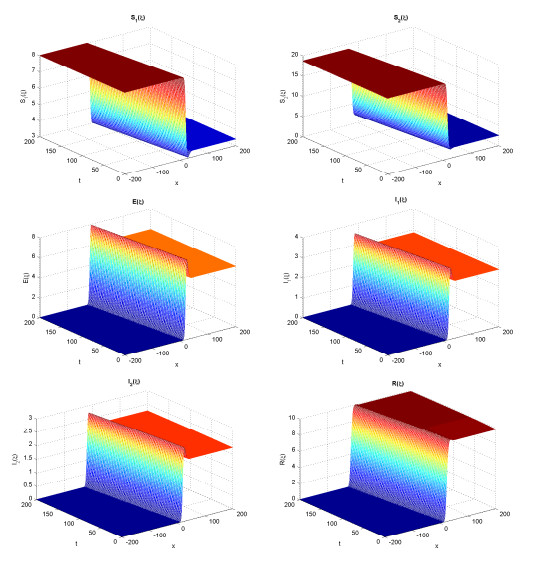
The rise in global ozone levels over the last few decades has harmed human health. This problem exists in several cities throughout South America due to dangerous levels of particulate matter in the air, particularly during the winter season, making it a public health issue. Lima, Peru, is one of the ten cities in South America with the worst levels of air pollution. Thus, efficient and precise modeling and forecasting are critical for ozone concentrations in Lima. The focus is on developing precise forecasting models to anticipate ozone concentrations, providing timely information for adequate public health protection and environmental management. This work used hourly O3 data in metropolitan areas for multi-step-ahead (one-, two-, three-, and seven-day-ahead) O3 forecasts. A multiple linear regression model was used to represent the deterministic portion, and four-time series models, autoregressive, nonparametric autoregressive, autoregressive moving average, and nonlinear neural network autoregressive, were used to describe the stochastic component. The various horizon out-of-sample forecast results for the considered data suggest that the proposed component-based forecasting technique gives a highly consistent, accurate, and efficient gain. This may be expanded to other districts of Lima, different regions of Peru, and even the global level to assess the efficacy of the proposed component-based modeling and forecasting approach. Finally, no analysis has been undertaken using a component-based estimation to forecast ozone concentrations in Lima in a multi-step-ahead manner.
Citation: Flor Quispe, Eddy Salcedo, Hasnain Iftikhar, Aimel Zafar, Murad Khan, Josué E. Turpo-Chaparro, Paulo Canas Rodrigues, Javier Linkolk López-Gonzales. Multi-step ahead ozone level forecasting using a component-based technique: A case study in Lima, Peru[J]. AIMS Environmental Science, 2024, 11(3): 401-425. doi: 10.3934/environsci.2024020
[1] | Ismail Ben Abdallah, Yassine Bouteraa, Saleh Mobayen, Omar Kahouli, Ali Aloui, Mouldi Ben Amara, Maher JEBALI . Fuzzy logic-based vehicle safety estimation using V2V communications and on-board embedded ROS-based architecture for safe traffic management system in hail city. Electronic Research Archive, 2023, 31(8): 5083-5103. doi: 10.3934/era.2023260 |
[2] | Miao Luo, Yousong Chen, Dawei Gao, Lijun Wang . Inversion study of vehicle frontal collision and front bumper collision. Electronic Research Archive, 2023, 31(2): 776-792. doi: 10.3934/era.2023039 |
[3] | Miguel Ferreira, Luís Moreira, António Lopes . Differential drive kinematics and odometry for a mobile robot using TwinCAT. Electronic Research Archive, 2023, 31(4): 1789-1803. doi: 10.3934/era.2023092 |
[4] | Longchao Da, Hua Wei . CrowdGAIL: A spatiotemporal aware method for agent navigation. Electronic Research Archive, 2023, 31(2): 1134-1146. doi: 10.3934/era.2023057 |
[5] | Hyunju Shin, Jieun Ko, Gunwoo Lee, Cheol Oh . Evaluating the environmental impact on connected vehicles during freeway accidents using VISSIM with probe vehicle data. Electronic Research Archive, 2024, 32(4): 2955-2975. doi: 10.3934/era.2024135 |
[6] | Lingling Zhang . Vibration analysis and multi-state feedback control of maglev vehicle-guideway coupling system. Electronic Research Archive, 2022, 30(10): 3887-3901. doi: 10.3934/era.2022198 |
[7] | Zhen-Zhen Tao, Bing Sun . A feedback design for numerical solution to optimal control problems based on Hamilton-Jacobi-Bellman equation. Electronic Research Archive, 2021, 29(5): 3429-3447. doi: 10.3934/era.2021046 |
[8] | Shuang Zhang, Songwen Gu, Yucong Zhou, Lei Shi, Huilong Jin . Energy efficient resource allocation of IRS-Assisted UAV network. Electronic Research Archive, 2024, 32(7): 4753-4771. doi: 10.3934/era.2024217 |
[9] | Hao Li, Zhengwu Wang, Shuiwang Chen, Weiyao Xu, Lu Hu, Shuai Huang . Integrated optimization of planning and operation of a shared automated electric vehicle system considering the trip selection and opportunity cost. Electronic Research Archive, 2024, 32(1): 41-71. doi: 10.3934/era.2024003 |
[10] | Xiaoxiao Wang, Liangjie Xu . Factors influencing drivers' queue-jumping behavior at urban intersections: A covariance-based structural equation modeling analysis. Electronic Research Archive, 2024, 32(3): 1439-1470. doi: 10.3934/era.2024067 |
The rise in global ozone levels over the last few decades has harmed human health. This problem exists in several cities throughout South America due to dangerous levels of particulate matter in the air, particularly during the winter season, making it a public health issue. Lima, Peru, is one of the ten cities in South America with the worst levels of air pollution. Thus, efficient and precise modeling and forecasting are critical for ozone concentrations in Lima. The focus is on developing precise forecasting models to anticipate ozone concentrations, providing timely information for adequate public health protection and environmental management. This work used hourly O3 data in metropolitan areas for multi-step-ahead (one-, two-, three-, and seven-day-ahead) O3 forecasts. A multiple linear regression model was used to represent the deterministic portion, and four-time series models, autoregressive, nonparametric autoregressive, autoregressive moving average, and nonlinear neural network autoregressive, were used to describe the stochastic component. The various horizon out-of-sample forecast results for the considered data suggest that the proposed component-based forecasting technique gives a highly consistent, accurate, and efficient gain. This may be expanded to other districts of Lima, different regions of Peru, and even the global level to assess the efficacy of the proposed component-based modeling and forecasting approach. Finally, no analysis has been undertaken using a component-based estimation to forecast ozone concentrations in Lima in a multi-step-ahead manner.
Epidemics are widespread around the world, and always jeopardize public lives and health, such as COVID-19, malaria, severe acute respiratory syndrome (SARS), influenza and so on. The public has taken all kinds of measures to struggle against the different infectious epidemics. Various of mathematical models are established to research dynamics and influences of prevention and control for different epidemics [12,23,22,10,9,15]. Recently, both the popularization of knowledge about infectious diseases and the improvement of treatment have built a solid foundation for epidemics' prevention. This popularization can also lead to the strengthening in public self-protection. For instance, since Chinese had owned strongly self-protection and governments of China had taken efficient treatments, COVID-19 was first under effective control in China [11,17,13]. As a result, it is necessary to integrate self-protection and treatment into epidemic models. Because epidemics are always discovered at one location and then spread to other areas [21], reaction-diffusion models become essential to describe this spatial spread. Simultaneously, epidemic models with spatial spread usually can result in a development from a diseases-free state to an infective state, which can be predicted a wave for this evolution of epidemics. Consequently, traveling wave solution becomes critical to study the spatial spread of epidemics [7,8,18,20].
Many people have analyzed traveling wave solution and the asymptotic speed of propagation of classic compartmental epidemic models [16,26,1,4,30,28,32,25]. Ducrot and Magal [3] studied the existence and the non-existence of traveling wave solution satisfying a diffusive epidemic model with age-structure and constant recruitment, and constructed a suitable Lyapunov functional to discuss their asymptotic behavior at infinity. Li et al. [14] found that the existence and the non-existence of traveling wave solution for the system which is a nonlocal dispersal delayed SIR model with constant recruitment and Holling-slowromancapii@ incidence rate are determined via both the minimal wave speed and the basic reproduction number
In this paper, we firstly integrate self-protection into the classical reaction-diffusion SEIR model with constant recruitment, so susceptible individuals are divided into two groups: susceptible individuals
{∂S1(x,t)∂t=d1ΔS1(x, t)+Λ−βS1(x, t)[θI1(x, t)+I2(x, t)]−(ρ+μ)S1(x, t),∂S2(x, t)∂t=d2ΔS2(x, t)+ρS1(x, t)−βσS2(x, t)[θI1(x, t)+I2(x, t)]−μS2(x, t),∂E(x, t)∂t=D0ΔE(x, t)+β[S1(x, t)+σS2(x, t)][θI1(x, t)+I2(x, t)]−(μ+γ)E(x, t),∂I1(x, t)∂t=D1ΔI1(x, t)+q1γE(x, t)−(μ+α1)I1(x, t),∂I2(x, t)∂t=D2ΔI2(x, t)+q2γE(x, t)−(μ+α2)I2(x, t),∂R(x, t)∂t=DΔR(x, t)+α1I1(x, t)+α2I2(x, t)−μR(x, t). | (1) |
Throughout this aritcle, we make the following assumption:
In fact, for making a living, the susceptible has to move, and their diffusion is almost same without special cases. Especially, the susceptible with self-protection may take some methods, such as wearing masks, avoiding crowed public places and so on, to decrease the likelihood of connects with the infectious. The infectious could reduce going out owing to discomfort by disease. Thus, the assumption can better close to reality. In mathematical analysis of this model, the assumption (A) also can help us get an upper bound for the different susceptible and infected components to research the asymptotic behavior as
Since the equations
{∂S1(x, t)∂t=dΔS1(x, t)+Λ−βS1(x, t)[θI1(x, t)+I2(x, t)]−(ρ+μ)S1(x, t),∂S2(x, t)∂t=dΔS2(x, t)+ρS1(x, t)−βσS2(x, t)[θI1(x, t)+I2(x, t)]−μS2(x, t),∂E(x, t)∂t=D0ΔE(x, t)+β[S1(x, t)+σS2(x, t)][θI1(x, t)+I2(x, t)]−(μ+γ)E(x, t),∂I1(x, t)∂t=D1ΔI1(x, t)+q1γE(x, t)−(μ+α1)I1(x, t),∂I2(x, t)∂t=D2ΔI2(x, t)+q2γE(x, t)−(μ+α2)I2(x, t), | (2) |
where
The structure of this article is as follows. In Section 2, we firstly obtain the basic reproduction number
In order to research traveling wave solution of system (2), the first step is to find what kind of constant equilibriums of system (2) exist. It is well known that there always exists an equilibrium
{dS1(t)dt=Λ−βS1(t)[θI1(t)+I2(t)]−(ρ+μ)S1(t),dS2(t)dt=ρS1(t)−βσS2(t)[θI1(t)+I2(t)]−μS2(t),dE(t)dt=β[S1(t)+σS2(t)][θI1(t)+I2(t)]−(μ+γ)E(t),dI1(t)dt=q1γE(t)−(μ+α1)I1(t),dI2(t)dt=q2γE(t)−(μ+α2)I2(t). | (3) |
The point
R0=[q1γθβ(μ+γ)(μ+α1)+q2γβ(μ+γ)(μ+α2)](S01+σS02). |
Furthermore, taking advantage of the direct Lyapunov functional method, which is similar in [15], can claim the below theorem.
