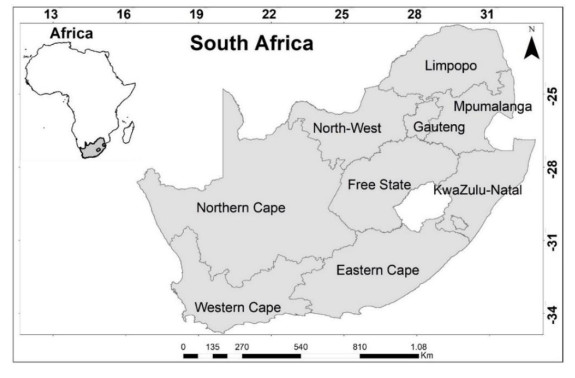
The global concentration of fine particulate matter (PM2.5) is experiencing an upward trend. This study investigates the utilization of space-time cubes to visualize and interpret PM2.5 data in South Africa over multiple temporal intervals spanning from 1998 to 2022. The findings indicated that the mean PM2.5 concentrations in Gauteng Province were the highest, with a value of 53 μg/m3 in 2010, whereas the lowest mean PM2.5 concentrations were seen in the Western Cape Province, with a value of 6.59 μg/m3 in 1999. In 2010, there was a rise in the average concentration of PM2.5 across all provinces. The increase might be attributed to South Africa being the host nation for the 2010 FIFA World Cup. In most provinces, there has been a general trend of decreasing PM2.5 concentrations over the previous decade. Nevertheless, the issue of PM2.5 remains a large reason for apprehension. The study also forecasts South Africa's PM2.5 levels until 2029 using simple curve fitting, exponential smoothing and forest-based models. Spatial analysis revealed that different areas require distinct models for accurate forecasts. The complexity of PM2.5 trends underscores the necessity for varied models and evaluation tools.
Citation: Tabaro H. Kabanda. Investigating PM2.5 pollution patterns in South Africa using space-time analysis[J]. AIMS Environmental Science, 2024, 11(3): 426-443. doi: 10.3934/environsci.2024021
[1] | Ernyasih, Anwar Mallongi, Anwar Daud, Sukri Palutturi, Stang, Abdul RazakThaha, Erniwati Ibrahim, Wesam Al Madhoun, Andriyani . Strategy for mitigating health and environmental risks from vehicle emissions in South Tangerang. AIMS Environmental Science, 2023, 10(6): 794-808. doi: 10.3934/environsci.2023043 |
[2] | Jordan Finch, Daniel W. Riggs, Timothy E. O’Toole, C. Arden Pope III , Aruni Bhatnagar, Daniel J. Conklin . Acute exposure to air pollution is associated with novel changes in blood levels of endothelin-1 and circulating angiogenic cells in young, healthy adults. AIMS Environmental Science, 2019, 6(4): 265-276. doi: 10.3934/environsci.2019.4.265 |
[3] | Nikolaos Barmparesos, Vasiliki D. Assimakopoulos, Margarita Niki Assimakopoulos, Evangelia Tsairidi . Particulate matter levels and comfort conditions in the trains and platforms of the Athens underground metro. AIMS Environmental Science, 2016, 3(2): 199-219. doi: 10.3934/environsci.2016.2.199 |
[4] | Suwimon Kanchanasuta, Sirapong Sooktawee, Natthaya Bunplod, Aduldech Patpai, Nirun Piemyai, Ratchatawan Ketwang . Analysis of short-term air quality monitoring data in a coastal area. AIMS Environmental Science, 2021, 8(6): 517-531. doi: 10.3934/environsci.2021033 |
[5] | K. Wayne Forsythe, Cameron Hare, Amy J. Buckland, Richard R. Shaker, Joseph M. Aversa, Stephen J. Swales, Michael W. MacDonald . Assessing fine particulate matter concentrations and trends in southern Ontario, Canada, 2003–2012. AIMS Environmental Science, 2018, 5(1): 35-46. doi: 10.3934/environsci.2018.1.35 |
[6] | Carolyn Payus, Siti Irbah Anuar, Fuei Pien Chee, Muhammad Izzuddin Rumaling, Agoes Soegianto . 2019 Southeast Asia Transboundary Haze and its Influence on Particulate Matter Variations: A Case Study in Kota Kinabalu, Sabah. AIMS Environmental Science, 2023, 10(4): 547-558. doi: 10.3934/environsci.2023031 |
[7] | Andrea L. Clements, Matthew P. Fraser, Pierre Herckes, Paul A. Solomon . Chemical mass balance source apportionment of fine and PM10 in the Desert Southwest, USA. AIMS Environmental Science, 2016, 3(1): 115-132. doi: 10.3934/environsci.2016.1.115 |
[8] | Scheitel M, Stanic M, Neuberger M . PM10, PM2.5, PM1, number and surface of particles at the child’s seat when smoking a cigarette in a car. AIMS Environmental Science, 2016, 3(4): 582-591. doi: 10.3934/environsci.2016.4.582 |
[9] | Barend L. Van Drooge, David Ramos García, Silvia Lacorte . Analysis of organophosphorus flame retardants in submicron atmospheric particulate matter (PM1). AIMS Environmental Science, 2018, 5(4): 294-304. doi: 10.3934/environsci.2018.4.294 |
[10] | Anwar Khan, Benjamin Razis, Simon Gillespie, Carl Percival, Dudley Shallcross . Global analysis of carbon disulfide (CS2) using the 3-D chemistry transport model STOCHEM. AIMS Environmental Science, 2017, 4(3): 484-501. doi: 10.3934/environsci.2017.3.484 |
The global concentration of fine particulate matter (PM2.5) is experiencing an upward trend. This study investigates the utilization of space-time cubes to visualize and interpret PM2.5 data in South Africa over multiple temporal intervals spanning from 1998 to 2022. The findings indicated that the mean PM2.5 concentrations in Gauteng Province were the highest, with a value of 53 μg/m3 in 2010, whereas the lowest mean PM2.5 concentrations were seen in the Western Cape Province, with a value of 6.59 μg/m3 in 1999. In 2010, there was a rise in the average concentration of PM2.5 across all provinces. The increase might be attributed to South Africa being the host nation for the 2010 FIFA World Cup. In most provinces, there has been a general trend of decreasing PM2.5 concentrations over the previous decade. Nevertheless, the issue of PM2.5 remains a large reason for apprehension. The study also forecasts South Africa's PM2.5 levels until 2029 using simple curve fitting, exponential smoothing and forest-based models. Spatial analysis revealed that different areas require distinct models for accurate forecasts. The complexity of PM2.5 trends underscores the necessity for varied models and evaluation tools.
