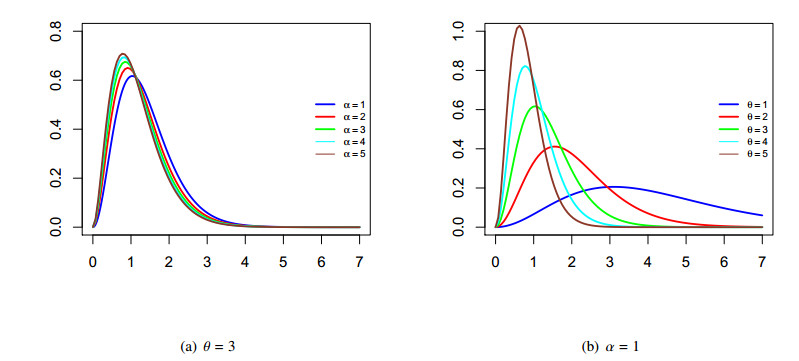
The physical and mechanical properties of graphene-reinforced epoxy (epoxy/graphene) in hygrothermal environment need to be comprehensively understood. This is because it is necessary to predict the durability of epoxy/graphene when epoxy/graphene is used in an aggressive environment with high humidity and high temperature. Based on the molecular dynamics method, the influences of water content (2, 4 and 6%) and temperature (298,333 and 368 K) on the physical and tensile properties of epoxy/graphene were studied in this research. The results showed that after the addition of graphene, the free volume fraction of epoxy and the diffusion coefficient of water molecules in the epoxy decreased, and the density, tensile strength and deformation performance of epoxy increased. In the hygrothermal environment, the tensile strength degradation rate of epoxy/graphene was lower than that of pure epoxy. The failure mechanism and mechanical response of epoxy/graphene during the tensile process in the nanoscale were revealed. The research results provide a reference for the design and performance optimization of epoxy/graphene composites in a hygrothermal environment.
Citation: Xiuli Zhang, Guangming He, Hui Yao, Xuanxi Wang, Guoru Ma, Junliang Li, Zulong Yu, Guozhong Lu, Zhifei Gao. Effect of graphene on the properties of epoxy in hygrothermal environment by molecular dynamics method[J]. Electronic Research Archive, 2023, 31(6): 3510-3533. doi: 10.3934/era.2023178
[1] | M. R. Irshad, S. Aswathy, R. Maya, Amer I. Al-Omari, Ghadah Alomani . A flexible model for bounded data with bathtub shaped hazard rate function and applications. AIMS Mathematics, 2024, 9(9): 24810-24831. doi: 10.3934/math.20241208 |
[2] | Doaa Basalamah, Bader Alruwaili . The weighted Lindley exponential distribution and its related properties. AIMS Mathematics, 2023, 8(10): 24984-24998. doi: 10.3934/math.20231275 |
[3] | Tabassum Naz Sindhu, Zawar Hussain, Naif Alotaibi, Taseer Muhammad . Estimation method of mixture distribution and modeling of COVID-19 pandemic. AIMS Mathematics, 2022, 7(6): 9926-9956. doi: 10.3934/math.2022554 |
[4] | M. G. M. Ghazal, Yusra A. Tashkandy, Oluwafemi Samson Balogun, M. E. Bakr . Exponentiated extended extreme value distribution: Properties, estimation, and applications in applied fields. AIMS Mathematics, 2024, 9(7): 17634-17656. doi: 10.3934/math.2024857 |
[5] | Rashad M. EL-Sagheer, Mohamed S. Eliwa, Khaled M. Alqahtani, Mahmoud El-Morshedy . Bayesian and non-Bayesian inferential approaches under lower-recorded data with application to model COVID-19 data. AIMS Mathematics, 2022, 7(9): 15965-15981. doi: 10.3934/math.2022873 |
[6] | Fiaz Ahmad Bhatti, Azeem Ali, G. G. Hamedani, Mustafa Ç. Korkmaz, Munir Ahmad . The unit generalized log Burr XII distribution: properties and application. AIMS Mathematics, 2021, 6(9): 10222-10252. doi: 10.3934/math.2021592 |
[7] | Ahmed M. Gemeay, Najwan Alsadat, Christophe Chesneau, Mohammed Elgarhy . Power unit inverse Lindley distribution with different measures of uncertainty, estimation and applications. AIMS Mathematics, 2024, 9(8): 20976-21024. doi: 10.3934/math.20241021 |
[8] | Refah Alotaibi, Hassan Okasha, Hoda Rezk, Abdullah M. Almarashi, Mazen Nassar . On a new flexible Lomax distribution: statistical properties and estimation procedures with applications to engineering and medical data. AIMS Mathematics, 2021, 6(12): 13976-13999. doi: 10.3934/math.2021808 |
[9] | Emrah Altun, Mustafa Ç. Korkmaz, M. El-Morshedy, M. S. Eliwa . The extended gamma distribution with regression model and applications. AIMS Mathematics, 2021, 6(3): 2418-2439. doi: 10.3934/math.2021147 |
[10] | Ayed. R. A. Alanzi, Muhammad Imran, M. H. Tahir, Christophe Chesneau, Farrukh Jamal, Saima Shakoor, Waqas Sami . Simulation analysis, properties and applications on a new Burr XII model based on the Bell-X functionalities. AIMS Mathematics, 2023, 8(3): 6970-7004. doi: 10.3934/math.2023352 |
The physical and mechanical properties of graphene-reinforced epoxy (epoxy/graphene) in hygrothermal environment need to be comprehensively understood. This is because it is necessary to predict the durability of epoxy/graphene when epoxy/graphene is used in an aggressive environment with high humidity and high temperature. Based on the molecular dynamics method, the influences of water content (2, 4 and 6%) and temperature (298,333 and 368 K) on the physical and tensile properties of epoxy/graphene were studied in this research. The results showed that after the addition of graphene, the free volume fraction of epoxy and the diffusion coefficient of water molecules in the epoxy decreased, and the density, tensile strength and deformation performance of epoxy increased. In the hygrothermal environment, the tensile strength degradation rate of epoxy/graphene was lower than that of pure epoxy. The failure mechanism and mechanical response of epoxy/graphene during the tensile process in the nanoscale were revealed. The research results provide a reference for the design and performance optimization of epoxy/graphene composites in a hygrothermal environment.
Recently, some researchers in statistics have been interested in generating new flexible statistical models based on different techniques such as the weight distributions which are commonly used in many fields of life situations such as sciences, ecology, biostatistics, medicine, engineering, pharmacy and environment and so on.
The concept of weighted distribution is suggested by [15] to study how verification methods can affect the form of the distribution of recorded observations. Later, the weighted distributions are unified and formulated by [31] in general terms in connection with modeling statistical data.
Suppose X is a non-negative random variable with a probability density function (pdf) f(x). Let W(x) be a non negative weight function, then the probability density function of the weighted random variable Xw is given by:
fw(x)=W(x)f(x)E(W(x)). | (1.1) |
If the weight function has the form W(x)=xλ, the resulting distribution is known as a size biased distribution of order λ with pdf given by:
fλ(x)=xλf(x)E(Xλ). | (1.2) |
If λ = or 2, the yielded distributions are known as the length biased and area biased distributions, respectively.
For example, the length-biased Suja distribution is proposed by [5] as a generalization of the Suja distribution. The size biased Ishita distribution is offered by [6] as a new modification of the Ishita distribution. The Marshall-Olkin length-biased exponential distribution is suggested by [18]. The length-biased weighted generalized Rayleigh distribution with its properties is proposed by [2]. The weighted Lomax distribution is introduced by [23]. Other types of distributions are also suggested based on other procedures as [8] for the exponentiated new Weibull-Pareto distribution. The Topp-Leone Mukherjee-Islam distribution is offered by [7]. Also, see [27,16,17,26].
To the best of our knowledge, the use of the weighted method to extend the generalized Quasi Lindley distribution introduced by [9] is still unexplored in the literature. In this study, the weighted generalized Quasi Lindley distribution is proposed. Indeed, the importance of the suggestion of the WGQLD arises from the fact it is a modification of the well known Quasi Lindley distribution which is considered by many researchers in different life situations.
The layout of this paper is organized as follows. Section 2 concerns with the pdf and cumulative distribution function (cdf) of the WGQLD and its shapes. Moment generating function and moments includes the rth moment, variance, the coefficient of skewness, kurtosis, and variation are presented in Section 3 theoretically and supported by some simulations. The distribution of order statistics, median deviations and harmonic mean are presented in Section 4. The stochastic ordering, reliability, hazard, reversed hazard rate and odds functions are given in Section 5. Bonferroni and Lorenz curves as well as the Gini index are provided in Section 6. In Section 7, different methods of estimation for the distribution parameters are discussed including maximum likelihood, maximum product of spacing's, ordinary least squares, weighted least squares, Cramer-von-Mises, and Anderson-Darling methods. A simulation study is conducted to compare the performance of the proposed estimators in Section 8. Illustrative examples of real data applications are given in Section 9. The paper is concluded with some suggestions for future works in Section 10.
This section introduces the pdf and cdf of the WGQLD along with the shapes of the model. The probability density function of the generalized Quasi Lindley distribution is given by:
fGQLD(x;θ,α)=θ2(θ2x36+αθx2+α2x)(α+1)2e−θx; x≥0, α>−1, θ≥0, | (2.1) |
and the corresponding cdf is:
FGQLD(x;θ,α)=1−(θ3x3+3(2α+1)θ2x2+6(α+1)2(θx+1))6(α+1)2e−θx. | (2.2) |
With reference to Eq 1.1, and in this work without loss of generality we considered the weight function as W(x)=x given that the mean of the GQLD is E(X)=2(2+α)θ(1+α). Hence, the weighted generalized Quasi Lindley distribution can be obtained by substituting fGQLD(x;θ,α), E(X) and W(x) in Eq 1.1 to get:
fWGQLD(x;θ,α)=θ32(α2+3α+2)(θ2x46+αθx3+α2x2)e−θx; x≥0, α>−1, θ≥0. | (2.3) |
Figures 1 and 2 demonstrate the graphs of the pdf of the WGQLD for different values of θ and α. Also, Figures 3 and 4 show various curves of the cdf of WGQLD for selected distribution parameters.
Based on Figures 1 and 2, it can be observed that the WGQLD is a unimodel and positively skewed. Also, it is approximately symmetric as in Figure 2 when θ=1 for some parameters. The curve of the distribution function is more flat for α=1,θ=0.25 and when α=θ=1. than other values.
The corresponding cdf equation of the WGQLD is given by
FWGQLD(x;θ,α)=1−24+6α2[2+xθ(2+xθ)]+6α[6+xθ(6+xθ(3+xθ))]+xθ[24+xθ(12+xθ(4+xθ))]12(1+α)(2+α)e−θx. | (2.4) |
Figure 3 reveals that the cdf plots are approach 1 when θ=3 (a) faster than that of α=1 (b). In comparing Figure 3(b) with Figure 4(b), it can be observed that the cdf plots are more spread with smaller values of θ. However, the same thing can be concluded in comparing Figure 3(a) with Figure 4(a).
Theorem 1. : Let X∼fWGQLD(x,θ,α), then the rth moment of X about the origin is:
E(Xr)=(r2+6(1+α)(2+α)+r(7+6α))Γ(3+r)12θr(2+3α+α2),α>−1,θ>0, r=1,2,3... | (3.1) |
Proof. : The proof is direct by using E(Xr)=∫∞0xrf(x,θ,α)dx as
E(Xr)=∞∫0xrθ32(α2+3α+2)(α2x2+αθx3+θ2x46)e−θxdx=θ32(α2+3α+2)∞∫0(xrα2x2e−θx+xrαθx3e−θx+xrθ2x46e−θx)dx=θ32(α2+3α+2)(∞∫0xrα2x2e−θxdx+∞∫0xrαθx3e−θxdx+∞∫0xrθ2x46e−θxdx)=θ32(α2+3α+2)(∞∫0xr+2α2e−θxdx+∞∫0xr+3αθe−θxdx+∞∫016xr+4θ2e−θxdx)=θ32(α2+3α+2)(α2(r+2)!θr+3+αθ(r+3)!θr+4+θ2(r+3)!6θr+5)=θ32(α2+3α+2)(α2(r+2)!θr+3+α(r+3)(r+2)!θr+3+(r+4)(r+3)(r+2)!6θr+3)=(r+2)!12θr(α2+3α+2)(6α2+6α(r+3)+(r+4)(r+3))=[r2+6(1+α)(2+α)+r(7+6α)]Γ[3+r]12θr(2+3α+α2). |
Based on Eq 7, it is simple to have the first, second, third and fourth moments of the WGQLD, respectively, as
E(X)=6(α+1)(α+2)+6α+82(α2+3α+2)θ=3α(α+4)+10(α+1)(α+2)θ, | (3.2) |
E(X2)=2(2(6α+7)+6(α+1)(α+2)+4)(α2+3α+2)θ2=12(α(α+5)+5)(α+1)(α+2)θ2, | (3.3) |
E(X3)=10(3(6α+7)+6(α+1)(α+2)+9)(α2+3α+2)θ3=60(α(α+6)+7)(α+1)(α+2)θ3, | (3.4) |
E(X4)=60(4(6α+7)+6(α+1)(α+2)+16)(α2+3α+2)θ4=120(3α(α+7)+28)(α+1)(α+2)θ4. | (3.5) |
Therefore, the variance of the WGQLD can be obtained as
V(X)=E(X2)−(E(X))2=3α4+24α3+60α2+60α+20(α+1)2(α+2)2θ2. | (3.6) |
The distribution shape analysis can be performed by studying the coefficient of skewness, coefficient of kurtosis, and coefficient of variation. For the WGQLD, these coefficients, respectively, are given by:
SKWGQLD=2(3α6+36α5+150α4+306α3+330α2+180α+40)(α+1)(α+2)(α+1)(α+2)(3α4+24α3+60α2+60α+20)32, | (3.7) |
KuWGQLD=315α8+240α7+1496α6+4968α5+9776α4+11760α3+8480α2+3360α+560(3α4+24α3+60α2+60α+20)2, | (3.8) |
CVWGQLD=(α+1)(α+2)√3α4+24α3+60α2+60α+20(3α2+12α+10)(α+1)(α+2). | (3.9) |
It is of interest to note here that the coefficient of skewness, coefficient of variation and coefficient of kurtosis are free of θ. Table (1) presents some values of the mean, standard deviation, coefficient of variation, coefficient of skewness and coefficient of kurtosis of WGQLD for various values of the parameters α and θ.
μWGQLD | σWGQLD | SKWGQLD | KuWGQLD | CVWGQLD | |
α | θ=1.25 | ||||
0.1 | 3.889177 | 1.786969 | 0.897106 | 4.205054 | 0.459472 |
0.2 | 3.793939 | 1.782395 | 0.903213 | 4.217285 | 0.469801 |
0.3 | 3.711037 | 1.776240 | 0.910968 | 4.233681 | 0.478637 |
0.4 | 3.638095 | 1.769174 | 0.919440 | 4.252427 | 0.486291 |
0.5 | 3.573333 | 1.761615 | 0.928126 | 4.272400 | 0.492989 |
0.6 | 3.515385 | 1.753829 | 0.936749 | 4.292896 | 0.498901 |
0.7 | 3.463181 | 1.745987 | 0.945154 | 4.313464 | 0.504157 |
0.8 | 3.415873 | 1.738199 | 0.953260 | 4.333820 | 0.508859 |
0.9 | 3.372777 | 1.730537 | 0.961027 | 4.353780 | 0.513090 |
1 | 3.333333 | 1.723046 | 0.968437 | 4.373230 | 0.516914 |
1.1 | 3.297081 | 1.715755 | 0.975492 | 4.392101 | 0.520386 |
1.2 | 3.263636 | 1.708680 | 0.982196 | 4.410356 | 0.523551 |
1.3 | 3.232675 | 1.701828 | 0.988563 | 4.427977 | 0.526446 |
1.4 | 3.203922 | 1.695202 | 0.994608 | 4.444961 | 0.529102 |
1.5 | 3.177143 | 1.688801 | 1.000346 | 4.461313 | 0.531547 |
θ | α=2 | ||||
0.1 | 38.333333 | 20.749833 | 1.025012 | 4.534152 | 0.5413 |
0.2 | 19.166667 | 10.374916 | 1.025012 | 4.534152 | 0.5413 |
0.3 | 12.777778 | 6.916611 | 1.025012 | 4.534152 | 0.5413 |
0.4 | 9.583333 | 5.187458 | 1.025012 | 4.534152 | 0.5413 |
0.5 | 7.666667 | 4.149967 | 1.025012 | 4.534152 | 0.5413 |
0.6 | 6.388889 | 3.458305 | 1.025012 | 4.534152 | 0.5413 |
0.7 | 5.476190 | 2.964262 | 1.025012 | 4.534152 | 0.5413 |
0.8 | 4.791667 | 2.593729 | 1.025012 | 4.534152 | 0.5413 |
0.9 | 4.259259 | 2.305537 | 1.025012 | 4.534152 | 0.5413 |
1 | 3.833333 | 2.074983 | 1.025012 | 4.534152 | 0.5413 |
1.1 | 3.484848 | 1.886348 | 1.025012 | 4.534152 | 0.5413 |
1.2 | 3.194444 | 1.729153 | 1.025012 | 4.534152 | 0.5413 |
1.3 | 2.948718 | 1.596141 | 1.025012 | 4.534152 | 0.5413 |
1.4 | 2.738095 | 1.482131 | 1.025012 | 4.534152 | 0.5413 |
1.5 | 2.555556 | 1.383322 | 1.025012 | 4.534152 | 0.5413 |
It can be noted that the mean and the standard deviation are decreasing when values of α and θ are increasing for fixed values of θ and α, respectively. The results of simulation emphasize that the coefficient of skewness, coefficient of variation and coefficient of kurtosis don't depend on θ and increasing when α values are increasing.
