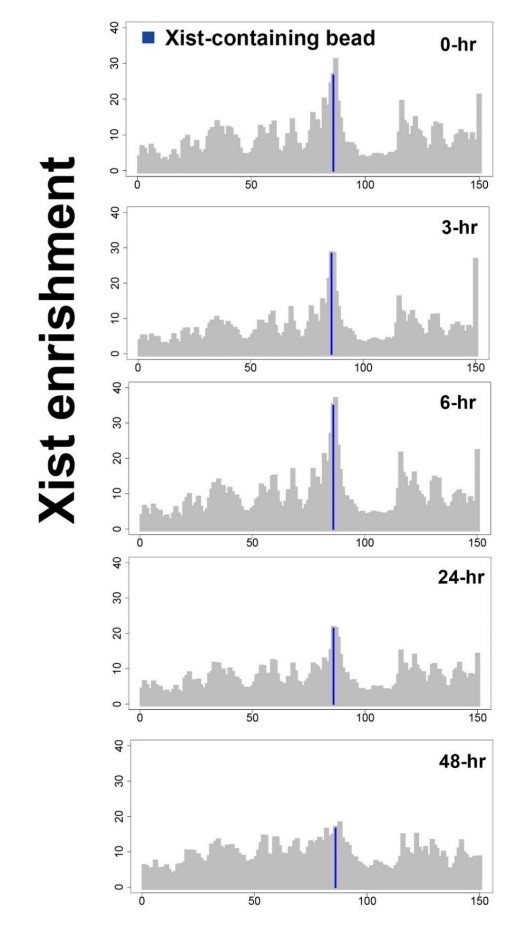
Many worldwide scientists have concentrated on using waste heat recovery technology in automotive applications because of increasingly strict fuel consumption. The thermoelectric generator (TEG) has garnered significant interest in the automobile sector as a viable waste heat recovery solution over the past several decades. A short survey of thermoelectric materials and heat exchangers for TEG systems is initially presented in this paper. To overcome the heat exchanger's current shortcomings, some previous studies designed a variety of the heat exchanger geometry of the TEG system. They suggested concentric cylindrical TEG system utilizes an annular thermoelectric module instead of the traditional square-shaped one. It uses the heat pipe's benefits to improve radial heat transmission. A comparison of the water-inside and gas-inside arrangements indicated that the water-inside concentric cylindrical TEG system provided a greater power output in our simulations to test the performance of the proposed system.
Citation: Tan Nguyen Tien, Quang Khong Vu, Vinh Nguyen Duy. Novel designs of thermoelectric generator for automotive waste heat recovery: A review[J]. AIMS Energy, 2022, 10(4): 922-942. doi: 10.3934/energy.2022042
[1] | Mohammadjavad Hosseinpoor, Hamid Parvin, Samad Nejatian, Vahideh Rezaie, Karamollah Bagherifard, Abdollah Dehzangi, Amin Beheshti, Hamid Alinejad-Rokny . Proposing a novel community detection approach to identify cointeracting genomic regions. Mathematical Biosciences and Engineering, 2020, 17(3): 2193-2217. doi: 10.3934/mbe.2020117 |
[2] | Yue Hu, Xiaoqin Mei, Dong Tang . Long non-coding RNA XIST is down-regulated and correlated to better prognosis in ovarian cancer. Mathematical Biosciences and Engineering, 2020, 17(3): 2070-2081. doi: 10.3934/mbe.2020110 |
[3] | Ping Zhou, Yuting Zhang, Yunlei Yu, Weijia Cai, Guangquan Zhou . 3D shape measurement based on structured light field imaging. Mathematical Biosciences and Engineering, 2020, 17(1): 654-668. doi: 10.3934/mbe.2020034 |
[4] | Xiaohong Tian, Rui Xu, Jiazhe Lin . Mathematical analysis of an age-structured HIV-1 infection model with CTL immune response. Mathematical Biosciences and Engineering, 2019, 16(6): 7850-7882. doi: 10.3934/mbe.2019395 |
[5] | Choonsung Shin, Sung-Hee Hong, Hieyoung Jeong, Hyoseok Yoon, Byoungsoo Koh . All-in-one encoder/decoder approach for non-destructive identification of 3D-printed objects. Mathematical Biosciences and Engineering, 2022, 19(12): 14102-14115. doi: 10.3934/mbe.2022657 |
[6] | Sukun Tian, Ning Dai, Linlin Li, Weiwei Li, Yuchun Sun, Xiaosheng Cheng . Three-dimensional mandibular motion trajectory-tracking system based on BP neural network. Mathematical Biosciences and Engineering, 2020, 17(5): 5709-5726. doi: 10.3934/mbe.2020307 |
[7] | Ning Bai, Rui Xu . Mathematical analysis of an HIV model with latent reservoir, delayed CTL immune response and immune impairment. Mathematical Biosciences and Engineering, 2021, 18(2): 1689-1707. doi: 10.3934/mbe.2021087 |
[8] | Cuicui Cai, Maosheng Fu, Xianmeng Meng, Chaochuan Jia, Mingjing Pei . Indoor high-precision visible light positioning system using Jaya algorithm. Mathematical Biosciences and Engineering, 2023, 20(6): 10358-10375. doi: 10.3934/mbe.2023454 |
[9] | Xianfeng Xu, Liping Wang, Xiaohong Cheng, Weilin Ke, Shenqiu Jie, Shen Lin, Manlin Lai, Linlin Zhang, Zhenzhou Li . Machine learning-based evaluation of application value of the USM combined with NIPT in the diagnosis of fetal chromosomal abnormalities. Mathematical Biosciences and Engineering, 2022, 19(4): 4260-4276. doi: 10.3934/mbe.2022197 |
[10] | Xudan Ma, Qijun Zhang, Haihong Zhu, Kefeng Huang, Weina Pang, Qin Zhang . Establishment and analysis of the lncRNA-miRNA-mRNA network based on competitive endogenous RNA identifies functional genes in heart failure. Mathematical Biosciences and Engineering, 2021, 18(4): 4011-4026. doi: 10.3934/mbe.2021201 |
Many worldwide scientists have concentrated on using waste heat recovery technology in automotive applications because of increasingly strict fuel consumption. The thermoelectric generator (TEG) has garnered significant interest in the automobile sector as a viable waste heat recovery solution over the past several decades. A short survey of thermoelectric materials and heat exchangers for TEG systems is initially presented in this paper. To overcome the heat exchanger's current shortcomings, some previous studies designed a variety of the heat exchanger geometry of the TEG system. They suggested concentric cylindrical TEG system utilizes an annular thermoelectric module instead of the traditional square-shaped one. It uses the heat pipe's benefits to improve radial heat transmission. A comparison of the water-inside and gas-inside arrangements indicated that the water-inside concentric cylindrical TEG system provided a greater power output in our simulations to test the performance of the proposed system.
Chromosome conformation capture (3C) [1] is a method that can be used to obtain the spatial organization of the DNA. Its data reflect the interaction frequencies between a single pair of loci on the genome ("one vs one"). The 3C-on-chip (4C) [2] technology was developed to map the interactions between a locus and all other regions ("one vs all"). The 3C-Carbon Copy (5C) [3] method was invented to detect the interactions between multiple pairs of genomic loci ("many vs many"). The interactions within the whole genome can be detected by the Hi-C technique [4], a method that combines proximity-based ligation with massively parallel sequencing to probe the three-dimensional proximation relationships within the genome ("all vs all"). Population Hi-C method is based on a population of cells and has been used in many studies, such as modeling the structures for balanced and unbalanced chromosomal rearrangements in primary human tumor samples [5], detecting the unknown 3D organization of chromosomes during human brain development [6], discovering that the 3D chromatin landscape is relatively stable once established in a particular cell type [7], and demonstrating that individual chromosomes maintain domain organization at the megabase scale [8]. Moreover, single-cell Hi-C method has been developed to reveal cell-to-cell variabilities in terms of chromosome structures [9]. Although we have developed computational approach [10] to reconstruct chromosomal 3D structures based on single-cell Hi-C data, this study will focus on using population Hi-C data due to the availability limitation of single-cell Hi-C data for X-chromosome inactivation.
The 3C, 4C, 5C, and population Hi-C data have been widely used to reconstruct the 3D structures of chromosomes by many computational methods. Duan et al. [11] constructed a 4C- based model that provided a way to observe the structure and function of a eukaryotic genome. Bau et al. [12] generated high-resolution 3D models of chromatins at megabase scale by developing an approach that combined 5C data with the Integrated Modeling Platform (IMP). Tanizawa et al. [13] explored the model organism fission yeast by utilizing an approach combining the 3C data and high throughput sequencing. The above-mentioned methods use multidimensional scaling (MDS), which model the 3D structures of chromosomes by making the Euclidean distance between each pair of beads as close as possible to the target distance that is converted from the number of 3C, 4C, or 5C contacts.
Zhang et al. [14] built the ChromeSDE method that improves the MDS objective function. Ben-Elazar et al. [15] proposed a statistical framework to reconstruct chromatin structures using a minimum set of assumptions. PASTIS [16] assumes the Hi-C contact counts following a Poisson distribution; and these contact counts decrease with the physical distances between genomic loci. Combing the Poisson counts with target distance, PASTIS was used to generate enzymes structures in different environments. Hu et al. [17] developed Bayesian probabilistic approaches BACH and BACH-MIX to study the structural variation of chromatin in a cell population using high resolution Hi-C dataset. Zou et al. [18] developed HSA, which globally searches the latent structure underlying different cleavage footprints; its robustness and accuracy outperform existing tools. Oluwadare et al. [19] developed a maximum likelihood algorithm called 3DMAX, which automatically re-estimates the conversion factor for converting interaction frequency to target distance and is more robust to structural variability and noise.