Particulate matter of a diameter of 2.5 micrometers or less (PM2.5) refers to solid and liquid particles that are floating in the atmosphere. These particles can transport harmful compounds across geographical and physical boundaries. PM2.5 pollution, which threatens public health, is mostly caused by industry, mining, mobile vehicles, residential, and garbage burning [1]. The rapid economic and urbanisation growth in South Africa has led to the emergence of PM2.5 as a significant air pollutant [2]. The PM2.5 concentration in South Africa was 4.5 times the World Health Organization's annual air quality guideline value in 2021 [3]. Coal plays a significant role in South Africa's energy sector, accounting for 69% of its primary energy and 90% of its electricity generation [4]. However, this reliance on coal also leads to elevated levels of PM2.5. Epidemiological research indicates that high levels of PM2.5 harm human health, leading to an elevated risk of cardiovascular disease morbidity and mortality [5]. Investigating the spatio-temporal patterns and trends of PM2.5 contributes to policymakers' effective implementation of preventive measures and carries significant implications for controlling air pollution [6].
The major sources for monitoring PM2.5 are aerosol optical depth (AOD) products and data from the ground monitoring network [7]. The ground measurement locations for PM2.5 exhibit a relatively discrete nature, consequently imposing constraints on the extent of spatial coverage. Satellite-derived AOD is a suitable method for evaluating ground-level PM2.5 pollution in regions where surface PM2.5 monitoring stations are scarce, owing to its extensive coverage, fine spatial resolution, and consistent repeated observation [8]. The quantification of light absorption by aerosols is accomplished by utilizing the AOD scale, which assesses the extent to which particles obstruct the passage of light into the atmosphere. The retrieval of visual AOD exhibits heightened sensitivity towards particles ranging from 0.1 to 2 m, which coincides with the particle size of PM2.5. This sensitivity is a crucial theoretical foundation for establishing the connection between AOD and PM2.5 [9].
In South Africa, Kneen et al. [10] conducted a study that demonstrated the potential of satellite technology as a reliable and effective alternative to traditional ground-based monitoring methods. Similarly, Muyemeki et al. [11] explored using satellite remote sensing as a possible replacement for ground-based surveillance. They used a dataset spanning eight years (2009–2016) and focused their research on the Vaal Triangle Airshed Priority Area (VTAPA) in South Africa. However, they generally observed a discrepancy between the PM2.5 estimates from satellite data and the PM2.5 measurements recorded at ground level. The root mean square error (RMSE) values exhibited a range of 6 to 11 μg/m3, whereas the correlation coefficient values spanned from –0.89 to 0.32. The paper proposed additional research to enhance the precision and accuracy of PM2.5 measurements obtained by satellite retrieval. Great progress has been made in developing regional algorithms, such as the Random Forest algorithm, enabling the retrieval of PM2.5 concentrations on a broad scale with high spatial resolutions [12]. These developments have demonstrated satisfactory concordance with ground measurements, as van Donkelaar et al. [13] reported. Retrievals of PM2.5 micrometers or less have proven to be effective in evaluating the extended regional and temporal trends in several regions, including West Europe, China, and Australia [12,14,15]. The spatiotemporal analysis in this study utilizes the global-scale compilation of PM2.5 big data conducted by [13]. The dataset presented in this study incorporates a geophysical-hybrid approach that combines satellite retrievals, chemical transport modelling, and ground monitor-based calibration. This methodology allows for reliable long-term global [16] and regional [17] estimates of PM2.5 concentrations.
Despite the valuable insights offered by most spatial analysis approaches, their limited temporal sensitivity hinders the identification of time-based clusters. To address this limitation, a series of discrete outputs is utilized to showcase the temporal impact, enabling the exploration of time-dependent patterns [18]. Utilizing space-time cube analysis offers a notable benefit compared to absolute and relative location mapping, spatial autocorrelation, Getis-Ord Gi*, and local Anselin Moran's I [19]. This advantage stems from its implementation of a three-dimensional model, which enables the depiction of space in a horizontal manner and time in a vertical manner. The space-time cube is a method that combines discrete events into bins, leading to the creation of precise representations of spatio-temporal data organized into time-based clusters. These clusters include new, consecutive, persistent, oscillating, and sporadic hot or cold spots [19]. The importance of spatial time cube has played a pivotal role in comprehending the spread of COVID-19, pedestrian collisions, and climate patterns [20,21,22]. This study uses space-time pattern mining tools described under the methods section to explore national differences in PM2.5 patterns in space and time, find areas with extreme or unusual pollution patterns, and forecast yearly average PM2.5 concentrations to 2029. Consequently, this research comprises two major analytical components. The first component involves quantitatively examining variations in PM2.5 concentration utilizing a space-time cube. The second component entails the forecasting of PM2.5 concentrations till the year 2029. Four distinct steps was derived from the cube, namely: 1) The Mann-Kendall trend test, which was employed to detect statistically significant upward or downward trends over time; 2) the emerging hot spot analysis, which was utilized to examine the pattern of trends; 3) the 3D visualisation of the space-time cube, which facilitated visual exploration of each time step throughout the entire study period and in each specific area of interest simultaneously; and 4) the time series forecast, which aimed to predict the future behaviour of PM2.5 pollution in space and time based on current patterns. This work presents several contributions. The study aims to offer a comprehensive analysis of PM2.5 concentrations in South Africa over twenty-three years (1998–2022) while employing a space-time cube methodology to conduct spatiotemporal data analysis. To overcome the limitations of traditional spatial analysis approaches, we employed a space-time cube methodology to capture the dynamic nature of PM2.5 pollution patterns.
The present investigation was conducted in South Africa (Figure 1), a country with nine provinces that exhibit significant variations in their respective sizes. Gauteng is the smallest province in terms of geographical size (18,179 km2) and accounts for only 1.5% of the country's land area, yet Gauteng houses more than a quarter of the entire nation's population, making up 26% of the total count [23]. On the other hand, the Northern Cape is the largest province in terms of land area, encompassing about one-third of the total land area of South Africa. Despite its vast expanse, the Northern Cape has the smallest population share among the provinces. South African economy is experiencing rapid development, characterised by notable strengths in natural resources, energy, and finance [2]. The Highveld Plateau, encompassing the provinces of Free State, North West, Mpumalanga, and Gauteng, is characterised by a significant presence of industrial zones and coal-fired power plants [1]. The Gauteng Province encompasses a substantial conurbation known as the Johannesburg-Tshwane-Ekurhuleni mega-city, wherein Johannesburg stands as the most populous urban center in the country.
This study examines the air quality in South Africa by utilizing annual PM2.5 (ug/m3) at 0.01° × 0.01° resolution data from the years 1998 to 2022. The data was obtained from an open-access worldwide database provided by Washington University in St. Louis, USA [24]. The estimation of PM2.5 data involves the integration of various satellite-based measurements, including NASA's MODIS, MISR, and SeaWiFS instruments. These measurements are combined with the GEOS chemical transport model, incorporating gridded emission inventories. The resulting estimates are then calibrated against global ground-based observations of PM2.5 using Geographically Weighted Regression (GWR), as described in the study by van Donkelaar et al. [13].
