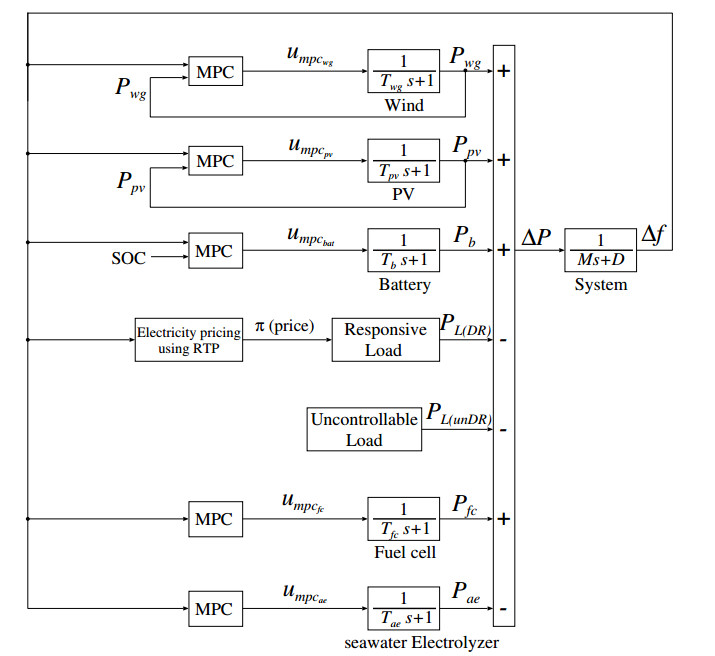
This work presents a load frequency control scheme in Renewable Energy Sources(RESs) power system by applying Model Predictive Control(MPC). The MPC is designed depending on the first model parameter and then investigate its performance on the second model to confirm its robustness and effectiveness over a wide range of operating conditions. The first model is 100% RESs system with Photovoltaic generation(PV), wind generation(WG), fuel cell, seawater electrolyzer, and storage battery. From the simulation results of the first case, it shows the control scheme is efficiency. And base on the good results of the first case study, to propose a second case using a 10-bus power system of Okinawa island, Japan, to verify the efficiency of proposed MPC control scheme again. In addition, in the second case, there also applied storage devices, demand-response technique and RESs output control to compensate the system frequency balance. Last, there have a detailed results analysis to compare the two cases simulation results, and then to Prospects for future research. All the simulations of this work are performed in Matlab®/Simulink®.
Citation: Lei Liu, Takeyoshi Kato, Paras Mandal, Alexey Mikhaylov, Ashraf M. Hemeida, Hiroshi Takahashi, Tomonobu Senjyu. Two cases studies of Model Predictive Control approach for hybrid Renewable Energy Systems[J]. AIMS Energy, 2021, 9(6): 1241-1259. doi: 10.3934/energy.2021057
[1] | Hassan Shirzeh, Fazel Naghdy, Philip Ciufo, Montserrat Ros . Stochastic energy balancing in substation energy management. AIMS Energy, 2015, 3(4): 810-837. doi: 10.3934/energy.2015.4.810 |
[2] | K. M. S. Y. Konara, M. L. Kolhe, Arvind Sharma . Power dispatching techniques as a finite state machine for a standalone photovoltaic system with a hybrid energy storage. AIMS Energy, 2020, 8(2): 214-230. doi: 10.3934/energy.2020.2.214 |
[3] | Khuthadzo Kgopana, Olawale Popoola . Improved utilization of hybrid energy for low-income houses based on energy consumption pattern. AIMS Energy, 2023, 11(1): 79-109. doi: 10.3934/energy.2023005 |
[4] | Mohamed Hamdi, Hafez A. El Salmawy, Reda Ragab . Optimum configuration of a dispatchable hybrid renewable energy plant using artificial neural networks: Case study of Ras Ghareb, Egypt. AIMS Energy, 2023, 11(1): 171-196. doi: 10.3934/energy.2023010 |
[5] | Ryuto Shigenobu, Oludamilare Bode Adewuyi, Atsushi Yona, Tomonobu Senjyu . Demand response strategy management with active and reactive power incentive in the smart grid: a two-level optimization approach. AIMS Energy, 2017, 5(3): 482-505. doi: 10.3934/energy.2017.3.482 |
[6] | Habibullah Fedayi, Mikaeel Ahmadi, Abdul Basir Faiq, Naomitsu Urasaki, Tomonobu Senjyu . BESS based voltage stability improvement enhancing the optimal control of real and reactive power compensation. AIMS Energy, 2022, 10(3): 535-552. doi: 10.3934/energy.2022027 |
[7] | Syed Sabir Hussain Rizvi, Krishna Teerth Chaturvedi, Mohan Lal Kolhe . A review on peak shaving techniques for smart grids. AIMS Energy, 2023, 11(4): 723-752. doi: 10.3934/energy.2023036 |
[8] | Mohamed G Moh Almihat, MTE Kahn . Centralized control system for islanded minigrid. AIMS Energy, 2023, 11(4): 663-682. doi: 10.3934/energy.2023033 |
[9] | Tilahun Nigussie, Wondwossen Bogale, Feyisa Bekele, Edessa Dribssa . Feasibility study for power generation using off- grid energy system from micro hydro-PV-diesel generator-battery for rural area of Ethiopia: The case of Melkey Hera village, Western Ethiopia. AIMS Energy, 2017, 5(4): 667-690. doi: 10.3934/energy.2017.4.667 |
[10] | Aaron St. Leger . Demand response impacts on off-grid hybrid photovoltaic-diesel generator microgrids. AIMS Energy, 2015, 3(3): 360-376. doi: 10.3934/energy.2015.3.360 |
This work presents a load frequency control scheme in Renewable Energy Sources(RESs) power system by applying Model Predictive Control(MPC). The MPC is designed depending on the first model parameter and then investigate its performance on the second model to confirm its robustness and effectiveness over a wide range of operating conditions. The first model is 100% RESs system with Photovoltaic generation(PV), wind generation(WG), fuel cell, seawater electrolyzer, and storage battery. From the simulation results of the first case, it shows the control scheme is efficiency. And base on the good results of the first case study, to propose a second case using a 10-bus power system of Okinawa island, Japan, to verify the efficiency of proposed MPC control scheme again. In addition, in the second case, there also applied storage devices, demand-response technique and RESs output control to compensate the system frequency balance. Last, there have a detailed results analysis to compare the two cases simulation results, and then to Prospects for future research. All the simulations of this work are performed in Matlab®/Simulink®.