Dieter W. Heermann et al. [20] built a computational model for simulating the 3D structure of the chromosome fiber on a coarse-grained level. Depending on the high complexity for establishing large-scale chromosomes, they systematically eliminated smaller details such as nucleosomes or small-scale chromatin loops to obtain an all-scale compact globular structure rather than the small-scale linear structures. They accomplished the modeling by using coarse-grained lattice Monte Carlo [21] that puts the chromosomes chain into a large cubic and randomly selected a monomer to move to the neighboring positions.
The X-linked genes in female mammal cells are twice of those in male cells because females have two X-chromosomes, whereas males only have one. The dose of double X-linked genes may pose a great harmful influence to the individual; and mammalian females have evolved a unique way called X-chromosome inactivation (XCI) to eliminate the gene imbalance [22]. The XCI does not delete the extra X-chromosome directly but rather, spreads Xist RNA transcripts on one of two X-chromosomes to silence the redundant gene expressions [23,24,25,26]. After XCI, one X-chromosome is compressed into a compact structure and silenced in terms of gene expression in the regular condition [27].
It is important to detect the 3D structure of the inactive X-chromosome during the XCI process. Many studies are based on the structure of the active and inactive X-chromosome to study the mechanism of the X-chromosome inactivation. For example, Bonora and Disteche [28] used traditional microscopy and super-resolution technology to reveal uneven compaction of the inactive X-chromosome. Naughton et al. [29] presented a high-resolution chromatin fiber analysis of transcriptionally active and inactive X and showed that the formation of facultative heterochromatin depends on factors at a level above the 30 nm fiber and transcription does not alter bulk chromatin fiber structures. By isolating a comprehensive protein interactome for Xist RNA [30], the study of Minajigi et al. unveiled many layers of inactive X-chromosome repression and demonstrated a central role for Xist RNA in the topological organization of mammalian chromosomes. Nora et al. [31] used 5C and super-resolution microscopy to analyze the spatial organization of a 4.5 Mb region including Xist RNA on active and inactive X-chromosome. They uncovered a series of discrete 200 kb–1 Mb topologically associating domains (TADs) and the disruption of a TAD boundary causing ectopic chromosomal contacts and long-range transcriptional mis-regulation. In the study of [32], Deng et al. applied a recently developed Hi-C assay to mouse F1 hybrid systems and discovered a specific bipartite organization of the mouse inactive X-chromosome that may reveal the maintenance of gene silencing. Chaumeil et al. [33] concluded that the chromatin and genome structure influenced the epigenetic control of XCI.
In mammal genomes, Xist, a long non-coding RNA (lncRNA) in the X-chromosome, can localize on the X-chromosome and silence gene expressions by condensing the 3D structure of the X-chromosome. Engreitz et al. [34] triggered the XCI process by using tetracycline on male mouse ES cells to start the expressions of the Xist transcripts. They detected the Xist localization intensities on the entire X-chromosome at five time points: 0 hours, 3 hours, 6 hours, 24 hours, and 48 hours after tetracycline was applied by using a biochemistry experiment named RNA Antisense Purification (RAP) [34]. They have found that Xist transcripts spread to spatially proximal sites and uses its a-repeat domain to spread over the active genes when encounters a new region. A-repeat domain may allow Xist to recruit PRC2 or other proteins to modify and compact chromatin that reposition nearby regions into the Xist RNA compartment. Through this process, Xist compartment will grow that pull the interacted region closer to the Xist transcription locus to propagate Xist spreading [34]. They have discovered that the regions with higher localization intensity of Xist usually have shorter spatial distances with the Xist locus (a significant correlation between the number of Hi-C contacts and Xist localization intensities). Recent conformation capture approaches [35] have generated the Hi-C data of the X-chromosome 0 hours and 48 hours after doxycycline induction (the start and end of the XCI process) that are associated with the active and inactive X-chromosomes, respectively. However, the Hi-C data of the X-chromosome during the process of XCI are still not available so that the 3D structures of the X-chromosome during XCI are still unknown.
In this study, we represented a chromosome as beads-on-a-string. Unlike other existing methods, we put it into a 3D cubic lattice and then conducted Metropolis-Hasting simulations. Based on the Xist RAP data, we then inferred the 3D structures of the X-chromosome at 0 hours, 3 hours, 6 hours, 24 hours, and 48 hours after the start of XCI.
The Hi-C data of Cast (Xa, or active X-chromosome, treated as the 0 hours X-chromosome in this research) and 129s (Xi, or inactive X-chromosome, treated as the 48-hour X-chromosome after XCI) of mouse neural progenitor cells (NPC) were downloaded from Gene Expression Omnibus (GEO, https://www.ncbi.nlm.nih.gov/geo/query/acc.cgi?acc=GSE72697) with ID GSM1868576. From the same GEO entry but with IDs GSM1868576 and GSM2053973, we downloaded the Hi-C data of mouse embryonic stem cells before and after the doxycycline induction, which were used as the data before and after XCI in our research.
The Xist RAP data from GEO IDs GSM1141197 to GSM1141201 are the Xist RNA-seq data at 0 hours, 3 hours, 6 hours, 24 hours, and 48 hours after the induction of doxycycline on mouse embryonic stem cells. We downloaded these data from GEO (https://www.ncbi.nlm.nih.gov/geo/query/acc.cgi?acc=GSE46918) that were published in the study of Engreitz et al. [34], which provides RAP data for all chromosomes. We only used the RAP data on X-chromosome for our research.
A chromosome is represented as a chain of DNA beads. Each bead is the size of the resolution, e.g., 1 Mb, 500 kb, 250 kb, and 40 kb. For initialization, the program puts the first bead randomly into the cubic lattice. When putting the n-th bead (n > = 2), the n-th bead must have a distance of (2,√10) with the (n-1)-th bead [36]. Also, to prevent knotting, the n-th bead cannot be smaller than 2 or equal √8 to all previously existent beads in the cubic lattice-based on the protocol in [36]. The restriction setting between (2,√10) with an exception of √8 are excluded volume constraints and the limitations on the changes in the covalent bond length, which have been used in simulating polymer molecules such as proteins [36,37]. Here, we adopt the same protocols when modeling the 3D structure of a chromosome.
The number of lattice sites or volume of the cubic lattice is:
V=s3 | (1) |
, where s is s=5n with n as the number of beads. This bigger space (compared to setting each side of the cubic lattice to be n) allows enough free space to simulate the 3D structure.
We used a cooling schedule based on [38], in which we set the starting temperature T0=10. The decrement of temperature is:
Tc=0.9c∗T0 | (2) |
, where c is the number of times that temperature has been decremented, and Tc is the current temperature. In this way, the temperature will keep decreasing with a rate of 0.9 every time the temperature's value is updated. At the beginning of the simulation process, the value of temperature is relatively high that makes the algorithm to have higher probability to accept non-optimal moves, that is, the moves that do not reduce the value of the loss function. In this way, the algorithm allows relatively larger alternations of the 3D structure exploring bigger conformational space. This is an important feature for the simulated annealing protocol because in this way the algorithm may jump out of local minima. Towards the later stages of the simulation process, the value of temperature will be gradually reduced, which will make it stricter and stricter at accepting non-optimal moves. In this way, the algorithm will be more and more constringent, which means it will only allow small refinements of the 3D structure when the simulation is reaching the later stages. The following two equations will further explain this mechanism.
We repeated trials at each temperature until the system stabilized at that temperature. At each temperature, if on average there are 10 accepted moves per DNA bead or the number of trials exceeds 100 times of the number of beads, the algorithm will decrease the temperature based on Eq. (2) and continue running with the new temperature. If the desired acceptance number, that is, on average 10 accepted moves per bead, is not achieved for three consecutive temperatures, the annealing process is stopped.
For each trial, the algorithm randomly selects a DNA bead to move and then accepts the move with probability:
P=e−ΔE/T | (3) |
if ∆E≥0 (∆E≥0 means it is not an optimal move, i.e., it is the move makes the 3D structure to have a bigger discrepancies with the target distances between all bead pairs), or always accepts the move if ∆E < 0 (∆E < 0 means the move reduced the value of loss function, i.e., making the new 3D structure to better fit the target distances between all bead pairs), where T is the current temperature and ∆E is the change of the loss value after the move:
ΔE=L(new)−L(old) | (4) |
The loss function we used is:
L=∑ni,jw∗(dij−δ′ij)2/δ′2ij | (5) |
, where w is a parameter to scale the value of the loss value (for this study w = 0.0001), dij is the actual Euclidean distance between beads i and j in the current structure, and δij' is the normalized target distance between beads i and j. The square of the target distance δ′2ij is also put as the denominator because doing that will better normalize extremely large or small target values. The δij' is re-scaled from δij that is:
δij=c−1/3ij,cij≠0 | (6) |
, where cij is the number of normalized Hi-C contacts between beads i and j. Eq. (6) including the parameter -1/3 is a classic approach to convert the number of Hi-C contacts to Euclidean distances in the 3D space that is motivated by polymer physics. Eq. (6) has been widely used by existing Hi-C-based 3D modeling methods [11,16], in which higher Hi-C values result in smaller Euclidean target distances. We normalized the value of the target distance by using:
δ′ij=n√3δijmax(δij) | (7) |
, where n√3 is the maximum allowed target distance in the 3D structure, that is also the diagonal length of a cubic lattice whose side length is the number of beads n, and max(δij) is the maximum target distance calculated from Hi-C contacts. This normalization process makes sure that the normalized target distance will fit within the size of our cubic lattice.