To gain a deeper comprehension of a geographical phenomenon, a space-time analysis considers space and time in investigating patterns and correlations [25]. Mining for space-time patterns allows for the detailed analysis of regional and temporal data trends. The subsequent steps delineate the procedure for creating space-time cubes using ArcGIS Pro. The process involves several steps: a) Initiating a blank mosaic dataset to accommodate the inclusion of raster files; b) incorporating 25 raster files, each representing a specific year within the range of 1998–2022, into the mosaic dataset; c) augmenting the footprint attribute table with two additional fields, one for the variable (PM2.5) and another for the timestamp; d) establishing multidimensional information; e) generating a multidimensional raster layer; and f) constructing a space-time cube based on the aforementioned multidimensional raster layer. Following the construction of the space-time cube, subsequent analysis is conducted on the cube to ascertain the trends and patterns of the concentration of PM2.5. The analysis encompass the Mann-Kendall trend test, identification of hot spots, and time series forecasting [25].
The Mann-Kendall method, first introduced by Mann and Kendall, is a statistical test used to analyze the correlation between the numerical values of geographical events and the ranks of corresponding time series [26]. The Mann-Kendall test method is considered non-parametric, as it does not rely on assumptions about the underlying distribution of the data. One advantage of this test is that it does not necessitate the data adhering to a specific distribution or being influenced by outliers [27]. Consequently, it may effectively assess the fluctuations in the data variables as they increase or decrease over time.
The present study used the Mann-Kendall test to examine the space-time cube's temporal and spatial distribution trends. The Mann-Kendall trend approach conducts tests on the time series of spatiotemporal columns at each data location. The temporal progression of each space-time bar is documented in the form of a z-score and a p-value. A p-value that is minimal in magnitude suggests that the observed trend possesses statistical significance. Symbols linked to a z-score can indicate an upward trend in spatiotemporal bars (indicated by a positive z-score) or a downward trend in space-time bars (shown by a negative z-score). For the time series, x = {x1, x2, …, xn, }, and the Mann-Kendall trend test is as shown in Eqs 1 and 2:
S=∑n−1i=1∑nj=i+1sgn(XJ−Xi), | (1) |
sgn(XJ−Xi)={1,xj>xi,0,xj=xi,−1,xj<xi. | (2) |
When n equals or exceeds 10, the spatiotemporal column statistic, S, for PM2.5, follows a normal distribution. With a mean value of 0, the variance is determined using Eqs 3 and 4:
Var(S)=n(n−1)(2n+5)18, | (3) |
Zc={S−1√Var(S),S>0,0,S=0,S+1√Var(S),S<0. | (4) |
The Mann-Kendall method is a statistical technique that can be employed to identify and assess the presence of a changing trend in PM2.5 levels and determine the statistical significance of such a trend [25].
A hotspot is a region with more events than predicted from a random distribution [28]. The Emerging Hot Spot Analysis (EHSA) tool identifies statistically significant spatio-temporal trends [29]. It uses a Hot spot analysis tool (Getis-Ord Gi*) to analyze varied spatiotemporal patterns [30]. Positive z-scores are hot spots, while negative z-scores are cold spots. The Mann-Kendall trend test then assesses these trends. The Mann-Kendall trend test evaluates notable trends of hotspot categories on every independent bin time-series test by determining the bin value and their time sequence [30]. Bins are the individual aggregation units that make up a space-time cube. In this study, EHSA categorises hot spot (increasing PM2.5) and cold spot (dropping PM2.5) patterns into 17 classes (eight hot spots, eight cold spots, and one unidentified pattern) based on temporal and spatial evolution tendencies. See the ESRI manual for a description of each model [25].
Time series forecasting estimates future events by understanding historical trends and assuming similar patterns. This is done by building best-fit models that match historical time series trends and extrapolating future values. The more time series data fed into the model, the higher the accuracy. Essential detection could forecast the value at t + 1 given previously measured values at times t = 1, ..., t [31]. Simple curve fitting, exponential smoothing and a forest-based technique (Table 1) can be employed to forecast time series data within ArcGIS Pro's space-time cube. These forecasting methods are executed concurrently on the space-time cube. The process of automatically selecting the optimal forecast is facilitated by Eq 5, which computes the validation root mean square error (RMSE). This metric is calculated based on the final time steps omitted during the fitting phase. The effectiveness of the prediction model in forecasting time series values is then assessed by a validation model [32]. In the validation model, the final time steps of each time series are withheld, and the forecasting model is applied to the remaining data. This validation model is then used to forecast the data values withheld. The forecasted values are compared to the observed values of the hidden data to calculate the validation RMSE. In this study, 18% of the time, steps are withheld for validation. In forecasting and other predictive techniques, at least 18 to 20% is held out for validation [33,34]. The exclusion of time steps should not exceed 25 percent of the total time steps [35]. The validation root mean square error (RMSE) is computed as the square root of the mean of the squared differences between the projected and the raw values of the missing time steps [32].
Validation RMSE=√∑Tt=T−m+1(ct−rt)2m, | (5) |
where T represents the total number of time steps, m denotes the number of time steps reserved for validation, ct symbolises the projected value derived from the initial T-m time steps, and rt represents the unprocessed value of the time series reserved for validation at time t.
Tool | Description |
Curve Fit Forecast (CFF) | Curve fitting is used to forecast the values of each. Linear, parabolic, exponential, or S-shaped curves are all possible (Gompertz). |
Evaluate Forecasts by Location | This allows various Time Series Forecasting tools with the same data to select the best forecast for each location. |
Exponential Smoothing Forecast (ESF) | Holt-Winters exponential smoothing predicts the values of each node in a space-time cube by breaking down the time series at each node into seasonal and trend components. |
Forest-based Forecast (FBF) | It uses a modified random forest prediction algorithm to predict values at each location. |
A profile chart is created on the multidimensional raster layer to examine pollution time series in different provinces of South Africa. Over the past two decades, the distribution of average PM2.5 concentrations (Figure 2) reveals that Gauteng Province had the highest mean PM2.5 concentrations (53 μg/m3) in 2010, while Western Cape had the lowest (6.59 μg/m3) in 1999. Several provinces' PM2.5 pollution levels are comparable. Most area readings exceed the South African National Ambient Air Quality Standards (NAAQS) of 20 μg/m3 for the yearly average [2]. In 2010, all provinces had an increase in mean PM2.5. The increase could be ascribed to South Africa hosting the 2010 FIFA World Cup. According to a Chilean study [36], the probability that the association between PM2.5 maxima and the 2014 FIFA World Cup is purely coincidental is 0.002%. Suggesting pollution spiked in Chile tied to the soccer event. Consequently, there is a strong association between these two gatherings, as evidenced by the prevalence of barbecues during sporting events. Due to the distances between match venues in South Africa, intercity transportation emissions were also a factor after international transit [37]. PM2.5 concentrations have declined in most provinces, except for an increase in Eastern Cape, Northern Cape, Western Cape, and Free State provinces after 2010.
The Mann-Kendall trend test was used for the independent spatio-temporal column time series at each place within the PM2.5 space-time cube. The spatio-temporal bars of the first period are being compared to the spatio-temporal bars of the second period in terms of their values. If the value of the former is smaller than the latter's, the comparison outcome is denoted as 1. If the value of the former exceeds that of the latter, the result of the comparison is represented by the integer -1. If the two entities being compared are equal, the comparison outcome will be represented by the numerical value of 0. The temporal pattern of each spatio-temporal bar is quantified using a z-score and a p-value [25].
