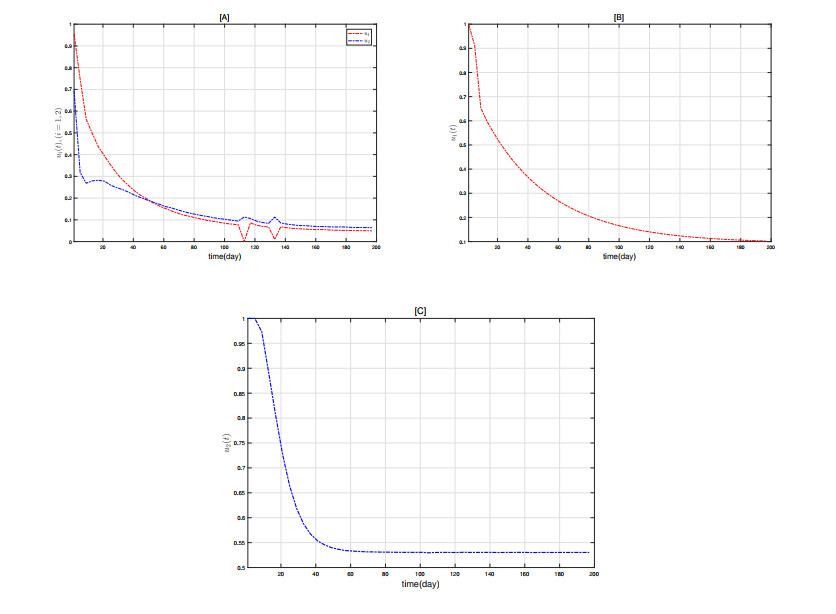
Chitin is the second most plentiful natural biomass after cellulose, with a yearly production of about 1 × 1010–1 × 1012 tonnes. It can be obtained mainly from sea crustaceans' shells, containing 15–40% chitin. Full or partial deacetylation of chitin generates chitosan. Chitin and chitosan are used in several industrial sectors, as they exhibit high biocompatibility, biodegradability and several biological functions (e.g., antioxidant, antimicrobial and antitumoral activities). These biopolymers' market trends are destined to grow in the coming years, confirming their relevance. As a result, low-cost and industrial-scale production is the main challenge. Scientific literature reports two major technologies for chitin and chitosan recovery from crustacean waste: chemical and biological methods. The chemical treatment can be performed using conventional solvents, typically strong acid and alkaline solutions, or alternative green solvents, such as deep eutectic solvents (DESs) and natural deep eutectic solvents (NADESs). Biological methods use enzymatic or fermentation processes. For each route, this paper reviews the advantages and drawbacks in terms of environmental and economic sustainability. The conventional chemical method is still the most used but results in high environmental impacts. Green chemical methods by DESs and NADESs use low-toxic and biodegradable solvents but require high temperatures and long reaction times. Biological methods are eco-friendly but have limitations in the upscaling process, and are affected by high costs and long reaction times. This review focuses on the methodologies available to isolate chitin from crustaceans, providing a comprehensive overview. At the same time, it examines the chemical, biological and functional properties of chitin and its derivative, along with their most common applications. Consequently, this work represents a valuable knowledge tool for selecting and developing the most suitable and effective technologies to produce chitin and its derivatives.
Citation: Alessandra Verardi, Paola Sangiorgio, Stefania Moliterni, Simona Errico, Anna Spagnoletta, Salvatore Dimatteo. Advanced technologies for chitin recovery from crustacean waste[J]. Clean Technologies and Recycling, 2023, 3(1): 4-43. doi: 10.3934/ctr.2023002
[1] | Hengjie Peng, Changcheng Xiang . A Filippov tumor-immune system with antigenicity. AIMS Mathematics, 2023, 8(8): 19699-19718. doi: 10.3934/math.20231004 |
[2] | Jun Moon . The Pontryagin type maximum principle for Caputo fractional optimal control problems with terminal and running state constraints. AIMS Mathematics, 2025, 10(1): 884-920. doi: 10.3934/math.2025042 |
[3] | Muhammad Farman, Aqeel Ahmad, Ali Akgül, Muhammad Umer Saleem, Kottakkaran Sooppy Nisar, Velusamy Vijayakumar . Dynamical behavior of tumor-immune system with fractal-fractional operator. AIMS Mathematics, 2022, 7(5): 8751-8773. doi: 10.3934/math.2022489 |
[4] | Meroua Medjoudja, Mohammed El hadi Mezabia, Muhammad Bilal Riaz, Ahmed Boudaoui, Saif Ullah, Fuad A. Awwad . A novel computational fractional modeling approach for the global dynamics and optimal control strategies in mitigating Marburg infection. AIMS Mathematics, 2024, 9(5): 13159-13194. doi: 10.3934/math.2024642 |
[5] | Hongyan Wang, Shaoping Jiang, Yudie Hu, Supaporn Lonapalawong . Analysis of drug-resistant tuberculosis in a two-patch environment using Caputo fractional-order modeling. AIMS Mathematics, 2024, 9(11): 32696-32733. doi: 10.3934/math.20241565 |
[6] | Sayed Saber, Azza M. Alghamdi, Ghada A. Ahmed, Khulud M. Alshehri . Mathematical Modelling and optimal control of pneumonia disease in sheep and goats in Al-Baha region with cost-effective strategies. AIMS Mathematics, 2022, 7(7): 12011-12049. doi: 10.3934/math.2022669 |
[7] | Zahra Pirouzeh, Mohammad Hadi Noori Skandari, Kamele Nassiri Pirbazari, Stanford Shateyi . A pseudo-spectral approach for optimal control problems of variable-order fractional integro-differential equations. AIMS Mathematics, 2024, 9(9): 23692-23710. doi: 10.3934/math.20241151 |
[8] | Ahmed J. Abougarair, Mohsen Bakouri, Abdulrahman Alduraywish, Omar G. Mrehel, Abdulrahman Alqahtani, Tariq Alqahtani, Yousef Alharbi, Md Samsuzzaman . Optimizing cancer treatment using optimal control theory. AIMS Mathematics, 2024, 9(11): 31740-31769. doi: 10.3934/math.20241526 |
[9] | Changjin Xu, Chaouki Aouiti, Zixin Liu, Qiwen Qin, Lingyun Yao . Bifurcation control strategy for a fractional-order delayed financial crises contagions model. AIMS Mathematics, 2022, 7(2): 2102-2122. doi: 10.3934/math.2022120 |
[10] | Devendra Kumar, Jogendra Singh, Dumitru Baleanu . Dynamical and computational analysis of a fractional predator-prey model with an infectious disease and harvesting policy. AIMS Mathematics, 2024, 9(12): 36082-36101. doi: 10.3934/math.20241712 |
Chitin is the second most plentiful natural biomass after cellulose, with a yearly production of about 1 × 1010–1 × 1012 tonnes. It can be obtained mainly from sea crustaceans' shells, containing 15–40% chitin. Full or partial deacetylation of chitin generates chitosan. Chitin and chitosan are used in several industrial sectors, as they exhibit high biocompatibility, biodegradability and several biological functions (e.g., antioxidant, antimicrobial and antitumoral activities). These biopolymers' market trends are destined to grow in the coming years, confirming their relevance. As a result, low-cost and industrial-scale production is the main challenge. Scientific literature reports two major technologies for chitin and chitosan recovery from crustacean waste: chemical and biological methods. The chemical treatment can be performed using conventional solvents, typically strong acid and alkaline solutions, or alternative green solvents, such as deep eutectic solvents (DESs) and natural deep eutectic solvents (NADESs). Biological methods use enzymatic or fermentation processes. For each route, this paper reviews the advantages and drawbacks in terms of environmental and economic sustainability. The conventional chemical method is still the most used but results in high environmental impacts. Green chemical methods by DESs and NADESs use low-toxic and biodegradable solvents but require high temperatures and long reaction times. Biological methods are eco-friendly but have limitations in the upscaling process, and are affected by high costs and long reaction times. This review focuses on the methodologies available to isolate chitin from crustaceans, providing a comprehensive overview. At the same time, it examines the chemical, biological and functional properties of chitin and its derivative, along with their most common applications. Consequently, this work represents a valuable knowledge tool for selecting and developing the most suitable and effective technologies to produce chitin and its derivatives.
Lung cancer is a prevalent cancer globally and a major cause of cancer deaths, responsible for 18% of cancer-related mortality and 11% of cancer cases[1,2,3,4]. It not only affects the health and quality of life of millions of people but also places a tremendous burden on global healthcare systems. The occurrence of lung cancer stems from gene mutations and unregulated growth control, leading to abnormal cell proliferation[5]. These abnormally proliferating cells gradually form tumors, which can infiltrate nearby tissues and metastasize.
Lung cancer risk factors are smoking, occupational hazards, air pollution, and genetics. Smoking is the most significant causative factor, greatly increasing the likelihood of developing the disease[6]. Additionally, prolonged exposure to air pollution, radiation, and chemicals like heavy metals can also raise lung cancer risk[7]. People working in the mining, construction, and chemical industries face higher risks due to occupational exposure[8]. Genetic factors also play a role in some cases, with individuals having a family history of lung cancer being at a higher risk[9]. These factors together raise the likelihood of lung cancer.
The metastasis and spread of cancer cells are among the primary reasons why lung cancer is so deadly [10]. Cancer cells spread via the blood and lymphatic systems, such as the brain, bones, and liver, forming metastatic tumors[11]. These tumors severely disrupt the structure and function of the affected organs and weaken the immune system, leading to decreased resistance and making patients more susceptible to infections and other diseases[12]. The presence of metastatic tumors not only affects the patient's quality of life but also makes treatment more complex and difficult, thereby further increasing the mortality rate of lung cancer.
Cancer treatments encompass chemotherapy, immunotherapy, targeted therapy, radiation, and surgical procedures[13,14]. Surgery is suitable for early-stage lung cancer and involves removing the tumor and affected tissues to control the disease[15]. Radiation therapy uses high-energy rays to kill cancer cells and is often combined with surgery or chemotherapy. Chemotherapy uses drugs to kill or inhibit the growth of cancer cells and is applicable for advanced lung cancer or as adjuvant therapy after surgery[16]. Targeted therapy employs specific drugs to attack the genes or proteins of cancer cells, reducing damage to normal cells[17]. Immunotherapy, an emerging treatment method, boosts the immune system to identify and destroy cancer cells. In order to quickly develop effective treatment strategies, we need to thoroughly study the interaction mechanisms between cancer cells and other cells.
Some researchers have attempted to investigate the interactions between tumor cells and immune cells using mathematical models to better understand the dynamic characteristics of cancer[18,19,20,21,22]. These models not only capture the interactions between different cell types but also account for factors such as immune evasion, changes in the tumor microenvironment, and the effects of drug treatments. By simulating tumor progression under various environmental and therapeutic conditions, researchers can predict the trajectory of tumor development, evaluate treatment efficacy, and provide support for personalized treatment strategies[23]. These models are particularly valuable in the context of immunotherapy, targeted therapy, and other emerging treatments as they help optimize therapeutic approaches, minimize side effects, and promote more precise cancer treatment.
With the development of fractional calculus, its application in modeling cancer growth has become increasingly widespread[24,25,26,27,28]. In particular, the Caputo derivative, which can more accurately describe systems with memory effects and historical dependence, has shown significant advantages in simulating complex biological processes[26]. Studies have shown that fractional-order models, when analyzing the impact of chemotherapy on cancer cell growth, align better with experimental data and more accurately reflect the interactions between cancer cells, chemotherapy drugs, and immune cells compared to integer-order models[29,30,31]. Since fractional-order models can capture the non-locality and long-term memory effects of the system, they have demonstrated higher precision and effectiveness in cancer modeling, drawing increasing attention from researchers.
Lung cancer, as a high-mortality cancer, has attracted many researchers who use fractional-order mathematical models to analyze the dynamic behavior of its cells and their interactions with other cells[32,33,34,35,36]. Amilo et al.[34] established a Nablara discrete fractional-order model, revealing how the growth of lung epithelial cells and their interactions with immune cells promote the increase in the number of lung cancer cells. At the same time, they combined immunotherapy with targeted therapy to analyze the effects of these two treatment strategies on cancer cells and explored in-depth the role of genetic mutations in the spread of cancer cells[35]. In addition, Özköse et al.[36] developed a model that includes lung cancer tumor cells, lung cancer stem cells, CD8+ T cells, interleukin-12 (IL-12), and natural immune cells, studying the dynamic relationships between these factors and cell types. The results showed that after tumor formation, an increase in the number of CD8+ T cells significantly reduced the numbers of cancer stem cells and tumor cells.
In the aforementioned studies, the dynamic interactions between cancer cells and immune cells, as well as the effects of different treatment strategies on cancer cells, have been effectively modeled. However, optimizing these interactions during the treatment process to achieve the best therapeutic outcomes remains an important and unresolved issue. To address this, many researchers have focused on optimal control under various treatment strategies, aiming to determine the best control policies to inhibit cancer growth and spread through mathematical models[37,38,39,40,41,42]. For example, one study analyzed the optimal treatment plan combining chemotherapy with a healthy lifestyle, and the results showed that this combination enhances the effectiveness of chemotherapy and reduces side effects [43]. Another study explored the optimal combination of surgery and programmed cell death ligand 1 (PD-L1) monoclonal antibody immunotherapy in the treatment of lung cancer, and the findings also indicated that the combined therapy significantly improves patient prognosis compared to single treatments[44]. Through these studies, researchers aim to provide more precise guidance for clinical treatment and optimize therapeutic outcomes.
This paper establishes a Caputo fractional-order model for lung cancer, aiming to explore the dynamics of lung cancer from two key aspects. First, the model focuses on the complex interactions between lung cancer cells, immune cells, healthy cells, and metastatic cancer cells, revealing the underlying mechanisms involved in tumor growth and cancer cell metastasis. Second, the model incorporates surgery and immunotherapy as intervention factors to evaluate the impact of different treatment strategies on tumor growth rate, immune response, and other dynamic changes. Through these studies, this paper aims to provide theoretical support for lung cancer treatment, enhance the understanding of tumor evolution under different treatment interventions, and provide scientific guidance for optimizing clinical treatment strategies.
The key contributions and innovations of this paper are as follows:
a. Unlike [37], the new model presented in this paper takes into account the interaction between healthy cells and tumor cells in nutrient competition. This competitive mechanism inhibits tumor cell growth, leading to a more comprehensive description of its growth dynamics.
b. Existing research on fractional-order optimization control often overlooks the time-dependent changes in control parameters and the performance of the objective function under different treatment strategies[38,39,40]. This paper analyzes these issues through numerical simulations and demonstrates the impact of different treatment strategies on control parameters and the objective function, as shown in Figures 1 and 2.
c. Based on real data regarding the changes in cancer cells in lung cancer patients, we evaluated the model parameters using the least squares method and performed curve fitting.
The remainder of this paper is organized as follows: Section 2 introduces the model construction and key definitions. Section 3 analyzes the conditions for the existence and uniqueness of the solution. Section 4 explores the stability theorems of the equilibrium points. Section 5 investigates the combined optimization strategy of surgery and immunotherapy, proposing the conditions for the existence of an optimal solution and deriving its expression. Section 6 validates the theory through numerical simulations. Section 7 performs parameter evaluation and curve fitting using real data. Finally, Section 8 summarizes the main conclusions of this paper.
