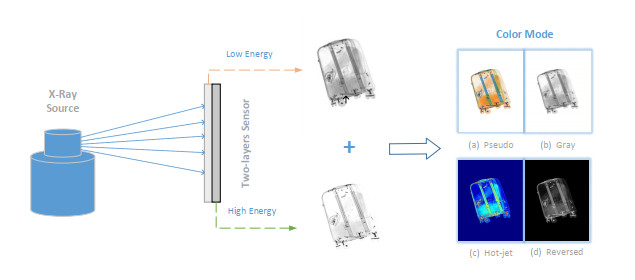
The merging of neural radiance fields with generative adversarial networks (GANs) can synthesize novel views of objects from latent code (noise). However, the challenge for generative neural radiance fields (NERFs) is that a single multiple layer perceptron (MLP) network represents a scene or object, and the shape and appearance of the generated object are unpredictable, owing to the randomness of latent code. In this paper, we propose a stylized generative radiance field (SGRF) to produce 3D-aware images with explicit control. To achieve this goal, we manipulated the input and output of the MLP in the model to entangle and disentangle label codes into/from the latent code, and incorporated an extra discriminator to differentiate between the class and color mode of the generated object. Based on the labels provided, the model could generate images of prohibited items varying in class, pose, scale, and color mode, thereby significantly increasing the quantity and diversity of images in the dataset. Through a systematic analysis of the results, the method was demonstrated to be effective in improving the detection performance of deep learning algorithms during security screening.
Citation: Jian Liu, Zhen Yu, Wenyu Guo. The 3D-aware image synthesis of prohibited items in the X-ray security inspection by stylized generative radiance fields[J]. Electronic Research Archive, 2024, 32(3): 1801-1821. doi: 10.3934/era.2024082
[1] | Shixiong Zhang, Jiao Li, Lu Yang . Survey on low-level controllable image synthesis with deep learning. Electronic Research Archive, 2023, 31(12): 7385-7426. doi: 10.3934/era.2023374 |
[2] | Kunxiao Liu, Guowu Yuan, Hongyu Liu, Hao Wu . Multiscale style transfer based on a Laplacian pyramid for traditional Chinese painting. Electronic Research Archive, 2023, 31(4): 1897-1921. doi: 10.3934/era.2023098 |
[3] | Jicheng Li, Beibei Liu, Hao-Tian Wu, Yongjian Hu, Chang-Tsun Li . Jointly learning and training: using style diversification to improve domain generalization for deepfake detection. Electronic Research Archive, 2024, 32(3): 1973-1997. doi: 10.3934/era.2024090 |
[4] | Wu Zeng, Heng-liang Zhu, Chuan Lin, Zheng-ying Xiao . A survey of generative adversarial networks and their application in text-to-image synthesis. Electronic Research Archive, 2023, 31(12): 7142-7181. doi: 10.3934/era.2023362 |
[5] | Longkui Jiang, Yuru Wang, Weijia Li . Regress 3D human pose from 2D skeleton with kinematics knowledge. Electronic Research Archive, 2023, 31(3): 1485-1497. doi: 10.3934/era.2023075 |
[6] | Yi Dong, Jinjiang Liu, Yihua Lan . A classification method for breast images based on an improved VGG16 network model. Electronic Research Archive, 2023, 31(4): 2358-2373. doi: 10.3934/era.2023120 |
[7] | Wangwei Zhang, Menghao Dai, Bin Zhou, Changhai Wang . MCADFusion: a novel multi-scale convolutional attention decomposition method for enhanced infrared and visible light image fusion. Electronic Research Archive, 2024, 32(8): 5067-5089. doi: 10.3934/era.2024233 |
[8] | Jinjiang Liu, Yuqin Li, Wentao Li, Zhenshuang Li, Yihua Lan . Multiscale lung nodule segmentation based on 3D coordinate attention and edge enhancement. Electronic Research Archive, 2024, 32(5): 3016-3037. doi: 10.3934/era.2024138 |
[9] | Longkui Jiang, Yuru Wang, Xinhe Ji . Calibrated deep attention model for 3D pose estimation in the wild. Electronic Research Archive, 2023, 31(3): 1556-1569. doi: 10.3934/era.2023079 |
[10] | Youzi He, Bin Li, Tingting Sheng, Xianchao Wang . Generating geometric body shapes with electromagnetic source scattering techniques. Electronic Research Archive, 2020, 28(2): 1107-1121. doi: 10.3934/era.2020061 |
The merging of neural radiance fields with generative adversarial networks (GANs) can synthesize novel views of objects from latent code (noise). However, the challenge for generative neural radiance fields (NERFs) is that a single multiple layer perceptron (MLP) network represents a scene or object, and the shape and appearance of the generated object are unpredictable, owing to the randomness of latent code. In this paper, we propose a stylized generative radiance field (SGRF) to produce 3D-aware images with explicit control. To achieve this goal, we manipulated the input and output of the MLP in the model to entangle and disentangle label codes into/from the latent code, and incorporated an extra discriminator to differentiate between the class and color mode of the generated object. Based on the labels provided, the model could generate images of prohibited items varying in class, pose, scale, and color mode, thereby significantly increasing the quantity and diversity of images in the dataset. Through a systematic analysis of the results, the method was demonstrated to be effective in improving the detection performance of deep learning algorithms during security screening.
X-ray security screening is a preeminent protection for public safety in various venues such as airports, railway stations, undergrounds, post offices, and customs. To this end, X-ray scanning machines are deployed to detect and expose prohibited items concealed within the baggage, luggage, or cargo. Human operators play pivotal roles in threat screening[1]. The expertise and proficiency of inspectors are indispensable for the accurate detection of threats; nevertheless, other factors, such as emotional fluctuation and physical fatigue, tend to divert their concentration. The necessity for a real-time, dependable, and automatic method for security screening is becoming increasingly pressing owing to the challenging conditions encountered during the checking process. Specifically, the random arrangement and extensive overlapping of content in luggage leads to highly occluded images, which significantly complicates the task of identifying prohibited items amidst cluttered backgrounds for inspectors [2].