Nowadays, diesel generators are still using in most areas of the world, especially at isolated islands area like Okinawa, Japan. The character of isolated island power system is it difficult to make a connection with the primary power grid, so thermal generation as one of the best way to provide power source in these areas. Many developed countries like Japan attach great importance to the development of thermal power generation technology. It makes the technology of thermal generation already very mature. Even though thermal power generation plays a critical role in major energy supply systems, but scholars also notice as its a bulky, lengthy installation process, and another most serious thing is the CO2 and other harmful gases emission. These shortcomings raised more and more attention along with the energy shortage in recent years.
The world's primary energy supply has increased 58% in the past 25 years, which means as current rates of production, the estimated proven coal reserves for 150 years, proven oil and gas reserves are equivalent to around 50 and 52 years. Thence, RESs has many advantages that make it a good substitute for fossil power generation in future decades [1]. And on the other hand, RESs have multiple forms and are virtually limitless. It has a low impact on the environment, and most RESs are positive environmental friendly. Another significant point is independence, it allows lots of nations could control their energy sources market pricing and availability, and it can help bring more revenue to the national finances to boosting the economy. At last, another advantage is that it could stabilize the global energy prices. The primary RES form includes PV, WG, biomass, fuel cell, geothermal generation, and hydroelectric energy generation [2].
With the rapid development of RESs, and the import capacity of RESs increasing, engineers and scholars realize that RESs are easily affected by weather factors that lead to the supply-load mismatch resulting in sharp fluctuation of system frequency [1]. Many researchers had worked on this point attempt to solve the problem. For Example, Vazquez et al. [3] review the principle and developing history of MPC, and point out the three elements of MPC implementation, forecast prediction model, cost function, and optimization algorithm. Furthermore, the author discussed the advanced technology and future trends of MPC in the nest few years. Serale et al. [4] show a study that compared the different MPC approaches for heating ventilation and air conditioning system management and building. The research method and article structure have a great enlightening effect on this work. Lu et al. [5] present a work about multi-area interconnected power system load frequency control problem by apply robust PI controller and constrained population extremal optimization. The model construction and research idea are inspired by our work to some extent. Al-Ghussain et al. [6] provide a hybrid PV/Wind system case study which for Lafarge cement factory in Al-Tafilah, Jordan. Moreover, by apply the Lithium-Ion bank batteries to examine the effect of technical and economic feasibility. And the simulation results indicate the Lithium-Ion batteries system is more feasible on technical and economic and has higher fraction of demand met the hybrid system compare with no storage bank case. Mazzeo et al. [7] show a literature review of 550 articles most relevant hybrid system in last twenty-five years during 1995 to 2020. Which give a qualitative-quantitative prospect and statistical analysis view, including but not limited to component configurations, auxiliary components used to support, operating mode and geographical. Anoune et al. [8] show a work of PV-Wind hybrid system provide electrical load demand for a immersed electrical heater, by applied the optimum configuration to ensure supplying energy within lowest cost. As last by compare two case of yearly average month and the worse month methods, to size a hybrid system with lower integration cost than the worst month method. Mazzeo et al. [9] propose a renewable hybrid trigeneration system (RHTS) within photovoltaic generator, wind micro-generator and an electric storage battery to supply power energy to a office system includes heat pump, electric office devices and an electric vehicle charging station, to analysis the dynamic and energy reliability. In different characteristic condition to study the simulation results with three electric loads, to identify the load and determine the system energy reliability. Mazzeo et al. [10] propose two Artificial Neural Networks to sizing and simulate a PV-wind hybrid system within energy storage devices and electric vehicle charging stations. One is to estimate the energy performance indicators, and the second on is to forecast the grid energy indication factor. By training the algorithm Within a large number of data of different climatic conditions, flexible power system configuration and varying electrical loads. Acuna et al. [11] base on the minimum hourly energy generate ability of solar radiation and wind, using a probabilistic scheme to proposed a new reliability indicator. By compared the new indicator with others, the results shows this indicator could increase the system reliability obviously.