Bashkirtseva et al. [23] developed a three-dimensional system based on the Lotka-Volterra competition model to study the dynamic interactions between tumor cells, immune cells, and healthy cells. The system considers the competition between healthy cells and tumor cells and introduces the stimulatory effect of tumor cell proliferation on immune cell proliferation. Specifically, tumor cell proliferation stimulates the growth of immune cells, but the proliferation of immune cells is not unlimited. Therefore, the authors use a second-type functional response function to describe this relationship. The system is
{˙T=r1T(1−Tk1)−a12TH−a13TE,˙H=r2H(1−Hk2)−a21HT,˙E=r3ETT+k3−a31ET−d3E, | (2.1) |
where T, H, and E represent tumor cells, healthy cells, and immune cells, respectively. ri and ki (i=1,2,3) denote the growth rate and the maximum carrying capacity of each cell type. a12 represents the competition coefficient between healthy cells and tumor cells, a13 represents the killing rate of tumor cells by effector cells, a21 represents the rate at which tumor cells inactivate healthy cells, a31 represents the rate at which tumor cells kill immune cells, and d3 represents the death rate of immune cells.
Meanwhile, in order to investigate the interactions between lung cancer cells, immune cells, and disseminated cancer cells, Amilo et al.[37] developed a three-dimensional system. In this system, in addition to considering the stimulatory effect of cancer cell proliferation on the immune system, it also takes into account the natural proliferation rate of immune cells in the absence of cancer cells. This three-dimensional system is
{dαN(t)dtα=λN(t)(1−N(t)K)−μN(t)P(t)−β1N(t)I(t),dαI(t)dtα=ϕ1I0+ϕ2N(t)2−ϕ3I(t)−β2I(t)N(t),dαP(t)dtα=γN(t)P(t)−δP(t)−β3I(t)P(t), | (2.2) |
where N(t), P(t), and I(t) represent the number of lung cancer cells, disseminated cancer cells, and immune cells, respectively. λ, K, μ, and β1 denote the growth rate of lung cancer cells, carrying capacity, spread rate, and the rate at which immune cells eliminate cancer cells. ϕ1, ϕ2, and ϕ3 are the factors influencing immune cells. β2 indicates the rate at which cancer cells kill immune cells, while γ, δ, and β3 represent the spread rate, death rate of disseminated cancer cells, and the rate at which immune cells destroy them. The fractional order of the Caputo derivative α ranges from 0<α≤1.
Inspired by systems (2.1) and (2.2) and considering that when the number of cancer cells becomes too high, the lack of nutrients or oxygen may lead to the death or slowed proliferation of cancer cells, resulting in a gradual reduction in the rate of cancer cell spread and eventually leading to stabilization. To better describe this phenomenon, we use a second-order functional response function to model the dynamic process of cancer cell diffusion. This function effectively simulates the rapid spread of cancer cells in the early stages, and as the number of tumor cells increases, resource limitations gradually become apparent, causing the diffusion rate to slow down and eventually stabilize. Therefore, we have established a four-dimensional fractional-order system that includes lung cancer cells, immune cells, healthy cells, and diffusing cancer cells to analyze the dynamic behaviors of these cells, as schematically shown in Figure 3. The specific form of the system is as follows:
{DαT(t)=r1T(t)(1−T(t)K1)−μ1I(t)T(t)−λ1H(t)T(t)−c1T(t)P(t)a1+T(t),DαI(t)=s+c2T(t)I(t)a2+T(t)−μ2I(t)T(t)−d1I(t),DαH(t)=r2H(t)(1−H(t)K2)−μ3H(t)T(t),DαP(t)=c1T(t)P(t)a1+T(t)−λ2I(t)P(t)−d2P(t), | (2.3) |
where T(t), I(t), H(t), and P(t), respectively, represent the tumor cell density, immune cell density, healthy cell density, and tumor cell density diffused out at time t in the lungs. Other parameters are detailed in Table 1. To better understand the following content, we will first introduce some basic definitions and concepts.
Parameter | Definition | Unit |
r1 | The net growth rate of tumor cells | day−1[45] |
r2 | The net growth rate of healthy cells | day−1[46] |
K1 | The maximum carrying capacity of lung tissue for tumor cells | cells[47] |
K2 | The maximum carrying capacity of lung tissue for healthy cells | cells[47] |
μ1 | The tumor cell mortality rate induced by the immune response | cell−1 day−1 |
μ2 | The mortality rate of healthy cells induced by the immune response | cell−1 day−1[48] |
μ3 | The rate of healthy cell death due to tumor cells | cell−1 day−1 |
λ1 | The tumor cell mortality rate caused by competition with healthy cells | cell−1 day−1 |
λ2 | The mortality rate of disseminated tumor cells caused by the immune response | cell−1 day−1 |
c1 | The speed at which tumor cells spread from the lungs to other tissues | cell−1 day−1 |
c2 | The rate at which tumor cell formation stimulates the production of immune cells | day−1[49] |
a1 | The half-maximal carrying capacity of the tumor cell environment | cells |
a2 | The maximum response threshold of immune cells | cells[50] |
d1 | The natural apoptotic rate of immune cells | day−1[46] |
d2 | The natural death rate of metastatic tumor cells | day−1 |
s | The generation rate of immune cells in the absence of tumors | cell day−1[49] |
Definition 2.1. [33] The Riemann-Liouville fractional integral is given by
0Iαth(t)=1Γ(α)∫t0(t−τ)α−1h(τ)dτ, |
where α>0 and Γ(⋅) is the Gamma function.
Definition 2.2. [33] The Caputo derivative is given by:
C0Dαth(t)=0In−αt=1Γ(n−α)∫t0h(n)(τ)(t−τ)α−n+1dτ. |
To simplify the notation, we use Dαh(t) to represent the Caputo derivative operator C0Dαth(t).
Definition 2.3. [37] Let (X,d) be a metric space and T:X→X be a function. If there exists a constant 0≤k<1 such that for any x,y∈X the following holds, d(T(x),T(y))≤k⋅d(x,y), then T is called a Banach contraction.
Definition 2.4. [37] If a function f:Rn→Rm on a set D⊆Rn has a constant K≥0 such that for any two points x and y in D it holds that |f(x)−f(y)|≤K|x−y|, then the function is Lipschitz continuous on D.
Definition 2.5. [37] The Laplace transform of the Caputo fractional derivative is given by the following formula:
L(Dαf(t))=ϑαF(ϑ)−n−1∑j=0f(j)(0)ϑα−j−1,n−1<α≤n. |
We first focus on the existence and uniqueness of the solution to system (2.3). Since directly proving the solution to system (2.3) is challenging, we will transform it into
{DαX(t)=A1X(t)+T(t)A2X(t)+I(t)A3X(t)+H(t)A4X(t)+T(t)a1+T(t)A5X(t)+T(t)a2+T(t)A6X(t)+A7,X(0)=X0, | (3.1) |
where
X(t)=(T(t)I(t)H(t)P(t)),X(0)=(T(0)I(0)H(0)P(0)),A1=(r10000−d10000r20000−d2), |
A2=(−r1K1−μ1−λ100−μ20000−μ300000),A4=(0000000000−r2K200000), |
A3=(000000000000000−λ2),A5=(000−c100000000000c1), |
A6=(00000c20000000000),A7=(0s00). |
According to [33], we can provide the following definition:
Definition 3.1. [33] Let C∗[0,a] be the set of continuous column vectors X(t), where the components T(t), I(t), H(t), and P(t) of X(t) are continuous functions on the interval [0,a]. The norm of X∈C∗[0,a] is defined as follows:
‖X‖=supt|e−NtT(t)|+supt|e−NtI(t)|+supt|e−NtH(t)|+supt|e−NtP(t)|, | (3.2) |
if t>ε≥m, it can be represented as C∗ε[0,a] and Cε[0,a].
Definition 3.2. [33] If X∈C∗[0,a] satisfies the following conditions:
(1) (t,X(t))∈B, t∈[0,a], where B=[0,a]×L,
L={(T,I,H,P)∈R4+:|T|<e1,|I|<e2,|H|<e3,|P|<e4}, |
e1, e2, e3, and e1 are real numbers.
(2) X(t) satisfies the initial value problem (3.1).
At this point, X(t) is called the solution to initial value problem (3.1).
Theorem 3.1. For the initial value problem (3.1), there exists a unique solution X∈C∗[0,a].
Proof. Based on the relevant properties of Caputo fractional order, (3.1) can be reformulated as
I(1−α)ddtX(t)=A1X(t)+T(t)A2X(t)+I(t)A3X(t)+H(t)A4X(t)+T(t)a1+T(t)A5X(t)+T(t)a2+T(t)A6X(t)+A7. |
Thus,
X(t)=X(0)+Iα(A1X(t)+T(t)A2X(t)+I(t)A3X(t)+H(t)A4X(t)+T(t)a1+T(t)A5X(t)+T(t)a2+T(t)A6X(t)+A7). | (3.3) |
Let F:C∗[0,a]→C∗[0,a], and we obtain
FX(t)=X(0)+Iα(A1X(t)+T(t)A2X(t)+I(t)A3X(t)+H(t)A4X(t)+T(t)a1+T(t)A5X(t)+T(t)a2+T(t)A6X(t)+A7), | (3.4) |
then
e−Nt(FX1−FX2)=e−NtIα(A1(X1(t)−X2(t))+T(t)A2(X1(t)−X2(t))+I(t)A3(X1(t)−X2(t))+H(t)A4(X1(t)−X2(t))+T(t)a1+T(t)A5(X1(t)−X2(t))+T(t)a2+T(t)A6(X1(t)−X2(t))),≤1Γ(α)∫t0(t−s)α−1e−N(t−s)(X1(s)−X2(s))×e−Ns(A1+e1A2+e2A3+e3A4+e1a1A5+e1a2A6)ds≤A1+e1A2+e2A3+e3A4+e1a1A5+e1a2A6Nα‖X1−X2‖∫t0sα−1Γ(α)ds. |
This suggests
‖FX1−FX2‖≤A1+e1A2+e2A3+e3A4+e1a1A5+e1a2A6Nα‖X1−X2‖. |
When N→∞, we have
A1+e1A2+e2A3+e3A4+e1a1A5+e1a2A6Nα<1 |
and
‖FX1−FX2‖<‖X1−X2‖. |
Based on the definition of operator F in Eq (3.4) having a unique fixed point, it follows that Eq (3.3) exists a unique solution X∈C∗[0,τ]. From Eq (3.3), we can deduce that
X(t)=X(0)+tαΓ(α+1)(A1X(0)+T(0)A2X(0)+I(0)A3X(0)+H(0)A4X(0)+T(0)a1+T(0)A5X(0)+T(0)a2+T(0)A6X(0)+A7)+Iα+1(A1X′(t)+T′(t)A2X(t)+T(t)A2X′(t)+I′(t)A3X(t)+I(t)A3X′(t)+H′(t)A4X(t)+H(t)A4X′(t)+a1T′(t)(a1+T(t))A5X(t)+T(t)a1+T(t)A5X′(t)+a2T′(t)(a2+T(t))A6X(t)+T(t)a2+T(t)A6X′(t)) |
and
dX(t)dt=tα−1Γ(α)(A1X(0)+T(0)A2X(0)+I(0)A3X(0) +H(0)A4X(0)+T(0)a1+T(0)A5X(0)+T(0)a2+T(0)A6X(0)+A7) +Iα(A1X′(t)+T′(t)A2X(t)+T(t)A2X′(t)+I′(t)A3X(t) +I(t)A3X′(t)+H′(t)A4X(t)+H(t)A4X′(t)+a1T′(t)(a1+T(t))A5X(t) +T(t)a1+T(t)A5X′(t)+a2T′(t)(a2+T(t))A6X(t)+T(t)a2+T(t)A6X′(t)),e−NtX′(t)=e−Nt[tα−1Γ(α)(A1X(0)+T(0)A2X(0)+I(0)A3X(0) +H(0)A4X(0)+T(0)a1+T(0)A5X(0)+T(0)a2+T(0)A6X(0)+A7) +Iα(A1X′(t)+T′(t)A2X(t)+T(t)A2X′(t)+I′(t)A3X(t) +I(t)A3X′(t)+H′(t)A4X(t)+H(t)A4X′(t)+a1T′(t)(a1+T(t))A5X(t) +T(t)a1+T(t)A5X′(t)+a2T′(t)(a2+T(t))A6X(t)+T(t)a2+T(t)A6X′(t))]. |
This implies that X∈C∗ε[0,a]. From (3.3), we can deduce
dX(t)dt=ddtIα(A1X(t)+T(t)A2X(t)+I(t)A3X(t)+H(t)A4X(t)+T(t)a1+T(t)A5X(t)+T(t)a2+T(t)A6X(t)+A7),Iα−1dX(t)dt=Iα−1ddtIα(A1X(t)+T(t)A2X(t)+I(t)A3X(t)+H(t)A4X(t)+T(t)a1+T(t)A5X(t)+T(t)a2+T(t)A6X(t)+A7),DαX(t)=A1X(t)+T(t)A2X(t)+I(t)A3X(t)+H(t)A4X(t)+T(t)a1+T(t)A5X(t)+T(t)a2+T(t)A6X(t)+A7,X(0)=X0+Iα(A1X(t)+T(t)A2X(t)+I(t)A3X(t)+H(t)A4X(t)+T(t)a1+T(t)A5X(t)+T(t)a2+T(t)A6X(t)+A7). |
Therefore, the initial value problem of Eq (3.3) corresponds to the solution of Eq (3.1).
Theorem 3.2. The solution of system (2.3) is positively invariant in the set
R4+={X∈R4;X(0)≥0} |
and
X(t)=(T,I,H,P)T. |
That is, if X(0)>0, then the model remains greater than zero for t>0.
Proof. Obviously,
DαT(t)|T=0≥0,DαI(t)|I=0>0,DαH(t)|H=0≥0,DαP(t)|P=0≥0. |
We can derive the following conclusion from the first equation of system (2.3)
DαT=r1T(1−TK1)−μ1IT−λ1HT−c1TPa1+T≥−(r1K1T+μ1I+λ1H+c1Pa1+T)T. |
Let
T≤e1,I≤e2,H≤e3,P≤e4 |
and
λ3=r1K1e1+μ1e2+λ1e3+c1e4a1+e1. |
Therefore, we can obtain
DαT(t)≥−λ3T(t). | (3.5) |
Next, by applying the Laplace transform to (3.5), we derive
L(DαT(t))≥L(−λ3T(t)). |
Based on the Laplace transform of the Caputo fractional derivative, we have
ϑαL{T}(ϑ)−T(0)ϑα−1≥−λ3L{T}(ϑ) |
and
ϑαL{T}(ϑ)(ϑα+λ3)ϑα≥T(0)ϑ. |
Thus,
L{T(t)}≥ϑα−1T(0)(ϑα+λ3). |
By applying the inverse Laplace transform,
T(t)≥L−1{ϑα−1T(0)(ϑα+λ3)}. | (3.6) |
As each term on the right side of Eq (3.6) is a positive constant, T(t) is positive for any t>0. The positivity of the remaining three variables in system (2.3) can be proven similarly to T(t).
