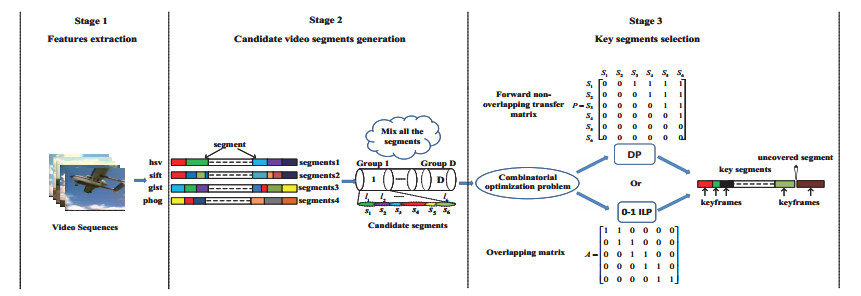
In this study, we employed a developed Fractional Cointegrating Vector Autoregressive (FCVAR) model to analyze the relationship between three different securities, i.e., housing prices, S&P500 stock prices and gold, and inflation rate, to determine the hedging properties of each type of asset against inflation shocks. Our analyses covered seven decades; ranging from January 1953 to January 2023. Our results suggested that housing prices and S&P500 performed partially against inflation and gold did not have hedging properties when attending to the full sample. Accounting for structural breaks, we discovered that these results changed. We found that housing prices and the S&P500 showed a superior hedging performance against inflation since the second regime. On the other hand, when we studied the behavior of gold, this security showed the inverse results, i.e., it showed no hedging performance in the second regime. Finally, these results were important for an optimal investment strategy, risk diversification, and monetarism.
Citation: Alejandro Almeida, Julia Feria, Antonio Golpe, José Carlos Vides. 2024: Financial assets against inflation: Capturing the hedging properties of gold, housing prices, and equities, National Accounting Review, 6(3): 314-332. doi: 10.3934/NAR.2024014
[1] | Zhenzhong Xu, Xu Chen, Linchao Yang, Jiangtao Xu, Shenghan Zhou . Multi-modal adaptive feature extraction for early-stage weak fault diagnosis in bearings. Electronic Research Archive, 2024, 32(6): 4074-4095. doi: 10.3934/era.2024183 |
[2] | Peng Lu, Yuchen He, Wenhui Li, Yuze Chen, Ru Kong, Teng Wang . An Informer-based multi-scale model that fuses memory factors and wavelet denoising for tidal prediction. Electronic Research Archive, 2025, 33(2): 697-724. doi: 10.3934/era.2025032 |
[3] | Jiange Liu, Yu Chen, Xin Dai, Li Cao, Qingwu Li . MFCEN: A lightweight multi-scale feature cooperative enhancement network for single-image super-resolution. Electronic Research Archive, 2024, 32(10): 5783-5803. doi: 10.3934/era.2024267 |
[4] | Wangwei Zhang, Menghao Dai, Bin Zhou, Changhai Wang . MCADFusion: a novel multi-scale convolutional attention decomposition method for enhanced infrared and visible light image fusion. Electronic Research Archive, 2024, 32(8): 5067-5089. doi: 10.3934/era.2024233 |
[5] | Huimin Qu, Haiyan Xie, Qianying Wang . Multi-convolutional neural network brain image denoising study based on feature distillation learning and dense residual attention. Electronic Research Archive, 2025, 33(3): 1231-1266. doi: 10.3934/era.2025055 |
[6] | Hui Xu, Jun Kong, Mengyao Liang, Hui Sun, Miao Qi . Video behavior recognition based on actional-structural graph convolution and temporal extension module. Electronic Research Archive, 2022, 30(11): 4157-4177. doi: 10.3934/era.2022210 |
[7] | Ruyu Yan, Jiafei Jin, Kun Han . Reinforcement learning for deep portfolio optimization. Electronic Research Archive, 2024, 32(9): 5176-5200. doi: 10.3934/era.2024239 |
[8] | Xu Chen, Wenbing Chang, Yongxiang Li, Zhao He, Xiang Ma, Shenghan Zhou . Resnet-1DCNN-REA bearing fault diagnosis method based on multi-source and multi-modal information fusion. Electronic Research Archive, 2024, 32(11): 6276-6300. doi: 10.3934/era.2024292 |
[9] | Wen Li, Jian Wang, Zhanpeng Du, Hongfeng Ma, Lijie Zhang, Libin Duan . Lightweight design method and application of MEWP bracket based on multi-level optimization. Electronic Research Archive, 2022, 30(12): 4416-4435. doi: 10.3934/era.2022224 |
[10] | Bo Wei, Xiao Jin, Li Deng, Yanrong Huang, Hongrun Wu . Feature selection via a multi-swarm salp swarm algorithm. Electronic Research Archive, 2024, 32(5): 3588-3617. doi: 10.3934/era.2024165 |
In this study, we employed a developed Fractional Cointegrating Vector Autoregressive (FCVAR) model to analyze the relationship between three different securities, i.e., housing prices, S&P500 stock prices and gold, and inflation rate, to determine the hedging properties of each type of asset against inflation shocks. Our analyses covered seven decades; ranging from January 1953 to January 2023. Our results suggested that housing prices and S&P500 performed partially against inflation and gold did not have hedging properties when attending to the full sample. Accounting for structural breaks, we discovered that these results changed. We found that housing prices and the S&P500 showed a superior hedging performance against inflation since the second regime. On the other hand, when we studied the behavior of gold, this security showed the inverse results, i.e., it showed no hedging performance in the second regime. Finally, these results were important for an optimal investment strategy, risk diversification, and monetarism.
Keyframe extraction algorithms select the most representative frames with a minimal level of redundancy from the videos [1]. Keyframe extraction plays an important role in several broad areas of video processing research such as real-time camera tracking [2], human shape and pose tracking [3], video annotation [4], video summarization [5,6,7,8,9,10], creating chapter titles in DVDs, video editing, 3D model reconstruction [11], human action recognition [12], and video retrieval and browsing [13]. The key challenges in keyframe extraction are twofold: i) covering the representative content of the video and ii) reducing redundancies between neighboring keyframes. Recent deep learning-based methods mainly extract relevant semantic features [14,15,16], while ignoring the whole image content, which is a key factor for keyframe extraction.