In addition to these papers, this article has also gained inspiration and inspiration from many other studies. Zhao et al. [12] show the work of a management and control system for microgrid power system by inducing RESs, which includes PV, WG, and storage battery. The power converters and control algorithms had been employed in this work. Moreover, this paper examined the stability of the designed method, and then the control approach had been applied in a three-area power system. Babahajiani et al. [13] display a research adopted fuzzy-logic frequency control for wind farms augmented with a wind-storage power system, and it shows the proposed control method how to compensated low-inertia hybrid power system to improves the primary frequency response. By considering load frequency fluctuation and frequency change rate, the controller proposed a bidirectional real power injection. By removing the inflexible deloading of the wind turbine and minimizing the storage capacity, to realize the optimization of the proposed fuzzy-logic frequency controller. Bahrami et al. [14] propose a controller connected two-districts thermal deregulated electricity power model, with reheat and a non-reheat unit, and gives a novel intelligent controller design using storage battery for load frequency control two-districts interconnected deregulated power systems. Vardakas et al. [15] show a plug-and-play style Load Frequency Control approach which could be use for generators and controllable loads.
Depending on all the previous reference works using PI and PID controller, this paper presented a load frequency control scheme by using MPC controller in two cases models, the first one is 100% hybrid RESs system with PV, WG, fuel cell, seawater electrolyzer, case two is a 10-bus Okinawa isolated island hybrid power system model. And by apply Demand-Response, storage battery and Real-time pricing. The goal of this work is to examine the robustness and effectiveness of MPC control sche me, and to discuss about the power system stability. Compare with other works, this paper presents a MPC control scheme in a 10-bus isolated island hybrid power system. Furthermore, based on the results of this model, a multi-area system two-level(Centralized and Distributed) MPC control scheme is looking forward to formulate in the future.
This article is arranged as: Section 2 introduce a 100% Renewable Energy power system configuration as case 1. Section 3 discusses the proposed control approaches. Section 4 presents the case 2 of Okinawa 10-bus Power system model. Section 5 the simulation results and analysis. Specific summaries and future work are written in Section 6.
Figure 1 shows the remote island power system model. The power system consists of a 20 MW Photovoltaic Generator (PV), 15 MW Wind power Generator (WG), storage battery (10 MW/40 MWh), fuel cell (5 MW), seawater electrolyzer (5 MW), and load. The parameters of the power system are shown in Table 1. This system is connected to the primary grid with an Energy Management System(EMS), which changes the electricity consumption by price. In Figure 1, decentralized MPCs designed in each part, PV, WG, storage battery, fuel cell, and seawater electrolyzer.
Parameters | Constants |
D | 0.015 pu/Hz |
Ms | 0.1667 pu/s |
Twg | 1.5 s |
Tpv | 1.8 s |
Tb | 0.1 s |
Tfc | 4.0 s |
Tae | 0.5 s |
MPC is an advanced control method to decide the operation parameters by using the plant controlled amount and manipulated variable to forecast the following step output anastomosis with the set value.
The MPC design process is shown below. The PV and seawater electrolyzer MPCs are neglected because the design process is identical WG and fuel cell controllers model, respectively. The following state-space function define the prediction model in each subsection. For all MPCs, the sample horizon time is set as 0.1 s, the prediction horizon set as 10 s, and the control horizon set as 2 s.
˙x=Ax(t)+Bu(t) | (2.1) |
y=Cx(t)+Du(t) | (2.2) |
Here, x is the state vector, u is the input vector, and y is the output vector. The real constant matrices A, B, C, and D associated with the above vectors are shown below.
The MPC design of storage battery is shown in Figure 2, frequency fluctuation and SOC as inputs of the MPC. In storage battery controller design, the frequency fluctuation compensation and storage battery SOC preserve are considered simultaneously. The frequency fluctuation allowable value is set at Δf=0 Hz, and the rate of SOC is set at 50% as the target values. Furthermore, the measured disturbance is considered in this model simulation as described below:
A=[−1Tb001M−DM0100]B=[1Tb001M00]C=[010001]D=[0000]x(t)=[ΔPbΔfSOC]Tu(t)=[umpcbatPdis]Ty(t)=[ΔfSOC]T | (2.3) |
In this part, the MPC constraint conditions are discussed. The output frequency deviation allowance value were set as ±0.05 Hz and the input values allowance range set as −0.15∼0.15 pu. The battery inverter assumed rating constraint is −0.15∼0.15 pu. The MPC weight of frequency deviation is 10, and the weight of SOC is 3. The weight of the frequency deviation control input is 0.1. The frequency fluctuation weight was more significant than the SOC. It is in order to take precedence of frequency fluctuation than the SOC.