In this section, we will investigate the equilibria of system (2.3). First, we analyze the equilibria in the absence of tumor cells. Second, we explore the equilibria when tumor cells coexist with other cell populations. Finally, we examine the local stability of these equilibria under different parameter conditions. Based on
{r1T(1−TK1)−μ1IT−λ1HT−c1TPa1+T=0,s+c2TIa2+T−μ2IT−d1I=0,r2H(1−HK2)−μ3HT=0,c3TPa3+T−λ2IP−d2P=0, | (4.1) |
we can obtain two equilibria without tumor cells, denoted as E1=(0,sd1,0,0) and E2=(0,sd1,K2,0).
Basic Reproduction Number: As an important indicator for assessing the progression of cancer, we will then apply the next-generation matrix[51,52] method for calculation, namely,
ℜ0=ρ(FV−1), | (4.2) |
where F=∂F∂T and V=∂V∂T. It follows from (2.3) that
F(x)=(r1T(1−TK1)),V(x)=(μ1IT+λ1HT+c1TPa1+T) |
and
F=∂F∂T=r1−2r1K1T,V=∂V∂T=μ1I+λ1H+a1c1P(a1+T)2. | (4.3) |
After substituting E1 and E2, we get
F1=r1,V1=sμ1d1,F2=r1,V2=sμ1d1+λ1K2 |
and
V−11=d1sμ1,V−12=d1sμ1+d1λ1K2. |
Therefore, it can be concluded that
ℜ10=ρ(F1V−11)=r1d1sμ1,ℜ20=ρ(F2V−12)=r1d1sμ1+d1λ1K2. | (4.4) |
Next, we will analyze the existence of equilibria of system (2.3) when T≠0. According to the first equation of system (4.1), we can derive
P∗=a1+T∗c1[r1(1−T∗K1)−μ1I∗−λ1H∗]. |
From the second equation of system (4.1), we have
I∗=−s(a2+T∗)c2T∗−(μ2T∗+d1)(a2+T∗). |
From the third equation of system (4.1), it can be deduced that
H∗=K2r2−K2μ3T∗r2. |
Based on the fourth equation of system (4.1), we can obtain
I∗=(c1−d2)T∗−a1d2λ2(a1+T∗). |
Therefore, system (2.3) has an interior equilibrium E3=(T∗,I∗,H∗,P∗).
This section examines the local stability of tumor-free equilibria.
Lemma 4.1. If r1<μ1sd1+λ1K2, equilibrium point E2 exhibits local asymptotic stability.
Proof. The Jacobian matrix of system (2.3) is
J=(r1−2r1K1T−μ1I−λ1H−a1c1P(a1+T)2−μ1T−λ1T−c1Ta1+Ta2c2I(a2+T)2−μ2Ic2Ta2+T−μ2T−d100−μ3H0r2−2r2K2H−μ3T0a1c1P(a1+T)2−λ2P0c1Ta1+T−λ1I−d2). |
Thus, the Jacobian matrix at E1 is
JE2=(r1−μ1sd1−λ1K2000−c2s−a2μ2sa2d1−d100−μ3K20−r20000−λ2s+d1d2d1). |
Obviously, the eigenvalues of matrix JE2 are
λ1=r1−μ1sd1−λ1K2,λ2=−d1,λ3=−r2,λ4=−λ2s+d1d2d1. |
This means that when r1<μ1sd1+λ1K2, E2 exhibits local asymptotic stability.
Next, we will examine the local stability of the internal equilibria.
Lemma 4.2. If C1>0, C2>0, C3>0, and C4>0, then E3 is locally asymptotically stable.
Proof. The Jacobian matrix at E3 is
JE3=(−B1−μ1T−λ1T−B2−B3−B400−μ3H0−B50−B6−B70−B8), |
where
B1=2r1K1T∗+μ1I∗+λ1H∗+a1c1P∗(a1+T∗)2−r1,B2=c1T∗a1+T∗, |
B3=μ2I∗−a2c2I∗(a2+T∗)2,B4=μ2T∗+d−c2T∗a2+T∗, |
B5=2r2K2H∗+μ3T∗−r2,B6=a1c1P∗(a1+T∗)2, |
B7=λ2P∗,B8=λ2I∗+d2−c1T∗a1+T∗. |
Therefore, the characteristic polynomial of matrix JE3 is
f(λ)=|λE−JE3|=|λ+B1μ1Tλ1TB2B3λ+B400μ3H0λ+B50B6B70λ+B8|. |
Through calculation, we can obtain
f(λ)=λ4+(B1+B4+B5+B8)λ3+[B1B8+B2B6+B4B5+(B1+B8)(B4+B5)]λ2+[B2B3B7+B2B4B6+B2B5B6+B4B5(B1+B8)+B1B8(B4+B5)]λ+B2B3B5B7+B2B4B5B6+B1B4B5B8. |
According to the Hurwitz criterion[37], if C1>0, C2>0, C3>0, and C4>0, then E3 is locally asymptotically stable, where
C1=B1+B4+B5+B8, |
C2=B1B8+B2B6+B4B5+(B1+B8)(B4+B5), |
C3=B2B3B7+B2B4B6+B2B5B6+B4B5(B1+B8)+B1B8(B4+B5), |
C4=B2B3B5B7+B2B4B5B6+B1B4B5B8. |
We will continue to formulate a fractional-order optimal control problem, targeting model (2.3), and study and design an optimal control strategy. By analyzing the basic reproduction number, it is evident that parameters r1 and μ1 play critical roles in tumor control. To effectively regulate tumor cell numbers, we need to decrease r1 while enhancing μ1. This will achieve the goal of reducing tumor density and minimizing control costs. This approach not only improves treatment efficacy but also reduces the burden on patients while controlling the tumor.
In our optimal control problem, we introduce two control functions: u1(t) and u2(t). Here, u1(t) represents surgical treatment and u2(t) represents immunotherapy. Surgical treatment reduces the number of tumor cells by directly removing tumor tissue, while immunotherapy boosts the immune system's ability to target cancer cells. Through these measures, our aim is to minimize the objective function, thereby achieving optimal treatment outcomes while controlling costs.
From the above description, we introduce the following control model on the basis of the existing system (2.3) to address this issue more systematically. This model considers both the dynamic interaction between tumor cells and immune cells, as well as the effects of surgery and immunotherapy, aiming to achieve effective tumor control through optimized control strategies.
{DαT(t)=r1T(t)(1−u1(t))(1−T(t)K1)−(1+u2(t))μ1I(t)T(t)−λ1H(t)T(t)−c1T(t)P(t)a1+T(t),DαI(t)=s+c2T(t)I(t)a2+T(t)−μ2I(t)T(t)−d1I(t),DαH(t)=r2H(t)(1−H(t)K2)−μ3H(t)T(t),DαP(t)=c1T(t)P(t)a1+T(t)−λ2I(t)P(t)−d2P(t), | (5.1) |
The initial condition is T(0)=T0, I(0)=I0, H(0)=H0, and P(0)=P0. Our objective function is as follows:
J(u1,u2)=min∫tf0T(t)+D1u21+D2u22dt, | (5.2) |
D1 and D2 are both positive constants, representing the weights of the control measures' costs in the objective function. Meanwhile, we define the following control set:
U={u=(u1,u2)|ui(t),0≤ui(t)≤1,t∈[0,tf],i=1,2}, | (5.3) |
where ui is measurable.
The main challenge is to discover an optimal control solution (u∗1,u∗2) that minimizes the objective function J(u1,u2). Therefore, our initial focus will be on exploring the existence of this optimal control solution.
Theorem 5.1. If these conditions are all fulfilled:
(1) The control set U contains at least one element.
(2) The control set is both convex and closed.
(3) The righthand side of system (5.1) is subject to linear constraints on the state and control variables.
(4) The objective function's integrand exhibits convexity.
(5) Exist a constant σ and two positive numbers k1 and k2 such that the integrated function G(T,u1,u2,t) satisfies
G(T,u1,u2,t)≥k1(|u1(t)|2+|u2(t)|2)σ2−k2, | (5.4) |
then a set of controls u∗1,u∗2∈U can be found such that
J(u∗1,u∗2)=minJ(u),u∈U. | (5.5) |
Proof. According to the existence of bounded system solutions in Lukes' literature[53], condition (1) holds, and based on the definition of the control set, condition (2) also holds. Our focus is on proving the last three conditions, and below is the proof for condition (3).
By simplifying (5.1), we obtain
DαX(t)=M(X)=NX+O(X), |
where
X(t)=(T(t),I(t),H(t),P(t))T |
and
N=(r1(1−u1)0000−d10000r20000−d2),O(X)=(−r1(1−u1)K1T2−(1+u2)μ1IT−λ1HT−c1TPa1+Ts+c2TPa2+T−μ2IT−r2K2H2−μ3HTc1TPa1+T−λ2IP). |
Obviously, we can find a constant e∗ such that
T(t),I(t),H(t),P(t)<e∗. |
Let
X1(t)=(T1(t),I1(t),H1(t),P1(t)) |
and
X2(t)=(T2(t),I2(t),H2(t),P2(t)). |
According to the Hölder inequality[54], we can derive
|O(X1)−O(X2)|=r1(1−u1)K1|T21−T22|+μ1(1+u2)|I1T1−I2T2|+λ1|H1T1−H2T2| +2c1|T1P1a1+T1−T2P2a1+T2|+c2|T1I1a2+T1−T2I2a2+T2|+μ2|I1T1−I2T2| +r2K2|H21−H22|+μ3|H1T1−H2T2|+λ2|I1P1−I2P2|. |
Therefore, we have
|O(X1)−O(X2)|=r1(1−u1)K1|T21−T22|+[μ1(1+u2)+μ2]|I1T1−I2T2|+(λ1+μ3)|H1T1−H2T2| +2c1|T1P1a1+T1−T2P2a1+T2|+c2|T1I1a2+T1−T2I2a2+T2|+r2K2|H21−H22|+λ2|I1P1−I2P2|≤r1K1|T1+T2||T1−T2|+(2μ1+μ2)|I1||T1−T2|+(2μ1+μ2)|T2||I1−I2| +(λ1+μ3)|H1||T1−T2|+(λ1+μ3)|T2||H1−H2|+2c1|T1T2||P1−P2| +2a1c1|T1||P1−P2|+2a1c1|P2||T1−T2|+c2|T1T2||I1−I2|+a2c2|T1||I1−I2| +a2c2|I2||T1−T2|+r2K2|H1+H2||H1−H2|+λ2|I1||P1−P2|+λ2|I2||I1−I2|≤r1K1(|T1|+|T2|)|T1−T2|+(2μ1+μ2)|I1||T1−T2|+(2μ1+μ2)|T2||I1−I2| +(λ1+μ3)|H1||T1−T2|+(λ1+μ3)|T2||H1−H2|+2c1|T1T2||P1−P2| +2a1c1|T1||P1−P2|+2a1c1|P2||T1−T2|+c2|T1T2||I1−I2|+a2c2|T1||I1−I2| +a2c2|I2||T1−T2|+r2K2(|H1|+|H2|)|H1−H2|+λ2|I1||P1−P2|+λ2|P2||I1−I2|=[r1K1(|T1|+|T2|)+(2μ1+μ2)|I1|+(λ1+μ3)|H1|+2a1c1|P2|a2c2|I2|]|T1−T2| +[(2μ1+μ2)|T2|+c2|T1T2|+a2c2|T1|+λ2|P2|]|I1−I2| +[(λ1+μ3)|T2|+r2K2(|H1|+|H2|)]|H1−H2| +(2c1|T1T2|+2a1c1|T1|)|P1−P2|≤ϖ1|T1−T2|+ϖ2|I1−I2|+ϖ3|H1−H2|+ϖ4|P1−P2|≤θ(|T1−T2|+|I1−I2|+|H1−H2|+|P1−P2|), |
where
ϖ1=(2r1K1+2μ1+μ2+μ3+λ1+2a1c1+a2c2)e∗, |
ϖ2=(2μ1+μ2+λ2+a2c2)e∗+c2e∗2,ϖ3=(μ3+λ1+2r2K2)e∗, |
ϖ4=(2a1c1+λ2)e∗+2c1e∗2,θ=max{ϖ1,ϖ2,ϖ3,ϖ4}. |
This implies
|M(X1)−M(X2)|≤η|X1−X2|, |
where η=max{θ,‖N‖}<∞. Therefore, M is Lipschitz continuous. This implies that condition (3) is satisfied.
Next, we will demonstrate condition (4). Let
S(t,T,→u)=T(t)+D1u21(t)+D2u22(t), |
where →u=(u1,u2)∈U,→w=(w1,w2)∈U, and 0≤β≤1. To prove that S is convex, it suffices to demonstrate
(1−β)S(t,T,→u)+βS(t,T,→w)≥S(t,T,(1−β)→u+β→w), |
namely,
(1−β)S(t,T,→u)+βS(t,T,→w)−S(t,T,(1−β)→u+β→w)≥0. |
According to the definition of convex functions,
(1−β)S(t,T,→u)+βS(t,T,→w)−S(t,T,(1−β)→u+β→w)=(1−β)[T(t)+D1u21(t)+D2u22(t)]+β[T(t)+D1w21(t)+D2w22(t)]−[T(t)+D1((1−β)u1+βw1)2+D2((1−β)u2+βw2)2]. |
Derived through simplification,
(1−β)S(t,T,→u)+βS(t,T,→w)−S(t,T,(1−β)→u+β→w)=D1u21−βD1u21+D2u22−βD2u22+βD1w21+βD1w22−D1[(1−β)u1+βw1]2−D2[(1−β)u2+βw2]2=D1(1−β)β(u21−2u1w1+w21)+D2(1−β)β(u22−2u2w2+w22)=D1(1−β)β(u1−w1)2+D2(1−β)β(u2−w2)2≥0. |
Finally, our proof of condition (5) is as follows:
As S(t,T,→u) is a convex function, therefore,
T(t)+D1u21(t)+D2u22(t)≥φ1(|u1(t)|2+|u2(t)|2)ς2−φ2. |
In this case, if we choose φ1=12min{D1,D2}, φ2>0, and ς=2, then condition (5) is also satisfied.
