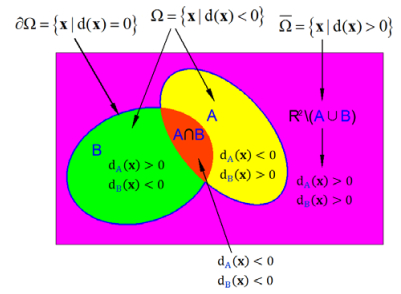
Improving energy efficiency is critical to breaking the resource curse. Using the GML Productivity Index, we measured the China's green total factor energy efficiency (GTFEE) and systematically explored the effects of environmental regulations on GTFEE. This article focuses on the threshold effect of environmental regulation (ER) on GTFEE at different skill premium levels. The conclusion shows that the impact of ER on GTFEE is expressed as a U-shaped relationship. ER can not only directly increase the skill premium, but also indirectly improve the GTFEE by increasing the skill premium. In addition, the threshold effect analysis suggests that skills premiums can enhance the role of ER in promoting GTFEE. Based on a new perspective on labor skills premiums, this study analyzes the mechanisms of environmental regulation to promote GTFEE, which has enlightening significance for improving the pollution control effect of ER and promoting carbon neutrality in China.
Citation: Siyu Ren, Haitao Wu. Path to green development: the role environmental regulation and labor skill premium on green total factor energy efficiency[J]. Green Finance, 2022, 4(4): 387-410. doi: 10.3934/GF.2022019
[1] | Xiaoming Peng, Yadong Shang . Attractors for a quasilinear viscoelastic equation with nonlinear damping and memory. AIMS Mathematics, 2021, 6(1): 543-563. doi: 10.3934/math.2021033 |
[2] | Jiangwei Zhang, Yongqin Xie . Asymptotic behavior for a class of viscoelastic equations with memory lacking instantaneous damping. AIMS Mathematics, 2021, 6(9): 9491-9509. doi: 10.3934/math.2021552 |
[3] | Zayd Hajjej, Sun-Hye Park . Asymptotic stability of a quasi-linear viscoelastic Kirchhoff plate equation with logarithmic source and time delay. AIMS Mathematics, 2023, 8(10): 24087-24115. doi: 10.3934/math.20231228 |
[4] | Xiaobin Yao . Random attractors for non-autonomous stochastic plate equations with multiplicative noise and nonlinear damping. AIMS Mathematics, 2020, 5(3): 2577-2607. doi: 10.3934/math.2020169 |
[5] | Adel M. Al-Mahdi, Mohammad M. Al-Gharabli, Mohamed Alahyane . Theoretical and numerical stability results for a viscoelastic swelling porous-elastic system with past history. AIMS Mathematics, 2021, 6(11): 11921-11949. doi: 10.3934/math.2021692 |
[6] | Narcisse Batangouna . A robust family of exponential attractors for a time semi-discretization of the Ginzburg-Landau equation. AIMS Mathematics, 2022, 7(1): 1399-1415. doi: 10.3934/math.2022082 |
[7] | Zayd Hajjej, Menglan Liao . Exponential stability of a system of coupled wave equations by second order terms with a past history. AIMS Mathematics, 2023, 8(12): 28450-28464. doi: 10.3934/math.20231456 |
[8] | Xiao Bin Yao, Chan Yue . Asymptotic behavior of plate equations with memory driven by colored noise on unbounded domains. AIMS Mathematics, 2022, 7(10): 18497-18531. doi: 10.3934/math.20221017 |
[9] | Peipei Wang, Yanting Wang, Fei Wang . Indirect stability of a 2D wave-plate coupling system with memory viscoelastic damping. AIMS Mathematics, 2024, 9(7): 19718-19736. doi: 10.3934/math.2024962 |
[10] | Yang Wang, Jihui Wu . Long-time dynamics of nonlinear MGT-Fourier system. AIMS Mathematics, 2024, 9(4): 9152-9163. doi: 10.3934/math.2024445 |
Improving energy efficiency is critical to breaking the resource curse. Using the GML Productivity Index, we measured the China's green total factor energy efficiency (GTFEE) and systematically explored the effects of environmental regulations on GTFEE. This article focuses on the threshold effect of environmental regulation (ER) on GTFEE at different skill premium levels. The conclusion shows that the impact of ER on GTFEE is expressed as a U-shaped relationship. ER can not only directly increase the skill premium, but also indirectly improve the GTFEE by increasing the skill premium. In addition, the threshold effect analysis suggests that skills premiums can enhance the role of ER in promoting GTFEE. Based on a new perspective on labor skills premiums, this study analyzes the mechanisms of environmental regulation to promote GTFEE, which has enlightening significance for improving the pollution control effect of ER and promoting carbon neutrality in China.
Topology optimization is an advanced method for structural optimization which has achieved vast progress over the last four decades. Topology optimization is mostly utilized to confirm the material(s) distribution within a prescribed spatial domain during the initial design stage, and it is realized by applying one or several certain conditions (volume constraints, perimeter constraints, mass constraints, etc.) to enhance structural performance. Topology optimization methods are of great significance in studies of the force transmission path and the performance of materials. By topology optimization, an optimal structure with better performance or less material consumption (while the structural performance is retained) can be obtained. So far, various distinctive approaches have become increasingly popular and have, therefore, been widely studied and applied for topology optimization [1,2,3], such as the homogenization theory [1], the density-based scheme (e.g., solid isotropic microstructure with penalty (SIMP)) [2], the evolutionary structural optimization (ESO) concept [4], the level-set approach [5,6,7,8,9], the parametric level-set method [10,11,12,13,14,15], the isogeometric method [16,17], the bionic approach [18], the meshless method [19,20], and so on.
Since Bendsøe and Kikuchi realized the structural topology optimization of porous materials successfully by applying the homogenization theory [1], structural topology optimization has garnered much attention for application to computational mechanics, material mechanics, and engineering structures. The research field of topology optimization can be mainly categorized as either continuum topology optimization or discrete structure topology optimization, both of which are closely related to finite element analysis (FEA). However, FEA is closely related to the mesh generation of structural design domains, so the generator of finite element meshes has significant influence on the efficiency of structure optimization. Most computationally oriented papers on topology optimization that rely on FEA apply unified low-order linear triangular meshes [21] or quadrilateral meshes [22] to discretize a design domain. However, numerical instability phenomena like the checkerboard patterns and the single-node connections often appear in the results of the variable density method [22,23]. Usually, a regularization scheme such as filtering [23] can be used to suppress such numerical instability phenomena, but regularization measures often involve the related parameters in the heuristic algorithm (for example, the SIMP method [23]) that can increase the complexity of topology optimization. A polygonal discretization method has been proposed by Talischi et al. [24] to achieve stable topology optimization formulations [25] by using lower-order polygonal elements. Jie et al. [26] extended the above method, introduced a simulated annealing algorithm into the process of mesh generation for finite element methods on the basis of the Centroidal Voronoi tessellations (CVTs), and solved the problems related to it being difficult to achieve convergence via the MacQueen's method and the Lloyd's algorithm. Recently, Bruggi [27] has proposed a topology optimization method that uses mixed finite elements on regular grids. The efficient mixed finite elements furnish accuracy and robustness on regular grids, and the topology optimization problem is solved only in terms of stresses. From the above references, the methods using the Voronoi elements have the ability to discretize the design domain with complex boundaries and solve the topology optimization problems of non-convex domains. Moreover, the methods have good numerical stability. Therefore, a topology optimization approach for multi-material structures is proposed on the basis of Voronoi elements in this paper.