Keyframe extraction has been tackled from various perspectives [17,18,19]. Below, we review the most representative works in three common approaches: i) shot detection or video segmentation, ii) clustering methods, and iii) the optimization problem, and differentiate our work from these previous works.
i) Shot detection or video segmentation: A shot is defined as an unbroken sequence of frames recorded from a single camera, which forms the building block of a video. During the shot-based keyframe extraction, the shot boundaries of the original video are first detected, and then one or more keyframes are extracted from each shot. The purpose of shot boundaries detection is to segment the video stream into multiple shots[20,21]. For example, a pixel-wise difference based metric with a threshold is used to detect the shot boundaries of the video presented in [20]. [21] uses k-means clustering on a two dimensional feature extracted from both histogram and spatial difference metrics, followed by a heuristic elimination process to detect the shot boundaries. After the shots are segmented, keyframes can be extracted from each shot based on it's contents[1,22]. A segment is defined as homogenous sequence of frames in the visual content domain. During the segment-based keyframe extraction approaches, a video is segmented into higher-level video components, where each component could be either a scene, an event, a set of shots, or even the entire video sequence. Then, each segment can be described by one or more keyframe(s)[23]. Han et al.[8] found key segments by agglomerative clustering followed by a variant of the 0-1 knapsack problem. Omidyeganeh et al.[24] employed generalized Gaussian density parameters of wavelet transform subbands along with the Kullback-Leibler distance measurement to address the keyframe extraction problem from each video segment. The performance of the keyframe extraction based on either the shot detection or the video segmentation approach relies on the accuracy of either shot detection or video segmentation.
ii) Clustering methods: The clustering methods [25,26,27,28] take all the frames of a shot together and classify them according to their content similarity. Then, the keyframes are determined as the representative frames of a cluster. A similarity-driven cluster merging method with a threshold parameter which controls the density of classification has been achieved in keyframe extraction [25]. In the approach presented in [26], the authors applied multiple partitional clustering to the whole video sequence in order to remove the visual-content redundancy among video frames. The keyframes were selected as centroids of the optimal clusters obtained by an unsupervised procedure based on a cluster-validity analysis. In [27], a hierarchical clustering was introduced when clustering the video, and the temporal constraints was used to filter out unsuitable clusters. A representative frame was selected from each cluster. Authors in [28] proposed a spectral clustering method for extracting keyframes based on a sparse representation. The disadvantage of clustering methods is that the temporal information of a video sequence is omitted. An inherent problem in this approach is the selection of appropriate thresholds. Although adaptive clustering methods can manipulate the threshold to produce a pre-designed number of keyframes, this iterative searching process makes these computational methods expensive. Compared to both the video segmentation and the shot detection approach, the above approach requires a proper visual content analysis and the selection of appropriate features. On the other hand, our method exploits the combinations of multiple clustering features without regard to the video contents, and can thus be easily applied to any complex video content. Many times, clustering can supply different contents of video segments. The longest non-overlapping segments can cover the contents of the video as much as possible. In addition, a combinatorial optimization problem without thresholds or a pre-designed number of keyframes was solved by either a quick dynamic programming [29] or a 0–1 integer linear programming approach [30].
iii) Optimization problem: The one main optimization problem is the set cover problem. Set cover problem: Given a universe U={e1,e2,...,en} of n elements, a collection of subsets of U, S={S1,S2,...,Sk}, and a cost function c:S⟶Q+, and find a minimum cost subcollection of S that covers all elements of U.
The minimum set cover problem can be formulated as the following integer linear program:
minc=wTys.t.yT1{ei∈S}≥1,∀i∈{1,2,...,n},y∈{0,1}k. | (1.1) |
where w is the weight vector and y is a binary vector. yi=1 denotes that Si is selected and 0 is otherwise. 1{ei∈S} is a indicator vector of k dimensions. If ei is in Sj, then the jth element in 1{ei∈S} is 1 and 0 otherwise.
It is an NP-complete problem [31]. The set cover problem has been widely applied in saliency detection [32] and keyframe extraction [1,33]. In [32], the social group candidates selection was formulated as the set cover problem, which was represented as the form of a quadratic integer programming, and the optimization was solved with a branch and bound method. In [1], the global keypoint pool is formed by matching keypoints, and the keypoints of the keyframes should cover the global keypoint pool as much as possible. It was formulated as a variation of the set cover problem, and a greedy algorithm was adopted to approximately tackle this issue. The set cover problem was also employed in [33], in which the suboptimal solution for the minimum covering problem was found using parts of the Quine-McCluskey algorithm. The disadvantage of this approach is obvious, since it can not obtain the optimal solution, but rather the suboptimal solution. The most closest to our approach is the combinatorial optimization problem. Combinatorial optimization is a topic that consists of finding an optimal object from a finite set of objects [34]. Chang et al. [33] constructed a tree-structured keyframe hierarchy in which a video segment for a given degree of fidelity was represented with a compact set of keyframes, and used a depth-first search scheme with pruning to enable an efficient content-based retrieval. The energy minimization or maximization based methods [35] extract keyframes by solving a rate-constrained problem, [36] extracting a small number of keyframes within a shot by maximizing the divergence between video objects in a feature space. This approach comes with two advantages: it's very intuitive and it can reach an optimal solution. The main contributions can be summarized as follows:
∙ We propose a keyframe extraction method based on multiple features via a novel combinatorial optimization algorithm.
∙ The proposed method achieves a promising performance on the Animal-world and Keyframe-Sydney (KFSYD) datasets. Extensive qualitative and quantitative experiments demonstrate the proposed method's effectiveness.
The rest of the paper is organized as follows. Section 2 introduces the proposed method, including features extraction, candidate video segments generation and key segments selection. In Section 3, the optimization methods are presented, including the dynamic programming approach and a 0–1 integer linear programming approach. In Section 4, some related analyses and discussions are presented. Experimental results are shown in Section 5 to verify the proposed approach. Finally, concluding remarks are given in Section 6.
Figure 1 shows the main framework of the proposed keyframe extraction algorithm. Our algorithm includes three stages, (i.e., features extraction, candidate video segments generation, and key segments selection). The details will be introduced as follows.
There is a strong relationship among neighbouring frames. Meanwhile, there may exist a blurring in the video caused by either the quick movement of objects in the shot or the jitter of the camera lens. This will interfere with the shot detection or the segmentation of video sequence. Illumination variation is another challenging point. Errors in key frames selection can arise by solely using the color or texture features to cluster the video. Intuitively, the more features there are in the video, the more detail will be presented. In our problem, we use the following features to cluster the video sequence: f1=HSVhistogram, f2=SIFT [37], f3=GIST [38], and f4=PHOG [39]. These features are usually used in keyframe extraction. These low-level visual features describe the image from various aspects such as the color, shape and context, which are in favour of accurately and comprehensively representing the video's contents.