From Figure 1, we can found MPC was used for the WG facility. The frequency deviation compensation and output power maximization are considered simultaneously. The load frequency deviation and WG output were utilized as inputs signals of the MPC. The reference values of frequency is Δf=0 Hz, and the MPPT (Maximum Power Point Tracking) controller real-time output of the generated power ΔPwg. Moreover, the measured disturbance is considered in the design of the proposed MPC control scheme as follows:
A=[−1Twg01M−DM]B=[1Twg0]TC=[0110]D=[0]x(t)=[ΔPwgΔf]Tu(t)=umpcbaty(t)=[ΔfΔPwg]T | (2.4) |
Here, the allowance value of frequency fluctuation is ±0.05 Hz and the input values allowance range is set as 0 pu ∼ the maximum power output by following the wind speed. The weight of load frequency fluctuation is set as 10, the weight of WG output power is set as 5. The weight value of frequency deviation is set more significant than the output power. It is to take precedence of the frequency fluctuation over the generated power.
In the fuel cell model, MPC is shown in Figure 1. By considering load frequency deviation and the solar generation output as MPC inputs, frequency fluctuation compensation and PV output maximization were considered simultaneously. The frequency deviation reference value is Δf=0 Hz. The prediction model of this MPC takes the measured disturbance into consideration as follows:
A=[−1Tfc01M−DM]B=[1Tfc001M]C=[01]D=[00]x(t)=[ΔPfcΔf]Tu(t)=[umpcbatPdis]Ty(t)=[Δf] | (2.5) |
The allowance value of frequency fluctuation is set as ±0.05 Hz, and the input values allowance range is −0.25∼0.25 pu. The weight value of the load frequency fluctuation is set at 1, as a constraint of the assumed fuel cell power output.
All the design of MPC controller for generation is shown in this part. Furthermore, real-time pricing and demand-response technique will explain in the following detail. To suppress the load frequency fluctuation by adjusting the power consumption from electricity price.
There are many pricing methods like Real-Time Pricing (RTP) and Time-Of-Use (TOU) pricing. Different methods can be chosen depending on different purposes of implement the situation. TOU is a method that prompts a peak shift by changing the electricity price during a given time range. Other hand, RTP could improve the supply-demand balance by changing the electricity price at any times. It differs from TOU, which could change by a static forecast from the previous usage. In this paper, RTP method is chosen as the pricing method.
The price calculate model shows in Figure 3, the price is determined by frequency fluctuation Δf.
π∗=−0.31+exp(−40⋅Δf)+0.35[Yen] | (3.1) |
Here, f is frequency, fref is reference frequency, π is power price.
Figure 4 shows how the frequency fluctuation affects the electricity price π. RTP is working by considering the relationship between the supply-demand balance and load frequency fluctuation. If a large load consumer occurs or the load frequency decrease, the price of the electricity will rise to drop the consumption. Conversely, the electricity price reduce when the light loads occur so that it can increase the load. As foreseeable, sharp frequency fluctuation would cause intense price fluctuations, shown in Eq (3.1). To effectively compensate the rapid fluctuation of electricity prices, a first-order lag filter time constant Tπ was applied to retard the change of price was shown in Eq (3.1).
In Figure 5, each load power consumption is presented with follow the price. The consumed power predetermined follow the electricity price. As the proposed method, the consumption will match the demand automatically according to the electricity price and energy management system, and it would realize the load automatical demand-response. For this paper, eight types of consumption loads were assumed. Eight types loads could divide into three categories roughly. As below, three types of loads are cited, and the characters had been described.
● Loads 1–4: Constantly fluctuating according to price fluctuations.
● Loads 5–6: For the purpose of managing the steep fluctuation of price, these loads are hysteresis characteristics for rises and drops output power cost. For instance, by obvious Load 5, if price increase to 30 yen, the consume power being 0 pu. Otherwise, if the price decreases to 25 yen, the load rises to 0.05 pu.
● Load 7–8: These two loads are hysteresis characteristic for rises and drops consumption.
As shown in Figure 6, the output power suppression control scheme of the PV due to the frequency fluctuation is presented by adding the reference value of frequency fluctuation control, the terminal voltage command value is decided PI controller. The output power of PV is determined by the PV curves and voltage value.
The effectiveness of assumed approach is inspected in the first model with various types of RESs and energy storage devices in Figure 1. Figure 7 shows solar radiation [Wh/m2]. Wind speed [m/s] is presented in Figure 8. The obtained results are held for 800 s as shown in Figures 9 and 10. The associated detailed analysis and comparison with second case results will be discussed in section 5.
In Figure 11, to inspect the proper performance of the assumed control approach under different operating conditions, Okinawa island 10-bus power system model was applied in this work. Where Gx, WGx, PVx refer to thermal power generation, PV, and WG, respectively. Table 2 shows the parameters of thermal generators, and the line admittance show in Table 3. Moreover, parameters of Gx, WGx, PVx are discussed in Table 4. Furthermore, the maximum consumption power of each load is discuss in Table 5.