The research on topology optimization currently focuses on solving the problems of stress constraints, nonlinear problems, and dynamic problems. However, it is not difficult to address the problems of single-material topology optimization [5,28]. With the development of topology optimization methods and engineering application requirements, research on topology optimization problems of multi-material structures is gradually becoming the mainstream. There are many works that focus on the solution of multi-material topology optimization [29,30,31,32,33,34,35,36]. In accordance with the concept of material distribution, Bendsøe and Sigmund put forward a SIMP method with a multi-material mixing principle [37], which has been successfully put to use for the compliance agencies of multiphysics [38]. Another method for optimizing the topology of multiple materials has been derived from the variable density method [39]. During the process of designing intelligent and sensitive structures, the multi-material topology optimization models have been used to find the optimal distribution of elastic and piezoelectric materials [40,41,42]. Hvejsel and Lund [43] proposed a couple of multi-material interpolation methods which summarized the well-known material interpolation schemes based on SIMP and the rational approximation of material properties. For topology optimization involving multiple materials, Tavakoli and Mohseni [44] put forward an alternating active-phase algorithm and a 115-line MATLAB implementation, through which a multi-material topology optimization problem is transformed into a range of two-material topology optimization sub-problems. Zhou and Wang [45] brought forward a universal method to tackle the multi-phase topology optimization problems on the basis of the Cahn-Hilliard equation. According to the penalization of objective functionals with the Ginzburg-Landau energy functional, Tavakoli [46] introduced a new method for multi-material topology optimization. The level-set method has been widely employed to multi-material structural topology optimization since the development of the SIMP method. For example, a "color" level-set approach was proposed by Wang and Wang [47] to solve the topology optimization problem involving compliance minimization with multiple materials. Moreover, Wang et al. [48] have put forward a level-set approach for multi-material topology optimization, in which M materials are expressed by M level sets with accurate mathematical expressions. By using the method, the redundant regions, as well as the overlaps between different materials, can be avoided. However, the traditional level-set method can only move or merge the existing holes, but not generate a new hole. Hence, it is generally decided by the initial design. The level-set method with moving morphable components (MMC) was proposed by Guo et al. [49] to realize topology optimization explicitly and geometrically. By utilizing this method, geometric and mechanical information can be directly combined and integrated with the topology optimization process. The method has greater flexibility in terms of handling topology optimization problems and reducing computational burden. Then, Zhang et al. [50] and Guo et al. [51] expanded the explicit structural topology optimization. Both of their methods are based on MMC and a level-set approach, which have shown great potential for use in multi-material topology optimization. Besides the SIMP method and the level-set approach, another procedure to address the problem of multi-material topology optimization is the evolutionary topology optimization involving multiple materials. For example, Huang and Xie [52] proposed a bi-directional ESO method which can be put to use to handle the binary-phase or multi-material topology optimization problem. In this article, the discretization method using Voronoi elements based on SIMP is considered. Therefore, a multi-material topology optimization method based on the alternating active-phase algorithm [44] is adopted as the framework to address the topology optimization problem involving multiple materials. The design variables are improved by using a filtering matrix and mapped to element variables so that the proposed method can be successfully implemented.
In existing methods for topology optimization involving multiple materials, the unified quadrilateral elements are usually utilized to discretize the design domains. However, it is difficult to optimize the structures with complex boundaries by using the unified quadrilateral elements, which confines the application range of the methods. To overcome the limitation, we propose a discretization method that uses the centroidal Voronoi elements to discretize the design domain of structures involving multiple materials. The centroidal Voronoi elements are based on the signed distance functions and the corresponding reflecting functions [53,54]. For an arbitrary mesh, the FEA routine can be implemented by using the unstructured and isoparametric polygonal elements. Therefore, the discretization method can deal with the design domains with complex boundaries, obtain good robustness, and avoid the checkerboard patterns [55,56].
The rest of this article is organized as follows. The polygonal meshes which can be used to discretize the design domain are introduced in Section 2. The model of topology optimization, combined with the alternating active-phase algorithm, is depicted in Section 3. The modified model of topology optimization is expressed in Section 4. The sensitivity analysis and the update scheme for design variables are described in Section 5. In Section 6, several numerical examples are provided to demonstrate the performance and effectiveness of this method. The conclusions are finally drawn in Section 7.
Inspired by References [24,25,57,58], we introduce the approximate hexagonal CVT elements to discretize the structural design domain with complex curve boundaries. In this section, we present the signed distance function, the corresponding mapping functions, the formation process for Voronoi elements, as well as the discretization method of the design domain. Finally, we discretize the design domain through the use of CVT elements.
Here, the signed distance function [24] is incorporated and used to implicitly express the design domain. Assuming that Ω is a subset of the two-dimensional real number space R2, then the distance function d(x) can be described as follows:
d(x)={−miny∈∂Ω||x−xb||,ifx∈Ω0,ifx∈∂Ωminy∈∂Ω||x−xb||,ifx∈R2∖Ω∖∂Ω | (1) |
where x represents the point set of Ω, ∂Ω signifies the boundary of Ω, and xb represents the point set of ∂Ω. ||∙|| signifies the standard Euclidean norm. Hence, the distance between x and xb can be denoted by ||x−xb|| here.
In this mesh algorithm, it is necessary to judge whether the point set x lies within the design domain, which is similar to the situation in which a level-set function is used to represent the design domain. Then, by means of Eq (1), the design domain can be represented as follows.
{Ω={x|d(x)<0}¯Ω={x|d(x)>0}∂Ω={x|d(x)=0} | (2) |
Figure 1 shows a schematic diagram of the relationship between the signed distance functions and the design domain. The green, orange, and yellow parts represent the solid design domain Ω, the magenta part represents R2∖Ω, and the indigo curve signifies the boundary of the design domain ∂Ω.
To describe the distance functions more clearly, the gradient of the signed distance function ∇d(x)|∇d(x)| is used to display the direction from an arbitrary point x to its nearest boundary point of the design domain. Certainly, it can also be said that ∇d(x)|∇d(x)| represents the direction closest to the boundary from point x. If the boundary of the design domain Ω is smooth and x is a point located on the boundary ∂Ω, then the length of the gradient vector ∇d(x)|∇d(x)| is equal to 1 and its direction remains the same as the normal direction of the boundary. In general, for almost all points x∈R2, we have
||∇d(x)|∇d(x)|||=1 | (3) |
The above-mentioned features of the gradient vector ∇d(x)|∇d(x)| can be used to determine the mirror reflection (mapping) point R(x) of the point x about its closest boundary ∂Ω. Correspondingly, the formula for the mirror reflection point R(x) can be expressed as below.
R(x)=x−2d(x)∇d(x)|∇d(x)|,x∈R2 | (4) |
Figure 2 explains the formation of the reflection R(x) in the form of a diagram, where the distance of x to xb remains the same as the distance of xb to R(x).
The signed distance functions and their corresponding mapping functions are used to describe the structural design domain in this article. Therefore, it is necessary to construct the signed distance functions about a given domain. For simple geometries, it is easy to confirm the signed distance function. For instance, the distance function of design domain Ω can be expressed as d(x)=||x−x0||−l/2 in the case that Ω indicates a quadrate with a side length l centered at point x0. The level curves of the signed distance function are a series of quadrates centered at point x0 and with a side length less than l. For the design domains with geometric complexity, they should first be discretized into a series of simple geometric configurations. Then, the set operation rules, namely, the union, intersection, difference, and complementarity, are very suitable for the purpose of piecing and connecting different simple geometric configurations. Given two regions A, B and the corresponding distance functions dA(x) and dB(x) as an example, the aforementioned set operation rules are described as follows.
UniondA∪B(x)=min(dA(x),dB(x))IntersectiondA∩B(x)=max(dA(x),dB(x))DifferencedA∖B(x)=max(dA(x),−dB(x))ComplementationdR2∖A(x)=−dA(x) | (5) |
where A and B are the different domains of a simple geometry, such as a circle, straight line, rectangle, triangle, etc. The characteristics for the signed distance functions of combined geometric configurations are shown in Figure 1.
For a given design domain with complex curve boundaries, it can be discretized into a series of simple geometric regions (Ωi, i = 1, 2, ..., n). The distance function of the ith simple geometric region is defined as di(xi). According to Eq (5), the signed distance function can be described by
d(x)=F(d1(x1),d2(x2),...,dn(xn)) | (6) |
where F means an appropriate multivariate function for the set operation rules mentioned above.