Theorem 5.2. In the optimal control problem formulated by Eqs (5.1) and (5.2), if u∗=(u∗1,u∗2), X∗=(T∗,I∗,H∗,P∗) is the optimal solution, then there exists an adjoint variable δi, i=1,2,3,4 such that
{Dαδ1(t)=1+δ1[r1(1+u1)−2r1(1+u1)K1T−(1+u2)μ1I−λ1H−a1c1P(a1+T)]+δ2[a2c2I(a2+T)−μ2I]−δ3μ3H+δ4a1c1P(a1+T),Dαδ2(t)=−δ1(1+u2)μ1T+δ2(c2Ta2+T−μ2T−d1)−δ4λ2P,Dαδ3(t)=−δ1λ1T+δ3(r2−2r2K2H−μ3T),Dαδ4(t)=−δ1c1Ta1+T+δ4(c1Ta1+T−λ2I−d2), | (5.6) |
and the intercept condition is δi(tf)=0. The optimal control solution is
u∗1=min{max{0,δ1r1T(1−TK1)2D1},umax}, |
u∗2=min{max{0,δ1μ1IT2D2},umax}. |
Proof. We begin by defining the Lagrangian function Z1 and the Hamiltonian function Z2 as follows:
Z1=T(t)+D1u21(t)+D2u22(t), |
Z2=Z1+δ1[r1(1−u1)T(1−TK1)−(1+u2)μ1IT−λ1HT−c1TPa1+T]+δ2[s+c2TIa2+T−μ2IT−d1I]+δ3[r2H(1−HK2)−μ3HT]+δ4[c1TPa1+T−λ2IP−d2P], |
where δi,i=1,2,3,4 is the adjoint variable. By further applying the Pontryagin maximum principle [37], the following state equation can be derived:
{Dαδ1(t)=∂Z2∂T=1+δ1[r1(1+u1)−2r1(1+u1)K1T−(1+u2)μ1I−λ1H−a1c1P(a1+T)]+δ2[a2c2I(a2+T)−μ2I]−δ3μ3H+δ4a1c1P(a1+T),Dαδ2(t)=∂Z2∂I=−δ1(1+u2)μ1T+δ2(c2Ta2+T−μ2T−d1)−δ4λ2P,Dαδ3(t)=∂Z2∂H=−δ1λ1T+δ3(r2−2r2K2H−μ3T),Dαδ4(t)=∂Z2∂P=−δ1c1Ta1+T+δ4(c1Ta1+T−λ2I−d2), |
simultaneously satisfying the transversality condition
δ1(tf)=δ2(tf)=δ3(tf)=δ4(tf)=0. |
According to the necessary conditions of the control equation,
∂Z2∂ui=0,i=1,2. |
Therefore, the optimal control solution u∗=(u∗1,u∗2) is
u∗1=δ1r1T2D1(1−TK1),u∗2=δ1μ1IT2D2. |
As a result of u∗1(t),u∗1(t)∈U, the expression for the optimal control solution is
u∗1=min{max{0,δ1r1T(1−TK1)2D1},umax}, |
u∗2=min{max{0,δ1μ1IT2D2},umax}. |
In this section, we will conduct numerical simulations to validate the theoretical derivations and assess the model's accuracy. Additionally, we will discuss the complex dynamic behaviors of the system under different parameters and conditions. These simulations help in understanding the stability and response characteristics of the system and reveal various dynamic phenomena that may be encountered in practical applications. Through numerical simulations, we can intuitively observe and explain the nonlinear characteristics of the system, providing a reference for further research and applications. In the following figures, when K1=100, we set the initial values to [10,12,15,2]; when K1=10, the initial values are set to [5,6,7,2].
From Figure 4, it can be seen that in the initial period, both tumor cells and healthy cells increase due to the immune system not being severely compromised. However, as time progresses, the immune system becomes increasingly damaged, and cancer cells continue to proliferate and absorb a large amount of nutrients, leading to a decrease in healthy cells. Eventually, the four state variables stabilize. Additionally, when α increases, the number of healthy cells and cancer cells at equilibrium becomes closer and when α=1, their numbers are almost equal. This indicates that α has a significant impact on the stability of system (2.3).
To better analyze the impact of α on each state variable, Figure 5 presents the time series of the same state variable under different α values. From Figure 5[A], it can be seen that as α increases, the number of tumor cells at equilibrium continuously rises, indicating that higher α values accelerate the growth of tumor cells. Figures 5[B–D] show that as α increases, the number of immune cells, healthy cells, and metastatic cancer cells at equilibrium continuously decreases. However, it is noteworthy that the maximum number of healthy cells that can be reached is also higher with larger α values, possibly due to the stronger protective effect on healthy cells during the initial stages. These results indicate that α has a significant overall impact on the system and that fractional-order models have an advantage in capturing the dynamic behavior of the system, making them more suitable for predicting and analyzing complex scenarios.
The basic reproduction number is crucial in cancer treatment. To delve deeper into the effects of different parameters in the basic regeneration expression on state variables, Figures 6–9 respectively show the comparative impacts of varying r1, μ1, d1, and s on each state variable. From the figures, it can be seen that as r1 increases, the quantities of state variables T and P at equilibrium gradually increase, while I and H gradually decrease. Conversely, increases in μ1 and s have the opposite effect of r1, leading to decreases in T and P quantities and increases in I and H quantities.
Notably, in Figure 8[A], during the initial period, as d1 increases, T continuously increases while H gradually decreases. This is due to the high death rate of immune cells, resulting in a sharp decrease in their number. However, over time, the increase in tumor cells stimulates the proliferation of immune cells. As a result, the proliferation rate of immune cells far exceeds their death rate, leading to a higher immune cell production rate with larger d1 values, ultimately reducing the number of tumor cells.
Figure 10 shows the time series and phase plots of the nodes. Subsequently, by fixing other parameters and adjusting α from 0.84 to 0.9, the state variables no longer stabilize at the nodes but exhibit periodic fluctuations, eventually settling into a limit cycle, as shown in Figure 11. This indicates that as α changes, the system (2.3) transitions from one stable state to another, with increasingly complex dynamic behavior. To more clearly analyze the impact of α changes on the system dynamics, Figure 12 presents the time series and phase plots of each state variable under different α values, providing a more intuitive validation of our analysis.
Next, we will analyze the existence of chaos in system (2.3). First, we computed the Lyapunov exponent of system (2.3), which is a numerical feature that represents the average exponential divergence rate of nearby trajectories in phase space and is one of the key indicators for identifying chaotic motion. The Lyapunov exponents of system (2.3) are shown in Table 2 and Figure 13. By observing the Lyapunov exponents, we can see that since there is a positive Lyapunov exponent, the system is expected to exhibit chaotic behavior within the parameter range specified in Table 2.
t | LET | LEI | LEH | LEP | t | LET | LEI | LEH | LEP |
10 | -0.1551 | -0.0131 | -1.2056 | -1.3859 | 100 | 0.8621 | -0.1742 | -0.6623 | -1.0283 |
20 | 0.5700 | -0.5235 | -0.5740 | -0.6670 | 300 | 0.8337 | -0.3379 | -0.5994 | -1.2045 |
30 | 0.7545 | -0.3418 | -0.6011 | -0.6055 | 500 | 0.8833 | -0.2905 | -0.5432 | -1.1698 |
40 | 0.5516 | -0.6181 | -1.3066 | -1.3565 | 700 | 0.8744 | -0.3094 | -0.5554 | -1.1951 |
50 | 0.5659 | -0.4543 | -0.9360 | -1.1777 | 1000 | 0.8918 | -0.2897 | -0.5413 | -1.1829 |
Additionally, the dimension of a chaotic attractor serves as a key numerical measure for assessing its characteristics. In this study, we use the Lyapunov dimension to quantify the chaotic attractor's dimension in system (2.3)[55]. The calculation formula is
dL=j+j∑i=1LEi|LEj+1|, | (6.1) |
where j∑i=1LEi>0 and j∑i=1LEi+LEj+1<0. For model (2.3), it follows from LET=0.8918, LEI=−0.2897, LEH=−0.2897, and LEP=−1.1829 that LET+LEI+LEH=0.06077>0 and LET+LEI+LEH+LEP=−1.12213<0. Therefore, the Lyapunov dimension is dL≈3.05137, indicating that the model is a fractal with a dimension between 3 and 4.
To further investigate the chaotic phenomena in system (2.3), we selected a new set of parameters and continuously adjusted the value of α. We found that the system tends to exhibit chaos when α is around 0.85, as shown in Figure 14. To more intuitively demonstrate the presence of chaos, we plotted two-dimensional phase diagrams of some combinations of state variables (Figure 15), three-dimensional phase diagrams of combinations of three state variables (Figure 16), and three-dimensional plots of two state variables over time (Figure 17). Chaotic phenomena can be explained by the irregular division of tumor cells and the complex dynamic relationships between cells. When the system exhibits chaos, the unpredictability and extreme sensitivity of chaotic behavior make cancer treatment more complex and challenging.
Combining the above analysis, it can be observed that varying the value of α leads to a transition of system (2.3) from chaotic behavior to a stable limit cycle, suggesting the presence of bifurcations within system (2.3). From a dynamical systems perspective, bifurcation refers to a phenomenon in which the continuous variation of a parameter causes a sudden and significant change in the system's behavior, thereby altering its dynamical properties. Bifurcation diagrams are crucial tools for analyzing how system properties change with respect to parameter variations, and they aid in uncovering the underlying dynamics of the system. In this study, four parameters are chosen as bifurcation parameters, and their relationships with the system state variable T are examined. The corresponding bifurcation diagrams are presented in Figure 18.
Figure 18[A, C, D] illustrates the occurrence of period-doubling bifurcations in system (2.3) under specific parameter values α=0.8, s=0.3, and μ1=0.00187. In Figure 18[A], a period-doubling bifurcation is observed when r1=1.12, and the relationship between the chaotic state and the intensity of immunotherapy μ1 is also evident. Before μ1=0.0196, the system exhibits period-doubling bifurcations, transitioning into chaotic behavior thereafter. This suggests that even small variations in these parameters can significantly influence the system's dynamic behavior. Therefore, in cancer treatment, adjusting these parameters could lead to more effective therapeutic strategies. For instance, the value of r1 can be altered through surgery, while μ1 can be regulated via immunotherapy. Next, we will conduct numerical simulations to investigate the effects of these two treatment methods on cancer, providing further insight into their role and effectiveness in modulating system parameters.
We designate the treatment method combining surgery and immunotherapy as Treatment Strategy A, surgery alone as Treatment Strategy B, and immunotherapy alone as Treatment Strategy C. Figure 1 shows the time series of the control parameter ui(i=1,2) under the three treatment strategies. From Figure 1[A], it can be seen that in the comprehensive Treatment Strategy A, the highest intensity of treatment is not required, and as the treatment progresses, the intensity gradually decreases, eventually reaching a very small value.
Interestingly, in the early stages of treatment, surgery dominates, while over time, immunotherapy becomes increasingly important. This aligns with real-world scenarios, where in the early stages, a larger number of tumor cells are present and surgery is used to remove most of the tumor, while the remaining tumor cells are eliminated through immunotherapy. This indicates that through comprehensive treatment, cancer can be effectively controlled without requiring extremely high treatment intensity, gradually reducing the treatment intensity and improving the sustainability and effectiveness of the treatment.
Figure 1[B] illustrates the scenario where only surgical treatment is performed. Compared to treatment strategy A, surgical treatment requires a higher intensity of treatment initially, and the treatment intensity remains higher even when it stabilizes in the later stages. This presents several issues: The high intensity of treatment not only demands a lot from doctors but also requires highly advanced medical facilities, increasing the complexity of the treatment. Therefore, the advantages of comprehensive treatment are particularly prominent, as it can reduce the intensity of treatment while improving the sustainability and effectiveness of the treatment.
Figure 1[C] shows the time series of the control function when only immunotherapy is administered. It can be seen that immunotherapy alone requires a period of high-intensity treatment, and the intensity remains relatively high in the later stages, which could result in higher costs. Therefore, among these three treatment strategies, treatment strategy A is preferred, as it can reduce the overall treatment intensity while improving treatment efficacy and sustainability.
After analyzing the treatment intensity under the three treatment strategies, we further examined their impact on the objective function, as shown in Figure 2. The objective function reflects the overall effect and cost of the treatment. From the figure, it can be seen that the objective function value for treatment strategy A is the lowest, indicating that the combined treatment (surgery and immunotherapy) is optimal in terms of effectiveness and cost. In contrast, the objective function value for treatment strategy B (surgery only) is higher. Although surgical treatment is effective in some aspects, it requires a higher initial treatment intensity, resulting in higher overall costs and less favorable outcomes.
The objective function value for treatment strategy C (immunotherapy only) is the highest, suggesting that immunotherapy alone not only requires a prolonged period of high-intensity treatment but also incurs higher costs. Considering both the treatment effect and cost, treatment strategy A is clearly superior to the other two strategies. This indicates that combining surgery and immunotherapy can achieve the best treatment outcomes while reducing treatment intensity and costs. Future research and practical treatments should focus on combined treatment. Finally, Figure 19 presents the time series of various state variables under different control strategies. It can be seen that treatment strategy A has the best control effect on cancer cells, followed by treatment strategy B, while treatment strategy C shows relatively poorer results, although all strategies are better than no treatment at all. Specifically, treatment strategy A not only significantly reduces the number of cancer cells but also effectively protects the immune system and healthy cells. In contrast, treatment strategy B is slightly less effective in controlling cancer cells than strategy A but still performs significantly better than strategy C and no treatment.
Moreover, although treatment strategy C is not as effective as the other two strategies, it still manages to control the growth of cancer cells to some extent and better protects healthy cells and the immune system compared to no treatment. This indicates that although single treatment methods (such as immunotherapy alone) have limited effectiveness, they still have some therapeutic value.
Overall, treatment strategy A, by combining surgery and immunotherapy, achieves the best results, not only excelling in inhibiting cancer cells but also maximizing the protection of the immune system and healthy cells. Although treatment strategy B is not as effective as strategy A, it still provides significant therapeutic benefits. Treatment strategy C, despite being weaker, still has its role as an adjunctive treatment. Therefore, in practical applications, comprehensive treatment strategy A should be prioritized to achieve the best clinical outcomes and patient prognosis.
Parameter estimation (PE) is crucial in the identification and validation of cancer models. The occurrence and progression of cancer involve various complex biological processes, and accurately translating these processes into mathematical models is a significant challenge. Through PE, model parameters can be determined using real data within a reasonable range, ensuring that the model can more accurately represent the biological characteristics of cancer. Accurate PE not only improves the predictive accuracy of the model but also helps reveal the underlying biological mechanisms of cancer progression, providing stronger support for clinical decision-making, ultimately driving the optimization and personalized development of cancer treatment plans.
In this section, we used real data on the growth of lung cancer cells over 14 days from a patient at Kayseri Erciyes University Hospital, with specific data points including 50, 000, 80, 000, 80, 000, 80, 000, 100, 000, 140, 000, 140, 000, 200, 000, 200, 000, 240, 000, 240, 000, 200, 000, 180, 000, and 180, 000, as mentioned in reference [33]. Given the limited real data points and the relatively large number of model parameters, we used interpolation to increase the number of data points for fitting and then applied the least squares method. The specific fitting process and details are as follows:
Step 1: First, define the real experimental data, including the time series and tumor cell count. Since the experimental data points are limited, which makes it difficult to fully capture the changes in tumor cell growth, we use spline interpolation to generate more data points, allowing for a more accurate reflection of tumor cell growth at different stages.
Step 2: Second, initialize the model parameters, such as tumor growth rate, carrying capacity, etc., and set reasonable upper and lower bounds for each parameter to ensure the optimization results are biologically plausible. At the same time, configure the parameters of the least squares optimizer, such as the maximum number of iterations and convergence tolerance, to ensure the stability of the optimization process.
Step 3: Next, the lsqcurvefit function is employed to optimize the parameters by minimizing the sum of squared residuals between the model predictions and experimental data, thereby improving the fitting accuracy. Additionally, the Akaike information criterion and the Bayesian information criterion are used to mitigate the risk of overfitting.
Step 4: Finally, a comparison plot of the fitting results is created, showing the real data points, interpolated data, and the model's predicted curve. Additionally, a residual analysis plot is generated to evaluate the model's prediction bias at different time periods, as shown in Figure 20.
Using this method, the optimal fitting parameter value at α=0.9 was obtained, with the specific results shown in Table 3. In Figure 20[A], the optimal fitting curve is presented, where red points represent the observed real data, the blue curve represents the fitted curve, and green points indicate the interpolated data points. Figure 20[B] shows the residual analysis, where smaller differences from zero indicate better fitting performance. As seen in Figure 20, the fitting performance is relatively good between days 0–9, while it worsens between days 9–14. This may be due to larger fluctuations in the later data and the significant influence of the estimated parameter values on the fitting process. This also suggests that different prediction strategies should be applied based on the data characteristics of different stages when making predictions.