The moment generating function (MGF) of the WGQLD is given in the following theorem.
Theorem 2. : Let X∼fWGQLD(x,θ,α), then the MGF of X about the origin is:
M(t)=θ3(θ+αθ−tα)((2+α)θ−tα)(2+3α+α2)(θ−t)5. | (3.10) |
Proof. : To prove the MGF of the WGQLD, let
E(etx)=∞∫0etxθ32(α2+3α+2)(α2x2+αθx3+θ2x46)e−θxdx=θ32(α2+3α+2)(∞∫0α2x2e−(θ−t)xdx+∞∫0αθx3e−(θ−t)xdx+∞∫0θ2x46e−(θ−t)xdx)=θ32(α2+3α+2)(α22!(θ−t)3+αθ3!(θ−t)4+θ24!6(θ−t)5)=2θ32(α2+3α+2)(α2(θ−t)3+3αθ(θ−t)4+2θ2(θ−t)5)=θ3(α2+3α+2)(θ−t)3(α2+3αθ(θ−t)+2θ2(θ−t)2)=θ3(α2+3α+2)(θ−t)3(t2α2−2tθα2+θ2α2−3tαθ+3αθ2+2θ2(θ−t)2)=θ3(αθ−αt+θ)((α+2)θ−αt)(α2+3α+2)(θ−t)5. |
Let X1,X2,…,Xm be a random sample of size m from a distribution with pdf f(x) and cdf F(x). The pdf of the ith order statistic X(j:m) for j=1,2,…,m are defined by [14] as
f(j:m)(x)=m!(j−1)(m−j)F(x)]j[1−F(x)]m−jf(x). | (4.1) |
Based on Eq (4.1), the pdf of the ith order statistic, X(i:m), from the WGQLD, will be
f(j:m)(x)=θ3(C+1)j−122j−2m−13j−mΓ(m+1)e−θxAm−j(θ2x46+αθx3+α2x2)(α+1)(α+2)Γ(j)Γ(−j+m+1), | (4.2) |
where
A=e−θx(12(α+1)(α+2)+θ4x4+2(3α+2)θ3x3+6(α+1)(α+2)θ2x2+12(α+1)(α+2)θx)(α+1)(α+2), |
and
C=e−θx(−6α2(θx(θx+2)+2)−6α(θx(θx(θx+3)+6)+6)−θx(θx(θx(θx+4)+12)+24)−24)12(α+1)(α+2). |
Based on Eq (18), the pdf of the minimum and maximum order statistics, respectively, are given by
f(1:m)(x)=θ321−2m31−mme−θmx(θ2x46+αθx3+α2x2)[−(−6α2(θx(θx+2)+2)−B)]m−1(α2+3α+2)m, |
where B={6α(θx(θx(θx+3)+6)+6)+θx(θx(θx(θx+4)+12)+24)+24}, and
f(m:m)(x)=θ3me−θx(θ2x46+αθx3+α2x2)(e−θxD12(α+1)(α+2)+1)m−12(α2+3α+2), |
where
D=−6α2(θx(θx+2)+2)−6α(θx(θx(θx+3)+6)+6)−θx(θx(θx(θx+4)+12)+24)−24 |
.
To measure the scatter in the population, the mean deviation about the median denoted by MD(x) can be used, where
MD(x)=∫∞0|x−M|f(x)dx=μ−2∫M0xf(x)dx, | (4.3) |
where M is the population median. The mean deviation about the median for the WGQL distribution is defined as:
MD=(6α2(θM(θM(θM+3)+6)+6)−6(3α(α+4)+10)eθM+6α(θM(θM(θM(θM+4)+12)+24)+24)+θM(θM(θM(θM(θM+5)+20)+60)+120)+120)6(α+1)(α+2)θe−θM. | (4.4) |
Let X∼fWGQLD(x,θ,α), then
E(1X)=(α+1)2θ2(α2+3α+2). |
Therefore, the harmonic mean of the WGQLD is given by
HM(θ,α)=2(α2+3α+2)(α+1)2θ. | (4.5) |
Table 2 presents some values of the harmonic mean of WGQLD for various values of the parameters θ and α.
α | HM(5,α) | α | HM(5,α) | α | HM(5,α) | θ | HM(θ,2) | θ | HM(θ,2) | θ | HM(θ,2) |
1 | 0.6000 | 16 | 0.4235 | 31 | 0.4125 | 1 | 2.6666 | 16 | 0.1666 | 31 | 0.0860 |
2 | 0.5333 | 17 | 0.4222 | 32 | 0.4121 | 2 | 1.3333 | 17 | 0.1568 | 32 | 0.0833 |
3 | 0.5000 | 18 | 0.4210 | 33 | 0.4117 | 3 | 0.8888 | 18 | 0.1481 | 33 | 0.0808 |
4 | 0.4800 | 19 | 0.4200 | 34 | 0.4114 | 4 | 0.6666 | 19 | 0.1403 | 34 | 0.0784 |
5 | 0.4666 | 20 | 0.4190 | 35 | 0.4111 | 5 | 0.5333 | 20 | 0.1333 | 35 | 0.0761 |
6 | 0.4571 | 21 | 0.4181 | 36 | 0.4108 | 6 | 0.4444 | 21 | 0.1269 | 36 | 0.0740 |
7 | 0.4500 | 22 | 0.4173 | 37 | 0.4105 | 7 | 0.3809 | 22 | 0.1212 | 37 | 0.0720 |
8 | 0.4444 | 23 | 0.4166 | 38 | 0.4102 | 8 | 0.3333 | 23 | 0.1159 | 38 | 0.0701 |
9 | 0.4400 | 24 | 0.4160 | 39 | 0.4100 | 9 | 0.2962 | 24 | 0.1111 | 39 | 0.0683 |
10 | 0.4363 | 25 | 0.4153 | 40 | 0.4097 | 10 | 0.2666 | 25 | 0.1066 | 40 | 0.0666 |
11 | 0.4333 | 26 | 0.4148 | 41 | 0.4095 | 11 | 0.2424 | 26 | 0.1025 | 41 | 0.0650 |
12 | 0.4307 | 27 | 0.4142 | 42 | 0.4093 | 12 | 0.2222 | 27 | 0.0987 | 42 | 0.0634 |
13 | 0.4285 | 28 | 0.4137 | 43 | 0.4090 | 13 | 0.2051 | 28 | 0.0952 | 43 | 0.0620 |
14 | 0.4266 | 29 | 0.4133 | 44 | 0.4088 | 14 | 0.1904 | 29 | 0.0919 | 44 | 0.0606 |
15 | 0.4250 | 30 | 0.4129 | 45 | 0.4086 | 15 | 0.1777 | 30 | 0.0888 | 45 | 0.0592 |
Based on Table 2, we can conclude that: the harmonic mean values are decreasing in α when θ=5 and are decreasing in θ when α=2. Also, in general the harmonic mean values when θ<α are larger than the case of θ>α.
The stochastic ordering is an important tool in finance and reliability theory to evaluate the comparative behaviour of the models or systems. Let the random variables X and Y having the probability density functions, cumulative distribution functions and survival functions f(x),f(y),F(x),F(y),¯F(x)=1−F(x), and ¯F(y)=1−F(y), respectively, then X≤Y in:
1. Mean residual life order denoted by X≤MRLOY, if mX(x)≤mY(x) for all x,
2. Hazard rate order denoted by X≤HROY, if ¯FX(x)/¯FY(x) is decreasing in x≥0,
3. Stochastic order denoted by X≤SOY, if ¯FX(x)≤≤¯FY(x) for all x,
4. Likelihood ratio order denoted by X≤LROY, if fx(x)fY(x) is decreasing in x≥0.
All these stochastic orders defined above are related to each other and [29] showed the following relation is hold.
X≤LROY⇒X≤HROY⇒X≤MRLOY.⇓X≤SoY. |
Theorem 3. : Let the random variables X and Y be independent follow the pdf fX(x,θ,α) and fY(x,μ,ω), respectively. If θ>μ and α>ω, then X≤LROY,X≤HROY,X≤MRLOY and X≤SOY.
Proof: Let X∼fX(x,θ,α), Y∼fY(x,μ,ω), then
fX(x,θ,α)fY(x,μ,ω)=θ3(θ2x46+αθx3+α2x2)e−θx2(α2+3α+2)μ3(μ2x46+ωμx3+ω2x2)e−μx2(ω2+3ω+2), |
and
log(fX(x,θ,α)fY(x,μ,ω))=log(θ3(θ2x46+αθx3+α2x2)e−θx2(α2+3α+2)μ3(μ2x46+ωμx3+ω2x2)e−μx2(ω2+3ω+2)),=log(θ3(ω2+3ω+2)μ3(α2+3α+2)(θ2x46+αθx3+α2x2μ2x46+ωμx3+ω2x2)exp((μ−θ)x)),=log(θ3(ω2+3ω+2)μ3(α2+3α+2))+log(θ2x46+αθx3+α2x2μ2x46+ωμx3+ω2x2)+(μ−θ)x. |
Taking the derivative of the last equation with respect to x yields
ddxlog(fX(x,θ,α)fY(x,μ,ω))=2θ2x+6αθθ2x2+6αθx+6α2−2μ2x+6μωμ2x2+6μωx+6ω2+μ−θ. |
Hence, if θ>μ, α>ω, then ddxlog(fX(x,θ,α)fY(x,μ,ω))<0. Therefore, X≤LROY, X≤HROY, X≤MRLOY and X≤SOY.
The corresponding reliability and hazard functions of the WGQLD distribution are given, respectively by:
RWGQLD(x;θ, α)=1−FWGQLD(x;θ, α)=(24+6α2[2+xθ(2+xθ)]+6α[6+xθ(6+xθ(3+xθ))]+xθ[24+xθ(12+xθ(4+xθ))])12(1+α)(2+α)e−θx, | (5.1) |
and
HWGQLD(x;θ,α)=fWGQLD(x;θ,α)1−FWGQLD(x;θ,α)=θ3(θ2x46+αθx3+α2x2)24+6α2[2+xθ(2+xθ)]+6α[6+xθ(6+xθ(3+xθ))]+xθ[24+xθ(12+xθ(4+xθ))]. | (5.2) |
Figure 5, reveals that the reliability of the WGQLD are decreasing while the hazard rate function is increasing for α=1,2,…,5. The reversed hazard rate and odds functions for the WGQLD distribution, respectively, are defined as
RHWGQLD(x;θ,α)=fWGQLD(x;θ,α)FWGQLD(x;θ,α)=θ3x2(θ2x2+6αθx+6α2)12(α+1)(α+2)eθx−12(α+1)(α+2)−θx(θx(θx(θx+6α+4)+6(α+1)(α+2))+12(α+1)(α+2)), |
and
OWGQLD(x;θ,α)=FWGQLD(x;θ,α)1−FWGQLD(x;θ,α)=12(α+1)(α+2)eθxθ4x+4θ3x3+12θ2x2+24θx+6αθ3x3+18αθ2x2+36αθx+36α+6α2θ2x2+12α2θx+12α2+24−1. |
As it is shown in Figure 6, the reversed hazard function is decreasing taking inverse J shape while the odds functions is increasing with J shape.
Assume that the random variable X is a non-negative with continuous and twice differentiable cumulative distribution function F(x). The Bonferroni curve of the random variable X is defined as
BC=1pμ(∫∞0xf(x)dx−∫∞qxf(x)dx)=1pμ(μ−∫∞qxf(x)dx), | (6.1) |
where q=F−1(p) and p∈(0,1]. The Lorenz curve is defined as
LC=1μ(∫∞0xf(x)dx−∫∞qxf(x)dx)=1μ(μ−∫∞qxf(x)dx). | (6.2) |
The Gini index is given by
GI=1−1μ∫∞0(1−F(x))2dx=1μ∫∞0F(x)(1−F(x))dx. | (6.3) |
Now, for the WGQL distribution, the Bonferroni curve, Lorenz curve and Gini index are given, respectively, as
BC=(12eqθ(10+3α(4+α))−120−6α2(6+qθ[6+qθ(3+qθ)])−6α(24+qθ(24+qθ[12+qθ(4+qθ)]))−qθ(120+qθ[60+qθ(20+qθ(5+qθ))]))12p(10+3α(4+α))e−qθ, | (6.4) |
LC=(−120+12eqθ(10+3α(4+α))−6α2(6+qθ(6+qθ(3+qθ)))−6α(24+qθ(24+qθ(12+qθ(4+qθ))))−qθ(120+qθ(60+qθ(20+qθ(5+qθ)))))12(10+3α(4+α))e−qθ, | (6.5) |
GI=5(63+α[189+4α(49+3α(7+α))])64(1+α)(2+α)[10+3α(4+α)]. | (6.6) |
Table 3 presents some values of the Gini Index for WGQLD for different values of α
α | GI(α) | α | GI(α) | α | GI(α) |
1 | 0.2833333 | 16 | 0.3115732 | 31 | 0.3122193 |
2 | 0.2956578 | 17 | 0.3116666 | 32 | 0.3122354 |
3 | 0.3014769 | 18 | 0.3117464 | 33 | 0.3122502 |
4 | 0.3047121 | 19 | 0.3118154 | 34 | 0.3122637 |
5 | 0.3067016 | 20 | 0.3118753 | 35 | 0.3122762 |
6 | 0.3080137 | 21 | 0.3119276 | 36 | 0.3122877 |
7 | 0.3089251 | 22 | 0.3119736 | 37 | 0.3122984 |
8 | 0.3095841 | 23 | 0.3120143 | 38 | 0.3123082 |
9 | 0.3100762 | 24 | 0.3120505 | 39 | 0.3123174 |
10 | 0.3104533 | 25 | 0.3120828 | 40 | 0.3123259 |
11 | 0.3107487 | 26 | 0.3121117 | 41 | 0.3123339 |
12 | 0.3109844 | 27 | 0.3121377 | 42 | 0.3123413 |
13 | 0.3111755 | 28 | 0.3121611 | 43 | 0.3123482 |
14 | 0.3113326 | 29 | 0.3121824 | 44 | 0.3123547 |
15 | 0.3114633 | 30 | 0.3122017 | 45 | 0.3123607 |
Table 3 explains that the Gini index values for the WGQL distribution are increasing as α values are increasing, and on the average its value is about 0.31.
In this section, we consider six methods of estimation for estimating the unknowns parameters α and θ of the WGQLD distribution. These methods include the (1) maximum likelihood (ML) method, (2) method of maximum product of spacings, (3) ordinary least square method, (4) weight least square method, (5) method of Cramer-Von-Mises, and (6) method of Anderson-Darling.
First of all, we investigate the ML estimates (MLEs) of θ and α. Let X1,X2…,Xn be a random sample of size n selected from the WGQLD. The likelihood function is given by:
L(x;θ, α)=n∏i=1f(xi, θ, α)=(θ32(α2+3α+2))nn∏i=1 (α2x2i+αθx3i+θ2x4i6)e−θxi, | (7.1) |
and the log-likelihood function Ξ=lnL (x;θ, α) is:
Ξ=nln(θ32(α2+3α+2))+n∑i=1ln(α2x2i+αθx3i+θ2x4i6)−θn∑i=1xi. | (7.2) |
The derivatives of Ξ with respect to θ and α are:
dΞdθ=3nθ+n∑i=12xi(xiθ+3α)x2iθ2+6αxiθ+6α2−n∑i=1xi, | (7.3) |
dΞdα=−n(2α+3)α2+3α+2+n∑i=112α+6θxi6α2+6θxiα+θ2x2i. | (7.4) |
Since there is no closed form for these equations, then the MLEs ˆθ and ˆα of θ and α, respectively, can be solved simultaneously using a numerical method as Newton Raphson method.