Define the set of seeds P={pi}Ni=1 which assembles the x and y coordinates of all N points of meshes into an N-by-2 array in the design domain Ω∈R2. The Voronoi tessellation of pi (pi is a seed in the set P), denoted by Vi (as shown in Figure 3), can be defined by
Vi={x∈Ω|||x−pi||<||x−pj||,∀pj∈P∖{pi},j≠i},i,j=1,...,n | (7) |
where ||∙|| is the distance function (i.e., the standard Euclidean norm). In other words, the Voronoi tessellation Vi consists of the points nearer to the point pi than the others inside P. The properties of Voronoi diagrams mainly include the following: 1) Each V-polygon has a generator element inside it; 2) The distance from the inner point of each V-polygon to the generating element is shorter than the distance to other generating elements; 3) The distance between each of the points on the polygon boundary and the generating element that generates this boundary is equal; 4) The Voronoi polygon boundaries of adjacent graphics are subsets of the original adjacent boundaries. It is worth noting that Voronoi diagrams are bounded convex polygons and play an important role in computational geometry due to their ability to partition regions based on point sets with the closest distance to points, making them essential in the FEA.
If each seed is coincident with the centroid of the corresponding Voronoi element, then the Voronoi tessellation Vi will be centroidal. Then, the distribution of discrete seeds is optimal, and Voronoi diagrams have minimal energy [59] that promotes the regularization of Voronoi tessellation. Additionally, a discretization method based on CVT elements can be used to prevent such imperfections as grid slivers and crossovers, which is very advantageous for the FEA in the future. Therefore, a multi-material topology optimization method combined with the CVT elements is put forward.
According to References [57,58], a minimum distance method of the interior points can eliminate the clustering of randomly generated points and generate the graded meshes. However, many distorted elements may be produced near the complex curve borders and affect the FEA. Taking inspiration from References [25] and [59], a method is proposed here, in combination with Lloyd's algorithm, to make the obtained Voronoi elements centroidal and consequently ensure the quality of meshes. The edge lengths of each polygonal element are employed as evaluation metrics for the quality of meshes. In addition, all edge lengths corresponding to each CVT element are equal. In terms of the quality of meshes, the CVT elements have clear and significant advantages over the triangular and quadrilateral elements. Lloyd's algorithm, which is used to update the seeds P and gain the CVTs, is expressed as follows.
Li(P)=∫Vi(P)∩Δxρ(x)dx∫Vi(P)∩Δρ(x)dx | (8) |
Pk+1=L(Pk) | (9) |
where ρ(x) represents a defined density function that exists throughout the entire design domain; k denotes the kth iteration. It can be observed that the seed set P is mapped by L to the seed set corresponding to the centroids of Voronoi elements. From Eqs (8) and (9), it is obvious that the Voronoi elements are CVTs if the seeds satisfy that P=L(P).
Once the set of discrete points P is determined, the set of reflections will be established by Eq (4), which enables the construction of the Voronoi diagram of P∪R(P). With Lloyd's algorithm, the convergence criterion is set to depend upon the motion of the seeds or the energy gradient function during the iterative process; meanwhile, the centroid set ¯P takes the place of the original point set P until the iterative process ultimately reaches convergence. Here, the energy gradient function with the CVT point ¯pi is chosen as the convergence criterion:
||∇ε||=||∑y∈P2(pi−¯pi)∫Vi(P)∩Δρ(x)dx||:=|Ω|1/2N∫Ωρ(x)dx | (10) |
A "non-dimensional" error parameter is defined by Eq (11):
Er=N||∇ε|||Ω|1/2∫Ωρ(x)dx | (11) |
The convergence criterion is described as follows:
Er<ξ r,0<ξ r<<1 | (12) |
In this work, the discretization for the interior of design domains is achieved through the relevant calculations corresponding to the discrete point set P, while the discretization for the boundary of design domains is achieved by using the Voronoi diagrams corresponding to the seeds P and their reflections R(P) (i.e., P∪R(P)). It is assumed that this work involves a bounded convex design domain Ω, and that, consequently, the mapping points are located outside of Ω. As for the design domain boundary, it approximates an edge which is formed by many tangents across the boundary points (as shown in Figure 3). Therefore, the boundary discretization approximation approach can be used to tackle the issue of complex curve boundaries by the Voronoi elements. For the specified set of points P, the design domain discretized by Voronoi diagrams is expressed below.
DΩ(P)={Vi∈((P∪R(P))∩Ω)|pi∈P},i=1,...,N | (13) |
Due to the fact that the grid discretization method using Voronoi elements is grounded in the variable density concept, this work adopts the use of a topology optimization method combined with the alternating active-phase algorithm [44], which decomposes a multi-phase topology optimization problem into a series of two-phase topology optimization problems and solves them step by step. It should be pointed out that we consider the void as a separate phase (or material) when expressing the formulas. The distribution of each material can be determined via the fields of local volume fraction, namely, ϑj (note that ϑj=ϑj(y)) (j=1,2,…,M), i.e., the local volume fraction of the corresponding material can be defined as the design variable. It is assumed that ϑj satisfies that ϑj∈V(Ω) (j=1,2,…,M), and V represents a function space that is sufficiently regular. Thus, the boundary constraints on each ϑj (j=1,2,…,M) are as follows:
lj⩽ | (14) |
where {{\mathbf{u}}_j} and {{\mathbf{l}}_j} indicate the lower and upper limits of design variables {\vartheta _j}, respectively.
One global volume constraint is usually defined as the constraint of topology optimization. Considering the volume fractions for all materials, the global volume constraint can be expressed as below.
\int_\Omega {{\vartheta _j}{\text{d}}{\mathbf{y}} = {{\Lambda}_j}} |\Omega|, j = 1, 2, ..., {\text{M}} | (15) |
where {\mathbf{y}} signifies a point in \Omega (here, {\mathbf{y}} \in {\mathbf{P}} and {\mathbf{y}} = {{\mathbf{p}}_i} = {\overline {\mathbf{p}} _i} ); {{\Lambda}_j} is a customization parameter; obviously, 0 \leqslant {{\Lambda}_j} \leqslant 1, and \sum\limits_{j = 1}^{\text{M}} {{{\Lambda}_j}} = 1.
In order to facilitate the process of solving the two-dimensional topology optimization problems, all design variables {\vartheta _j} (j = 1, 2, \ldots, {\text{M}}) are compressed into one vector field which is marked with {\mathbf{J}}, namely, {\mathbf{J}} = \{ {\vartheta _1}, ..., {\vartheta _j}, ..., {\vartheta _{\text{M}}}\} . After the discretization for the design domain is finished, the admissible design space [44] \Pi can be defined as below.
\Pi : = \left\{ {\vartheta \in {{\{ {\vartheta _j} \in {\text{V}}\left( \Omega \right)\} }_{j \in \{ 1, 2, ..., {\text{M}}\} }}\left| {\begin{array}{*{20}{c}} {\sum\limits_j^{\text{M}} {{\vartheta _j} = 1} } \\ {\int_\Omega {{\vartheta _j}{\text{d}}{\mathbf{y}} = {{\Lambda}_j}} |\Omega|} \\ {{{\mathbf{l}}_j} \leqslant {\vartheta _j} \leqslant {{\mathbf{u}}_j}} \end{array}, j = 1, 2, ..., {\text{M}}} \right.} \right\} | (16) |
In this article, the performance parameters of each material are given in advance. Here, \overline {\text{M}} (\vartheta) represents the material performance interpolation functions for the volume fraction. The solutions to the partial differential equation (PDE) constraints are represented by {\text{U}}(\vartheta ) = \Theta (\vartheta ({\mathbf{y}})), where \Theta indicates a function space that is sufficiently regular. The partial differential operator subject to PDE constraints can be represented by the operator \Gamma(...). Accordingly, the discrete form for PDE constraints is expressed below.