Theorem 2.1. If
In the rest of Section 2, we always assume
(S1(ξ), S2(ξ), E(ξ), I1(ξ), I2(ξ)),ξ=x+ct∈R. | (4) |
Substitute formula (4) into systmen (2), and we can obtain the wave form equations as follows:
{dS″1(ξ)−cS′1(ξ)+Λ−βS1(ξ)[θI1(ξ)+I2(ξ)]−(ρ+μ)S1(ξ)=0,dS″2(ξ)−cS′2(ξ)+ρS1(ξ)−βσS2(ξ)[θI1(ξ)+I2(ξ)]−μS2(ξ)=0,D0E″(ξ)−cE′(ξ)+β[θI1(ξ)+I2(ξ)][S1(ξ)+σS2(ξ)]−(μ+γ)E(ξ)=0,D1I″1(ξ)−cI′1(ξ)+q1γE(ξ)−(μ+α1)I1(ξ)=0,D2I″2(ξ)−cI′2(ξ)+q2γE(ξ)−(μ+α2)I2(ξ)=0, | (5) |
for
Si(−∞)=S0i, E(−∞)=0, Ii(−∞)=0,Si(+∞)=S∗i, E(+∞)=E∗, Ii(+∞)=I∗i,i=1, 2. | (6) |
Linearizing the
{D0E″(ξ)−cE′(ξ)+β[θI1(ξ)+I2(ξ)](S01+σS02)−(μ+γ)E(ξ)=0,D1I″1(ξ)−cI′1(ξ)+q1γE(ξ)−(μ+α1)I1(ξ)=0,D2I″2(ξ)−cI′2(ξ)+q2γE(ξ)−(μ+α2)I2(ξ)=0. |
Set
{D0η0λ2−cη0λ+β(θη1+η2)(S01+σS02)−(μ+γ)η0=0,D1η1λ2−cη1λ+q1γη0−(μ+α1)η1=0,D2η2λ2−cη2λ+q2γη0−(μ+α2)η2=0. | (7) |
Let
˜A=(D0000D1000D2),˜B=(c000c000c), |
˜V=(μ+γ000μ+α1000μ+α2), |
˜F=(0βθ(S01+σS02)β(S01+σS02)q1γ00q2γ00). |
Denote
Θ(λ, c)(η0, η1, η2)T=0. |
And define
(−Aλ2+Bλ+I)−1Fη=η, | (8) |
and
(−Aλ2+Bλ+I)−1F=(0βθ(S01+σS02)m0(λ, c)β(S01+σS02)m0(λ, c)q1γm1(λ, c)00q2γm2(λ, c)00), |
where
M(λ, c)η=η. |
Let
λc=min{c+√c2−4D0(μ+γ)2D0, c+√c2−4D1(μ+α1)2D1,c+√c2−4D2(μ+α2)2D2}. |
For
ρ(λ, c)=[(βθq1γm0(λ, c)m1(λ, c)+βq2γm0(λ, c)m2(λ, c))(S01+σS02)]12. | (9) |
Moreover, some properties of
Lemma 2.2. Below statements hold:
(ⅰ)
(ⅱ)
(ⅲ) for
Proof. It is well known that (ⅰ) is established, so we only prove (ⅱ) and (ⅲ). It follows from the definitions of both
ddλρ(λ, 0)=ρ−12(λ, 0)(βθq1γ(S01+σS02)(D0λm1(λ, 0)+D1λm0(λ, 0))m20(λ, 0)m21(λ, 0)+βq2γ(S01+σS02)(D0λm2(λ, 0)+D2λm0(λ, 0))m20(λ, 0)m22(λ, 0))>0 |
for
∂∂cρ(λ, c)=−12λρ−12(λ, c)(βθq1γ(S01+σS02)m20(λ, c)m21(λ, c)+βq2γ(S01+σS02)m20(λ, c)m22(λ, c))<0, |
for
According to Lemma 2.2, we define
ˆλ(c)=minλ∈[0, λc)ρ(λ, c),∀c≥0. |
Then we conclude
λ∗=inf{λ∈[0, λc∗): ρ(λ, c∗)=1}, |
it indicates that
λ1(c)=sup{λ∈(0, λ∗): ρ(λ, c)=1, ρ(λ′, c)≥1, ∀λ′∈(0, λ)}. |
Because of
Lemma 2.3. Assume that
(ⅰ)
(ⅱ)
(ⅲ) as
Since the
Lemma 2.4. Assume that
M(λ1(c), c)η(c)=η(c), |
M(λ1(c)+εn(c), c)ζn(c)=ρ(λ1(c)+εn(c), c)ζn(c). |
Fix
{−m0(λ1, c)η0+(S01+σS02)(θη1+η2)=0,−m1(λ1, c)η1+q1γη0=0,−m2(λ1, c)η2+q2γη0=0, | (10) |
and
{−m0(λ1+εn, c)ζn0+(S01+σS02)(θζn1+ζn2)<0,−m1(λ1+εn, c)ζn1+q1γζn0<0,−m2(λ1+εn, c)ζn2+q2γζn0<0. | (11) |
According to the above argument, we can construct suitable sub- and super-solutions which are defined in below lemmas. And then the local existence of solution for system (5) is proved via the Schauder fixed point theorem.
Lemma 2.5. Let the vector function
{D0p″0(ξ)−cp′0(ξ)+β(S01+σS02)(θp1(ξ)+p2(ξ))−(μ+γ)p0(ξ)=0,D1p″1(ξ)−cp′1(ξ)+q1γp0(ξ)−(μ+α1)p1(ξ)=0,D2p″2(ξ)−cp′2(ξ)+q2γp0(ξ)−(μ+α2)p2(ξ)=0. |
Lemma 2.6. For each
cS−′1(ξ)≤dS−″1(ξ)+Λ−(μ+ρ)S−1(ξ)−βS−1(ξ)(θp1(ξ)+p2(ξ)), | (12) |
cS−′2(ξ)≤dS−″2(ξ)+ρS−1(ξ)−μS−2(ξ)−βσS−2(ξ)(θp1(ξ)+p2(ξ)), | (13) |
for
Proof. When
(−dω+c)S01ωMeωξ+Λ−(μ+ρ)S01(1−Meωξ)−β(θη1+η2)S01(1−Meωξ)eλ1ξ=(−dω+c)S01ωMeωξ+(μ+ρ)S01Meωξ−β(θη1+η2)S01(1−Meωξ)eλ1ξ≥[(−dω+c)S01ωM+(μ+ρ)S01M−β(θη1+η2)S01e−λ1−ωωlnM]eωξ≥0, |
so the inequality (12) is established. Furthermore,
(−dω+c)S02ωMeωξ+ρS01(1−Meωξ)−βσ(θη1+η2)S02(1−Meωξ)eλ1ξ−μS02(1−Meωξ)=(−dω+c)S02ωMeωξ−βσ(θη1+η2)S02(1−Meωξ)eλ1ξ≥[(−dω+c)S01ωM−βσ(θη1+η2)S01e−λ1−ωωlnM]eωξ≥0, |
and the inequality (13) is set up. The lemma is completely proved.
Lemma 2.7. Let
min{1ϵlnKζ0η0, 1ϵlnKζ1η1, 1ϵlnKζ2η2}>1ωlnM, |
then the vector map
ch′0(ξ)≤D0h″0(ξ)−(μ+γ)h0(ξ)+β(S−1(ξ)+σS−2(ξ))(θh1(ξ)+h2(ξ)),ξ<1ϵlnη0Kζ0, | (14) |
ch′1(ξ)≤D1h″1(ξ)+q1γh0(ξ)−(μ+α1)h1(ξ), ξ<1ϵlnη1Kζ1, | (15) |
ch′2(ξ)≤D2h″2(ξ)+q2γh0(ξ)−(μ+α2)h2(ξ), ξ<1ϵlnη2Kζ2. | (16) |
Proof. Firstly, we prove the inequality (14). For
K[−D0ζ0(λ1+ϵ)2+cζ0(λ1+ϵ)+(μ+γ)ζ0]e(λ1+ϵ)ξ+β(S−1+σS−2)[θh1(ξ)+h2(ξ)]+D0η0λ21eλ1ξ−cη0λ1eλ1ξ−(μ+γ)η0eλ1ξ≥0, | (17) |
which is
−Kζ0m0(λ1+ϵ, c)e(λ1+ϵ)ξ+η0m0(λ1, c)eλ1ξ−β(S−1+σS−2)[θh1(ξ)+h2(ξ)]≤0. |
According to equations (10), the proof of the inequality (17) can be replaced by proving
−Kζ0m0(λ1+ϵ, c)e(λ1+ϵ)ξ+β(S01+σS02)(θη1+η2)eλ1ξ−β(S−1+σS−2)[θh1(ξ)+h2(ξ)]≤0. | (18) |
Because
β(S01+σS02)(θη1+η2)eλ1ξ−β(S−1+σS−2)[θh1(ξ)+h2(ξ)]=β(S01+σS02)(θη1+η2)eλ1ξ−β(S01+σS02)[θh1(ξ)+h2(ξ)]+β(S01+σS02)[θh1(ξ)+h2(ξ)]−β(S−1+σS−2)[θh1(ξ)+h2(ξ)]=β(S01+σS02)[θ(η1eλ1ξ−h1(ξ))+(η2eλ1ξ)−h2(ξ)]+β[θh1(ξ)+h2(ξ)][(S01−S−1)+σ(S02−S−2)]≤β(S01+σS02)(θKζ1e(λ1+ω)ξ+Kζ2e(λ1+ω)ξ)+β[θh1(ξ)+h2(ξ)](S01Meωξ+σS02Meωξ). |
Thus, for the proof of the inequality (18), we only need to prove
Ke(λ1+ϵ)ξ[−m0(λ1+ϵ)ζ0+β(S01+σS02)(θζ1+ζ2)]+Mβ(S01+σS02)[θh1(ξ)+h2(ξ)]eωξ≤0, |
which is
K[−m0(λ1+ϵ)ζ0+β(S01+σS02)(θζ1+ζ2)]+Mβ(S01+σS02)[θη1(ξ)+η2(ξ)]e(ω−ϵ)ξ≤0. | (19) |
For
Now, we set
ΓX={χ1(⋅), χ2(⋅), φ0(⋅), φ1(⋅), φ2(⋅)∈C([−X, X], R5)|χi(±X)=S−i(±X),φj(±X)=hj(±X),S−i(ξ)≤χi(ξ)≤S0i,h−j(ξ)≤φj(ξ)≤pj(ξ),} |
where
For any
(χ1(⋅), χ2(⋅), φ0(⋅), φ1(⋅), φ2(⋅))∈ΓX, |
we consider the following boundary-value problem for
