, we have the following result:
Theorem 5. For given , , and , if and , then Filippov system (2.5) has a unique pseudo-equilibrium .
For system (2.8), we have
To ensure that and do not intersect, it requires that , in such case is below . Let be the isolines in system, that is,
According to the relative position between , , and , two situations are discussed for the dynamic of system (2.8):
Case Ⅰ: , , that is, both and lie below in .
Case Ⅱ: , , that is, both and lies above in .
Definition 6 (Successor function). For a point , if the trajectory from directly intersects , denote the intersection point by . And then is impulsed to due to impulse effect. In such case, we can define : , . If the trajectory from first passes through , and then intersects , then we can define : , .
Theorem 6. For given , and and Case I, an order-1 periodic solution exists in system (2.8) for any one of the following conditions: I-1) , , ; I-2) , ; I-3) , ; I-4) , ; I-5) , , ; I-6) , .
Proof. For case Ⅰ-1) , is real equilibrium, is virtual equilibrium. Moreover, is locally asymptotically stable.
Denote as the interaction point between and . Since , then . Let such that
The coordinates of are obtained from the following equations:
Define . Then,
1) for , there is , that is, the orbit from forms an order-1 periodic orbit;
2) for , there is . Then, such that ;
3) for , there is . Then, . Besides, for d, and so that , then it has . Thus such that .
To sum up, , the trajectory of system (2.8) starting from forms an order-l periodic solution. Similarly, the results for case Ⅰ-2)–Ⅰ-6) can be proved.
Theorem 7. For given , , and and Case II, an order-1 periodic solution exists in system (2.8) for any one of following two conditions: II-1) , , ; II-2) , , .
Proof. The proof is similar to that of Theorem 5 and is omitted here.
Let , , be the order-1 periodic solution. Denote
and define
Theorem 8. For given , , and and Case I, , , is orbitally asymptotically stable if
Proof. For Case I, lies in the region . Then,
so that
Thus, it has
and
Therefore,
To sum up, there is if
Remark 1. For Case Ⅱ, it is only necessary to consider that , interacts with . In such situation, , is always orbitally asymptotically stable since it always leaves the sliding region from the same point.
To illustrate the theoretical results, we present the numerical simulations, which are implemented in MATLAB simulations. The model parameters are assumed to be , , , , , , , which are arbitrarily selected within their reasonable range.
For system (2.7), three aspects of the imprecision indicator , the coefficient of refuge , and fishing efforts together affect the dynamical behavior of the system. To illustrate the effect of different parameters on the system, we will verify it by fixing two parameters and changing one parameter.
First, we consider a scenario where fishing activity is not allowed, that is, . For , that is, about 25% prey fishes can go into the shelter, the dynamics of the system (2.7) for different imprecise index on the system are presented in Figures 2–4. It can be observed that for , when is small (for example ), is a virtual equilibrium and is a real equilibrium; when is big (for example ), is a real equilibrium and is a virtual equilibrium; when is small (for example ), is a virtual equilibrium and is a real equilibrium for smaller ; with the increasing of (for example ), both and are virtual equilibria; while for bigger (for example ), is a real equilibrium and is a virtual equilibrium. Next, for , the impact of the refuge coefficient on the dynamics of the system (2.7) is demonstrated, where is selected to characterize the relative size of the refuge. The dynamics of the system for different is presented in Figure 5. Obviously, the prey's refuge level does affect the system's dynamic behaviour.
Second, we consider a scenario where fishing activity is allowed. For , , the dynamics of the system for different are presented in Figure 6. It can be observed that the fishing efforts have a certain impact on the dynamics of system (2.7). For smaller fishing efforts (for example ), is a virtual equilibrium and is a real equilibrium; with the increasing of fishing efforts (for example ), both and are virtual equilibria; for bigger fishing efforts (for example ), is a real equilibrium and is a virtual equilibrium.
Given that . For control parameters , , , when , an order-1 periodic solution exists for case I, which totally lies in the region , as presented in Figure 7(a); when , an order-1 periodic solution exists for case Ⅱ, which totally lies in the region , as presented in Figure 7(b). While for control parameters , , , , an order-I periodic solution exists for case Ⅱ, which includes a sliding trajectory in region , as shown in Figure 7(c).