A high z-value suggests a discernible rising trend in the sequence of PM2.5 increases. When the z-value approaches zero, a substantial change trend is absent. The significance of the change trend is then assessed and graded according to the information provided in Table 2.
Classification | Z-Score | p-value | Confidence (%) | Trend of Change |
–3 | Z < –2.58 | p < 0.01 | 99 | Downward |
–2 | –2.58 ≤ Z < –1.96 | 0.01 ≤ p < 0.05 | 95 | Downward |
–1 | –1.96 ≤ Z < –1.65 | 0.05 ≤ p < 0.1 | 90 | Downward |
0 | –1.65 ≤ Z < 1.65 | p ≥ 0.1 | – | no significant trend |
1 | 1.65 ≤ Z < 1.96 | 0.05 ≤ p < 0.1 | 90 | Upward |
2 | 1.96 ≤ Z < 2.58 | 0.01 < p ≤ 0.05 | 95 | Upward |
3 | Z ≥ 2.58 | p ≤ 0.1 | 99 | Upward |
The hierarchical visualisation of the data obtained from the Mann-Kendall trend test is depicted in Figure 3, with the values of Trend Bin being used as the basis for the hierarchy. The figure illustrates the presence of green regions, which correspond to the PM2.5 data bars exhibiting a declining trend. Out of the 9907 data cube locations representing PM2.5 sites, 1099 data cube locations showed a discernible decrease trend. In general, the spatial distribution of these declining space-time cube bars is mainly concentrated in Mpumalanga (36.6%), Limpopo (16.5%), KwaZulu Natal (10.7%), and in the Gauteng region, comprising 1.8% of the total percentage. The areas in purple in Figure 3 exhibit a temporal and spatial increase in PM2.5 levels. No significant trend occurred in the 6762 data cube locations, accounting for 68.3%. Using the map in Figure 3, it is possible to see the overall trends of PM2.5 in the different South African provinces. Over this period (1998–2022), annual PM2.5 has steadily declined in Limpopo, North West, Gauteng, Mpumalanga, and Kwa-Zulu Natal regions to the point where it is now consistently below the long-term average. In the Northern Cape and Eastern Cape provinces, the PM2.5 has increased. Annual PM2.5 trends in the Western Cape and Free State provinces are more challenging to identify as the upward and downward trends are balanced, but it appears they have slowly but steadily increased.
Areas with no significant trend in the central area (Figure 3) are identified as Intensifying Hot Spots in Figure 4. This might seem to show a discrepancy, but the difference lies in the focus of the two methods. The Mann-Kendall trend test focuses on trends in the data values over time, while the Emerging Hot Spot Analysis focuses on the spatial clustering of these values and how it changes over time. Therefore, an area could show no significant trend in the Mann-Kendall trend test (meaning the values do not consistently increase or decrease over time), but be identified as an "Intensifying Hot Spot" in the Emerging Hot Spot Analysis. This could occur if the area has been a significant hot spot for most of the time steps and the intensity of clustering in each time step is significantly increasing. This means that even though the values themselves may not show a consistent trend over time, the spatial clustering of these values is becoming more intense, which is what the Emerging Hot Spot Analysis is designed to detect.
This study utilised the space-time cube representation of PM2.5 concentrations in South Africa to identify areas with elevated and reduced levels of PM2.5 dispersion. It also examined the dynamic aggregation characteristics of these areas from 1998 to 2022. The findings indicate that 9.3% of sites were Intensifying Hot Spots, 6.9% were Persistent Hot Spots, 2.7% were Diminishing Hot Spots, 21.4% were Persistent Cold Spots, and 42.6% exhibited no discernible pattern (Figure 4). Hotspots were notably concentrated in provinces such as North West, Free State, KwaZulu-Natal, Gauteng, Mpumalanga, and Limpopo's foothills, which collectively account for over fifty percent of South Africa's population [38]. Specifically, in Mpumalanga, hotspots were observed around Mbombela, Secunda, Ermelo, Delmas, and Ogies, regions known for a notable density of coal-fired power plants. Emissions from 14 facilities in these areas resulted in 305 to 650 premature fatalities in 2016 [39]. Similarly, the Gauteng conurbation, South Africa's most densely populated province, exhibited the largest concentration of anthropogenic emissions from various sources [40]. Weather patterns transport contaminated air, particularly from Mpumalanga's power stations and biomass burning, to major metropolises like Johannesburg and the country's capital, Pretoria, in Gauteng province [41]. Persistent Hotspot areas in the North West Province include Rustenburg, Brits, Lichtenburg, and Sun City. The provinces of North West, Gauteng, Mpumalanga, and portions of Limpopo persistently exhibited elevated PM2.5 levels for 90% of the period from 1998 to 2022.
In the Free State, Sasolburg town is classified as an Intensifying Hot Spot, Harrismith town as a Persistent Hot Spot, and Petrusburg and Boshof towns as Sporadic Hot Spots. Air quality in Sasolburg is influenced by the existence of coal and the making of liquid fuels out of coal [42]. PM2.5 concentrations range from Persistent, Intensifying, Consecutive, and Sporadic Hot spots from the western portions of KwaZulu Natal to Durban. KwaZulu Natal and Free State are generally designated as Sporadic Hot Spots, which means they have had high PM2.5 readings less than 90% of the time during the past 25 years. In addition, they have never had statistically low PM2.5 levels. The statistically significant spatial clusters of high values (Hot Spots) identified in the above areas form part of the Vaal Triangle Airshed Priority Area (VTAPA). The VTAPA is confronted with intricate and enduring air pollution challenges due to its proximity to various commercial, agricultural, residential, industrial, and mining activities [43]. It was estimated that the source group comprising industrial, mining, and institutional fuel-burning in the Vaal triangle accounted for 65% of the cases of chronic bronchitis [44].
Western Cape, Northern Cape, and Eastern Cape are statistically insignificant. Hence, no pattern for PM2.5 was identified. South Africa's southern and western coastline regions have the lowest quantities of pollution, whereas inland cities have the highest concentrations. Coastal regions exhibit lower exposure levels to emissions from power plants compared to inland urban areas. In their study, Adeyemi et al. [41] observed the presence of air masses that originated from countries located to the north of South Africa. These air masses were found to migrate inland due to biomass-burning activities primarily conducted for space heating. The researchers further noted that these air masses have a significant impact on the air quality conditions, particularly in the city of Johannesburg, as compared to coastal regions [41,42,44]. The geographical disparities in emission sources between inland and coastal areas exhibit variations. For instance, in provinces such as KwaZulu Natal and Western Cape, the emission sources are susceptible to the influence of transboundary air pollution, which occurs when air masses from neighbouring jurisdictions settle in these areas. Conversely, inland emissions predominantly affect Johannesburg, although transboundary sources also contribute to the overall pollution levels [45].