Parameter | Value (α=0.9) | Status |
r1 | 3.001424e-01 | Fitted |
r2 | 4.886303e-01 | Fitted |
K1 | 2.686256e+05 | Fitted |
K2 | 2.283360e+05 | Fitted |
μ1 | 1.000000e-05 | Fitted |
μ2 | 1.000000e-05 | Fitted |
μ3 | 1.000000e-05 | Fitted |
λ1 | 8.912330e-05 | Fitted |
λ2 | 8.875427e-05 | Fitted |
c1 | 9.836971e-04 | Fitted |
c2 | 9.874923e-04 | Fitted |
a1 | 2.942564e+04 | Fitted |
a2 | 2.959367e+04 | Fitted |
d1 | 1.144475e-02 | Fitted |
d2 | 1.004176e-02 | Fitted |
s | 4.801490e-01 | Fitted |
Cancer treatment remains a significant challenge. This paper introduces a new Caputo fractional-order model to describe the progression of lung cancer. The model includes tumor cells, immune cells, healthy cells, and the spread of tumor cells from the lungs to surrounding organs. The main objective of this paper is to analyze the complex dynamic behaviors between these cell populations and assess the impact of different treatment strategies on cancer cell growth. By incorporating Caputo fractional-order differential equations, we are able to capture the complex interactions between cells more accurately. Compared to traditional integer-order models, the fractional-order model has a clear advantage in describing the memory effects and genetic characteristics of biological systems. Its flexibility and accuracy make it better suited for simulating the growth and spread dynamics of tumor cells.
Our model not only considers the growth of lung tumors but also includes the process of tumor cell dissemination to surrounding organs. This spread is crucial in the progression of lung cancer, and an accurate model to predict tumor dissemination is essential for devising effective treatment strategies. Additionally, the dynamic changes of immune cells and healthy cells included in the model help us understand the role of the immune system in tumor progression and the impact of different treatment regimens on the immune system.
We investigated the system's solutions to ensure that subsequent research is both reasonable and biologically meaningful, focusing on their existence, uniqueness, and positivity. First, we discussed the existence and stability conditions of tumor-free equilibria and internal equilibria. Furthermore, we calculated the basic reproduction number to determine whether the tumor will deteriorate. These analyses lay the theoretical foundation for introducing control terms and conducting numerical simulations in subsequent studies, particularly in selecting sensitive parameters, laying the groundwork for analyzing how parameters affect the system.
Considering the different impacts of surgery and immunotherapy on tumors, we introduced control parameters into the model. Surgical treatment directly affects the natural growth rate r1 of tumor cells, while immunotherapy increases the killing rate μ1 of immune cells against tumor cells. By incorporating control parameters into these two aspects, we can simulate the effects of surgery and immunotherapy on the system. After introducing the control terms, we first theoretically proved the necessary conditions for the existence of optimal control solutions and then used Pontryagin's maximum principle to calculate the expressions for the optimal solutions.
We used numerical simulations to verify the correctness of the above theoretical derivations and explored some complex dynamic behaviors that are difficult to prove theoretically. First, we studied the system without the control terms and analyzed the effects of key parameters α, r1, and μ1 on the system's dynamics. The results showed that small variations in these parameters significantly affect the system's dynamic behavior. Since fractional-order systems exhibit more complex dynamic behaviors, they are more suitable for predicting the growth of tumor cells.
By continuously adjusting the parameters, we found that the system stabilizes at a node, as shown in Figure 8. This is a positive signal for cancer patients, indicating a lower number of cancer cells and higher numbers of healthy and immune cells. This suggests that as long as the immune system is strong enough, it can control tumor cells at a low level and ensure normal immune function, even if it cannot completely eliminate the tumor cells. Therefore, regular exercise and a consistent daily routine are crucial for both cancer prevention and treatment.
When only modifying α, the system transitions from a stable node to a stable limit cycle, as illustrated in Figure 9. Further parameter adjustments lead to chaotic phenomena, which can be explained by the irregular proliferation of tumor cells and the complex dynamic interactions between cells. Chaotic behavior is detrimental to cancer treatment because it complicates monitoring cell growth, necessitating multiple treatment approaches to stabilize the condition before devising a reasonable treatment strategy. Analysis shows that the system may exhibit bifurcation phenomena. Therefore, we selected r1, μ1, α, and s as bifurcation parameters and plotted the corresponding period-doubling or period-halving bifurcation diagrams. These diagrams indicate that small perturbations in these parameters can shift the system from one state to another, highlighting the importance of closely monitoring these parameter changes during cancer treatment.
After introducing controls, we studied three treatment strategies: simultaneous surgery and immunotherapy (Strategy A), surgery alone (Strategy B), and immunotherapy alone (Strategy C). Through numerical simulations, we evaluated the required control intensity, the size of the objective function, and the dynamic changes of each state variable for these three treatment strategies during the treatment process. The results showed that the combined treatment strategy performed best in terms of treatment intensity, efficacy, and cost, consistent with theoretical predictions. Surgery treatment was the next most effective, followed by immunotherapy. Regardless of the treatment strategy adopted, all were more effective than no treatment.
To validate the accuracy of the model, we used real data from lung cancer patients, recording the changes in cancer cells over a 14-day period. Given the limited amount of real data, we first applied spline interpolation to augment the dataset, then estimated parameters using the least squares method and performed curve fitting. Figure 18[A] shows the fitting results when α=0.9, while Figure 18[B] presents the residual analysis between the real data and the fitted data. It can be observed that the model fits well in the early stages, but the deviation increases in the later stages. This indicates that while the model effectively captures the trend of cancer cell changes, there are potential limitations in its ability to predict changes over longer periods.
Considering that in actual treatment processes, the monitored data may have a certain time delay, we plan to introduce time-delay parameters in future research. This will make the model more accurately reflect the dynamic processes in clinical treatment, simulating and predicting changes in tumor cells, immune cells, and healthy cells. By calibrating with actual clinical data, we can optimize treatment strategies, improve treatment outcomes, and reduce side effects and costs, thus providing more scientific theoretical support and effective clinical guidance for cancer treatment. Ultimately, this will help enhance patient outcomes.
Xingxiao Wu: Software, Writing; Lidong Huang: Idea, Methodology; Zhang Shan: Formal analysis; Wenjie Qin: Project Administration and Review Editing. All authors read and approved the final manuscript.
The authors declare they have not used Artificial Intelligence (AI) tools in the creation of this article.
This work is supported by National Natural Science Foundation of China (Nos. 12261104, 12361104), the Youth Talent Program of Xingdian Talent Support Plan (No. XDYC-QNRC-2022-0708), and the Yunnan Provincial Basic Research Program Project (Nos. 202401AT070036, 202301AT070016).
Declare that there are no conflicts of interest regarding the publication of this paper.
[1] |
Pighinelli L (2019) Methods of chitin production a short review. Am J Biomed Sci Res 3: 307–314. https://doi.org/10.34297/AJBSR.2019.03.000682 doi: 10.34297/AJBSR.2019.03.000682
![]() |
[2] | Research and Markets, Chitin and Chitosan Derivatives: Global Strategic Business Report. Research and Markets, 2022. Available from: https://www.researchandmarkets.com/reports/338576/chitin_and_chitosan_derivatives_global_strategic#: ~: text = In%20the%20changed%20post%20COVID-19%20business%20landscape%2C%20the, CAGR%20of%2018.4%25%20over%20the%20analysis%20period%202020-2027. |
[3] |
Ahmad SI, Ahmad R, Khan MS, et al. (2020) Chitin and its derivatives: Structural properties and biomedical applications. Int J Biol Macromol 164: 526–539. https://doi.org/10.1016/j.ijbiomac.2020.07.098 doi: 10.1016/j.ijbiomac.2020.07.098
![]() |
[4] |
Aranaz I, Alcántara AR, Civera MC, et al. (2021) Chitosan: An overview of its properties and applications. Polymers 13: 3256. https://doi.org/10.3390/polym13193256 doi: 10.3390/polym13193256
![]() |
[5] |
Islam N, Hoque M, Taharat SF (2023) Recent advances in extraction of chitin and chitosan. World J Microbiol Biotechnol 39: 28. https://doi.org/10.1007/s11274-022-03468-1 doi: 10.1007/s11274-022-03468-1
![]() |
[6] | Crini G (2019) Historical landmarks in the discovery of chitin, In: Crini G, Lichtfouse E, Sustainable Agriculture Reviews 35, 1 Ed., Cham: Springer, 1–47. https://doi.org/10.1007/978-3-030-16538-3_1 |
[7] |
Iber BT, Kasan NA, Torsabo D, et al. (2022) A review of various sources of chitin and chitosan in nature. J Renew Mater 10: 1097. https://doi.org/10.32604/jrm.2022.018142 doi: 10.32604/jrm.2022.018142
![]() |
[8] | Broek LA, Boeriu CG, Stevens CV (2019) Chitin and Chitosan: Properties and Applications, Hoboken: Wiley. https://doi.org/10.1002/9781119450467 |
[9] |
El Knidri H, Belaabed R, Addaou A, et al. (2018) Extraction, chemical modification and characterization of chitin and chitosan. Int J Biol Macromol 120: 1181–1189. https://doi.org/10.1016/j.ijbiomac.2018.08.139 doi: 10.1016/j.ijbiomac.2018.08.139
![]() |
[10] | Sawada D, Nishiyama Y, Langan P, et al. (2012) Water in crystalline fibers of dihydrate β-chitin results in unexpected absence of intramolecular hydrogen bonding. PLoS One 7: e39376. https://doi.org/10.1371/journal.pone.0039376 |
[11] |
Abidin NAZ, Kormin F, Abidin NAZ, et al. (2020) The potential of insects as alternative sources of chitin: An overview on the chemical method of extraction from various sources. Int J Mol Sci 21: 1–25. https://doi.org/10.3390/ijms21144978 doi: 10.3390/ijms21144978
![]() |
[12] |
Hahn T, Roth A, Ji R, et al. (2020) Chitosan production with larval exoskeletons derived from the insect protein production. J Biotechnol 310: 62–67. https://doi.org/10.1016/j.jbiotec.2019.12.015 doi: 10.1016/j.jbiotec.2019.12.015
![]() |
[13] | FAO, The State of World Fisheries and Aquaculture 2020. Sustainability in Action. FAO, 2020. Available from: https://doi.org/10.4060/ca9229en. |
[14] |
Kurita K (2006) Chitin and chitosan: Functional biopolymers from marine crustaceans. Marine Biotechnol 8: 203–226. https://doi.org/10.1007/s10126-005-0097-5 doi: 10.1007/s10126-005-0097-5
![]() |
[15] | Özogul F, Hamed I, Özogul Y, et al. (2018) Crustacean by-products, In: Varelis P, Melton L, Shahidi F, Encyclopedia of Food Chemistry, Amsterdam: Elsevier, 33–38. https://doi.org/10.1016/B978-0-08-100596-5.21690-9 |
[16] | Bastiaens L, Soetemans L, D'Hondt E, et al. (2019) Sources of chitin and chitosan and their isolation, In Broek LA, Boeriu CG, Stevens CV, Chitin and Chitosan: Properties and Applications, 1 Ed., Amsterdam: Elsevier, 1–34. https://doi.org/10.1002/9781119450467.ch1 |
[17] |
Jones M, Kujundzic M, John S, et al. (2020) Crab vs. mushroom: A review of crustacean and fungal chitin in wound treatment. Mar Drugs 18: 64. https://doi.org/10.3390/md18010064 doi: 10.3390/md18010064
![]() |
[18] |
Arbia W, Arbia L, Adour L, et al. (2013) Chitin extraction from crustacean shells using biological methods—A review. Food Hydrocolloid 31: 392–403. https://doi.org/10.1016/j.foodhyd.2012.10.025 doi: 10.1016/j.foodhyd.2012.10.025
![]() |
[19] | Chakravarty J, Yang CL, Palmer J, et al. (2018) Chitin extraction from lobster shell waste using microbial culture-based methods. Appl Food Biotechnol 5: 141–154. |
[20] |
Maddaloni M, Vassalini I, Alessandri I (2020) Green routes for the development of chitin/chitosan sustainable hydrogels. Sustain Chem 1: 325–344. https://doi.org/10.3390/suschem1030022 doi: 10.3390/suschem1030022
![]() |
[21] |
Kozma M, Acharya B, Bissessur R (2022) Chitin, chitosan, and nanochitin: Extraction, synthesis, and applications. Polymers 14: 3989. https://doi.org/10.3390/polym14193989 doi: 10.3390/polym14193989
![]() |
[22] | Morin-Crini N, Lichtfouse E, Torri G, et al. (2019) Fundamentals and applications of chitosan, In: Crini G, Lichtfouse E, Sustainable Agriculture Reviews 35, 1 Ed., Cham: Springer, 49–123. https://doi.org/10.1007/978-3-030-16538-3_2 |