{−dS″1,X(ξ)+cS′1,X(ξ)−Λ+(μ+ρ)S1,X(ξ)+βS1,X(ξ)[θφ1(ξ)+φ2(ξ)]=0,−dS″2,X(ξ)+cS′2,X(ξ)−ρχ1(ξ)+μS2,X(ξ)+βσS2,X(ξ)[θφ1(ξ)+φ2(ξ)]=0,−D0E″X(ξ)+cE′X(ξ)−β[χ1(ξ)+σχ2(ξ)][θφ1(ξ)+φ2(ξ)]+(μ+γ)EX(ξ)=0,−D1I″1,X(ξ)+cI′1,X(ξ)−q1γφ0(ξ)+(μ+α1)I1,X(ξ)=0,−D2I″2,X(ξ)+cI′2,X(ξ)−q2γφ0(ξ)+(μ+α2)I2,X(ξ)=0, | (20) |
satisfying the below boundary condition:
S1,X(±X)=S−1(±X), S2,X(±X)=S−2(±X),EX(±X)=h0(±X), I1,X(±X)=h1(±X), I2,X(±X)=h2(±X). | (21) |
Applying the Gilbarg and Trudinger's Corollary 9.18 in [6], we can claim that there exists a unique solution
(S1,X, S2,X, EX, I1,X, I2,X), |
satisfying the problems (20)-(21), where
(S1,X, S2,X, EX, I1,X, I2,X)∈W2,p((−X, X), R5)∩C([−X, X], R5), |
for
S1,X=T1(χ1, χ2, φ0, φ1, φ2),S2,X=T2(χ1, χ2, φ0, φ1, φ2),EX=T3(χ1, χ2, φ0, φ1, φ2),I1,X=T4(χ1, χ2, φ0, φ1, φ2),I1,X=T5(χ1, χ2, φ0, φ1, φ2), |
for any
Lemma 2.8. The operator
Proof. Firstly, we consider
0≥−dS−″1(ξ)+cS−′1(ξ)−Λ+(μ+ρ)S−1(ξ)+βS−1(ξ)(θp1(ξ)+p2(ξ))≥−dS−″1(ξ)+cS−′1(ξ)−Λ+(μ+ρ)S−1(ξ)+βS−1(ξ)(θφ1(ξ)+φ2(ξ)), |
and
0≥−dS−″2(ξ)+cS−′2(ξ)−ρS−1(ξ)+μS−2(ξ)+βσS−2(ξ)(θp1(ξ)+p2(ξ))≥−dS−″2(ξ)+cS−′2(ξ)−ρχ1(ξ)+μS−2(ξ)+βσS−2(ξ)(θφ1(ξ)+φ2(ξ)). |
for any
Secondly, we consider about
0=−D0p″0(ξ)+cp′0(ξ)−β(S01+σS02)(θp1(ξ)+p2(ξ))+(μ+γ)p0(ξ)≤−D0p″0(ξ)+cp′0(ξ)−β(S01+σS02)(θφ1(ξ)+φ2(ξ))+(μ+γ)p0(ξ),0=−D1p″1(ξ)+cp′1(ξ)−q1γp0(ξ)+(μ+α1)p1(ξ)≤−D1p″1(ξ)+cp′1(ξ)−q1γφ0(ξ)+(μ+α1)p1(ξ), |
and
0=−D2p″2(ξ)+cp′2(ξ)−q2γp0(ξ)+(μ+α2)p2(ξ)≤−D2p″2(ξ)+cp′2(ξ)−q2γφ0(ξ)+(μ+α2)p2(ξ), |
for any
0≥−D0h″0(ξ)+ch′0(ξ)+(μ+γ)h0(ξ)−β(S−1(ξ)+σS−2)(ξ)(θh1(ξ)+h2(ξ))≥−D0h″0(ξ)+ch′0(ξ)+(μ+γ)h0(ξ)−β(χ−1(ξ)+σχ−2)(ξ)(θφ1(ξ)+φ2(ξ)), |
for
0≥−D1h″1(ξ)+ch′1(ξ)−q1γh0(ξ)+(μ+α1)h1(ξ)≥−D1h″1(ξ)+ch′1(ξ)−q1γφ0(ξ)+(μ+α1)h1(ξ), |
for
0≥−D2h″2(ξ)+ch′2(ξ)−q2γh0(ξ)+(μ+α2)h2(ξ)≥−D2h″2(ξ)+ch′2(ξ)−q2γφ0(ξ)+(μ+α2)h2(ξ), |
for
By taking advantage of the classic embedding theorem,
(S1,X, S2,X, EX, I1,X, I2,X)=T(S1,X, S2,X, EX, I1,X, I2,X), |
for
{−dS″1,X(ξ)+cS′1,X(ξ)−Λ+(μ+ρ)S1,X(ξ)+βS1,X(ξ)[θI1,X(ξ)+I2,X(ξ)]=0,−dS″2,X(ξ)+cS′2,X(ξ)−ρS1,X(ξ)+μS2,X(ξ)+βσS2,X(ξ)[θI1,X(ξ)+I2,X(ξ)]=0,−D0E″X(ξ)+cE′X(ξ)−β[S1,X(ξ)+σS2,X(ξ)][θI1,X(ξ)+I2,X(ξ)]+(μ+γ)EX(ξ)=0,−D1I″1,X(ξ)+cI′1,X(ξ)−q1γEX(ξ)+(μ+α1)I1,X(ξ)=0,−D2I″2,X(ξ)+cI′2,X(ξ)−q2γEX(ξ)+(μ+α2)I2,X(ξ)=0,Si,X(±X)=S−i(±X), EX(±X)=h0(±X), Ii,X(±X)=hi(±X). | (22) |
We have proved the local existence of solution for system (5). In order to obtain the global existence, we need the following estimate.
Lemma 2.9. For a given
∥Si,X∥C3[−Y, Y]≤NSi(Y), ∥EX∥C3[−Y, Y]≤NE(Y), ∥Ii,X∥C3[−Y, Y]≤NIi(Y), | (23) |
with
X>max{Y, 1ωln1M, 1ϵlnη0Kζ0, 1ϵlnη1Kζ1, 1ϵlnη2Kζ2 }. |
Proof. We always set
∥S1,X∥W2,p(−Y,Y)≤Ω1(Λ+βS01[θ^NI1(Y)+^NI2(Y)]+∥ϕ1∥W2,p(−Y,Y)), |
and
∥S2,X∥W2,p(−Y,Y)≤Ω2(ρS01+βσS02[θ^NI1(Y)+^NI2(Y)]+∥ϕ2∥W2,p(−Y,Y)), |
where
Set a sequence of positive numbers
S−1(ξ)≤S1(ξ)≤S01, S−2(ξ)≤S2(ξ)≤S02,h0(ξ)≤E(ξ)≤p0(ξ), h1(ξ)≤I1(ξ)≤p1(ξ), h2(ξ)≤I2(ξ)≤p2(ξ) | (24) |
with
limξ→−∞S1(ξ)=S01, limξ→−∞S2(ξ)=S02, limξ→−∞E(ξ)=0, |
limξ→−∞I1(ξ)=0, limξ→−∞I2(ξ)=0. |
Now, we need to show some estimates about solution
Lemma 2.10. Let
0≤E(ξ)+I1(ξ)+I2(ξ)≤√dDminΛr | (25) |
and
Λμ+ρ+β√dDminΛr≤S1(ξ)≤S01,ρΛ(μ+ρ+β√dDminΛr)(μ+βσ√dDminΛr)≤S2(ξ)≤S02, | (26) |
where
Proof. In this proof, we still set
m1(ξ)=ρS1(ξ)+βS1(ξ)[θI1(ξ)+I2(ξ)], m2(ξ)=ρS1(ξ)−βσS2(ξ)[θI1(ξ)+I2(ξ)], |
and
n1(ξ)=q1γE(ξ), n2(ξ)=q1γE(ξ). |
Due to the define of
{−dS″1(ξ)+cS′1(ξ)+rS1(ξ)≤Λ−m1(ξ),−dS″2(ξ)+cS′2(ξ)+rS2(ξ)≤m2(xi),−D0E″(ξ)+cE′(ξ)+rE(ξ)≤m1(ξ)−m2(ξ)−n1(ξ)−n2(ξ),−D1I″1(ξ)+cI′1(ξ)+rI1(ξ)≤n1(ξ),−D2I″2(ξ)+cI′2(ξ)+rI2(ξ)≤n2(ξ). ∀ξ∈R, | (27) |
So we need to consider the following Cauchy problems
{∂∂tu1(t, ξ)−d∂∂ξ2u1(t, ξ)+c∂∂ξu1(t, ξ)+ru1(t, ξ)=Λ−m1(ξ),u1(0, ξ)=S1(ξ),{∂∂tu2(t, ξ)−d∂∂ξ2u2(t, ξ)+c∂∂ξu2(t, ξ)+ru2(t, ξ)=m2(ξ),u2(0, ξ)=S2(ξ),{∂∂tv0(t, ξ)−d∂∂ξ2v0(t, ξ)+c∂∂ξv0(t, ξ)+rv0(t, ξ)=m1(ξ)−m2(ξ)−n1(ξ)−n2(ξ),v0(0, ξ)=E(ξ),{∂∂tvi(t, ξ)−d∂∂ξ2vi(t, ξ)+c∂∂ξvi(t, ξ)+rvi(t, ξ)=ni(ξ),vi(0, ξ)=Ii(ξ), |
for
u1(t, ξ)=e−rt∫R1√4πdte−(ξ−ct−y)24dtS1(y)dy+∫t0∫Re−rs√4πdse−(ξ−ct−y)24ds(Λ−m1(y))dyds,u2(t, ξ)=e−rt∫R1√4πdte−(ξ−ct−y)24dtS2(y)dy+∫t0∫Re−rs√4πdse−(ξ−ct−y)24dsm2(y)dyds,v0(t, ξ)=e−rt∫R1√4πD0te−(ξ−ct−y)24D0tE(y)dy+∫t0∫Re−rs√4πD0se−(ξ−ct−y)24D0s(m1(y)−m2(y)−n(y)−n(y))dyds,vi(t, ξ)=e−rt∫R1√4πDite−(ξ−ct−y)24DitIi(y)dy+∫t0∫Re−rs√4πDise−(ξ−ct−y)24Disni(y)dyds, |
for
Si(ξ)≤ui(t, ξ), E(ξ)≤v0(t, ξ), Ii(ξ)≤vi(t, ξ), ∀t>0, ∀ξ∈R. |
Let
S1(ξ)≤Λr−f1(ξ), S2(ξ)≤f2(ξ), E(ξ)≤f0(ξ)−g0(ξ), Ii(ξ)≤gi(ξ), |
for
fi(ξ)=∫+∞0e−−rt√4πdt∫+∞−∞mi(ξ−y−ct)e−y24dtdydt,f0(ξ)=∫+∞0e−−rt√4πD0t∫+∞−∞[m1(ξ−y−ct)−m2(ξ−y−ct)]e−y24D0tdydt,g0(ξ)=∫+∞0e−−rt√4πD0t∫+∞−∞[n1(ξ−y−ct)+n2(ξ−y−ct)]e−y24D0tdydt,gi(ξ)=∫+∞0e−−rt√4πDit∫+∞−∞ni(ξ−y−ct)e−y24Ditdydt. |
Owing to
√D0g0(ξ)≥√D1g1(ξ)+√D2g2(ξ) |
and
√d[f1(ξ)−f2(ξ)]≥√D0f0(ξ) |
for any
E(ξ)+√D1D0I1(ξ)+√D2D0I2(ξ)≤f0(ξ)≤√dD0f1(ξ)−√dD0f2(ξ)≤√dD0[λr−S1(ξ)]−√dD0S2(ξ)≤√dD0Λr, |
which implies
E(ξ)+I1(ξ)+I2(ξ)≤√D0DminE(ξ)+√D1DminI1(ξ)+√D2DminI2(ξ)≤√dDminΛr, |
for
On the other hand, one has
dS″1(ξ)−cS′1(ξ)+Λ−βS1(ξ)√dDminΛr−(μ+ρ)S1(ξ). |
for any
Λμ+ρ+β√dDminΛr≤S1(ξ), ∀ξ∈R. |
Via the similar argument on
ρΛ(μ+ρ+β√dDminΛr)(μ+βσ√dDminΛr)≤S2(ξ), ∀ξ∈R. |
This lemma is completely proved.