The candidate video segments generation includes the following three steps, in the given order.
● Step 1: For each feature, the k-means clustering method is used to obtain the initial video segments by clustering the video sequence.
● Step 2: The initial video segments are further pruned to remove some invalid segments.
● Step 3: The ultimate candidate video segments are produced by mixing the video segments generated by all the features in Step 2.
For example, as shown in Figure 2, the video sequence is clustered into four clusters with the GIST feature according to Step 1. We can obtain five initial video segments, where the first video segment and the last video segment belong to the same cluster c1. In Step 2, the second video segment, which belongs to cluster c2, is pruned, since the frame length is less than 10. Such a segment is unlikely to compose a complete shot. The left video segments are labeled as s1∼s4. In Step 3, we start to mix the segments set {svn}V,Nvv=1,n=1, where V refers to the number of features, and Nv denotes the number of segments generated by v-th feature in Step 2. There may exist such a case that more than two segments in {svn}V,Nvv=1,n=1 have the same beginning and ending times. Then, only one of these reduplicated segments will ultimately remained in the segments set by random.
The remaining video segments are called candidate segments. These candidate segments are sorted by the beginning time, followed by being divided into D groups that don't overlap in time. The whole process of generating candidate video segments is similar to the Stage 2 of Figure 1.
The candidate segments in the dth group are denoted as a set Sd={sj},j∈{1…n}, where n is the number of candidate segments in the group. The segments selected from the candidate segments are called key segments.
Combinatorial optimization problem: The target of our problem is to find the optimal subset S∗d that covers all the video sequences as possible. S∗d has the property that there is no overlap in time for any segment that are selected from the dth group. S∗d is the set of keysegments that we seek. Then, the problem then becomes how to find the longest non-overlapping segments in each group. For the dth group, it can be formulated as follows:
maxk,qik∑i=1lqis.t.lsqi⋂sqj=0,∀i≠j∈{1,...,k},qi,qj∈{1,...,n},sqi∈Sd,∀i∈{1,...,k},qi∈{1,...,n}. | (2.1) |
where n is the number of selected candidate segments in the dth group and qi is the index of qthi segment in this group. The ith element li in column vector L=(l1,…,ln) denotes the number of frames of ith candidate segment. The k denotes the number of keysegments selected from the current group. The first constraint denotes that the selected segment sqi and the selected segment sqj don't overlap.
A forward non-overlapping transfer matrix is defined to represent a directed graph G=(V,E) with a temporal non-overlapping relationship, as shown in Figure 3, where the segments correspond to the nodes V={v1,⋯,v6}, and a directed edge eij is connected between node i and j with non-overlapping segments, where the corresponding segment si is in front of segment sj. The forward non-overlapping transfer matrix can be formulated in the n×n upper triangular matrix, where n is the number of nodes in the graph:
P=[0000Pi,j⋱⋱0000] |
where Pij=1,i≠j denotes that the ith and jth segment has no overlap, and 0 if jth segment is in front of the ith segment or there is no overlap between them. For any i∈{1,2,...n−1}, Pi,i+1=0, (i.e., the elements in the diagonal right above the main diagonal are all zeros). It is because the ith segment and the i+1th segment have overlapped with each other; otherwise, these two segments belong to different groups. Additionally, the element Pij in matrix P means that there exists a path of length 1 from the node i to the node j if Pij=1 and no path if Pij=0, according to [40]. In the same way, the element Pkij in the power matrix Pk means that there exist Pkij path(s) of length k from the node i to the node j. The transfer matrix serves as the building block throughout our following algorithm.
We define a selection matrix Ak, where k is the number of key segments. The elements of Ak are the total lengths of the key segments. We set P0=I, where I is an identity matrix. An AND operator for non negative integer vectors is defined as c=a∘b, where c=logical(a)&logical(b), logical(∙) denotes that the vector is an element-wise binarization operation and & is a logical AND operator.
As mentioned previously, the candidate segments in each group can be represented as a graph. The difficulties in solving Eq (1.1) include the following: the variable is binary and the number of key segments is not given in advance and has to been optimized. To deal these problems, we propose the following dynamic programming based on the graph. For dth subgraph, our dynamic programming algorithm is described as follows:
● Step 1: Construct a transfer matrix P and initialize a selection matrix Ak=l⋅1T,k=1, where l is a column vector of length.
● Step 2: Determine whether Pk is all zeros matrix. If not, determine which elements are activated in Ak(i,:) in the ith row based on the activated indicator vector a=Pk−1(i,:)∘P(:,j), where Pk−1(i,:),P(:,j) denote the elements in the ith row of Pk−1 and in the jth column of P, respectively. Then, the maximum value Ak(i,r) in Ak(i,:) and the corresponding rth column segment lr are added to Ak+1(i,j). If so, go to Step 4.
● Step 3: k=k+1, and go to Step 2.
● Step 4: Return the maximum value in {A1,⋯,Ak} and its corresponding key segments.
Figure 4 displays a toy example and is a subgraph in graph G according to our dynamic programming. The forward non-overlapping transfer matrix and initial selection matrix are represented as follows:
P=s1s2s3s4s5s6s1s2s3s4s5s6(001111000111000011000001000000000000) |
![]() |
As mentioned earlier, the forward non-overlapping transfer matrix represents whether or not there exists a path of step length 1 from one node to subsequent node. In other words, P(i,j)=1 denotes that si and sj do not overlap in time. The activated indicator vector a=P0(1,:)∘P(:,3), a=[100000] denotes that only one path A1(1,1) is activated. The segment s3 is added to path A2(1,3)=A1(1,1)+l3. In the same way, we can calculate A2(i,j), where i,j are the index of the non zeros elements in P. Since A2 and P have the same data structure, the other elements in A2 are all zeros. The elements with a box around them are activated as shown in A1 and A2:
![]() |
where A2 is a upper triangular matrix, and A2i,j≠0 denotes that two candidate segments are selected.
