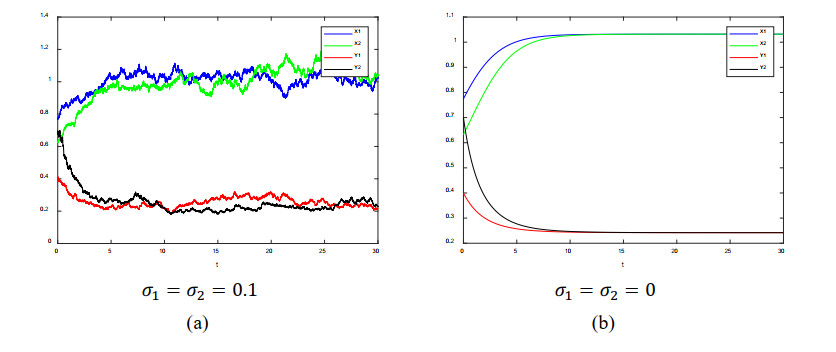
In this paper, a stochastic predator-prey system with mutual interference is studied, which provides guidance on creating appropriate biodegradable environments. By utilizing Mawhin's coincidence degree theorem and constructing a suitable Lyapunov function, a Volterra model with mutual interference is studied. A few sufficient conditions are obtained for existence, extinction and global asymptomatic stability of the positive solution of the model. Then we perform various numerical simulations to verify the stochastic and deterministic systems are global asymptotically stable. It is significant that such a model is firstly proposed with stochastic mutual interference.
Citation: Pinglan Wan. Dynamic behavior of stochastic predator-prey system[J]. Electronic Research Archive, 2023, 31(5): 2925-2939. doi: 10.3934/era.2023147
[1] | Xuemin Fan, Wenjie Zhang, Lu Xu . Global dynamics of a predator-prey model with prey-taxis and hunting cooperation. Electronic Research Archive, 2025, 33(3): 1610-1632. doi: 10.3934/era.2025076 |
[2] | Érika Diz-Pita . Global dynamics of a predator-prey system with immigration in both species. Electronic Research Archive, 2024, 32(2): 762-778. doi: 10.3934/era.2024036 |
[3] | Yuan Tian, Hua Guo, Wenyu Shen, Xinrui Yan, Jie Zheng, Kaibiao Sun . Dynamic analysis and validation of a prey-predator model based on fish harvesting and discontinuous prey refuge effect in uncertain environments. Electronic Research Archive, 2025, 33(2): 973-994. doi: 10.3934/era.2025044 |
[4] | Jialu Tian, Ping Liu . Global dynamics of a modified Leslie-Gower predator-prey model with Beddington-DeAngelis functional response and prey-taxis. Electronic Research Archive, 2022, 30(3): 929-942. doi: 10.3934/era.2022048 |
[5] | Miao Peng, Rui Lin, Zhengdi Zhang, Lei Huang . The dynamics of a delayed predator-prey model with square root functional response and stage structure. Electronic Research Archive, 2024, 32(5): 3275-3298. doi: 10.3934/era.2024150 |
[6] | Xiaowen Zhang, Wufei Huang, Jiaxin Ma, Ruizhi Yang . Hopf bifurcation analysis in a delayed diffusive predator-prey system with nonlocal competition and schooling behavior. Electronic Research Archive, 2022, 30(7): 2510-2523. doi: 10.3934/era.2022128 |
[7] | Yujia Xiang, Yuqi Jiao, Xin Wang, Ruizhi Yang . Dynamics of a delayed diffusive predator-prey model with Allee effect and nonlocal competition in prey and hunting cooperation in predator. Electronic Research Archive, 2023, 31(4): 2120-2138. doi: 10.3934/era.2023109 |
[8] | Yichao Shao, Hengguo Yu, Chenglei Jin, Jingzhe Fang, Min Zhao . Dynamics analysis of a predator-prey model with Allee effect and harvesting effort. Electronic Research Archive, 2024, 32(10): 5682-5716. doi: 10.3934/era.2024263 |
[9] | Ailing Xiang, Liangchen Wang . Boundedness of a predator-prey model with density-dependent motilities and stage structure for the predator. Electronic Research Archive, 2022, 30(5): 1954-1972. doi: 10.3934/era.2022099 |
[10] | Ruizhi Yang, Dan Jin . Dynamics in a predator-prey model with memory effect in predator and fear effect in prey. Electronic Research Archive, 2022, 30(4): 1322-1339. doi: 10.3934/era.2022069 |
In this paper, a stochastic predator-prey system with mutual interference is studied, which provides guidance on creating appropriate biodegradable environments. By utilizing Mawhin's coincidence degree theorem and constructing a suitable Lyapunov function, a Volterra model with mutual interference is studied. A few sufficient conditions are obtained for existence, extinction and global asymptomatic stability of the positive solution of the model. Then we perform various numerical simulations to verify the stochastic and deterministic systems are global asymptotically stable. It is significant that such a model is firstly proposed with stochastic mutual interference.