In Figure 5, the vertical columns extending from the bottom to the top illustrate the evolution of PM2.5 values over time. The bottom cube represents the year 1998, and the top cube represents the year 2022. Red blocks represent locations and times with elevated PM2.5 readings. Blue blocks represent low-level values. Figure 5(a) depicts a Diminishing Hot Spot at eMahlaleni, where values have declined to below 30 μg/m3 since 2012. eMahlaleni is located inside the highveld priority area, which is characterised by intensive mining, coal-fired power plants, and industry. Similarly, a study by Matandirotya and Burger [46] identified a decreasing trend for PM2.5 values with a confidence interval of p0.001 in eMahlaleni. A Diminishing Hot Spot has been a statistically significant hot spot for 90% of the time-step intervals, including the last time step. In addition, the level of clustering in each time step is statistically and significantly decreasing over time. In Figure 5(b), Sasolburg shows space-time cubes are Hot and increasing over time. Sebokeng, as illustrated in Figure 5(c), is a Persistent Hot Spot characterised by relatively stable values (39.9 to 49.1 μg/m3) across the entire time series, exhibiting minimal variation. Sebokeng and Sasolburg are constituent localities within the Vaal Triangle, an extensively industrialised region officially classified as a priority area for air pollution mitigation. This designation is primarily motivated by public health concerns arising from the region's elevated levels of air pollution, as acknowledged by Shikwambana et al. [47]. One enduring consequence of the planning policies implemented during the Apartheid era was the deliberate clustering of industry and working-class neighbourhoods near one another. This was helpful in the 1960s and 1970s. However, the subsequent population growth in these towns and the concurrent rise in industrial production have given rise to a significant environmental predicament [48]. The main contributors to PM2.5 air pollution in Sebokeng include particulate matter from dust, emissions from vehicles, and the combustion of coal in residential areas [46]. Also, the regions above experience the highest amounts of PM2.5 in winter [1].
Due to the increasing demand for space heating, residential fuel consumption in low-income communities increases throughout the winter. The Sasol Chemical Industries Complex in Sasolburg is a prominent source of PM2.5 and generates secondary aerosols in surrounding areas [49]. During the winter season, a significant portion of the trajectory, approximately 75%, is influenced by an air mass originating from northern South Africa. This air mass follows a brief journey through Botswana and the mining regions of the North West Province. This observation highlights the contribution of both local and regional sources of pollutants. The summer/autumn air mass originates from Mozambique and traverses the mining and industrial districts of the Mpumalanga region along its trajectory. The predominant air mass is responsible for most (55%) of the arriving trajectories towards the regions next to Sasolburg and Sebokeng [49]. The presence of these air masses may influence the observed high concentrations of PM2.5 in certain places. Figure 5(d) depicts a Consecutive Hot Spot in Edendale, where statistically significant hot spots have occurred continuously over numerous years. The PM2.5 concentration ranges from 23.98 to 33.15 μg/m3. The areas close to Thabazimbi (Figure 5(e)) are Sporadic Hot Spots, where an "on-again, off-again hot spot" is depicted by a switch between lighter and darker colours multiple times over the time series. The PM2.5 concentration ranges from 17.32 to 33.15 μg/m3.
In this study, South Africa's PM2.5 yearly average concentration is projected to 2029 using the Time Series Forecasting tool. After applying three forecasting algorithms to the space-time cube of PM2.5 concentrations, the Evaluate Forecast by Location tool determines the ideal forecast for each area in South Africa. The outcome is a hybrid forecast in which each site is predicted using the optimal technique. The Vaal Triangle, particularly Vereeniging, is notorious for its air pollution and respiratory diseases [50]. The major causes of pollution include mining, energy production, and other industrial activities.
With a Validation Root Mean Square Error of 1.45 μg/m3, the Curve Fit Forecast tool determined that the exponential curve type best fits Vereeniging. The blue dots in the graph of Figure 6 represent the actual measured PM2.5 values, while the orange dots represent the fitted and predicted values. The South African map (top right corner) in Figure 6 has a heavier shade of blue, indicating places with high PM2.5 forecasts, while the lighter shade of blue indicates areas with low PM2.5 forecasts. The map in Figure 6 displays the results of the Exponential Smoothing Forecast on PM2.5 data in South Africa. Figure 6 also displays the PM2.5 time series graph that exhibits cyclic increases and decreases for Vereeniging (green dot on the map).
A confidence interval is also supplied to show the forecast's reliability. The fluctuating trend in Vereeniging increases the confidence interval. The approach produces a 90% confidence interval for the estimates, represented by the orange translucent cone surrounding the predicted line in Figure 6.
The map in Figure 7 depicts PM2.5 prediction for South Africa to 2029 using a Forest-based Forecast. The darker shades represent higher PM2.5 concentrations, while lighter shades represent lower concentrations. Figure 7 also shows the pop-up graph displaying Vereeniging's forecast values and confidence interval (green dot on the map). According to the graph, the overall PM2.5 concentration in Vereeniging is anticipated to remain above the South African annual air quality guideline of 20 μg/m3. It will not meet the current national PM2.5 standard within the forecasted years.
Different areas in South Africa exhibit varying upward and downward trend patterns. Hence, a single forecast model cannot be applied to all areas. Once all three forecasting algorithms have been used for the dataset, the Evaluate Forecasts by Location tool becomes instrumental in discerning the most suitable forecast for each location. The result is a hybrid forecast that employs the most appropriate technique for predicting PM2.5 levels across various areas in South Africa. Figure 8 demonstrates that the Forest-based Forecast can accurately capture the complex trends and patterns of PM2.5 concentrations in Vereeniging.
In this study, the performance of three distinct models was assessed using validation RMSE, which measures the models' forecasting capability during the validation run. According to the data presented in Figure 8 and Table 3, it can be observed that the validation RMSE values at Vereeniging were the lowest for the Forest-based Forecast model (1.38), followed by Curve Fit (1.45) and Exponential Smoothing (1.57). This suggests that the Forest-based Forecast model exhibits higher prediction accuracy, aligning with the findings of [51], who also reported superior performance of forest-based models in predicting air quality parameters.
Indicators | Models | ||
CFF | ESF | FBF | |
FRMSE | 1.96 | 2.01 | 1.18 |
VRMSE | 1.45 | 1.57 | 1.38 |
This suggests that the Forest-based Forecast model exhibits higher prediction accuracy. A larger root mean square error (RMSE) signifies a significant discrepancy between the observed and predicted values. Because this study adopts a spatial approach to this problem and allows these models to differ from location to location, one of the most effective methods to comprehend spatiotemporal trends is the Evaluate Forecasts by Location method. The Evaluate Forecasts by location technique reveals that complicated time series patterns in Hot Spot areas of North West, Free State, KwaZulu Natal, Gauteng, and Mpumalanga are primarily modeled using Forest-based forecasting or exponential smoothing. In South Africa, Exponential smoothing and Forest-based Forecasting account for 24.6% and 20.9% of forecasting methods, respectively. Both exponential smoothing and the random forest-based technique effectively handle complex time series, including seasonal or cyclical characteristics in the data that can make forecasting difficult, a finding that corroborates the work of Liu et al. [52]. Curve Fit Forecast is primarily used to model places with cold spots and no significant trends. Curve Fit Forecast makes up 54.5% (Exponential, 8.7; Gompertz, 23.4; Linear, 13.8; Parabolic, 8.6). The Evaluate Forecasts by Site tool allows the mapping of several forecasting models over the study region based on how well they correspond to each location. If the PM2.5 levels exhibited a consistent pattern throughout all regions in South Africa, it would be anticipated that the map in Figure 8 would predominantly display a uniform colour. Given the absence of this circumstance, it becomes evident that the process of modeling PM2.5 is highly intricate, underscoring the significance of possessing multiple methods available and the tools to evaluate them. This finding corroborates previous research highlighting the multifaceted nature of air quality forecasting and the importance of employing diverse modelling techniques [53]. Observing different locations for which the same model is applied provides significant insight into the likelihood that these locations also share comparable characteristics.