Since the system consisted by
max{max[ξ−1,ξ+1]E, max[ξ−1,ξ+1]I1, max[ξ−1,ξ+1]I2}≤M1min{min[ξ−1,ξ+1]E, min[ξ−1,ξ+1]I1, min[ξ−1,ξ+1]I2}. | (28) |
Furthermore, there exists a constant
|E′(ξ)E(ξ)|+|I′1(ξ)I1(ξ)|+|I′2(ξ)I2(ξ)|≤ˆM, | (29) |
for
max{∥E∥W2,p(ξ−12,ξ+12), ∥I1∥W2,p(ξ−12,ξ+12), ∥I2∥W2,p(ξ−12,ξ+12)}≤M2{∥E∥Lp(ξ−1,ξ+1)+∥I1∥Lp(ξ−1,ξ+1)+∥I2∥Lp(ξ−1,ξ+1)}≤6M2max{max[ξ−1,ξ+1]E, max[ξ−1,ξ+1]I1, max[ξ−1,ξ+1]I2}, |
for any
max{∥E′∥C[x−12,x+12], ∥I′1∥C[x−12,x+12], ∥I′2∥C[x−12,x+12]}≤M3max{max[x−1,x+1]E, max[x−1,x+1]I1, max[x−1,x+1]I2}.∀ξ∈R. | (30) |
Set
In order to take advantage of a suitable Lyapunov functional to research the asymptotic behavior of
ˆE={S1(⋅), S2(⋅), E(⋅), I1(⋅), I2(⋅)∈C1(R, (0, +∞))×⋯×C1(R, (0, +∞)),S1(⋅)>0, S2(⋅)>0, E(⋅)>0, I1(⋅)>0, I2(⋅)>0,∃ˆM>0, |E′(ξ)E(ξ)|+|I′1(ξ)I1(ξ)|+|I′2(ξ)I2(ξ)|≤ˆM.}, |
Let
V(S1, S2, E, I1, I2)(ξ)=S∗1[dS′1(1S1(ξ)−1S∗1)+cg(S1(ξ)S∗1)]+S∗2[dS′2(1S2(ξ)−1S∗2)+cg(S2(ξ)S∗2)]+E∗[D0E′(1E(ξ)−1E∗)+cg(E(ξ)E∗)]+C1I∗1[D1I′1(1I1(ξ)−1I∗1)+cg(I1(ξ)I∗1)]+C2I∗2[D2I′2(1I2(ξ)−1I∗2)+cg(I2(ξ)I∗2)], | (31) |
where
C1=βθI∗1(S∗1+σS∗2)q1γE∗,C2=βI∗2(S∗1+σS∗2)q2γE∗, |
for each
Lemma 2.11. Let (A) be satisfied and
1N≤Si(ξ)≤S∗i, | (32) |
E(ξ)≤NE∗, | (33) |
Ii(ξ)≤NI∗i, | (34) |
and
|E′(ξ)E(ξ)|+|I′1(ξ)I1(ξ)|+|I′2(ξ)I2(ξ)|≤N | (35) |
for any
−m≤V(ξ)<+∞,∀ξ∈R, | (36) |
where the map
Proof. The previous description has shown
|d2∑i=1S∗iS′i(ξ)(1Si(ξ)−1S∗i)+D0E∗E′(ξ)(1E(ξ)−1E∗)+2∑i=1CiI∗iDiI′i(xi)(1Ii(ξ)−1I∗i)|≤d2∑i=1S∗i∥Si∥∞(N+1S∗i)+ˆDˆCN+ˆDˆC(|E′(ξ)E∗|+|I′1(ξ)I∗1|+|I′2(ξ)I∗2|)≤d2∑i=1S∗i∥Si∥∞(N+1S∗i)+ˆDˆCN+ˆDˆCN2, | (37) |
where
Φ(ξ)=cg(S1(ξ)S∗1)+cg(S2(ξ)S∗2)+cg(E(ξ)E∗)+cC1g(I1(ξ)I∗1)+cC2g(I2(ξ)I∗2). | (38) |
According to both the definition of
Since a direct calculation with letting
dV(ξ)dξ=(Λ−βθS∗1I∗1−βS∗1I∗2−ρS∗1)(2−x1−1x1)+(ρS∗1−βσθS∗2I∗1−βσS∗2I∗2)(3−1x1−x2−x1x2)+βθS∗1I∗1(3−1x1−x1x4x3−x3x4)+βS∗1I∗2(3−1x1−x1x5x3−x3x5)+βσθS∗2I∗1(4−1x1−x1x2−x2x4x3−x3x4)+βσS∗2I∗2(4−1x1−x1x2−x2x5x3−x3x5). |
Therefore, via the mean inequality, we conclude that
dV(ξ)dξ≤0,∀ξ∈R, |
which implies that the map
dV(ξ)dξ=0,∀ξ∈R, |
the map
S1(ξ)≡S∗1, S2(ξ)≡S∗2, E(ξ)≡E∗, I1(ξ)≡I∗1, I2(ξ)≡I∗2,∀ξ∈R. |
This completes the proof.
Now, we gain the first theorem for existence of traveling wave solution for system (2) as below:
Theorem 2.12. If (A) and
Proof. We still set
(S1(ξ), S2(ξ), E(ξ), I1(ξ), I2(ξ)) |
satisfying system (5), and
limξ→−∞S1(ξ)=S01, limξ→−∞S2(ξ)=S02, limξ→−∞E(ξ)=0,limξ→−∞I1(ξ)=0, limξ→−∞I2(ξ)=0, |
for any
limξ→+∞S1(ξ)=S∗1, limξ→+∞S2(ξ)=S∗2, limξ→+∞E(ξ)=E∗,limξ→+∞I1(ξ)=I∗1, limξ→+∞I2(ξ)=I∗2, |
Take an arbitrary increasing sequence
Si,m(ξ)=Si(ξ+ξm), Em(ξ)=E(ξ+ξm), Ii,m(ξ)=Ii(ξ+ξm). |
Via the elliptic estimate, it may assume that the sequence
V(S1,m, S2,m, Em, I1,m, I2,m)(ξ)≤V(S1, S2, Em, I1, I2)(ξ) |
for any
limm→+∞V(S1,m, S2,m, Em, I1,m, I2,m)(ξ)=ˆG,∀ξ∈R, |
which implies that
V(S1,∞, S2,∞, E∞, I1,∞, I2,∞)(ξ)≡ˆG |
in
Si,∞=S∗i, E∞=E∗, Ii,∞=I∗i,S′i,∞=0, E′∞=0, I′i,∞=0. |
Via the arbitrariness of the sequence
limξ→+∞Si(ξ)=S∗i, limξ→+∞E(ξ)=E∗, limξ→+∞Ii(ξ)=I∗i. |
The proof is completed.
Furthermore, the second theorem of existence for traveling wave solution for system (2) is stated as below:
Theorem 2.13. Assume that (A) is satisfied and
Proof. Step 1. Take a decreasing sequence
S1,m(0)=S01+S∗12. |
The interior elliptic estimates, Arzela-Ascoli theorem and a diagonalization argument can indicate a subsequence of
(S1,m, S2,m, Em, I1,m. I2,m)→(S1, S2, E, I1. I2) |
as
S1(0)=S01+S∗12, | (39) |
which implies that
(S_{1},\ S_{2},\ E,\ I_{1}.\ I_{2})\not\equiv(S_{1}^0,\ S_{2}^0,\ 0,\ 0,\ 0). |
Moreover, we yield
\begin{equation*} S_i(+\infty) = S_i^*,\ E(+\infty) = E^*,\ I_i(+\infty) = I_i^*,\ i = 1,\ 2. \end{equation*} |
Next, we need to prove what the solution converges to as
\begin{equation} \lim\limits_{\xi\rightarrow-\infty}V(\xi) = L < +\infty, \end{equation} | (40) |
or
\begin{equation} \lim\limits_{\xi\rightarrow-\infty}V(\xi) = +\infty. \end{equation} | (41) |
If the formula (40) holds, via the similar way in Lemma 2.11, it can claim that
\begin{equation*} S_i(-\infty) = S_i^*,E(-\infty) = E^*,\ I_i(-\infty) = I^*,\ i = 1,\ 2, \end{equation*} |
and then
\begin{equation*} S_i(\xi)\equiv S_i^*,\ E(\xi)\equiv E^*,\ I_i(\xi)\equiv I_i^*,\ \forall\xi\in\mathbb{R},\ i = 1,\ 2, \end{equation*} |
which contradicts the equation (39). Thus, the equation (41) must be only workable. Due to the inequality (37), it is shown that
\begin{equation} \lim\limits_{\xi\rightarrow-\infty}\Phi(\xi) = +\infty, \end{equation} | (42) |
where
\begin{equation} \lim\limits_{\xi\rightarrow-\infty}\inf E(\xi) = 0. \end{equation} | (43) |
On the contrary, if
I_i(\xi) > \hat\delta,\quad\forall\xi\in\mathbb{R}, |
which indicates that
\begin{equation*} \lim\limits_{\xi\rightarrow-\infty}\Phi(\xi) < +\infty, \end{equation*} |
and contradicts the equation (42). Therefore, the equation (43) holds. Secondly, we prove that
\lim\limits_{\xi\rightarrow-\infty}E(\xi) = 0. |
If
\begin{equation*} \lim\limits_{\xi\rightarrow-\infty}\sup E(\xi) = \delta > 0, \end{equation*} |
there exists a sequence
\begin{equation*} \lim\limits_{j\rightarrow\infty}E(\xi_j) = \delta, \end{equation*} |
Via the estimate (28), we can have
I_i(\xi_j)\leq\frac{1}{2M_1}\delta,\quad i = 1,\ 2. |
Then, it is implied that
\begin{equation*} \lim\limits_{j\rightarrow\infty}\sup V(\xi_j) < +\infty, \end{equation*} |
which contradicts the equation (41). Therefore,
\begin{equation*} \lim\limits_{\xi\rightarrow-\infty}E(\xi) = 0. \end{equation*} |
In the similar way, we can also claim that
\begin{equation*} \lim\limits_{\xi\rightarrow-\infty}I_i(\xi) = 0. \end{equation*} |
Finally, set
\begin{equation*} \lim\limits_{\xi\rightarrow-\infty}S_i(\xi) = S_i^0 \end{equation*} |
In order to hit the target, we firstly prove the existence of
\begin{equation*} \lim\limits_{\xi\rightarrow-\infty}\inf\{S_1(\xi)+S_2(\xi)\} < \lim\limits_{\xi\rightarrow-\infty}\sup\{S_1(\xi)+S_2(\xi)\}\leq \frac{\Lambda}{\mu}. \end{equation*} |
Take a sequence
\begin{align} &\lim\limits_{n\rightarrow+\infty}[S_1(\xi_n)+S_2(\xi_n)] = \lim\limits_{\xi\rightarrow-\infty}\inf\{S_1(\xi)+S_2(\xi)\} < \frac{\Lambda}{\mu},\\ &\frac{\mathrm{d}}{\mathrm{d}\xi_n}[S_1(\xi_n)+S_2(\xi_n)] = 0,\ \frac{\mathrm{d}^2}{\mathrm{d}\xi_n^2}[S_1(\xi_n)+S_2(\xi_n)]\leq0. \end{align} | (44) |
Since
\begin{equation*} \lim\limits_{n\rightarrow+\infty}[S_1(\xi_n)+S_2(\xi_n)]\geq\frac{\Lambda}{\mu}, \end{equation*} |
which leads to a contradiction to inequalities (44). Thus,
Step 2. This step is to prove
\begin{equation*} -dS_1^{''}(\xi)+cS_1^{'}(\xi)+(\mu+\rho)S_1(\xi) = \Lambda-\beta S_1(\xi)[\theta I_1(\xi)+I_2(\xi)],\quad\xi\in\mathbb{R}, \end{equation*} |
we can obtain that
\begin{align*} S_1(\xi) = &\frac{1}{z}\int_{-\infty}^{\xi}e^{A_1(\xi-x)}[\lambda-\beta S_1(x)(\theta I_1(x)+I_2(x))]\mathrm{d}x\\ &+\frac{1}{z}\int_{\xi}^{+\infty}e^{A_2(\xi-x)}[\lambda-\beta S_1(x)(\theta I_1(x)+I_2(x))]\mathrm{d}x\\ = &\frac{1}{z}\int_{-\infty}^{0}e^{A_1x}[\lambda-\beta S_1(\xi-x)(\theta I_1(\xi-x)+I_2(\xi-x))]\mathrm{d}x\nonumber\\ &+\frac{1}{z}\int_{0}^{+\infty}e^{A_2x}[\lambda-\beta S_1(\xi-x)(\theta I_1(\xi-x)+I_2(\xi-x))]\mathrm{d}x, \end{align*} |
where
\begin{equation*} z = d(A_1-A_2),\ A_1 = \frac{c-\sqrt{c^2+4d(\mu+\rho)}}{2},\ A_2 = \frac{c-\sqrt{c^2+4d(\mu+\rho)}}{2}. \end{equation*} |
As
\begin{equation*} k_1 = \frac{\Lambda}{z}\left(\int_{-\infty}^{0}s^{A_1x}\mathrm{d}x+\int_{0}^{+\infty}s^{A_2x}\mathrm{d}x\right), \end{equation*} |
which leads to
k_1 = \frac{\Lambda}{\mu+\rho} = S_1^0. |
Via the similar argument on
k_2 = \frac{\rho k_1}{\mu} = S_2^0. |
This completes the proof.