[23] |
Arnold ND, Brück WM, Garbe D, et al. (2020) Enzymatic modification of native chitin and conversion to specialty chemical products. Mar Drugs 18: 93. https://doi.org/10.3390/md18020093 doi: 10.3390/md18020093
![]() |
[24] |
Fernando LD, Widanage MCD, Penfield J, et al. (2021) Structural polymorphism of chitin and chitosan in fungal cell walls from solid-state NMR and principal component analysis. Front Mol Biosci 8: 727053. https://doi.org/10.3389/fmolb.2021.727053 doi: 10.3389/fmolb.2021.727053
![]() |
[25] |
Kaya M, Mujtaba M, Ehrlich H, et al. (2017) On chemistry of γ-chitin. Carbohyd Polym 176: 177–186. https://doi.org/10.1016/j.carbpol.2017.08.076 doi: 10.1016/j.carbpol.2017.08.076
![]() |
[26] |
Cao S, Liu Y, Shi L, et al. (2022) N-Acetylglucosamine as a platform chemical produced from renewable resources: opportunity, challenge, and future prospects. Green Chem 24: 493–509. https://doi.org/10.1039/D1GC03725K doi: 10.1039/D1GC03725K
![]() |
[27] |
de Queiroz Antonino RSCM, Fook BRPL, de Oliveira Lima VA, et al. (2017) Preparation and characterization of chitosan obtained from shells of shrimp (Litopenaeus vannamei Boone). Mar Drugs 15: 141. https://doi.org/10.3390/md15050141 doi: 10.3390/md15050141
![]() |
[28] |
Yu Z, Lau D (2017) Flexibility of backbone fibrils in α-chitin crystals with different degree of acetylation. Carbohyd Polym 174: 941–947. https://doi.org/10.1016/j.carbpol.2017.06.099 doi: 10.1016/j.carbpol.2017.06.099
![]() |
[29] |
Philibert T, Lee BH, Fabien N (2017) Current status and new perspectives on chitin and chitosan as functional biopolymers. Appl Biochem Biotech 181: 1314–1337. https://doi.org/10.1007/s12010-016-2286-2 doi: 10.1007/s12010-016-2286-2
![]() |
[30] |
Philippova OE, Korchagina EV, Volkov EV, et al. (2012) Aggregation of some water-soluble derivatives of chitin in aqueous solutions: Role of the degree of acetylation and effect of hydrogen bond breaker. Carbohyd Polym 87: 687–694. https://doi.org/10.1016/j.carbpol.2011.08.043 doi: 10.1016/j.carbpol.2011.08.043
![]() |
[31] |
Younes I, Sellimi S, Rinaudo M, et al. (2014) Influence of acetylation degree and molecular weight of homogeneous chitosans on antibacterial and antifungal activities. Int J Food Microbiol 185: 57–63. https://doi.org/10.1016/j.ijfoodmicro.2014.04.029 doi: 10.1016/j.ijfoodmicro.2014.04.029
![]() |
[32] |
Hattori H, Ishihara M (2015) Changes in blood aggregation with differences in molecular weight and degree of deacetylation of chitosan. Biomed Mater 10: 015014. https://doi.org/10.1088/1748-6041/10/1/015014 doi: 10.1088/1748-6041/10/1/015014
![]() |
[33] |
Mourya VK, Inamdar NN, Choudhari YM (2011) Chitooligosaccharides: Synthesis, characterization and applications. Polym Sci Ser A+ 53: 583–612. https://doi.org/10.1134/S0965545X11070066 doi: 10.1134/S0965545X11070066
![]() |
[34] |
Errico S, Spagnoletta A, Verardi A, et al. (2022) Tenebrio molitor as a source of interesting natural compounds, their recovery processes, biological effects, and safety aspects. Compr Rev Food Sci Food Saf 21: 148–197. https://doi.org/10.1111/1541-4337.12863 doi: 10.1111/1541-4337.12863
![]() |
[35] | Elieh-Ali-Komi D, Hamblin MR (2016) Chitin and chitosan: Production and application of versatile biomedical nanomaterials. Int J Adv Res 4: 411–427. |
[36] |
Liang S, Sun Y, Dai X (2018) A review of the preparation, analysis and biological functions of chitooligosaccharide. Int J Mol Sci 19: 2197. https://doi.org/10.3390/ijms19082197 doi: 10.3390/ijms19082197
![]() |
[37] |
Park PJ, Je JY, Kim SK (2003) Angiotensin I converting enzyme (ACE) inhibitory activity of hetero-chitooligosaccharides prepared from partially different deacetylated chitosans. J Agr Food Chem 51: 4930–4934. https://doi.org/10.1021/jf0340557 doi: 10.1021/jf0340557
![]() |
[38] |
Muanprasat C, Chatsudthipong V (2017) Chitosan oligosaccharide: Biological activities and potential therapeutic applications. Pharmacol Therapeut 170: 80–97. https://doi.org/10.1016/j.pharmthera.2016.10.013 doi: 10.1016/j.pharmthera.2016.10.013
![]() |
[39] |
Abd El-Hack ME, El-Saadony MT, Shafi ME, et al. (2020) Antimicrobial and antioxidant properties of chitosan and its derivatives and their applications: A review. Int J Biol Macromol 164: 2726–2744. https://doi.org/10.1016/j.ijbiomac.2020.08.153 doi: 10.1016/j.ijbiomac.2020.08.153
![]() |
[40] |
Haktaniyan M, Bradley M (2022) Polymers showing intrinsic antimicrobial activity. Chem Soc Rev 51: 8584–8611. https://doi.org/10.1039/D2CS00558A doi: 10.1039/D2CS00558A
![]() |
[41] |
Karagozlu MZ, Karadeniz F, Kim SK (2014) Anti-HIV activities of novel synthetic peptide conjugated chitosan oligomers. Int J Biol Macromol 66: 260–266. https://doi.org/10.1016/j.ijbiomac.2014.02.020 doi: 10.1016/j.ijbiomac.2014.02.020
![]() |
[42] |
Jang D, Lee D, Shin YC, et al. (2022) Low molecular weight chitooligosaccharide inhibits infection of SARS-CoV-2 in vitro. J Appl Microbiol 133: 1089–1098. https://doi.org/10.1111/jam.15618 doi: 10.1111/jam.15618
![]() |
[43] |
Hemmingsen LM, Panchai P, Julin K, et al. (2022) Chitosan-based delivery system enhances antimicrobial activity of chlorhexidine. Front Microbiol 13: 1023083. https://doi.org/10.3389/fmicb.2022.1023083 doi: 10.3389/fmicb.2022.1023083
![]() |
[44] |
Odjo K, Al-Maqtari QA, Yu H, et al. (2022) Preparation and characterization of chitosan-based antimicrobial films containing encapsulated lemon essential oil by ionic gelation and cranberry juice. Food Chem 397: 133781. https://doi.org/10.1016/j.foodchem.2022.133781 doi: 10.1016/j.foodchem.2022.133781
![]() |
[45] |
Dai X, Hou W, Sun Y, et al. (2015) Chitosan oligosaccharides inhibit/disaggregate fibrils and attenuate amyloid β-mediated neurotoxicity. Int J Mol Sci 16: 10526–10536. https://doi.org/10.3390/ijms160510526 doi: 10.3390/ijms160510526
![]() |
[46] |
Einarsson JM, Bahrke S, Sigurdsson BT, et al. (2013) Partially acetylated chitooligosaccharides bind to YKL-40 and stimulate growth of human osteoarthritic chondrocytes. Biochem Biophys Res Commun 434: 298–304. https://doi.org/10.1016/j.bbrc.2013.02.122 doi: 10.1016/j.bbrc.2013.02.122
![]() |
[47] |
Hong S, Ngo DN, Kim MM (2016) Inhibitory effect of aminoethyl-chitooligosaccharides on invasion of human fibrosarcoma cells. Environ Toxicol Phar 45: 309–314. https://doi.org/10.1016/j.etap.2016.06.013 doi: 10.1016/j.etap.2016.06.013
![]() |
[48] |
Neyrinck AM, Catry E, Taminiau B, et al. (2019) Chitin–glucan and pomegranate polyphenols improve endothelial dysfunction. Sci Rep 9: 1–12. https://doi.org/10.1038/s41598-019-50700-4 doi: 10.1038/s41598-019-50700-4
![]() |
[49] |
Satitsri S, Muanprasat C (2020) Chitin and chitosan derivatives as biomaterial resources for biological and biomedical applications. Molecules 25: 5961. https://doi.org/10.3390/molecules25245961 doi: 10.3390/molecules25245961
![]() |
[50] |
Fernandez JG, Ingber DE (2014) Manufacturing of large-scale functional objects using biodegradable chitosan bioplastic. Macromol Mater Eng 299: 932–938. https://doi.org/10.1002/mame.201300426 doi: 10.1002/mame.201300426
![]() |
[51] |
Atay HY, Doğan LE, Ç elik E (2013) Investigations of self-healing property of chitosan-reinforced epoxy dye composite coatings. J Mater 2013: 1–7. https://doi.org/10.1155/2013/613717 doi: 10.1155/2013/613717
![]() |
[52] |
Sangiorgio P, Verardi A, Dimatteo S, et al. (2022) Valorisation of agri-food waste and mealworms rearing residues for improving the sustainability of Tenebrio molitor industrial production. J Insects Food Feed 8: 509–524. https://doi.org/10.3920/JIFF2021.0101 doi: 10.3920/JIFF2021.0101
![]() |
[53] | Xu D, Qin H, Ren D, et al. (2018) Influence of coating time on the preservation performance of chitosan/montmorillonite composite coating on tangerine fruits, 21st IAPRI World Conference on Packaging 2018—Packaging: Driving a Sustainable Future. https://doi.org/10.12783/iapri2018/24422 |
[54] |
Liu Y, Xu R, Tian Y, et al. (2023) Exogenous chitosan enhances the resistance of apple to Glomerella leaf spot. Sci Hortic-Amsterdam 309: 111611. https://doi.org/10.1016/j.scienta.2022.111611 doi: 10.1016/j.scienta.2022.111611
![]() |
[55] | Kardas I, Struszczyk MH, Kucharska M, et al. (2012) Chitin and chitosan as functional biopolymers for industrial applications, In: Navard P, The European Polysaccharide Network of Excellence (EPNOE), 1 Ed., Vienna: Springer, 329–373. https://doi.org/10.1007/978-3-7091-0421-7_11 |
[56] |
Kopacic S, Walzl A, Zankel A, et al. (2018) Alginate and chitosan as a functional barrier for paper-based packaging materials. Coatings 8: 235. https://doi.org/10.3390/coatings8070235 doi: 10.3390/coatings8070235
![]() |
[57] |
Kurek M, Benbettaieb N, Ščetar M, et al. (2021) Novel functional chitosan and pectin bio-based packaging films with encapsulated Opuntia-ficus indica waste. Food Biosci 41: 100980. https://doi.org/10.1016/j.fbio.2021.100980 doi: 10.1016/j.fbio.2021.100980
![]() |
[58] |
Hafsa J, ali Smach M, Khedher MR, et al. (2016) Physical, antioxidant and antimicrobial properties of chitosan films containing Eucalyptus globulus essential oil. LWT-Food Sci Technol 68: 356–364. https://doi.org/10.1016/j.lwt.2015.12.050 doi: 10.1016/j.lwt.2015.12.050
![]() |
[59] |
Elmacı SB, Gülgö r G, Tokatlı M, et al. (2015) Effectiveness of chitosan against wine-related microorganisms. Anton Leeuw Int J G 3: 675–686. https://doi.org/10.1007/s10482-014-0362-6 doi: 10.1007/s10482-014-0362-6
![]() |
[60] |
Quintela S, Villaran MC, de Armentia LI, et al. (2012) Ochratoxin a removal from red wine by several oenological fining agents: Bentonite, egg albumin, allergen-free adsorbents, chitin and chitosan. Food Addit Contam 29: 1168–1174. https://doi.org/10.1080/19440049.2012.682166 doi: 10.1080/19440049.2012.682166
![]() |
[61] |
Gassara F, Antzak C, Ajila CM, et al. (2015) Chitin and chitosan as natural flocculants for beer clarification. J Food Eng 166: 80–85. https://doi.org/10.1016/j.jfoodeng.2015.05.028 doi: 10.1016/j.jfoodeng.2015.05.028
![]() |
[62] |
Li K, Xing R, Liu S, et al. (2020) Chitin and chitosan fragments responsible for plant elicitor and growth stimulator. J Agr Food Chem 68: 12203–12211. https://doi.org/10.1021/acs.jafc.0c05316 doi: 10.1021/acs.jafc.0c05316
![]() |
[63] |
Rkhaila A, Chtouki T, Erguig H, et al. (2021) Chemical proprieties of biopolymers (chitin/chitosan) and their synergic effects with endophytic Bacillus species: Unlimited applications in agriculture. Molecules 26: 1117. https://doi.org/10.3390/molecules26041117 doi: 10.3390/molecules26041117
![]() |
[64] |
Feng S, Wang J, Zhang L, et al. (2020) Coumarin-containing light-responsive carboxymethyl chitosan micelles as nanocarriers for controlled release of pesticide. Polymers 12: 1–16. https://doi.org/10.3390/polym12102268 doi: 10.3390/polym12102268
![]() |
[65] |
Abdel-Ghany HM, Salem MES (2020) Effects of dietary chitosan supplementation on farmed fish; a review. Rev Aquac 12: 438–452. https://doi.org/10.1111/raq.12326 doi: 10.1111/raq.12326
![]() |
[66] |
Fadl SE, El-Gammal GA, Abdo WS, et al. (2020) Evaluation of dietary chitosan effects on growth performance, immunity, body composition and histopathology of Nile tilapia (Oreochromis niloticus) as well as the resistance to Streptococcus agalactiae infection. Aquac Res 51: 1120–1132. https://doi.org/10.1111/are.14458 doi: 10.1111/are.14458
![]() |
[67] |
Kong A, Ji Y, Ma H, et al. (2018) A novel route for the removal of Cu (Ⅱ) and Ni (Ⅱ) ions via homogeneous adsorption by chitosan solution. J Clean Prod 192: 801–808. https://doi.org/10.1016/j.jclepro.2018.04.271 doi: 10.1016/j.jclepro.2018.04.271
![]() |
[68] |
Lin Y, Wang H, Gohar F, et al. (2017) Preparation and copper ions adsorption properties of thiosemicarbazide chitosan from squid pens. Int J Biol Macromol 95: 476–483. https://doi.org/10.1016/j.ijbiomac.2016.11.085 doi: 10.1016/j.ijbiomac.2016.11.085
![]() |
[69] |
Zia Q, Tabassum M, Gong H, et al. (2019) a review on chitosan for the removal of heavy metals ions. J Fiber Bioeng Inform 12: 103–128. https://doi.org/10.3993/jfbim00301 doi: 10.3993/jfbim00301
![]() |
[70] |
Labidi A, Salaberria AM, Fernandes SCM, et al. (2019) Functional chitosan derivative and chitin as decolorization materials for methylene blue and methyl orange from aqueous solution. Materials 12: 361. https://doi.org/10.3390/ma12030361 doi: 10.3390/ma12030361
![]() |
[71] |
Song Z, Li G, Guan F, et al. (2018) Application of chitin/chitosan and their derivatives in the papermaking industry. Polymers 10: 389. https://doi.org/10.3390/polym10040389 doi: 10.3390/polym10040389
![]() |
[72] |
Kaur B, Garg KR, Singh AP (2020) Treatment of wastewater from pulp and paper mill using coagulation and flocculation. J Environ Treat Tech 9: 158–163. https://doi.org/10.47277/JETT/9(1)163 doi: 10.47277/JETT/9(1)163
![]() |
[73] |
Renault F, Sancey B, Charles J, et al. (2009) Chitosan flocculation of cardboard-mill secondary biological wastewater. Chem Eng J 155: 775–783. https://doi.org/10.1016/j.cej.2009.09.023 doi: 10.1016/j.cej.2009.09.023