The biodegradable materials have been a dominant theme due to the in-depth study of the ESG [1]. Under properly controlled conditions, the materials will be decompose into little to no harmful residue. In order to provide guidance on how to create suitable biodegradable [2,3,4,5] environments, this article will investigate a two kinds of populations stochastic prey-predator model.
There are three relationships between two kinds of populations: cooperation, competition, and predator-prey. And the predator-prey relationship is the most popular. In this paper, we consider the dynamic behavior of predator-prey model. The classical deterministic predator-prey model has been widely studied [6,7,8,9,10,11]. In the early 20th century, Lotka and Volterra jointly proposed the classic Lotka-Volterra model in the field of chemistry and the observation on the competitive behavior of fish which have an important role in the modeling of special classes of nonlinear systems such as interaction of microorganisms [12] and population ecology [13]. Through the continuous efforts of scientists, plenty of improved predator-prey models have been obtained which can analyze the complex processes of life phenomena more accurately. The immediate advantage is that individuals can better predict and control the dynamics of the population and then numerous practical problems that human beings encounter in life can be solved [14,15]). Ahmad [17,18] investigates the critical normal form coefficients for two discrete-time Bazykin-Berezovskaya prey-predator models with a strong Allee effect and Mixed Functional Response respectively. There are not a sea of papers researching the mutual interference between the predators and prey while the research on the interaction between predatory predators is of great application value and research meanings. The idea of mutual interference was first proposed by Hassell [19,20] in 1971.
{˙x=xg(t,x)−φ(t,x)ym)˙y=y(−d(t)+kφ(t,x)ym−1−q(t,y)) | (1) |
This can also be generalized to include group or herd defense, toxin production and mimicry [16]. Kai Wang [21] studied the existence, global attractivity and uniqueness of a Volterra model with mutual interference, which can be described by the following form:
{˙x=x(r1−b1x−c1ymk+x)˙y=y(−r2−b2y+c2xym−1k+x) | (2) |
where x(t) and y(t) denote the densities of the prey and the predator at time t, respectively. The parameters r1, r2; b1, b2; c1, c2; m and k are positive constants from the viewpoint of ecology which denote the intrinsic growth rate for the prey, intrinsic death rate of the predator; effect of the density of species on the rate of growth rate of themselves; efficiency of biomass conversion from prey to predator; mutual interference parameter and value of population density at which per capita removal rate is half of x, respectively.
Meanwhile, the noise in the real world always exists. In order to describe clearly, one should consider the effect of noise. On the other hand, by the theory of central limit theorem, we know that the error term obeys the normal distribution, and the stochastic nonautonomous system can be obtained from the deterministic system [22].
r1→r1+σ1(t)dB1(t)−r2→−r2+σ2(t)dB2(t) |
Bi(t),i=1,2 are Brownian motion defined on a complete probability space (Ω,F,P), then corresponding to the deterministic system (2), the similar stochastic nonautonomous is as follow.
{dx=x(r1−b1x−c1ymk+x)dt+σ1(t)xdB1(t)dy=y(−r2−b2y+c2xym−1k+x)dt+σ2(t)ydB2(t)x(0)=x0>0,y(0)=y0>0 | (3) |
For stochastic predator-prey model with mutual interference, Wang and Zhu [23,24] consider the following system
{dx=x(r1−b1x−c1ym)dt+σ1(t)xdB1(t)dy=y(−r2−b2y+c2xym−1)dt+σ2(t)ydB2(t)x(0)=x0>0,y(0)=y0>0 | (4) |
In this paper, the dynamic behavior of the system (3) is studied. Recently, the predator-prey system with different function response has been studied by [25,26,27,28,29,30]. But the predator-prey of the system (3) has not been studied until now. Generally speaking, coincidence degree theory, Jacobi matrix and Fokker-Planck equation are the approaches for studying stochastic system. However, these classical approaches are not useful for the system (3). Therefore, the novelty of this paper is unique system and the extinction and global asymptotic stability characteristic.
The rest of this paper is organized as follows. In Section 2, the existence and uniqueness of the system (3) are given. we will prove that the positive solution of system (3) is globally existent which is the solution is not explosive at finite time. In Section 3, we will carry out the survival analysis for the model and obtain a sufficient condition for the extinction of each species in many cases. In Section 4, sufficient conditions for global asymptotical stability of system (3) are established. In Section 5, the numeric simulations are shown to support the result. Finally, the conclusions and discussions are given in Section 6.
In this section, we present a few results on the existence and uniqueness of global positive solution of system (SM).
{dx=x(r1(t)−b1(t)x−c1(t)ymk+x)dt+σ1(t)xdB1(t)dy=y(−r2(t)−b2(t)y+c2(t)xym−1k+x)dt+σ2(t)ydB2(t)x(0)=x0>0,y(0)=y0>0.(SM) |
In the SM, x(t) and y(t) are the densities of prey population and predator population respectively, only the non-negative solution has ecological significance. In order to make the stochastic differential equation have a global solution, generally speaking, the equation needs to satisfy the linear growth condition and the local Lipschitz condition. However, SM neither satisfies the linear growth condition nor the local Lipschitz condition. Therefore, we use the following method which is a combination of a few stochastic calculus and marting. If f(t) is a continuous bounded function on R+, define
fu=supt∈R+f(t),fl=inft∈R+f(t) |
Throughout this paper, suppose ri(t) , bi(t) and ci(t) , i=1,2 are continuous bounded functions on R+ and ri(t)>0,bi(t)>0,ci(t)>0,i= 1, 1.