The population Hi-C contact matrices may contain many zero values due to reasons such as experimental imperfection, especially at high resolution. These zero values cannot be converted to target distances by Eq. (6) (would be infinity). Therefore, we use a 2D Gaussian function Eq. (8) to impute the zero values in the Hi-C contact matrices. This mechanism is based on 2D sequential proximity between DNA bead pairs. Specifically, if one bead pair on the DNA has lots of Hi-C contacts, its sequentially adjacent bead pairs may not have zero Hi-C contact. In other words, the number of Hi-C contacts for a zero-Hi-C bead pair can be modeled based on the Hi-C contact values of its adjacent bead pairs. Specifically, it can be modeled as:
c′ij=∑cij=0,cab>0|a−i|≤d0 and |b−j|≤d0(−((a−i)2μ+(b−j)2μ))n′ | (8) |
In Eq. (8), cij indicates the original zero Hi-C value between beads i and j; c'ij indicates the imputed Hi-C value between beads i and j. The non-zero values of normalized Hi-C contact between beads a and b are used to impute the value between beads i and j. The value n' indicates the number of neighboring bead pairs whose non-zero Hi-C values are used to impute the value for beads i and j. The a and b values are the X and Y coordinates in the 2D Hi-C contact matrix, which are in the range of (i-d0, i+d0) and (j-d0, j+d0). The value d0 is a distance threshold that controls the amount of surrounding non-zero values that will be used for the imputation. The parameter μ controls how much influence a non-zero value can generate to the originally zero value. A larger μ results in smaller influence. The default values of μ and d0 are determined by the resolutions of the Hi-C data and the percentage of zero Hi-C values. The values of these parameters can be freely changed when executing our source code.
To generate a 3D structure at a high-resolution, for example, 40 kb resolution, our algorithm at first generates the 3D structure at 400 kb resolution using the protocol defined above and then adds nine beads in between every two consecutive 400 kb beads. The resulting resolution of the 3D structure will be 40 kb. The procedure of adding beads is the same as the way of initializing the 3D structure in the cubic lattice as previously mentioned.
After beads are added, the system starts new simulations on the high-resolution structure. The initial temperature for the simulations is set to 0.1, and the number of trials at each temperature is five times of the number of the beads at 40 kb resolution. The reason why we used fixed temperature and trials here is that these simulations are performed on the 400 kb structure that has already been optimized. Therefore, we would only need to refine the structure instead of largely altering it. This will also help ensure to be able to generate high-resolution structures within reasonable amount of time.
The distance of each bead pair traveling towards each other or further away from each other from 0 hours (before XCI) to 48 hours (after XCI) should equal the sum of travelled distances at each time interval, that is, from 0 hours to 3 hours, 3 hours to 6 hours, 6 hours to 24 hours, and 24 hours to 48 hours. This is modeled by the equation:
v0h_ij(3−0)+(R3h_ijR0h_ij)v0h_ij(6−3)+(R6h_ijR3h_ij)v3h_ij(24−6)+(R24h_ijR6h_ij)v6h_ij(48−24)=δ48h_ij−δ0h_ij | (9) |
In Equation (9), v0h_ij is the relative average traveling speed between beads i and j during the 0 hours to 3 hours period; (3-0) is the traveling time for beads i and j from 0 hours to 3 hours with speed v0h_ij. We used v0h_ij(3−0) to model the distance the beads i and j have traveled towards or further away from each other from 0 hours to 3 hours after XCI starts. Similarity, (R3h_ijR0h_ij)v0h_ij(6−3) is the distance beads i and j have traveled from the 3 hours to 6 hours after XCI starts, in which:
R0h_ij=(RAP0h_i+RAP0h_j)/2 | (10) |
, where RAP0h_i and RAP0h_j are the RAP values on beads i and j respectively at 0 hours that were used to indicate the intensity of Xist localization on DNA. Therefore, the average of these two values, which is the R0h_ij value in Eq. (10), is the Xist localization intensity of the beads i and j. The R3h_ij, R6h_ij, and R24h_ij in Eq. (9) are calculated using the same way.
At first, we used constant speed and assumed no acceleration for bead pairs' traveling (we would later model it with acceleration considered). We further assume that higher average RAP value on two beads will result in higher speed for the two beads' traveling:
v3h_ij=(R3h_ijR0h_ij)v0h_ij | (11) |
, where v3h_ij is the constant speed from 3 hours to 6 hours after XCI starts. This assumption is based on the study in [34] that proposes a model for how Xist exploits and alters 3D genome structure to spread across the X-chromosome. Specifically, Engreitz et al. [34] proposed that Xist exploits the existing 3D structure of the X-chromosome to search for target sites to localize. After encountering a new site, Xist transcripts bind to that region and accumulate at spatially proximal sites of active gene-dense regions. By silencing the active region into Xist silenced compartment, Xist effectively pulls new regions of active chromatin closer to the Xist transcription locus, which eventually changes the 3D conformation of the chromosome.
Similarity, we set (R6h_ijR3h_ij) as the ratio between v3h_ij and v6h_ij:
v6h_ij=(R6h_ijR3h_ij)v3h_ij | (12) |
, where v6h_ij is the constant speed from 6 hours to 24 hours after XCI starts. In Eq. (9), (R6h_ijR3h_ij)v3h_ij(24−6) is the travelled distance between beads i and j from 6 to 24 hours after XCI starts. We used the same method to calculate the distance beads i and j have travelled between 24 hours and the end of inactivation (48 hours).
The right of the equal sign in Eq. (9) indicates the total travelled distance from the beginning of XCI (0 hours) to the end of XCI (48 hours), in which δ0h_ij and δ48h_ij are the target distances between beads beads i and j at 0 hours and 48 hours that are converted from the number of normalized Hi-C contacts. From Eq. (9), we can solve the equation and get the value of v0h_ij. After that, we can calculate v3h_ij,v6h_ij, and v24h_ij, i.e., the speeds from 3 hours to 6 hours, 6 hours to 24 hours, and 24 to 48 hours. This allows us to further calculate the target distance between each bead pair at 3 hours, 6 hours, and 24 hours since we know the target distance at 0 hours and the speed and traveled time during each period of time.
Figure 1 shows the Xist localization intensity (RAP data) on the X-chromosome 0 hours, 3 hours, 6 hours, 24 hours, and 48 hours after XCI starts. It can be found that at the beginning of XCI, e.g., between 0 hours and 3 hours, the Xist locus has the highest level of Xist RNA, followed by the regions that are spatially proximate to Xist locus. We assume this high intensity of Xist localization results in higher speed of Xist pulling the proximate region towards Xist locus. In comparison, the 5' region of the X-chromosome tends to have less localized Xist RNA. Therefore, the 3D conformation at that region is not intensively altered, corresponding to smaller traveling speed at that specific region.
At 24 and 48 hours after XCI, the localization intensities of Xist transcripts in the Xist locus dropped (see Figure 1), similar to some other spatially proximal regions. In that case, our model assigns reduced traveling speed for the bead pairs in those regions. This makes sense as the 3D conformation in those regions have already been altered at the beginning of XCI. Therefore, the intensity of the 3D conformation being changed is reduced, which indicates smaller traveling speed at the later stage of XCI.
We also model the travelling of bead pairs considering a different acceleration value for every periods of time. Similarly as in Eq. (9), the distance of each bead pair that had travelled towards each other or further away from each other from 0 hours (before XCI) to 48 hours (after XCI) after XCI starts should equal the sum of travelled distances at each time period, that is, from 0 hours to 3 hours, 3 hours to 6 hours, 6 hours to 24 hours, and 24 hours to 48 hours. This is modeled by the equation:
[v0h−ij(3−0)+12⋅a0h−ij(3−0)2]+[v3h−ij⋅(6−3)+12⋅a3h−ij⋅(6−3)2]+[v6h−ij⋅(24−6)+12⋅a6h−ij⋅(24−6)2]+[v24h−ij⋅(48−24)+12⋅a24h−ij⋅(48−24)2]=δ48h−ij−δ0h−ij | (13) |
, where v0h_ij is the initial velocity of bead i and bead j at 0 hours; and we assumed it as zero. The travelling time for the first time period is (3−0) hours. The a0h_ij is the acceleration of bead i and bead j during 0 to 3 hours.
The first two terms in Eq. (13):v0h_ij⋅(3−0)+12⋅a0h_ij⋅(3−0)2 represent the travelled distance of bead i and bead j during 0 to 3 hours after XCI starts. Similarity, v3h_ij,v6h_ij, and v24h_ij are the initial velocities of bead i and bead j for three time periods: 3 to 6 hours, 6 to 24 hours, and 24 to 48 hours. The a3h_ij,a6h_ij, and a24h_ij are the accelerations for bead i and bead j in different time periods respectively.
As Xist transcripts cause the structural change of the X-chromosome, we model the acceleration with respect to the localization intensity of Xist transcripts or the RAP values as:
a3h_ij=R3h_ijR0h_ija0h_ij | (14) |
, where R0h_ij is the average Xist localization intensity of bead i and bead j at 0 hours calculated as:
R0h_ij=12⋅(RAPi_0h+RAPj_0h) | (15) |
, where parameters RAPi_0h and RAPj_0h are the Xist localization intensities for bead i and bead j at 0 hours respectively.
From Eq. (13), the value of a0h_ij can be calculated. After that, we can get all the acceleration values for all time periods. Thus, the distances of bead i and bead j approaching or moving away from each other in each XCI time period can be calculated, and we can further obtain the target distance between every bead pair at 3 hours, 6 hours, and 24 hours after XCI starts.
We resized the Hi-C data downloaded from [35] at 500 kb resolution of the active and inactive NPC X-chromosomes into 1 Mb low resolution. Figure 2 (a) shows the 1 Mb resolution Hi-C contact heatmaps of the X-chromosome of NPC. These heatmaps indicate the normalized Hi-C data, in which the "none" entries have been deleted with darker color indicating higher Hi-C values. The Hi-C matrices in Figure 2 (a) contains zero values.
Figure 2 (b) shows the heatmaps of Hi-C contacts for the NPC X-chromosome, in which the "none" entries have been removed and the zero values have been imputed by the 2D Gaussian function. Therefore, there are no zero values in the Hi-C matrices; all of the values in the Hi-C contact maps can be converted to target distances between bead pairs. Target distances are the Euclidean distances between bead pairs in the 3D space that our method uses as the target to achieve. In other words, our method tries to make the real distances between all bead pairs as close as possible to their target distances.