\Gamma(\overline {\text{M}} (\vartheta {\text{), U}}(\vartheta )) = 0 | (17) |
Considering the above terminology, the structural minimum compliance is defined as the objective of topology optimization. Generally speaking, the objective function \Phi , which is dependent upon \vartheta and {\text{U}}, can be expressed as an integral spanning the entire region of \Omega. Therefore, the discrete mathematical model for each multi-material topology optimization problem can be abstractly represented as follows.
\left\{ {\begin{array}{*{20}{c}} {\mathop {{\text{min}}}\limits_{\vartheta \in \Pi } \Phi (\vartheta , {\text{U}}(\vartheta ))} \\ {{\text{R}}(\overline {\text{M}} (\vartheta ), {\text{U}}(\vartheta )) = 0} \end{array}} \right. | (18) |
According to the alternating active-phase topology optimization algorithm, the optimization process can be implemented by the nested inner and outer loops. In the outer loop, it is necessary to solve {\text{M(M}} - 1)/2 two-phase topology optimization sub-problems, that is, to solve the 0-1-type single-material topology optimization problem shown in the inner loop.
All topology optimization sub-problems in the inner loop are solved via the modified density method. Therefore, in each topology optimization of the inner loop, {\text{M}} - 2 materials are fixed, and the remaining two materials are handled by the binary phase method (as illustrated in Figure 4). The two active materials are represented by subscripts "a" and "b", respectively, and the corresponding volume fractions of the two active materials are represented by {\vartheta _{\text{a}}} and {\vartheta _{\text{b}}}. The volume fraction field of the two active materials is represented by {{\mathbf{r}}_{{\text{ab}}}}.
\mathbf{r}_{\mathrm{ab}}=\mathbf{1}-\sum\limits_{\substack{j=1 \\ j \neq \mathrm{a}, \mathrm{b}}}^{\mathrm{M}} \vartheta_j | (19) |
Since \sum\limits_{j = 1}^{\text{M}} {{\vartheta _j}} = 1 (i.e., \sum\limits_{j = 1}^{\text{M}} {{{\text{v}}_j}} = 1), the volume fraction of material a is required as the design variable for the topology optimization sub-problem of binary phases (material a and material b). After the sub-problem of material a is solved, the volume fraction field of material b can be calculated by solving {\vartheta _{\text{b}}} = {{\mathbf{r}}_{{\text{ab}}}} - {\vartheta _{\text{a}}}.
Considering Eqs (14) and (19), in the sub-problem of binary-phase topology optimization, the temporary upper limit of material a, {{\mathbf{u}}^{{\text{a, temp}}}} (which is adopted for updating the design variables), can be calculated as follows:
{{\mathbf{u}}^{{\text{a, temp}}}} = \min ({{\mathbf{u}}_{\text{a}}}, {{\mathbf{r}}_{{\text{ab}}}}) | (20) |
where {{\mathbf{u}}_a} denotes the upper limit of the volume fraction corresponding to material a (as described by Eq (14)), and the lower bound of material a does not need to change. With the "active phases" a and b, the parameter {\vartheta _{{\text{ab}}}} denotes the design vector of the active materials (a and b) and fixed materials (j \ne a, b). Thus, the topology optimization sub-problem corresponding to the inner loop becomes a 0-1-type (binary phase) counterpart, which is formulated as below.
\left\{ {\begin{array}{*{20}{l}} {{\text{min}}:\Phi ({\vartheta _{{\text{ab}}}}, {\text{U}}({\vartheta _{{\text{ab}}}})) = \frac{1}{2}\int_\Omega {({\mathbf{E}}:{\text{D}}({\mathbf{u}})):{\text{D}}({\mathbf{u}}){\text{d}}{\mathbf{y}}} } \\ {{\text{s}}{\text{.t}}{\text{.}}:\Gamma (\overline {\text{M}} ({\vartheta _{{\text{ab}}}}), {\text{U}}({\vartheta _{{\text{ab}}}})) = 0} \end{array}} \right., \begin{array}{*{20}{c}} {{\text{within}}}&\Omega \end{array} | (21) |
In this work, the objective function of topology optimization is set to minimize the structural compliance, and the material property function \overline {\text{M}} is obtained via analogy by using the elasticity tensor {\mathbf{E}}({\mathbf{y}}){\text{ = }}{\mathbf{E}}(\vartheta ({\mathbf{y}})). Note that {\mathbf{E}} is a fourth-order super-symmetric tensor (symmetric in both the right and the left Cartesian index pair, together with symmetry under the interchange of the pairs). According to References [44] and [45], the SIMP version, as improved by linear interpolation, can be applied in multi-material interpolation as follows.
{\mathbf{E}}(\vartheta ) = \sum\limits_{{\text{j}} = 1}^{\text{M}} {\vartheta _j^q} {{\mathbf{E}}_j} | (22) |
where {{\mathbf{E}}_j} indicates the constant elasticity tensor corresponding to material j (j = 1, 2, \ldots, {\text{M}}); q signifies a power in the SIMP interpolation; {\mathbf{E}}(\vartheta) represents the structural stiffness to be used for subsequent FEA and sensitivity analyses.
For a given volume fraction vector of each material, the PDE operator can be formulated as below.
\left\{ {\begin{array}{*{20}{l}} {\nabla \cdot ({\mathbf{E}}:{\text{D}}({\mathbf{u}})) = {\mathbf{f}}({\mathbf{y}})}&{{\text{within}}}&\Omega \\ {{\mathbf{u}}({\mathbf{y}}) = {\mathbf{\hat u}}({\mathbf{y}})}&{{\text{on}}}&{{\gamma _u}} \\ {({\mathbf{E}}:{\text{D}}({\mathbf{u}})) \cdot {\mathbf{n}} = 0}&{{\text{on}}}&{{\gamma _f}} \\ {({\mathbf{E}}:{\text{D}}({\mathbf{u}})) \cdot {\mathbf{n}} = {\mathbf{\hat t}}({\mathbf{y}})}&{{\text{on}}}&{{\gamma _t}} \end{array}} \right. | (23) |
where {\mathbf{u}} represents the displacement field corresponding to the PDE constraints; {\mathbf{f}} represents the volumetric body force; {\mathbf{\hat u}} indicates the prescribed displacement of boundaries {\gamma _u} ; {\gamma _f} indicates the traction-free part of boundaries; {\mathbf{\hat t}} indicates the traction of a structure through the traction boundaries {\gamma _t} ; the integrated boundary of the design domain is \partial \Omega = {\gamma _u} \cup {\gamma _f} \cup {\gamma _t}. Furthermore, {\text{D}}({\mathbf{u}}): = \frac{1}{2}(\nabla {\mathbf{u}} + {(\nabla {\mathbf{u}})^T}).
In this work, the design domain with the complex curve boundaries is discretized by using the CVT elements, and the main framework for handling topology optimization involving multiple materials is the alternating active-phase algorithm. To overcome the challenges of applying CVT elements in topology optimization involving multiple materials, the design variables denoted by {\vartheta _j} (j = 1, 2, \ldots, {\text{M}}) are improved and regularized by the filtering matrix {{\mathbf{F}}_t} and consequently mapped to the element variables denoted by {{\mathbf{z}}_j} (j = 1, 2, \ldots, {\text{M}}). Taking inspiration from References [23,24,25,44], we propose a new model which combines the alternating active-phase algorithm with centroidal Voronoi elements.