The following theorems give a few sufficient conditions for the existence and uniqueness of the global positive solution.
Theorem 1. [22] There is a unique positive local solution (x(t),y(t)) for t∈[0,τe) to SM almost surely (a. s.) for the initial x0 > 0, y0 > 0, where τe is the explosion time.
Proof. Let us suppose x(t)=eu(t),y(t)=ev(t). then by using Itô's formula, we obtain
{du=(r1(t)−b1(t)eu−c1(t)emvk+eu)dt+σ1(t)dB1(t)dv=(−r2(t)−b2(t)ev+c2(t)eue(m−1)vk+eu)dt+σ2(t)dB2(t)(SM') |
Along with u(0)=ln x0,v(0)=ln y0,
We denote the above system along with the initial conditions as (SM). Note that the coefficients of (SM') satisfy the local Lipschitz condition, then for given initial values u0>0,v0>0 there is a unique maximal local solution (u(t),v(t),τe), where τe is the explosion time of the solution. By Ito's formula, x(t)=eu(t),y(t)=ev(t) is the positive local solution to (SM') with initial value x0>0,y0>0.
Theorem 2. For any given initial value (x0,y0)∈R2+ , there is a unique solution (x(t),y(t))ont≥0 to (SM), and this solution will remain in R2+ with probability 1, where R2+={(x,y)∈R2|x,y>0,i=1,2} .
Proof. For convenience, let
F(x,y)=r1(t)−b1(t)x−c1(t)ymk+x |
G(x,y)=−r2(t)−b2(t)ev+c2(t)eue(m−1)vk+eu |
Let n0>0, be large enough, such that for x0 and y0 given, they lie in the interval [1n0,n0]. For each integer n>n0, define the stopping times:
τn=inf{t∈[0,τn]:x(t)∉(1n,n)or y(t)∉(1n,n)} |
Obviously, τn is increasing as n→∞.Let τ∞=limn→∞τn, whence τ∞<τe a.s. We only need to show τ∞=∞. If this statement is false, there exist constants T>0 and ε∈(0,1) such that P{τ∞≤T}>ε. Consequently, there exists an integer n1≥n0 such that
P{τn≤T}>ε;n≥n1 | (5) |
Define a C2 function V:R2+→R+ by
V(x,y)=(√x−1−0.5ln x)+(√y−1−0.5ln y) |
If (x,y) ∈R2, then Ito's formula [8] yields:
dV(x,y)=(x0.5−1)F(x,y)dt+18(−x0.5+2)σ12(t)dt+(x0.5−1)σ1(t)dB1(t)+(y0.5−1)G(x,y)dt+18(−y0.5+2)σ22(t)dt+(y0.5−1)σ2(t)dB2(t) |
Compute
(x0.5−1)F(x,y)=(x0.5−1)(r1(t)−b1(t)x−c1(t)ymk+x)=r1(t)x0.5−b1(t)x1.5−r1(t)+b1(t)x+c1(t)x0.5ymk+x−c1(t)ymk+x≤r1(t)x0.5−b1(t)x1.5−r1(t)+b1(t)x≤K1 |
And
(y0.5−1)G(x,y)=(y0.5−1)(r2(t)−b2(t)y−c2(t)xym−1k+x)=r2(t)y0.5−b2(t)y1.5−r2(t)+b2(t)y+c2(t)xym−0.5k+x−c2(t)ym−1k+x≤r2(t)y0.5−b2(t)y1.5−r2(t)+b2(t)y≤K2 |
where K1 and K2 are given positive constants. Integrating both sides of the above inequality from 0 to τn∧T and then taking the expectation leads to
EV(x(τn∧T),y(τn∧T))≤V(x0,y0)+(K1+K2)T | (6) |
Set Ωn={τn≤T}, by (5) we have P(Ωn)≥ε. Note that for each ω∈Ωn, there are a few i such that xi(τn,ω) equals n or 1/n for i = 1, 2. Hence V(x(τn∧T),y(τn∧T)) is no less than
min{√n−1−0.5ln n,√1n−1−0.5ln 1n} |
Thus by (6) we have
V(x0,y0)+(K1+K2)T≥E[1Ωn(ω)V(x(τn∧T),y(τn∧T))]≥εmin{√n−1−0.5ln n,√1n−1−0.5ln 1n} |
where 1Ωn is the indicator function of Ωn. Letting n→∞, leads to the contraction
∞>V(x0,y0)+(K1+K2)T=∞ |
This completes the proof.
In this section, we investigate extinction criteria in the model, finding that the stochastic forcing can be responsible for extinction of both species, under certain conditions.
Theorem 3. [30,31] Let conditions r1−0.5σ21<0 and c2−r2−0.5σ21<0 hold. The populations x and y by (SM) will become extinct exponentially with probability one.