Figure 2 (c) shows the heatmaps of the target distances. We used these target-distance matrices as the input when modeling the 3D structures of the X-chromosome. The 3D structures were generated using the algorithms discussed in the Methods section. The structures of the active (0 hours) and inactive X-chromosomes (48 hours after Xist expression) of NPC at 1 Mb resolution are shown in Figure 2 (d).
The results for the mouse ES cells can be found in Supplementary Figure S1.
Supplementary Figure S2 shows the values of the loss function from the first trial to the last trial during the simulation process. It can be seen that our simulated annealing algorithm was able to keep reducing the loss function.
To validate the accuracy of our lattice-based model, we compared the 3D structure our method generated with the one constructed by PASTIS. We executed PASTIS using the same loss function as we used in Eq. (5) with the difference that PASTIS uses the software IPOPT [16] to solve the optimization problem.
We constructed the 1 Mb resolution 3D structure of the active X-chromosome (Xa) that was derived from neural progenitor cells (NPC) of hybrid mice [35]. In total, 40 jobs were executed independently to 40 3D structures in total. In this way, a pool of 40 structures, an ensemble, was generated. After that, we used Q-score [39] to select the top three structures. The Q-score of a target structure is the average of the pair-wise comparison, that is, the TM-score [40], between the target structure and all other structures in the pool (in this case, other 39 structures). TM-score [40] is an algorithm for measuring the structural similarity between two protein 3D structures, in which zero indicates no similarity between the two structures and one indicates that the two structures are exactly the same. It has been proven in the protein structure prediction community that the top predicted structure(s) ranked by the Q-score (also called clustering method) usually best match the native structure [41]. Therefore, Q-score has been largely used to pick up the best predicted structure from a pool of predicted protein structures. Here, we also used Q-score to pick up the most representative top three structures and then compare them with the structure generated by PASTIS.
Figure 3 (a)–(c) show our top three structures of the inactive X-chromosome at 1 Mb resolution ranked by Q-score. Figure 3 (d) shows the 3D structure constructed by PASTIS. The TM-scores between the top three structures and PASTIS structure are all 0.96. Besides using the top-three structures, we also compared all of the 40 structures with the PASTIS structure. The lowest TM-score between our 40 structures and PASTIS structure is 0.93 and the highest is 0.98 (the distribution of the 40 TM-scores is plotted in Supplementary Figure S3). These high TM-scores indicate that our lattice-based method was correctly implemented, and our approach can generate stable structures.
Figure 3 (e) shows the heatmap of the target distances for the inactive X-chromosome (48 hours) and the heatmap of the Euclidean distances parsed from the top-one 3D structure we reconstructed. The Pearson's correlation between the two heatmaps (matrices) is 0.93 with a p-value < 0.00001 indicating that our 3D modeling method successfully reconstructed the 3D structure, i.e., made a high correlation between target and actual distances in the reconstructed 3D structure. The correlations and p-values at different stages of the XCI process are shown in Supplementary Figure S4 (1 Mb resolution) and Supplementary Figure S5 (250 kb resolution).
Figure 4 (a) shows the travelling speed (assuming no acceleration) of each bead pair at different time periods during XCI. The travelling speeds between the bead that contains Xist locus and all other beads are indicated in Figure 4 (b), in which the X-axis represents every bead in the X-chromosome and the Y-axis represents the speed.
Based on the speed values shown in Figure 4 (a), we calculated the target distances at 3 hours, 6 hours, and 24 hours after XCI starts as shown in Figure 4 (c). Based on the target distances, we modeled the 1 Mb resolution structures at 3 hours, 6 hours, and 24 hours after XCI starts, as shown in Figure 4 (d).
Figure 5 shows the similar contents when acceleration is considered. Specifically, Figure 5 (a) shows the heatmaps of acceleration values at different time periods of XCI. Figure 5 (b) shows the acceleration between the Xist-locus-containing bead and all other beads. Figure 5 (c) indicates the target distances at three time points during the XCI (3 hours, 6 hours, and 24 hours after XCI starts).
Our lattice-based model can also generate high-resolution 3D structures of the X-chromosome. Figure 6 (a)–(d) show the 3D structures we reconstructed (when assuming constant travelling speed) for the NPC X-chromosome at 0 (before XCI starts), 3, 6, and 24 hours (during XCI), and 48 hours (after XCI) at 1 Mb, 500 kb, 250 kb, and 40 kb resolutions. It clearly shows how the 3D structure of the X-chromosome gradually changed into two large domains, also known as a bipartite structure. Figure 7 shows the structures when acceleration is considered.
We developed a new approach to model the chromosome 3D structure based on population Hi-C data. Our approach is based on simulations performed on a 3D lattice. Furthermore, we used the method to reconstruct the 3D structures of the X-chromosome during the XCI process. We first modeled the X-chromosome in mouse NPC cells. From 40 independent simulations, we selected the top three structures based on the Q-score and then compared them with the structure generated by PASTIS. Each of the top three structures has a TM-score of 0.96. We then compared all of the 40 structures with PASTIS structure and found that all of them have a TM-score at around 0.96. This indicates that our approach has been correctly implemented and that the structures generated by our approach are stable. The Pearson's correlation between the target distances converted from the Hi-C contact matrices and the Euclidean distances parsed from the reconstructed 3D structure at 1 Mb resolution is 0.93. It proves that our model can successfully generate the 3D structures.
We also proposed and implemented a 2D Gaussian method to model the zero values in the Hi-C contact maps, that is, making the zero values non-zero based on their sequential distances to the neighboring bead pairs that originally have non-zero Hi-C contacts. This approach can be largely used as an imputation procedure on the Hi-C contact maps.
We downloaded the Xist localization intensity data (RAP) data for NPC and ES cells at 0, 3, 6, 24, and 48 hours after XCI starts. Based on the previous finding that Xist spreads to spatially proximal site from the Xist locus and pulls the interacting segments to the Xist locus, we developed two methods to model the traveling speed and acceleration between every bead pair in the X-chromosome. The speed or acceleration of two beads traveling closer to or away from each other can be used to infer the target distances between all bead pairs at different time points in the XCI process.
Based on the traveling speed and acceleration, we further calculated the target distances between all bead pairs at different time points of XCI. Then, we reconstructed the 3D structures of the X-chromosome during XCI. These structures show how the active X-chromosome gradually changed into the inactive X-chromosome.
One may argue that the findings from [34] indicate that Xist pulls regions of active chromatin closer to the Xist transcription locus, but here our mathematical model assigns speeds not only for the bead pairs between the Xist-locus-containing bead and other beads, but also the bead pairs none of which contains Xist locus. We believe our mathematical model does not violate the previous finding that the regions of active chromatin are pulled towards the Xist locus. This is because the speed we assign to a bead pair that neither of which is Xist-locus-containing bead can be considered as the relative speed between the two beads even if both beads travel towards the Xist locus. Also, the speed in the middle of XCI process is based on the 3D structures before and after XCI (0 hours and 48 hours) that are inferred from Hi-C data. Therefore, if the spatial distance between a bead pair do change during the XCI process (even these two beads may both travel towards the Xist locus), it can be indicated by our mathematical model with a none zero speed (can be getting closer or apart). If the spatial distance between a bead pair did not change during XCI, their relative speed would be zero. Either way, it can be modeled by our mathematical model and fits the Hi-C (also the 3D structures inferred from Hi-C) before and after the XCI process.
Our 3D lattice-based approach can generate accurate and stable high-resolution 3D chromosome structures based on population Hi-C data. We used Xist localization intensity to infer the traveling speed and acceleration between DNA bead pairs during the process of XCI. We reconstructed the 3D structure of the X-chromosome at different time points of XCI. For the first time, this can show the changing of 3D structure of the X-chromosome during the XCI process.
We thank Dr. Andrew Sung from the University of Southern Mississippi for his scientific advice. We thank Michelle Sun, a high school student from Academic Magnet High School at North Charleston, South Carolina, to revise the English writing of the manuscript. This research was supported by the NIGMS grant R15GM120650 to ZW and start-up funding from the University of Miami to ZW. The content is solely the work and responsibility of the authors and does not necessarily represent the official views of the National Institutes of Health or the University of Miami.
All authors declare no conflicts of interest in this paper.