In the natural ecosystem, prey species may exhibit refuge effect when facing the threat of predator species. When the number of predators is relative high compared to the prey, certain percentages of the prey will hide, and when the number of predators is relative small compared to the prey, prey choose not to hide. In addition, natural species are affected by environmental changes in ecosystems, resulting in certain inaccuracies or uncertainties in some key biological parameters. Considering this phenomenon, a Filippov-type fishery model with discontinuous refuge effect and triangle fuzzy number was proposed. Through the Filippov theorem, the sliding mode dynamics of Filippov-type predator-prey system with continuous harvesting were analyzed. The results show that the system may present different types (real, virtual, and pseudo) of equilibrium under different conditions (Theorem 4, Theorem 5, Figures 2–6).
Considering the exploitation of fish resources, a fishing model with threshold control was established by adopting a linear dependent feedback fishing strategy. The complex dynamic properties of the control model are analyzed, including the existence and stability of coexisting order-1 periodic solutions. For two special cases, we provide conditions for the existence of first-order periodic solutions that depend on the relative values of and (Theorem 6, Theorem 7, Figure 7). The results show that different dynamic behaviors can be obtained in the system with discontinuous refuge and different type of harvesting activities.
The authors declare they have not used Artificial Intelligence (AI) tools in the creation of this article.
The work was supported by the National Natural Science Foundation of China (No. 11401068).
The authors declare there is no conflicts of interest.
[1] |
F. Wang, R. Yang, Dynamics of a delayed reaction-diffusion predator-prey model with nonlocal competition and double Allee effect in prey, Int. J. Biomath., 18 (2025), 2350097. https://doi.org/10.1142/S1793524523500973 doi: 10.1142/S1793524523500973
![]() |
[2] |
Y. Ma, R. Yang, Hopf-Hopf bifurcation in a predator-prey model with nonlocal competition and refuge in prey, Discrete Contin. Dyn. Syst.-Ser. B, 29 (2024), 2582–2609. https://doi.org/10.3934/dcdsb.2023193 doi: 10.3934/dcdsb.2023193
![]() |
[3] | A. J. Lotka, Elements of Physical Biology, Williams and Wilkins Company, Baltimore, 1925. |
[4] |
V. Volterra, Fluctuations in the abundance of a species considered mathematically, Nature, 119 (1927), 12–13. https://doi.org/10.1038/119012b0 doi: 10.1038/119012b0
![]() |
[5] |
C. S. Holling, The functional response of predators to prey density and its role in mimicry and population regulation, Mem. Entomol. Soc. Can., 97 (1965), 5–60. https://doi.org/10.4039/entm9745fv doi: 10.4039/entm9745fv
![]() |
[6] |
Y. Kuang, E. Beretta, Global qualitative analysis of a ratio-dependent predator-prey system, J. Math. Biol., 36 (1998), 389–406. https://doi.org/10.1007/s002850050105 doi: 10.1007/s002850050105
![]() |
[7] | J. Smith, Models in Ecology, Cambridge University Press, Cambridge, 1974. |
[8] |
Z Ma, W. Li, Y. Zhao, W. Wang, H. Zhang, Z. Li, Effects of prey refuges on a predator-prey model with a class of functional responses: The role of refuges, Math. Biosci., 218 (2009), 73–79. https://doi.org/10.1016/j.mbs.2008.12.008 doi: 10.1016/j.mbs.2008.12.008
![]() |
[9] |