The primary contribution of this work is the investigation of spatio-temporal dynamic 3D properties of PM2.5 concentrations at a high resolution using emerging geographical and temporal hot spots. This study shows fine particulate matter remains a significant concern, particularly in the North West, Gauteng, Free State, and Mpumalanga. The temporal profile chart and space-time cube depicted an overall decrease in PM2.5 concentration across the research period. The Mann-Kendall trend test shows downward trending space-time cube bars are mainly distributed in Mpumalanga (36.6%) and Limpopo (16.5%). At the current rate, compliance with existing requirements will take years and decades at specific sites. The results obtained in this study are similar to those obtained by [10]. However, this study expands the literature using space-time mining analysis and locational forecasting. Space-time pattern mining tools are utilized to analyze and comprehend pollutant data. 3D visualisation enhanced comprehension of the patterns that each hot spot reflects. The Time Series Forecasting tool identifies future patterns and recommends the most appropriate statistical model to employ in a given area. Even though time series forecasting is not explicitly spatial, it is now possible to detect spatial patterns in the data by observing which model is selected at various areas, making capturing these hybrid results quite intriguing. Future studies will benefit from improved accuracy of satellite retrieved PM2.5 if monitoring stations in South Africa are optimised to have monitoring data that are more spatially representative.
The authors declare they have not used Artificial Intelligence (AI) tools in the creation of this article.
The data was obtained from an open-access worldwide database provided by Washington University in St. Louis, USA (WUSTL, 2022). Available from: https://sites.wustl.edu/acag/datasets/surface-pm2-5/.
The author confirms that no known conflict of interest is associated with this publication, and there has been no financial support for this work that could have influenced its outcome.
[1] |
Katoto PDMC, Byamungu L, Brand AS, et al. (2019) Ambient air pollution and health in Sub-Saharan Africa: Current evidence, perspectives and a call to action. Environ Res 173: 174–188. https://doi.org/10.1016/j.envres.2019.03.029 doi: 10.1016/j.envres.2019.03.029
![]() |
[2] |
Edlund KK, Killman F, Molnár P, et al. (2021) Health risk assessment of PM2.5 and PM2.5-bound trace elements in Thohoyandou, South Africa. Int J Environ Res 18: 1359. https://doi.org/10.3390/ijerph18031359 doi: 10.3390/ijerph18031359
![]() |
[3] | Indoor Quality Air. Air quality in South Africa, 2022. Available from: https://www.iqair.com/south-africa. |
[4] | Zulu T, Aphane O, Audat T, et al. (2019) South Africa energy sector report. Available from: http://www.energy.gov.za/files/media/explained/2019-South-African-Energy-Sector-Report.pdf. |
[5] |
Zhang R, Di B, Luo Y, et al. (2018) A nonparametric approach to filling gaps in satellite-retrieved aerosol optical depth for estimating ambient PM2.5 levels. Environ Pollut 243: 998–1007. https://doi.org/10.1016/j.envpol.2018.09.052 doi: 10.1016/j.envpol.2018.09.052
![]() |
[6] |
Yan JW, Tao F, Zhang SQ, et al. (2021) Spatiotemporal distribution characteristics and driving forces of PM2.5 in three urban agglomerations of the Yangtze River Economic Belt. Int J Env Res Pub He 18: 2222. https://doi.org/10.3390/ijerph18052222 doi: 10.3390/ijerph18052222
![]() |
[7] |
Chudnovsky AA, Koutrakis P, Kloog I, et al. (2014) Fine particulate matter predictions using high-resolution aerosol optical depth (AOD) retrievals. Atmos Environ 89: 189–198. https://doi.org/10.1016/j.atmosenv.2014.02.019 doi: 10.1016/j.atmosenv.2014.02.019
![]() |
[8] |
Stowell JD, Bi J, Al-Hamdan MZ, et al. (2020) Estimating PM2.5 in Southern California using satellite data: Factors that affect model performance. Environ Res Lett 15: 094004. https://doi.org/10.1088/1748-9326/ab9334 doi: 10.1088/1748-9326/ab9334
![]() |
[9] |
Hu X, Waller LA, Al-Hamdan MZ, et al. (2013) Estimating ground-level PM2.5 concentrations in the southeastern U.S. using geographically weighted regression. Environ Res 121: 1–10. https://doi.org/10.1016/j.envres.2012.11.003 doi: 10.1016/j.envres.2012.11.003
![]() |
[10] |
Kneen MA, Lary DJ, Harrison WA, et al. (2016) Interpretation of satellite retrievals of PM2.5 over the southern African Interior. Atmos Environ 128: 53–64. https://doi.org/10.1016/j.atmosenv.2015.12.016 doi: 10.1016/j.atmosenv.2015.12.016
![]() |
[11] |
Muyemeki L, Burger R, Piketh SJ (2020) Evaluating the potential of remote sensing imagery in mapping ground-level fine particulate matter (PM25) for the Vaal triangle priority area. Clean Air J 30: 1–7. https://doi.org/10.17159/caj/2020/30/1.8066 doi: 10.17159/caj/2020/30/1.8066
![]() |
[12] |
Hu X, Belle JH, Meng X, et al (2017) Estimating PM2.5 concentrations in the conterminous United States using the random forest approach. Environ Sci Technol 51: 6936–6944. https://doi.org/10.1021/acs.est.7b01210.s001 doi: 10.1021/acs.est.7b01210.s001
![]() |
[13] |
van Donkelaar A, Hammer M, Bindle L, et al. (2021) Monthly global estimates of fine particulate matter and their uncertainty. Environ Sci Technol 55: 15287–15300. https://doi.org/10.1021/acs.est.1c05309 doi: 10.1021/acs.est.1c05309
![]() |
[14] |
Knibbs LD, van Donkelaar A, Martin RV, et al. (2018) Satellite-based land-use regression for continental-scale long-term ambient PM2.5 exposure assessment in Australia. Environ Sci Technol 52: 12445–12455. https://doi.org/10.1021/acs.est.8b02328 doi: 10.1021/acs.est.8b02328
![]() |
[15] |