[1] |
Amar AB, Kouki AB, Cao H (2015) Power approaches for implantable medical devices. Sensors (Switzerland) 15: 28889–28914. https://doi.org/10.3390/s151128889 doi: 10.3390/s151128889
![]() |
[2] |
Jaziri N, Boughamoura A, Müller J, et al. (2020) A comprehensive review of thermoelectric generators: Technologies and common applications. Energy Rep 6: 264–287. https://doi.org/10.1016/j.egyr.2019.12.011 doi: 10.1016/j.egyr.2019.12.011
![]() |
[3] |
Bombarda P, Invernizzi CM, Pietra C (2010) Heat recovery from diesel engines: A thermodynamic comparison between Kalina and ORC cycles. Appl Therm Eng 30: 212–219. https://doi.org/10.1016/j.applthermaleng.2009.08.006 doi: 10.1016/j.applthermaleng.2009.08.006
![]() |
[4] |
Crane D, Jackson G, Holloway D (2001) Towards optimization of automotive waste heat recovery using thermoelectrics. SAE Tech Pap 1: 1–13. https://doi.org/10.4271/2001-01-1021 doi: 10.4271/2001-01-1021
![]() |
[5] |
Jaziri N, Boughamoura A, Müller J, et al. (2020) A comprehensive review of Thermoelectric generators: Technologies and common applications. Energy Rep 6: 264–287. https://doi.org/10.1016/j.egyr.2019.12.011 doi: 10.1016/j.egyr.2019.12.011
![]() |
[6] |
Karvonen M, Kapoor R, Uusitalo A, et al. (2016) Technology competition in the internal combustion engine waste heat recovery: A patent landscape analysis. J Clean Prod 112: 3735–3743. https://doi.org/10.1016/j.jclepro.2015.06.031 doi: 10.1016/j.jclepro.2015.06.031
![]() |
[7] |
Jia N, Cao J, Tan XY, et al. (2021) Thermoelectric materials and transport physics. Mater Today Phys 21: 100519. https://doi.org/10.1016/j.mtphys.2021.100519 doi: 10.1016/j.mtphys.2021.100519
![]() |
[8] |
Knecht W (2008) Diesel engine development in view of reduced emission standards. Energy 33: 264–271. https://doi.org/10.1016/j.energy.2007.10.003 doi: 10.1016/j.energy.2007.10.003
![]() |
[9] |
Saqr KM, Mansour MK, Musa MN (2008) Thermal design of automobile exhaust based Thermoelectric generators: Objectives and challenges. Int J Automot Technol 9: 155160. https://doi.org/10.1007/s12239-008-0020-y doi: 10.1007/s12239-008-0020-y
![]() |
[10] |
Zhang X, Chau KT, Chan CC (2008) Overview of Thermoelectric generation for hybrid vehicles. J Asian Electr Veh 6: 1119–1124. https://doi.org/10.4130/jaev.6.1119 doi: 10.4130/jaev.6.1119
![]() |
[11] |
Abedin MJ, Masjuki HH, Kalam MA, et al. (2013) Energy balance of internal combustion engines using alternative fuels. Renewable Sustainable Energy Rev 26: 20–33. https://doi.org/10.1016/j.rser.2013.05.049 doi: 10.1016/j.rser.2013.05.049
![]() |
[12] |
Yüksel F, Ceviz MA (2003) Thermal balance of a four stroke SI engine operating on hydrogen as a supplementary fuel. Energy 28: 1069–1080. https://doi.org/10.1016/S0360-5442(03)00090-2 doi: 10.1016/S0360-5442(03)00090-2
![]() |
[13] |
Durgun O, Şahin Z (2009) Theoretical investigation of heat balance in direct injection (DI) diesel engines for neat diesel fuel and gasoline fumigation. Energy Convers Manage 50: 43–51. https://doi.org/10.1016/j.enconman.2008.09.007 doi: 10.1016/j.enconman.2008.09.007
![]() |
[14] |
Özcan H, Söylemez MS (2006) Thermal balance of a LPG fuelled, four stroke SI engine with water addition. Energy Convers Manage 47: 570–581. https://doi.org/10.1016/j.enconman.2005.05.019 doi: 10.1016/j.enconman.2005.05.019
![]() |
[15] |
Mukai K, Iijima T, Miyazaki H, et al. (2005) The effects of design factors of the combustion chamber on heat balance in a gasoline engine. SAE Tech Pap 1: 1–9. https://doi.org/10.4271/2005-01-2021 doi: 10.4271/2005-01-2021
![]() |
[16] |
Rakopoulos CD, Giakoumis EG (2006) Second-law analyses applied to internal combustion engines operation. Prog Energy Combust Sci 32: 2–47. https://doi.org/10.1016/j.pecs.2005.10.001 doi: 10.1016/j.pecs.2005.10.001
![]() |
[17] |
Şahin Z, Durgun O (2007) Theoretical investigation of effects of light fuel fumigation on diesel engine performance and emissions. Energy Convers Manage 48: 1952–1964. https://doi.org/10.1016/j.enconman.2007.01.027 doi: 10.1016/j.enconman.2007.01.027
![]() |
[18] |
Ramadhas AS, Jayaraj S, Muraleedharan C (2006) Theoretical modeling and experimental studies on biodiesel-fueled engine. Renewable Energy 31: 1813–1826. https://doi.org/10.1016/j.renene.2005.09.011 doi: 10.1016/j.renene.2005.09.011
![]() |
[19] | Liu Y, Reitz RD (1998) Modeling of heat conduction within chamber walls for multidimensional internal combustion engine simulations. Int J Heat Mass Transf 41: 859–869. |
[20] |
Lounici MS, Loubar K, Balistrou M, et al. (2011) Investigation on heat transfer evaluation for a more efficient two-zone combustion model in the case of natural gas SI engines. Appl Therm Eng 31: 319–328. https://doi.org/10.1016/j.applthermaleng.2010.09.012 doi: 10.1016/j.applthermaleng.2010.09.012
![]() |
[21] |
Dado B, Gelbstein Y, Dariel MP (2010) Nucleation of nanosize particles following the spinodal decomposition in the pseudo-ternary Ge0.6Sn0.1Pb0.3Te compound. Scr Mater 62: 89–92. https://doi.org/10.1016/j.scriptamat.2009.09.029 doi: 10.1016/j.scriptamat.2009.09.029
![]() |
[22] |
Komisarchik G, Gelbstein Y, Fuks D (2017) Solubility of Ti in thermoelectric PbTe compound. Intermetallics 89: 16–21. https://doi.org/10.1016/j.intermet.2017.05.016 doi: 10.1016/j.intermet.2017.05.016
![]() |
[23] | Meroz O, Gelbstein Y (2018) Thermoelectric Bi2Te3-xSex alloys for efficient thermal to electrical energy conversion. Phys Chem Chem Phys 20: 4092–4099. |
[24] |
Sadia Y, Elegrably M, Ben-Nun O, et al. (2013) Submicron features in higher manganese silicide. J Nanomater 2013: 5–10. https://doi.org/10.1155/2013/701268 doi: 10.1155/2013/701268
![]() |
[25] |
Zilber T, Cohen S, Fuks D, et al. (2019) TiNiSn half-Heusler crystals grown from metallic flux for thermoelectric applications. J Alloys Comp 781: 1132–1138. https://doi.org/10.1016/j.jallcom.2018.12.165 doi: 10.1016/j.jallcom.2018.12.165
![]() |
[26] |
Suwardi A, Cao J, Hu L, et al. (2020) Tailoring the phase transition temperature to achieve high-performance cubic GeTe-based thermoelectrics. J Mater Chem A 8: 18880–18890. https://doi.org/10.1039/D0TA06013E doi: 10.1039/D0TA06013E
![]() |
[27] |
Suwardi A, Cao J, Zhao Y, et al. (2020) Achieving high thermoelectric quality factor toward high figure of merit in GeTe. Mater Today Phys 14: 100239. https://doi.org/10.1016/j.mtphys.2020.100239 doi: 10.1016/j.mtphys.2020.100239
![]() |
[28] |
Chen X, Dai W, Wu T, et al. (2018) Thin film thermoelectric materials: Classification, characterization, and potential for wearable applications. Coatings 8: 1–16. https://doi.org/10.3390/coatings8070244 doi: 10.3390/coatings8070244
![]() |
[29] |
Ren P, Liu Y, He J, et al. (2018) Recent advances in inorganic material thermoelectrics. Inorg Chem Front 5: 2380–2398. https://doi.org/10.1039/C8QI00366A doi: 10.1039/C8QI00366A
![]() |
[30] |
Wong-Ng W, Yan Y, Otani M, et al. (2015) High throughput screening tools for thermoelectric materials. J Electron Mater 44: 1688–1696. https://doi.org/10.1007/s11664-014-3519-1 doi: 10.1007/s11664-014-3519-1
![]() |
[31] |
Bhattacharya S, Madsen GKH (2015) High-throughput exploration of alloying as design strategy for thermoelectrics. Phys Rev B: Condens Matter Mater Phys 92: 1–9. https://doi.org/10.48550/arXiv.1508.03477 doi: 10.48550/arXiv.1508.03477
![]() |
[32] |
Gibbs ZM, Ricci F, Li G, et al. (2017) Effective mass and Fermi surface complexity factor from ab initio band structure calculations. Npj Comput Mater 3: 1–6. https://doi.org/10.1038/s41524-017-0013-3 doi: 10.1038/s41524-017-0013-3
![]() |
[33] |
Liu R, Chen H, Zhao K, et al. (2017) Entropy as a gene-like performance indicator promoting thermoelectric materials. Adv Mater 29: 1–7. https://doi.org/10.1002/adma.201702712 doi: 10.1002/adma.201702712
![]() |
[34] |
Fan Z, Wang H, Wu Y, et al. (2017) Thermoelectric performance of PbSnTeSe high-entropy alloys. Mater Res Lett 5: 187–194. https://doi.org/10.1080/21663831.2016.1244116 doi: 10.1080/21663831.2016.1244116
![]() |
[35] |
Fan Z, Wang H, Wu Y, et al. (2016) Thermoelectric high-entropy alloys with low lattice thermal conductivity. RSC Adv 6: 52164–52170. https://doi.org/10.1039/C5RA28088E doi: 10.1039/C5RA28088E
![]() |
[36] | Zhu Q, Wang S, Wang X, et al. (2021) Bottom-up engineering strategies for high-performance thermoelectric materials. Nano-Micro Letters 13: 1–38. Available from: https://link.springer.com/article/10.1007/s40820-021-00637-z. |
[37] |