D. Barman, J. Roy, S. Alam, Modelling hiding behaviour in a predator-prey system by both integer order and fractional order derivatives, Ecol. Inf., 67 (2022), 101483. https://doi.org/10.1016/j.ecoinf.2021.101483 doi: 10.1016/j.ecoinf.2021.101483
![]() |
[10] |
S. Tang, J. Liang, Global qualitative analysis of a non-smooth Gause predator-prey model with a refuge, Nonlinear Anal. Theory Methods Appl., 76 (2013), 165–180. https://doi.org/10.1016/j.na.2012.08.013 doi: 10.1016/j.na.2012.08.013
![]() |
[11] |
X. Chen, L. Huang, A Filippov system describing the effect of prey refuge use on a ratio-dependent predator-prey model, J. Math. Anal. Appl., 428 (2015), 817–837. https://doi.org/10.1016/j.jmaa.2015.03.045 doi: 10.1016/j.jmaa.2015.03.045
![]() |
[12] |
W. Li, L. Huang, J. Wang, Global dynamics of Filippov-type plant disease models with an interaction ratio threshold, Math. Methods Appl. Sci., 43 (2020), 6995–7008. https://doi.org/10.1002/mma.6450 doi: 10.1002/mma.6450
![]() |
[13] |
W. Li, Y. Chen, L. Huang, J. Wang, Global dynamics of a filippov predator-prey model with two thresholds for integrated pest management, Chaos Solitons Fractals, 157 (2022), 111881. https://doi.org/10.1016/j.chaos.2022.111881 doi: 10.1016/j.chaos.2022.111881
![]() |
[14] |
D. Pal, G. S. Mahapatra, G. P. Samanta, Optimal harvesting of prey-predator system with interval biological parameters: A bioeconomic model, Math. Biosci., 241 (2013), 181-187. https://doi.org/10.1016/j.mbs.2012.11.007 doi: 10.1016/j.mbs.2012.11.007
![]() |
[15] |
X. Zhang, H. Zhao, Bifurcation and optimal harvesting of a diffusive predator-prey system with delays and interval biological parameters, J. Theor. Biol. 363 (2014), 390-403. https://doi.org/10.1016/j.jtbi.2014.08.031 doi: 10.1016/j.jtbi.2014.08.031
![]() |
[16] |
D. Pal, G. S. Mahapatra, G. P. Samanta, Stability and bionomic analysis of fuzzy parameter based prey-predator harvesting model using UFM, Nonlinear Dyn., 79 (2015), 1939–1955. https://doi.org/10.1007/s11071-014-1784-4 doi: 10.1007/s11071-014-1784-4
![]() |
[17] |
D. Pal, G. S. Mahapatra, G. P. Samanta, Stability and bionomic analysis of fuzzy prey-predator harvesting model in presence of toxicity: A dynamic approach, Bull. Math. Biol. 78 (2016), 1493-1519. https://doi.org/10.1007/s11538-016-0192-y doi: 10.1007/s11538-016-0192-y
![]() |
[18] |
Q. Xiao, B. Dai, L. Wang, Analysis of a competition fishery model with interval-valued parameters: Extinction, coexistence, bionomic equilibria and optimal harvesting policy, Nonlinear Dyn., 80 (2015), 1631–1642. https://doi.org/10.1007/s11071-015-1967-7 doi: 10.1007/s11071-015-1967-7
![]() |
[19] |
Q. Wang, Z. Liu, X. Zhang, R. A. Cheke, Incorporating prey refuge into a predator-prey system with imprecise parameter estimates, Comput. Appl. Math., 36 (2017), 1067–1084. https://doi.org/10.1007/s40314-015-0282-8 doi: 10.1007/s40314-015-0282-8
![]() |
[20] |
X. Yu, S. Yuan, T. Zhang, About the optimal harvesting of a fuzzy predator-prey system: A bioeconomic model incorporating prey refuge and predator mutual interference, Nonlinear Dyn., 94 (2018), 2143–2160. https://doi.org/10.1007/s11071-018-4480-y doi: 10.1007/s11071-018-4480-y
![]() |
[21] |
X. Meng, Y. Wu, Dynamical analysis of a fuzzy phytoplankton-zooplankton model with refuge, fishery protection and harvesting, J. Appl. Math. Comput., 63 (2020), 361–389. https://doi.org/10.1007/s12190-020-01321-y doi: 10.1007/s12190-020-01321-y