The previous section has stated the existence of traveling wave solution connecting disease-free equilibrium and endemic equilibrium when
Theorem 3.1. If
Proof. On the contrary, assume that there exists a solution
\begin{equation*} D_iI_i^{''}(\xi)-cI_i^{'}(\xi)+q_i\gamma E_{\sup}-(\mu+\alpha_i)I_i(\xi)\geq0,\quad\forall\xi\in\mathbb{R}. \end{equation*} |
It follows from the comparison principle that
\begin{equation*} I_i(\xi)\leq\frac{q_i\gamma E_{\sup}}{\mu+\alpha_i},\quad\forall\xi\in\mathbb{R}. \end{equation*} |
Next we research the following equation
\begin{equation*} D_0\bar{E}^{''}(\xi)-c\bar{E}^{'}(\xi)+\bar{\Lambda}\beta\left(\theta\frac{q_1\gamma E_{\sup}}{\mu+\alpha_1}+\frac{q_2\gamma E_{\sup}}{\mu+\alpha_2}\right)-(\mu+\gamma)\bar{E}(\xi) = 0, \end{equation*} |
where
\begin{equation*} E(\xi)\leq\bar{\Lambda}\left(\theta\frac{q_1\gamma}{\mu+\alpha_1}+\frac{q_2\gamma}{\mu+\alpha_2}\right)E_{\sup}, \end{equation*} |
which indicates
\begin{equation*} \bar{\Lambda}\left(\theta\frac{q_1\gamma}{\mu+\alpha_1}+\frac{q_2\gamma}{\mu+\alpha_2}\right)\geq1. \end{equation*} |
and contradicts the fact
Theorem 3.2. As
Proof. Set a new sequence
\begin{equation*} \lim\limits_{m\rightarrow+\infty}E(\xi_m) = \hat{B} = \sup\limits_{\xi\in\mathbb{R}}E(\xi). \end{equation*} |
Now, we need to show
\begin{equation*} (S_{1,m}(\xi),\ S_{2,m}(\xi),\ E_{m}(\xi),\ I_{1,m}(\xi),\ I_{2,m}(\xi))\rightarrow(\tilde{S_1}(\xi),\ \tilde{S_2}(\xi),\ \tilde{E}(\xi),\ \tilde{I_1}(\xi),\ \tilde{I_2}(\xi)), \end{equation*} |
as
\begin{equation*} \left\{ \begin{split} &d\tilde{S_1}^{''}(\xi)-c\tilde{S_1}^{'}(\xi)+\Lambda-\beta \tilde{S_1}(\xi)(\theta \tilde{I_1}(\xi)+\tilde{I_2}(\xi))-(\rho+\mu)\tilde{S_1}(\xi) = 0,\\ &d\tilde{S_2}^{''}(\xi)-c\tilde{S_2}^{'}(\xi)+\rho \tilde{S_1}(\xi)-\beta\sigma\tilde{S_2}(\xi)(\theta\tilde{I_1}(\xi)+\tilde{I_2}(\xi))-\mu \tilde{S_2}(\xi) = 0,\\ &D_0\tilde{E}^{''}(\xi)-c\tilde{E}^{'}(\xi)+\beta\left[\theta \tilde{I_1}(\xi)+\tilde{I_2}(\xi)\right]\left[\tilde{S_1}(\xi)+\sigma \tilde{S_2}(\xi)\right]-(\mu+\gamma)\tilde{E}(\xi) = 0,\\ &D_1\tilde{I_1}^{''}(\xi)-c\tilde{I_1}^{'}(\xi)+q_1\gamma \tilde{E}(\xi)-(\mu+\alpha_1)\tilde{I_1}(\xi) = 0,\\ &D_2\tilde{I_2}^{''}(\xi)-c\tilde{I_2}^{'}(\xi)+q_2\gamma \tilde{E}(\xi)-(\mu+\alpha_2)\tilde{I_2}(\xi) = 0,\\ &\tilde{E}(0) = \hat{B},\ \tilde{E}(\xi)\leq\hat{B},\\ &0\leq\tilde{S_i}(\xi)\leq S_i^0,\ i = 1,\ 2,\ \forall\xi\in\mathbb{R}.\\ \end{split} \right. \end{equation*} |
The maximum principle implies that
\begin{equation*} \tilde{I_i}(\xi)\leq\frac{q_i\gamma\hat{B}}{\mu+\alpha_i}. \end{equation*} |
Moreover, we can yields
\begin{equation*} 0\leq D_0\tilde{E}^{''}(0)+\beta[\tilde{S_1}(0)+\sigma\tilde{S_1}(0)]\left(\theta\frac{q_1\gamma}{\mu+\alpha_1}+\frac{q_2\gamma}{\mu+\alpha_2}\right)\tilde{B}-(\mu+\gamma)\tilde{B}. \end{equation*} |
Owing to
In this case, we attempt to utilize the two-sides Laplace transform to gain the non-existence of traveling wave solution for system (2). Therefore, we firstly need to imply the exponential boundedness for traveling wave solution via next two lemmas.
Lemma 3.3. Assume that
\begin{equation*} \int_{-\infty}^{\xi}E(x)\mathrm{d}x < \mathcal{J},\ \int_{-\infty}^{\xi}I_1(x)\mathrm{d}x < \mathcal{J},\ \int_{-\infty}^{\xi}I_2(x)\mathrm{d}x < \mathcal{J}, \end{equation*} |
for
Proof. Set
S_i(\xi) > S_i^0(1-\varepsilon),\quad\xi\in(-\infty,\ -2G). |
For any
\begin{align} \beta(S_1(\xi)+\sigma S_2(\xi))(\theta I_1(\xi)+I_2(\xi))-(\mu+\gamma)E(\xi)\geq&\beta(S_1^0+\sigma S_2^0)(1-\varepsilon)[\theta I_1(\xi)\\ &+I_2(\xi)]-(\mu+\gamma)E(\xi) \end{align} | (45) |
For
\begin{equation*} \bar{J_0}(\xi,\ y) = \int_{y}^{\xi}E(x)\mathrm{d}x,\ \bar{J_1}(\xi,\ y) = \int_{y}^{\xi}I_1(x)\mathrm{d}x,\ \bar{J_2}(\xi,\ y) = \int_{y}^{\xi}I_2(x)\mathrm{d}x. \end{equation*} |
Integrate both sides of inequalities (45) from
\begin{align} &\beta(S_1^0+\sigma S_2^0)(1-\varepsilon)(\theta\bar{J_1}(\xi,\ y)+\bar{J_2}(\xi,\ y))-(\mu+\gamma)\bar{J_0}(\xi,\ y)\\ &\leq\int_{y}^{\xi}[\beta(S_1(x)+\sigma S_2(x))(\theta I_1(x)+I_2(x))-(\mu+\gamma)E(x)]\mathrm{d}x. \end{align} | (46) |
It follows from Lemma 2.10 and inequality (29) that
\begin{equation} \parallel E(\cdot)\parallel_{C^2(\mathbb{R})}\leq\hat{P}, \parallel I_i(\cdot)\parallel_{C^2(\mathbb{R})}\leq\hat{P}, \end{equation} | (47) |
and
\begin{equation} \lim\limits_{\xi\rightarrow-\infty}E^{'}(\xi) = \lim\limits_{\xi\rightarrow-\infty}I_i^{'}(\xi) = 0, \end{equation} | (48) |
where
\begin{align} &\int_{-\infty}^{\xi}[\beta(S_1(x)+\sigma S_2(x))(\theta I_1(x)+I_2(x))-(\mu+\gamma)E(x)]\mathrm{d}x \end{align} | (49) |
\begin{align} &\qquad = \lim\limits_{y\rightarrow-\infty}\int_{y}^{\xi}[-D_0E^{''}(x)+cE^{'}(x)]\mathrm{d}x = -D_0E^{'}(\xi)+cE(\xi),\\ &\int_{-\infty}^{\xi}[q_i\gamma E(x)-(\mu+\alpha_i)I_i(x)]\mathrm{d}x = \lim\limits_{y\rightarrow-\infty}\int_{y}^{\xi}[-D_iI_i^{''}(x)+cI_i^{'}(x)]\mathrm{d}x\\ &\qquad = -D_iI_i^{'}(\xi)+cI_i(\xi). \end{align} | (50) |
In the following, we aim to prove that there exists a constant
\begin{equation*} \int_{-\infty}^{\xi}E(x)\mathrm{d}x < \mathcal{J},\quad \int_{-\infty}^{\xi}I_i(x)\mathrm{d}x < \mathcal{J},\quad \forall\xi < -2G. \end{equation*} |
Let
\begin{equation*} A = \left( \begin{array}{ccc} \mu+\gamma & \beta\theta(S_1^0+\sigma S_2^0)(1-\varepsilon) & \beta(S_1^0+\sigma S_2^0)(1-\varepsilon)\\ q_1\gamma & \mu+\alpha_1 & 0\\ q_2\gamma & \mu+\alpha_2 & 0\\ \end{array} \right), \end{equation*} |
and we conclude
\begin{align*} \bar{J}(\xi,\ y) = &-(\mu+\alpha_2)\beta\theta(S_1^0+\sigma S_2^0)(1-\varepsilon)(q_1\gamma\bar{J_0}(\xi,\ y)-(\mu+\alpha_1)\bar{J_1}(\xi,\ y))\\ &-(\mu+\alpha_1)\beta(S_1^0+\sigma S_2^0)(1-\varepsilon)(q_2\gamma\bar{J_0}(\xi,\ y)-(\mu+\alpha_2)\bar{J_2}(\xi,\ y)) \end{align*} |
to both sides of the inequality (46) multiplied by
\begin{align} -|A|\bar{J_0}(\xi,\ y)\leq&(\mu+\alpha_1)(\mu+\alpha_2)\int_{y}^{\xi}[\beta(S_1(x)+\sigma S_2(x))(\theta I_1(x)+I_2(x))\\ &-(\mu+\gamma)E(x)]\mathrm{d}x-\bar{J}(\xi,\ y), \end{align} | (51) |
for
\begin{equation*} \int_{-\infty}^{\xi}E(x)\mathrm{d}x < \mathcal{J},\quad \forall\xi < -2G. \end{equation*} |
In the similar way, we also can claim that
\begin{equation*} \int_{-\infty}^{\xi}I_i(x)\mathrm{d}x < \mathcal{J},\quad \forall\xi < -2G. \end{equation*} |
The proof is completed.