Despite the growing interest in computer-assisted techniques to enhance the alertness and detection capabilities of screeners, research in this area has been understudied owing to the scarcity of extensive datasets and sophisticated deep-learning algorithms. Previous studies have primarily focused on conventional image analysis [3,4,5] and machine learning. Most recently, deep learning methods, especially convolutional neural networks (CNN), have demonstrated superior performance over conventional machine learning methods for threat object classification [6,7,8], detection [9,10] and segmentation [11,12]. Nevertheless, most methods merely achieve high accuracy and recovery rates on specific datasets.
The principle underlying X-ray imaging is the penetration of an X-ray beam through a scanned object and its subsequent detection using a photoelectric sensor. The intensity of the X-ray signal is inversely proportional to the density of the object material, thereby enabling determination of the internal structure of the object. The formula for attenuation is Ix=I0e−μx, where I0 is the initial density, Ix is the attenuated density at x cm, and μ is the linear attenuation coefficient [13]. The formulation implies that Ix is correlated with the object thickness x and the nature of its material. The influence of the object thickness is mitigated through the utilization of dual- or multi-energy imaging technology, which enables the determination of the object's density and properties, in particular, the effective atomic number Zeff. Owing to the sensitivity of human perception to color, the density and effective atom number are converted to pseudo-color images or other color modes using lookup tables. The process of generating X-ray inspection images is depicted in Figure 1. In pseudo-color mode, different materials exhibit different colors during X-ray imaging. Conventionally, blue, orange, and green refer to the inorganic, organic, and mixed materials, respectively.
Data generation is an effective strategy for improving the performance of deep-learning methods, particularly in the context of prohibited object detection during X-ray security inspections. This is because the process of acquiring and annotating a large number of X-ray images in a real-world setting is both expensive and time-consuming. Nevertheless, in recent years, researchers have made tremendous efforts to create large-scale X-ray inspection datasets. Several commonly cited benchmarks include GDXray, UCL TIP, SIXray, and OPIXray [14,15,16,17]. With flourishing contemporary generative adversarial network (GAN) technology, its outstanding image-generation capacity has received wide attention. Various attempts have been made to synthesize X-ray images of prohibited items using GANs. For instance, Yang et al. [18] proposed a generative model based on improvements to WGAN-GP to generate ten classes of prohibited items using real images. Zhu et al. [19] attempted to synthesize images using SAGAN and CycleGAN to enrich the diversity of the threat image database, although the quality of the generated images could be improved. Liu et al. [20] developed a comprehensive framework based on GAN to synthesize X-ray inspection images for data enhancement.
Inspired by the concept of generative radiance fields (GRAF), we propose a stylized generative radiance fields (SGRF) that enables controllable synthesis of 3D-aware images. The framework of the model is shown in Figure 2, which will be described in detail in other sections. This model can generate images of prohibited items based on label prompts and serves as an implicit representation database, producing a large number of images with rich diversity in class, color mode, pose, etc. More specifically, we make the following contributions.
● We implemented a stylized generative radiance field to learn the implicit representation of multiple objects with different color styles using a single multilayer perceptron (MLP).
● We attained explicit control over the generation of 3D-aware images by entangling and disentangling class and style labels into/from random latent codes.
● We utilized the model to significantly augment the X-ray inspection datasets and effectively increase the generalization ability of state-of-the-art detection algorithms in security screening.
The rest of this paper is organized as follows. Section 2 presents a literature review of the 2D and 3D image synthesis methods. Section 3 describes stylized generative radiance field methodology. Section 4 describes the experimental setup and details. Section 5 presents the experimental results for the proposed model. Section 6 concludes the paper and provides recommendations for future research.
GAN: In 2014, Goodfellow et al. [21] introduced GANs, which are deep generative models inspired by the game theory. During the training process, generator G and discriminator D compete to reach a Nash equilibrium state. The principle of G is to generate as much fake data as possible to fit the potential distribution of the real data, whereas the principle of D is to correctly distinguish real data from fake data. The input of G is a random noise vector z (usually a uniform or normal distribution). The noise was mapped to a new data space via G to obtain a fake sample G(z), which is a multidimensional vector. The discriminator D is a binary classifier that takes both the real sample from the dataset and the fake sample generated by G as the input, and the output of D represents the probability that the sample is real rather than fake. The architecture of GAN is illustrated in Figure 3.
Following this principle, various GANs were developed for image generation and translation [22,23]. Initially, GAN adopted a fully connected MLP as the generator and discriminator. Taking advantage of a CNN, Radford et al. [24] proposed a deep convolutional generative adversarial network (DCGAN) that achieved superior performance in image generation. Owing to the use of random latent vectors as inputs, unrestricted variables may result in collapse of the training process. To address this issue, conditional GANs, such as CGAN, ACGAN, and InfoGAN [25,26,27] have incorporated conditional variables (including labels, text, or other relevant data) into both the generator and discriminator of the model. These modifications result in a more robust training process and the ability to generate images based on specific conditions. Moreover, considerable effort has been devoted to optimizing the objective function for stabilizing Gan's training. For example, Arjovsky et al. [28] proposed a Wasserstein generative adversarial network (WGAN). They first showed theoretically that the Earth-Mover (EM) distance produces better gradient behaviors than other distance metrics. Gulrajani et al. [29] presented a gradient penalty named WGAN-GP to enforce the Lipschitz constraint, which performs better than the original WGAN. Petzka et al. [30] introduced a new penalty term known as the WGAN-LP to enforce the Lipschitz constraint.
Image translation, or converting images from one domain to another, is another primary task of GANs. Isola et al. [31] utilized a pix-to-pix GAN to perform image adaptation between pairs of images. Subsequently, the HD pix-to-pix GAN [32] enhanced the quality and resolution of the generated images up to 2048 × 1024 pixels. Owing to the difficulties in obtaining paired data in real-world scenarios, CycleGAN, DiscoGAN, and DualGAN [33,34,35] employed a term of cyclic consistency to train the model using unpaired data. Choi et al. [36] proposed StarGAN, which accomplished image translation across multiple domains by using a single model.