Kinsella CE, O'Shaughnessy SM, Deasy MJ, et al. (2014) Battery charging considerations in small scale electricity generation from a thermoelectric module. Appl Energy 114: 80–90. https://doi.org/10.1016/j.apenergy.2013.09.025 doi: 10.1016/j.apenergy.2013.09.025
![]() |
[38] |
Kawajiri K, Kishita Y, Shinohara Y (2021) Life cycle assessment of thermoelectric generators (TEGs) in an automobile application. Sustainability 13: 1–13. https://doi.org/10.3390/su132413630 doi: 10.3390/su132413630
![]() |
[39] |
Karri MA, Thacher EF, Helenbrook BT (2011) Exhaust energy conversion by thermoelectric generator: Two case studies. Energy Convers Manage 52: 1596–1611. https://doi.org/10.1016/j.enconman.2010.10.013 doi: 10.1016/j.enconman.2010.10.013
![]() |
[40] |
Liu X, Deng YD, Li Z, et al. (2015) Performance analysis of a waste heat recovery thermoelectric generation system for automotive application. Energy Convers Manage 90: 121–127. https://doi.org/10.1016/j.enconman.2014.11.015 doi: 10.1016/j.enconman.2014.11.015
![]() |
[41] |
Wang Y, Dai C, Wang S (2013) Theoretical analysis of a thermoelectric generator using exhaust gas of vehicles as heat source. Appl Energy 112: 1171–1180. https://doi.org/10.1016/j.apenergy.2013.01.018 doi: 10.1016/j.apenergy.2013.01.018
![]() |
[42] |
Liu X, Li C, Deng YD, et al. (2015) An energy-harvesting system using thermoelectric power generation for automotive application. Int J Electr Power Energy Syst 67: 510–516. https://doi.org/10.1016/j.ijepes.2014.12.045 doi: 10.1016/j.ijepes.2014.12.045
![]() |
[43] |
Wang P, Ji C, Tang B, et al. (2010) Effect of common rail system on vehicle engine combustion performance. Proc-2010 Int Conf Optoelectron Image Process ICOIP 2010 1: 464–467. https://doi.org/10.1109/CCIENG.2011.6007975 doi: 10.1109/CCIENG.2011.6007975
![]() |
[44] |
Huang K, Chang H, Qin J, et al. (2011) The exploration of HCCI combustion in the high-power direct-injection diesel engine. CCIE 2011-Proc 2011 IEEE 2nd Int Conf Comput Control Ind Eng 1: 133–135. https://doi.org/10.1109/CCIENG.2011.6007975 doi: 10.1109/CCIENG.2011.6007975
![]() |
[45] |
Arsie I, Cricchio A, Pianese C, et al. (2014) A comprehensive powertrain model to evaluate the benefits of electric turbo compound (ETC) in reducing CO2 emissions from small diesel passenger cars. SAE Tech Pap 1. https://doi.org/10.4271/2014-01-1650 doi: 10.4271/2014-01-1650
![]() |
[46] |
Hatami M, Ganji DD, Gorji-Bandpy M (2014) A review of different heat exchangers designs for increasing the diesel exhaust waste heat recovery. Renewable Sustainable Energy Rev 37: 168–181. https://doi.org/10.1016/j.rser.2014.05.004 doi: 10.1016/j.rser.2014.05.004
![]() |
[47] |
Thomas J (2014) Drive cycle powertrain efficiencies and trends derived from EPA vehicle dynamometer results. SAE Int J Passeng Cars-Mech Syst 7: 1374–1384. https://doi.org/10.4271/2014-01-2562 doi: 10.4271/2014-01-2562
![]() |
[48] |
Liming F, Wenzhi G, Hao Q, et al. (2010) Heat recovery from internal combustion engine with Rankine cycle. Asia-Pacific Power Energy Eng Conf APPEEC 1: 2–5. https://doi.org/10.1109/APPEEC.2010.5448861 doi: 10.1109/APPEEC.2010.5448861
![]() |
[49] |
Mingshan W, Jinli F, Chaochen M, et al. (2011) Waste heat recovery from heavy-duty diesel engine exhaust gases by medium temperature ORC system. Sci China Technol Sci 54: 2746–2753. https://doi.org/10.1007/s11431-011-4547-1 doi: 10.1007/s11431-011-4547-1
![]() |
[50] |
Amicabile S, Lee JI, Kum D (2015) A comprehensive design methodology of organic Rankine cycles for the waste heat recovery of automotive heavy-duty diesel engines. Appl Therm Eng 87: 574–585. https://doi.org/10.1016/j.applthermaleng.2015.04.034 doi: 10.1016/j.applthermaleng.2015.04.034
![]() |
[51] |
Kobayashi H, Yaji K, Yamasaki S, et al. (2021) Topology design of two-fluid heat exchange. Struct Multidiscip Optim 63: 821–834. https://doi.org/10.48550/arXiv.2005.08870 doi: 10.48550/arXiv.2005.08870
![]() |
[52] |
Li J, Zhou L, Pan K, et al. (1995) Evaluation of the thermodynamic process of indirect injection diesel engines by the first and second law. SAE Tech Pap: 09: 1–13. https://doi.org/10.4271/952055 doi: 10.4271/952055
![]() |
[53] |
Ghazikhani M, Feyz ME, Joharchi A (2010) Experimental investigation of the exhaust gas recirculation effects on irreversibility and brake specific fuel consumption of indirect injection diesel engines. Appl Therm Eng 30: 1711–1718. https://doi.org/10.1016/j.applthermaleng.2010.03.030 doi: 10.1016/j.applthermaleng.2010.03.030
![]() |
[54] |
Ozcan H (2010) Hydrogen enrichment effects on the second law analysis of a lean burn natural gas engine. Int J Hydrogen Energy 35: 1443–1452. https://doi.org/10.1016/j.ijhydene.2009.11.039 doi: 10.1016/j.ijhydene.2009.11.039
![]() |
[55] |
Rakopoulos CD, Kyritsis DC (2001) Comparative second-law analysis of internal combustion enginge operation for methane, methanol, and dodecane fuels. Energy 26: 705–722. https://doi.org/10.1016/S0360-5442(01)00027-5 doi: 10.1016/S0360-5442(01)00027-5
![]() |
[56] |
Saidur R, Rezaei M, Muzammil WK, et al. (2012) Technologies to recover exhaust heat from internal combustion engines. Renewable Sustainable Energy Rev 16: 5649–5659. https://doi.org/10.1016/j.rser.2012.05.018 doi: 10.1016/j.rser.2012.05.018
![]() |
[57] |
Duparchy A, Leduc P, Bourhis G, et al. (2009) Heat recovery for next generation of hybrid vehicles: Simulation and design of a Rankine cycle system. 24th Int Batter Hybrid Fuel Cell Electr Veh Symp Exhib 2009, EVS 24. 2: 1408–1424. https://doi.org/10.3390/wevj3030440 doi: 10.3390/wevj3030440
![]() |
[58] |
Conklin JC, Szybist JP (2010) A highly efficient six-stroke internal combustion engine cycle with water injection for in-cylinder exhaust heat recovery. Energy 35: 1658–1664. https://doi.org/10.1016/j.energy.2009.12.012 doi: 10.1016/j.energy.2009.12.012
![]() |
[59] |
Hosseini MJ, Ranjbar AA, Sedighi K, et al. (2012) A combined experimental and computational study on the melting behavior of a medium temperature phase change storage material inside shell and tube heat exchanger. Int Commun Heat Mass Transf 39: 1416–1424. https://doi.org/10.1016/j.icheatmasstransfer.2012.07.028 doi: 10.1016/j.icheatmasstransfer.2012.07.028
![]() |
[60] |
Jamshidi N, Farhadi M, Ganji DD, et al. (2013) Experimental analysis of heat transfer enhancement in shell and helical tube heat exchangers. Appl Therm Eng 51: 644–652. https://doi.org/10.1016/j.applthermaleng.2012.10.008 doi: 10.1016/j.applthermaleng.2012.10.008
![]() |
[61] |
Xu HJ, Xing ZB, Wang FQ, et al. (2019) Review on heat conduction, heat convection, thermal radiation and phase change heat transfer of nanofluids in porous media: Fundamentals and applications. Chem Eng Sci 195: 462–483. https://doi.org/10.1016/j.ces.2018.09.045 doi: 10.1016/j.ces.2018.09.045
![]() |
[62] |
Xu HJ, Gong L, Zhao CY, et al. (2015) Analytical considerations of local thermal non-equilibrium conditions for thermal transport in metal foams. Int J Therm Sci 95: 73–87. https://doi.org/10.1016/j.ijthermalsci.2015.04.007 doi: 10.1016/j.ijthermalsci.2015.04.007
![]() |
[63] |
Boomsma K, Poulikakos D (2011) Erratum: On the effective thermal conductivity of a three-dimensionally structured fluid-saturated metal foam. Int J Heat Mass Transf 54: 746–748. https://doi.org/10.1016/S0017-9310(00)00123-X doi: 10.1016/S0017-9310(00)00123-X
![]() |
[64] |
Brinkman HC (1952) The viscosity of concentrated suspensions and solutions. J Chem Phys 20: 571. https://doi.org/10.1063/1.1700493 doi: 10.1063/1.1700493
![]() |
[65] |
Buongiorno J (2006) Convective transport in nanofluids. J Heat Transfer 128: 240–250. https://doi.org/10.1115/1.2150834 doi: 10.1115/1.2150834
![]() |
[66] |
Calmidi VV, Mahajan RL (1999) The effective thermal conductivity of high porosity fibrous metal foams. J Heat Transfer 121: 466–471. https://doi.org/10.1115/1.2826001 doi: 10.1115/1.2826001
![]() |
[67] |
Das SK, Putra N, Thiesen P, et al. (2003) Temperature dependence of thermal conductivity enhancement for nanofluids. J Heat Transfer 125: 567–574. https://doi.org/10.1115/1.1571080 doi: 10.1115/1.1571080
![]() |
[68] |
Gao L, Zhou XF (2006) Differential effective medium theory for thermal conductivity in nanofluids. Phys Lett Sect A Gen At Solid State Phys 348: 355–360. https://doi.org/10.1016/j.physleta.2005.08.069 doi: 10.1016/j.physleta.2005.08.069
![]() |
[69] |