P2=s1s2s3s4s5s6s1s2s3s4s5s6(000012000001000000000000000000000000) |
As for P21,6=2, there exist two paths of step length 2, while only the longest one is selected and stored in A31,6. The activation indicator vector a=P(1,:)∘P(:,6), a = [0 0 1 1 0 0] denotes that A21,3,A21,4 are both activated as in the first row of A2 with a box around them. The largest value between the activation paths A21,3,A21,4 is transferred to A31,6. As A21,3=l1+l3,A21,3=30,A21,4=l1+l4,A21,4=45, A21,4>A21,3; then, the segment s6 is added to path A21,4, A31,6=A21,4+l6. If a=[000000], the corresponding A3i,j=0. The whole A3 is shown as follows:
A3=[0000l1+l3+l5l1+l4+l600000l2+l4+l6000000000000000000000000] |
When P3=0, then A4=0. There is no path of step length 3. Then, the algorithm stops. The maximum value in {A1,A2,A3} is A32,6=l2+l4+l6, and the corresponding key segments are s2,s4,s6 in the subgraph.
Complexity analysis: Our algorithm is parallelizable and it's very easy to predict the iteration step k subjects to Pk=0 for each subgraph. In addition, the transfer matrix P is zero on the first diagonal above the main diagonal and below it for a subgraph of any, which will facilitate the acceleration of calculating Pn,{n=2,3,…,k}. It is very easy to verify that Pn has all zeros on the (2n−1)th diagonal above and below the main diagonal. Since An+1 and Pn have the same data structure, An+1 can be calculated quickly. The computation complexity is C(m36−7m224−m12) if m is even, or C(m32−m28−m2+18) if m is odd, where m is the maximum number of nodes in all subgraphs. Once the longest key segments were found, the frames which are closest to the corresponding feature centroid of key segments are selected as keyframes.
The pairwise overlapping relationship of segments are represented as an overlapping matrix A∈{0,1}m×n, where m is the number of pairwise overlapping segments, and n is the number of segments. If the ith segment and the jth(j>i) problem overlap in time, then Ai,i=1,Ai,j=1. In addition, A⋅1=2, i.e., only one pairwise overlapping segments are presented in each row of matrix A, where 1 is a column vector of all ones, and 2 are a column vector of all twos. For example, take the toy game in Figure 2. The overlapping matrix can be represented as follows:
A=[110000011000001100000110000011] |
We define a selection indicator vector y∈{0,1}n, i.e., yi=1 denotes that the ith segment is selected, and 0 otherwise. Equation (1.1) can be represented as a form of 0-1 integer linear programming.
maxLTys.t.Ay≤1,y∈{0,1}6 | (3.1) |
The inequality constraint means that, at most, one segment can be selected from the pairwise overlapping segments. The embedded matlab function bintprog is used to optimize the aforementioned problem. Once the optimal key segments are found, the frames which are closest to the corresponding feature centroids of key segments are selected as the keyframes.
The main differences between our problem and the set cover problem, as well as the shortest path problem, are described as follows.
There are four main differences between our combinational problem and the set cover problem: 1) the subsets in S have no order in the set cover problem, but they are sorted in time order in our problem; 2) All the elements in the universe should be covered in set cover problem, but they should be covered as much as possible in our problem; 3) The subsets should not overlap with each other in our problem, but it has no such constraint in the set cover problem; and 4) The set cover problem is a minimal problem, but ours is a maximal problem.
Shortest path problem: In graph theory, the shortest path problem is the problem of finding a path between two vertices (or nodes) in a graph such that the sum of the weights of its constituent edges is minimized. The main difference between our problem and the shortest path problem is that the start node and the end node are not given in our problem, which should be inferred by our proposed combinatorial optimization.
The 0–1 inter linear program (ILP) approach is very time consuming when the dimensions of the constraint matrix are very large, but it is very easy to implement. It takes about 10 minutes to select key segments from one groups candidate segments on the Animal-world dataset with the 0–1 ILP approach, while the DP approach take less than 20 s to extract the same length of key segments. To obtain the longest key segments, the combination of key segments is not unique. The dynamic program (DP) approach searches optimal key segments from a smaller combination of candidate segments to a larger combination of candidate segments. In this process, it selects the minimal combination of candidate segments but with the longest sequences coverage. However, the 0–1 ILP approach selects more key segments than the dynamic approach with the same length of sequence coverage. The average gap between neighboring keyframes extracted by the DP approach is larger than the 0–1 ILP approach. The larger average gap between neighboring keyframes can reduce the redundancies between neighboring keyframes. The longer key segments can cover the representative content of the video as much as possible. In the experimental part, the dynamic approach is utilized to extract the key segments.
When the number of keyframes is specified as k, all of the video segments will serve as a whole. In our DP approach or the 0–1 ILP approach, the forward non-overlapping transfer matrix will include the whole video segments. For the DP approach, the element Pi,i+1=1, if ith and i+1th segments are in different groups. The selection matrix Ak can be obtained after running the dynamic programming algorithm. The combinatorial segments, which correspond to the maximum value in Ak, are the desired key segments. For the 0–1 ILP approach, a similar overlapping matrix is constructed. The new constraint yT1=k means that the k segments are selected as key segments.
To evaluate the performance of the proposed keyframes extraction algorithm, two types of experiments were performed. The first one compared the performance of video segmentation by giving the groundtruth of keyframes on the Animal-world dataset. The second one was a quantitative experiment evaluated on the KFSYD dataset [1].
Databases: 1) The Animal-world dataset is a long video sequence of 8000 frames constructed by ourselves. The resolution of the frames is 352×288. It contains six different types of objects including leopard, rhinoceros, hyena, zebra, elephant, and human. We chose 36 shots, which included 4213 frames of the Animal-world dataset to evaluate our experiment. The same object class in the same frames were given different labels according to the order of their emergence. The groundtruth were annotated frame by frame, which took almost two months to complete this task. There existed frequent shot changes, visual transitions (such as dissolves), occlusions and complex scenes. 2) The KFSYD dataset [1] was constructed from the open video project (http://www.open-video.org) for quantitative evaluation. It consists of 10 video shots across several genres. The resolution of frames is 352×240. The ground-truth keyframes of the videos were manually selected by three students with video processing backgrounds. The main differences between these two datasets are listed in Table 1.
# of Videos | # of frames | # of shots per video | given ground truth keyframes | evaluation | |
Animal-world | 1 | 4213 | 36 | no | The quality of video segmentation |
KFSYD | 10 | 95 ∼ 352 | 1 | yes | The quality of keyframes extraction |
Experimental settings: For feature f1, 16 histogram bins were used to represent the features in each color channel. For feature f2, SIFT features were computed on a uniform grid and a k-means cluster method was used to obtain 300 centroids for the bag-of-words [41] representation. The whole video sequence with different features were all clustered into C clusters by k-means clustering. In our experiment, the HSV (Hue, Saturation, Value) histogram had 32 bins, and the SIFT (scale-invariant feature transform) descriptor [37], which was quantized into visual words had 300 dimensions, the GIST [38] had 512 dimensions, and the PHOG (pyramid histogram of oriented gradients) [39] had 628 dimensions. All the experiments were conducted on an Intel(R) Core(TM) i7-8700K CPU @ 3.70GHz and implemented with the MATLAB programming software.