To date, contemporary GAN technology has achieved outstanding performance in synthesizing high-resolution photo-realistic 2D images; however, GAN cannot synthesize novel views of objects, and the generated images unable to maintain 3D consistency.
NERF: Neural radiance fields [37] are powerful for learning 3D scene implicit representations, where the scene is represented as a continuous field and stored in a neural network. The neural radiation field can render high-fidelity images from any perspective by training on a set of posed images. The implicit rendering process is illustrated in Figure 4. The inputs are the position o, direction d(θ,ϕ), and the corresponding coordinates (x,y,z) of the emitted light from a certain perspective, which are fed into the neural radiation field to obtain the volume density σ and color (r,g,b) and obtain the final image through volume rendering. To obtain clear images, positional encoding optimizes the networks by mapping a 5D input to a high-dimensional space to represent the high-resolution geometry and appearance. Additionally, a coarse-to-fine strategy was adopted for hierarchical volume sampling to increase rendering efficiency.
NERF has achieved impressive success in view synthesis, image processing, controllable editing, digital human body, and multimodal applications. The limitations of NERF are its slow training and rendering, restriction to static scenes, lack of generalization, and the requirement of a large number of perspectives. To address these issues, Garbin et al. [38] proposed FastNeRF that can render high-fidelity and realistic images at 200 Hz on high-end consumer GPUs. Li et al. [39] presented the neural scene flow fields to expand it to dynamic scenes by learning implicit representation of scenes from monocular videos. Because the NERF requires retraining for a new scene and cannot be extended to unseen scenes, academic research on this topic comprises pixelNeRF and IBRNet [40,41]. Moreover, NERF stores one scene in one fully connected multilayer network (MLP), which restricts the representation of multiple scenes in a single model.
GRAF: Taking advantage of GAN and NERF, GRAF [42] designed a generative model of NERF by integrating a neural field into a GAN generator. The model can generate 3D-aware images from the latent code (noise) and can be trained using a set of unposed images. In addition to changing the perspective, GRAF also allows the modification of the shape and appearance of the generated objects. Although GRAF has demonstrated remarkable capabilities in generating high-resolution images with 3D consistency, its performance in more complex real-world settings is limited, owing to its inability to effectively handle multi-object scenes. GIRAFFE [43] aims to represent scenes as synthetic neural feature fields that can control the camera's pose, position, and angle of objects placed in the scene as well as the shape and appearance of objects. GIRAFFE is a combination of multiple MLP networks, each of which representing a scene or an object. Our goal was to utilize a single MLP to store various objects or scenes. Inspired by previous studies, we designed stylized generative radiance fields to accomplish this objective. Using this model, we exercised explicit control over the generation of objects based on the class and style labels.
GAN is a generative model primarily utilized for image generation and translation tasks, but the synthesized images are unable to maintain multi-view consistency. By contrast, NERFs enable the generation of 3D-aware images owing to the inherent nature of the radiance field. GRAF is a combination of GAN and NERF, and has been proven successful for novel view synthesis from a random latent code, which also allows modification of the shape and appearance of the generated object based on latent code. However, the shapes and appearances of the objects are randomly generated without explicit control. Moreover, the GRAF prototype employs an MLP to learn the implicit representation of one object or scene, leading to high memory consumption in multiple-scene cases. Therefore, we argue for representing multiple scenes using a single MLP and generating objects with explicit control through the SGRF, which has evolved from GRAF. In the following section, we briefly review the NERF and GRAF models, which form the basis of our model.
Neural radiance fields [37] provide the foundation for various NERF-derived models. Its contributions include implicit representation of 3D geometry and differentiable volume rendering.
Implicit representation: Radiance fields provide implicit representations of 3D geometry. The radiance field is a continuous function with the input of the 3D location x = (x,y,z), viewing direction d = (θ,ϕ), output of the emitted color c = (r,g,b), and volume density values σ. The mapping of the function from input to output is implemented using an MLP network FΘ:(x,d)→(c,σ) and its weights are optimized to map each input 5D coordinate to its corresponding volume density and directional emitted color. The network FΘ directly operated on 5D input coordinates performs poorly at representing high-frequency variations in color and geometry because deep networks are biased toward learning low-frequency functions. Positional encoding was used to map a 3D location x∈R3 and viewing direction d ∈S2 into a higher-dimensional space to enable the MLP to more easily approximate a higher-frequency function. Formally, the positional encoding function is defined in Eq (3.1) [37].
γ(p)=[sin(20πp),cos(20πp),(sin(21πp),cos(21πp),…,(sin(2L−1πp),cos(2L−1πp)] | (3.1) |
This function γ(·) is applied separately to each of the three coordinate values in x and the three components of the Cartesian viewing-direction unit vector d. In the experiments, L = 10 for γ(x) and L = 4 for γ(d). In Eq (3.2) [37], an MLP network fΘ(·) is applied to map the resulting features to a color value c∈R3 and volume density σ∈R+.
FΘ:RLx×RLd→R3×R+γ(x),γ(d)↦(c,σ) | (3.2) |
Volume rending: To render a 2D image from the radiance field fΘ(·), the volume density σ(x) can be interpreted as the differential probability of a ray terminating at an infinitesimal particle at the location x. The expected color C(r) of the camera ray r(t) = o + td with near and far bounds tn and tf is calculated using Eq (3.3) [37].
C(r)=∫tftnT(t)σ(r(t))c(r(t),d))dt,whereT(t)=exp(−∫ttnσ(r(s))ds). | (3.3) |
Rendering a view from a continuous NERF requires estimating integral C(r) for a camera ray traced through each pixel of the camera. This continuous integral of C(r) is numerically estimated using deterministic quadrature, which limits the resolution of the representation, because the MLP would only be queried at a fixed discrete set of locations. Instead, a stratified sampling approach was used to partition [tn, tf] into N evenly spaced bins and draw one sample uniformly at random from each bin. This approximation approach estimates the integral value from a discrete set of samples. In Eq (3.4) [37], let (cir,σir)Ni=1 denote the color and volume density values of N random samples along camera ray r. The rendering function π(·) maps these values onto the color value cr. The color value, cr, was calculated using Eq (3.5) [37].