The maximum product of spacing (MPS) method is proposed by [11,12] as an alternative to the maximum likelihood method. The MPS method requires a maximization of the geometric mean of the spacings in the data with respect to the parameters. Consider a random sample of size n, X1,X2,…,Xn from WGQLD distribution, then uniform spacings is given as:
Υi(θ,α)=F(xi:n|θ,α)−F(xi−1:n|θ,α),i=1,…,n, |
where F(x0:n|θ,α)=0 and F(xn+1:n|θ,α)=1. Clearly ∑n+1i=1Υi(θ,α)=1.
The MPSs, ˆαMPS and ˆθMPS, are the values of α and θ, which maximize the geometric mean of the spacing:
Z(θ,α|x)=[n+1∏i=1Υi(θ,α)]1n+1. | (7.5) |
The natural logarithm of (7.5) is:
H(θ,α|x)=1n+1n+1∑i=1logΥi(θ,α). |
The MPSs estimators ˆαMPS and ˆθMPS of the parameters α and θ, respectively, can also be obtained by solving the nonlinear equations:
∂∂θH(θ,α)=1n+1n+1∑i=11Υi(θ,α)[Δ1(xi:n|θ,α)−Δ1(xi−1:n|θ,α)]=0, |
∂∂αH(θ,α)=1n+1n+1∑i=11Υi(θ,α)[Δ2(xi:n|θ,α)−Δ2(xi−1:n|θ,α)]=0, |
where
Δ1(xi:n|θ,α)=∂∂θF(xi:n|θ,α)=θ3x3i:ne−θxi:n(6α2+(2θxi:n+8)α+3θxi:n)12(α+1)2(α+2)2, | (7.6) |
Δ2(xi:n|θ,α)=∂∂αF(xi:n|θ,α)=x3i:nθ2(x2i:nθ2+6αxi:nθ+6α2)e−xi:nθ12(α+1)(α+2), | (7.7) |
which can be obtained numerically.
The least square methods are introduced by [28] to estimate the parameters of beta distribution. Let Xi:n be the ith order statistic of the random sample X1,X2,…,Xn with distribution function F(x), then a main result in probability theory indicates that F(Xi:n)∼Beta(i,n−i+1). Moreover, we have
E[F(Xi:n)]=in+1 and Var[F(Xi:n)]=i(n−i+1)(n+1)2(n+2).
Using the expectations and variances, we obtain two variants of the least squares methods.
For the WGQLD distribution parameters estimation, the ordinary least square estimators ˆθOLS and ˆαOLS of the parameters θ and α, respectively can be obtained by minimizing the function:
Ω(θ,α|x)=n∑i=1[F(xi:n|θ,α)−in+1]2=n∑i=1[1−(24+6α2[2+xi:nθ(2+xi:nθ)]+6α[6+xi:nθ(6+xi:nθ(3+xi:nθ))]+xθ[24+xi:nθ(12+xi:nθ(4+xi:nθ))])12(1+α)(2+α)e−θxi:n−in+1]2, |
with respect to θ and α. Alternatively, these estimates can also be obtained by solving the following nonlinear equations:
n∑i=1[F(xi:n|θ,α)−in+1]Δ1(xi:n|θ,α)=0, |
n∑i=1[F(xi:n|θ,α)−in+1]Δ2(xi:n|θ,α)=0, |
where Δ1(xi:n|θ,α) and Δ2(xi:n|θ,α) are defined as in 7.6 and 7.7, respectively.
For the WGQLD distribution, the weighted least square estimators of θ and α say, ˆθWLS and ˆαWLS, respectively can be obtained by minimizing the function:
W(θ,α|x)=n∑i=1(n+1)2(n+2)i(n−i+1)[F(xi:n|θ,α)−in+1]2=n∑i=1(n+1)2(n+2)i(n−i+1)[1−(24+6α2[2+xi:nθ(2+xi:nθ)]+6α[6+xi:nθ(6+xi:nθ(3+xi:nθ))]+xθ[24+xi:nθ(12+xi:nθ(4+xi:nθ))])12(1+α)(2+α)e−θxi:n−in+1]2, |
with respect to θ and α. Equivalently, these estimators are the solution of the following nonlinear equations:
n∑i=1(n+1)2(n+2)i(n−i+1)[F(xi:n|θ,α)−in+1]Δ1(xi:n|θ,α)=0, |
n∑i=1(n+1)2(n+2)i(n−i+1)[F(xi:n|θ,α)−in+1]Δ2(xi:n|θ,α)=0, |
where Δ1(xi:n|θ,α) and Δ2(xi:n|θ,α) are specified as in 7.6 and 7.7, respectively.
Here, we use two popular methods based on the minimization of test statistics between the theoretical and empirical cumulative distribution functions. The methods are Cramer-von-Mises method and the method of Anderson-Darling (for more details see [13] and [24]).
The Cramer-von-Mises estimators (CVEs) ˆθ and ˆα of θ and α respectively, are obtained by minimizing the following function:
CV(θ,α)=112n+n∑i=1(n+1)2(n+2)i(n−i+1)[F(x(i:n);θ,α)−2i−12n]2=112n+n∑i=1(n+1)2(n+2)i(n−i+1)[1−(24+6α2[2+xi:nθ(2+xi:nθ)]+6α[6+xi:nθ(6+xi:nθ(3+xi:nθ))]+xθ[24+xi:nθ(12+xi:nθ(4+xi:nθ))])12(1+α)(2+α)e−θxi:n−2i−12n]2, |
with respect to θ and α. Equivalently, these estimators are the solution of the following nonlinear equations:
n∑i=1(n+1)2(n+2)i(n−i+1)[F(xi:n|θ,α)−2i−12n]Δ1(xi:n|θ,α)=0, |
n∑i=1(n+1)2(n+2)i(n−i+1)[F(xi:n|θ,α)−2i−12n]Δ2(xi:n|θ,α)=0, |
where Δ1(xi:n|θ,α) and Δ2(xi:n|θ,α) are given in 7.6 and 7.7, respectively.
The Anderson-Darling (AD) estimates of the WGQLD distribution parameters θ and α denoted by ˆθAD and ˆαAD can be obtained by minimizing the following function
A(α, θ)=−n−1nn∑i=1(2i−1){log F(xi:n|α, θ)+log¯F(xn−i+1:n|α, θ)}, |
with respect to θ and α, or by solving the following two equations
∂A(α,λ)∂λ=n∑i=1(2i−1){Δ1(xi:n|α,λ)F(xi:n|α,λ)−Δ1(xn−i+1:n|α,λ)¯F(xn−i+1:n|α,λ)}=0, |
and
∂A(α,λ)∂α=n∑i=1(2i−1){Δ2(xi:n|α,λ)F(xi:n|α,λ)−Δ2(xn−i+1:n|α,λ)¯F(xn−i+1:n|α,λ)}=0, |
where Δ1(xi:n|θ,α) and Δ2(xi:n|θ,α) are specified in 7.6 and 7.7, respectively.
This section compares the performances of the proposed estimators of the WGQLD parameters α and θ. This comparison is carried out by taking random samples of different sizes (n=20, 40, 60, 80,100 and 200) with various pairs of parameters values (θ,α) = (0.25, 1), (0.5, 1.5), (0.75, 2), (1, 1), (0.3, 2), (0.2, 3), (0.8, 1), (1, 3). The estimators are compared in terms of there mean square errors (MSE) and the estimated (Es) values of the parameters. The results are summarized in the Tables 4–7.
Parameters | MLEs | MPS | OLS | WLS | CVEs | AD | ||||||
Es | MSE | Es | MSE | Es | MSE | Es | MSE | Es | MSE | Es | MSE | |
α=0.25 | 0.426 | 0.3075 | 0.377 | 0.2919 | 0.486 | 0.4311 | 0.472 | 0.3890 | 0.456 | 0.3717 | 0.463 | 0.3680 |
θ=1 | 1.010 | 0.0118 | 1.026 | 0.0129 | 1.006 | 0.0134 | 1.005 | 0.0127 | 1.008 | 0.0127 | 1.005 | 0.0121 |
α=0.5 | 0.781 | 1.0029 | 0.718 | 1.0197 | 0.835 | 1.1394 | 0.822 | 1.0732 | 0.804 | 1.0553 | 0.811 | 1.0250 |
θ=1.5 | 1.523 | 0.0288 | 1.550 | 0.0325 | 1.516 | 0.0335 | 1.515 | 0.0319 | 1.521 | 0.0321 | 1.515 | 0.0304 |
α=0.75 | 1.133 | 1.8082 | 1.075 | 1.9053 | 1.196 | 2.0488 | 1.177 | 1.9076 | 1.157 | 1.8866 | 1.167 | 1.8744 |
θ=2 | 2.031 | 0.0562 | 2.068 | 0.0626 | 2.018 | 0.0639 | 2.017 | 0.0604 | 2.025 | 0.0607 | 2.017 | 0.0583 |
α=1 | 1.485 | 2.5509 | 1.429 | 2.6727 | 1.552 | 2.7457 | 1.530 | 2.6005 | 1.511 | 2.5964 | 1.514 | 2.528 |
θ=1 | 1.016 | 0.0140 | 1.036 | 0.0159 | 1.012 | 0.0165 | 1.010 | 0.0156 | 1.015 | 0.0156 | 1.010 | 0.0148 |
α=0.3 | 0.496 | 0.4830 | 0.441 | 0.4607 | 0.546 | 0.5756 | 0.534 | 0.5275 | 0.518 | 0.5111 | 0.527 | 0.5156 |
θ=2 | 2.021 | 0.0487 | 2.053 | 0.0538 | 2.010 | 0.0551 | 2.008 | 0.0527 | 2.015 | 0.0528 | 2.009 | 0.0509 |
α=0.2 | 0.412 | 0.3789 | 0.368 | 0.3773 | 0.464 | 0.5029 | 0.455 | 0.4562 | 0.440 | 0.4340 | 0.445 | 0.4371 |
θ=3 | 3.046 | 0.1080 | 3.094 | 0.1213 | 3.034 | 0.1251 | 3.033 | 0.1202 | 3.044 | 0.1202 | 3.033 | 0.1134 |
α=0.8 | 1.202 | 2.0044 | 1.148 | 2.1251 | 1.266 | 2.2173 | 1.248 | 2.0845 | 1.228 | 2.0673 | 1.237 | 2.0439 |
θ=1 | 1.014 | 0.0141 | 1.034 | 0.0161 | 1.009 | 0.0166 | 1.009 | 0.0157 | 1.013 | 0.0157 | 1.009 | 0.0151 |
α=1 | 1.480 | 2.6725 | 1.429 | 2.8627 | 1.546 | 2.9849 | 1.519 | 2.8085 | 1.502 | 2.7973 | 1.511 | 2.7333 |
θ=3 | 3.043 | 0.1308 | 3.103 | 0.1497 | 3.027 | 0.154 | 3.023 | 0.1462 | 3.036 | 0.1464 | 3.024 | 0.1392 |
Parameters | MLEs | MPS | OLS | WLS | CVEs | AD | ||||||
Es | MSE | Es | MSE | Es | MSE | Es | MSE | Es | MSE | Es | MSE | |
α=0.25 | 0.315 | 0.0771 | 0.283 | 0.0674 | 0.335 | 0.0949 | 0.331 | 0.0878 | 0.323 | 0.0849 | 0.329 | 0.0868 |
θ=1 | 1.001 | 0.0047 | 1.010 | 0.0049 | 0.999 | 0.0053 | 0.999 | 0.0050 | 1.001 | 0.0050 | 0.999 | 0.0049 |
α=0.5 | 0.576 | 0.1816 | 0.534 | 0.1741 | 0.604 | 0.2142 | 0.596 | 0.1981 | 0.586 | 0.1943 | 0.595 | 0.1965 |
θ=1.5 | 1.505 | 0.0113 | 1.520 | 0.0120 | 1.504 | 0.0130 | 1.503 | 0.0123 | 1.506 | 0.0123 | 1.503 | 0.0121 |
α=0.75 | 0.875 | 0.4170 | 0.829 | 0.4199 | 0.902 | 0.4771 | 0.894 | 0.4451 | 0.884 | 0.4409 | 0.892 | 0.4370 |
θ=2 | 2.012 | 0.0217 | 2.033 | 0.0231 | 2.007 | 0.0256 | 2.007 | 0.0238 | 2.011 | 0.0239 | 2.007 | 0.0234 |
α=1 | 1.145 | 0.5971 | 1.101 | 0.6059 | 1.174 | 0.6490 | 1.163 | 0.6149 | 1.153 | 0.6132 | 1.162 | 0.6064 |
θ=1 | 1.004 | 0.0051 | 1.014 | 0.0054 | 1.002 | 0.0058 | 1.002 | 0.0055 | 1.004 | 0.0055 | 1.002 | 0.0054 |
α=0.3 | 0.363 | 0.0849 | 0.328 | 0.0754 | 0.389 | 0.1047 | 0.383 | 0.0972 | 0.375 | 0.0941 | 0.381 | 0.0957 |
θ=2 | 2.007 | 0.0180 | 2.025 | 0.0192 | 2.003 | 0.0211 | 2.003 | 0.0198 | 2.007 | 0.0198 | 2.003 | 0.0195 |
α=0.2 | 0.281 | 0.0654 | 0.252 | 0.0560 | 0.306 | 0.0862 | 0.300 | 0.0780 | 0.293 | 0.0748 | 0.298 | 0.0777 |
θ=3 | 3.015 | 0.0415 | 3.040 | 0.0441 | 3.010 | 0.0479 | 3.010 | 0.0452 | 3.015 | 0.0453 | 3.009 | 0.0444 |
α=0.8 | 0.935 | 0.4789 | 0.891 | 0.4883 | 0.958 | 0.5383 | 0.953 | 0.5067 | 0.943 | 0.5026 | 0.950 | 0.5011 |
θ=1 | 1.005 | 0.0054 | 1.016 | 0.0058 | 1.003 | 0.0062 | 1.003 | 0.0059 | 1.005 | 0.0059 | 1.003 | 0.0058 |
α=1 | 1.157 | 0.7315 | 1.115 | 0.7467 | 1.187 | 0.8219 | 1.174 | 0.7597 | 1.164 | 0.7562 | 1.175 | 0.7566 |
θ=3 | 3.010 | 0.0528 | 3.042 | 0.0561 | 3.004 | 0.0615 | 3.003 | 0.0577 | 3.009 | 0.0578 | 3.003 | 0.0570 |
Parameters | MLEs | MPS | OLS | WLS | CVEs | AD | ||||||
Es | MSE | Es | MSE | Es | MSE | Es | MSE | Es | MSE | Es | MSE | |
α=0.25 | 0.287 | 0.0343 | 0.265 | 0.0312 | 0.3042 | 0.0433 | 0.298 | 0.0393 | 0.294 | 0.0383 | 0.299 | 0.0395 |
θ=1 | 1.003 | 0.0023 | 1.008 | 0.0024 | 1.003 | 0.0026 | 1.002 | 0.0025 | 1.003 | 0.0025 | 1.002 | 0.0024 |
α=0.5 | 0.540 | 0.0819 | 0.511 | 0.0797 | 0.551 | 0.0967 | 0.547 | 0.0892 | 0.542 | 0.0882 | 0.548 | 0.0892 |
θ=1.5 | 1.504 | 0.0057 | 1.513 | 0.0059 | 1.503 | 0.0066 | 1.503 | 0.0062 | 1.504 | 0.0062 | 1.503 | 0.0061 |
α=0.75 | 0.797 | 0.1301 | 0.768 | 0.1295 | 0.811 | 0.1509 | 0.806 | 0.1397 | 0.801 | 0.1387 | 0.807 | 0.1392 |
θ=2 | 2.006 | 0.0099 | 2.018 | 0.0104 | 2.003 | 0.0116 | 2.003 | 0.0107 | 2.005 | 0.0107 | 2.003 | 0.0106 |
α=1 | 1.096 | 0.2412 | 1.069 | 0.2411 | 1.107 | 0.2800 | 1.104 | 0.2593 | 1.098 | 0.2578 | 1.104 | 0.2577 |
θ=1 | 1.005 | 0.0028 | 1.011 | 0.0029 | 1.003 | 0.0033 | 1.004 | 0.0030 | 1.005 | 0.0031 | 1.003 | 0.0030 |
α=0.3 | 0.326 | 0.0369 | 0.302 | 0.0344 | 0.336 | 0.0442 | 0.333 | 0.0412 | 0.329 | 0.0404 | 0.334 | 0.0411 |
θ=2 | 2.002 | 0.0092 | 2.012 | 0.0094 | 1.999 | 0.0102 | 1.999 | 0.0096 | 2.001 | 0.0096 | 1.999 | 0.0096 |
α=0.2 | 0.240 | 0.0262 | 0.220 | 0.0228 | 0.252 | 0.0320 | 0.248 | 0.0297 | 0.244 | 0.0288 | 0.249 | 0.0296 |
θ=3 | 3.009 | 0.0194 | 3.024 | 0.0201 | 3.005 | 0.0220 | 3.005 | 0.0207 | 3.008 | 0.0207 | 3.005 | 0.0205 |
α=0.8 | 0.846 | 0.1625 | 0.818 | 0.1621 | 0.854 | 0.1858 | 0.852 | 0.1748 | 0.847 | 0.1739 | 0.852 | 0.1725 |