By giving the groundtruth of keyframes extracted by [42,43], we used the publicly available code [42]* to segment the whole video. The algorithm can detect the shot change and determine the pixel-level label of each frame by either transferring the groundtruth of the nearest left keyframe or the nearest right keyframe based on propagation of errors.
*http://vision.cs.utexas.edu/projects/active_frame_selection/
In our approach, the k-means parameter C was experimentally set to 50 for each feature. The ultimate 52 key segments produced by our dynamic programming approach covered 4197 frames. The mean keyframe interval between neighbourhood keyframes was 84.1. For keyframe extraction approach in [42], a cost matrix was calculated with accumulative errors from the pixel flow propagation. A dynamic programming algorithm was used to extract the keyframes based on the cost matrix. The number of keyframes was set to 52. The Markov Random Field (MRF) model was utilized to achieve the video segmentation. For the keyframe extraction approach in [43], the keyframe extraction was formulated as a conditional k keyframe selection problem. A dynamic programming algorithm was proposed to search for the optimal k keyframes. The number of keyframes was also set to 52. By giving the groundtruth of keyframes extracted by three different algorithms, a similar MRF-based approach was used to segment the video.
The comparison between an algorithm's output and the groundtruth was performed on four evaluation metrics (i.e., average precision (AP), average recall (AR), average F-score (AF) and average intersection-over-union (AIoU)).
AP=1NN∑i=11nini∑k=1mi∑ˆk=1Prekˆkiσ(yki=gˆki),Prekˆki=|Rki∩Gˆki||Rki| | (5.1) |
AR=1NN∑i=11mini∑k=1mi∑ˆk=1Reckˆkiσ(yji=gˆki),Reckˆki=|Rki∩Gˆki||Gˆki| | (5.2) |
AF=1NN∑i=11Nini∑k=1mi∑ˆk=11.3Prekˆki×Reckˆki0.3Prekˆki+Reckˆkiσ(yki=gˆki),Ni=ni+mi−ni∑k=1mi∑ˆk=1σ(yki=gˆki) | (5.3) |
AIoU=1NN∑i=11Nini∑k=1mi∑ˆk=1|Rki∩Gˆki||Rki∪Gˆki|σ(yki=gˆki) | (5.4) |
where Rki is the kth region of semantic segmentation in the ith frame and yki is the label of it, Gˆki is the ˆkth groundtruth semantic region of the ith frame and gˆki is the groundtruth label of it, ni is the number of segmentation objects including background in the ith frame, mi is the number of groundtruth objects including background in the ith frame and σ is a indicator function.
The results of the video segmentation are shown in Figure 5. As illustrated, our algorithm includes the 0–1 integer linear programming approach (ILP) and the dynamic programming approach (DP-1) yields a much better result. The higher value of AF and AIoU, the more representative of the keyframes. The illumination variation, blurring, and occlusion in the Animal-world dataset have a big impact in extracting keyframes based on the label propagation errors approach and the appearance transfer [42]. Though the algorithm can detect the shot change, it may fail in some cases mentioned above.
The video segmentation performance can be further improved by merging the uncovered frames, as shown in Figure 1, into key segments and transferring the appearance model based on flows between neighboring frames within key segments in our dynamic programming approach, as shown in ILP-2 and DP-2. The video segmentation is restricted within each segments. According to the segmentation method proposed in [42] Section 3.1, the unary terms include two terms (i.e., a unary potential based on an appearance model and a unary potential based on transferred label). The binary term is based on both the appearance and motion similarity of neighbouring pixels within each frame. Then, an MRF method is used to infer the labels of each pixels. As shown in Figure 5, the promotion of the segmentation performance is very obvious.
Execution times: The computational cost of our approach is largely affected by the multiple feature extraction and clustering. It takes about 20 minutes to complete the feature extraction and clustering. However, the dynamic programming optimization process takes less than 30 seconds, while the 0–1 integer linear programming approach takes more than 20 minutes in key segments selection. [42] takes about 40 hours in computing its cost matrix. The keyframe selection process takes about five minutes. [43] has a execution times of 90 minutes.
A keyframe is considered a true keyframe if it is no more than 15 frames apart from a ground-truth keyframe in the KFSYD dataset. A groundtruth keyframe will be matched with, at most, one keyframe. The matching procedure can be achieved by the hungarian algorithm[44].
To evaluate the quality of keyframes, we used three evaluations metrics (i.e., the average precision (AP) metric, the average recall (AR), and the average F-score (AF) metric), which are defined as follows:
AP=13010∑i=13∑j=1MijBi | (5.5) |
AR=13010∑i=13∑j=1MijNij | (5.6) |
AF=11510∑i=13∑j=1MijBi+Nij | (5.7) |
where Mij denotes the number of keyframes extracted from the ith video, which are matched with the groundtruth keyframes selected by the jth student from the ith video, Bi is the number of keyframes extracted from the ith video by the proposed approach, and Nij is the number of the groundtruth keyframes selected by the jth student from the ith video.
In our approach, the number of feature clustering are all set to C=5. As illustrated in Figure 6, our two approaches achieve an improved performance with regards to the state of the art clustering approach [25] and the method in [19]. A clustering-based method is unlikely to select either the first or the last frames as a keyframe, but our approach still gives as good of a result as in ILP-1 and DP-1. As shown in Figure 6, the performance of the proposed method is lower than existing the KBKS and KBKS-fast methods. The main reason is that the proposed clustering-based method is unlikely to select either the first or the last frames as keyframe. If the beginning frame of the first key segment and the ending frame of the last key segment are selected as the keyframes, just like the method in [42], our approach can achieve a further improvement of the experimental performance in the ILP-2 or DP-2. These results show that the keyframes extracted with our approach are efficient. The visualization result of the DP-1 are shown in Figure 7. Our method can cover the primary contents of the video. As shown in Figure 7(a), our method can capture not only the posture change of an airplane, but also the shape change of the clouds. The keyframes extracted by our method have few redundancies. As shown in Figure 7(i), there are few similarities of visual content among them.