de Hoogh K, Gulliver J, van Donkelaar A, et al. (2016) Development of West-European PM2.5 and NO2 land use regression models incorporating satellite-derived and chemical transport modelling data. Environ Res 151: 1–10. https://doi.org/10.1016/j.envres.2016.07.005 doi: 10.1016/j.envres.2016.07.005
![]() |
[16] |
Hammer MS, van Donkelaar A, Li C, et al. (2020) Global estimates and long-term trends of fine particulate matter concentrations (1998–2018). Environ Sci Technol 54: 7879–7890. https://doi.org/10.1021/acs.est.0c01764 doi: 10.1021/acs.est.0c01764
![]() |
[17] |
van Donkelaar A, Martin RV, Li C, et al. (2019) Regional estimates of chemical composition of fine particulate matter using a combined geoscience-statistical method with information from satellites, models, and monitors. Environ Sci Technol 53: 2595. https://doi.org/10.1021/acs.est.8b06392 doi: 10.1021/acs.est.8b06392
![]() |
[18] |
Fenderson LE, Kovach AI, Llamas B (2020) Spatiotemporal landscape genetics: Investigating ecology and evolution through space and time. Mol Ecol 29: 218–246. https://doi.org/10.1111/mec.15315 doi: 10.1111/mec.15315
![]() |
[19] | Osman A, Owusu AB, Adu-Boahen K, et al. (2023) Space-time cube approach in analysing conflicts in Africa. Soc Sci Humanit Open 8. https://doi.org/10.1016/j.ssaho.2023.100557 |
[20] |
Yoon J, Lee S (2021) Spatio-temporal patterns in pedestrian crashes and their determining factors: Application of a space-time cube analysis model. Accident Anal Prev 161. https://doi.org/10.1016/j.aap.2021.106291 doi: 10.1016/j.aap.2021.106291
![]() |
[21] |
Allen MJ, Allen TR, Davis C (2021) Exploring spatial patterns of Virginia tornadoes using kernel density and space-time cube analysis (1960–2019). ISPRS Int J Geo-Inf 10: 310. https://doi.org/10.3390/ijgi10050310 doi: 10.3390/ijgi10050310
![]() |
[22] |
Mo C, Tan D, Mai T, et al. (2020) An analysis of spatiotemporal pattern for COVID-19 in China based on space‐time cube. J Med Virol 92: 1587–1595. https://doi.org/10.1002/jmv.25834 doi: 10.1002/jmv.25834
![]() |
[23] | South African Yearbook (2021) South Africa Yearbook 2021/22. Available: https://www.gcis.gov.za/south-africa-yearbook-202122. |
[24] | WUSTL (Washington University in St. Louis) (2022) Atmospheric composition analysis group-surface PM2.5. Available from: https://sites.wustl.edu/acag/datasets/surface-pm2-5/. |
[25] | ESRI (2022) How Emerging Hot Spot Analysis Works. Available from: https://pro.arcgis.com/en/pro-app/latest/tool-reference/space-time-pattern-mining/learnmoreemerging.htm. |
[26] |
Malik A, Kumar A, Pham QB, et al. (2020) Identification of EDI trend using Mann-Kendall and innovative trend methods (Uttarakhand, India). Arab J Geosci 13: 951. https://doi.org/10.1007/s12517-020-05926-2 doi: 10.1007/s12517-020-05926-2
![]() |
[27] |
Cui J, Liu Y, Sun J, et al. (2021) G-STC-M spatiotemporal analysis method for archaeological sites. ISPRS Int J Geo-Inf 10: 312. https://doi.org/10.3390/ijgi10050312 doi: 10.3390/ijgi10050312
![]() |
[28] |
Zhang H, Tripathi NK (2018) Geospatial hot spot analysis of lung cancer patients correlated to fine particulate matter (PM2.5) and industrial wind in Eastern Thailand. J Clean Prod 170: 407–424. https://doi.org/10.1016/j.jclepro.2017.09.185 doi: 10.1016/j.jclepro.2017.09.185
![]() |
[29] |
Harris NL, Goldman C, Gabris J, et al. (2017) Using spatial statistics to identify emerging hot spots of forest loss using spatial statistics to identify emerging hot spots of forest loss. Environ Res Lett 12. https://doi.org/10.1088/1748-9326/aa5a2f doi: 10.1088/1748-9326/aa5a2f
![]() |
[30] |
Wan Y, Beydoun MA (2007) The obesity epidemic in the United States—gender, Age, socioeconomic, racial/ethnic, and geographic characteristics: A systematic review and meta-regression analysis. Epidemiol Rev 29. https://doi.org/10.1093/epirev/mxm007 doi: 10.1093/epirev/mxm007
![]() |
[31] | Barazzetti L, Previtali M, Roncoroni F (2022) Visualisation and processing of structural monitoring data using space-time cubes, International Conference on Computational Science and Its Applications, Springer, Cham. https://doi.org/10.1007/978-3-031-10450-3_2 |
[32] |
Zhou R, Chen H, Chen H, et al. (2021) Research on traffic situation analysis for urban road network through spatiotemporal data mining: A case study of Xi'an, China. IEEE Access 9: 75553–75567. https://doi.org/10.1109/access.2021.3082188 doi: 10.1109/access.2021.3082188
![]() |
[33] |
Cherchi E, Cirillo C (2010) Validation and forecasts in models estimated from multiday travel survey. Transport Res Rec 2175: 57–64. https://doi.org/10.3141/2175-07 doi: 10.3141/2175-07
![]() |
[34] | Arsham H (2020) Time-critical decision-making for business administration. Time Series Ana Bus Forecast. |
[35] | ESRI (2023) Train time series forecasting model. Available from: https://pro.arcgis.com/en/pro-app/latest/tool-reference/geoai/train-time-series-forecasting-model.htm. |
[36] |
Lapere R, Menut L, Mailler S, et al. (2020) Soccer games and record-breaking PM2.5 pollution events in Santiago, Chile. Atmos Chem Phys 20: 4681–4694. https://doi.org/10.5194/acp-20-4681-2020 doi: 10.5194/acp-20-4681-2020
![]() |
[37] | van der Merwe C (2010) The World Cup's 2, 7 MT carbon footprint and what's being done about it. Available from: https://www.engineeringnews.co.za/article/the-world-cups-27-million-ton-carbon-footprint-2010-01-22. |
[38] | Paul M (2022) Different air under one sky: Almost everyone in South Africa breathes polluted air. Available from: https://www.downtoearth.org.in/news/health-in-africa/different-air-under-one-sky-almost-everyone-in-south-africa-breathes-polluted-air-84743. |
[39] | Gray HA (2019) Air quality impacts and health effects due to sizeable stationary source emissions in and around South Africa's Mpumalanga highveld priority area, San Rafael, CA USA: Gray Sky Solutions. |
[40] |