Manohara R, Harinath MA (2019) Application of Taguchi method for optimization of process parameters in drilling operation. Int J Trend Sci Res Dev 3: 1052–1057. https://doi.org/10.31142/ijtsrd24003 doi: 10.31142/ijtsrd24003
![]() |
[70] |
Alkidas AC, Battiston PA, Kapparos DJ (2004) Thermal studies in the exhaust system of a diesel-powered light-duty vehicle. SAE Tech Pap 1: 1–21. https://doi.org/10.4271/2004-01-0050 doi: 10.4271/2004-01-0050
![]() |
[71] |
Kishore RA, Kumar P, Priya S (2018) A comprehensive optimization study on Bi2Te3 -based thermoelectric generators using the Taguchi method. Sustainable Energy Fuels 2: 175–190. https://doi.org/10.1039/C7SE00437K doi: 10.1039/C7SE00437K
![]() |
[72] |
Kang CC, Yamauchi KA, Vlassakis J, et al. (2016) Single cell-resolution western blotting. Nat Protoc 11: 1508–1530. https://doi.org/10.1038/nprot.2016.089 doi: 10.1038/nprot.2016.089
![]() |
[73] |
Silaipillayarputhur K, Idem S (2013) A general matrix approach to model steady-state performance of crossflow heat exchangers. Heat Transf Eng 34: 338–348. https://doi.org/10.1080/01457632.2013.716347 doi: 10.1080/01457632.2013.716347
![]() |
[74] |
Silaipillayarputhur K, Al Mughanam T (2018) Performance charts for multi-pass parallel crossflow heat exchangers. Int J Mech Eng Robot Res 7: 478–482. https://doi.org/10.18178/ijmerr.7.5.478-482 doi: 10.18178/ijmerr.7.5.478-482
![]() |
[75] |
Romie FE (1983) Transient response of gas-to-gas crossflow heat exchangers with neither gas mixed. J Heat Transfer 105: 563–570. https://doi.org/10.1016/0017-9310(91)90249-E doi: 10.1016/0017-9310(91)90249-E
![]() |
[76] |
Gao T, Geer J, Sammakia B (2014) Nonuniform temperature boundary condition effects on data center cross flow heat exchanger dynamic performance. Int J Heat Mass Transf 79: 1048–1058. https://doi.org/10.1016/j.ijheatmasstransfer.2014.09.011 doi: 10.1016/j.ijheatmasstransfer.2014.09.011
![]() |
[77] |
Gao T, Sammakia BG, Geer FJ, et al. (2014) Dynamic analysis of cross flow heat exchangers in data centers using transient effectiveness method. IEEE Trans Components, Packag Manuf Technol 4: 1925–1935. https://doi.org/10.1109/TCPMT.2014.2369256 doi: 10.1109/TCPMT.2014.2369256
![]() |
[78] |
Yuan P (2003) Effect of inlet flow maldistribution on the thermal performance of a three-fluid crossflow heat exchanger. Int J Heat Mass Transf 46: 3777–3787. https://doi.org/10.1016/S0017-9310(03)00196-0 doi: 10.1016/S0017-9310(03)00196-0
![]() |
[79] |
Mishra M, Das PK, Sarangi S (2008) Effect of temperature and flow nonuniformity on transient behaviour of crossflow heat exchanger. Int J Heat Mass Transf 51: 2583–2592. https://doi.org/10.1016/j.ijheatmasstransfer.2007.07.054 doi: 10.1016/j.ijheatmasstransfer.2007.07.054
![]() |
[80] |
Guo D, Gao J, Yu YJ, et al. (2014) Design and modeling of a fluid-based micro-scale electrocaloric refrigeration system. Int J Heat Mass Transf 72: 559–564. https://doi.org/10.1016/j.ijheatmasstransfer.2014.01.043 doi: 10.1016/j.ijheatmasstransfer.2014.01.043
![]() |
[81] |
Jia Y, Sungtaek Ju Y (2012) A solid-state refrigerator based on the electrocaloric effect. Appl Phys Lett 100. https://doi.org/10.1063/1.4729038 doi: 10.1063/1.4729038
![]() |
[82] | Ossmer H, Wendler F, Gueltig M, et al. (2016) Energy-efficient miniature-scale heat pumping based on shape memory alloys. Smart Mater Struct 25: 1–13. Avaiable from: https://iopscience.iop.org/article/10.1088/0964-1726/25/8/085037. |
[83] |
Park SIl (1996) Performance analysis of a moving-bed heat exchanger in vertical pipes. Energy 21: 911–918. https://doi.org/10.1016/0360-5442(96)00027-8 doi: 10.1016/0360-5442(96)00027-8
![]() |
[84] |
Rahimi A, Niksiar A (2013) A general model for moving-bed reactors with multiple chemical reactions part Ⅰ: Model formulation. Int J Miner Process 124: 58–66. https://doi.org/10.1016/j.minpro.2013.02.015 doi: 10.1016/j.minpro.2013.02.015
![]() |
[85] |
García-Triñanes P, Seville JPK, Ansart R, et al. (2018) Particle motion and heat transfer in an upward-flowing dense particle suspension: Application in solar receivers. Chem Eng Sci 177: 313–322. https://doi.org/10.1016/j.ces.2017.11.041 doi: 10.1016/j.ces.2017.11.041
![]() |
[86] |
Yusuf R, Melaaen MC, Mathiesen V (2005) Convective heat and mass transfer modeling in gas-fluidized beds. Chem Eng Technol 28: 13–24. https://doi.org/10.1002/ceat.200407014 doi: 10.1002/ceat.200407014
![]() |
[87] |
Zhang Y, Jiang C, Yang Z, et al. (2016) Numerical study on heat transfer enhancement in capsule-type plate heat exchangers. Appl Therm Eng 108: 1237–1242. https://doi.org/10.1016/j.applthermaleng.2016.08.033 doi: 10.1016/j.applthermaleng.2016.08.033
![]() |
[88] |
Song JW, Wang F, Cheng L (2012) Experimental study and analysis of a novel multi-media plate heat exchanger. Sci China Technol Sci 55: 2157–2162. https://doi.org/10.1007/s11431-012-4931-5 doi: 10.1007/s11431-012-4931-5
![]() |
[89] |
Villanueva HHS, de Mello PEB (2015) Heat transfer and pressure drop correlations for finned plate ceramic heat exchangers. Energy 88: 118–125. https://doi.org/10.1016/j.energy.2015.04.017 doi: 10.1016/j.energy.2015.04.017
![]() |
[90] |
Kim M, Baik YJ, Park SR, et al. (2010) Experimental study on corrugated crossflow air-cooled plate heat exchangers. Exp Therm Fluid Sci 34: 1265–1272. https://doi.org/10.1016/j.expthermflusci.2010.05.007 doi: 10.1016/j.expthermflusci.2010.05.007
![]() |
[91] |
Zhang L, Che D (2011) Influence of corrugation profile on the thermalhydraulic performance of cross-corrugated plates. Numer Heat Transf Part A Appl 59: 267–296. https://doi.org/10.1080/10407782.2011.540963 doi: 10.1080/10407782.2011.540963
![]() |
[92] |
Gulenoglu C, Akturk F, Aradag S, et al. (2014) Experimental comparison of performances of three different plates for gasketed plate heat exchangers. Int J Therm Sci 75: 249–256. https://doi.org/10.1016/j.ijthermalsci.2013.06.012 doi: 10.1016/j.ijthermalsci.2013.06.012
![]() |
[93] |
Yang J, Jacobi A, Liu W (2017) Heat transfer correlations for single-phase flow in plate heat exchangers based on experimental data. Appl Therm Eng 113: 1547–1557. https://doi.org/10.1016/j.applthermaleng.2016.10.147 doi: 10.1016/j.applthermaleng.2016.10.147
![]() |
[94] |
Durmuş A, Benli H, Kurtbaş I, et al. (2009) Investigation of heat transfer and pressure drop in plate heat exchangers having different surface profiles. Int J Heat Mass Transf 52: 1451–1457. https://doi.org/10.1016/j.ijheatmasstransfer.2008.07.052 doi: 10.1016/j.ijheatmasstransfer.2008.07.052
![]() |
[95] |
Seo JW, Kim YH, Kim D, et al. (2015) Heat transfer and pressure drop characteristics in straight microchannel of printed circuit heat exchangers. Entropy 17: 3438–3457. https://doi.org/10.3390/e17053438 doi: 10.3390/e17053438
![]() |
[96] | Gao T, Sammakia BG, Geer J, et al. (2014) Dynamic analysis of cross flow heat exchangers in data centers using transient effectiveness Method. IEEE Trans Components, Packag Manuf Technol 4: 1925–1935. Avaiable from: https://ieeexplore.ieee.org/document/6963402. |
[97] |
Vintrou S, Bougeard D, Russeil S, et al. (2013) Quantitative infrared investigation of local heat transfer in a circular finned tube heat exchanger assembly. Int J Heat Fluid Flow 44: 197–207. https://doi.org/10.1016/j.ijheatfluidflow.2013.05.019 doi: 10.1016/j.ijheatfluidflow.2013.05.019
![]() |
[98] |
Monteiro DB, de Mello PEB (2012) Thermal performance and pressure drop in a ceramic heat exchanger evaluated using CFD simulations. Energy 45: 489–496. https://doi.org/10.1016/j.energy.2012.02.012 doi: 10.1016/j.energy.2012.02.012
![]() |
[99] |
Zhang C, Wang D, Han Y, et al. (2017) Experimental and numerical investigation on the exergy and entransy performance of a novel plate heat exchanger. Exp Heat Transf 30: 162–177. https://doi.org/10.1080/08916152.2016.1179358 doi: 10.1080/08916152.2016.1179358
![]() |
[100] |
Doo JH, Ha MY, Min JK, et al. (2012) An investigation of cross-corrugated heat exchanger primary surfaces for advanced intercooled-cycle aero engines (Part-Ⅰ: Novel geometry of primary surface). Int J Heat Mass Transf 55: 5256–5267. https://doi.org/10.1016/j.ijheatmasstransfer.2013.01.084 doi: 10.1016/j.ijheatmasstransfer.2013.01.084
![]() |
[101] |
Lee JM, Kwan PW, Son CM, et al. (2015) Characterizations of aerothermal performance of novel cross-corrugated plate heat exchangers for advanced cycle aero-engines. Int J Heat Mass Transf 85: 166–180. https://doi.org/10.1016/j.ijheatmasstransfer.2013.01.084 doi: 10.1016/j.ijheatmasstransfer.2013.01.084