The improved design variables and the filtering sub-matrix are defined as follows:
\left\{ {\begin{array}{*{20}{c}} {{\mathbf{z}} = {{\mathbf{F}}_t}\vartheta } \\ {{{({{\mathbf{F}}_t})}_{lk}} = \frac{{{\text{max}}(0, |{{\text{V}}^k}|(1 - |\vartheta _l^* - \vartheta _k^*|/{\text{R}}))}}{{\sum\nolimits_{k \in S(l)} {|{{\text{V}}^k}|} (1 - |\vartheta _l^* - \vartheta _k^*|/{\text{R}})}}} \end{array}} \right. | (24) |
where {\mathbf{z}} indicates the improved design variables; {{\mathbf{F}}_t} represents the global filtering matrix which is derived from the classic filtering formulas, corresponding to the linear hat filter [23]; {({{\mathbf{F}}_t})_{lk}} indicates the sub-matrix of {{\mathbf{F}}_t} ; {\text{R}} indicates the filtering radius; |{{\text{V}}^k}| represents the kth Voronoi element.
According to optimization criteria of the SIMP method and the filtered elements, the structural volume fraction is described as follows:
{\text{V}}(\vartheta ) = \int_\Omega {{\mathbf{z}}{\text{d}}{\mathbf{y}} = \int_\Omega {{{\mathbf{F}}_t}\vartheta } } {\text{d}}{\mathbf{y}} | (25) |
There are obvious similarities between the volume fraction for each material [44] and the element density in the SIMP method [23,60]. Therefore, the optimal model with the objective function to minimize compliance can be specified as follows:
\left\{ {\begin{array}{*{20}{l}} {\mathop {{\text{min}}}\limits_{\vartheta \in [l, u]} :{{\mathbf{F}}^T}{\mathbf{U}}} \\ {{\text{s}}{\text{.t}}{\text{.}}:\Gamma ({\text{M}}({\vartheta _{ab}}), {\text{U}}({\vartheta _{ab}})) = \frac{1}{\Omega}\int_\Omega {{\mathbf{z}}{\text{d}}{\mathbf{y}} - {{\text{V}}^*}} } \end{array}} \right. | (26) |
where {{\text{V}}^*} represents the upper limit of the structural volume fraction.
Figure 5 describes the specific implementation steps in a topology optimization process for the proposed method. In this work, all CVT elements are generated by random seeds, so the optimal structures may be slightly different from each other for the same numerical example. Nevertheless, the very little difference can be neglected. Thus, topology optimization for all of the following numerical examples is addressed by using random seeds.
The discrete problems are solved by using the gradient-based optimization algorithm in this article. Therefore, it is necessary to calculate the cost function gradient with respect to the original design variables. Note that the sensitivity analysis only involves its own internal parameters {\mathbf{E}} and {\mathbf{V}} , and these two parameters are functions of the design variables. The sensitivity analysis of the cost function is separated along the same line. The sensitivity analysis of the linear separation cost function is usually performed by using the gradient-based algorithms, and it can be described by a chain rule as follows.
\frac{{\partial \Gamma }}{{\partial \vartheta }} = \frac{{\partial {\mathbf{E}}}}{{\partial \vartheta }}\frac{{\partial \Gamma }}{{\partial {\mathbf{E}}}} + \frac{{\partial {\mathbf{V}}}}{{\partial \vartheta }}\frac{{\partial \Gamma }}{{\partial {\mathbf{V}}}} | (27) |
In the compliance problem {\text{C}} = {{\mathbf{F}}^T}{\mathbf{U}}, according to References [23] and [25], there are the expressions of sensitivities that are given below.
\frac{{\partial {\text{C}}}}{{\partial {{\text{E}}^e}}} = - {{\mathbf{U}}^T}\frac{{\partial {\mathbf{K}}}}{{\partial {{\text{E}}^e}}}{\mathbf{U}} = - {{\mathbf{U}}^T}{{\mathbf{k}}_e}{\mathbf{U}}, \frac{{\partial {\text{C}}}}{{\partial {{\text{V}}^e}}} = 0 | (28) |
Thus, the design sensitivities are converted into the following expressions:
\left\{\begin{array}{l}\frac{\partial \mathrm{C}}{\partial \vartheta}=\mathbf{F}_t^{\prime}\left(\frac{\partial \mathbf{E}}{\partial \mathbf{z}} \frac{\partial \mathrm{C}}{\partial \mathbf{E}}+\frac{\partial \mathbf{V}}{\partial \mathbf{z}} \frac{\partial \mathrm{C}}{\partial \mathbf{V}}\right) \\ \frac{\partial \Gamma}{\partial \vartheta}=\mathbf{F}_t^{\prime}\left(\frac{\partial \mathbf{E}}{\partial \mathbf{z}} \frac{\partial \Gamma}{\partial \mathbf{E}}+\frac{\partial \mathbf{V}}{\partial \mathbf{z}} \frac{\partial \Gamma}{\partial \mathbf{V}}\right)\end{array}\right. | (29) |
According to the methods for updating design variables under the condition of variable density [23,60], and combined with Eq (29), the new active-phase design variable \vartheta _a^{new} which satisfies the box constraints is defined as follows:
\vartheta _a^{new} = \left\{ {\begin{array}{*{20}{l}} {\min ({\mathbf{u}}{}_a, {\vartheta _a} + {\text{m}}), }&{{{\text{z}}_{\min }} + ({\vartheta _a} - {{\text{z}}_{\min }}){{( - \frac{{\frac{{\partial {\text{C}}}}{{\partial \vartheta }}}}{{{\rm{\rlap{-} \lambda }} \frac{{\partial \Gamma }}{{\partial \vartheta }}}})}^{\text{η }}} \geqslant \min (u{}_a, {\vartheta _a} + {\text{m}})} \\ {\max ({{\mathbf{l}}_a}, {\vartheta _a} - {\text{m}}), }&{{{\text{z}}_{\min }} + ({\vartheta _a} - {{\text{z}}_{\min }}){{( - \frac{{\frac{{\partial {\text{C}}}}{{\partial \vartheta }}}}{{{\rm{\rlap{-} \lambda }} \frac{{\partial \Gamma }}{{\partial \vartheta }}}})}^{\text{η }}} \leqslant \max ({l_a}, {\vartheta _a} - {\text{m}})} \\ { - \frac{{\frac{{\partial {\text{C}}}}{{\partial \vartheta }}}}{{{\rm{\rlap{-} \lambda }} \frac{{\partial \Gamma }}{{\partial \vartheta }}}}, }&{{\text{otherwise}}} \end{array}} \right. | (30) |
where m is a constant, {\text{m}} = 0.2; {{\text{z}}_{\min }} denotes the minimum value of {z} ; {\text{η }} denotes the damping coefficient; {\rm{\rlap{-} \lambda }} denotes the Lagrange multiplier, which can be solved via the bisection method.
To demonstrate the effectiveness and feasibility of the proposed method, several typical numerical examples of topology optimization are provided in this section. It should be noted that the maximum number of iterations to discretize the structural design domain was set to 500 so as to ensure the quality of Voronoi elements. In addition, the termination criterion for topology optimization was set such that a change in the volume fraction is less than the user-defined threshold. However, it is hard to achieve the termination criterion at times due to the numerical oscillation, so we set the user-defined maximum iteration number to 800, which serves as an additional termination criterion for topology optimization iterations to save program running time.
Taking a semi-Messerschmitt-Bölkow-Blohm (semi-MBB) beam as this numerical example, Figure 6 shows its design domain and boundary conditions with an aspect ratio of 3:1. The horizontal displacement at the left boundary of the design domain and the vertical displacement at the bottom-right corner of the design domain are both constrained. Moreover, there is an external downward force {\mathbf{F}} = 1 at the top-left corner of the design domain. In this section, to explore the impact of different factors on the optimization results from different perspectives, we divided the examples into different cases (i.e., the following cases 1–6). The topology optimization design of a semi-MBB beam involving three different materials (where voids are considered as one material) was first studied (i.e., case 1). In case 1, for materials 1, 2, and 3 (void), their corresponding Young's moduli were set to 3, 1, and 10-9, respectively. For all the materials involved, a Poisson's ratio of 0.3 was equally set. The filtering radius used here was set to 0.08. In addition, for materials 1, 2, and 3, their corresponding volume fractions were set to 0.3, 0.1, and 0.6, respectively.