Zhang D, Du L, Wang W, et al. (2021) A machine learning model to estimate ambient PM2.5 concentrations in industrialized highveld region of South Africa. Remote Sens Environ 266: 112713. https://doi.org/10.1016/j.rse.2021.112713 doi: 10.1016/j.rse.2021.112713
![]() |
[41] |
Adeyemi A, Molnar P, Boman J, et al. (2022) Particulate matter (PM2.5) characterization, air quality level and origin of air masses in an urban background in Pretoria. Arch Environ Con Tox 83: 77–94. https://doi.org/10.1007/s00244-022-00937-4 doi: 10.1007/s00244-022-00937-4
![]() |
[42] |
Mollo VM, Nomngongo PN, Ramontja J (2022) Evaluation of surface water quality using various indices for heavy metals in Sasolburg, South Africa. Water 14: 2375. https://doi.org/10.3390/w1415237 doi: 10.3390/w1415237
![]() |
[43] |
Moreoane L, Mukwevho P, Burger R (2021) The quality of the first and second Vaal triangle airshed priority area air quality management plans. Clean Air J 31: 1–14. https://doi.org/10.17159/caj/2020/31/2.12178 doi: 10.17159/caj/2020/31/2.12178
![]() |
[44] |
Scorgie Y, Kneen A, Annegarn HJ, et al. (2003) Air pollution in the Vaal triangle-quantifying source contributions and identifying cost-effective solutions. Clean Air J 13: 5–18. https://doi.org/10.17159/caj/2003/13/2.7152 doi: 10.17159/caj/2003/13/2.7152
![]() |
[45] |
Venter AD, Beukes JP, Van Zyl PG (2012) An air quality assessment in the industrialised western Bushveld Igneous Complex, South Africa. S Afr J Sci 108: 1–10. https://doi.org/10.4102/sajs.v108i9/10.1059 doi: 10.4102/sajs.v108i9/10.1059
![]() |
[46] |
Matandirotya NR, Burger R (2023) An assessment of NO2 atmospheric air pollution over three cities in South Africa during 2020 COVID-19 pandemic. Air Qual Atmos Hlth 16: 263–276. https://doi.org/10.1007/s11869-022-01271-3 doi: 10.1007/s11869-022-01271-3
![]() |
[47] |
Shikwambana L, Mhangara P, Mbatha N (2020) Trend analysis and first time observations of sulphur dioxide and nitrogen dioxide in South Africa using TROPOMI/Sentinel-5 P data. Int J Appl Earth Obs 91. https://doi.org/10.1016/j.jag.2020.102130 doi: 10.1016/j.jag.2020.102130
![]() |
[48] | Department of Environmental Affairs (DEA) (2019) The second generation Vaal triangle airshed priority area air quality management plan: Draft baseline assessment report, Pretoria: DEA. |
[49] | Norman R, Cairncross E, Witi J, et al. (2007) Estimating the burden of disease attributable to urban outdoor air pollution in South Africa in 2000. S Afr Med J 97: 748–753. |
[50] |
Muyemeki L, Burger R, Piketh SJ, et al. (2021) Source apportionment of ambient PM10-25 and PM2.5 for the Vaal triangle, South Africa. S Afr J Sci 117: 1–11. https://doi.org/10.17159/sajs.2021/8617 doi: 10.17159/sajs.2021/8617
![]() |
[51] |
Oosthuizen MA, Mundackal AJ, Wright CY (2014) The prevalence of asthma among children in South Africa is increasing-is the need for medication increasing as well? A case study in the Vaal triangle. Clean Air J 24: 28–30. https://doi.org/10.17159/caj/2014/24/1.7050 doi: 10.17159/caj/2014/24/1.7050
![]() |
[52] |
Liu H, Yan G, Duan Z, et al. (2021) Intelligent modeling strategies for forecasting air quality time series: A review. Appl Soft Comput 102. https://doi.org/10.1016/j.asoc.2020.106957 doi: 10.1016/j.asoc.2020.106957
![]() |
[53] |
Gilliam RC, Hogrefe C, Rao ST (2006) New methods for evaluating meteorological models used in air quality applications. Atmos Environ 40: 5073–5086. https://doi.org/10.1016/j.atmosenv.2006.01.023 doi: 10.1016/j.atmosenv.2006.01.023
![]() |
Tool | Description |
Curve Fit Forecast (CFF) | Curve fitting is used to forecast the values of each. Linear, parabolic, exponential, or S-shaped curves are all possible (Gompertz). |
Evaluate Forecasts by Location | This allows various Time Series Forecasting tools with the same data to select the best forecast for each location. |
Exponential Smoothing Forecast (ESF) | Holt-Winters exponential smoothing predicts the values of each node in a space-time cube by breaking down the time series at each node into seasonal and trend components. |
Forest-based Forecast (FBF) | It uses a modified random forest prediction algorithm to predict values at each location. |
Classification | Z-Score | p-value | Confidence (%) | Trend of Change |
–3 | Z < –2.58 | p < 0.01 | 99 | Downward |
–2 | –2.58 ≤ Z < –1.96 | 0.01 ≤ p < 0.05 | 95 | Downward |
–1 | –1.96 ≤ Z < –1.65 | 0.05 ≤ p < 0.1 | 90 | Downward |
0 | –1.65 ≤ Z < 1.65 | p ≥ 0.1 | – | no significant trend |
1 | 1.65 ≤ Z < 1.96 | 0.05 ≤ p < 0.1 | 90 | Upward |
2 | 1.96 ≤ Z < 2.58 | 0.01 < p ≤ 0.05 | 95 | Upward |
3 | Z ≥ 2.58 | p ≤ 0.1 | 99 | Upward |
Indicators | Models | ||
CFF | ESF | FBF | |
FRMSE | 1.96 | 2.01 | 1.18 |
VRMSE | 1.45 | 1.57 | 1.38 |
Tool | Description |
Curve Fit Forecast (CFF) | Curve fitting is used to forecast the values of each. Linear, parabolic, exponential, or S-shaped curves are all possible (Gompertz). |
Evaluate Forecasts by Location | This allows various Time Series Forecasting tools with the same data to select the best forecast for each location. |
Exponential Smoothing Forecast (ESF) | Holt-Winters exponential smoothing predicts the values of each node in a space-time cube by breaking down the time series at each node into seasonal and trend components. |
Forest-based Forecast (FBF) | It uses a modified random forest prediction algorithm to predict values at each location. |
Classification | Z-Score | p-value | Confidence (%) | Trend of Change |
–3 | Z < –2.58 | p < 0.01 | 99 | Downward |
–2 | –2.58 ≤ Z < –1.96 | 0.01 ≤ p < 0.05 | 95 | Downward |
–1 | –1.96 ≤ Z < –1.65 | 0.05 ≤ p < 0.1 | 90 | Downward |
0 | –1.65 ≤ Z < 1.65 | p ≥ 0.1 | – | no significant trend |
1 | 1.65 ≤ Z < 1.96 | 0.05 ≤ p < 0.1 | 90 | Upward |
2 | 1.96 ≤ Z < 2.58 | 0.01 < p ≤ 0.05 | 95 | Upward |
3 | Z ≥ 2.58 | p ≤ 0.1 | 99 | Upward |
Indicators | Models | ||
CFF | ESF | FBF | |
FRMSE | 1.96 | 2.01 | 1.18 |
VRMSE | 1.45 | 1.57 | 1.38 |