Execution times: For a video shot with 300 frames, KBKS [1]-1 needs roughly 150 seconds and KBKS [1]-2 needs about 15 seconds. The clustering method [25] needs about 13 seconds. The iso-content distance [19]-1 needs about 15 seconds and the iso-content distance [19]-2 needs about 16 seconds. The running time of our dynamic programming approach is roughly 250 seconds, where the feature extraction consumes a great deal of time. The running time of our 0–1 integer linear programming approach is roughly 300 seconds.
In order to explore the influence of the k-means parameter C in the number of keyframes, the experiments are conducted on the Animal-world dataset by varying C at five intervals from 20 to 200. As shown in Figure 7, the parameter C does influence the number of keyframes. As C increases, the video will be separated into more segments, and the number of candidate segments also increases. The final number of keyframes becomes larger. When C is more than 50, the number of keyframes is far less than the parameter C as C increases. It shows that the relation between them is not very strong.
The main advantages of our proposed method are listed as follows: 1) From the result on the Animal-world dataset, our proposed method is adaptive to long video content, including complex video; 2) Since our proposed method is based on multiple features clustering, it is also easy to integrate other image descriptors; 3) The number of keyframes can be determined by semiautomatic, which has some influence from the parameter C, or specified by the users; and 4) Our proposed method is easy to implement and can obtain an optimal solution. However, every coin has two sides. Our proposed method is no exception. The main disadvantages or limitations are given as follows: (ⅰ) The procedures for feature extraction and clustering take too much time. It is not ideal for a real-time application. Through current popular hash technology [45], the large scale image clustering problem can be addressed very fast; (ⅱ) The k-means parameter C has some influence on the number of keyframes. It requires human intervention to determine parameter C; and (ⅲ) The motion information between frames or local descriptors within frames has not been taken into consideration. It is very valuable to mine these information and will require further investigation regarding integrating them into the keyframe extraction.
In this paper, we present a key frame extraction approach based on a combinatorial optimization problem. A novel dynamic programming approach and a 0–1 integer linear programming approach is developed to solve the combinatorial optimization problem. The experimental results on the Animal-world dataset and the KFSYD dataset demonstrated that the performance of the proposed approach is very promising. More experiments on other large and diverse datasets will be explored in future.
The authors declare they have not used Artificial Intelligence (AI) tools in the creation of this article.
This work is supported by the National Natural Science Foundation of China under Grants No.62201406, No.62171329 and the Startup Foundation of Wuhan Institute of Technology under Grant No.20QD16.
The authors declare there is no conflicts of interest.
[1] |
Adekoya OB, Oliyide JA, Oduyemi GO (2021) How COVID-19 upturns the hedging potentials of gold against oil and stock markets risks: Nonlinear evidences through threshold regression and markov-regime switching models. Resour Policy 70: 101926. https://doi.org/10.1016/j.resourpol.2020.101926 doi: 10.1016/j.resourpol.2020.101926
![]() |
[2] |
Ahmed S, Cardinale M (2005) Does inflation matter for equity returns?. J Asset Manag 6: 259–273. https://doi.org/10.1057/palgrave.jam.2240180 doi: 10.1057/palgrave.jam.2240180
![]() |
[3] | Alderman L (2022) Eurozone Inflation Hits its Highest Level since the Creation of the Euro in 1999. The New York Times. https://www.nytimes.com/2022/05/31/business/eurozone-inflation.html. |
[4] |
Anari A, Kolari J (2001) Stock prices and inflation. J Financ Res 24: 587–602. https://doi.org/10.1111/j.1475-6803.2001.tb00832.x doi: 10.1111/j.1475-6803.2001.tb00832.x
![]() |
[5] |
Anari A, Kolari J (2002) House prices and inflation. Real Estate Econ 30: 67–84. https://doi.org/10.1111/1540-6229.00030 doi: 10.1111/1540-6229.00030
![]() |
[6] |
Antonakakis N, Gupta R, Tiwari AK (2017) Has the correlation of inflation and stock prices changed in the United States over the last two centuries?. Res Int Bus Financ 42: 1–8. https://doi.org/10.1016/j.ribaf.2017.04.005 doi: 10.1016/j.ribaf.2017.04.005
![]() |
[7] | Arif I, Khan L, Iraqi KM (2019) Relationship between oil price and white precious metals return: a new evidence from quantile-on-quantile regression. Pak J Commer Soc Sci 13: 515–528. |
[8] |
Arnold S, Auer BR (2015) What do scientists know about inflation hedging?. N Am J Econ Financ 34: 187–214. https://doi.org/10.1016/j.najef.2015.08.005 doi: 10.1016/j.najef.2015.08.005
![]() |
[9] |
Aye GC, Chang T, Gupta R (2016) Is gold an inflation-hedge? Evidence from an interrupted Markov-switching cointegration model. Resour Policy 48 77–84. https://doi.org/10.1016/j.resourpol.2016.02.011 doi: 10.1016/j.resourpol.2016.02.011
![]() |
[10] |
Aye GC, Carcel H, Gil-Alana LA, et al. (2017) Does gold act as a hedge against inflation in the UK? Evidence from a fractional cointegration approach over 1257 to 2016. Resour Policy 54, 53–57. https://doi.org/10.1016/j.resourpol.2017.09.001 doi: 10.1016/j.resourpol.2017.09.001
![]() |
[11] |
Baillie RT, Booth GG, Tse Y, et al. (2002) Price Discovery and Common Factor Models. J Financ Mark 5: 309–321. https://doi.org/10.1016/s1386-4181(02)00027-7 doi: 10.1016/s1386-4181(02)00027-7