θ=1 | 1.000 | 0.0027 | 1.007 | 0.0028 | 0.999 | 0.0032 | 0.999 | 0.0030 | 1.000 | 0.0030 | 0.999 | 0.0029 |
α=1 | 1.071 | 0.2538 | 1.044 | 0.2564 | 1.087 | 0.2868 | 1.080 | 0.2664 | 1.074 | 0.2651 | 1.081 | 0.2669 |
θ=3 | 3.006 | 0.0248 | 3.025 | 0.0258 | 3.003 | 0.0298 | 3.002 | 0.0274 | 3.006 | 0.0274 | 3.002 | 0.0273 |
Parameters | MLEs | MPS | OLS | WLS | CVEs | AD | ||||||
Es | MSE | Es | MSE | Es | MSE | Es | MSE | Es | MSE | Es | MSE | |
α=0.25 | 0.263 | 0.0162 | 0.249 | 0.0154 | 0.271 | 0.0204 | 0.268 | 0.0185 | 0.266 | 0.0183 | 0.269 | 0.0185 |
θ=1 | 1.000 | 0.0011 | 1.003 | 0.0011 | 1.000 | 0.0013 | 1.000 | 0.0012 | 1.001 | 0.0012 | 1.000 | 0.0012 |
α=0.5 | 0.512 | 0.0364 | 0.495 | 0.0362 | 0.519 | 0.0430 | 0.517 | 0.0397 | 0.514 | 0.0395 | 0.517 | 0.0397 |
θ=1.5 | 1.501 | 0.0028 | 1.506 | 0.0028 | 1.500 | 0.0032 | 1.500 | 0.0030 | 1.501 | 0.0030 | 1.500 | 0.0030 |
α=0.75 | 0.762 | 0.0563 | 0.745 | 0.0564 | 0.770 | 0.0637 | 0.767 | 0.0594 | 0.764 | 0.0592 | 0.767 | 0.0592 |
θ=2 | 2.000 | 0.0048 | 2.007 | 0.0049 | 1.999 | 0.0054 | 1.999 | 0.00511 | 2.000 | 0.0051 | 1.998 | 0.0050 |
α=1 | 1.026 | 0.0976 | 1.011 | 0.0979 | 1.034 | 0.1101 | 1.031 | 0.1031 | 1.028 | 0.1028 | 1.031 | 0.1033 |
θ=1 | 1.000 | 0.0013 | 1.004 | 0.0013 | 0.999 | 0.0015 | 1.000 | 0.0014 | 1.000 | 0.0014 | 1.000 | 0.0014 |
α=0.3 | 0.307 | 0.0191 | 0.291 | 0.0186 | 0.315 | 0.0229 | 0.313 | 0.0212 | 0.310 | 0.0210 | 0.313 | 0.0212 |
θ=2 | 2.000 | 0.0046 | 2.007 | 0.0047 | 1.999 | 0.0051 | 1.999 | 0.0048 | 2.000 | 0.0048 | 1.999 | 0.0048 |
α=0.2 | 0.215 | 0.0125 | 0.202 | 0.0114 | 0.222 | 0.0149 | 0.220 | 0.0138 | 0.217 | 0.0136 | 0.220 | 0.0138 |
θ=3 | 2.999 | 0.0100 | 3.008 | 0.0102 | 2.998 | 0.0113 | 2.998 | 0.0107 | 2.999 | 0.0107 | 2.998 | 0.0106 |
α=0.8 | 0.837 | 0.0749 | 0.821 | 0.0744 | 0.842 | 0.0846 | 0.841 | 0.0793 | 0.838 | 0.0790 | 0.842 | 0.0792 |
θ=1 | 1.002 | 0.0013 | 1.006 | 0.0014 | 1.001 | 0.0015 | 1.002 | 0.0014 | 1.002 | 0.0014 | 1.002 | 0.0014 |
α=1 | 1.030 | 0.0935 | 1.015 | 0.0935 | 1.040 | 0.1044 | 1.036 | 0.0978 | 1.033 | 0.0975 | 1.036 | 0.0976 |
θ=3 | 3.003 | 0.0116 | 3.014 | 0.0119 | 3.002 | 0.0133 | 3.002 | 0.0125 | 3.004 | 0.0125 | 3.001 | 0.0124 |
Based on Tables 4–7 it is clear that:
● The MSEs values are decreasing as the sample sizes values are increasing for all cases considered in this section. As an example, for α=1,θ=3, with n=50 based on the AD, the MSEs are 0.7566 and 0.0570 compared to 0.2669 and 0.0273 for n=100, respectively.
● The bias values of the suggested estimators are decreasing as the sample sizes are increasing, and approaches zero for all cases for large n. For illustration, for α=0.3,θ=2, with n=100 the Es values are 0.302 and 2.012 using the MPS as compared to 0.291 and 2.007 for n=200, respectively.
● It can be observed that for most of the cases, the MLEs method has the smallest values of the MSEs among all methods of estimation.
In this section, we use data sets to illustrate the usefulness of the WGQL model, where four different data sets are used related to the environment, engineering and two medical data sets are considered. We compare the WGQL distribution to some well known distributions of two parameters as the generalized Quasi Lindley distribution, Quasi Lindley distribution, two-parameter Sujatha distribution, and the Pareto distribution.
The first data set is taken from [22] represents the 100 annual maximum precipitation (inches) for one rain gauge in Fort Collins, Colorado, from 1900 through 1999. The data are given below:
Data Set 1: 239,232,434, 85,302,174,170,121,193,168,148,116,132,132,144,183,223, 96,298, 97,116,146, 84,230,138,170,117,115,132,125,156,124,189,193, 71,176,105, 93,354, 60,151,160,219,142,117, 87,223,215,108,354,213,306,169,184, 71, 98, 96,218,176,121,161,321,102,269, 98,271, 95,212,151,136,240,162, 71,110,285,215,103,443,185,199,115,134,297,187,203,146, 94,129,162,112,348, 95,249,103,181,152,135,463,183,241 5.
The second data set from [25] consists of 100 observations on breaking stress of carbon fibers (in Gba). The data are as follows:
Data Set 2: 3.7, 2.74, 2.73, 2.5, 3.6, 3.11, 3.27, 2.87, 1.47, 3.11, 4.42, 2.41, 3.19, 3.22, 1.69, 3.28, 3.09, 1.87, 3.15, 4.9, 3.75, 2.43, 2.95, 2.97, 3.39, 2.96, 2.53, 2.67, 2.93, 3.22, 3.39, 2.81, 4.2, 3.33, 2.55, 3.31, 3.31, 2.85, 2.56, 3.56, 3.15, 2.35, 2.55, 2.59, 2.38, 2.81, 2.77, 2.17, 2.83, 1.92, 1.41, 3.68, 2.97, 1.36, 0.98, 2.76, 4.91, 3.68, 1.84, 1.59, 3.19, 1.57, 0.81, 5.56, 1.73, 1.59, 2, 1.22, 1.12, 1.71, 2.17, 1.17, 5.08, 2.48, 1.18, 3.51, 2.17, 1.69, 1.25, 4.38, 1.84, 0.39, 3.68, 2.48, 0.85, 1.61, 2.79, 4.7, 2.03, 1.8, 1.57, 1.08, 2.03, 1.61, 2.12, 1.89, 2.88, 2.82, 2.05, 3.655.
Due to the importance of the studies about the Covid-19 in the last years, we considered two sets of Covid-19 related to Algeria and Saudi Arabia in various times. The third data set is the Covid-19 data for the daily new cases in Algeria from 12 August 2020 to 09 November 2020 and it is available on the following electronic address https://sehhty.com/dz-covid/. It is given as follows
Data Set 3: 642,670,581,631,642,548,405,302,330,291,319,306,320,287,276,263,250,273,276,252,213,214,199,205,221,193,185,174,153,132,136,146,138,121,129,134,141,148,157,160,162,155,146,153,160,175,179,186,191,197,203,210,219,228,232,238,242,247,255,264,272,278,285,289,293,298,304,311,325,339,348,365,378,387,397,391,370,398,392,401,409,411,403,419,442,450,469,477,488,495.
The box and TTT plots for the above data are given in Figures 7 and 8, respectively.
The fourth data set is calling the Covid-19 data which present the daily new cases in Saudi Arabia from 24 March 2020 to 24 April 2020 and it is given by
Data Set 4: 1, 1, 1, 0, 1, 4, 0, 2, 6, 5, 4, 4, 5, 4, 3, 0, 3, 3, 5, 7, 6, 8, 6, 4, 4, 5, 5, 6, 6, 5, 7, 6. The descriptive statistics for the data is given in Table 8.
n | Min | Max | Mean | Median | St derivation | Kurtosis | Skweness | |
Data Set 1: | 100 | 60.000 | 463.000 | 175.670 | 158.000 | 83.166 | 1.713 | 1.316 |
Data Set 2: | 100 | 0.390 | 5.560 | 2.621 | 2.700 | 1.013 | 0.043 | 0.362 |
Data Set 3: | 90 | 121.000 | 670.000 | 294.322 | 274.500 | 131.618 | 0.369 | 0.927 |
Data Set 4: | 32 | 0 | 8 | 3.97 | 4 | 2.24 | -0.98 | -3.35 |
The WGQLD distribution is fitted to these two real data sets and compared with the following models:
● The generalized Quasi Lindley distribution: f(x)=θ2(θ2x36+αθx2+α2x)e−θx(α+1)2.
● Quasi Lindley distribution: f(x)=θ(α+xθ)α+1e−θx.
● The Pareto distribution: f(x)=αθαxα+1.
● Two-parameter Sujatha distribution: f(x)=θ3(x2+αx+1)e−θxθ2+αθ+2.
To choose the best model fitting, we considered Akaike information criterion (AIC) introduced by [1], Baysian information criterion (BIC) proposed by [30], Hannan Quinn Information Criterion (HQIC) suggested by [21], Consistent Akaike Information Criterion (CAIC) by [10], Kolmogorov-Smirnov (KS), where AIC = −2L+k,, CAIC = −2L+22knn−k−1, HQIC = 2loglog(n)[k−2L], BIC = −2L+klog(n), KS = sup|Fn(x)−F(x)|,Fn(x)=1n∑niłxi≤x, where k is the number of parameters and n is the sample size and L is the value of maximum log-likelihood function.
Based on the results reported in Tables 9, 10, 11 and 12, we observe that the WGQLD provides the better fit with the smallest values of AIC, AICc, BIC, HQIC and K-S with maximum P-values as compared to its competitive models considered in this study. Figures 9, 10, 11 and 12 support this claim.
Model | AIC | CAIC | BIC | HQIC | K-S | p-value |
WGQLD | 1142.145 | 1142.268 | 1147.355 | 1144.253 | 0.059902 | 0.865573 |
GQLD | 1145.844 | 1145.968 | 1151.055 | 1147.953 | 0.095349 | 0.323229 |
QLD | 1180.179 | 1180.303 | 1185.389 | 1182.288 | 0.216170 | 0.000174 |
PD | 1237.721 | 1237.845 | 1242.932 | 1239.83 | 0.340971 | 1.59e-10 |
TSPD | 1156.301 | 1156.425 | 1161.511 | 1158.41 | 0.143704 | 0.032159 |
Model | AIC | AICc | BIC | HQIC | K-S | p-value |
WGQLD | 295.1091 | 295.2328 | 300.3194 | 297.2178 | 0.105898 | 0.212049 |
GQLD | 306.1634 | 306.2871 | 311.3737 | 308.2721 | 0.123234 | 0.095915 |
QLD | 346.108 | 346.2317 | 351.3183 | 348.2167 | 0.223871 | 8.86e-05 |
PD | 396.7418 | 396.8655 | 401.9522 | 398.8505 | 0.320272 | 2.46-09 |
TSPD | 350.3233 | 350.447 | 355.5336 | 352.432 | 0.220935 | 0.000115 |
Model | AIC | AICc | BIC | HQIC | K-S | p-value |
WGQLD | 1118.42 | 1118.558 | 1123.420 | 1120.436 | 0.0606885 | 0.8946827 |
GQLD | 1122.41 | 1122.548 | 1127.410 | 1124.426 | 0.0900125 | 0.4593636 |
QLD | 1154.697 | 1154.835 | 1159.696 | 1156.713 | 0.2081433 | 0.0008209 |
PD | 1207.242 | 1207.38 | 1212.241 | 1209.258 | 0.3435296 | 1.190e-09 |
TSPD | 1132.559 | 1132.697 | 1137.559 | 1134.575 | 0.1352032 | 0.0744752 |
Model | AIC | AICc | BIC | HQIC | K-S | p-value |
WGQLD | 161.0522 | 161.4966 | 163.8546 | 161.9487 | 0.3077698 | 0.006804395 |
GQLD | 166.9975 | 167.4419 | 169.7999 | 167.894 | 0.3318577 | 0.00269967 |
In this article, we proposed the WGQLD distribution along with some of its properties such as, stochastic ordering, Median deviation, Harmonic mean, some plots of the pdf and cdf, Bonferroni and Lorenz curves and Gini index moments, coefficient of variation, coefficient of skewness and coefficient of kurtosis. Also, the hazard rate function, reliability function, reversed hazard rate and odds functions are presented. The maximum likelihood estimates is computed as well as the maximum product of spacing's, ordinary least squares, weighted least squares, Cramer-von-Mises, and Anderson-Darling methods are obtained. The results show that the best method of estimation is the MLE method. Applications of various real data sets are analyzed for illustration. It is proved that the WGQLD is empirically better than other competitors models considered in this study including the base GQLD. Therefore, in the future, the authors intend to investigate the performance of different estimators of the WGQLD based on ranked set sampling method and its modifications, see [3,4,19,20,32].
The author declare that they have no conflict of interest
The authors extend their appreciation to the deputy ship for research and innovation, "Ministry of Education" in Saudi Arabia for funding this research work through project No. IFKSURG-1438-086.