Lemma 3.4. Assume that
\begin{align} &\sup\limits_{\xi\in\mathbb{R}}E(\xi)e^{-\mu_0\xi} < \infty,\ \sup\limits_{\xi\in\mathbb{R}}|E^{'}(\xi)|e^{-\mu_0\xi} < \infty,\ \sup\limits_{\xi\in\mathbb{R}}|E^{''}(\xi)|e^{-\mu_0\xi} < \infty,\\ &\sup\limits_{\xi\in\mathbb{R}}I_1(\xi)e^{-\mu_0\xi} < \infty,\ \sup\limits_{\xi\in\mathbb{R}}|I_1^{'}(\xi)|e^{-\mu_0\xi} < \infty,\ \sup\limits_{\xi\in\mathbb{R}}|I_1^{''}(\xi)|e^{-\mu_0\xi} < \infty,\\ &\sup\limits_{\xi\in\mathbb{R}}I_2(\xi)e^{-\mu_0\xi} < \infty,\ \sup\limits_{\xi\in\mathbb{R}}|I_2^{'}(\xi)|e^{-\mu_0\xi} < \infty,\ \sup\limits_{\xi\in\mathbb{R}}|I_2^{''}(\xi)|e^{-\mu_0\xi} < \infty. \end{align} | (52) |
Proof. Fix
S_1(\xi) > S_1^0(1-\varepsilon),\quad S_2(\xi) > S_2^0(1-\varepsilon),\quad \forall\xi < -2G. |
And
\begin{equation} \frac{[q_1\gamma\beta\theta(\mu+\alpha_2)+q_2\gamma\beta(\mu+\alpha_1)](S_1^0+\sigma S_0^2)(1-\varepsilon)}{(\mu+\gamma)(\mu+\alpha_1)(\mu+\alpha_2)} > 1. \end{equation} | (53) |
Thus, for any
\begin{align} &cE^{'}(\xi)\geq D_0E^{''}(\xi)+\beta(S_1^0+S_2^0)(1-\varepsilon)[\theta I_1(\xi)+I_2(\xi)]-(\mu+\gamma)E(\xi), \end{align} | (54) |
\begin{align} &cI_1^{'}(\xi) = D_1I_1^{''}(\xi)+q_1\gamma E(\xi)-(\mu+\alpha_1)I_1(\xi), \end{align} | (55) |
\begin{align} &cI_2^{'}(\xi) = D_2I_2^{''}(\xi)+q_2\gamma E(\xi)-(\mu+\alpha_2)I_2(\xi). \end{align} | (56) |
Set
\bar{J_0}(\xi) = \int_{-\infty}^{\xi}E(x)\mathrm{d}x < \mathcal{J},\ \bar{J_i}(\xi) = \int_{-\infty}^{\xi}I_i(x)\mathrm{d}x < \mathcal{J}, |
for any
\begin{equation} cE(\xi)\geq D_0E^{'}(\xi)+\beta(S_1^0+\sigma S_2^0)(1-\varepsilon)[\theta\bar{J_1}(\xi)+\bar{J_2}(\xi)]-(\mu+\gamma)\bar{J_0}(\xi). \end{equation} | (57) |
Integrating two sides of the inequality (57) from
\begin{align} &\beta(S_1^0+\sigma S_2^0)(1-\varepsilon)[\theta\int_{-\infty}^{\xi}\bar{J_1}(x)\mathrm{d}x+\int_{-\infty}^{\xi}\bar{J_2}(x)\mathrm{d}x]-(\mu+\gamma)\int_{-\infty}^{\xi}\bar{J_0}(x)\mathrm{d}x\\ &\quad+D_0E(\xi)\geq c\bar{J_0}(\xi). \end{align} | (58) |
Via the similar way in equations (55) and (56), we can yield
\begin{equation} q_1\gamma\int_{-\infty}^{\xi}\bar{J_0}(x)\mathrm{d}x-(\mu+\alpha_1)\int_{-\infty}^{\xi}\bar{J_1}(x)\mathrm{d}x+D_1I_1(\xi) = c\bar{J_1}(\xi), \end{equation} | (59) |
and
\begin{equation} q_2\gamma\int_{-\infty}^{\xi}\bar{J_0}(x)\mathrm{d}x-(\mu+\alpha_2)\int_{-\infty}^{\xi}\bar{J_2}(x)\mathrm{d}x+D_2I_2(\xi) = c\bar{J_2}(\xi), \end{equation} | (60) |
which reduces to
\begin{equation} \int_{-\infty}^{\xi}\bar{J_1}(x)\mathrm{d}x = \frac{1}{\mu+\alpha_1}\left(q_1\gamma\int_{-\infty}^{\xi}\bar{J_0}(x)\mathrm{d}x+D_1I_1(\xi)-c\bar{J_1}(\xi)\right), \end{equation} | (61) |
and
\begin{equation} \int_{-\infty}^{\xi}\bar{J_2}(x)\mathrm{d}x = \frac{1}{\mu+\alpha_2}\left(q_2\gamma\int_{-\infty}^{\xi}\bar{J_0}(x)\mathrm{d}x+D_2I_2(\xi)-c\bar{J_2}(\xi)\right). \end{equation} | (62) |
Next, we need to claim there exist two positive constants
\begin{equation} a\sum\limits_{j = 0}^{2}\int_{-\infty}^{\xi}\bar{J_j}(x)\mathrm{d}x\leq b\sum\limits_{j = 0}^{2}\bar{J_j}(x)\mathrm{d}x. \end{equation} | (63) |
Substituting equations (61) and (62) into the equation (59) has
\begin{align*} c\bar{J_0}(\xi)\geq&\beta(S_1^0+\sigma S_2^0)(1-\varepsilon)\left[\frac{\theta}{\mu+\alpha_1}\left(q_1\gamma\int_{-\infty}^{\xi}\bar{J_0}(x)\mathrm{d}x+D_1I_1(\xi)-c\bar{J_1}(\xi)\right)\right.\\ &\left.+\frac{1}{\mu+\alpha_2}\left(q_2\gamma\int_{-\infty}^{\xi}\bar{J_0}(x)\mathrm{d}x+D_2I_2(\xi)-c\bar{J_2}(\xi)\right)\right]\\ &+D_0E(\xi)-(\mu+\gamma)\int_{-\infty}^{\xi}\bar{J_0}(x)\mathrm{d}x\\ = &\left[\beta(S_1^0+\sigma S_2^0)(1-\varepsilon)\left(\frac{q_1\gamma\theta}{\mu+\alpha_1}+\frac{q_2\gamma}{\mu+\alpha_2}\right)-(\mu+\gamma)\right]\int_{-\infty}^{\xi}\bar{J_0}(x)\mathrm{d}x\\ &+\beta(S_1^0+\sigma S_2^0)(1-\varepsilon)\left(\frac{\theta D_1}{\mu+\alpha_1}I_1(\xi)+\frac{D_2}{\mu+\alpha_2}I_2(\xi)\right)+D_0E(\xi)\\ &-c\beta(S_1^0+\sigma S_2^0)(1-\varepsilon)\left(\frac{\theta}{\mu+\alpha_1}\bar{J_1}(\xi)+\frac{1}{\mu+\alpha_2}\bar{J_2}(\xi)\right), \end{align*} |
which implies
\begin{align*} &c\left[\bar{J_0}(\xi)+\beta(S_1^0+\sigma S_2^0)(1-\varepsilon)\left(\frac{\theta}{\mu+\alpha_1}\bar{J_1}(\xi)+\frac{1}{\mu+\alpha_2}\bar{J_2}(\xi)\right) \right]\\ &\quad\geq\left[\beta(S_1^0+\sigma S_2^0)(1-\varepsilon)\left(\frac{q_1\gamma\theta}{\mu+\alpha_1}+\frac{q_2\gamma}{\mu+\alpha_2}\right)-(\mu+\gamma)\right]\int_{-\infty}^{\xi}\bar{J_0}(x)\mathrm{d}x\\ &\quad\quad+\beta(S_1^0+\sigma S_2^0)(1-\varepsilon)\left(\frac{\theta D_1}{\mu+\alpha_1}I_1(\xi)+\frac{D_2}{\mu+\alpha_2}I_2(\xi)\right)+D_0E(\xi). \end{align*} |
Because
\begin{align*} &\left[\beta(S_1^0+\sigma S_2^0)(1-\varepsilon)\left(\frac{q_1\gamma\theta}{\mu+\alpha_1}+\frac{q_2\gamma}{\mu+\alpha_2}\right)-(\mu+\gamma)\right]\int_{-\infty}^{\xi}\bar{J_0}(x)\mathrm{d}x\\ &\quad\leq c\left[\bar{J_0}(\xi)+\beta(S_1^0+\sigma S_2^0)(1-\varepsilon)\left(\frac{\theta}{\mu+\alpha_1}\bar{J_1}(\xi)+\frac{1}{\mu+\alpha_2}\bar{J_2}(\xi)\right) \right]. \end{align*} |
According to the inequality (53), we can claim that there exist two positive constants
\begin{equation} \bar{a_0}\int_{-\infty}^{\xi}\bar{J_0}(x)\mathrm{d}x\leq\bar{b_0}(\bar{J_0}(\xi)+\bar{J_1}(\xi)+\bar{J_2}(\xi)). \end{equation} | (64) |
Plug the inequality (64) into the inequality (58), and it is shown that there are two constants
\begin{equation} \bar{a}\left(\int_{-\infty}^{\xi}\bar{J_1}(x)\mathrm{d}x+\int_{-\infty}^{\xi}\bar{J_2}(x)\mathrm{d}x\right)\leq\bar{b}(\bar{J_0}(\xi)+\bar{J_1}(\xi)+\bar{J_2}(\xi)). \end{equation} | (65) |
Hence, for any
\begin{equation*} \mathcal{J}(\xi) = \bar{J_0}(\xi)+\bar{J_1}(\xi)+\bar{J_2}(\xi), \end{equation*} |
then we gain that
a\int_{-\infty}^{\xi}\mathcal{J}(x)\mathrm{d}x\leq b\mathcal{J}(\xi),\quad\forall\xi < -2G, |
that is
a\int_{-\infty}^{0}\mathcal{J}(x+\xi)\mathrm{d}x\leq b\mathcal{J}(\xi),\quad\forall\xi < -2G. |
Because
\mathcal{J}(\xi-\xi_0)\leq\omega_0\mathcal{J}(\xi)\quad\forall\xi < -2G. |
Let
\begin{equation*} \Psi(\xi-\xi_0) = \mathcal{J}(\xi-\xi_0)e^{-\mu_0(\xi-\xi_0)}\leq\omega_0\mathcal{J}(\xi)e^{-\mu_0(\xi-\xi_0)} = \Psi(\xi), \end{equation*} |
for any
\begin{equation*} E(\xi)\leq p_0e^{\mu_0\xi},\quad I_i(\xi)\leq p_0e^{\mu_0\xi},\quad\forall\xi < -2G. \end{equation*} |
Since
\begin{equation*} E(\xi)\leq p_0e^{\mu_0\xi},\quad I_i(\xi)\leq p_0e^{\mu_0\xi},\quad\forall\xi\in\mathbb{R}. \end{equation*} |
According to estimates (29) and (57), it yields
\sup\limits_{\xi\in\mathbb{R}}|E^{'}(\xi)|e^{-\mu_0\xi} < \infty. |
Via the
\sup\limits_{\xi\in\mathbb{R}}|E^{''}(\xi)|e^{-\mu_0\xi} < \infty. |
Finally, applying the similar argument on
\begin{equation*} \sup\limits_{\xi\in\mathbb{R}}I_i(\xi)e^{-\mu_0\xi} < \infty,\quad \sup\limits_{\xi\in\mathbb{R}}|I_i^{'}(\xi)|e^{-\mu_0\xi} < \infty,\quad \sup\limits_{\xi\in\mathbb{R}}|I_i^{''}(\xi)|e^{-\mu_0\xi} < \infty. \end{equation*} |
This completes the proof.
According to above two theorems, we can obtain the following non-existence theorem.