Proof. Define V(x)=c1x+c2y. By the Itô formula, we have
d[ln x(t)]=(r1−b1x−c1(t)ymk+x−12σ12)dt+σ1(t)dB1(t) | (7) |
Integrating both sides of (7) leads to
ln (x(t)x0)t=∫t0[r1−0.5σ21]dst−∫t0b1xdst |
−∫t0c1ymk+xdst+∫t0σ1dB1(s)t | (8) |
In the same way
ln (y(t)y0)t=∫t0[r2−0.5σ22]dst−∫t0b2ydst |
−∫t0c2xym−1k+xdst+∫t0σ2dB2(s)t | (9) |
Set Mi(t)=∫t0σidBi(t),i=1,2. Then Mi(t) is a local martingale whose quadratic variation is
⟨Mi,Mi⟩t=∫t0σ2ids≤(σ2i)ut | (10) |
By the strong law of large numbers for Martingales [8] we have
limt→∞Mi(t)t=0 a.s. | (11) |
From (8), (10) and r1(t)−0.5σ21<0, we have
limt→+∞supln (x(t))t≤∫t0[r1−0.5σ21]dst<0 a.s. |
Consider m = 1, by the Itô formula, we have
d[ln y(t)]=(−r2−b2y+c2xk+x−12σ22)dt+σ2dB2(t) | (12) |
Integrating both sides of (12) leads to
ln y(t)−ln y(0)=(−r2−12σ22)t−∫t0b2yds+∫t0c2(k+x−k)k+xds+∫t0σ2dB2(s) |
=(c2−r2−12σ22)t−∫t0b2y(s)ds−∫t0c2kk+x(s)ds+σ2dB2(s) | (13) |
By the strong law of large numbers for Martingales, similarly we have
ln y(t)−ln y(0)≤(c2−r2−12σ22)t−∫t0b2yds−∫t0c2kk+xds | (14) |
From (14) and c2−r2−12σ22<0, we have
ln y(t)−ln y(0)≤(c2−r2−12σ22)t<0 |
As t→+∞.
Thus lim→+∞supln y(t)<0a.s.
Consider 0 < m < 1, and the term ∫t0c2(s)x(s)ym−1k+xdst in (9).
From y(t)≥1 , (9) and c2−r2−0.5σ21<0, we have
−∫t0b2(s)y(s)dst−∫t0c2(s)x(s)ym−1k+xdst≤∫t0(c2(s)−b2(s))y(s)dst<0 |
As t→+∞.
We obtain the same result limt→+∞sup ln y(t)<0 a.s.
Definition 4. SM is said to be globally asymptotical stable if
limt→+∞ |x1(t)−x2(t)|=limt→+∞ |y1(t)−y2(t)|=0a.s. |
for any two positive solutions (x1(t),y1(t))and(x2(t),y2(t)) of SM, we first give a few lemmas.
Lemma 5. [18] Let x(t),y(t) be a solution to (SM) with initial value (x0,y0) . If bl2−cu2>0 , then for all p>1 , there exist constants L(p) and G(p) such that
E[xp(t)]≤L(p);E[yp(t)]≤G(p) |
Proof. Define V(x)=xp for all x∈R+,p>1. By the Itô formula, we have
dV(x)=pxp−1dx+0.5p(p−1)xp−2(dx)2=pxp[(r1(t)−b1(t)x−c1(t)ymk+x)+0.5(p−1)σ21(t)]dt+pxpσ1(t)dB1(t) |
Using Itô formula again to etV(x) results in
d[etV(x)]=etV(x)dt+etdV(x)={etxp+petxp[(r1(t)−b1(t)x−c1(t)ymk+x)+0.5(p−1)σ21(t)]}dt+petxpσ1(t)dB1(t) |
Taking expectations on both sides, we have
E[etxp(t)]≤xp0+pE∫t0 es{xp(s)[1p+ru1+0.5(p−1)(σ21)u−bl1x(s)]}ds |
≤xp0+∫t0 esL1(p)ds=xp0+L1(p)(et−1) |
L1(p)=[1+rμ1p+0.5p(p−1)(σ21)u]p+1(p+1)p+1(b'1)p. |
There exists a T>0 such that E[xp(t)]≤1.5L1(p) for all t≥T. At the same time, an application of the continuity of E[xp(t)] results in that there exists ˜L1(p)>0 such that E[xp(t)]≤˜L1(p) for all t≤T. Let L(p)=max{1.5L1(p),˜L1(p)} , then for all t≥0 , we have E[xp(t)]≤L(p).