[1] |
D. Xin, Q. Han, A molecular dynamics investigation on the compression of cross-linked epoxy resins, Mol. Simul., 41 (2015), 1509–1514. https://doi.org/10.1080/08927022.2014.994623 doi: 10.1080/08927022.2014.994623
![]() |
[2] | L. Shen, L. Zou, M. Ding, T. Zhao, L. Zhang, Q. Li, Molecular dynamics simulation on dielectric constant and thermal conductivity of crosslink epoxy/functionalized graphene nano-composites, in IOP Conference Series: Materials Science and Engineering, 761 (2020), 012009. https://doi.org/10.1088/1757-899X/761/1/012009 |
[3] |
W. Sun, S. Yu, S. Gao, X. Yao, H. Xu, B. Qian, et al., Molecular dynamics simulation of water molecule diffusion in graphene-reinforced epoxy resin anticorrosive coatings, J. Chin. Soc. Corros. Prot., 41 (2021), 411–416. https://doi.org/10.11902/1005.4537.2020.227 doi: 10.11902/1005.4537.2020.227
![]() |
[4] |
Y. Li, S. Wang, Q. Wang, A molecular dynamics simulation study on enhancement of mechanical and tribological properties of polymer composites by introduction of graphene, Carbon, 111 (2017), 538–545. http://doi.org/10.1016/j.carbon.2016.10.039 doi: 10.1016/j.carbon.2016.10.039
![]() |
[5] |
Q. Xie, K. Fu, S. Liang, B. Liu, L. Lu, X. Yang, et al., Micro-structure and thermomechanical pproperties of crosslinked epoxy composite modified by nano-SiO2: A molecular dynamics simulation, Polymers (Basel), 10 (2018), 801. https://doi.org/10.3390/polym10070801 doi: 10.3390/polym10070801
![]() |
[6] |
Y. Yang, G. Xian, H. Li, L. Sui, Thermal aging of an anhydride-cured epoxy resin, Polym. Degrad. Stab., 118 (2015), 111–119. https://doi.org/10.1016/j.polymdegradstab.2015.04.017 doi: 10.1016/j.polymdegradstab.2015.04.017
![]() |
[7] | D. Li, Evolution of the Properties of an Epoxy Resin Submitted to Water and Alkaline Immersion and the molecular Dynamic Simulation, Master's thesis, Harbin Institute of Technology in Harbin, 2015. |
[8] |
Y. Xiao, G. Xian, Effects of moisture ingress on the bond between carbon fiber and epoxy resin investigated with molecular dynamics simulation, Polym. Compos., 39 (2018), E2074–E2083. https://doi.org/10.1002/pc.24459 doi: 10.1002/pc.24459
![]() |
[9] |
F. Zhao, H. Ba, X. Gao, The durability of epoxy resin coating, J. Wuhan Univ. Technol. Mater. Sci. Ed., 23 (2008), 242–244. https://doi.org/10.1007/s11595-006-2242-z doi: 10.1007/s11595-006-2242-z
![]() |
[10] |
J. Arias, M. M. Escobar, C. Bernal, A. Vázquez, Aging in water and in an alkaline medium of unsaturated polyester and epoxy resins: experimental study and modeling, Adv. Polym. Technol., 37 (2018), 450–460. https://doi.org/10.1002/adv.21684 doi: 10.1002/adv.21684
![]() |
[11] |
A. Uthaman, G. Xian, S. Thomas, Y. Wang, Q. Zheng, X. Liu, Durability of an epoxy resin and its carbon fiber-reinforced polymer composite upon immersion in water, acidic, and alkaline solutions, Polymers (Basel), 12 (2020), 614. https://doi.org/10.3390/polym12030614 doi: 10.3390/polym12030614
![]() |
[12] |
B. Wang, D. Li, G. Xian, C. Li, Effect of Immersion in water or alkali solution on the structures and properties of epoxy resin, Polymers (Basel), 13 (2021), 1902. https://doi.org/10.3390/polym13121902 doi: 10.3390/polym13121902
![]() |
[13] |
F. Jeyranpour, G. Alahyarizadeh, H. Minuchehr, The thermo-mechanical properties estimation of fullerene-reinforced resin epoxy composites by molecular dynamics simulation-A comparative study, Polymer, 88 (2016), 9–18. https://doi.org/10.1016/j.polymer.2016.02.018 doi: 10.1016/j.polymer.2016.02.018
![]() |
[14] |
Y. Zhang, J. Ma, C. Wu, X. Han, W. Zhang, Effects of moisture ingress on the mesoscale mechanical properties of epoxy adhesives under elevated temperature, Polym. Test., 94 (2021), 107049. https://doi.org/10.1016/j.polymertesting.2020.107049 doi: 10.1016/j.polymertesting.2020.107049
![]() |
[15] |
H. Chai, X. Wang, W. U. Rehman, X. Yang, T. Meng, Study on water absorption and mechanical properties of CNF-Ti reinforced epoxy resin composites, Plast., Rubber Compos., 52 (2021), 47–58. https://doi.org/10.1080/14658011.2021.2017127 doi: 10.1080/14658011.2021.2017127
![]() |
[16] |
P. Penjumras, R. A. Rahman, R. A. Talib, K. Abdan, Mechanical properties and water absorption behaviour of durian rind cellulose reinforced poly (lactic acid) biocomposites, Int. J. Adv. Sci. Eng. Inf. Technol., 5 (2015), 343–349. https://doi.org/10.18517/ijaseit.5.5.574 doi: 10.18517/ijaseit.5.5.574
![]() |
[17] |
A. King, G. Johnson, D. Engelberg, W. Ludwig, L. Marrow, Observations of intergranular stress corrosion cracking in a grain-mapped polycrystal, Science, 321 (2008), 382–385. https://doi.org/10.1126/science.abq1274 doi: 10.1126/science.abq1274
![]() |
[18] |
X. Sun, H. Sun, H. Li, H. Peng, Developing polymer composite materials: carbon nanotubes or graphene?, Adv. Mater., 25 (2013), 5153–5176. https://doi.org/10.1002/adma.201301926 doi: 10.1002/adma.201301926
![]() |
[19] |
L. C. O. Silva, G. G. Silva, P. M. Ajayan, B. G. Soares, Long-term behavior of epoxy/graphene-based composites determined by dynamic mechanical analysis, J. Mater. Sci., 50 (2015), 6407–6419. https://doi.org/10.1007/s10853-015-9193-8 doi: 10.1007/s10853-015-9193-8
![]() |
[20] |
W. Hou, Y. Gao, J. Wang, D. J. Blackwood, S. Teo, Recent advances and future perspectives for graphene oxide reinforced epoxy resins, Mater. Today Commun., 23 (2020), 100883. https://doi.org/10.1016/j.mtcomm.2019.100883 doi: 10.1016/j.mtcomm.2019.100883
![]() |
[21] |
Y. Ni, L. Chen, K. Teng, J. Shi, X. Qian, Z. Xu, et al., Superior mechanical properties of epoxy composites reinforced by 3D interconnected graphene skeleton, ACS Appl. Mater. Interfaces, 7 (2015), 11583–11591. https://doi.org/10.1021/acsami.5b02552 doi: 10.1021/acsami.5b02552
![]() |
[22] |
T. Hu, D. Zhao, C. Cheng, S. Meng, Z. Ding, L. Wu, Preparation and mechanical properties of graphene oxide reinforced epoxy composites, J. Beijing Univ. Chem. Technol. (Nat. Sci. Ed.), 45 (2018), 29–33. https://doi.org/10.13543/j.bhxbzr.2018.06.005 doi: 10.13543/j.bhxbzr.2018.06.005
![]() |
[23] |
W. Xiao, Y. Liu, S. Guo, Composites of graphene oxide and epoxy resin assuming a uniform 3D graphene oxide network structure, RSC Adv., 6 (2016), 8694–8698. https://doi.org/10.1039/c6ra16335a doi: 10.1039/c6ra16335a
![]() |
[24] |
H. Yao, S. A. Hawkins, H. Sue, Preparation of epoxy nanocomposites containing well-dispersed graphene nanosheets. Compos. Sci. Technol., 146 (2017), 161–168. https://doi.org/10.1016/j.compscitech.2017.04.026 doi: 10.1016/j.compscitech.2017.04.026
![]() |
[25] |
S. A. Bansal, A. P. Singh, A. Kumar, S. Kumar, N. Kumar, J. K. Goswamy, Improved mechanical performance of bisphenol-A graphene-oxide nano-composites, J. Compos. Mater., 52 (2018), 2179–2188. https://doi.org/10.1177/0021998317741952 doi: 10.1177/0021998317741952
![]() |
[26] |
B. Hu, Y. Cong, B. Zhang, L. Zhang, Y. Shen, H. Huang, Enhancement of thermal and mechanical performances of epoxy nanocomposite materials based on graphene oxide grafted by liquid crystalline monomer with Schiff base, J. Mater. Sci., 55 (2020), 3712–3727. https://doi.org/10.1007/s10853-019-04273-2 doi: 10.1007/s10853-019-04273-2
![]() |
[27] |
Y. Zhang, D. Zhang, X. Wei, S. Zhong, J. Wang, Enhanced tribological properties of polymer composite coating containing graphene at room and elevated temperatures, Coatings, 8 (2018), 91. https://doi.org/10.3390/coatings8030091 doi: 10.3390/coatings8030091
![]() |
[28] |
A. Wolk, M. Rosenthal, J. Weiss, M. Voigt, J. Wesendahl, M. Hartmann, et al., Graphene oxide as flexibilizer for epoxy amine resins, Prog. Org. Coat., 122 (2018), 280–289. https://doi.org/10.1016/j.porgcoat.2018.05.028 doi: 10.1016/j.porgcoat.2018.05.028
![]() |
[29] |
S. C. Her, L. Y. Chen, Fabrication and characterization of graphene/epoxy nanocomposites, Mater. Sci., 25 (2019), 433–440. https://doi.org/10.5755/j01.ms.25.4.19462 doi: 10.5755/j01.ms.25.4.19462
![]() |
[30] |
P. Bian, W. Verestek, S. Yan, X. Xu, H. Qing, S. Schmauder, A multiscale modeling on fracture and strength of graphene platelets reinforced epoxy, Eng. Fract. Mech., (2020), 107197. https://doi.org/10.1016/j.engfracmech.2020.107197 doi: 10.1016/j.engfracmech.2020.107197
![]() |
[31] |
P. Bian, S. Schmauder, H. Qing, Strength and damage of nanoplatelets reinforced polymer: A 3D finite element modeling and simulation, Compos. Struct., 245 (2020), 112337. https://doi.org/10.1016/j.compstruct.2020.112337 doi: 10.1016/j.compstruct.2020.112337
![]() |
[32] |
A. Yadav, A. Kumar, P. K. Singh, K. Sharma, Glass transition temperature of functionalized graphene epoxy composites using molecular dynamics simulation, Integr. Ferroelectr., 186 (2018), 106–114. https://doi.org/10.1080/10584587.2017.1370331 doi: 10.1080/10584587.2017.1370331
![]() |
[33] |
A. Salehi, S. Rash-Ahmadi, Effect of adsorption, hardener, and temperature on mechanical properties of epoxy nanocomposites with functionalized graphene: A molecular dynamics study, J. Mol. Graphics Modell., 117 (2022), 108311. https://doi.org/10.1016/j.jmgm.2022.108311 doi: 10.1016/j.jmgm.2022.108311
![]() |
[34] |
O. Starkova, S. Gaidukovs, O. Platnieks, A. Barkane, K. Garkusina, E. Palitis, et al., Water absorption and hydrothermal ageing of epoxy adhesives reinforced with amino-functionalized graphene oxide nanoparticles. Polym. Degrad. Stab., 191 (2021), 109670. https://doi.org/10.1016/j.polymdegradstab.2021.109670 doi: 10.1016/j.polymdegradstab.2021.109670
![]() |
[35] |
S. Prolongo, A. Jiménez-Suárez, R. Moriche, A. Ureña, Influence of thickness and lateral size of graphene nanoplatelets on water uptake in epoxy/graphene nanocomposites, Appl. Sci., 8 (2018), 1550. https://doi.org/10.3390/app8091550 doi: 10.3390/app8091550
![]() |
[36] |
W. Li, L. Zhang, M. Zhang, S. Chen, Structures of graphene-reinforced epoxy coatings and the dynamic diffusion of guest water: a molecular dynamics study, Ind. Eng. Chem. Res., 59 (2020), 20749–20756. https://doi.org/10.1021/acs.iecr.0c04673 doi: 10.1021/acs.iecr.0c04673
![]() |
[37] |
L. Zhu, C. Feng, Y. Cao, Corrosion behavior of epoxy composite coatings reinforced with reduced graphene oxide nanosheets in the high salinity environments, Appl. Surf. Sci., 493 (2019), 889–896. https://doi.org/10.1016/j.apsusc.2019.06.271 doi: 10.1016/j.apsusc.2019.06.271
![]() |
[38] |
L. Yao, J. Zhao, Mechanism study of doped graphene on improving mechanical properties and corrosion resistance of epoxy resin, J. At. Mol. Phys., 38 (2021), 131–135. https://doi.org/10.19855/j.1000-0364.2021.016003 doi: 10.19855/j.1000-0364.2021.016003
![]() |
[39] |
P. Bian, H. Qing, A phase-field based finite element method for modeling graphene flake reinforced composites, Mech. Adv. Mater. Struct., (2022), 1–16. https://doi.org/10.1080/15376494.2022.2048146 doi: 10.1080/15376494.2022.2048146
![]() |
[40] |