Gas-turbine generators | G1 | G2 | G3 | G4 | |
rated power | PG [MW] | 324 | 482 | 292 | 417 |
Inertia constant | H [s] | 6.3 | 7.2 | 5.8 | 6.8 |
d-axis synchronous reactance | xd [pu] | 1.57 | 1.63 | 1.53 | 1.68 |
d-axis transient reactance | x′d [pu] | 0.261 | 0.268 | 0.298 | 0.312 |
q-axis synchronous reactance | xq [pu] | 1.06 | 0.98 | 1.12 | 0.98 |
q-axis transient reactance | x′q [pu] | 0.177 | 0.189 | 0.173 | 0.192 |
d-axis open-circuit transient time constant | T′d0 [s] | 6.1 | 7.5 | 5.7 | 7.6 |
q-axis open-circuit transient time constant | T′q0 [s] | 0.64 | 0.41 | 0.72 | 0.47 |
Amplifier gain | KA | 50 | 50 | 50 | 50 |
Amplifier time constant | TA [s] | 0.06 | 0.06 | 0.06 | 0.06 |
Governor time constant | TG [s] | 4.0 | 4.0 | 4.0 | 4.0 |
line resistance | r [pu] | 1.1048 |
line reactance | x [pu] | 4.6954 |
Wind-turbine generator | WG1 | WG2 | WG3 | ||
Rated power | Pwg [MW] | 10 | 7 | 8 | |
Photovoltaic generator | PV1 | PV2 | PV3 | ||
Rated power | Ppv [MW] | 140 | 140 | 140 | |
Battery | 1 | 2 | 3 | 4 | |
Inverter capacity | [MVA] | 25 | 25 | 25 | 25 |
Storage capacity | [MWh] | 100 | 100 | 100 | 100 |
Load 1, 2, 3, 5 | Uncontrollable load | 100 MW |
Controllable load | 75 MW | |
Load 4, 6 | Uncontrollable load | 200 MW |
Controllable load | 150 MW | |
Total | Uncontrollable load | 800 MW |
Controllable load | 600 MW |
The simulations are held for 1 hour and the obtained results are shown in Figure 14–Figure 27. Detailed analysis for the simulation outcomes will be held in the next section.
A) Case 1: Figure 7 shows solar radiation [Wh/m2]. Wind speed [m/s] is presented in Figure 8, 600 [Wh/m2] average solar radiation is considered with rapid decrease to 200 [Wh/m2] within the interval from 600 to 700 second and rapid increase from 700 to 800 second. To investigate the performance of the proposed control scheme for wind speed, a range of (8.5–10) m/s is considered in this case. Figure 9 shows the capability of the proposed control approach to damp frequency fluctuation and keep it in the permissible range. Moreover, the novel utility demand response scheme succeeds in controlling the various categories of load demand as shown in Figure 10 according to the mismatch between generation and load to dampen the associated frequency fluctuation.
B) Case 2: Okinawa island 10-bus power system model is applied as a second case study to investigate the performance of the proposed control methodology to confirm its ability to work properly under wide range of operating conditions. Simulations are held for 1 hour. The associated solar radiation and wind speed parameters adopted this time are shown in Figures 12 and 13, respectively.
From Figure 14, the proposed scheme succeeded in damping the frequency fluctuations and keeping them in the permissible range. Most of the tome, the system frequency deviation was controlled between 59.9–60.1 Hz. Considering that this model introduced variety and large-scale RESs, the controllers had a pretty good simulation performance. Moreover, depending on the electricity price variation shown in Figure 15, the electricity price increase twice during 50–450 s and 1300–2300 s, Demand-Response affects the balance of supply and demand at both ends of the system by adjusting electricity prices. Furthermore, the proposed approach managed the various load demand of each categories as shown in Figure 16 to ensure the stability of the power system even in emergency cases. Furthermore, the consumption load power of each bus is shown in Figure 17. On the other hand, as shown in Figure 18, the proposed control method succeeded to get the optimal matching between the generation and load side by introducing RESs in the generation side and applying novel a Demand-Response approach on the load side, it clearly shows the effect of the control scheme on the system supply and demand balance. Active power of each battery was presented in Figure 19 while the associated SOC is shown in Figure 20 which confirms the ability of the control methodology to keep SOC within the allowed range (20%–80%). The storage battery in the system can reduce both high and low operation ratio frequency fluctuations. Before the storage battery price and endurance are the main factors that restrict the application of battery equipment in the power system. However, with the development of storage battery technology in recent years, the battery's endurance has been dramatically improved, and the price has been decreasing year by year. In the near future, storage batteries will be more and more apply in power systems. The active power of each thermal generator is shown in Figure 21 and the associated operation rate is presented in Figure 22. From Figure 22, the proposed control scheme succeed to keep thermal generators an optimal operation rate (70%–80%) to decrease the losses for low operation ratio and at the same time to avoid high operation ratio and its lifetime effect problems. The output power of wind turbines are shown in Figure 23 with average power 0.01–0.02 pu. Moreover, Figure 24 presents the output power of PV power plants implemented in this system. In this part it can find that the output of PV and WG both followed the solar radiation and wind speed curve within applied MPPT. Also by update case two model as more RES generation even 100% RES sources, it will be a new research subject in next step. The reactive power of each battery is shown in Figure 25. Here can see before 2400s, there is a fluctuation between -0.05–0.05, and after 2400s to 3600s, the reactive power stable at -0.05. The terminal voltage of each bus is presented in Figure 26 and Figure 27, respectively. These figures confirms the performance of the assumed control approach to keep bus voltage within the permissible range 0.95–1.05 irrespective of the RESs output variation or load demand change.