On the other hand, we can show that
d[etV(y)]=etV(y)dt+etdV(y)={etyp+petyp[(−r2(t)−b2(t)y+c2(t)xym−1k+x+0.5(p−1)σ22(t)]}dt+petypσ2(t)dB2(t) |
Consider k = 1, Taking expectations on both sides results in
E[etyp(t)]≤yp0+pE∫t0 es{yp(s)[1p+cu2x(s)+0.5(p−1)(σ22)u−bl2y(s)]}ds≤yp0+p∫t0 es{E[yp(s)[1p+0.5(p−1)(σ22)u−bl2y(s)]]+cu2E[yp(s)x(s)]}ds,≤yp0+p∫t0 esE[yp(s)[1p+0.5(p−1)(σ22)u−bl2y(s)]]ds+pcu2∫t0 esE[yp+1(s)]+pcu2p+1∫t0 esE[xp+1(s)]ds=yp0+pE∫t0 esyp(s)[1p+0.5(p−1)(σ22)u−(bl2−cu2)y(s)]ds+pcu2p+1∫t0 esE[xp+1(s)]ds≤yp0+∫t0 esL2(p)ds+pcu2p+1L(p+1)∫t0 esds=yp0+[L2(p)+pcu2p+1L(p+1)](et−1) |
where L2(p)=[1+0.5p(p−1)(σ22)u]p+1(p+1)p+1 (bl2−cμ2)p. Thus we get
limt→+∞ sE[yp(t)]≤L2(p)+pcu2p+1L(p+1)=:L3(p) |
Then there exists a T>0 such that E(yp(t))≤1.5L3(p) for all t≥T. There also exists E(yp(t))≤˜L3(p) for t<T. Let G(p)=max{1.5L3(p),˜L3(p)}, then for all t≥0, E(yp(t))≤G(p) .
Lemma 6. [32] Suppose that an n-dimensional stochastic process X(t) on t≥0 satisfies the condition
E|X(t)−X(s)|α1≤c|t−s|1+α2,0≤s,t<∞ |
for a few positive constants α1,α2 and c. Then there exists a continuous modification ˜X(t) of X(t) which has the property that for every ϑ∈(0,α2/α1), there is a positive random variable h(ω) such that
P{ω:sup0<|t−s|<h(ω),0≤s,t<∞ ˜X(t,ω)−X(t,ω)|t−s|ϑ≤21−2−ϑ}=1 |
Lemma 7. [33] Let (x(t),y(t)) be a solution of (SM) on t≥0 . If bl2−cu2>0 , then almost every sample path of (x(t),y(t)) is uniformly continuous.
Proof. The first equation of SM is equivalent to the following stochastic integral equation
x(t)=x0+∫t0 x(s)(r1(s)−b1(s)x(s)−c1(s)ymk+x)ds+∫t0 σ1(s)x(s)dB1(s) |
Note that
E|x(r1(s)−b1(s)x−c1(s)ymk+x)|p=E|x|p|(r1(s)−b1(s)x−c1(s)ymk+x)|p≤0.5E|x|2p+0.5E|(r1(s)−b1(s)x−c1(s)ymk+x)|2p≤0.5{L(2p)+32p−1[(|r1|u)2p+bu1E|x(t)|2p+cu1E|y(t)|2p]}≤0.5{L(2p)+32p−1[(|r1|u)2p+bu1L(2p)+cu1G(2p)]}:=K2(p) |
Moreover, in the view of the moment inequality for stochastic integrals we can obtain that for 0≤t1≤t2 and p>2,
E|∫t2t1 σ1(s)x(s)dB1(s)|p≤[(σ21)u]p[p(p−1)2]p/2(t2−t1)(p−2)∫t2t1 E|x(s)|pds≤[(σ21)u]p[p(p−1)2]p/2(t2−t1)p/2L(p) |
Then for 0<t1<t2<∞,t2−t1≤1,1/p+1/q=1, we have
E(|x(t2)−x(t1)|p)=E|∫t2t1 x(s)[r1(s)−b1(s)x−c1(s)ymk+x]ds+∫t2t1 σ1(s)x(s)dB1(s)|p≤2p−1E|∫t2t1 x(s)[r1(s)−b1(s)x−c1(s)ymk+x]ds|p+2p−1E|∫t2t1 σ1(s)x(s)dB1(s)|p≤2p−1(t2−t1)p/q|∫t2t1 E|x(s)[r1(s)−b1(s)x−c1(s)ymk+x]pds+2p−1[p(p−1)2]p/2(t2−t1)p/2[(σ21)u]pL(p)≤2p−1(t2−t1)p/q+1K2(p)+2p−1[p(p−1)2]p/2(t2−t1)p/2[(σ21)u]pL(p) |
≤2p−1(t2−t1)p/2[(t2−t1)p/2+(p(p−1)2)p/2]K3(p) |
≤2p−1(t2−t1)p/2[1+(p(p−1)2)p/2]K3(p) |
where K3(p)=max{K2(p),[(σ21)u]pL(p)}. By Lemma 6, variable ϑ for every ϑ∈(0,(p−2)/2p) and each sample path of x(t) is uniformly continuous on t≥0. Similarly, we can almost verify y(t) is uniformly continuous.
Lemma 8. [33] Let f be a non-negative function defined on R+ such that f is integrable and is uniformly continuous. Then limt→∞ f(t)=0 .
Theorem 9. If bl2−cu2>0 , then SM is globally asymptotically stable.