P. L. Bian, H. Qing, S. Schmauder, A novel phase-field based cohesive zone model for modeling interfacial failure in composites, Int. J. Numer. Methods Eng., (2021), 1–24. https://doi.org/10.1002/nme.6821 doi: 10.1002/nme.6821
![]() |
[41] |
W. Li, W. Chai, L. Zhang, Y. Guo, W. Wang, S. Chen, Atomic insight into the influences of moisture ingress on the structures and dynamics of graphene-epoxy interfaces, Compos. Sci. Technol., 219 (2022), 109222. https://doi.org/10.1016/j.compscitech.2021.109222 doi: 10.1016/j.compscitech.2021.109222
![]() |
[42] |
C. Sheng, G. Wu, X. Sun, S. Liu, Molecular dynamics investigation of the thermo-mechanical properties of the moisture invaded and cross-linked epoxy system, Polymers (Basel), 14 (2022), 103, https://doi.org/10.3390/polym14010103 doi: 10.3390/polym14010103
![]() |
[43] |
J. Fan, P. Li, Z. Wang, J. Yang, The temperature-dependent properties of epoxy-functionalized graphene oxide/epoxy nanocomposites: insights from simulation and experiment, J. Mater. Sci., 57 (2022), 15298–15313, https://doi.org/10.1007/s10853-022-07575-0 doi: 10.1007/s10853-022-07575-0
![]() |
[44] |
K. Fu, Q. Xie, F. LÜ, Q. Duan, X. Wang, Q. Zhu, et al., Molecular dynamics simulation and experimental studies on the thermomechanical properties of epoxy resin with different anhydride curing agents, Polymers (Basel), 11 (2019), 975, https://doi:10.3390/polym11060975 doi: 10.3390/polym11060975
![]() |
[45] |
B. S. Sindu, S. Sasmal, Evaluation of mechanical characteristics of nano modified epoxy based polymers using molecular dynamics, Comput. Mater. Sci., 96 (2015), 146–158, http://dx.doi.org/10.1016/j.commatsci.2014.09.003 doi: 10.1016/j.commatsci.2014.09.003
![]() |
[46] |
Q. Yang, X. Yang, X. Li, L. Shi, G. Sui, The curing and thermal transition behavior of epoxy resin: a molecular simulation and experimental study, RSC Adv., 3 (2013), 7452–7459. https://doi.org/10.1039/c3ra40699g doi: 10.1039/c3ra40699g
![]() |
[47] |
C. Sheng, G. Wu, X. Sun, S. Liu, Molecular dynamics investigation of the thermo-mechanical properties of the moisture invaded and cross-linked epoxy system, Polymers (Basel), 14 (2022), 103. https://doi.org/10.3390/polym14010103 doi: 10.3390/polym14010103
![]() |
[48] |
S. Ma, P. Chen, J. Xu, X. Xiong, Molecular dynamics simulations of key physical properties and microstructure of epoxy resin cured with different curing agents, J. Mater. Sci., 57 (2022), 1123–1133. https://doi.org/10.1007/s10853-021-06799-w doi: 10.1007/s10853-021-06799-w
![]() |
[49] | Y. Jin, X. Han, P. Hu, Modelling and Experimental Study on Diffusion Characteristics of Epoxy Adhesive Immersed in Aqueous Environment, The 26th Annual Conference of Beijing Adhesion Society, Beijing, 2017. |
[50] | K. Li, Molecular Dynamic Simulations of Crosslinked Epoxy Resin and Properties of Nano-Composites Containing Phenyl POSS, Ph.D thesis, Beijing University of Chemical Technology, Beijing, 2017. |
[51] | Y. Liu, Research on the Aging Properties of Epoxy Resin in Hygrothermal Environment, Master's thesis, Chongqing University in Chongqing, 2018 |
[52] |
R. Cai, J. Zhao, N. Lv, A. Fu, C. Yin, C. Song, et al., Curing and molecular dynamics simulation of MXene/Phenolic epoxy composites with different amine curing agent systems, Nanomaterials, 12 (2022), 2249. https://doi.org/10.3390/nano12132249 doi: 10.3390/nano12132249
![]() |
[53] |
Y. Xie, H. Lei, H. Huang, H. Pan, S. Zhao, Y. Liu, et al., Molecular simulation on hygrothermal ageing process of epoxy resin, Insul. Mater., 52 (2019), 70–77. https://doi.org/10.16790/j.cnki.1009-9239.im.2019.09.013 doi: 10.16790/j.cnki.1009-9239.im.2019.09.013
![]() |
[54] |
H. Yan, Z. Wang, F. Sun, R. Gao, Y. Fu, Molecular dynamics simulation of crosslinked epoxy resin tensile properties, Eng. Plast. Appl., 50 (2022), 113–117, https://doi.org/10.3969/j.issn.1001-3539.2022.12.019 doi: 10.3969/j.issn.1001-3539.2022.12.019
![]() |
[55] |
S. Yang, J. Qu, Computing thermomechanical properties of crosslinked epoxy by molecular dynamic simulations, Polymer, 53 (2012), 4806–4817, http://dx.doi.org/10.1016/j.polymer.2012.08.045 doi: 10.1016/j.polymer.2012.08.045
![]() |
[56] |
C. Li, A. Strachan, Molecular dynamics predictions of thermal and mechanical properties of thermoset polymer EPON862/DETDA, Polymer, 52 (2011), 2920–2928, https://doi:10.1016/j.polymer.2011.04.041 doi: 10.1016/j.polymer.2011.04.041
![]() |
[57] |
M. Y. Fard, Y. Liu, A. Chattopadhyay, Characterization of epoxy resin including strain rate effects using digital image correlation system, J. Aerosp. Eng., 25 (2012), 308–319. http://dx.doi.org/10.1061/(ASCE)AS.1943-5525.0000127 doi: 10.1061/(ASCE)AS.1943-5525.00001
![]() |
[58] | D. Xin, Mechanical Properties and the Interface Failure Mechanism of Epoxy Resin under Hygrothermal Condition, Ph.D thesis, South China University of Technology, Guangzhou, 2013. |
1. | Saurabh L. Raikar, Dr. Rajesh S. Prabhu Gaonkar, Jaya algorithm in estimation of P[X > Y] for two parameter Weibull distribution, 2021, 7, 2473-6988, 2820, 10.3934/math.2022156 | |
2. | Lishamol Tomy, Veena G, Christophe Chesneau, Applications of the Sine Modified Lindley Distribution to Biomedical Data, 2022, 27, 2297-8747, 43, 10.3390/mca27030043 | |
3. | SidAhmed Benchiha, Amer Ibrahim Al-Omari, Ghadah Alomani, Goodness-of-Fit Tests for Weighted Generalized Quasi-Lindley Distribution Using SRS and RSS with Applications to Real Data, 2022, 11, 2075-1680, 490, 10.3390/axioms11100490 | |
4. | Amer Ibrahim Al-Omari, Rehab Alsultan, Ghadah Alomani, Asymmetric Right-Skewed Size-Biased Bilal Distribution with Mathematical Properties, Reliability Analysis, Inference and Applications, 2023, 15, 2073-8994, 1578, 10.3390/sym15081578 | |
5. | SidAhmed Benchiha, Laxmi Prasad Sapkota, Aned Al Mutairi, Vijay Kumar, Rana H. Khashab, Ahmed M. Gemeay, Mohammed Elgarhy, Said G. Nassr, A New Sine Family of Generalized Distributions: Statistical Inference with Applications, 2023, 28, 2297-8747, 83, 10.3390/mca28040083 |
μWGQLD | σWGQLD | SKWGQLD | KuWGQLD | CVWGQLD | |
α | θ=1.25 | ||||
0.1 | 3.889177 | 1.786969 | 0.897106 | 4.205054 | 0.459472 |
0.2 | 3.793939 | 1.782395 | 0.903213 | 4.217285 | 0.469801 |
0.3 | 3.711037 | 1.776240 | 0.910968 | 4.233681 | 0.478637 |
0.4 | 3.638095 | 1.769174 | 0.919440 | 4.252427 | 0.486291 |
0.5 | 3.573333 | 1.761615 | 0.928126 | 4.272400 | 0.492989 |
0.6 | 3.515385 | 1.753829 | 0.936749 | 4.292896 | 0.498901 |
0.7 | 3.463181 | 1.745987 | 0.945154 | 4.313464 | 0.504157 |
0.8 | 3.415873 | 1.738199 | 0.953260 | 4.333820 | 0.508859 |
0.9 | 3.372777 | 1.730537 | 0.961027 | 4.353780 | 0.513090 |
1 | 3.333333 | 1.723046 | 0.968437 | 4.373230 | 0.516914 |
1.1 | 3.297081 | 1.715755 | 0.975492 | 4.392101 | 0.520386 |
1.2 | 3.263636 | 1.708680 | 0.982196 | 4.410356 | 0.523551 |
1.3 | 3.232675 | 1.701828 | 0.988563 | 4.427977 | 0.526446 |
1.4 | 3.203922 | 1.695202 | 0.994608 | 4.444961 | 0.529102 |
1.5 | 3.177143 | 1.688801 | 1.000346 | 4.461313 | 0.531547 |
θ | α=2 | ||||
0.1 | 38.333333 | 20.749833 | 1.025012 | 4.534152 | 0.5413 |
0.2 | 19.166667 | 10.374916 | 1.025012 | 4.534152 | 0.5413 |
0.3 | 12.777778 | 6.916611 | 1.025012 | 4.534152 | 0.5413 |
0.4 | 9.583333 | 5.187458 | 1.025012 | 4.534152 | 0.5413 |
0.5 | 7.666667 | 4.149967 | 1.025012 | 4.534152 | 0.5413 |
0.6 | 6.388889 | 3.458305 | 1.025012 | 4.534152 | 0.5413 |
0.7 | 5.476190 | 2.964262 | 1.025012 | 4.534152 | 0.5413 |
0.8 | 4.791667 | 2.593729 | 1.025012 | 4.534152 | 0.5413 |
0.9 | 4.259259 | 2.305537 | 1.025012 | 4.534152 | 0.5413 |
1 | 3.833333 | 2.074983 | 1.025012 | 4.534152 | 0.5413 |
1.1 | 3.484848 | 1.886348 | 1.025012 | 4.534152 | 0.5413 |
1.2 | 3.194444 | 1.729153 | 1.025012 | 4.534152 | 0.5413 |
1.3 | 2.948718 | 1.596141 | 1.025012 | 4.534152 | 0.5413 |
1.4 | 2.738095 | 1.482131 | 1.025012 | 4.534152 | 0.5413 |
1.5 | 2.555556 | 1.383322 | 1.025012 | 4.534152 | 0.5413 |
α | HM(5,α) | α | HM(5,α) | α | HM(5,α) | θ | HM(θ,2) | θ | HM(θ,2) | θ | HM(θ,2) |
1 | 0.6000 | 16 | 0.4235 | 31 | 0.4125 | 1 | 2.6666 | 16 | 0.1666 | 31 | 0.0860 |
2 | 0.5333 | 17 | 0.4222 | 32 | 0.4121 | 2 | 1.3333 | 17 | 0.1568 | 32 | 0.0833 |
3 | 0.5000 | 18 | 0.4210 | 33 | 0.4117 | 3 | 0.8888 | 18 | 0.1481 | 33 | 0.0808 |
4 | 0.4800 | 19 | 0.4200 | 34 | 0.4114 | 4 | 0.6666 | 19 | 0.1403 | 34 | 0.0784 |
5 | 0.4666 | 20 | 0.4190 | 35 | 0.4111 | 5 | 0.5333 | 20 | 0.1333 | 35 | 0.0761 |
6 | 0.4571 | 21 | 0.4181 | 36 | 0.4108 | 6 | 0.4444 | 21 | 0.1269 | 36 | 0.0740 |
7 | 0.4500 | 22 | 0.4173 | 37 | 0.4105 | 7 | 0.3809 | 22 | 0.1212 | 37 | 0.0720 |
8 | 0.4444 | 23 | 0.4166 | 38 | 0.4102 | 8 | 0.3333 | 23 | 0.1159 | 38 | 0.0701 |
9 | 0.4400 | 24 | 0.4160 | 39 | 0.4100 | 9 | 0.2962 | 24 | 0.1111 | 39 | 0.0683 |
10 | 0.4363 | 25 | 0.4153 | 40 | 0.4097 | 10 | 0.2666 | 25 | 0.1066 | 40 | 0.0666 |
11 | 0.4333 | 26 | 0.4148 | 41 | 0.4095 | 11 | 0.2424 | 26 | 0.1025 | 41 | 0.0650 |
12 | 0.4307 | 27 | 0.4142 | 42 | 0.4093 | 12 | 0.2222 | 27 | 0.0987 | 42 | 0.0634 |
13 | 0.4285 | 28 | 0.4137 | 43 | 0.4090 | 13 | 0.2051 | 28 | 0.0952 | 43 | 0.0620 |
14 | 0.4266 | 29 | 0.4133 | 44 | 0.4088 | 14 | 0.1904 | 29 | 0.0919 | 44 | 0.0606 |
15 | 0.4250 | 30 | 0.4129 | 45 | 0.4086 | 15 | 0.1777 | 30 | 0.0888 | 45 | 0.0592 |
α | GI(α) | α | GI(α) | α | GI(α) |
1 | 0.2833333 | 16 | 0.3115732 | 31 | 0.3122193 |
2 | 0.2956578 | 17 | 0.3116666 | 32 | 0.3122354 |
3 | 0.3014769 | 18 | 0.3117464 | 33 | 0.3122502 |
4 | 0.3047121 | 19 | 0.3118154 | 34 | 0.3122637 |
5 | 0.3067016 | 20 | 0.3118753 | 35 | 0.3122762 |
6 | 0.3080137 | 21 | 0.3119276 | 36 | 0.3122877 |
7 | 0.3089251 | 22 | 0.3119736 | 37 | 0.3122984 |
8 | 0.3095841 | 23 | 0.3120143 | 38 | 0.3123082 |
9 | 0.3100762 | 24 | 0.3120505 | 39 | 0.3123174 |
10 | 0.3104533 | 25 | 0.3120828 | 40 | 0.3123259 |
11 | 0.3107487 | 26 | 0.3121117 | 41 | 0.3123339 |
12 | 0.3109844 | 27 | 0.3121377 | 42 | 0.3123413 |
13 | 0.3111755 | 28 | 0.3121611 | 43 | 0.3123482 |
14 | 0.3113326 | 29 | 0.3121824 | 44 | 0.3123547 |
15 | 0.3114633 | 30 | 0.3122017 | 45 | 0.3123607 |
Parameters | MLEs | MPS | OLS | WLS | CVEs | AD | ||||||
Es | MSE | Es | MSE | Es | MSE | Es | MSE | Es | MSE | Es | MSE | |
α=0.25 | 0.426 | 0.3075 | 0.377 | 0.2919 | 0.486 | 0.4311 | 0.472 | 0.3890 | 0.456 | 0.3717 | 0.463 | 0.3680 |
θ=1 | 1.010 | 0.0118 | 1.026 | 0.0129 | 1.006 | 0.0134 | 1.005 | 0.0127 | 1.008 | 0.0127 | 1.005 | 0.0121 |
α=0.5 | 0.781 | 1.0029 | 0.718 | 1.0197 | 0.835 | 1.1394 | 0.822 | 1.0732 | 0.804 | 1.0553 | 0.811 | 1.0250 |
θ=1.5 | 1.523 | 0.0288 | 1.550 | 0.0325 | 1.516 | 0.0335 | 1.515 | 0.0319 | 1.521 | 0.0321 | 1.515 | 0.0304 |
α=0.75 | 1.133 | 1.8082 | 1.075 | 1.9053 | 1.196 | 2.0488 | 1.177 | 1.9076 | 1.157 | 1.8866 | 1.167 | 1.8744 |
θ=2 | 2.031 | 0.0562 | 2.068 | 0.0626 | 2.018 | 0.0639 | 2.017 | 0.0604 | 2.025 | 0.0607 | 2.017 | 0.0583 |
α=1 | 1.485 | 2.5509 | 1.429 | 2.6727 | 1.552 | 2.7457 | 1.530 | 2.6005 | 1.511 | 2.5964 | 1.514 | 2.528 |