![]() |
[102] |
Nilpueng K, Wongwises S (2015) Experimental study of single-phase heat transfer and pressure drop inside a plate heat exchanger with a rough surface. Exp Therm Fluid Sci 68: 268–275. https://doi.org/10.1016/j.expthermflusci.2015.04.009 doi: 10.1016/j.expthermflusci.2015.04.009
![]() |
[103] |
Kundu B (2015) Beneficial design of unbaffled shell-and-tube heat exchangers for attachment of longitudinal fins with trapezoidal profile. Case Stud Therm Eng 5: 104–112. https://doi.org/10.1016/j.csite.2015.03.001 doi: 10.1016/j.csite.2015.03.001
![]() |
[104] |
Parikshit B, Spandana KR, Krishna V, et al. (2015) A simple method to calculate shell side fluid pressure drop in a shell and tube heat exchanger. Int J Heat Mass Transf 84: 700–712. https://doi.org/10.1016/j.ijheatmasstransfer.2015.01.068 doi: 10.1016/j.ijheatmasstransfer.2015.01.068
![]() |
[105] |
Chang TB, Yu LY (2015) Optimal nozzle spray cone angle for triangular-pitch shell-and-tube interior spray evaporator. Int J Heat Mass Transf 85: 463–472. https://doi.org/10.1016/j.ijheatmasstransfer.2015.01.123 doi: 10.1016/j.ijheatmasstransfer.2015.01.123
![]() |
[106] |
Alimoradi A, Veysi F (2017) Optimal and critical values of geometrical parameters of shell and helically coiled tube heat exchangers. Case Stud Therm Eng 10: 73–78. https://doi.org/10.1016/j.csite.2017.03.003 doi: 10.1016/j.csite.2017.03.003
![]() |
[107] |
Raja BD, Jhala RL, Patel V (2017) Many-objective optimization of shell and tube heat exchanger. Therm Sci Eng Prog 2: 87–101. https://doi.org/10.1016/j.tsep.2017.05.003 doi: 10.1016/j.tsep.2017.05.003
![]() |
[108] |
Gao B, Bi Q, Nie Z, et al. (2015) Experimental study of effects of baffle helix angle on shell-side performance of shell-and-tube heat exchangers with discontinuous helical baffles. Exp Therm Fluid Sci 68: 48–57. https://doi.org/10.1016/j.expthermflusci.2015.04.011 doi: 10.1016/j.expthermflusci.2015.04.011
![]() |
[109] |
Salahuddin U, Bilal M, Ejaz H (2015) A review of the advancements made in helical baffles used in shell and tube heat exchangers. Int Commun Heat Mass Transf 67: 104–108. https://doi.org/10.1016/j.icheatmasstransfer.2015.07.005 doi: 10.1016/j.icheatmasstransfer.2015.07.005
![]() |
[110] |
Yang JF, Zeng M, Wang QW (2015) Numerical investigation on combined single shell-pass shell-and-tube heat exchanger with two-layer continuous helical baffles. Int J Heat Mass Transf 84: 103–113. https://doi.org/10.1016/j.ijheatmasstransfer.2014.12.042 doi: 10.1016/j.ijheatmasstransfer.2014.12.042
![]() |
[111] |
Zhou GY, Xiao J, Zhu L, et al. (2015) A Numerical Study on the Shell-Side Turbulent Heat Transfer Enhancement of Shell-and-Tube Heat Exchanger with Trefoil-Hole Baffles. Energy Procedia 75: 3174–3179. https://doi.org/10.1016/j.egypro.2015.07.656 doi: 10.1016/j.egypro.2015.07.656
![]() |
[112] |
Wen J, Yang H, Jian G, et al. (2016) Energy and cost optimization of shell and tube heat exchanger with helical baffles using Kriging metamodel based on MOGA. Int J Heat Mass Transf 98: 29–39. https://doi.org/10.1016/j.ijheatmasstransfer.2016.02.084 doi: 10.1016/j.ijheatmasstransfer.2016.02.084
![]() |
[113] |
Du BC, He YL, Wang K, et al. (2017) Convective heat transfer of molten salt in the shell-and-tube heat exchanger with segmental baffles. Int J Heat Mass Transf 113: 456–465. https://doi.org/10.1016/j.ijheatmasstransfer.2017.05.075 doi: 10.1016/j.ijheatmasstransfer.2017.05.075
![]() |
[114] |
Wen J, Yang H, Wang S, et al. (2017) PIV experimental investigation on shell-side flow patterns of shell and tube heat exchanger with different helical baffles. Int J Heat Mass Transf 104: 247–259. https://doi.org/10.1016/j.ijheatmasstransfer.2016.08.048 doi: 10.1016/j.ijheatmasstransfer.2016.08.048
![]() |
[115] |
Crane D, Lagrandeur J, Jovovic V, et al. (2013) TEG on-vehicle performance and model validation and what it means for further teg development. J Electron Mater 42: 1582–1591. https://doi.org/10.1007/s11664-012-2327-8 doi: 10.1007/s11664-012-2327-8
![]() |
[116] |
Mori M, Yamagami T, Sorazawa M, et al. (2011) Simulation of fuel economy effectiveness of exhaust heat recovery system using thermoelectric generator in a series hybrid. SAE Int J Mater Manuf 4: 1268–1276. https://doi.org/10.4271/2011-01-1335 doi: 10.4271/2011-01-1335
![]() |
[117] |
Hussain QE, Brigham DR, Maranville CW (2009) Thermoelectric exhaust heat recovery for hybrid vehicles. SAE Tech Pap 2: 1132–1142. https://doi.org/10.4271/2009-01-1327 doi: 10.4271/2009-01-1327
![]() |
[118] |
Espinosa N, Lazard M, Aixala L, et al. (2010) Modeling a thermoelectric generator applied to diesel automotive heat recovery. J Electron Mater 39: 1446–1455. https://doi.org/10.1007/s11664-010-1305-2 doi: 10.1007/s11664-010-1305-2
![]() |
[119] |
Gonçalves VM, Maia CA, Hardouin L (2012) On the solution of Max-plus linear equations with application on the control of TEGs. IFAC Proc 45: 91–97. https://doi.org/10.3182/20121003-3-MX-4033.00018 doi: 10.3182/20121003-3-MX-4033.00018
![]() |
[120] |
Brito FP, Goncalves LM, Martins J, et al. (2013) Influence of heat pipe operating temperature on exhaust heat thermoelectric generation. SAE Int J Passeng Cars-Mech Syst 6: 652–664. https://doi.org/10.4271/2013-01-0559 doi: 10.4271/2013-01-0559
![]() |
[121] | Martins J, Goncalves LM, Antunes J, et al. (2011) Thermoelectric exhaust energy recovery with temperature control through heat pipes. SAE 2011 World Congr Exhib 1: 1–16. Avaiable from: https://core.ac.uk/download/pdf/55616825.pdf. |
[122] |
Jang JC, Chi RG, Rhi SH, et al. (2015) Heat pipe-assisted thermoelectric power generation technology for waste heat recovery. J Electron Mater 44: 2039–2047. https://doi.org/10.1007/s11664-015-3653-4 doi: 10.1007/s11664-015-3653-4
![]() |
[123] |
Lan S, Yang Z, Chen R, et al. (2018) A dynamic model for thermoelectric generator applied to vehicle waste heat recovery. Appl Energy 210: 327–338. https://doi.org/10.1016/j.apenergy.2017.11.004 doi: 10.1016/j.apenergy.2017.11.004
![]() |
[124] |
Tang ZB, Deng YD, Su CQ, et al. (2015) A research on thermoelectric generator's electrical performance under temperature mismatch conditions for automotive waste heat recovery system. Case Stud Therm Eng 5: 143–150. https://doi.org/10.1016/j.csite.2015.03.006 doi: 10.1016/j.csite.2015.03.006
![]() |
[125] |
Orr B, Akbarzadeh A (2017) Prospects of waste heat recovery and power generation using thermoelectric generators. Energy Procedia 110: 250–255. https://doi.org/10.1016/j.egypro.2017.03.135 doi: 10.1016/j.egypro.2017.03.135
![]() |
[126] |
He W, Wang S, Yue L (2017) High net power output analysis with changes in exhaust temperature in a thermoelectric generator system. Appl Energy 196: 259–267. https://doi.org/10.1016/j.apenergy.2016.12.078 doi: 10.1016/j.apenergy.2016.12.078
![]() |
[127] | Fankai M, Lingen Ch, Sun FR (2012) Effects of temperature dependence of thermoelectric properties on the power and efficiency of a multielement thermoelectric generator. Int J Energy Environ Eng 3: 137–150. Available from: https://www.ijee.ieefoundation.org/vol3/issue1/IJEE_14_v3n1.pdf. |
[128] |
Gou X, Yang S, Xiao H, et al. (2013) A dynamic model for thermoelectric generator applied in waste heat recovery. Energy 52: 201–209. https://doi.org/10.1016/j.apenergy.2017.11.004 doi: 10.1016/j.apenergy.2017.11.004
![]() |
[129] |
Meng JH, Zhang XX, Wang XD (2014) Dynamic response characteristics of thermoelectric generator predicted by a three-dimensional heat-electricity coupled model. J Power Sources 245: 262–269. https://doi.org/10.1016/j.jpowsour.2013.06.127 doi: 10.1016/j.jpowsour.2013.06.127
![]() |
[130] |
Hsu CT, Huang GY, Chu HS, et al. (2011) An effective Seebeck coefficient obtained by experimental results of a thermoelectric generator module. Appl Energy 88: 5173–5179. https://doi.org/10.1016/j.apenergy.2011.07.033 doi: 10.1016/j.apenergy.2011.07.033
![]() |
1. | Juexin Wang, Yan Wang, Towards Machine Learning in Molecular Biology, 2020, 17, 1551-0018, 2822, 10.3934/mbe.2020156 | |
2. | Hang Zhao, Lin Wang, Lijuan Zhang, Hongyu Zhao, Phytochemicals targeting lncRNAs: A novel direction for neuroprotection in neurological disorders, 2023, 162, 07533322, 114692, 10.1016/j.biopha.2023.114692 | |
3. | Hao Zhu, Tong Liu, Zheng Wang, scHiMe: predicting single-cell DNA methylation levels based on single-cell Hi-C data, 2023, 24, 1467-5463, 10.1093/bib/bbad223 |