Proof. Define V(t)=|lnx1(t)−lnx2(t)|+|lny1(t)−lny2(t)|
For d+V(t) of V(t), applying Itö's formula we obtain
d+V(t)=sgn(x1−x2){[dx1x1−(dx1)22x21]−[dx2x2−(dx2)22x22]} |
+sgn(y1−y2){[dy1y1−(dy1)22y21]−[dy2y2−(dy2)22y22]}=sgn(x1−x2){−b1(t)(x1−x2)−c1(t)(ym1−ym2)k+x}dt+sgn(y1−y2){−b2(t)(y1−y2)+c2(t)(x1ym−11−x2ym−12)k+x}dt≤{−b1(t)|x1−x2|+c1(t)|ym1−ym2|−b2(t)|y1−y2|+c2(t)|x1ym−11−x2ym−12|}dt |
Integrating both sides lead to
V(t)≤V(0)+∫t0 {−b1(s)|x1−x2|+c1(s)|ym1−ym2|k+x−b2(s)|y1−y2|+c2(s)|x1ym−11−x2ym−12|k+x}ds |
thus
V(t)+∫t0 {b1(s)|x1−x2|−c1(s)|ym1−ym2|k+x+b2(s)|y1−y2|−c2(s)|x1ym−11−x2ym−12|k+x}ds≤V(0)<∞ |
It then follows from V(t)≥0 that
|x1(t)−x2(t)|∈L1[0,∞),|y1(t)−y2(t)|∈L1[0,∞) |
This completes the proof.
In this section, we will use Milstein method mentioned in Higham [34] to illustrate our main results, we get the discretization equation of (2) as
{x(k+1)=x(k)+x(k)(r1−b1x(k)−c1(y(k))mk+x(k))Δt+σ1x(k)√Δtξ(k)−σ212x(k)[(ξ(k))2−1]Δty(k+1)=y(k)+y(k)(−r2−b2y(k)+c2x(k)(y(k))m−1k+x(k))Δt+σ2y(k)√Δtη(k)−σ222y(k)[(η(k))2−1]Δt | (15) |
where ξ(k) and η(k) are the Gaussian random variable which follow N(0,1).
Choosing the suitable parameters, the numerical simulation has been given as follow. Without loss of generality, we choose the parameters r1=0.65,b1=0.55,c1=0.27,r2=0.15,b2=0.55,c2=0.27,m=0.5,k=1,Δt=0.0001 . and initial value (x1(0),y1(0))=(0.6,0.4) and (x2(0),y2(0))=(0.4,0.7). The numerical simulation results are shown in Figure 1, the vertical axis represents the population sizes and the horizontal axis represents the time t.
In Figure 1(a), we choose σ1=σ2=0.1. By Theorem 5, SM is global asymptotically stable. In Figure 1(b), we choose σ1=σ2=0. Similarly, system (2) is global asymptotically stable. By Figure 1(a), (b), if the positive equilibrium state of the deterministic system is asymptotic stable, then the corresponding positive equilibrium state of the stochastic system will be globally asymptotic stable when the parameter b2 > c2 and the effect of noise is sufficiently small.
In this paper, we investigate the existence of globally positive solution, extinction and global asymptomatic stability of the populations to Hassell-Varley model with mutual interference in the form (SM), some interest results are obtained under a few natural and simple conditions. The biosphere environment in which the population located is often highly stochastic, and stochastic noise is one of the reasons leading to the extinction of individuals. The impact of mutual interference and environmental perturbation is important so it is of practical significance to study the non-autonomous model with white noise disturbance. We have added white Gaussian noise in the growth rate parameter of the Predator and prey population and observe that the stochastic system preserves the property of the deterministic system when the noise is sufficiently small while the intensity of environmental disturbance is large enough, it may lead to the extinction of the population. Therefore, the model can be used to study the effects of small disturbances as sunlight and rain on population dynamics, as well as to study large disturbances as hail and greenhouse effect on population dynamics. Furthermore, the results of this paper can be applied to biological communities on biodegradable materials, which provides reference value for the sustainable development of ESG and offers advice on creating suitable biodegradable environment.
In the studies of models with white noise and mutual interference, current research concerning optimal predation strategy is not sufficient. Therefore, In future studies we hope to consider this kind of questions to the crowley-Martin or Beddington-DeAngelis models. The main challenge of how to apply this model to biodegradable communities now is to effectively combine and conjugate the requisite cross disciplinary approaches.
The author wishes to acknowledge The Hong Kong Polytechnic University (PolyU).
The author declares no conflict of interest.