π:(R3×R+)N→R3,{(cir,σir)}↦cr, | (3.4) |
cr=N∑i=1Tirαircir,Tir=i−1∏j=1(1−αjr),αir=1−exp(−σirδir), | (3.5) |
where Tir and αir denote the transmittance and alpha value of sample point i along ray r and δir=‖xi+1r−xir‖2 is the distance between neighboring sample points. Network FΘ is trained with a set of posed images by minimizing the reconstruction loss between observations and predictions. In addition, a hierarchical volume sampling strategy was applied to improve the rending efficiency.
The GRAF [42] comprises a radiance field-based generator and a multi-scale patch discriminator. It differs from NERF in that it attempts to learn a model using unposed images rather than posed images.
The generator takes the camera intrinsic matrix K, camera pose ξ, 2D sampling pattern ν, and shape/appearance codes zs/za as inputs, and predicts the image patches P′. The camera poses ξ=[R|t] sampled from a pose distribution pξ and ν(u, s) determines the center u∈R2 and scale s∈R+ of the virtual K×K patch drawn from a uniform distribution. The shape and appearance variables zs and za are obtained from the shape and appearance distributions ps and pa, respectively.
Ray sampling: K×K patch P(u,s) is determined by a set of 2D image coordinates that describe the location of every pixel of the patch in the image domain Ω. In Eq (3.6) [42], the corresponding 3D rays are uniquely determined by P(u,s), the camera pose ξ, and the intrinsic matrix K.
P(u,s)={(sx+u,sy+v)|x,y∈{−K2,…,K2−1}} | (3.6) |
3D point sampling: As with the NERF, stratified sampling was used to sample N points {xir}Ni=1 along each ray r for the numerical integration of the radiance field.
Conditional radiance fields: The radiance field was implemented using a fully connected neural network with parameter θ. In addition to a regular radiance field, it is conditional on two latent codes: shape code zs and appearance code za, which determine the object's shape and appearance. As shown in Figure 5, the shape encoding h is derived from the positional encoding of γ(x) and shape code zs and then transformed to the volume density σ by density head σθ. The volume density is computed independently without using viewpoint d and appearance code za to disentangle the shape from the appearance during the inference. To predict the color c, the concatenating vector of the shape encoding h, positional encoding of γ(d), and appearance code za are passed to the color head cθ.
Volume rendering: Given the color and volume density (cir,σir)Ni=1 of N points along a ray, the color cr∈R3 of the pixel corresponding to the ray is obtained using the volume rendering operator π. The predicted patch P′ is generated by combining the results of all sampling rays.
The discriminator was implemented as a convolutional neural network with leaky ReLU activation. To accelerate training and inference, the discriminator compares the synthesized patch P′ with a patch P extracted from a real image I drawn from the data distribution pD. To extract a K×K patch from a real image, the real patch P is sampled by querying a real image at 2D image coordinates P(u, s) using a bilinear interpolation operation, which is referred to as Γ(I,ν). It was proven that the discriminator with shared weights was sufficient for all patches even though they were sampled at random locations with different scales. As the scale determines the receptive field of the patch, starting with patches of larger receptive fields to capture the global context, patches with smaller receptive fields are progressively sampled to refine the local details.
During adversarial training, the goal of generator G(θ) is to minimize the objective function, and that of discriminator D(ϕ) is to maximize the objective function. The non-saturating objective function V(θ,ϕ) with R1-regularization is defined in Eq (3.7) [42].
V(θ,ϕ)=Ezs,za,ξ,ν[f(Dϕ(Gθ(zs,za,ξ,ν)))]+EI,ν[f(−Dϕ(Γ(I,ν)))−λ‖∇Dϕ(Γ(I,ν))‖2], | (3.7) |
where f(t)=−log(1+exp(−t)), I denotes an image from the data distribution pD, pν denotes the distribution over random patches, and λ controls the strength of regularization. Spectral and instance normalization were used for the discriminator. RMSprop was used as an optimizer with a batch size of 8 and learning rates of 0.0005 and 0.0001 for the generator and discriminator, respectively.
GRAF can synthesize novel views of an object from latent code, although the generation of the shape and appearance of an object is random and lacks explicit control. To address this issue, we propose the use of SGRF that enable the implicit representation of multiple 3D objects using a single MLP, providing precise control over 3D-aware image synthesis according text prompts (labels).
In the generator, the label codes of the class and style are entangled with a latent vector as the input of the conditional radiance field. This encourages the generator to use label-embedding latent vectors to synthesize objects with explicit control in terms of class and style. The schema of label embedding is illustrated in Figure 6.
Input: The random latent code z∈N(0 ∽ 1) is split into shape code zs and appearance code za. The class and style labels are embedded into the embedding layers, and the label-embedded codes z′s and z′a are produced by multiplying the shape code zs and appearance code za with the label codes.
Output: In contrast to GRAF, which employs a single value of σ and c, the conditional radiance field g(θ) in our model produces a volume density array [σ(i)]N−1i=0 and color array [c(j)]M−1j=0, both indexed by numerical labels. In practice, the number of classes was set to N = 5 and the number of styles at M = 4.
Conditional radiance field: The network structure of conditional radiance fields g(θ) is illustrated in Figure 7. The mechanism of prediction for volume density σ and color c is the similar to that of GRAF. However, the input and output of g(θ) are manipulated for entangling and disentangling label codes accordingly. In practice, a total of 1024 rays are sampled for one image with parameters P(u,s), camera pose ξ and intrinsic K, then 64 points are sampled along each ray r. Here, γ(x), and γ(d) are the positional encoding of the 3D coordinates x and ray direction d of the point. z′s and z′a are the latent codes associated with class and style, respectively.