In summary, the previous results clarity the ability of the proposed control approach to adjust the power system stable through maintaining frequency deviation between 59.9–60.1 Hz, control the generation-load balance via novel both generation and load control scheme to force each side to follow the other side so as to mitigate the frequency deviations. In addition, the proposed approach also keep battery SOC at 50% to extend its lifetime.
In this paper, a novel load frequency control scheme is proposed to investigate the system frequency deviation suppression. Two case studies are considered to confirm the effectiveness of the proposed control approach for a wide range of operating conditions. First model is the 100% RESs power system while the second one is the real 10-bus Okinawa power system model. The proposed methodology utilizes MPC, RESs, demand-response and storage battery systems. The simulation results show the ability of the proposed control method to damp the frequency fluctuations and keep them in the permissible range. Moreover, it succeeded in controlling the various load demand types to mitigate the generation-load mismatch and keep the power system stable. Furthermore, the proposed scheme could keep the battery SOC around 50% to extend its lifetime. Also, the proposed approach could keep the bus voltage in the allowed range (0.95–1.05) irrespective of the RESs variation or load-demand changes. The performance of the test models is significantly improved with the application of the proposed control scheme.
For future work, based on the current work, a two-step MPC load frequency control scheme of multi-area power system is considered. In addition to the existing controllers, a central MPC controller is designed and apply to the entire multi-regional power system. While considering the tie-power between each regional system, verify the effectiveness and robustness of the two-step MPC control scheme through simulation results.
This research received no external funding.
The authors declare no conflict of interest.
[1] | Stott PA, Mueller MA (2006) Modelling fully variable speed hybrid wind diesel systems. Proceedings of the 41st International Universities Power Engineering Conference, 9486735, Newcastle upon Tyne, UK. |
[2] | Alternative Energy Solutions for the 21st Century, Renewable Energy. [Online]. Available from: http://www.altenergy.org/renewables/renewables.htmll. |
[3] | Vazquez S, Rodriguez J, Rivera M, et al. (2016) Model eredictive control for power converters and drives: Advances and trends. IEEE Trans Ind Electron 64: 935-947. |
[4] | Serale G, Fiorentini M, Capozzoli A, et al. (2018) Model Predictive Control (MPC) for enhancing building and HVAC system energy efficiency: Problem formulation, applications and opportunities. Energies 11: 631. |
[5] | Lu K, Zhou W, Zeng G, et al. (2019) Constrained population extremal optimization-based robust load frequency control of multi-area interconnected power system. Int J Electr Power Energy Syst 105: 249-271. |
[6] | Al-Ghussain L, Ahmed H, Haneef F (2018) Optimization of hybrid PV-wind system: Case study Al-Tafilah cement factory, Jordan. Sustainable Energy Technol Assess 30: 24-36. |
[7] | Mazzeo D, Matera N, De Luca P, et al. (2021) A literature review and statistical analysis of photovoltaic-wind hybrid renewable system research by considering the most relevant 550 articles: An upgradable matrix literature database. J Cleaner Prod 295: 126070. |
[8] | Anoune K, Laknizia A, Bouyaa M, et al. (2018) Sizing a PV-Wind based hybrid system using deterministic approach. Energy Convers Manage 169: 137-148. |
[9] | Mazzeo D, Matera N, Oliveti G (2018) Interaction between a wind-PV-battery-heat pump trigeneration system and office building electric energy demand including vehicle charging. 2018 IEEE International Conference on Environment and Electrical Engineering and 2018 IEEE Industrial and Commercial Power Systems Europe (EEEIC/ICPS Europe) 18182476, Palermo, Italy. |