![]() |
[12] |
Bai J, Perron P (2003) Computation and analysis of multiple structural change models. J Appl Econom 18 1–22. https://doi.org/10.1002/jae.659 doi: 10.1002/jae.659
![]() |
[13] |
Bampinas G, Panagiotidis T (2015) Are gold and silver a hedge against inflation? A two century perspective. Int Rev Financ Anal 41 267–276. https://doi.org/10.1016/j.irfa.2015.02.007 doi: 10.1016/j.irfa.2015.02.007
![]() |
[14] |
Beckmann J, Czudaj R (2013) Gold as an inflation hedge in a time-varying coefficient framework. The North American J Econ Financ 24 208–222. https://doi.org/10.1016/j.najef.2012.10.007 doi: 10.1016/j.najef.2012.10.007
![]() |
[15] | Bureau of Labor Statistics (2022) Consumer Prices up 8.6 Percent over Year Ended May 2022: the Economics Daily. U.S. Bureau of Labor Statistics. Available from: https://www.bls.gov/opub/ted/2022/consumer-prices-up-8-6-percent-over-year-ended-may-2022.htm. https://doi.org/10.1787/d1e962ba-en. |
[16] | Comin DA, Johnson RC, Jones CJ (2023) Supply chain constraints and inflation (No. w31179). National Bureau of Economic Research. https://doi.org/10.3386/w31179 |
[17] |
Christou C, Gupta R, Nyakabawo W, et al. (2018) Do house prices hedge inflation in the US? A quantile cointegration approach. Int Rev Econ Financ 54 15–26. https://doi.org/10.1016/j.iref.2017.12.012 doi: 10.1016/j.iref.2017.12.012
![]() |
[18] |
Chua J, Woodward RS (1982) Gold as an inflation hedge: a comparative study of six major industrial countries. J Bus Finan Account 9 191–197. https://doi.org/10.1111/j.1468-5957.1982.tb00985.x doi: 10.1111/j.1468-5957.1982.tb00985.x
![]() |
[19] |
Dolatabadi S, Nielsen MØ , Xu K (2015) A Fractionally Cointegrated VAR Analysis of Price Discovery in Commodity Futures Markets. J Futures Mark 35: 339–356. https://doi.org/10.1002/fut.21693 doi: 10.1002/fut.21693
![]() |
[20] |
Dolatabadi S, Nielsen MØ , Xu K (2016) A Fractionally Cointegrated VAR Model with Deterministic Trends and Application to Commodity Futures Markets. J Empir Financ 38: 623–639. https://doi.org/10.1016/j.jempfin.2015.11.005 doi: 10.1016/j.jempfin.2015.11.005
![]() |
[21] |
Dolatabadi S, Narayan PK, Nielsen MØ , et al. (2018) Economic Significance of Commodity Return Forecasts from the Fractionally Cointegrated VAR Model. J Futures Mark 38: 219–242. https://doi.org/10.1002/fut.21866 doi: 10.1002/fut.21866
![]() |
[22] |
Erb CB, Harvey CR (2013) The golden dilemma. Financ Anal J 69: 10–42. https://doi.org/10.3386/w18706 doi: 10.3386/w18706
![]() |
[23] |
Engsted T, Tanggaard C (2002) The relation between asset returns and inflation at short and long horizons. J Int Financ Mark I 12: 101–118. https://doi.org/10.1016/s1042-4431(01)00052-x doi: 10.1016/s1042-4431(01)00052-x
![]() |
[24] |
Fama EF, Schwert GW (1977) Asset returns and inflation. J Financ Econ 5: 115–146. https://doi.org/10.1016/s0014-2921(98)00090-7 doi: 10.1016/s0014-2921(98)00090-7
![]() |
[25] | Fisher I (1930) The theory of interest, New York: MacMillan Company. |
[26] |
Geweke J, Porter‐Hudak S (1983) The estimation and application of long memory time series models. J Time Ser Anal 4: 221–238. https://doi.org/10.1111/j.1467-9892.1983.tb00371.x doi: 10.1111/j.1467-9892.1983.tb00371.x
![]() |
[27] |
Hillier D, Draper P, Faff R (2006) Do precious metals shine? An investment perspective. Financ Anal J 62: 98–106. https://doi.org/10.2469/faj.v62.n2.4085 doi: 10.2469/faj.v62.n2.4085
![]() |
[28] |
Van Hoang TH, Lahiani A, Heller D (2016) Is gold a hedge against inflation? New evidence from a nonlinear ARDL approach. Econ Model 54: 54–66. https://doi.org/10.1016/j.econmod.2015.12.013 doi: 10.1016/j.econmod.2015.12.013
![]() |
[29] |
Hong G, Khil J, Lee BS (2013) Stock returns, housing returns and inflation: is there an inflation illusion?. Asia‐Pac J Financ Stud 42: 511–562. https://doi.org/10.1111/ajfs.12023 doi: 10.1111/ajfs.12023
![]() |
[30] |
Iqbal J (2017) Does gold hedge stock market, inflation and exchange rate risks? An econometric investigation. Int Rev Econ Financ 48: 1–17. https://doi.org/10.1016/j.iref.2016.11.005 doi: 10.1016/j.iref.2016.11.005
![]() |
[31] |
Jain A, Ghosh S (2013) Dynamics of global oil prices, exchange rate and precious metal prices in India. Resour Policy 38: 88–93. https://doi.org/10.1016/j.resourpol.2012.10.001 doi: 10.1016/j.resourpol.2012.10.001
![]() |
[32] | Johansen S (1995) Likelihood-based inference on cointegration in the vector autoregressive model, Oxford, UK: Oxford University Press. https://doi.org/10.1093/0198774508.001.0001 |
[33] |
Johansen S (2008a) A Representation Theory For A Class Of Vector Autoregressive Models For Fractional Processes. Economet Theory 24: 651–676. https://doi.org/10.1017/s0266466608080274 doi: 10.1017/s0266466608080274
![]() |
[34] |
Johansen S (2008b) Representation of Cointegrated Autoregressive Processes with Application to Fractional Processes. Economet Reviews 28: 121–145. https://doi.org/10.1080/07474930802387977 doi: 10.1080/07474930802387977
![]() |
[35] |
Johansen S, Nielsen MØ (2010) Likelihood Inference for a Non-stationary Fractional Autoregressive Model. J Econom 158: 51–66. https://doi.org/10.2139/ssrn.1150164 doi: 10.2139/ssrn.1150164
![]() |
[36] |
Johansen S, Nielsen MØ (2012) Likelihood Inference for a Fractionally Cointegrated Vector Autoregressive Model. Econometrica 80: 2667–2732. https://doi.org/10.2139/ssrn.1618653 doi: 10.2139/ssrn.1618653
![]() |
[37] |
Kasa K (1992) Common stochastic trends in international stock markets. J Monetary Econ 29: 95–124. https://doi.org/10.1016/0304-3932(92)90025-w doi: 10.1016/0304-3932(92)90025-w
![]() |