θ=1 | 1.016 | 0.0140 | 1.036 | 0.0159 | 1.012 | 0.0165 | 1.010 | 0.0156 | 1.015 | 0.0156 | 1.010 | 0.0148 |
α=0.3 | 0.496 | 0.4830 | 0.441 | 0.4607 | 0.546 | 0.5756 | 0.534 | 0.5275 | 0.518 | 0.5111 | 0.527 | 0.5156 |
θ=2 | 2.021 | 0.0487 | 2.053 | 0.0538 | 2.010 | 0.0551 | 2.008 | 0.0527 | 2.015 | 0.0528 | 2.009 | 0.0509 |
α=0.2 | 0.412 | 0.3789 | 0.368 | 0.3773 | 0.464 | 0.5029 | 0.455 | 0.4562 | 0.440 | 0.4340 | 0.445 | 0.4371 |
θ=3 | 3.046 | 0.1080 | 3.094 | 0.1213 | 3.034 | 0.1251 | 3.033 | 0.1202 | 3.044 | 0.1202 | 3.033 | 0.1134 |
α=0.8 | 1.202 | 2.0044 | 1.148 | 2.1251 | 1.266 | 2.2173 | 1.248 | 2.0845 | 1.228 | 2.0673 | 1.237 | 2.0439 |
θ=1 | 1.014 | 0.0141 | 1.034 | 0.0161 | 1.009 | 0.0166 | 1.009 | 0.0157 | 1.013 | 0.0157 | 1.009 | 0.0151 |
α=1 | 1.480 | 2.6725 | 1.429 | 2.8627 | 1.546 | 2.9849 | 1.519 | 2.8085 | 1.502 | 2.7973 | 1.511 | 2.7333 |
θ=3 | 3.043 | 0.1308 | 3.103 | 0.1497 | 3.027 | 0.154 | 3.023 | 0.1462 | 3.036 | 0.1464 | 3.024 | 0.1392 |
Parameters | MLEs | MPS | OLS | WLS | CVEs | AD | ||||||
Es | MSE | Es | MSE | Es | MSE | Es | MSE | Es | MSE | Es | MSE | |
α=0.25 | 0.315 | 0.0771 | 0.283 | 0.0674 | 0.335 | 0.0949 | 0.331 | 0.0878 | 0.323 | 0.0849 | 0.329 | 0.0868 |
θ=1 | 1.001 | 0.0047 | 1.010 | 0.0049 | 0.999 | 0.0053 | 0.999 | 0.0050 | 1.001 | 0.0050 | 0.999 | 0.0049 |
α=0.5 | 0.576 | 0.1816 | 0.534 | 0.1741 | 0.604 | 0.2142 | 0.596 | 0.1981 | 0.586 | 0.1943 | 0.595 | 0.1965 |
θ=1.5 | 1.505 | 0.0113 | 1.520 | 0.0120 | 1.504 | 0.0130 | 1.503 | 0.0123 | 1.506 | 0.0123 | 1.503 | 0.0121 |
α=0.75 | 0.875 | 0.4170 | 0.829 | 0.4199 | 0.902 | 0.4771 | 0.894 | 0.4451 | 0.884 | 0.4409 | 0.892 | 0.4370 |
θ=2 | 2.012 | 0.0217 | 2.033 | 0.0231 | 2.007 | 0.0256 | 2.007 | 0.0238 | 2.011 | 0.0239 | 2.007 | 0.0234 |
α=1 | 1.145 | 0.5971 | 1.101 | 0.6059 | 1.174 | 0.6490 | 1.163 | 0.6149 | 1.153 | 0.6132 | 1.162 | 0.6064 |
θ=1 | 1.004 | 0.0051 | 1.014 | 0.0054 | 1.002 | 0.0058 | 1.002 | 0.0055 | 1.004 | 0.0055 | 1.002 | 0.0054 |
α=0.3 | 0.363 | 0.0849 | 0.328 | 0.0754 | 0.389 | 0.1047 | 0.383 | 0.0972 | 0.375 | 0.0941 | 0.381 | 0.0957 |
θ=2 | 2.007 | 0.0180 | 2.025 | 0.0192 | 2.003 | 0.0211 | 2.003 | 0.0198 | 2.007 | 0.0198 | 2.003 | 0.0195 |
α=0.2 | 0.281 | 0.0654 | 0.252 | 0.0560 | 0.306 | 0.0862 | 0.300 | 0.0780 | 0.293 | 0.0748 | 0.298 | 0.0777 |
θ=3 | 3.015 | 0.0415 | 3.040 | 0.0441 | 3.010 | 0.0479 | 3.010 | 0.0452 | 3.015 | 0.0453 | 3.009 | 0.0444 |
α=0.8 | 0.935 | 0.4789 | 0.891 | 0.4883 | 0.958 | 0.5383 | 0.953 | 0.5067 | 0.943 | 0.5026 | 0.950 | 0.5011 |
θ=1 | 1.005 | 0.0054 | 1.016 | 0.0058 | 1.003 | 0.0062 | 1.003 | 0.0059 | 1.005 | 0.0059 | 1.003 | 0.0058 |
α=1 | 1.157 | 0.7315 | 1.115 | 0.7467 | 1.187 | 0.8219 | 1.174 | 0.7597 | 1.164 | 0.7562 | 1.175 | 0.7566 |
θ=3 | 3.010 | 0.0528 | 3.042 | 0.0561 | 3.004 | 0.0615 | 3.003 | 0.0577 | 3.009 | 0.0578 | 3.003 | 0.0570 |
Parameters | MLEs | MPS | OLS | WLS | CVEs | AD | ||||||
Es | MSE | Es | MSE | Es | MSE | Es | MSE | Es | MSE | Es | MSE | |
α=0.25 | 0.287 | 0.0343 | 0.265 | 0.0312 | 0.3042 | 0.0433 | 0.298 | 0.0393 | 0.294 | 0.0383 | 0.299 | 0.0395 |
θ=1 | 1.003 | 0.0023 | 1.008 | 0.0024 | 1.003 | 0.0026 | 1.002 | 0.0025 | 1.003 | 0.0025 | 1.002 | 0.0024 |
α=0.5 | 0.540 | 0.0819 | 0.511 | 0.0797 | 0.551 | 0.0967 | 0.547 | 0.0892 | 0.542 | 0.0882 | 0.548 | 0.0892 |
θ=1.5 | 1.504 | 0.0057 | 1.513 | 0.0059 | 1.503 | 0.0066 | 1.503 | 0.0062 | 1.504 | 0.0062 | 1.503 | 0.0061 |
α=0.75 | 0.797 | 0.1301 | 0.768 | 0.1295 | 0.811 | 0.1509 | 0.806 | 0.1397 | 0.801 | 0.1387 | 0.807 | 0.1392 |
θ=2 | 2.006 | 0.0099 | 2.018 | 0.0104 | 2.003 | 0.0116 | 2.003 | 0.0107 | 2.005 | 0.0107 | 2.003 | 0.0106 |
α=1 | 1.096 | 0.2412 | 1.069 | 0.2411 | 1.107 | 0.2800 | 1.104 | 0.2593 | 1.098 | 0.2578 | 1.104 | 0.2577 |
θ=1 | 1.005 | 0.0028 | 1.011 | 0.0029 | 1.003 | 0.0033 | 1.004 | 0.0030 | 1.005 | 0.0031 | 1.003 | 0.0030 |
α=0.3 | 0.326 | 0.0369 | 0.302 | 0.0344 | 0.336 | 0.0442 | 0.333 | 0.0412 | 0.329 | 0.0404 | 0.334 | 0.0411 |
θ=2 | 2.002 | 0.0092 | 2.012 | 0.0094 | 1.999 | 0.0102 | 1.999 | 0.0096 | 2.001 | 0.0096 | 1.999 | 0.0096 |
α=0.2 | 0.240 | 0.0262 | 0.220 | 0.0228 | 0.252 | 0.0320 | 0.248 | 0.0297 | 0.244 | 0.0288 | 0.249 | 0.0296 |
θ=3 | 3.009 | 0.0194 | 3.024 | 0.0201 | 3.005 | 0.0220 | 3.005 | 0.0207 | 3.008 | 0.0207 | 3.005 | 0.0205 |
α=0.8 | 0.846 | 0.1625 | 0.818 | 0.1621 | 0.854 | 0.1858 | 0.852 | 0.1748 | 0.847 | 0.1739 | 0.852 | 0.1725 |
θ=1 | 1.000 | 0.0027 | 1.007 | 0.0028 | 0.999 | 0.0032 | 0.999 | 0.0030 | 1.000 | 0.0030 | 0.999 | 0.0029 |
α=1 | 1.071 | 0.2538 | 1.044 | 0.2564 | 1.087 | 0.2868 | 1.080 | 0.2664 | 1.074 | 0.2651 | 1.081 | 0.2669 |
θ=3 | 3.006 | 0.0248 | 3.025 | 0.0258 | 3.003 | 0.0298 | 3.002 | 0.0274 | 3.006 | 0.0274 | 3.002 | 0.0273 |
Parameters | MLEs | MPS | OLS | WLS | CVEs | AD | ||||||
Es | MSE | Es | MSE | Es | MSE | Es | MSE | Es | MSE | Es | MSE | |
α=0.25 | 0.263 | 0.0162 | 0.249 | 0.0154 | 0.271 | 0.0204 | 0.268 | 0.0185 | 0.266 | 0.0183 | 0.269 | 0.0185 |
θ=1 | 1.000 | 0.0011 | 1.003 | 0.0011 | 1.000 | 0.0013 | 1.000 | 0.0012 | 1.001 | 0.0012 | 1.000 | 0.0012 |
α=0.5 | 0.512 | 0.0364 | 0.495 | 0.0362 | 0.519 | 0.0430 | 0.517 | 0.0397 | 0.514 | 0.0395 | 0.517 | 0.0397 |
θ=1.5 | 1.501 | 0.0028 | 1.506 | 0.0028 | 1.500 | 0.0032 | 1.500 | 0.0030 | 1.501 | 0.0030 | 1.500 | 0.0030 |
α=0.75 | 0.762 | 0.0563 | 0.745 | 0.0564 | 0.770 | 0.0637 | 0.767 | 0.0594 | 0.764 | 0.0592 | 0.767 | 0.0592 |
θ=2 | 2.000 | 0.0048 | 2.007 | 0.0049 | 1.999 | 0.0054 | 1.999 | 0.00511 | 2.000 | 0.0051 | 1.998 | 0.0050 |
α=1 | 1.026 | 0.0976 | 1.011 | 0.0979 | 1.034 | 0.1101 | 1.031 | 0.1031 | 1.028 | 0.1028 | 1.031 | 0.1033 |
θ=1 | 1.000 | 0.0013 | 1.004 | 0.0013 | 0.999 | 0.0015 | 1.000 | 0.0014 | 1.000 | 0.0014 | 1.000 | 0.0014 |
α=0.3 | 0.307 | 0.0191 | 0.291 | 0.0186 | 0.315 | 0.0229 | 0.313 | 0.0212 | 0.310 | 0.0210 | 0.313 | 0.0212 |
θ=2 | 2.000 | 0.0046 | 2.007 | 0.0047 | 1.999 | 0.0051 | 1.999 | 0.0048 | 2.000 | 0.0048 | 1.999 | 0.0048 |
α=0.2 | 0.215 | 0.0125 | 0.202 | 0.0114 | 0.222 | 0.0149 | 0.220 | 0.0138 | 0.217 | 0.0136 | 0.220 | 0.0138 |
θ=3 | 2.999 | 0.0100 | 3.008 | 0.0102 | 2.998 | 0.0113 | 2.998 | 0.0107 | 2.999 | 0.0107 | 2.998 | 0.0106 |
α=0.8 | 0.837 | 0.0749 | 0.821 | 0.0744 | 0.842 | 0.0846 | 0.841 | 0.0793 | 0.838 | 0.0790 | 0.842 | 0.0792 |
θ=1 | 1.002 | 0.0013 | 1.006 | 0.0014 | 1.001 | 0.0015 | 1.002 | 0.0014 | 1.002 | 0.0014 | 1.002 | 0.0014 |
α=1 | 1.030 | 0.0935 | 1.015 | 0.0935 | 1.040 | 0.1044 | 1.036 | 0.0978 | 1.033 | 0.0975 | 1.036 | 0.0976 |
θ=3 | 3.003 | 0.0116 | 3.014 | 0.0119 | 3.002 | 0.0133 | 3.002 | 0.0125 | 3.004 | 0.0125 | 3.001 | 0.0124 |
n | Min | Max | Mean | Median | St derivation | Kurtosis | Skweness | |
Data Set 1: | 100 | 60.000 | 463.000 | 175.670 | 158.000 | 83.166 | 1.713 | 1.316 |
Data Set 2: | 100 | 0.390 | 5.560 | 2.621 | 2.700 | 1.013 | 0.043 | 0.362 |
Data Set 3: | 90 | 121.000 | 670.000 | 294.322 | 274.500 | 131.618 | 0.369 | 0.927 |
Data Set 4: | 32 | 0 | 8 | 3.97 | 4 | 2.24 | -0.98 | -3.35 |
Model | AIC | CAIC | BIC | HQIC | K-S | p-value |
WGQLD | 1142.145 | 1142.268 | 1147.355 | 1144.253 | 0.059902 | 0.865573 |
GQLD | 1145.844 | 1145.968 | 1151.055 | 1147.953 | 0.095349 | 0.323229 |
QLD | 1180.179 | 1180.303 | 1185.389 | 1182.288 | 0.216170 | 0.000174 |
PD | 1237.721 | 1237.845 | 1242.932 | 1239.83 | 0.340971 | 1.59e-10 |
TSPD | 1156.301 | 1156.425 | 1161.511 | 1158.41 | 0.143704 | 0.032159 |
Model | AIC | AICc | BIC | HQIC | K-S | p-value |
WGQLD | 295.1091 | 295.2328 | 300.3194 | 297.2178 | 0.105898 | 0.212049 |
GQLD | 306.1634 | 306.2871 | 311.3737 | 308.2721 | 0.123234 | 0.095915 |
QLD | 346.108 | 346.2317 | 351.3183 | 348.2167 | 0.223871 | 8.86e-05 |
PD | 396.7418 | 396.8655 | 401.9522 | 398.8505 | 0.320272 | 2.46-09 |
TSPD | 350.3233 | 350.447 | 355.5336 | 352.432 | 0.220935 | 0.000115 |
Model | AIC | AICc | BIC | HQIC | K-S | p-value |
WGQLD | 1118.42 | 1118.558 | 1123.420 | 1120.436 | 0.0606885 | 0.8946827 |
GQLD | 1122.41 | 1122.548 | 1127.410 | 1124.426 | 0.0900125 | 0.4593636 |
QLD | 1154.697 | 1154.835 | 1159.696 | 1156.713 | 0.2081433 | 0.0008209 |
PD | 1207.242 | 1207.38 | 1212.241 | 1209.258 | 0.3435296 | 1.190e-09 |
TSPD | 1132.559 | 1132.697 | 1137.559 | 1134.575 | 0.1352032 | 0.0744752 |
Model | AIC | AICc | BIC | HQIC | K-S | p-value |
WGQLD | 161.0522 | 161.4966 | 163.8546 | 161.9487 | 0.3077698 | 0.006804395 |
GQLD | 166.9975 | 167.4419 | 169.7999 | 167.894 | 0.3318577 | 0.00269967 |
μWGQLD | σWGQLD | SKWGQLD | KuWGQLD | CVWGQLD | |
α | θ=1.25 | ||||
0.1 | 3.889177 | 1.786969 | 0.897106 | 4.205054 | 0.459472 |
0.2 | 3.793939 | 1.782395 | 0.903213 | 4.217285 | 0.469801 |
0.3 | 3.711037 | 1.776240 | 0.910968 | 4.233681 | 0.478637 |
0.4 | 3.638095 | 1.769174 | 0.919440 | 4.252427 | 0.486291 |
0.5 | 3.573333 | 1.761615 | 0.928126 | 4.272400 | 0.492989 |
0.6 | 3.515385 | 1.753829 | 0.936749 | 4.292896 | 0.498901 |
0.7 | 3.463181 | 1.745987 | 0.945154 | 4.313464 | 0.504157 |
0.8 | 3.415873 | 1.738199 | 0.953260 | 4.333820 | 0.508859 |
0.9 | 3.372777 | 1.730537 | 0.961027 | 4.353780 | 0.513090 |
1 | 3.333333 | 1.723046 | 0.968437 | 4.373230 | 0.516914 |
1.1 | 3.297081 | 1.715755 | 0.975492 | 4.392101 | 0.520386 |
1.2 | 3.263636 | 1.708680 | 0.982196 | 4.410356 | 0.523551 |
1.3 | 3.232675 | 1.701828 | 0.988563 | 4.427977 | 0.526446 |
1.4 | 3.203922 | 1.695202 | 0.994608 | 4.444961 | 0.529102 |
1.5 | 3.177143 | 1.688801 | 1.000346 | 4.461313 | 0.531547 |
θ | α=2 | ||||
0.1 | 38.333333 | 20.749833 | 1.025012 | 4.534152 | 0.5413 |
0.2 | 19.166667 | 10.374916 | 1.025012 | 4.534152 | 0.5413 |
0.3 | 12.777778 | 6.916611 | 1.025012 | 4.534152 | 0.5413 |
0.4 | 9.583333 | 5.187458 | 1.025012 | 4.534152 | 0.5413 |
0.5 | 7.666667 | 4.149967 | 1.025012 | 4.534152 | 0.5413 |
0.6 | 6.388889 | 3.458305 | 1.025012 | 4.534152 | 0.5413 |
0.7 | 5.476190 | 2.964262 | 1.025012 | 4.534152 | 0.5413 |
0.8 | 4.791667 | 2.593729 | 1.025012 | 4.534152 | 0.5413 |
0.9 | 4.259259 | 2.305537 | 1.025012 | 4.534152 | 0.5413 |
1 | 3.833333 | 2.074983 | 1.025012 | 4.534152 | 0.5413 |
1.1 | 3.484848 | 1.886348 | 1.025012 | 4.534152 | 0.5413 |
1.2 | 3.194444 | 1.729153 | 1.025012 | 4.534152 | 0.5413 |
1.3 | 2.948718 | 1.596141 | 1.025012 | 4.534152 | 0.5413 |
1.4 | 2.738095 | 1.482131 | 1.025012 | 4.534152 | 0.5413 |
1.5 | 2.555556 | 1.383322 | 1.025012 | 4.534152 | 0.5413 |
α | HM(5,α) | α | HM(5,α) | α | HM(5,α) | θ | HM(θ,2) | θ | HM(θ,2) | θ | HM(θ,2) |
1 | 0.6000 | 16 | 0.4235 | 31 | 0.4125 | 1 | 2.6666 | 16 | 0.1666 | 31 | 0.0860 |
2 | 0.5333 | 17 | 0.4222 | 32 | 0.4121 | 2 | 1.3333 | 17 | 0.1568 | 32 | 0.0833 |
3 | 0.5000 | 18 | 0.4210 | 33 | 0.4117 | 3 | 0.8888 | 18 | 0.1481 | 33 | 0.0808 |
4 | 0.4800 | 19 | 0.4200 | 34 | 0.4114 | 4 | 0.6666 | 19 | 0.1403 | 34 | 0.0784 |
5 | 0.4666 | 20 | 0.4190 | 35 | 0.4111 | 5 | 0.5333 | 20 | 0.1333 | 35 | 0.0761 |
6 | 0.4571 | 21 | 0.4181 | 36 | 0.4108 | 6 | 0.4444 | 21 | 0.1269 | 36 | 0.0740 |