In addition to using a regular discriminator D(ϕ) to compare the synthesized patch P′ with a real patch P, another auxiliary classifier Dvgg based on VGG16 is added to distinguish the class and style of an object or scene. VGG16 [44] is a classical deep CNN that is typically used for multi-classification tasks owing to its superior generalization ability. Initially, the discriminator Dvgg is trained using the annotated images I′, which are downsampled from real image I.
In the training phase, the loss function guides network optimization. The discriminator D(ϕ) is trained using a real patch P and a synthesized patch P′ with labels. The discriminator Dvgg was trained using a rescaled real image I′. The loss function of G(θ) is expressed by Eq (3.8).
L(G(θ))=Ladv(D(ϕ)|P′i,j)+λ1Lcls(Dvgg|P′i,j)+λ2Lsty(Dvgg|P′i,j), | (3.8) |
where Ladv is the adversarial loss of P and P′, Lcls and Lsty denote the classification and style loss of the generator, respectively, and λ1 and λ2 are weights for Lcls and Lsty. In this experiment, MSE and RMSprop were used as the adversarial loss function and optimizer, respectively, with parameters λ1 = 2.0 and λ2 = 3.0 and a batch size of 8. During the inference phase, class and style labels are embedded into latent codes zs and za, respectively, for controllable synthesis of 3D-aware images.
Owing to the difficulty in obtaining X-ray data in a real scene, we trained the model on a synthetic dataset. In a 3D editing software, such as Blender, an object was set at the origin and the camera was on the surface of the upper hemisphere facing toward the origin of the coordinate system. By manipulating the camera's pose, we can capture natural images of an object from a variable perspective. Subsequently, the captured images were binarized and converted into a semantic map as an input to an HD pix-to-pix GAN. The four types of semantic maps correspond to the four display styles individually. The image translator is a pretrained HD pix-to-pix GAN that is responsible for the style transfer of the captured images. The dataset comprises five classes of images of prohibited items, such as guns, forks, pliers, knives, and scissors, and each class is displayed in four color modes: grey, pseudo, hot jet, and reversed. The image synthesis pipeline is illustrated in Figure 8.
We trained the model on the synthesized dataset prepared in Section 4.1 and compared its results with those of the NERF, GRAF, and GIRAFFE models, respectively. NERF learns 3D geometry from posed images and synthesizes novel views using differentiable volumetric rendering. GRAF generates high-quality 3D-aware images from latent codes of shape and appearance without requiring posed images. GIRAFFE is a combination of multiple MLP networks that represent multiple objects in a given scene.
Frechet inception distance (FID) scores have been extensively adopted to evaluate the quality and diversity of generated images. We calculate the FID scores using Eq (4.1) [45].
FID=d2((mr,Cr),(mg,Cg))=||mr−mg||22+Tr(Cr+Cg−2(CrCg)1/2), | (4.1) |
where pair (mr, Cr) corresponds to real images, pair (mg, Cg) corresponds to generated images, and m and C are the mean and covariance, respectively. We also introduce kernel inception distance (KID) [46], which does not require a normal distribution hypothesis such as FID and is an unbiased estimate. In addition, average precision (AP) and mean average precision (mAP) are the most popular metrics used to evaluate object detection performance.
The model was trained on a synthetic dataset (Section 4.1). We employed an RMSprop optimizer with learning rates of 0.0001 and 0.0005 for the discriminator and the generator, respectively. During the inference phase, the inputs of the MLP are label-embedded latent codes z′s and z′a and view direction d(θ,ϕ). The average inference time was 0.7723 s for each image with a size of 128. In Figures 9 and 10, some X-ray images were predicted based on the class and style labels after 50,000 iterations. In Figure 11, novel views of prohibited items are synthesized by altering the pose of the virtual camera. Here, θ,ϕ are the pitch and yaw angles of the camera in a spherical coordinate system.
The FID score indicates the differences between the real and generated images; the lower the FID score, the better the generative model's performance. As shown in Figure 12, the 'guns' and 'pliers' presented superior quality and diversity compared to other category because they have more complex details in the internal structures than other categories.
Our model was derived from the GRAF model by modifying the inputs and outputs of the MLP in the prototype. Therefore, we performed ablation studies by comparing the results of our model with those of its counterparts. In Table 1, we quantitatively compared the FID and KID scores of GRAF with our model under the pseudo-color mode, indicating no significant variance between the two models.
Class | Gun | Fork | Knife | Pliers | Scissor | |
GRAF | (FID) | 43.55 | 45.25 | 80.14 | 46.92 | 55.35 |
/without | (KID) | 0.038 | 0.029 | 0.084 | 0.042 | 0.048 |
Ours | (FID) | 47.74 | 51.81 | 78.43 | 48.27 | 54.52 |
/with | (KID) | 0.042 | 0.032 | 0.079 | 0.039 | 0.050 |
By fusing the prohibited item image with a benign background image, we synthesized X-ray inspection images using pixel-by-pixel alpha-blending algorithms [20]. A schematic of the synthesis of X-ray inspection images is shown in Figure 13. Some samples of the synthesized images are shown in Figure 14. The prohibited objects were located within red bounding boxes.
In the experiment, we first prepared two datasets: Dataset A, which included 2000 images selected from the real dataset, and Dataset B, which included 1000 images from the real dataset and 1000 synthesized images. Each dataset comprises five classes of prohibited items: guns, forks, knives, pliers, and scissors. The two datasets were then split into training and validation sets in a ratio of 4:1. For validation, we employed YOLOv8 [47] as the object detection paradigm. The model was trained on Datasets A and B separately. The training curves demonstrated that the model trained on Dataset B outperformed the model trained on Dataset A, achieving improvements of approximately 4.4% in mAP0.5 and 11.9% in mAP0.5:0.95.
During the inference phase, Model A was the pretrained model on Dataset A and Model B was the pretrained model on Dataset B. Both models were tested on the same test set, which comprised 500 real images from an available dataset. By evaluating the mAP values of both models in Table 2, Model B achieves superior performance over Model A, demonstrating that augmented Dataset B effectively improves the detection accuracy and generalization ability of the deep learning algorithms. We expect to further enhance the detection performance by adding additional synthesized X-ray inspection images to the training set.