[1] |
M. Brogi, V. Lagasio, Environmental, social, and governance and company profitability: Are financial intermediaries different?, Corporate Soc. Responsib. Environ. Manage., 26 (2019), 576–587. https://doi.org/10.1002/csr.1704 doi: 10.1002/csr.1704
![]() |
[2] |
M. A. White, Environmental finance: Value and risk in an age of ecology, Bus. Strategy Environ., 5 (1996), 198–206. https://doi.org/10.1002/(SICI)1099-0836(199609)5:3<198::AID-BSE66>3.0.CO;2-4 doi: 10.1002/(SICI)1099-0836(199609)5:3<198::AID-BSE66>3.0.CO;2-4
![]() |
[3] |
M. V. Suarez, V. F. Cruz, H. L. Tavera, Biodegradation of polyacrylic and polyester polyurethane coatings by enriched microbial communities, Appl. Microbiol. Biotechnol., 103 (2019), 3225–3236. https://doi.org/10.1007/s00253-019-09660-y doi: 10.1007/s00253-019-09660-y
![]() |
[4] |
F. Kawai, The biochemistry and molecular biology of xenobiotic polymer degradation by microorganisms, Biosci. Biotechnol. Biochem., 74 (2010), 1743–1759. https://doi.org/10.1271/bbb.100394 doi: 10.1271/bbb.100394
![]() |
[5] |
E. Nikolaivits, Progressing plastics circularity: A review of mechano-biocatalytic approaches for waste plastic (Re) valorization, Front. Bioeng. Biotechnol., 9 (2021). https://doi.org/10.3389/fbioe.2021.696040 doi: 10.3389/fbioe.2021.696040
![]() |
[6] |
H. Li, L. Zhang, Z. Teng, Y. Jiang, Dynamic behaviors of holling type ii predator-prey system with mutual interference and impulses, Discrete Dyn. Nat. Soc., 2014 (2014), https://doi.org/10.1155/2014/793761 doi: 10.1155/2014/793761
![]() |
[7] |
H. Liu, F. Meng, Existence of positive periodic solutions for a predator-prey system of holling type iv function response with mutual interference and impulsive effects, Discrete Dyn. Nat. Soc., 2015 (2015), 1–12. https://doi.org/10.1155/2015/138984 doi: 10.1155/2015/138984
![]() |
[8] |
K. Wang, Permanence and global asymptotical stability of a predator-prey model with mutual interference, Nonlinear Anal. Real World Appl., 12 (2011), 1062–1071. https://doi.org/10.1016/j.nonrwa.2010.08.028 doi: 10.1016/j.nonrwa.2010.08.028
![]() |
[9] |
R. Wu, Permanence of a discrete periodic volterra model with mutual interference and Beddington-Deangelis functional response, Discrete Dyn. Nat. Soc., 2010 (2010), 1038–1045. https://doi.org/10.1155/2010/246783 doi: 10.1155/2010/246783
![]() |
[10] |
E. Moustafa, M. A. Asmaa, Deterministic and stochastic fractional-order hastings-powell food chain model, Cmc-comput. Mater. Contin., 70 (2022), 2277–2296. https://doi.org/10.32604/cmc.2022.019314 doi: 10.32604/cmc.2022.019314
![]() |
[11] | B. Vahid, Y. M. Javad, T. M. Saleh, Global stabilization of Lotka-Volterra systems with interval uncertainty, 64 (2019), 1209–1213. https://doi.org/10.1109/TAC.2018.2845659 |
[12] |
X. Zheng, D. Li, Interaction of Acidithiobacillus ferrooxidans, Rhizobium phaseoli and Rhodotorula sp. in bioleaching process based on Lotka-Volterra model, Electron. J. Biotechnol., 22 (2016), 90–97. https://doi.org/10.1016/j.ejbt.2016.06.004 doi: 10.1016/j.ejbt.2016.06.004
![]() |
[13] |
R. A. Cropp, J. Norbury, Population interactions in ecology: A rule-based approach to modeling ecosystems in a mass-conserving framework, SIAM Rev., 57 (2015), 437–465. https://doi.org/10.1137/140962528 doi: 10.1137/140962528
![]() |
[14] |
R. Arditi, H. Saiah, Empirical evidence of the role of heterogeneity in ratio-dependent consumption, Ecology, 73 (2008), 1544–1551. https://doi.org/10.2307/1940007 doi: 10.2307/1940007
![]() |
[15] |
X. Zhao, Z. Zeng, Stochastic dynamics of a two-species patch-system with ratio-dependent functional response, Qual. Theory Dyn. Syst., 21 (2022), 1–15. https://doi.org/10.1007/s12346-022-00594-x doi: 10.1007/s12346-022-00594-x
![]() |
[16] |
Q. Yang, X. Zhang, D. Jiang, M. Shao, Analysis of a stochastic predator-prey model with weak Allee effect and Holling-(n+1) functional response, Commun. Nonlinear Sci. Numer. Simul., 111 (2022), 106454. https://doi.org/10.1016/j.cnsns.2022.106454 doi: 10.1016/j.cnsns.2022.106454
![]() |
[17] |
P. A. Naik, Z. Eskandari, M. Yavuz, J. Zu, Complex dynamics of a discrete-time Bazykin-Berezovskaya prey-predator model with a strong Allee effect, J. Comput. Appl. Math., 413 (2022), 114401. https://doi.org/10.1016/j.cam.2022.114401 doi: 10.1016/j.cam.2022.114401
![]() |
[18] |