![]() |
[22] |
Q. Wang, S. Zhai, Q. Liu, Z. Liu, Stability and optimal harvesting of a predator-prey system combining prey refuge with fuzzy biological parameters, Math. Biosci. Eng., 18 (2021), 9094–9120. http://doi.org/10.3934/mbe.2021448 doi: 10.3934/mbe.2021448
![]() |
[23] |
M. Chen, Q. Zheng, Diffusion-driven instability of a predator-prey model with interval biological coefficients, Chaos Solitons Fractals, 172 (2023), 113494. https://doi.org/10.1016/j.chaos.2023.113494 doi: 10.1016/j.chaos.2023.113494
![]() |
[24] |
J. Xu, S. Yuan, T. Zhang, Optimal harvesting of a fuzzy water hyacinth-fish model with Kuznets curve effect, Int. J. Biomath., 16 (2023), 2250082. https://doi.org/10.1142/S1793524522500826 doi: 10.1142/S1793524522500826
![]() |
[25] |
H. Guo, Y Tian, K. Sun, X. Song, Dynamic analysis of two fishery capture models with a variable search rate and fuzzy biological parameters, Math. Biosci. Eng., 20 (2023), 21049–21074. http://doi.org/10.3934/mbe.2023931 doi: 10.3934/mbe.2023931
![]() |
[26] |
X. Cao, Q. Wang, J. Liu, Hopf bifurcation in a predator-prey model under fuzzy parameters involving prey refuge and fear effects, AIMS Math., 9 (2024), 23945–23970. http://doi.org/10.3934/math.20241164 doi: 10.3934/math.20241164
![]() |
[27] |
Y. Kwon, M. Bae, S. Hwang, S. Kim, Y. Park, Predicting potential impacts of climate change on freshwater fish in Korea, Ecol. Inf., 29 (2015), 156–165. https://doi.org/10.1016/j.ecoinf.2014.10.002 doi: 10.1016/j.ecoinf.2014.10.002
![]() |
[28] |
Y. Tian, C. Li, J. Liu, Complex dynamics and optimal harvesting strategy of competitive harvesting models with interval-valued imprecise parameters, Chaos Solitons Fractals, 167 (2023), 113084. https://doi.org/10.1016/j.chaos.2022.113084 doi: 10.1016/j.chaos.2022.113084
![]() |
[29] |
Q. Zhang, S. Tang, X. Zou, Rich dynamics of a predator-prey system with state-dependent impulsive controls switching between two means, J. Differ. Equations, 364 (2023), 336–377. https://doi.org/10.1016/j.jde.2023.03.030 doi: 10.1016/j.jde.2023.03.030
![]() |
[30] |
L. Nie, Z. Teng, H. Lin, J. Peng, The dynamics of a Lotka-Volterra predator-prey model with state dependent impulsive harvest for predator, Biosystems, 98 (2009), 67–72. https://doi.org/10.1016/j.biosystems.2009.06.001 doi: 10.1016/j.biosystems.2009.06.001
![]() |
[31] |
Q. Zhang, S. Tang, Bifurcation analysis of an ecological model with nonlinear state-dependent feedback control by poincaré map defined in phase set, Commun. Nonlinear Sci. Numer. Simul., 108 (2022) 106212. https://doi.org/10.1016/j.cnsns.2021.106212 doi: 10.1016/j.cnsns.2021.106212
![]() |
[32] |
Y. Tian, Y. Gao, K. Sun, A fishery predator-prey model with anti-predator behavior and complex dynamics induced by weighted fishing strategies, Math. Biosci. Eng., 20 (2023), 1558–1579. https://doi.org/10.3934/mbe.2023071 doi: 10.3934/mbe.2023071
![]() |
[33] |
W. Li, J. Ji, L. Huang, Global dynamic behavior of a predator-prey model under ratio-dependent state impulsive control, Appl. Math. Modell., 77 (2020), 1842–1859. https://doi.org/10.1016/j.apm.2019.09.033 doi: 10.1016/j.apm.2019.09.033
![]() |
[34] |
L. A. Cherkas, A. A. Grin', Bendixson-Dulac criterion and reduction to global uniqueness in the problem of estimating the number of limit cycles, Differ. Equations 46 (2010), 61–69. https://doi.org/10.1134/S0012266110010076 doi: 10.1134/S0012266110010076
![]() |