7 | 0.4500 | 22 | 0.4173 | 37 | 0.4105 | 7 | 0.3809 | 22 | 0.1212 | 37 | 0.0720 |
8 | 0.4444 | 23 | 0.4166 | 38 | 0.4102 | 8 | 0.3333 | 23 | 0.1159 | 38 | 0.0701 |
9 | 0.4400 | 24 | 0.4160 | 39 | 0.4100 | 9 | 0.2962 | 24 | 0.1111 | 39 | 0.0683 |
10 | 0.4363 | 25 | 0.4153 | 40 | 0.4097 | 10 | 0.2666 | 25 | 0.1066 | 40 | 0.0666 |
11 | 0.4333 | 26 | 0.4148 | 41 | 0.4095 | 11 | 0.2424 | 26 | 0.1025 | 41 | 0.0650 |
12 | 0.4307 | 27 | 0.4142 | 42 | 0.4093 | 12 | 0.2222 | 27 | 0.0987 | 42 | 0.0634 |
13 | 0.4285 | 28 | 0.4137 | 43 | 0.4090 | 13 | 0.2051 | 28 | 0.0952 | 43 | 0.0620 |
14 | 0.4266 | 29 | 0.4133 | 44 | 0.4088 | 14 | 0.1904 | 29 | 0.0919 | 44 | 0.0606 |
15 | 0.4250 | 30 | 0.4129 | 45 | 0.4086 | 15 | 0.1777 | 30 | 0.0888 | 45 | 0.0592 |
α | GI(α) | α | GI(α) | α | GI(α) |
1 | 0.2833333 | 16 | 0.3115732 | 31 | 0.3122193 |
2 | 0.2956578 | 17 | 0.3116666 | 32 | 0.3122354 |
3 | 0.3014769 | 18 | 0.3117464 | 33 | 0.3122502 |
4 | 0.3047121 | 19 | 0.3118154 | 34 | 0.3122637 |
5 | 0.3067016 | 20 | 0.3118753 | 35 | 0.3122762 |
6 | 0.3080137 | 21 | 0.3119276 | 36 | 0.3122877 |
7 | 0.3089251 | 22 | 0.3119736 | 37 | 0.3122984 |
8 | 0.3095841 | 23 | 0.3120143 | 38 | 0.3123082 |
9 | 0.3100762 | 24 | 0.3120505 | 39 | 0.3123174 |
10 | 0.3104533 | 25 | 0.3120828 | 40 | 0.3123259 |
11 | 0.3107487 | 26 | 0.3121117 | 41 | 0.3123339 |
12 | 0.3109844 | 27 | 0.3121377 | 42 | 0.3123413 |
13 | 0.3111755 | 28 | 0.3121611 | 43 | 0.3123482 |
14 | 0.3113326 | 29 | 0.3121824 | 44 | 0.3123547 |
15 | 0.3114633 | 30 | 0.3122017 | 45 | 0.3123607 |
Parameters | MLEs | MPS | OLS | WLS | CVEs | AD | ||||||
Es | MSE | Es | MSE | Es | MSE | Es | MSE | Es | MSE | Es | MSE | |
α=0.25 | 0.426 | 0.3075 | 0.377 | 0.2919 | 0.486 | 0.4311 | 0.472 | 0.3890 | 0.456 | 0.3717 | 0.463 | 0.3680 |
θ=1 | 1.010 | 0.0118 | 1.026 | 0.0129 | 1.006 | 0.0134 | 1.005 | 0.0127 | 1.008 | 0.0127 | 1.005 | 0.0121 |
α=0.5 | 0.781 | 1.0029 | 0.718 | 1.0197 | 0.835 | 1.1394 | 0.822 | 1.0732 | 0.804 | 1.0553 | 0.811 | 1.0250 |
θ=1.5 | 1.523 | 0.0288 | 1.550 | 0.0325 | 1.516 | 0.0335 | 1.515 | 0.0319 | 1.521 | 0.0321 | 1.515 | 0.0304 |
α=0.75 | 1.133 | 1.8082 | 1.075 | 1.9053 | 1.196 | 2.0488 | 1.177 | 1.9076 | 1.157 | 1.8866 | 1.167 | 1.8744 |
θ=2 | 2.031 | 0.0562 | 2.068 | 0.0626 | 2.018 | 0.0639 | 2.017 | 0.0604 | 2.025 | 0.0607 | 2.017 | 0.0583 |
α=1 | 1.485 | 2.5509 | 1.429 | 2.6727 | 1.552 | 2.7457 | 1.530 | 2.6005 | 1.511 | 2.5964 | 1.514 | 2.528 |
θ=1 | 1.016 | 0.0140 | 1.036 | 0.0159 | 1.012 | 0.0165 | 1.010 | 0.0156 | 1.015 | 0.0156 | 1.010 | 0.0148 |
α=0.3 | 0.496 | 0.4830 | 0.441 | 0.4607 | 0.546 | 0.5756 | 0.534 | 0.5275 | 0.518 | 0.5111 | 0.527 | 0.5156 |
θ=2 | 2.021 | 0.0487 | 2.053 | 0.0538 | 2.010 | 0.0551 | 2.008 | 0.0527 | 2.015 | 0.0528 | 2.009 | 0.0509 |
α=0.2 | 0.412 | 0.3789 | 0.368 | 0.3773 | 0.464 | 0.5029 | 0.455 | 0.4562 | 0.440 | 0.4340 | 0.445 | 0.4371 |
θ=3 | 3.046 | 0.1080 | 3.094 | 0.1213 | 3.034 | 0.1251 | 3.033 | 0.1202 | 3.044 | 0.1202 | 3.033 | 0.1134 |
α=0.8 | 1.202 | 2.0044 | 1.148 | 2.1251 | 1.266 | 2.2173 | 1.248 | 2.0845 | 1.228 | 2.0673 | 1.237 | 2.0439 |
θ=1 | 1.014 | 0.0141 | 1.034 | 0.0161 | 1.009 | 0.0166 | 1.009 | 0.0157 | 1.013 | 0.0157 | 1.009 | 0.0151 |
α=1 | 1.480 | 2.6725 | 1.429 | 2.8627 | 1.546 | 2.9849 | 1.519 | 2.8085 | 1.502 | 2.7973 | 1.511 | 2.7333 |
θ=3 | 3.043 | 0.1308 | 3.103 | 0.1497 | 3.027 | 0.154 | 3.023 | 0.1462 | 3.036 | 0.1464 | 3.024 | 0.1392 |
Parameters | MLEs | MPS | OLS | WLS | CVEs | AD | ||||||
Es | MSE | Es | MSE | Es | MSE | Es | MSE | Es | MSE | Es | MSE | |
α=0.25 | 0.315 | 0.0771 | 0.283 | 0.0674 | 0.335 | 0.0949 | 0.331 | 0.0878 | 0.323 | 0.0849 | 0.329 | 0.0868 |
θ=1 | 1.001 | 0.0047 | 1.010 | 0.0049 | 0.999 | 0.0053 | 0.999 | 0.0050 | 1.001 | 0.0050 | 0.999 | 0.0049 |
α=0.5 | 0.576 | 0.1816 | 0.534 | 0.1741 | 0.604 | 0.2142 | 0.596 | 0.1981 | 0.586 | 0.1943 | 0.595 | 0.1965 |
θ=1.5 | 1.505 | 0.0113 | 1.520 | 0.0120 | 1.504 | 0.0130 | 1.503 | 0.0123 | 1.506 | 0.0123 | 1.503 | 0.0121 |
α=0.75 | 0.875 | 0.4170 | 0.829 | 0.4199 | 0.902 | 0.4771 | 0.894 | 0.4451 | 0.884 | 0.4409 | 0.892 | 0.4370 |
θ=2 | 2.012 | 0.0217 | 2.033 | 0.0231 | 2.007 | 0.0256 | 2.007 | 0.0238 | 2.011 | 0.0239 | 2.007 | 0.0234 |
α=1 | 1.145 | 0.5971 | 1.101 | 0.6059 | 1.174 | 0.6490 | 1.163 | 0.6149 | 1.153 | 0.6132 | 1.162 | 0.6064 |
θ=1 | 1.004 | 0.0051 | 1.014 | 0.0054 | 1.002 | 0.0058 | 1.002 | 0.0055 | 1.004 | 0.0055 | 1.002 | 0.0054 |
α=0.3 | 0.363 | 0.0849 | 0.328 | 0.0754 | 0.389 | 0.1047 | 0.383 | 0.0972 | 0.375 | 0.0941 | 0.381 | 0.0957 |
θ=2 | 2.007 | 0.0180 | 2.025 | 0.0192 | 2.003 | 0.0211 | 2.003 | 0.0198 | 2.007 | 0.0198 | 2.003 | 0.0195 |
α=0.2 | 0.281 | 0.0654 | 0.252 | 0.0560 | 0.306 | 0.0862 | 0.300 | 0.0780 | 0.293 | 0.0748 | 0.298 | 0.0777 |
θ=3 | 3.015 | 0.0415 | 3.040 | 0.0441 | 3.010 | 0.0479 | 3.010 | 0.0452 | 3.015 | 0.0453 | 3.009 | 0.0444 |
α=0.8 | 0.935 | 0.4789 | 0.891 | 0.4883 | 0.958 | 0.5383 | 0.953 | 0.5067 | 0.943 | 0.5026 | 0.950 | 0.5011 |
θ=1 | 1.005 | 0.0054 | 1.016 | 0.0058 | 1.003 | 0.0062 | 1.003 | 0.0059 | 1.005 | 0.0059 | 1.003 | 0.0058 |
α=1 | 1.157 | 0.7315 | 1.115 | 0.7467 | 1.187 | 0.8219 | 1.174 | 0.7597 | 1.164 | 0.7562 | 1.175 | 0.7566 |
θ=3 | 3.010 | 0.0528 | 3.042 | 0.0561 | 3.004 | 0.0615 | 3.003 | 0.0577 | 3.009 | 0.0578 | 3.003 | 0.0570 |
Parameters | MLEs | MPS | OLS | WLS | CVEs | AD | ||||||
Es | MSE | Es | MSE | Es | MSE | Es | MSE | Es | MSE | Es | MSE | |
α=0.25 | 0.287 | 0.0343 | 0.265 | 0.0312 | 0.3042 | 0.0433 | 0.298 | 0.0393 | 0.294 | 0.0383 | 0.299 | 0.0395 |
θ=1 | 1.003 | 0.0023 | 1.008 | 0.0024 | 1.003 | 0.0026 | 1.002 | 0.0025 | 1.003 | 0.0025 | 1.002 | 0.0024 |
α=0.5 | 0.540 | 0.0819 | 0.511 | 0.0797 | 0.551 | 0.0967 | 0.547 | 0.0892 | 0.542 | 0.0882 | 0.548 | 0.0892 |
θ=1.5 | 1.504 | 0.0057 | 1.513 | 0.0059 | 1.503 | 0.0066 | 1.503 | 0.0062 | 1.504 | 0.0062 | 1.503 | 0.0061 |
α=0.75 | 0.797 | 0.1301 | 0.768 | 0.1295 | 0.811 | 0.1509 | 0.806 | 0.1397 | 0.801 | 0.1387 | 0.807 | 0.1392 |
θ=2 | 2.006 | 0.0099 | 2.018 | 0.0104 | 2.003 | 0.0116 | 2.003 | 0.0107 | 2.005 | 0.0107 | 2.003 | 0.0106 |
α=1 | 1.096 | 0.2412 | 1.069 | 0.2411 | 1.107 | 0.2800 | 1.104 | 0.2593 | 1.098 | 0.2578 | 1.104 | 0.2577 |
θ=1 | 1.005 | 0.0028 | 1.011 | 0.0029 | 1.003 | 0.0033 | 1.004 | 0.0030 | 1.005 | 0.0031 | 1.003 | 0.0030 |
α=0.3 | 0.326 | 0.0369 | 0.302 | 0.0344 | 0.336 | 0.0442 | 0.333 | 0.0412 | 0.329 | 0.0404 | 0.334 | 0.0411 |
θ=2 | 2.002 | 0.0092 | 2.012 | 0.0094 | 1.999 | 0.0102 | 1.999 | 0.0096 | 2.001 | 0.0096 | 1.999 | 0.0096 |
α=0.2 | 0.240 | 0.0262 | 0.220 | 0.0228 | 0.252 | 0.0320 | 0.248 | 0.0297 | 0.244 | 0.0288 | 0.249 | 0.0296 |
θ=3 | 3.009 | 0.0194 | 3.024 | 0.0201 | 3.005 | 0.0220 | 3.005 | 0.0207 | 3.008 | 0.0207 | 3.005 | 0.0205 |
α=0.8 | 0.846 | 0.1625 | 0.818 | 0.1621 | 0.854 | 0.1858 | 0.852 | 0.1748 | 0.847 | 0.1739 | 0.852 | 0.1725 |
θ=1 | 1.000 | 0.0027 | 1.007 | 0.0028 | 0.999 | 0.0032 | 0.999 | 0.0030 | 1.000 | 0.0030 | 0.999 | 0.0029 |
α=1 | 1.071 | 0.2538 | 1.044 | 0.2564 | 1.087 | 0.2868 | 1.080 | 0.2664 | 1.074 | 0.2651 | 1.081 | 0.2669 |
θ=3 | 3.006 | 0.0248 | 3.025 | 0.0258 | 3.003 | 0.0298 | 3.002 | 0.0274 | 3.006 | 0.0274 | 3.002 | 0.0273 |
Parameters | MLEs | MPS | OLS | WLS | CVEs | AD | ||||||
Es | MSE | Es | MSE | Es | MSE | Es | MSE | Es | MSE | Es | MSE | |
α=0.25 | 0.263 | 0.0162 | 0.249 | 0.0154 | 0.271 | 0.0204 | 0.268 | 0.0185 | 0.266 | 0.0183 | 0.269 | 0.0185 |
θ=1 | 1.000 | 0.0011 | 1.003 | 0.0011 | 1.000 | 0.0013 | 1.000 | 0.0012 | 1.001 | 0.0012 | 1.000 | 0.0012 |
α=0.5 | 0.512 | 0.0364 | 0.495 | 0.0362 | 0.519 | 0.0430 | 0.517 | 0.0397 | 0.514 | 0.0395 | 0.517 | 0.0397 |
θ=1.5 | 1.501 | 0.0028 | 1.506 | 0.0028 | 1.500 | 0.0032 | 1.500 | 0.0030 | 1.501 | 0.0030 | 1.500 | 0.0030 |
α=0.75 | 0.762 | 0.0563 | 0.745 | 0.0564 | 0.770 | 0.0637 | 0.767 | 0.0594 | 0.764 | 0.0592 | 0.767 | 0.0592 |
θ=2 | 2.000 | 0.0048 | 2.007 | 0.0049 | 1.999 | 0.0054 | 1.999 | 0.00511 | 2.000 | 0.0051 | 1.998 | 0.0050 |
α=1 | 1.026 | 0.0976 | 1.011 | 0.0979 | 1.034 | 0.1101 | 1.031 | 0.1031 | 1.028 | 0.1028 | 1.031 | 0.1033 |
θ=1 | 1.000 | 0.0013 | 1.004 | 0.0013 | 0.999 | 0.0015 | 1.000 | 0.0014 | 1.000 | 0.0014 | 1.000 | 0.0014 |
α=0.3 | 0.307 | 0.0191 | 0.291 | 0.0186 | 0.315 | 0.0229 | 0.313 | 0.0212 | 0.310 | 0.0210 | 0.313 | 0.0212 |
θ=2 | 2.000 | 0.0046 | 2.007 | 0.0047 | 1.999 | 0.0051 | 1.999 | 0.0048 | 2.000 | 0.0048 | 1.999 | 0.0048 |
α=0.2 | 0.215 | 0.0125 | 0.202 | 0.0114 | 0.222 | 0.0149 | 0.220 | 0.0138 | 0.217 | 0.0136 | 0.220 | 0.0138 |
θ=3 | 2.999 | 0.0100 | 3.008 | 0.0102 | 2.998 | 0.0113 | 2.998 | 0.0107 | 2.999 | 0.0107 | 2.998 | 0.0106 |
α=0.8 | 0.837 | 0.0749 | 0.821 | 0.0744 | 0.842 | 0.0846 | 0.841 | 0.0793 | 0.838 | 0.0790 | 0.842 | 0.0792 |
θ=1 | 1.002 | 0.0013 | 1.006 | 0.0014 | 1.001 | 0.0015 | 1.002 | 0.0014 | 1.002 | 0.0014 | 1.002 | 0.0014 |
α=1 | 1.030 | 0.0935 | 1.015 | 0.0935 | 1.040 | 0.1044 | 1.036 | 0.0978 | 1.033 | 0.0975 | 1.036 | 0.0976 |
θ=3 | 3.003 | 0.0116 | 3.014 | 0.0119 | 3.002 | 0.0133 | 3.002 | 0.0125 | 3.004 | 0.0125 | 3.001 | 0.0124 |
n | Min | Max | Mean | Median | St derivation | Kurtosis | Skweness | |
Data Set 1: | 100 | 60.000 | 463.000 | 175.670 | 158.000 | 83.166 | 1.713 | 1.316 |
Data Set 2: | 100 | 0.390 | 5.560 | 2.621 | 2.700 | 1.013 | 0.043 | 0.362 |
Data Set 3: | 90 | 121.000 | 670.000 | 294.322 | 274.500 | 131.618 | 0.369 | 0.927 |
Data Set 4: | 32 | 0 | 8 | 3.97 | 4 | 2.24 | -0.98 | -3.35 |
Model | AIC | CAIC | BIC | HQIC | K-S | p-value |
WGQLD | 1142.145 | 1142.268 | 1147.355 | 1144.253 | 0.059902 | 0.865573 |
GQLD | 1145.844 | 1145.968 | 1151.055 | 1147.953 | 0.095349 | 0.323229 |
QLD | 1180.179 | 1180.303 | 1185.389 | 1182.288 | 0.216170 | 0.000174 |
PD | 1237.721 | 1237.845 | 1242.932 | 1239.83 | 0.340971 | 1.59e-10 |
TSPD | 1156.301 | 1156.425 | 1161.511 | 1158.41 | 0.143704 | 0.032159 |
Model | AIC | AICc | BIC | HQIC | K-S | p-value |
WGQLD | 295.1091 | 295.2328 | 300.3194 | 297.2178 | 0.105898 | 0.212049 |
GQLD | 306.1634 | 306.2871 | 311.3737 | 308.2721 | 0.123234 | 0.095915 |
QLD | 346.108 | 346.2317 | 351.3183 | 348.2167 | 0.223871 | 8.86e-05 |
PD | 396.7418 | 396.8655 | 401.9522 | 398.8505 | 0.320272 | 2.46-09 |
TSPD | 350.3233 | 350.447 | 355.5336 | 352.432 | 0.220935 | 0.000115 |
Model | AIC | AICc | BIC | HQIC | K-S | p-value |
WGQLD | 1118.42 | 1118.558 | 1123.420 | 1120.436 | 0.0606885 | 0.8946827 |
GQLD | 1122.41 | 1122.548 | 1127.410 | 1124.426 | 0.0900125 | 0.4593636 |
QLD | 1154.697 | 1154.835 | 1159.696 | 1156.713 | 0.2081433 | 0.0008209 |
PD | 1207.242 | 1207.38 | 1212.241 | 1209.258 | 0.3435296 | 1.190e-09 |
TSPD | 1132.559 | 1132.697 | 1137.559 | 1134.575 | 0.1352032 | 0.0744752 |
Model | AIC | AICc | BIC | HQIC | K-S | p-value |
WGQLD | 161.0522 | 161.4966 | 163.8546 | 161.9487 | 0.3077698 | 0.006804395 |
GQLD | 166.9975 | 167.4419 | 169.7999 | 167.894 | 0.3318577 | 0.00269967 |