[10] | Mazzeo D, Herdem MS, Matera N, et al. (2021) Artificial intelligence application for the performance prediction of a clean energy community. Energy 232: 120999. |
[11] | Acuna LG, Padilla RV, Mercado AS (2016) Measuring reliability of hybrid photovoltaic-wind energy systems: A new indicator. Renewable Energy 8426: 152-178. |
[12] | Zhao C, Mallada E, Low SH, et al. (2018) Distributed plug-and-play optimal generator and load control for power system frequency regulation. Int J Electr Power Energy Syst 101: 1-12. |
[13] | Babahajiani P, Shafiee Q, Bevrani H (2016) Intelligent demand response contribution in frequency control of Multi-Area power systems. IEEE Trans Smart Grid 9: 1282-1291. |
[14] | Bahrami S, Sheikhi A (2015) From demand response in smart grid toward integrated demand response in smart energy hub. IEEE Trans Smart Grid 7: 650-658. |
[15] | Vardakas JS, Zorba N, Verikoukis CV (2014) A Survey on demand response programs in smart grids: Pricing methods and optimization algorithms. IEEE Commun Surv Tutorials 17: 152-178. |
1. | Mir Sayed Shah Danish, Gabor Pinter, 2022, Chapter 10, 978-3-031-12957-5, 115, 10.1007/978-3-031-12958-2_10 | |
2. | Miraj Ahmed Bhuiyan, Nora Hegedusne Baranyai, 2022, Chapter 10, 978-3-031-13145-5, 117, 10.1007/978-3-031-13146-2_10 | |
3. | Santi Agatino Rizzo, Editorial to the 'Special Issue—Distribution network reliability in Smart Grids and Microgrids' of AIMS Energy, 2022, 10, 2333-8334, 533, 10.3934/energy.2022026 | |
4. | Nikita Makarichev, Tsangyao Chang, 2022, Chapter 9, 978-3-031-12957-5, 101, 10.1007/978-3-031-12958-2_9 | |
5. | Georgy Shilov, András Vincze, 2022, Chapter 12, 978-3-031-12957-5, 139, 10.1007/978-3-031-12958-2_12 | |
6. | Ivan Udalov, Almakul Abdimomynova, Svetlana Moldagulova, 2022, Chapter 12, 978-3-031-13145-5, 147, 10.1007/978-3-031-13146-2_12 | |
7. | Andrey Kraykin, Artur Meynkhard, Tomonobu Senjyu, 2022, Chapter 11, 978-3-031-13145-5, 131, 10.1007/978-3-031-13146-2_11 | |
8. | Konstantin Panasenko, Fi-John Chang, 2022, Chapter 9, 978-3-031-13145-5, 105, 10.1007/978-3-031-13146-2_9 | |
9. | Miraj Ahmed Bhuiyan, Elizaveta Ibragimova, 2022, Chapter 11, 978-3-031-12957-5, 127, 10.1007/978-3-031-12958-2_11 | |
10. | Lyailya Maratovna Mutaliyeva, Ulf Henning Richter, 2023, 978-1-80382-884-8, 1, 10.1108/978-1-80382-883-120231001 | |
11. | Khayrilla Abdurasulovich Kurbonov, Gabor Pinter, 2023, 978-1-80382-884-8, 31, 10.1108/978-1-80382-883-120231003 | |
12. | David Philippov, Tomonobu Senjyu, 2023, 978-1-80382-884-8, 177, 10.1108/978-1-80382-883-120231014 | |
13. | Raya Hojabaevna Karlibaeva, Anthony Nyangarika, 2023, 978-1-80382-884-8, 153, 10.1108/978-1-80382-883-120231012 | |
14. | Mahmoud Zadehbagheri, Ashraf Hemeida, 2024, Chapter 14, 978-3-031-51531-6, 173, 10.1007/978-3-031-51532-3_14 | |
15. | Iman Mahmoud, Emerson Guzzi Zuan Esteves, 2024, Chapter 11, 978-3-031-51531-6, 135, 10.1007/978-3-031-51532-3_11 | |
16. | Alexey Mikhaylov, 2024, Chapter 4, 978-3-031-51531-6, 39, 10.1007/978-3-031-51532-3_4 | |
17. | Solomon Eghosa Uhunamure, Tsangyao Chang, 2024, Chapter 7, 978-3-031-51531-6, 85, 10.1007/978-3-031-51532-3_7 | |
18. | Laura M. Baitenova, Lyailya M. Mutaliyeva, Fi-John Chang, 2023, Chapter 2, 978-3-031-26595-2, 13, 10.1007/978-3-031-26596-9_2 | |
19. | Mikhail Dorofeev, Hooi Hooi Lean, 2024, Chapter 20, 978-3-031-51531-6, 245, 10.1007/978-3-031-51532-3_20 | |
20. | Mikhail Dorofeev, Kanato Tamashiro, 2023, Chapter 14, 978-3-031-26595-2, 165, 10.1007/978-3-031-26596-9_14 | |
21. | Solomon Eghosa Uhunamure, Tsangyao Chang, 2023, Chapter 10, 978-3-031-26595-2, 115, 10.1007/978-3-031-26596-9_10 | |
22. | Md. Mominur Rahman, Rizwan Ullah Khan, 2024, Chapter 18, 978-3-031-51531-6, 221, 10.1007/978-3-031-51532-3_18 | |
23. | Mikhail Dorofeev, Vikas Khare, 2024, Chapter 19, 978-3-031-51531-6, 233, 10.1007/978-3-031-51532-3_19 | |