Figure 7 shows the discretization of the initial design domain, as obtained by utilizing 4000 Voronoi elements. For the polygonal elements and seeds, especially those on the boundaries, their distribution is uniform.
Figure 8 illustrates the topology optimization process corresponding to the semi-MBB beam with three materials in case 1, where red regions denote material 1, indigo blue regions denote material 2, and the light green regions denote material 3 (void). The solutions after 50,100,200,400,600, and 800 iterations are shown in Figure 8(a)–(f), respectively. The minimum compliance of the semi-MBB beam with three materials is {\text{C}} = {\text{22}}{\text{.8265 }} after 800 iterations, which corresponds to the optimal structure in Figure 8(f). The results in Figure 8 indicate that a reasonable distribution of materials can be obtained by using the proposed method under volume constraints. Therefore, the feasibility and effectiveness of the proposed method are verified. Figure 9 illustrates the convergence history for structural compliance in the topology optimization design process for the semi-MBB beam with three materials in case 1. Figure 10 illustrates the changes in volume fraction of the three materials in the topology optimization process for the semi-MBB beam with three materials in case 1. The results in Figures 9 and 10 indicate that the compliance value (i.e., objective function) can be quickly minimized and a reasonable material distribution can be obtained under volume constraints by using the proposed method, with little change in the volume fraction of each material. Therefore, the feasibility and effectiveness of the proposed method are further confirmed.
It is necessary to study the influence of different parameters, such as the number of elements, filtering radius, different stiffness ratios, and different volume fraction ratios of three-material structures. Therefore, other cases of the semi-MBB beam are further provided to investigate the influence of the above-mentioned parameters on the results with the same seeds {\mathbf{P}} .
All parameters set in case 2 remained the same as those set in case 1, except that the semi-MBB structure was initially discretized into 2000 and 5000 Voronoi elements, as respectively illustrated in Figure 11(a), (c). The optimal structures obtained in case 2 are illustrated in Figure 11(b), (d), respectively. The results in Figure 11 indicate that, although the structure is discretized into different numbers of Voronoi elements, the material distributions with little difference under volume constraints can still be obtained by using this method. Specifically, in the case of the optimal structure, the distribution of the material with a high elastic modulus (material 1) remains basically unchanged, whereas the distribution of the material with a low elastic modulus (material 2) is slightly different. Therefore, it can be concluded that different numbers of Voronoi elements have little impact on the optimization results obtained via this method.
In case 3, the filtering radius was changed from 0.08 to 0.03 and 0.1, respectively. The initial discretized semi-MBB beam structure is also shown in Figure 7. Figure 12(a), (b) illustrate the optimal structures with the different filter radii (0.03 and 0.1, respectively). The results in Figure 12 indicate that significantly different material distributions in the optimal structure can be obtained by using the proposed method under the condition of setting different filtering radii. Specifically, in the optimal structure, setting a smaller filtering radius leads to a more dispersed distribution of materials, while setting a larger filtering radius leads to a more concentrated distribution of materials. Therefore, it can be concluded that different filtering radii have a significant impact on the optimization results obtained via this method.
In case 4, the stiffness ratios (i.e., Young's modulus ratios) between different materials are changeable. Under the condition that the values of the Young's modulus of material 2 remained the same as that in case 1, the values of the Young's modulus of material 1 were set to 10 and 50, respectively. The optimal structures with different stiffness ratios for material 1 to material 2 are illustrated in Figure 13(a), (b), respectively. The results in Figure 13 indicate that the proposed method can realize significantly different material distributions and structural compliance in the optimal structure by setting different material stiffness ratios. Specifically, in the optimal structure with the unchanged Young's modulus of material 2, setting a smaller Young's modulus of material 1 results in greater structural compliance, while setting a larger Young's modulus of material 1 results in smaller structural compliance. Therefore, it can be concluded that different material stiffness ratios have a significant impact on the optimization results obtained via this method.
In case 5, the values of volume fraction that were set for the three materials differ from each other, and the corresponding optimal solutions are shown in Figure 14. The optimal structure with values of volume fraction for the three materials equal to 0.2, 0.2 and 0.6 is shown in Figure 14(a). The optimal structure with values of volume fraction for the three materials equal to 0.1, 0.3 and 0.6 is shown in Figure 14(b). The results in Figure 14 indicate that the proposed method can realize significantly different material distributions and structural compliance in the optimal structure by setting different values of volume fractions for the three materials. Specifically, the larger the volume fraction of a strong material (i.e., material 1 with a high Young's modulus) and the smaller the volume fraction of a weak material (i.e., material 2 with a low Young's modulus), the smaller the objective function (structural compliance) of the optimal structure. In other words, the distributions of the strong material (i.e., material 1) and weak material (i.e., material 2) are changed by setting different material volume fractions. Therefore, it can be concluded that different material volume fractions have a significant impact on the optimization results obtained via this method.
To investigate the effect of material quantity on the optimization design results, another new numerical case (i.e., case 6) is given here. The parameters in case 6 were set as follows. The quantity of materials involved in this case is 4, and the Young's moduli for the materials were respectively set to 4, 2, 1, and 10-9. The values of volume fraction for the materials were respectively set to 0.24, 0.08, 0.08, and 0.6. Moreover, the Poisson's ratio, filtering radius, and quantity of polygonal elements were set to the same as those in case 1.
Figure 15 illustrates the topology optimization process for the semi-MBB beam involving four materials in case 6, where the red area represents material 1, the indigo blue area represents material 2, the green area represents material 3, and the black area represents material 4 (void). The solutions after 50,100,200,400,600, and 731 iterations are shown in Figure 15(a)–(f), respectively. The minimum compliance is {\text{C}} = {\text{18}}{\text{.8914}} after 731 iterations. The change in structural compliance for the semi-MBB beam with four materials in the optimization process of case 6 is illustrated in Figure 16. The variation in the volume fraction of each of the four materials in the optimization process of case 6 is illustrated in Figure 17. The results in Figures 15–17 indicate that the structural compliance (i.e., objective function) can be quickly minimized and a reasonable material distribution can be obtained via the proposed method under volume constraints, with little change in the volume fraction of each material. Therefore, the feasibility and effectiveness of the proposed method are further confirmed.
Figure 18 shows the different optimal semi-MBB structures for three and four materials with quadrilateral elements, both of which were discretized by using 60×20 quadrilateral elements. In this case, the filtering radius was set to 1.5. Comparing the objective function values, the results were obtained as follows: {{{C_{3 - q}}} \mathord{\left/ {\vphantom {{{C_{3 - q}}} {{C_{3 - p}}}}} \right. } {{C_{3 - p}}}} \approx 4.6994, {{{C_{4 - q}}} \mathord{\left/ {\vphantom {{{C_{4 - q}}} {{C_{4 - p}}}}} \right. } {{C_{4 - p}}}} \approx 4.4113. Additionally, {C_{{\text{M}} - el}} denotes the objective function value, M signifies the material number, and e l \quad(e l = q \text { or } e l = p) signifies the discretization method (i.e., q signifies the discretization method with quadrilateral elements, or p signifies the discretization method with polygonal elements).
From the above numerical cases, the effectiveness and feasibility of this method are verified. Moreover, a reasonable distribution of materials is shown in each optimal structure, where the strong material mainly undertakes the task of power transmission, and the other materials assume auxiliary roles. It certifies that the proposed method can be used for the topology optimization of multi-material structures with linear boundaries.
The hook structures with three and four materials were studied as another example. The design domain, constraints, and loads for this example are shown in Figure 19. For the first case, the hook structure involves three different materials (where voids are considered as one material), i.e., M = 3. The Young's moduli for the materials were respectively set to 3, 1 and 10-9(for voids), respectively. For all materials involved, a Poisson's ratio of 0.3 was equally set. The filtering radius used here was set to 4. In addition, for materials 1, 2 and 3, their corresponding volume fractions were set to 0.3 (for solid material), 0.1 (for solid material), and 0.6 (for voids), respectively. Here, 4000 Voronoi elements were used to discretize the design domain, as shown in Figure 20.