Model | Class | P | R | mAP (0.5) | mAP (0.5:0.95) |
Gun | 0.79 | 0.81 | 0.85 | 0.56 | |
Fork | 0.77 | 0.63 | 0.71 | 0.34 | |
Model | Knife | 0.62 | 0.52 | 0.54 | 0.30 |
(A) | Pliers | 0.72 | 0.68 | 0.73 | 0.47 |
Scissor | 0.71 | 0.46 | 0.60 | 0.42 | |
Mean | 0.72 | 0.62 | 0.68 | 0.42 | |
Gun | 0.93 | 0.91 | 0.92 | 0.67 | |
Fork | 0.78 | 0.55 | 0.61 | 0.37 | |
Model | Knife | 0.65 | 0.43 | 0.56 | 0.38 |
(B) | Pliers | 0.82 | 0.65 | 0.83 | 0.51 |
Scissor | 0.74 | 0.51 | 0.62 | 0.44 | |
Mean | 0.78 | 0.61 | 0.71↑ | 0.47↑ |
In this study, we propose a novel stylized generative radiance field for the controllable synthesis of 3D-aware images. By manipulating the input and output of the conditional radiance field (MLP) and incorporating a new discriminator (VGG16), we enabled the entanglement and disentanglement of class and style labels into and from random latent vectors, thereby achieving explicit control over the generation of prohibited items. Moreover, our model can generate nonexistent images in the training set using transfer learning. The main advantages of this model are that it can learn multiple objects using a single MLP and synthesize novel views according to class and style labels. The experimental results reveal that our image generation model significantly increases the quantity and diversity of the images of prohibited items, and the augmented dataset effectively promotes the accuracy and generalization of object detection algorithms. Furthermore, our proposed model has the potential to be extended to other fields such as medial image generation. In the future, we plan to add more subclasses to a class, such as different types of guns in a gun class, enriching the diversity in the 3D geometry of prohibited items within one category.
The authors declare they have not used Artificial Intelligence (AI) tools in the creation of this article.
This work has been supported by Henan Province Program for Science and Technology Development under Grant No. 162102210009.
The authors declare there are no conflicts of interest.
[1] |
A. Chavaillaz, A. Schwaninger, S. Michel, J. Sauer, Expertise, automation and trust in X-ray screening of cabin baggage, Front. Psychol., 10 (2019), 256. https://doi.org/10.3389/fpsyg.2019.00256 doi: 10.3389/fpsyg.2019.00256
![]() |
[2] | D. Turcsany, A. Mouton, T. P. Breckon, Improving feature-based object recognition for X-ray baggage security screening using primed visual words, in 2013 IEEE International Conference on Industrial Technology (ICIT), IEEE, (2013), 1140–1145. https://doi.org/10.1109/ICIT.2013.6505833 |
[3] | Z. Chen, Y. Zheng, B. R. Abidi, D. L. Page, M. A. Abidi, A combinational approach to the fusion, de-noising and enhancement of dual-energy X-ray luggage images, in 2005 IEEE Conference on Computer Vision and Pattern Recognition (CVPR) Workshops, IEEE, (2005), 2. https://doi.org/10.1109/CVPR.2005.386 |
[4] |
B. R. Abidi, Y. Zheng, A. V. Gribok, M. A. Abidi, Improving weapon detection in single energy X-ray images through pseudo coloring, IEEE Trans. Syst. Man Cybern. Part C Appl. Rev., 36 (2006), 784–796. https://doi.org/10.1109/TSMCC.2005.855523 doi: 10.1109/TSMCC.2005.855523
![]() |
[5] |
Q. Lu, R. W. Conners, Using image processing methods to improve the explosive detection accuracy, IEEE Trans. Syst. Man Cybern. Part C Appl. Rev., 36 (2006), 750–760. https://doi.org/10.1109/TSMCC.2005.855532 doi: 10.1109/TSMCC.2005.855532
![]() |
[6] | T. W. Rogers, N. Jaccard, E. J. Morton, L. D. Griffin, Detection of cargo container loads from X-ray images, in 2nd IET International Conference on Intelligent Signal Processing 2015 (ISP), (2015), 1–6. https://doi.org/10.1049/cp.2015.1762 |
[7] | M. Kundegorski, S. Akçay, M. Devereux, A. Mouton, T. Breckon, On using feature descriptors as visual words for object detection within X-ray baggage security screening, in 7th International Conference on Imaging for Crime Detection and Prevention (ICDP), (2016), 1–6. https://doi.org/10.1049/ic.2016.0080 |
[8] | D. Mery, E. Svec, M. Arias, Object recognition in baggage inspection using adaptive sparse representations of X-ray images, in Image and Video Technology, Springer, 9431 (2016), 709–720. https://doi.org/10.1007/978-3-319-29451-3_56 |
[9] | T. Franzel, U. Schmidt, S. Roth, Object detection in multi-view X-ray images, in Pattern Recognition, Springer, 7476 (2012), 144–154. https://doi.org/10.1007/978-3-642-32717-9_15 |
[10] |
M. Bastan, Multi-view object detection in dual-energy X-ray images, Mach. Vision Appl., 26 (2015), 1045–1060. https://doi.org/10.1007/s00138-015-0706-x doi: 10.1007/s00138-015-0706-x
![]() |