Theorem 3.5. If
Proof. We intend to prove this theorem by contradiction. Fix
\begin{align} &cK_1^{'}(\xi)-dK_1^{''}(\xi)+(\mu+\rho)K_1^{'}(\xi)-\beta S_1(\xi)[\theta I_1(\xi)+I_2(\xi)] = 0, \end{align} | (66) |
\begin{align} &cK_2^{'}(\xi)-dK_2^{''}(\xi)-\rho K_2^{''}(\xi)+\mu K_2^{'}(\xi)-\beta\sigma S_2(\xi)[\theta I_1(\xi)+I_2(\xi)] = 0. \end{align} | (67) |
According to the inequality
\begin{equation*} \parallel K_i^{'}\parallel_{C((\infty,0],\mathbb{R})}\leq2\parallel K_i^{''}\parallel_{C((\infty,0],\mathbb{R})}^{\frac{1}{2}}\parallel K_i\parallel_{C((\infty,0],\mathbb{R})}^{\frac{1}{2}}, \end{equation*} |
and the fact that
\begin{equation} \lim\limits_{\xi\rightarrow-\infty}K_i(\xi) = 0, \end{equation} | (68) |
we can claim
\begin{equation} \lim\limits_{\xi\rightarrow-\infty}K_i^{'}(\xi) = 0, \end{equation} | (69) |
for
\begin{align*} &c[K_1(\xi)+K_2(\xi)]-d[K_1^{'}(\xi)+K_2^{'}(\xi)]+\mu\int_{-\infty}^{\xi}[K_1(x)+K_2(x)]\mathrm{d}x\\&\quad-\int_{-\infty}^{\xi}\beta[S_1(x)+\sigma S_2(x)][\theta I_1(x)+I_2(x)]\mathrm{d}x = 0. \end{align*} |
Let
\begin{align*} K_1(\xi)+K_2(\xi)& = [K_1(0)+K_2(0)]e^{\frac{c}{d}\xi}+\frac{1}{d}e^{\frac{c}{d}\xi}\int_{\xi}^{0}e^{-\frac{c}{d}x}[-K_\mu(x)+f(x)]\mathrm{d}x\\ &\leq[K_1(0)+K_2(0)]e^{\frac{c}{d}\xi}+\frac{1}{d}e^{\frac{c}{d}\xi}\int_{\xi}^{0}e^{-\frac{c}{d}x}f(x)\mathrm{d}x, \end{align*} |
for
\begin{equation*} \sup\limits_{\xi\in\mathbb{R}}[K_1(\xi)+K_2(\xi)]e^{-\mu_{0}^{'}\xi} < \infty, \end{equation*} |
which implies
\begin{equation} \sup\limits_{\xi\in\mathbb{R}}K_1(\xi)e^{-\mu_{0}^{'}\xi} < \infty,\quad\sup\limits_{\xi\in\mathbb{R}}K_2(\xi)e^{-\mu_{0}^{'}\xi} < \infty. \end{equation} | (70) |
On the basis of the above discussion, we define the one-sided Laplace transforms for
\begin{equation} L_0(\hat{\lambda}) = \int_{-\infty}^{0}e^{-\hat{\lambda}\xi}E(\xi)\mathrm{d}\xi,\ L_1(\hat{\lambda}) = \int_{-\infty}^{0}e^{-\hat{\lambda}\xi}I_1(\xi)\mathrm{d}\xi,\ L_2(\hat{\lambda}) = \int_{-\infty}^{0}e^{-\hat{\lambda}\xi}I_2(\xi)\mathrm{d}\xi. \end{equation} | (71) |
Next, we only consider
\begin{align*} \mathcal{L}_0(\hat{\lambda})& = \int_{-\infty}^{+\infty}e^{-\hat{\lambda}\xi}E(\xi)\mathrm{d}\xi,\quad\mathcal{L}_1(\hat{\lambda}) = \int_{-\infty}^{+\infty}e^{-\hat{\lambda}\xi}I_1(\xi)\mathrm{d}\xi,\quad\mathcal{L}_2(\hat{\lambda})\\ & = \int_{-\infty}^{+\infty}e^{-\hat{\lambda}\xi}I_2(\xi)\mathrm{d}\xi, \end{align*} |
and we also only research
\begin{equation*} \int_{0}^{+\infty}e^{-\hat{\lambda}\xi}E(\xi)\mathrm{d}\xi < \infty,\quad\int_{0}^{+\infty}e^{-\hat{\lambda}\xi}I_1(\xi)\mathrm{d}\xi < \infty,\quad\int_{0}^{+\infty}e^{-\hat{\lambda}\xi}I_2(\xi)\mathrm{d}\xi < \infty, \end{equation*} |
for any
In the following, we indicate that there indeed exists a
\begin{align*} &D_0E^{''}(\xi)-cE^{'}(\xi)+\beta(S_1^0+\sigma S_2^0)[\theta I_1(\xi)+I_2(\xi)]-(\mu+\gamma)E(\xi)\\&\quad = \beta[S_1^0-S_1(\xi)+\sigma S_2^0-S_2(\xi)][\theta I_1(\xi)+I_2(\xi)],\qquad\forall\xi\in\mathbb{R}, \end{align*} |
it is implied that
\begin{align} &[D_0\hat{\lambda}^2-c\hat{\lambda}-(\mu+\gamma)]L_0(\hat{\lambda})+\beta(S_1^0+\sigma S_2^0)[\theta \mathcal{L}_1(\hat{\lambda})+\mathcal{L}_2(\hat{\lambda})]\\ &\quad = \beta\int_{-\infty}^{+\infty}[S_1^0-S_1(\xi)+\sigma (S_2^0-S_2(\xi))][\theta I_1(\xi)+I_2(\xi)]e^{-\hat{\lambda}\xi}\mathrm{d}\xi,\qquad\forall\xi\in\mathbb{R}. \end{align} | (72) |
In the same way, we can obtain
\begin{equation} [D_1\hat{\lambda}^2-c\hat{\lambda}-(\mu+\alpha_1)]\mathcal{L}_1(\hat{\lambda})+q_1\gamma\mathcal{L}_0(\hat{\lambda}) = 0, \end{equation} | (73) |
and
\begin{equation} [D_2\hat{\lambda}^2-c\hat{\lambda}-(\mu+\alpha_2)]\mathcal{L}_2(\hat{\lambda})+q_2\gamma\mathcal{L}_0(\hat{\lambda}) = 0. \end{equation} | (74) |
Thus, it follows from equations (73) and (74) that
\begin{align*} &\beta\int_{-\infty}^{+\infty}[S_1^0-S_1(\xi)+\sigma (S_2^0-S_2(\xi))][\theta I_1(\xi)+I_2(\xi)]e^{-v\xi}\mathrm{d}\xi\\ &\quad\leq\beta\sup\limits_{\xi\in\mathbb{R}}\left\{[S_1^0-S_1(\xi)+\sigma (S_2^0-S_2(\xi))]e^{-\mu_0^{'}\xi}\right\}\left[\int_{-\infty}^{-\infty}\theta I_1(\xi)e^{-(v-\mu_0^{'})\xi}\mathrm{d}\xi\right.\\ &\qquad\left.+\int_{-\infty}^{-\infty}I_2(\xi) e^{-(v-\mu_0^{'})\xi}\mathrm{d}\xi\right]\\ &\quad\leq\infty. \end{align*} |
However, the left side tends to
\begin{align} m_0(\hat{\lambda},\ c)\mathcal{L}_0({\hat{\lambda}})[\rho^2(\hat{\lambda},\ c)-1] = &\beta\int_{-\infty}^{+\infty}[S_1^0-S_1(\xi)+\sigma (S_2^0-S_2(\xi))][\theta I_1(\xi)\\ &+I_2(\xi)]e^{-\hat{\lambda}\xi}\mathrm{d}\xi, \end{align} | (75) |
and
If the case (ⅱ) is established, similarly, let
Section 2 and Section 3 have proved the existence and non-existence of traveling wave solution for system (2) satisfying the boundary condition (6). In this section, we aim to visually display the existence of traveling wave solution for system (2) connecting disease-free equilibrium and endemic equilibrium. Now, we firstly take a set of parameters for system (2) as follows:
\begin{align*} &d = 0.02,\ D_0 = 0.015,\ D_1 = 0.005,\ D_2 = 0.01,\ D = 0.02,\\ &\Lambda = 8,\ \mu = 0.3,\ \beta = 0.375,\ \rho = 0.7,\\ &\sigma = 0.5,\ \gamma = 0.75,\ \alpha_1 = 0.65,\ \alpha_2 = 0.5,\\ &\theta = 0.5,\ q_1 = 0.6,\ q_2 = 0.4. \end{align*} |
As a result, we can obtain the disease-free equilibrium
\begin{eqnarray*} &&S_i(x,\ t) = \left\{ \begin{split} &S_i^*,\quad0 < x\leq200,\quad t = 0,\quad i = 1,\ 2,\\ &S_i^0,\quad-200\leq x\leq0,\quad t = 0,\quad i = 1,\ 2, \end{split} \right.\\ &&E(x,\ t) = \left\{ \begin{split} &E^*,\quad0 < x\leq200,\quad t = 0,\\ &0,\quad-200\leq x\leq0,\quad t = 0, \end{split} \right.\\ &&I_i(x,\ t) = \left\{ \begin{split} &I_i^*,\quad0 < x\leq200,\quad t = 0,\quad i = 1,\ 2,\\ &0,\quad-200\leq x\leq0,\quad t = 0,\quad i = 1,\ 2, \end{split} \right.\\ &&R(x,\ t) = \left\{ \begin{split} &R^*,\quad0 < x\leq200,\quad t = 0,\\ &0,\quad-200\leq x\leq0,\quad t = 0. \end{split} \right. \end{eqnarray*} |
The figure 1, simulations with applying above conditions, indicate that there exists a traveling wave solution of system (2) connecting disease-free equilibrium and endemic equilibrium. Meanwhile, we cross section curves of traveling wave solution in figure 1 as
Since we pay more attention to influences of self-protection and treatment in the spatial spread for an epidemic, it is critical to research the change of the minimal spread speed
\begin{equation} \frac{\partial\rho}{\partial\theta} > 0,\quad \frac{\partial\rho}{\partial\sigma} > 0, \end{equation} | (76) |
respectively, where
In this paper, we mainly construct a non-monotonic reaction diffusion SEIR model with effects of self-protection and treatment in incident rate, and determine the existence and non-existence of traveling wave solution connecting disease-free equilibrium and endemic equilibrium. We prove the existence as
We would like to thank you for following the instructions above very closely in advance. It will definitely save us lot of time and expedite the process of your paper's publication.
[1] | Ghorani-Azam, A, Riahi-Zanjani B, Balali-Mood M (2016) Effects of air pollution on human health and practical measures for prevention in Iran. J Res Med Sci 21. https://doi.org/10.4103/1735-1995.189646 |
[2] | Hailstone J. Hailstone J (2023) https://www.forbes.com/sites/jamiehailstone/2023/03/07/nearly-nowhere-on-earth-safe-from-air-pollution-study-finds/?sh=1e0d9fd9da1d/ (accessed july 25, 2023). |
[3] |
Ordóñez C, Garrido-Perez J M, García-Herrera R (2020) Early spring near-surface ozone in Europe during the COVID-19 shutdown: Meteorological effects outweigh emission changes. Sci Total Environ 747: 141322. https://doi.org/10.1016/j.scitotenv.2020.141322 doi: 10.1016/j.scitotenv.2020.141322
![]() |
[4] |
Mostafa M K, Gamal G, Wafiq A (2021) The impact of COVID 19 on air pollution levels and other environmental indicators-A case study of Egypt. J Environ Manage 277: 111496. https://doi.org/10.1016/j.jenvman.2020.111496 doi: 10.1016/j.jenvman.2020.111496
![]() |
[5] |
Gagliardi R V, Andenna C (2020) A machine learning approach to investigate the surface ozone behavior. Atmosphere 11: 1173. https://doi.org/10.3390/atmos11111173 doi: 10.3390/atmos11111173
![]() |
[6] |
Jaffe DA, Cooper OR, Fiore AM, et al. (2018) Scientific assessment of background ozone over the US: Implications for air quality management. Elem Sci Anth 6: 56. https://doi.org/10.1525/elementa.309 doi: 10.1525/elementa.309
![]() |
[7] |
Lu H, Lyu X, Cheng H, et al.(2019) Overview on the spatial–temporal characteristics of the ozone formation regime in China. Environmental Science: Processes & Impacts 21: 916-929. https://doi.org/10.1039/C9EM00098D doi: 10.1039/C9EM00098D
![]() |
[8] |
Käffer M I, Domingos M, Lieske I, et al. (2019) Predicting ozone levels from climatic parameters and leaf traits of Bel-W3 tobacco variety. Environ Pollut 248: 471-477. https://doi.org/10.1016/j.envpol.2019.01.130 doi: 10.1016/j.envpol.2019.01.130
![]() |
[9] |
Li Y, Xue Y, Guang J, et al. (2018) Ground-level PM2. 5 concentration estimation from satellite data in the Beijing area using a specific particle swarm extinction mass conversion algorithm. Remote Sens 10: 1906. https://doi.org/10.3390/rs10121906 doi: 10.3390/rs10121906
![]() |
[10] |
Velasco E, Retama A (2017) Ozone's threat hits back Mexico City. Sustain Cities Soc 31: 260-263. https://doi.org/10.1016/j.scs.2016.12.015 doi: 10.1016/j.scs.2016.12.015
![]() |
[11] |
Carbo-Bustinza N, Belmonte M, Jimenez V, et al. (2022) A machine learning approach to analyse ozone concentration in metropolitan area of Lima, Peru. Sci Rep 12: 22084. https://doi.org/10.1038/s41598-022-26575-3 doi: 10.1038/s41598-022-26575-3
![]() |
[12] |
Cohen AJ, Brauer M, Burnett R, et al. (2017) Estimates and 25-year trends of the global burden of disease attributable to ambient air pollution: an analysis of data from the Global Burden of Diseases Study 2015. The Lancet 389: 1907-1918. https://doi.org/10.1016/S0140-6736(17)30505-6 doi: 10.1016/S0140-6736(17)30505-6
![]() |
[13] |
Iftikhar H, Khan M, Khan Z, et al. (2023). A Comparative Analysis of Machine Learning Models: A Case Study in Predicting Chronic Kidney Disease. Sustainability 15: 2754. https://doi.org/10.3390/su15032754 doi: 10.3390/su15032754