![]() |
[74] |
Yang R, Li H, Huang M, et al. (2016) A review on chitosan-based flocculants and their applications in water treatment. Water Res 95: 59–89. https://doi.org/10.1016/j.watres.2016.02.068 doi: 10.1016/j.watres.2016.02.068
![]() |
[75] |
Kjellgren H, Gä llstedt M, Engströ m G, et al. (2006) Barrier and surface properties of chitosan-coated greaseproof paper. Carbohyd Polym 65: 453–460. https://doi.org/10.1016/j.carbpol.2006.02.005 doi: 10.1016/j.carbpol.2006.02.005
![]() |
[76] | Agusnar H, Nainggolan I (2013) Mechanical properties of paper from oil palm pulp treated with chitosan from horseshoe crab. Adv Environ Biol 7: 3857–3861. |
[77] |
Habibie S, Hamzah M, Anggaravidya M, et al. (2016) The effect of chitosan on physical and mechanical properties of paper. J Chem Eng Mater Sci 7: 1–10. https://doi.org/10.5897/JCEMS2015.0235 doi: 10.5897/JCEMS2015.0235
![]() |
[78] | Dev VRG, Neelakandan R, Sudha S, et al. (2005) Chitosan A polymer with wider applications. Text Mag 46: 83. |
[79] | Enescu D (2008) Use of chitosan in surface modification of textile materials. Rom Biotechnol Lett 13: 4037–4048. |
[80] |
Zhou CE, Kan CW, Sun C, et al. (2019) A review of chitosan textile applications. AATCC J Res 6: 8–14. https://doi.org/10.14504/ajr.6.S1.2 doi: 10.14504/ajr.6.S1.2
![]() |
[81] |
Jimtaisong A, Saewan N (2014) Utilization of carboxymethyl chitosan in cosmetics. Int J Cosmet Sci 36: 12–21. https://doi.org/10.1111/ics.12102 doi: 10.1111/ics.12102
![]() |
[82] |
Aranaz I, Acosta N, Civera C, et al. (2018) Cosmetics and cosmeceutical applications of chitin, chitosan and their derivatives. Polymers 10: 213. https://doi.org/10.3390/polym10020213 doi: 10.3390/polym10020213
![]() |
[83] |
Ruocco N, Costantini S, Guariniello S, et al. (2016) Polysaccharides from the marine environment with pharmacological, cosmeceutical and nutraceutical potential. Molecules 21: 551. https://doi.org/10.3390/molecules21050551 doi: 10.3390/molecules21050551
![]() |
[84] |
Islam MM, Shahruzzaman M, Biswas S, et al. (2020) Chitosan based bioactive materials in tissue engineering applications—A review. Bioact Mater 5: 164–183. https://doi.org/10.1016/j.bioactmat.2020.01.012 doi: 10.1016/j.bioactmat.2020.01.012
![]() |
[85] |
Balagangadharan K, Dhivya S, Selvamurugan N (2017) Chitosan based nanofibers in bone tissue engineering. Int J Biol Macromol 104: 1372–1382. https://doi.org/10.1016/j.ijbiomac.2016.12.046 doi: 10.1016/j.ijbiomac.2016.12.046
![]() |
[86] |
Doench I, Tran TA, David L, et al. (2019) Cellulose nanofiber-reinforced chitosan hydrogel composites for intervertebral disc tissue repair. Biomimetics 4: 19. https://doi.org/10.3390/biomimetics4010019 doi: 10.3390/biomimetics4010019
![]() |
[87] |
Shamshina JL, Zavgorodnya O, Berton P, et al. (2018) Ionic liquid platform for spinning composite chitin–poly (lactic acid) fibers. ACS Sustain Chem Eng 6: 10241–10251. https://doi.org/10.1021/acssuschemeng.8b01554 doi: 10.1021/acssuschemeng.8b01554
![]() |
[88] |
Chakravarty J, Rabbi MF, Chalivendra V, et al. (2020) Mechanical and biological properties of chitin/polylactide (PLA)/hydroxyapatite (HAP) composites cast using ionic liquid solutions. Int J Biol Macromol 151: 1213–1223. https://doi.org/10.1016/j.ijbiomac.2019.10.168 doi: 10.1016/j.ijbiomac.2019.10.168
![]() |
[89] |
Xing F, Chi Z, Yang R, et al. (2021) Chitin-hydroxyapatite-collagen composite scaffolds for bone regeneration. Int J Biol Macromol 184: 170–180. https://doi.org/10.1016/j.ijbiomac.2021.05.019 doi: 10.1016/j.ijbiomac.2021.05.019
![]() |
[90] |
Almeida A, Silva D, Gonç alves V, et al. (2018) Synthesis and characterization of chitosan-grafted-polycaprolactone micelles for modulate intestinal paclitaxel delivery. Drug Deliv Transl Res 8: 387–397. https://doi.org/10.1007/s13346-017-0357-8 doi: 10.1007/s13346-017-0357-8
![]() |
[91] |
Andersen T, Mishchenko E, Flaten GE, et al. (2017) Chitosan-based nanomedicine to fight genital Candida Infections: Chitosomes. Mar Drugs 15: 64. https://doi.org/10.3390/md15030064 doi: 10.3390/md15030064
![]() |
[92] |
Parhi R (2020) Drug delivery applications of chitin and chitosan: a review. Environ Chem Lett 18: 577–594. https://doi.org/10.1007/s10311-020-00963-5 doi: 10.1007/s10311-020-00963-5
![]() |
[93] |
Kim Y, Park RD (2015) Progress in bioextraction processes of chitin from crustacean biowastes. J Korean Soc Appl Biol Chem 58: 545–554. https://doi.org/10.1007/s13765-015-0080-4 doi: 10.1007/s13765-015-0080-4
![]() |
[94] |
Hossin MA, Al Shaqsi NHK, Al Touby SSJ, et al. (2021) A review of polymeric chitin extraction, characterization, and applications. Arab J Geosci 14: 1870. https://doi.org/10.1007/s12517-021-08239-0 doi: 10.1007/s12517-021-08239-0
![]() |
[95] |
Yang X, Yin Q, Xu Y, et al. (2019) Molecular and physiological characterization of the chitin synthase B gene isolated from Culex pipiens pallens (Diptera: Culicidae). Parasit Vectors 12: 1–11. https://doi.org/10.1186/s13071-019-3867-z doi: 10.1186/s13071-019-3867-z
![]() |
[96] |
Hosney A, Ullah S, Barčauskaitė K (2022) A review of the chemical extraction of chitosan from shrimp wastes and prediction of factors affecting chitosan yield by using an artificial neural network. Mar Drugs 20: 675. https://doi.org/10.3390/md20110675 doi: 10.3390/md20110675
![]() |
[97] |
Pohling J, Dave D, Liu Y, et al. (2022) Two-step demineralization of shrimp (Pandalus Borealis) shells using citric acid: An environmentally friendly, safe and cost-effective alternative to the traditional approach. Green Chem 24: 1141–1151. https://doi.org/10.1039/D1GC03140F doi: 10.1039/D1GC03140F
![]() |
[98] |
Kumar AK, Sharma S (2017) Recent updates on different methods of pretreatment of lignocellulosic feedstocks: a review. Bioresour Bioprocess 4: 7. https://doi.org/10.1186/s40643-017-0137-9 doi: 10.1186/s40643-017-0137-9
![]() |
[99] |
Kou SG, Peters LM, Mucalo MR (2021) Chitosan: A review of sources and preparation methods. Int J Biol Macromol 169: 85–94. https://doi.org/10.1016/j.ijbiomac.2020.12.005 doi: 10.1016/j.ijbiomac.2020.12.005
![]() |
[100] |
Percot A, Viton C, Domard A (2003) Optimization of chitin extraction from shrimp shells. Biomacromolecules 4: 12–18. https://doi.org/10.1021/bm025602k doi: 10.1021/bm025602k
![]() |
[101] |
Hahn T, Tafi E, Paul A, et al. (2020) Current state of chitin purification and chitosan production from insects. J Chem Technol Biot 95: 2775–2795. https://doi.org/10.1002/jctb.6533 doi: 10.1002/jctb.6533
![]() |
[102] |
Greene BHC, Robertson KN, Young JCOC, et al. (2016) Lactic acid demineralization of green crab (Carcinus maenas) shells: effect of reaction conditions and isolation of an unusual calcium complex. Green Chem Lett Rev 9: 1–11. https://doi.org/10.1080/17518253.2015.1119891 doi: 10.1080/17518253.2015.1119891
![]() |
[103] |
Yang H, Gö zaydln G, Nasaruddin RR, et al. (2019) Toward the shell biorefinery: Processing crustacean shell waste using hot water and carbonic acid. ACS Sustain Chem Eng 7: 5532–5542. https://doi.org/10.1021/acssuschemeng.8b06853 doi: 10.1021/acssuschemeng.8b06853
![]() |
[104] |
Borić M, Vicente FA, Jurković DL, et al. (2020) Chitin isolation from crustacean waste using a hybrid demineralization/DBD plasma process. Carbohyd Polym 246: 116648. https://doi.org/10.1016/j.carbpol.2020.116648 doi: 10.1016/j.carbpol.2020.116648
![]() |
[105] |
Abbott AP, Capper G, Davies DL, et al. (2003) Novel solvent properties of choline chloride/urea mixtures. Chem Commun 2003: 70–71. https://doi.org/10.1039/b210714g doi: 10.1039/b210714g
![]() |
[106] |
Hikmawanti NPE, Ramadon D, Jantan I, et al. (2021) Natural deep eutectic solvents (Nades): Phytochemical extraction performance enhancer for pharmaceutical and nutraceutical product development. Plants 10: 2091. https://doi.org/10.3390/plants10102091 doi: 10.3390/plants10102091
![]() |
[107] |
Zhou C, Zhao J, Yagoub AEGA, et al. (2017) Conversion of glucose into 5-hydroxymethylfurfural in different solvents and catalysts: Reaction kinetics and mechanism. Egypt J Pet 26: 477–487. https://doi.org/10.1016/j.ejpe.2016.07.005 doi: 10.1016/j.ejpe.2016.07.005
![]() |
[108] |
Huang J, Guo X, Xu T, et al. (2019) Ionic deep eutectic solvents for the extraction and separation of natural products. J Chromatogr A 1598: 1–19. https://doi.org/10.1016/j.chroma.2019.03.046 doi: 10.1016/j.chroma.2019.03.046
![]() |
[109] |
Florindo C, Oliveira FS, Rebelo LPN, et al. (2014) Insights into the synthesis and properties of deep eutectic solvents based on cholinium chloride and carboxylic acids. ACS Sustain Chem Eng 2: 2416–2425. https://doi.org/10.1021/sc500439w doi: 10.1021/sc500439w
![]() |
[110] |
Saravana PS, Ho TC, Chae SJ, et al. (2018) Deep eutectic solvent-based extraction and fabrication of chitin films from crustacean waste. Carbohyd Polym 195: 622–630. https://doi.org/10.1016/j.carbpol.2018.05.018 doi: 10.1016/j.carbpol.2018.05.018
![]() |
[111] |
Zhao D, Huang WC, Guo N, et al. (2019) Two-step separation of chitin from shrimp shells using citric acid and deep eutectic solvents with the assistance of microwave. Polymers 11: 409. https://doi.org/10.3390/polym11030409 doi: 10.3390/polym11030409
![]() |
[112] |
Huang WC, Zhao D, Xue C, et al. (2022) An efficient method for chitin production from crab shells by a natural deep eutectic solvent. Mar Life Sci Technol 4: 384–388. https://doi.org/10.1007/s42995-022-00129-y doi: 10.1007/s42995-022-00129-y
![]() |
[113] |
Bradić B, Novak U, Likozar B (2020) Crustacean shell bio-refining to chitin by natural deep eutectic solvents. Green Process Synt 9: 13–25. https://doi.org/10.1515/gps-2020-0002 doi: 10.1515/gps-2020-0002
![]() |
[114] |
Younes I, Rinaudo M (2015) Chitin and chitosan preparation from marine sources. Structure, properties and applications. Mar Drugs 13: 1133–1174. https://doi.org/10.3390/md13031133 doi: 10.3390/md13031133
![]() |
[115] |
Rao MS, Stevens WF (2005) Chitin production by Lactobacillus fermentation of shrimp biowaste in a drum reactor and its chemical conversion to chitosan. J Chem Technol Biot 80: 1080–1087. https://doi.org/10.1002/jctb.1286 doi: 10.1002/jctb.1286
![]() |
[116] |
Neves AC, Zanette C, Grade ST, et al. (2017) Optimization of lactic fermentation for extraction of chitin from freshwater shrimp waste. Acta Sci Technol 39: 125–133. https://doi.org/10.4025/actascitechnol.v39i2.29370 doi: 10.4025/actascitechnol.v39i2.29370
![]() |
[117] | Castro R, Guerrero-Legarreta I, Bórquez R (2018) Chitin extraction from Allopetrolisthes punctatus crab using lactic fermentation. Biotechnology Rep 20: e00287. https://doi.org/10.1016/j.btre.2018.e00287 |
[118] |
Jung WJ, Jo GH, Kuk JH, et al. (2006) Extraction of chitin from red crab shell waste by cofermentation with Lactobacillus paracasei subsp. tolerans KCTC-3074 and serratia marcescens FS-3. Appl Microbiol Biot 71: 234–237. https://doi.org/10.1007/s00253-005-0126-3 doi: 10.1007/s00253-005-0126-3
![]() |
[119] |
Aytekin O, Elibol M (2010) Cocultivation of Lactococcus lactis and Teredinobacter turnirae for biological chitin extraction from prawn waste. Bioproc Biosyst Eng 33: 393–399. https://doi.org/10.1007/s00449-009-0337-6 doi: 10.1007/s00449-009-0337-6
![]() |
[120] |
Zhang H, Jin Y, Deng Y, et al. (2012) Production of chitin from shrimp shell powders using Serratia marcescens B742 and Lactobacillus plantarum ATCC 8014 successive two-step fermentation. Carbohyd Res 362: 13–20. https://doi.org/10.1016/j.carres.2012.09.011 doi: 10.1016/j.carres.2012.09.011
![]() |
[121] |
Xie J, Xie W, Yu J, et al. (2021) Extraction of chitin from shrimp shell by successive two-step fermentation of Exiguobacterium profundum and Lactobacillus acidophilus Front Microbiol 12: 677126. https://doi.org/10.3389/fmicb.2021.677126 doi: 10.3389/fmicb.2021.677126
![]() |
[122] |
Ghorbel-Bellaaj O, Hajji S, et al. (2013) Optimization of chitin extraction from shrimp waste with Bacillus pumilus A1 using response surface methodology. Int J Biol Macromol 61: 243–250. https://doi.org/10.1016/j.ijbiomac.2013.07.001 doi: 10.1016/j.ijbiomac.2013.07.001
![]() |
[123] |
Younes I, Hajji S, Frachet V, et al. (2014) Chitin extraction from shrimp shell using enzymatic treatment. Antitumor, antioxidant and antimicrobial activities of chitosan. Int J Biol Macromol 69: 489–498. https://doi.org/10.1016/j.ijbiomac.2014.06.013 doi: 10.1016/j.ijbiomac.2014.06.013
![]() |
[124] |
Hajji S, Ghorbel-Bellaaj O, Younes I, et al. (2015) Chitin extraction from crab shells by Bacillus bacteria. Biological activities of fermented crab supernatants. Int J Biol Macromol 79: 167–173. https://doi.org/10.1016/j.ijbiomac.2015.04.027 doi: 10.1016/j.ijbiomac.2015.04.027
![]() |
[125] |
Oh YS, Shih IL, Tzeng YM, et al. (2000) Protease produced by Pseudomonas aeruginosa K-187 and its application in the deproteinization of shrimp and crab shell wastes. Enzyme Microb Tech 27: 3–10. https://doi.org/10.1016/S0141-0229(99)00172-6 doi: 10.1016/S0141-0229(99)00172-6
![]() |
[126] |