[11] | G. Heitz, G. Chechik, Object separation in X-ray image sets, in 2010 IEEE Conference on Computer Vision and Pattern Recognition (CVPR), IEEE, (2010), 2093–2100. https://doi.org/10.1109/CVPR.2010.5539887 |
[12] | O. K. Stamatis, N. Aouf, D. Nam, C. Belloni, Automatic X-ray image segmentation and clustering for threat detection, in Proceedings Volume 10432, Target and Background Signatures III, (2017), 104320O. https://doi.org/10.1117/12.2277190 |
[13] | D. Mery, Computer Vision Technology for X-ray Testing, Springer, 2015. https://doi.org/10.1007/978-3-319-20747-6 |
[14] |
D. Mery, V. Riffo, U. Zscherpel, G. Mondragón, I. Lillo, I. Zuccar, et al., GDXray: The database of X-ray images for nondestructive testing, J. Nondestr. Eval., 34 (2015), 42. https://doi.org/10.1007/s10921-015-0315-7 doi: 10.1007/s10921-015-0315-7
![]() |
[15] | T. W. Rogers, N. Jaccard, E. D. Protonotarios, J. Ollier, E. J. Morton, L. D. Griffin, Threat image projection (TIP) into X-ray images of cargo containers for training humans and machines, in 2016 IEEE International Carnahan Conference on Security Technology (ICCST), IEEE, (2016), 1–7. https://doi.org/10.1109/CCST.2016.7815717 |
[16] | C. Miao, L. Xie, F. Wan, C. Su, H. Liu, J. Jiao, et al., Sixray : A large-scale security inspection x-ray benchmark for prohibited item discovery in overlapping images, in 2019 IEEE/CVF Conference on Computer Vision and Pattern Recognition (CVPR), IEEE, (2019), 2114–2123. https://doi.org/10.1109/CVPR.2019.00222 |
[17] | Y. Wei, R. Tao, Z. Wu, Y. Ma, L. Zhang, X. Liu, Occluded prohibited items detection: an X-ray security inspection benchmark and de-occlusion attention module, in Proceedings of the 28th ACM International Conference on Multimedia, ACM, (2020), 138–146. https://doi.org/10.1145/3394171.3413828 |
[18] |
J. Yang, Z. Zhao, H. Zhang, Y. Shi, Data augmentation for X-ray prohibited item images using generative adversarial networks, IEEE Access, 7 (2019), 28894–28902. https://doi.org/10.1109/ACCESS.2019.2902121 doi: 10.1109/ACCESS.2019.2902121
![]() |
[19] |
Y. Zhu, Y. Zhang, H. Zhang, J. Yang, Z. Zhao, Data augmentation of X-ray images in baggage inspection based on generative adversarial networks, IEEE Access, 8 (2020), 86536–86544. https://doi.org/10.1109/ACCESS.2020.2992861 doi: 10.1109/ACCESS.2020.2992861
![]() |
[20] |
J. Liu, T. H. Lin, A framework for the synthesis of X-ray security inspection images based on generative adversarial networks, IEEE Access, 11 (2023), 63751–63760. https://doi.org/10.1109/ACCESS.2023.3288087 doi: 10.1109/ACCESS.2023.3288087
![]() |
[21] | I. Goodfellow, NIPS 2016 Tutorial: Generative adversarial networks, preprint, arXiv: 1701.00160. |
[22] |
X. Wu, K. Xu, P. Hall, A survey of image synthesis and editing with generative adversarial networks, Tsinghua Sci. Technol., 22 (2017), 660–674. https://doi.org/10.23919/TST.2017.8195348 doi: 10.23919/TST.2017.8195348
![]() |
[23] |
Z. Pan, W. Yu, X. Yi, A. Khan, F. Yuan, Y. Zheng, Recent progress on generative adversarial networks (GANs): A survey, IEEE Access, 7 (2019), 36322–36333. https://doi.org/10.1109/ACCESS.2019.2905015 doi: 10.1109/ACCESS.2019.2905015
![]() |
[24] | A. Radford, L. Metz, S. Chintala, Unsupervised representation learning with deep convolutional generative adversarial networks, preprint, arXiv: 1511.06434. |
[25] | M. Mirza, S. Osindero, Conditional generative adversarial nets, preprint, arXiv: 1411.1784. |
[26] | A. Odena, C. Olah, J. Shlens, Conditional image synthesis with auxiliary classifier GANs, preprint, arXiv: 1610.09585. |
[27] | X. Chen, Y. Duan, R. Houthooft, J. Schulman, I. Sutskever, P. Abbeel, InfoGAN: Interpretable representation learning by information maximizing generative adversarial nets, preprint, arXiv: 1606.03657. |
[28] | M. Arjovsky, S. Chintala, L. Bottou, Wasserstein generative adversarial networks, in International Conference on Machine Learning, PMLR, (2017), 214–223. |
[29] | I. Gulrajani, F. Ahmed, M. Arjovsky, V. Dumoulin, A. C. Courville, Improved training of wasserstein GANs, in Proceedings of the 31st International Conference on Neural Information Processing Systems, Curran Associates, Inc., (2017), 5769–5779. |
[30] | H. Petzka, A. Fischer, D. Lukovnicov, On the regularization of wasserstein GANs, preprint, arXiv: 1709.08894. |
[31] | P. Isola, J. Y. Zhu, T. Zhou, A. A. Efros, Image-to-image translation with conditional adversarial networks, in 2017 IEEE Conference on Computer Vision and Pattern Recognition (CVPR), IEEE, (2017), 5967–5976. https://doi.org/10.1109/CVPR.2017.632 |
[32] | T. C. Wang, M. Y. Liu, J. Y. Zhu, A. Tao, J. Kautz, B. Catanzaro, High-resolution image synthesis and semantic manipulation with conditional GANs, in 2018 IEEE/CVF Conference on Computer Vision and Pattern Recognition, IEEE, (2018), 8798–8807. https://doi.org/10.1109/CVPR.2018.00917 |