![]() |
[14] |
Jakovlev A R, Smyshlyaev S P et al. (2019) Numerical simulation of world ocean effects on temperature and ozone in the lower and middle atmosphere. Russ Meteorol Hydrol 44: 594-602. https://doi.org/10.3103/S1068373919090036 doi: 10.3103/S1068373919090036
![]() |
[15] |
Gaudel A, Cooper OR, Ancellet G, et al. (2018) Assessment Report: Present-day distribution and trends of tropospheric ozone relevant to climate and global atmospheric chemistry model evaluation. Elem Sci Anth 6: 39. https://doi.org/10.1525/elementa.291 doi: 10.1525/elementa.291
![]() |
[16] |
Rodríguez-Urrego D, Rodríguez-Urrego L (2020) Air quality during the COVID-19: PM2. 5 analysis in the 50 most polluted capital cities in the world. Environ Pollut 266: 115042. https://doi.org/10.1016/j.envpol.2020.115042 doi: 10.1016/j.envpol.2020.115042
![]() |
[17] |
Rybarczyk Y, Zalakeviciute R (2018) Machine learning approaches for outdoor air quality modelling: A systematic review. Appl Sci 8: 2570. https://doi.org/10.3390/app8122570 doi: 10.3390/app8122570
![]() |
[18] |
Iftikhar H, Khan N, Raza MA, et al. (2024). Electricity theft detection in smart grid using machine learning. Front Energy Res 12: 1383090. https://doi.org/10.3389/fenrg.2024.1383090 doi: 10.3389/fenrg.2024.1383090
![]() |
[19] |
Comrie A C (1997) Comparing neural networks and regression models for ozone forecasting. J Air Waste Manage 47: 653-663. https://doi.org/10.1080/10473289.1997.10463925 doi: 10.1080/10473289.1997.10463925
![]() |
[20] |
Carbo-Bustinza N, Iftikhar H, Belmonte M, et al. (2023). Short-term forecasting of Ozone concentration in metropolitan Lima using hybrid combinations of time series models. Appl Sci 13: 10514. https://doi.org/10.3390/app131810514 doi: 10.3390/app131810514
![]() |
[21] | Harrou F, Fillatre L, Bobbia M, et al. (2013) Statistical detection of abnormal ozone measurements based on constrained generalized likelihood ratio test. In 52nd IEEE Conference on Decision and Control, Firenze, Italy, 10-13 December 2013. https://doi.org/10.1109/CDC.2013.6760673 |
[22] |
Duenas C, Fernandez M C, Canete S, et al. (2005) Stochastic model to forecast ground-level ozone concentration at urban and rural areas. Chemosphere 61: 1379-1389. https://doi.org/10.1016/j.chemosphere.2005.04.079 doi: 10.1016/j.chemosphere.2005.04.079
![]() |
[23] |
Iftikhar H, Khan M, Turpo-Chaparro J E, et al. (2024). Forecasting stock prices using a novel filtering-combination technique: Application to the Pakistan stock exchange. AIMS Math 9: 3264-3288. https://doi.org/10.3934/math.2024159 doi: 10.3934/math.2024159
![]() |
[24] |
Petetin H, Bowdalo D, Soret A, et al. (2020) Meteorology-normalized impact of the COVID-19 lockdown upon NO 2 pollution in Spain. Atmos Chem Phys 20: 19-11141. https://doi.org/10.5194/acp-20-11119-2020 doi: 10.5194/acp-20-11119-2020
![]() |
[25] |
Aljanabi M, Shkoukani M, Hijjawi M (2020) Ground-level ozone prediction using machine learning techniques: A case study in Amman, Jordan. Int J Autom Comput 17: 667-677. https://doi.org/10.1007/s11633-020-1233-4 doi: 10.1007/s11633-020-1233-4
![]() |
[26] |
Sousa S I V, Martins F G, Alvim-Ferraz M C, et al. (2007) Multiple linear regression and artificial neural networks based on principal components to predict ozone concentrations. Environ Modell Softw 22: 97-103. https://doi.org/10.1016/j.envsoft.2005.12.002 doi: 10.1016/j.envsoft.2005.12.002
![]() |
[27] |
Chelani A B (2010) Prediction of daily maximum ground ozone concentration using support vector machine. Environmental monitoring and assessment 162: 169-176. https://doi.org/10.1007/s10661-009-0785-0 doi: 10.1007/s10661-009-0785-0
![]() |
[28] |
Ren X, Mi Z, Georgopoulos P G (2020) Comparison of Machine Learning and Land Use Regression for fine scale spatiotemporal estimation of ambient air pollution: Modeling ozone concentrations across the contiguous United States. Environ Int 142: 105827. https://doi.org/10.1016/j.envint.2020.105827 doi: 10.1016/j.envint.2020.105827
![]() |
[29] |
Yafouz A, AlDahoul N, Birima AH, et al. (2022) Comprehensive comparison of various machine learning algorithms for short-term ozone concentration prediction. Alex Eng J 61: 4607-4622. https://doi.org/10.1016/j.aej.2021.10.021 doi: 10.1016/j.aej.2021.10.021
![]() |
[30] |
Pan Q, Harrou F, Sun Y A (2023) comparison of machine learning methods for ozone pollution prediction. J Big Data 10: 63. https://doi.org/10.1186/s40537-023-00748-x doi: 10.1186/s40537-023-00748-x
![]() |
[31] |
Iftikhar H, Zafar A, Turpo-Chaparro J E, et al. (2023) Forecasting Day-Ahead Brent Crude Oil Prices Using Hybrid Combinations of Time Series Models. Mathematics 16: 3548. https://doi.org/10.3390/math11163548 doi: 10.3390/math11163548
![]() |
[32] |
Iftikhar H, Bibi N, Canas Rodrigues P, et al. (2023) Multiple Novel Decomposition Techniques for Time Series Forecasting: Application to Monthly Forecasting of Electricity Consumption in Pakistan. Energies 16: 2579. https://doi.org/10.3390/en16062579 doi: 10.3390/en16062579
![]() |
[33] |
Alshanbari H M, Iftikhar H, Khan F, et al. (2023). On the Implementation of the Artificial Neural Network Approach for Forecasting Different Healthcare Events. Diagnostics 13: 1310. https://doi.org/10.3390/diagnostics13071310 doi: 10.3390/diagnostics13071310
![]() |
[34] | Iftikhar H (2018) Modeling and Forecasting Complex Time Series: A Case of Electricity Demand. Master's Thesis, Quaidi-Azam University, Islamabad, Pakistan, 1-94. |
[35] |
Shah I, Iftikhar H, Ali S (2020) Modeling and forecasting medium-term electricity consumption using component estimation technique. Forecasting 2: 163–179. https://doi.org/10.3390/forecast2020009 doi: 10.3390/forecast2020009
![]() |
[36] |
Iftikhar H, Turpo-Chaparro J E, Canas Rodrigues P, et al. (2023). Day-Ahead Electricity Demand Forecasting Using a Novel Decomposition Combination Method. Energies 16: 6675. https://doi.org/10.3390/en16186675 doi: 10.3390/en16186675
![]() |
[37] |
Shah I, Iftikhar H, Ali S, et al. (2019) Short-term electricity demand forecasting using components estimation technique. Energies 12: 2532. https://doi.org/10.3390/en12132532 doi: 10.3390/en12132532
![]() |
[38] | Van Buuren S, Oudshoorn C G (2000) Multivariate imputation by chained equations. |
[39] |
Iftikhar H, Turpo-Chaparro J E, Canas Rodrigues P, et al. (2023). Forecasting Day-Ahead Electricity Prices for the Italian Electricity Market Using a New Decomposition—Combination Technique. Energies 16: 6669. https://doi.org/10.3390/en16186669 doi: 10.3390/en16186669
![]() |
[40] |
Diebold F X, Mariano R S (2022) Comparing predictive accuracy. J Bus Econ Stat 20: 134–144. https://doi.org/10.1198/073500102753410444 doi: 10.1198/073500102753410444
![]() |
[41] |
Iftikhar H, Khan M, Khan M S, et al. (2023). Short-Term Forecasting of Monkeypox Cases Using a Novel Filtering and Combining Technique. Diagnostics 13: 1923. https://doi.org/10.3390/diagnostics13111923 doi: 10.3390/diagnostics13111923
![]() |
[42] |
Shah I, Iftikhar H, Ali S (2022) Modeling and forecasting electricity demand and prices: A comparison of alternative approaches. J Math 2022: 3581037. https://doi.org/10.1155/2022/3581037 doi: 10.1155/2022/3581037
![]() |
[43] |
Iftikhar H, Daniyal M, Qureshi M, et al. (2023). A hybrid forecasting technique for infection and death from the mpox virus. Digit Health 9: 20552076231204748. https://doi.org/10.1177/20552076231204748 doi: 10.1177/20552076231204748
![]() |
[44] |
Dickey D A, Fuller W A (1979) Distribution of the estimators for autoregressive time series with a unit root. J Am Stat Assoc 74: 427–431. https://doi.org/10.1080/01621459.1979.10482531 doi: 10.1080/01621459.1979.10482531
![]() |
[45] | Romero Y, Diaz C, Meldrum I, et al. (2020) Temporal and spatial analysis of traffic–Related pollutant under the influence of the seasonality and meteorological variables over an urban city in Peru. Heliyon https://doi.org/10.1016/j.heliyon.2020.e04029 |
[46] | Leon C A M, Felix M F M, Olivera C A C, et al (2022) Influence of Social Confinement by COVID-19 on Air Quality in the District of San 503 Juan de Lurigancho in Lima, Perù. Chem Eng Trans 91: 475–480. |
[47] |
Aaker D A, Jacobson R (1987). The sophistication of 'naive'modeling. Int J Forecast 3: 449-451. https://doi.org/10.1016/0169-2070(87)90039-2 doi: 10.1016/0169-2070(87)90039-2
![]() |
[48] |
Gonzales Javier L L, Calili Rodrigo F, Souza Reinaldo C, et al. (2016) Simulation of the energy efficiency auction prices in Brazil. Renew Energ Power Qual J 1: 574-579. https://doi.org/10.24084/repqj14.396 doi: 10.24084/repqj14.396
![]() |
[49] |
López-Gonzales J L, Souza RC, Da Silva FLC, et al. (2020) Simulation of the energy efficiency auction prices via the markov chain monte carlo method. Energies 13: 4544. https://doi.org/10.3390/en13174544 doi: 10.3390/en13174544
![]() |
[50] | da Silva KLS, López-Gonzales J L, Turpo-Chaparro JE, et al. Spatio-temporal visualization and forecasting of PM10 in the Brazilian state of Minas Gerais. Sci Rep 13: 3269. https://doi.org/10.1038/s41598-023-30365-w |
[51] |
Jeldes N, Ibacache-Pulgar G, Marchant C, et al. (2022) Modeling Air Pollution Using Partially Varying Coefficient Models with Heavy Tails. Mathematics 10: 3677. https://doi.org/10.3390/math10193677 doi: 10.3390/math10193677
![]() |
[52] |
Cabello-Torres RJ, Estela MAP, Sánchez-Ccoyllo O, et al. (2022) Statistical modeling approach for PM10 prediction before and during confinement by COVID-19 in South Lima, Perú. Sci Rep 12: 1. https://doi.org/10.1038/s41598-022-20904-2 doi: 10.1038/s41598-022-20904-2
![]() |
[53] |
Orrego Granados D, Ugalde J, Salas R, et al. (2022) Visual-Predictive Data Analysis Approach for the Academic Performance of Students from a Peruvian University. Appl Sci 12: 11251. https://doi.org/10.3390/app122111251 doi: 10.3390/app122111251
![]() |
1. | Guangbing Xiao, Kang Liu, Ning Sun, Yong Zhang, Game-Based Vehicle Strategy Equalization Algorithm for Unsignalized Intersections, 2024, 15, 2032-6653, 146, 10.3390/wevj15040146 |