Figure 21 demonstrates the topology optimization design process for the hook structure with three materials, where the red area represents material 1, the indigo blue area represents material 2, and the green area represents material 3 (void). The solutions after 20,100,200,400,600 and 800 iterations are shown in Figure 21(a)–(f), respectively. For the case of 800 iterations, the optimal solution is shown in Figure 21(f), with the minimum compliance of {\text{C}} = {\text{311}}{\text{.3395}}. The change in structural compliance in the optimization process for the three-material hook structure is illustrated in Figure 22. The variation in the volume fraction corresponding to three materials in the optimization process for this case is illustrated in Figure 23. The results in Figures 21–23 indicate that the structural compliance (i.e., objective function) can be quickly minimized and a reasonable material distribution can be obtained via the proposed method under volume constraints, with little change in the volume fraction of each material. Therefore, the feasibility and effectiveness of this method are further confirmed.
To further examine the performance of this method, similar to the hook structure with three materials in the first case, a hook structure with four materials (M = 4) was considered as another case. The Young's moduli for the materials were set to 9, 3, 1, and 10-9, respectively. The volume fractions were set to 0.24, 0.08, 0.08, and 0.6, signifying that the total volume fraction for solid materials was 40%. The Poisson's ratios, filtering radius, and number of polygonal elements were the same as those in the first case.
Figure 24 describes the topology optimization design process for the hook structure with four materials, where the red area represents material 1, the indigo blue area represents material 2, the green area represents material 3, and the gray area represents material 4 (void). The solutions after 20,100,200,400,600 and 800 iterations are shown in Figure 24(a)–(f), respectively. The minimum compliance is {\text{C}} = {\text{119}}{\text{.0761}} after 800 iterations. Moreover, it is clear that a reasonable distribution of material can also be obtained via this method under volume constraints for each material. The change in structural compliance in the optimization process for the four-material hook structure is illustrated in Figure 25. The variation in the volume fraction for the four materials in the optimization process for this case is illustrated in Figure 26. The results in Figures 24–26 indicate that the structural compliance (i.e., objective function) can be quickly minimized and a reasonable material distribution can be obtained via the proposed method under volume constraints, with little change in the volume fraction of each material. Therefore, the feasibility and effectiveness of this method are further confirmed.
From the above two numerical cases for the hook structure, it is found that this method can effectively address the structural topology optimization problem involving multiple materials with complex curve boundaries, eliminate the checkerboard patterns, and realize a reasonable solution for topology optimization.
A serpentine beam structure was considered as another example to further confirm the feasibility and effectiveness of this method. The design domain, constraints, and loading situation are shown in Figure 27. Similar to the example above (the hook structure), this example for the serpentine beam structure was also studied by analyzing two cases: one with three materials and the other with four materials. In both cases, the serpentine beam was discretized into 4000 Voronoi elements as shown in Figure 28.
First, one case of a three-material serpentine beam structure is considered in this example. In this case, several parameters were set as follows: the Young's moduli for the materials were set to 3, 1, and 10-9, respectively; the filtering radius was set to 0.3; the material volume fractions were set to 0.3, 0.1, and 0.6, respectively; Poisson's ratio was set to 0.3 for all materials.
Figure 29 describes the topology optimization design process for the serpentine beam structure with three materials, where the red regions indicate material 1, the indigo blue regions indicate material 2, and the green regions indicate material 3 (void). The solutions after 20,100,200,400,600, and 800 iterations are shown in Figure 29(a)–(f), respectively. The minimum compliance is {\text{C}} = {\text{186}}{\text{.0482}} after 800 iterations. The change in the structural compliance in the optimization process for the three-material serpentine beam structure is illustrated in Figure 30. The variation in the volume fraction for the three materials in the optimization process for this case is illustrated in Figure 31. The results in Figures 29–31 indicate that the structural compliance (i.e., objective function) can be quickly minimized and a reasonable material distribution can be obtained via the proposed method under volume constraints, with little change in the volume fraction of each material. Therefore, the feasibility and effectiveness of this method are further confirmed.
Then, we consider the other case of a serpentine beam with four materials in this example. In this case, the Young's moduli for the materials were set to 4, 2, 1, and 10-9, respectively; the material volume fractions were set to 0.24, 0.08, 0.08, and 0.6, respectively. The Poisson's ratios, filtering radius, and quantity of polygonal elements were the same as those in the case above.
Figure 32 describes the topology optimization design process for the serpentine beam structure with four materials, where the red area indicates material 1, the indigo blue area indicates material 2, the green area indicates material 3, and the gray area indicates material 4 (void). The solutions after 20,100,150,200,300, and 362 iterations are shown in Figure 32(a)–(f), respectively. The optimal result is realized at 362 iterations, with the corresponding structural compliance of {\text{C}} = {\text{ 149}}{\text{.4421}}. The change in the structural compliance in the optimization process for the four-material serpentine beam structure is illustrated in Figure 33. The variation in the volume fraction for the four materials in the optimization process for this case is illustrated in Figure 34. The results in Figures 32–34 also indicate that the structural compliance (i.e., objective function) can be quickly minimized and a reasonable material distribution can be obtained via the proposed method under volume constraints, with little change in the volume fraction of each material. Therefore, the feasibility and effectiveness of this method are further confirmed.
From the above numerical cases for the serpentine beam structure, it is also found that this method can effectively solve the structural topology optimization problem involving multiple materials and complex curve boundaries, eliminate the checkerboard patterns, and realize a reasonable distribution of materials.
For all of the above examples, it should be noted that the objective function is pursued in the optimization process and multiple materials contribute to the stiffness of the structure as a whole. Therefore, the volume convergence law for structural materials is related to the type of structure, the number of materials, and the type of load. Therefore, the convergence law for structural material volume during the optimization process varies for different examples due to different structural types, material quantities, and load types.
A topology optimization method for the multi-material problems has been developed in this work, and it combines the polygonal discretization method with the alternating active-phase algorithm. The topology optimization objective function serves to minimize structural compliance, with the volume fraction as the constraint condition. The volume fraction of each material is considered as a design variable, which is mapped to its corresponding element variable through a filtering matrix. The structural design domain for topology optimization involving multiple materials has been discretized by using the CVT elements. The feasibility and effectiveness of the proposed method have been verified and confirmed via topology optimization of semi-MBB beam, hook, and serpentine beam structures, respectively. All numerical examples involve three-material and four-material problems, which were divided into multiple cases to study the impact of various factors on the obtained results. By means of this method, the problems of topology optimization involving structures with complex curve boundaries can be effectively solved. Therefore, the applicability scope of this method for structural topology optimization has been substantively expanded. In addition, with the proposed method using polygonal elements, the results of multi-material topology optimization have better numerical stability and higher calculation accuracy than those obtained via topology optimization methods using quadrilateral elements or triangle elements. Furthermore, the sensitivity filtering is avoidable and a relatively concise optimal structure can be obtained via this method, so this work is innovative and contributes to the development of multi-material structural topology optimization design.
The authors declare they have not used Artificial Intelligence (AI) tools in the creation of this article.
This work was supported by Natural Science Foundation of Shaanxi Province (2020JM-200). The authors would like to express thanks to the reviewers for their useful and constructive suggestions that have improved the manuscript.
The authors declare there is no conflict of interest.