[38] | Lothian JR, McCarthy CH (2001) Equity returns and inflation: The puzzlingly long lags. |
[39] |
Oloko TF, Ogbonna AE, Adedeji AA, et al. (2021) Fractional cointegration between gold price and inflation rate: Implication for inflation rate persistence. Resour Policy 74: 102369. https://doi.org/10.1016/j.resourpol.2021.102369 doi: 10.1016/j.resourpol.2021.102369
![]() |
[40] |
Qian L, Jiang Y, Long H (2023) Extreme risk spillovers between China and major international stock markets. Modern Financ 1: 30–34. https://doi.org/10.61351/mf.v1i1.6 doi: 10.61351/mf.v1i1.6
![]() |
[41] |
Reboredo JC (2013) Is gold a hedge or safe haven against oil price movements?. Resour Policy 38 130–137. https://doi.org/10.1016/j.resourpol.2013.02.003 doi: 10.1016/j.resourpol.2013.02.003
![]() |
[42] |
Robinson PM (1995) Log-periodogram regression of time series with long-range dependence. Ann Stat, 1048–1072. https://doi.org/10.1214/aos/1176324636 doi: 10.1214/aos/1176324636
![]() |
[43] | Rödel MG (2012) Inflation Hedging: An Empirical Analysis on Inflation Nonlinearities, Infrastructure, and International Equities (Doctoral dissertation). |
[44] |
Rödel M (2014) Inflation hedging with international equities. J Portf Manage 40: 41–53. https://doi.org/10.3905/jpm.2014.40.2.041 doi: 10.3905/jpm.2014.40.2.041
![]() |
[45] |
Rushdi M, Kim JH, Silvapulle P (2012) ARDL bounds tests and robust inference for the long run relationship between real stock returns and inflation in Australia. Econ Model 29: 535–543. https://doi.org/10.1016/j.econmod.2011.12.017 doi: 10.1016/j.econmod.2011.12.017
![]() |
[46] |
Salisu AA, Raheem ID, Ndako UB (2020) The inflation hedging properties of gold, stocks and real estate: A comparative analysis. Resour Policy 66: 101605. https://doi.org/10.1016/j.resourpol.2020.101605 doi: 10.1016/j.resourpol.2020.101605
![]() |
[47] | Schnabel I (2023) New challenges for the Economic and Monetary Union in the post-crisis environment. Speech by Isabel Schnabel, Member of the Executive Board of the ECB, at the Euro50 Group conference. Luxembourg, 19 June 2023. |
[48] |
Shahzad SJH, Mensi W, Hammoudeh S, et al. (2019) Does gold act as a hedge against different nuances of inflation? Evidence from Quantile-on-Quantile and causality-in-quantiles approaches. Resour Policy 62: 602–615. https://doi.org/10.1016/j.resourpol.2018.11.008 doi: 10.1016/j.resourpol.2018.11.008
![]() |
[49] |
Shimotsu K, Phillips PC (2002) Pooled log periodogram regression. J Time Ser Anal 23: 57–93. https://doi.org/10.1111/1467-9892.00575 doi: 10.1111/1467-9892.00575
![]() |
[50] |
Spierdijk L, Umar Z (2015) Stocks, bonds, T-bills and inflation hedging: From great moderation to great recession. J Econ Bus 79: 1–37. https://doi.org/10.1016/j.jeconbus.2014.12.002 doi: 10.1016/j.jeconbus.2014.12.002
![]() |
[51] |
Taderera M, Akinsomi O (2020) Is commercial real estate a good hedge against inflation? Evidence from South Africa. Res Int Bus Financ 51: 101096. https://doi.org/10.2139/ssrn.3373626 doi: 10.2139/ssrn.3373626
![]() |
[52] |
Tiwari AK, Cunado J, Gupta R, et al. (2018) Are stock returns an inflation hedge for the UK? Evidence from a wavelet analysis using over three centuries of data. Stud Nonlinear Dyn Econom 23: 20170049. https://doi.org/10.1515/snde-2017-0049 doi: 10.1515/snde-2017-0049
![]() |
[53] |
Tkacz G (2001) Estimating the Fractional Order of Integration of Interest Rates Using a Wavelet OLS Estimator. Stud Nonlinear Dyn Econom 5: 19–32. https://doi.org/10.2202/1558-3708.1068 doi: 10.2202/1558-3708.1068
![]() |
[54] |
Valadkhani A, Nguyen J, Chiah M (2022) When is gold an effective hedge against inflation?. Resour Policy 79: 103009. https://doi.org/10.1016/j.resourpol.2022.103009 doi: 10.1016/j.resourpol.2022.103009
![]() |
[55] |
Valcarcel VJ (2012) The dynamic adjustments of stock prices to inflation disturbances. J Econ Bus 64: 117–144. https://doi.org/10.1016/j.jeconbus.2011.11.002 doi: 10.1016/j.jeconbus.2011.11.002
![]() |
[56] |
Velasco C (1999) Non-stationary log-periodogram regression. J Econom 91: 325–371. https://doi.org/10.1016/s0304-4076(98)00080-3 doi: 10.1016/s0304-4076(98)00080-3
![]() |
[57] | World Bank (2020) Global economic prospects, June 2020. The World Bank. https://doi.org/10.1596/978-1-4648-1553-9 |
[58] |
Yaya O, Adenikinju O, Olayinka HA (2024) African stock markets' connectedness: Quantile VAR approach. Modern Financ 2: 51–68. https://doi.org/10.61351/mf.v2i1.70 doi: 10.61351/mf.v2i1.70
![]() |
[59] |
Yeap GP, Lean HH (2017) Asymmetric inflation hedge properties of housing in Malaysia: New evidence from nonlinear ARDL approach. Habitat Int 62: 11–21. https://doi.org/10.1016/j.habitatint.2017.02.006 doi: 10.1016/j.habitatint.2017.02.006
![]() |
[60] |
Zaremba A, Umar Z, Mikutowski M (2019) Inflation hedging with commodities: A wavelet analysis of seven centuries worth of data. Econ Lett 181: 90–94. https://doi.org/10.1016/j.econlet.2019.05.002 doi: 10.1016/j.econlet.2019.05.002
![]() |
[61] |
Zaremba A, Szczygielski JJ, Umar Z, et al. (2021) Inflation hedging in the long run: Practical perspectives from seven centuries of commodity prices. J Altern Invest 24: 119–134. https://doi.org/10.3905/jai.2021.1.136 doi: 10.3905/jai.2021.1.136
![]() |
1. | Manjusha Rajan, Latha Parameswaran, Key frame extraction algorithm for surveillance videos using an evolutionary approach, 2025, 15, 2045-2322, 10.1038/s41598-024-84324-0 |
# of Videos | # of frames | # of shots per video | given ground truth keyframes | evaluation | |
Animal-world | 1 | 4213 | 36 | no | The quality of video segmentation |
KFSYD | 10 | 95 ∼ 352 | 1 | yes | The quality of keyframes extraction |