24. | Mir Sayed Shah Danish, Emerson Guzzi Zuan Esteves, 2023, Chapter 12, 978-3-031-26595-2, 141, 10.1007/978-3-031-26596-9_12 | |
25. | Solomon Eghosa Uhunamure, Abderrahmen Bouchenine, 2024, Chapter 23, 978-3-031-51531-6, 283, 10.1007/978-3-031-51532-3_23 | |
26. | Md. Mominur Rahman, 2024, Chapter 17, 978-3-031-51531-6, 209, 10.1007/978-3-031-51532-3_17 | |
27. | Kanato Tamashiro, Raya Karlibaeva, Diana Stepanova, 2024, Chapter 12, 978-3-031-51531-6, 147, 10.1007/978-3-031-51532-3_12 | |
28. | Tomonobu Sengyu, Vikas Khare, 2023, Chapter 8, 978-3-031-26595-2, 87, 10.1007/978-3-031-26596-9_8 | |
29. | Alexey Mikhaylov, 2024, Chapter 9, 978-3-031-51531-6, 111, 10.1007/978-3-031-51532-3_9 | |
30. | Laura M. Baitenova, Lyailya M. Mutaliyeva, Tarek Ismail Mohamed, 2024, Chapter 2, 978-3-031-51531-6, 13, 10.1007/978-3-031-51532-3_2 | |
31. | Yulia Budaeva, David Philippov, Tsangyao Chang, 2023, Chapter 5, 978-3-031-26595-2, 47, 10.1007/978-3-031-26596-9_5 |
Parameters | Constants |
D | 0.015 pu/Hz |
Ms | 0.1667 pu/s |
Twg | 1.5 s |
Tpv | 1.8 s |
Tb | 0.1 s |
Tfc | 4.0 s |
Tae | 0.5 s |
Gas-turbine generators | G1 | G2 | G3 | G4 | |
rated power | PG [MW] | 324 | 482 | 292 | 417 |
Inertia constant | H [s] | 6.3 | 7.2 | 5.8 | 6.8 |
d-axis synchronous reactance | xd [pu] | 1.57 | 1.63 | 1.53 | 1.68 |
d-axis transient reactance | x′d [pu] | 0.261 | 0.268 | 0.298 | 0.312 |
q-axis synchronous reactance | xq [pu] | 1.06 | 0.98 | 1.12 | 0.98 |
q-axis transient reactance | x′q [pu] | 0.177 | 0.189 | 0.173 | 0.192 |
d-axis open-circuit transient time constant | T′d0 [s] | 6.1 | 7.5 | 5.7 | 7.6 |
q-axis open-circuit transient time constant | T′q0 [s] | 0.64 | 0.41 | 0.72 | 0.47 |
Amplifier gain | KA | 50 | 50 | 50 | 50 |
Amplifier time constant | TA [s] | 0.06 | 0.06 | 0.06 | 0.06 |
Governor time constant | TG [s] | 4.0 | 4.0 | 4.0 | 4.0 |
line resistance | r [pu] | 1.1048 |
line reactance | x [pu] | 4.6954 |
Wind-turbine generator | WG1 | WG2 | WG3 | ||
Rated power | Pwg [MW] | 10 | 7 | 8 | |
Photovoltaic generator | PV1 | PV2 | PV3 | ||
Rated power | Ppv [MW] | 140 | 140 | 140 | |
Battery | 1 | 2 | 3 | 4 | |
Inverter capacity | [MVA] | 25 | 25 | 25 | 25 |
Storage capacity | [MWh] | 100 | 100 | 100 | 100 |
Load 1, 2, 3, 5 | Uncontrollable load | 100 MW |
Controllable load | 75 MW | |
Load 4, 6 | Uncontrollable load | 200 MW |
Controllable load | 150 MW | |
Total | Uncontrollable load | 800 MW |
Controllable load | 600 MW |
Parameters | Constants |
D | 0.015 pu/Hz |
Ms | 0.1667 pu/s |
Twg | 1.5 s |
Tpv | 1.8 s |
Tb | 0.1 s |
Tfc | 4.0 s |
Tae | 0.5 s |
Gas-turbine generators | G1 | G2 | G3 | G4 | |
rated power | PG [MW] | 324 | 482 | 292 | 417 |
Inertia constant | H [s] | 6.3 | 7.2 | 5.8 | 6.8 |
d-axis synchronous reactance | xd [pu] | 1.57 | 1.63 | 1.53 | 1.68 |
d-axis transient reactance | x′d [pu] | 0.261 | 0.268 | 0.298 | 0.312 |
q-axis synchronous reactance | xq [pu] | 1.06 | 0.98 | 1.12 | 0.98 |
q-axis transient reactance | x′q [pu] | 0.177 | 0.189 | 0.173 | 0.192 |
d-axis open-circuit transient time constant | T′d0 [s] | 6.1 | 7.5 | 5.7 | 7.6 |
q-axis open-circuit transient time constant | T′q0 [s] | 0.64 | 0.41 | 0.72 | 0.47 |
Amplifier gain | KA | 50 | 50 | 50 | 50 |
Amplifier time constant | TA [s] | 0.06 | 0.06 | 0.06 | 0.06 |
Governor time constant | TG [s] | 4.0 | 4.0 | 4.0 | 4.0 |
line resistance | r [pu] | 1.1048 |
line reactance | x [pu] | 4.6954 |
Wind-turbine generator | WG1 | WG2 | WG3 | ||
Rated power | Pwg [MW] | 10 | 7 | 8 | |
Photovoltaic generator | PV1 | PV2 | PV3 | ||
Rated power | Ppv [MW] | 140 | 140 | 140 | |
Battery | 1 | 2 | 3 | 4 | |
Inverter capacity | [MVA] | 25 | 25 | 25 | 25 |
Storage capacity | [MWh] | 100 | 100 | 100 | 100 |
Load 1, 2, 3, 5 | Uncontrollable load | 100 MW |
Controllable load | 75 MW | |
Load 4, 6 | Uncontrollable load | 200 MW |
Controllable load | 150 MW | |
Total | Uncontrollable load | 800 MW |
Controllable load | 600 MW |