P. A. Naik, Z. Eskandari, Z. Avazzadeh, J. Zu, Multiple bifurcations of a discrete-time prey-predator model with mixed functional response, Int. J. Bifurcation Chaos, 32 (2022), 2250050. https://doi.org/10.1142/S021812742250050X doi: 10.1142/S021812742250050X
![]() |
[19] |
M. Hassell, Mutual interference between searching insect parasites, J. Anim. Ecol., 40 (1971), 473–486. https://doi.org/10.2307/3256 doi: 10.2307/3256
![]() |
[20] |
A. Watkinson, Density-dependence in single-species population, J. Anim. Ecol., 83 (1980), 345–357. https://doi.org/10.1016/0022-5193(80)90297-0 doi: 10.1016/0022-5193(80)90297-0
![]() |
[21] |
K. Wang, Y. Zhu, Global attractivity of positive periodic solution for a volterra model, Appl. Math. Comput., 203 (2008), 493–501. https://doi.org/10.1016/j.amc.2008.04.005 doi: 10.1016/j.amc.2008.04.005
![]() |
[22] | K. Wang, Stochastic biomathematics model, Sci. Press, 1 (2010), 1–207. |
[23] |
K. Wang, Y. Zhu, Periodic solutions, permanence and global attractivity of a delayed impulsive prey-predator system with mutual interference, Nonlinear Anal. Real World Appl., 14 (2013). https://doi.org/10.1016/j.nonrwa.2012.08.016 doi: 10.1016/j.nonrwa.2012.08.016
![]() |
[24] |
K. Wang, Y. Zhu, Dynamics of a stochastic predator-prey model with mutual interference, Int. J. Biomath., 7 (2014), 1–22. https://doi.org/10.1142/S1793524514500260 doi: 10.1142/S1793524514500260
![]() |
[25] |
L. Wu, H. Zheng, S. Zhang, Dynamics of a non-autonomous predator-prey system with hassell-varley-hollingⅡfunction response and mutual interference, AIMS Math., 6 (2021), 6033–6049. https://doi.org/10.3934/math.2021355 doi: 10.3934/math.2021355
![]() |
[26] |
K. Gupta, S. Gakkhar, The filippov approach for predator-prey system involving mixed type of functional responses, Differ. Equations Dyn. Syst., 28 (2020), 1–21. https://doi.org/10.1007/s12591-016-0322-x doi: 10.1007/s12591-016-0322-x
![]() |
[27] |
X. Li, X. Lin, J. Liu, Existence and global attractivity of positive periodic solutions for a predator-prey model with crowley-martin functional response, Electron. J. Differ. Equations, 191 (2018), 1–17. https://doi.org/10.2298/FIL1901043L doi: 10.2298/FIL1901043L
![]() |
[28] |
L. Chen, F. Chen, Y. Wang, Influence of predator mutual interference and prey refuge on lotka-volterra predator-prey dynamics, Commun. Nonlinear Sci. Numer. Simul., 18 (2013), 3174–180. https://doi.org/10.1016/j.cnsns.2013.04.004 doi: 10.1016/j.cnsns.2013.04.004
![]() |
[29] |
Z. Ma, F. Chen, C. Wu, W. Chen, Dynamic behaviors of a lotka-volterra predator-prey model incorporating a prey refuge and predator mutual interference, Appl. Math. Comput., 219 (2013), 7945–7953. https://doi.org/10.1016/j.amc.2013.02.033 doi: 10.1016/j.amc.2013.02.033
![]() |
[30] |
C. Zhang, N. Huang, D. O'Regan, Almost periodic solutions for a Volterra model with mutual interference and Holling type Ⅲ functional response, Appl. Math. Comput., 225 (2013), 503–511. https://doi.org/10.1016/j.amc.2013.09.047 doi: 10.1016/j.amc.2013.09.047
![]() |
[31] |
X. Lin, F. Chen, Almost periodic solution for a Volterra model with mutual interference and Beddington-DeAngelis functional response, Appl. Math. Comput., 214 (2009), 548–556. https://doi.org/10.1016/j.amc.2009.04.028 doi: 10.1016/j.amc.2009.04.028
![]() |
[32] |
S. Arik, Global asymptotic stability analysis of bidirectional associative memory neural networks with time delays, IEEE Trans. Neural Networks, 16 (2003), 1–7. https://doi.org/10.1109/TNN.2005.844910 doi: 10.1109/TNN.2005.844910
![]() |
[33] |
B. Du, Existence, extinction and global asymptotical stability of a stochastic predator-prey model with mutual interference, J. Appl. Math. Comput., 46 (2014), 79–91. https://doi.org/10.1007/s12190-013-0738-1 doi: 10.1007/s12190-013-0738-1
![]() |
[34] |
D. J. Higham, An algorithmic introduction to numerical simulation of stochastic differential equations, SIAM Rev., 43 (2001), 525–546. https://doi.org/10.1137/S0036144500378302 doi: 10.1137/S0036144500378302
![]() |
1. | Xiangwen Yin, Promoting peer learning in education: Exploring continuous action iterated dilemma and team leader rotation mechanism in peer-led instruction, 2023, 31, 2688-1594, 6552, 10.3934/era.2023331 | |
2. | Xin Du, Quansheng Liu, Yuanhong Bi, Bifurcation analysis of a two–dimensional p53 gene regulatory network without and with time delay, 2023, 32, 2688-1594, 293, 10.3934/era.2024014 |