[33] | J. Y. Zhu, T. Park, P. Isola, A. A. Efros, Unpaired image-to-image translation using cycle-consistent adversarial networks, in 2017 IEEE International Conference on Computer Vision (ICCV), IEEE, (2018), 2242–2251. https://doi.org/10.1109/ICCV.2017.244 |
[34] | T. Kim, M. Cha, H. Kim, J. K. Lee, J. Kim, Learning to discover cross-domain relations with generative adversarial networks, in Proceedings 34th International Conference Machine Learning, PMLR, (2017), 1857–1865. |
[35] | Z. Yi, H. Zhang, P. Tan, M. Gong, DualGAN: Unsupervised dual learning for image-to-image translation, in Proceedings of the IEEE International Conference on Computer Vision (ICCV), IEEE, (2017), 2849–2857. |
[36] | Y. Choi, M. Choi, M. Kim, J. W. Ha, S. Kim, J. Choo, StarGAN: Unified generative adversarial networks for multi-domain image-to-image translation, in 2018 Conference on Computer Vision and Pattern Recognition (CVPR), IEEE, (2018), 8789–8797. https://doi.org/10.1109/CVPR.2018.00916 |
[37] | B. Mildenhall, P. Srinivasan, M. Tancik, J. T. Barron, R. Ramamoorthi, R. Ng, NeRF: Representing scenes as neural radiance fields for view Synthesis, preprint, arXiv: 2003.08934. |
[38] | S. J. Garbin, M. Kowalski, M. Johnson, J. Shotton, J. Valentin, Fastnerf: High-fidelity neural rendering at 200fps, in Proceedings of the IEEE/CVF Conference on Computer Vision and Pattern Recognition (CVPR), IEEE, (2021), 14346–14355. |
[39] | Z. Li, S. Niklaus, N. Snavely, N. Snavely, O. Wang, Neural scene flow fields for space-time view synthesis of dynamic scenes, in Proceedings of the IEEE/CVF Conference on Computer Vision and Pattern Recognition (CVPR), IEEE, (2021), 6498–6508. |
[40] | A. Yu, V. Ye, M. Tancik, M. Tancik, A. Kanazawa, Pixelnerf: Neural radiance fields from one or few images, in Proceedings of the IEEE/CVF Conference on Computer Vision and Pattern Recognition (CVPR), IEEE, (2021), 4578–4587. |
[41] | Q. Wang, Z. Wang, K. Genova, P. P. Srinivasan, H. Zhou, J. T. Barron, et al., IBRnet: Learning multi-view image-based rendering, in 2021 Conference on Computer Vision and Pattern Recognition (CVPR), IEEE, (2021), 4688–4697. https://doi.org/10.1109/CVPR46437.2021.00466 |
[42] | K. Schwarz, Y. Liao, M. Niemeyer, A. Geiger, GRAF: Generative radiance fields for 3D-aware image synthesis, preprint, arXiv: 2007.02442. |
[43] | M. Niemeyer, A. Geiger, GIRAFFE: Representing scenes as compositional generative neural feature fields, in 2021 Conference on Computer Vision and Pattern Recognition (CVPR), IEEE, (2021), 11448–11459. https://doi.org/10.1109/CVPR46437.2021.01129 |
[44] | K. Simonyan, A. Zisserman, Very deep convolutional networks for large-scale image recognition, preprint, arXiv: 1409.1556. |
[45] | Q. Xu, G. Huang, Y. Yuan, C. Guo, Y. Sun, F. Wu, et al., An empirical study on evaluation metrics of generative adversarial net, preprint, arXiv: 1806.07755. |
[46] | M. Bińkowski, D. J. Sutherland, M. Arbel, A. Gretton, Demystifying MMD GANs, preprint, arXiv: 1801.01401. |
[47] | Ultralytics, YOLOv8 Project, GitHub. Available from: https://github.com/ultralytics/ultralytics. |
Class | Gun | Fork | Knife | Pliers | Scissor | |
GRAF | (FID) | 43.55 | 45.25 | 80.14 | 46.92 | 55.35 |
/without | (KID) | 0.038 | 0.029 | 0.084 | 0.042 | 0.048 |
Ours | (FID) | 47.74 | 51.81 | 78.43 | 48.27 | 54.52 |
/with | (KID) | 0.042 | 0.032 | 0.079 | 0.039 | 0.050 |
Model | Class | P | R | mAP (0.5) | mAP (0.5:0.95) |
Gun | 0.79 | 0.81 | 0.85 | 0.56 | |
Fork | 0.77 | 0.63 | 0.71 | 0.34 | |
Model | Knife | 0.62 | 0.52 | 0.54 | 0.30 |
(A) | Pliers | 0.72 | 0.68 | 0.73 | 0.47 |
Scissor | 0.71 | 0.46 | 0.60 | 0.42 | |
Mean | 0.72 | 0.62 | 0.68 | 0.42 | |
Gun | 0.93 | 0.91 | 0.92 | 0.67 | |
Fork | 0.78 | 0.55 | 0.61 | 0.37 | |
Model | Knife | 0.65 | 0.43 | 0.56 | 0.38 |
(B) | Pliers | 0.82 | 0.65 | 0.83 | 0.51 |
Scissor | 0.74 | 0.51 | 0.62 | 0.44 | |
Mean | 0.78 | 0.61 | 0.71↑ | 0.47↑ |
Class | Gun | Fork | Knife | Pliers | Scissor | |
GRAF | (FID) | 43.55 | 45.25 | 80.14 | 46.92 | 55.35 |
/without | (KID) | 0.038 | 0.029 | 0.084 | 0.042 | 0.048 |
Ours | (FID) | 47.74 | 51.81 | 78.43 | 48.27 | 54.52 |
/with | (KID) | 0.042 | 0.032 | 0.079 | 0.039 | 0.050 |
Model | Class | P | R | mAP (0.5) | mAP (0.5:0.95) |
Gun | 0.79 | 0.81 | 0.85 | 0.56 | |
Fork | 0.77 | 0.63 | 0.71 | 0.34 | |
Model | Knife | 0.62 | 0.52 | 0.54 | 0.30 |
(A) | Pliers | 0.72 | 0.68 | 0.73 | 0.47 |
Scissor | 0.71 | 0.46 | 0.60 | 0.42 | |
Mean | 0.72 | 0.62 | 0.68 | 0.42 | |
Gun | 0.93 | 0.91 | 0.92 | 0.67 | |
Fork | 0.78 | 0.55 | 0.61 | 0.37 | |
Model | Knife | 0.65 | 0.43 | 0.56 | 0.38 |
(B) | Pliers | 0.82 | 0.65 | 0.83 | 0.51 |
Scissor | 0.74 | 0.51 | 0.62 | 0.44 | |
Mean | 0.78 | 0.61 | 0.71↑ | 0.47↑ |