Oh KT, Kim YJ, Nguyen VN, et al. (2007) Demineralization of crab shell waste by Pseudomonas aeruginosa F722. Process Biochem 42: 1069–1074. https://doi.org/10.1016/j.procbio.2007.04.007 doi: 10.1016/j.procbio.2007.04.007
![]() |
[127] |
Teng WL, Khor E, Tan TK, et al. (2001)Concurrent production of chitin from shrimp shells and fungi. Carbohyd Res 332: 305–316. https://doi.org/10.1016/S0008-6215(01)00084-2 doi: 10.1016/S0008-6215(01)00084-2
![]() |
[128] |
Sedaghat F, Yousefzadi M, Toiserkani H, et al. (2017) Bioconversion of shrimp waste Penaeus merguiensis using lactic acid fermentation: An alternative procedure for chemical extraction of chitin and chitosan. Int J Biol Macromol 104: 883–888. https://doi.org/10.1016/j.ijbiomac.2017.06.099 doi: 10.1016/j.ijbiomac.2017.06.099
![]() |
[129] |
Tan YN, Lee PP, Chen WN (2020) Microbial extraction of chitin from seafood waste using sugars derived from fruit waste-stream. AMB Express 10: 1–11. https://doi.org/10.1186/s13568-020-0954-7 doi: 10.1186/s13568-020-0954-7
![]() |
[130] |
He Y, Xu J, Wang S, et al. (2014) Optimization of medium components for production of chitin deacetylase by Bacillus amyloliquefaciens Z7, using response surface methodology. Biotechnol Biotechnol Equip 28: 242–247. https://doi.org/10.1080/13102818.2014.907659 doi: 10.1080/13102818.2014.907659
![]() |
[131] |
Gamal RF, El-Tayeb TS, Raffat EI, et al. (2016) Optimization of chitin yield from shrimp shell waste by bacillus subtilis and impact of gamma irradiation on production of low molecular weight chitosan. Int J Biol Macromol 91: 598–608. https://doi.org/10.1016/j.ijbiomac.2016.06.008 doi: 10.1016/j.ijbiomac.2016.06.008
![]() |
[132] |
Jabeur F, Mechri S, Kriaa M, et al. (2020) Statistical experimental design optimization of microbial proteases production under co-culture conditions for chitin recovery from speckled shrimp metapenaeus monoceros by-product. Biomed Res Int 2020: 1–10. https://doi.org/10.1155/2020/3707804 doi: 10.1155/2020/3707804
![]() |
[133] |
Ghorbel-Bellaaj O, Hmidet N, Jellouli K, et al. (2011) Shrimp waste fermentation with Pseudomonas aeruginosa A2: Optimization of chitin extraction conditions through Plackett–Burman and response surface methodology approaches. Int J Biol Macromol 48: 596–602. https://doi.org/10.1016/j.ijbiomac.2011.01.024 doi: 10.1016/j.ijbiomac.2011.01.024
![]() |
[134] |
Doan CT, Tran TN, Wen IH, et al. (2019) Conversion of shrimp headwaste for production of a thermotolerant, detergent-stable, alkaline protease by paenibacillus sp. Catalysts 9: 798. https://doi.org/10.3390/catal9100798 doi: 10.3390/catal9100798
![]() |
[135] |
Nasri R, Younes I, Lassoued I, et al. (2011) Digestive alkaline proteases from zosterisessor ophiocephalus, raja clavata, and scorpaena scrofa : Characteristics and application in chitin extraction. J Amino Acids 2011: 1–9. https://doi.org/10.4061/2011/913616 doi: 10.4061/2011/913616
![]() |
[136] |
Mathew GM, Huang CC, Sindhu R, et al. (2021) Enzymatic approaches in the bioprocessing of shellfish wastes. 3 Biotech 11: 1–13. https://doi.org/10.1007/s13205-021-02912-7 doi: 10.1007/s13205-021-02912-7
![]() |
[137] |
Venugopal V (2021) Valorization of seafood processing discards: Bioconversion and bio-refinery approaches. Front Sustain Food Syst 5: 611835. https://doi.org/10.3389/fsufs.2021.611835 doi: 10.3389/fsufs.2021.611835
![]() |
[138] |
Younes I, Hajji S, Rinaudo M, et al. (2016) Optimization of proteins and minerals removal from shrimp shells to produce highly acetylated chitin. Int J Biol Macromol 84: 246–253. https://doi.org/10.1016/j.ijbiomac.2015.08.034 doi: 10.1016/j.ijbiomac.2015.08.034
![]() |
[139] | Rifaat HM, El-Said OH, Hassanein SM, et al. (2007) Protease activity of some mesophilic streptomycetes isolated from Egyptian habitats. J Cult Collect 5: 16–24. |
[140] |
Hamdi M, Hammami A, Hajji S, et al. (2017) Chitin extraction from blue crab (Portunus segnis) and shrimp (Penaeus kerathurus) shells using digestive alkaline proteases from P. segnis viscera. Int J Biol Macromol 101: 455–463. https://doi.org/10.1016/j.ijbiomac.2017.02.103 doi: 10.1016/j.ijbiomac.2017.02.103
![]() |
[141] |
Razzaq A, Shamsi S, Ali A, et al. (2019) Microbial proteases applications. Front Bioeng Biotechnol 7: 110. https://doi.org/10.3389/fbioe.2019.00110 doi: 10.3389/fbioe.2019.00110
![]() |
[142] |
Contesini FJ, Melo RR de, Sato HH (2018) An overview of Bacillus proteases: from production to application. Crit Rev Biotechnol 38: 321–334. https://doi.org/10.1080/07388551.2017.1354354 doi: 10.1080/07388551.2017.1354354
![]() |
[143] |
Mhamdi S, Ktari N, Hajji S, et al. (2017) Alkaline proteases from a newly isolated Micromonospora chaiyaphumensis S103: Characterization and application as a detergent additive and for chitin extraction from shrimp shell waste. Int J Biol Macromol 94: 415–422. https://doi.org/10.1016/j.ijbiomac.2016.10.036 doi: 10.1016/j.ijbiomac.2016.10.036
![]() |
[144] |
Caruso G, Floris R, Serangeli C, et al. (2020) Fishery wastes as a yet undiscovered treasure from the sea: Biomolecules sources, extraction methods and valorization. Mar Drugs 18: 622. https://doi.org/10.3390/md18120622 doi: 10.3390/md18120622
![]() |
[145] |
Sila A, Mlaik N, Sayari N, et al. (2014) Chitin and chitosan extracted from shrimp waste using fish proteases aided process: Efficiency of chitosan in the treatment of unhairing effluents. J Polym Environ 22: 78–87. https://doi.org/10.1007/s10924-013-0598-7 doi: 10.1007/s10924-013-0598-7
![]() |
[146] |
Klomklao S, Benjakul S, Visessanguan W, et al. (2007) Trypsin from the pyloric caeca of bluefish (Pomatomus saltatrix). Comp Biochem Phys B 148: 382–389. https://doi.org/10.1016/j.cbpb.2007.07.004 doi: 10.1016/j.cbpb.2007.07.004
![]() |
[147] |
Sila A, Nasri R, Jridi M, et al. (2012) Characterisation of trypsin purified from the viscera of Tunisian barbel (barbus callensis) and its application for recovery of carotenoproteins from shrimp wastes. Food Chem 132: 1287–1295. https://doi.org/10.1016/j.foodchem.2011.11.105 doi: 10.1016/j.foodchem.2011.11.105
![]() |
[148] |
Batav C (2020) Valorization of catla visceral waste by obtaining industrially important enzyme: trypsin. Annu Rev Mater Res 6: 555680. https://doi.org/10.19080/ARR.2020.06.555680 doi: 10.19080/ARR.2020.06.555680
![]() |
[149] | Minh TLT, Truc TT, Osako K (2022) The effect of deproteinization methods on the properties of glucosamine hydrochloride from shells of white leg shrimp (litopenaeus vannamei) and black tiger shrimp (Penaeus monodon). Cienc Rural 52: e20200723. https://doi.org/10.1590/0103-8478cr20200723 |
[150] |
Duong NTH, Nghia ND (2014) Kinetics and optimization of the deproteinization by pepsin in chitin extraction from white shrimp shell. J Chitin Chitosan Sci 2: 21–28. https://doi.org/10.1166/jcc.2014.1054 doi: 10.1166/jcc.2014.1054
![]() |
[151] |
Hongkulsup C, Khutoryanskiy VV, Niranjan K (2016) Enzyme assisted extraction of chitin from shrimp shells (litopenaeus vannamei). J Chem Technol Biot 91: 1250–1256. https://doi.org/10.1002/jctb.4714 doi: 10.1002/jctb.4714
![]() |
[152] |
Manni L, Ghorbel-Bellaaj O, Jellouli K, et al. (2010) Extraction and characterization of chitin, chitosan, and protein hydrolysates prepared from shrimp waste by treatment with crude protease from bacillus cereus SV1. Appl Biochem Biotech 162: 345–357. https://doi.org/10.1007/s12010-009-8846-y doi: 10.1007/s12010-009-8846-y
![]() |
[153] |
Deng JJ, Mao HH, Fang W, et al. (2020) Enzymatic conversion and recovery of protein, chitin, and astaxanthin from shrimp shell waste. J Clean Prod 271: 122655. https://doi.org/10.1016/j.jclepro.2020.122655 doi: 10.1016/j.jclepro.2020.122655
![]() |
[154] |
Benmrad MO, Mechri S, Jaouadi NZ, et al. (2019) Purification and biochemical characterization of a novel thermostable protease from the oyster mushroom Pleurotus sajor-caju strain CTM10057 with industrial interest. BMC Biotechnol 19: 1–18. https://doi.org/10.1186/s12896-019-0536-4 doi: 10.1186/s12896-019-0536-4
![]() |
[155] |
Foophow T, Sittipol D, Rukying N, et al. (2022) Purification and characterization of a novel extracellular haloprotease Vpr from bacillus licheniformis strain KB111. Food Technol Biotech 60: 225–236. https://doi.org/10.17113/ftb.60.02.22.7301 doi: 10.17113/ftb.60.02.22.7301
![]() |
[156] |
Amiri H, Aghbashlo M, Sharma M, et al. (2022) Chitin and chitosan derived from crustacean waste valorization streams can support food systems and the UN Sustainable Development Goals. Nat Food 3: 822–828. https://doi.org/10.1038/s43016-022-00591-y doi: 10.1038/s43016-022-00591-y
![]() |
Parameter | Definition | Unit |
r1 | The net growth rate of tumor cells | day−1[45] |
r2 | The net growth rate of healthy cells | day−1[46] |
K1 | The maximum carrying capacity of lung tissue for tumor cells | cells[47] |
K2 | The maximum carrying capacity of lung tissue for healthy cells | cells[47] |
μ1 | The tumor cell mortality rate induced by the immune response | cell−1 day−1 |
μ2 | The mortality rate of healthy cells induced by the immune response | cell−1 day−1[48] |
μ3 | The rate of healthy cell death due to tumor cells | cell−1 day−1 |
λ1 | The tumor cell mortality rate caused by competition with healthy cells | cell−1 day−1 |
λ2 | The mortality rate of disseminated tumor cells caused by the immune response | cell−1 day−1 |
c1 | The speed at which tumor cells spread from the lungs to other tissues | cell−1 day−1 |
c2 | The rate at which tumor cell formation stimulates the production of immune cells | day−1[49] |
a1 | The half-maximal carrying capacity of the tumor cell environment | cells |
a2 | The maximum response threshold of immune cells | cells[50] |
d1 | The natural apoptotic rate of immune cells | day−1[46] |
d2 | The natural death rate of metastatic tumor cells | day−1 |
s | The generation rate of immune cells in the absence of tumors | cell day−1[49] |
t | LET | LEI | LEH | LEP | t | LET | LEI | LEH | LEP |
10 | -0.1551 | -0.0131 | -1.2056 | -1.3859 | 100 | 0.8621 | -0.1742 | -0.6623 | -1.0283 |
20 | 0.5700 | -0.5235 | -0.5740 | -0.6670 | 300 | 0.8337 | -0.3379 | -0.5994 | -1.2045 |
30 | 0.7545 | -0.3418 | -0.6011 | -0.6055 | 500 | 0.8833 | -0.2905 | -0.5432 | -1.1698 |
40 | 0.5516 | -0.6181 | -1.3066 | -1.3565 | 700 | 0.8744 | -0.3094 | -0.5554 | -1.1951 |
50 | 0.5659 | -0.4543 | -0.9360 | -1.1777 | 1000 | 0.8918 | -0.2897 | -0.5413 | -1.1829 |
Parameter | Value (α=0.9) | Status |
r1 | 3.001424e-01 | Fitted |
r2 | 4.886303e-01 | Fitted |
K1 | 2.686256e+05 | Fitted |
K2 | 2.283360e+05 | Fitted |
μ1 | 1.000000e-05 | Fitted |
μ2 | 1.000000e-05 | Fitted |
μ3 | 1.000000e-05 | Fitted |
λ1 | 8.912330e-05 | Fitted |
λ2 | 8.875427e-05 | Fitted |
c1 | 9.836971e-04 | Fitted |
c2 | 9.874923e-04 | Fitted |
a1 | 2.942564e+04 | Fitted |
a2 | 2.959367e+04 | Fitted |
d1 | 1.144475e-02 | Fitted |
d2 | 1.004176e-02 | Fitted |
s | 4.801490e-01 | Fitted |
Parameter | Definition | Unit |
r1 | The net growth rate of tumor cells | day−1[45] |
r2 | The net growth rate of healthy cells | day−1[46] |
K1 | The maximum carrying capacity of lung tissue for tumor cells | cells[47] |
K2 | The maximum carrying capacity of lung tissue for healthy cells | cells[47] |
μ1 | The tumor cell mortality rate induced by the immune response | cell−1 day−1 |
μ2 | The mortality rate of healthy cells induced by the immune response | cell−1 day−1[48] |
μ3 | The rate of healthy cell death due to tumor cells | cell−1 day−1 |
λ1 | The tumor cell mortality rate caused by competition with healthy cells | cell−1 day−1 |
λ2 | The mortality rate of disseminated tumor cells caused by the immune response | cell−1 day−1 |
c1 | The speed at which tumor cells spread from the lungs to other tissues | cell−1 day−1 |
c2 | The rate at which tumor cell formation stimulates the production of immune cells | day−1[49] |
a1 | The half-maximal carrying capacity of the tumor cell environment | cells |
a2 | The maximum response threshold of immune cells | cells[50] |
d1 | The natural apoptotic rate of immune cells | day−1[46] |
d2 | The natural death rate of metastatic tumor cells | day−1 |
s | The generation rate of immune cells in the absence of tumors | cell day−1[49] |
t | LET | LEI | LEH | LEP | t | LET | LEI | LEH | LEP |
10 | -0.1551 | -0.0131 | -1.2056 | -1.3859 | 100 | 0.8621 | -0.1742 | -0.6623 | -1.0283 |
20 | 0.5700 | -0.5235 | -0.5740 | -0.6670 | 300 | 0.8337 | -0.3379 | -0.5994 | -1.2045 |
30 | 0.7545 | -0.3418 | -0.6011 | -0.6055 | 500 | 0.8833 | -0.2905 | -0.5432 | -1.1698 |
40 | 0.5516 | -0.6181 | -1.3066 | -1.3565 | 700 | 0.8744 | -0.3094 | -0.5554 | -1.1951 |
50 | 0.5659 | -0.4543 | -0.9360 | -1.1777 | 1000 | 0.8918 | -0.2897 | -0.5413 | -1.1829 |
Parameter | Value (α=0.9) | Status |
r1 | 3.001424e-01 | Fitted |
r2 | 4.886303e-01 | Fitted |
K1 | 2.686256e+05 | Fitted |
K2 | 2.283360e+05 | Fitted |
μ1 | 1.000000e-05 | Fitted |
μ2 | 1.000000e-05 | Fitted |
μ3 | 1.000000e-05 | Fitted |
λ1 | 8.912330e-05 | Fitted |
λ2 | 8.875427e-05 | Fitted |
c1 | 9.836971e-04 | Fitted |
c2 | 9.874923e-04 | Fitted |
a1 | 2.942564e+04 | Fitted |
a2 | 2.959367e+04 | Fitted |
d1 | 1.144475e-02 | Fitted |
d2 | 1.004176e-02 | Fitted |
s | 4.801490e-01 | Fitted |