[1] | Alpay E, Hari A, Kambouri M, et al. (2010) Gender issues in the university research environment. Eur J Eng Educ 35: 135–145. |
[2] |
Ambec S, Cohen MA, Elgie S, et al. (2013) The Porter hypothesis at 20: can environmental regulation enhance innovation and competitiveness? Rev Environ Econ Pol 7: 222. https://doi.org/10.1093/reep/res016 doi: 10.1093/reep/res016
![]() |
[3] |
Becker RA, Pasurka Jr C, Shadbegian RJ (2013) Do environmental regulations disproportionately affect small businesses? Evidence from the Pollution Abatement Costs and Expenditures survey. J Environ Econo Manage 66: 523538. https://doi.org/10.1016/j.jeem.2013.08.001 doi: 10.1016/j.jeem.2013.08.001
![]() |
[4] |
Berman E, Bui LT (2001) Environmental regulation and productivity: evidence from oil refineries. Rev Econ Stat 83: 498510. https://doi.org/10.1162/00346530152480144 doi: 10.1162/00346530152480144
![]() |
[5] |
Blackman, A. (2008) Can voluntary environmental regulation work in developing countries? Lessons from case studies. Pol Stud J 36: 119141. https://doi.org/10.1111/j.1541-0072.2007.00256.x doi: 10.1111/j.1541-0072.2007.00256.x
![]() |
[6] |
Blackman A, Li Z, Liu AA (2018) Efficacy of command-and-control and market-based environmental regulation in developing countries. Annu Rev Resour Econ 10: 381404. https://doi.org/10.1146/annurev-resource-100517-023144 doi: 10.1146/annurev-resource-100517-023144
![]() |
[7] |
Cai W, Lai KH, Liu C, et al. (2019) Promoting sustainability of manufacturing industry through the lean energy-saving and emission-reduction strategy. Sci Total Environ 665: 2332. https://doi.org/10.1016/j.scitotenv.2019.02.069 doi: 10.1016/j.scitotenv.2019.02.069
![]() |
[8] |
Cai X, Zhu B, Zhang H, et al. (2020) Can direct environmental regulation promote green technology innovation in heavily polluting industries? Evidence from Chinese listed companies. Sci Total Environ 746: 140810. https://doi.org/10.1016/j.scitotenv.2020.140810 doi: 10.1016/j.scitotenv.2020.140810
![]() |
[9] | Callan SJ, Thomas JM (2013) Environmental economics and management: Theory, policy, and applications. Cengage Learning. |
[10] |
Chen H, Hao Y, Li J, et al. (2018) The impact of environmental regulation, shadow economy, and corruption on environmental quality: Theory and empirical evidence from China. J Clean Prod 195: 200214. https://doi.org/10.1016/j.jclepro.2018.05.206 doi: 10.1016/j.jclepro.2018.05.206
![]() |
[11] |
Chen X, Chen YE, Chang CP (2019) The effects of environmental regulation and industrial structure on carbon dioxide emission: a non-linear investigation. Environ Sci Pollut Res 26: 3025230267. https://doi.org/10.1007/s11356-019-06150-6 doi: 10.1007/s11356-019-06150-6
![]() |
[12] |
Chen X, Fu Q, Chang CP (2021) What are the shocks of climate change on clean energy investment: A diversified exploration. Energy Econ 95: 105136. https://doi.org/10.1016/j.eneco.2021.105136 doi: 10.1016/j.eneco.2021.105136
![]() |
[13] | Copeland BR, Taylor MS (2013) Trade and the Environment. Princeton University Press. |
[14] |
Fan M, Yang P, Li Q (2022) Impact of environmental regulation on green total factor productivity: a new perspective of green technological innovation. Environ Sci Pollut Res 29: 116. https://doi.org/10.1007/s11356-022-19576-2 doi: 10.1007/s11356-022-19576-2
![]() |
[15] |
Guo X, Fu L, Sun X (2021) Can Environmental Regulations Promote Greenhouse Gas Abatement in OECD Countries? Command-and-Control vs. Market-Based Policies. Sustainability 13: 6913. https://doi.org/10.3390/su13126913 doi: 10.3390/su13126913
![]() |
[16] |
Hao Y, Ba N, Ren S, et al. (2021) How does international technology spillover affect China's carbon emissions? A new perspective through intellectual property protection. Sust Prod Consumption 25: 577590. https://doi.org/10.1016/j.spc.2020.12.008 doi: 10.1016/j.spc.2020.12.008
![]() |
[17] |
Hao Y, Guo Y, Wu H (2022) The role of information and communication technology on green total factor energy efficiency: Does environmental regulation work? Bus Strategy Environ 31: 403424. https://doi.org/10.1002/bse.2901 doi: 10.1002/bse.2901
![]() |
[18] |
Hassan ST, Khan SUD, Xia E, et al. (2020) Role of institutions in correcting environmental pollution: An empirical investigation. Sust Cities Soc 53: 101901. https://doi.org/10.1016/j.scs.2019.101901 doi: 10.1016/j.scs.2019.101901
![]() |
[19] |
Hazilla M, Kopp RJ (1990) Social cost of environmental quality regulations: A general equilibrium analysis. J Polit Econ 98: 853873. https://doi.org/10.1086/261709 doi: 10.1086/261709
![]() |
[20] |
Hu JL, Wang SC (2006) Total-factor energy efficiency of regions in China. Energy Policy 34: 32063217. https://doi.org/10.1016/j.enpol.2005.06.015 doi: 10.1016/j.enpol.2005.06.015
![]() |
[21] | Huang QH, Hu JF, Chen XD (2018) Environmental regulation and green total factor productivity: Dilemma or win-win. China Popul Resour Environ 28: 140149. |
[22] |
Jia S, Qiu Y, Yang C (2021) Sustainable Development Goals, Financial Inclusion, and Grain Security Efficiency. Agronomy 11: 2542. https://doi.org/10.3390/agronomy11122542 doi: 10.3390/agronomy11122542
![]() |
[23] |
Jin W, Zhang HQ, Liu SS, et al. (2019) Technological innovation, environmental regulation, and green total factor efficiency of industrial water resources. J Clean Prod 211: 6169. https://doi.org/10.1016/j.jclepro.2018.11.172 doi: 10.1016/j.jclepro.2018.11.172
![]() |
[24] |
Kim RE, Bosselmann K (2015) Operationalizing sustainable development: ecological integrity as a grundnorm of international law. Rev Eur Comp Int Environ Law 24: 194208. https://doi.org/10.1111/reel.12109 doi: 10.1111/reel.12109
![]() |
[25] |
Li P, Chen Y (2019) The influence of enterprises' bargaining power on the green total factor productivity effect of environmental regulation—evidence from China. Sustainability 11: 4910. https://doi.org/10.3390/su11184910 doi: 10.3390/su11184910
![]() |
[26] |
Lin J, Xu C (2017) The impact of environmental regulation on total factor energy efficiency: A cross-region analysis in China. Energies 10: 1578. https://doi.org/10.3390/en10101578 doi: 10.3390/en10101578
![]() |
[27] | Liu H, Lei H, Zhou Y (2022) How does green trade affect the environment? Evidence from China. J Econ Anal 1: 127. |
[28] |
Liu M, Tan R, Zhang B (2021) The costs of "blue sky": Environmental regulation, technology upgrading, and labor demand in China. J Dev Econ 150: 102610. https://doi.org/10.1016/j.jdeveco.2020.102610 doi: 10.1016/j.jdeveco.2020.102610
![]() |
[29] |
Liu Y, Wang J (2020) Environmental pollution, environmental regulation, and labor income share. Environ Sci Pollut Res 27: 4516145174. https://doi.org/10.1007/s11356-020-10408-9 doi: 10.1007/s11356-020-10408-9
![]() |
[30] |
Lv C, Shao C, Lee CC (2021) Green technology innovation and financial development: Do environmental regulation and innovation output matter? Energy Econ 98: 105237. https://doi.org/10.1016/j.eneco.2021.105237 doi: 10.1016/j.eneco.2021.105237
![]() |
[31] |
Mandal SK (2010) Do undesirable output and environmental regulation matter in energy efficiency analysis? Evidence from Indian cement industry. Energy Policy 38: 60766083. https://doi.org/10.1016/j.enpol.2010.05.063 doi: 10.1016/j.enpol.2010.05.063
![]() |
[32] |
Mbanyele W, Wang F (2022). Environmental regulation and technological innovation: Evidence from China. Environ Sci Pollut Res 29: 1289012910. https://doi.org/10.1007/s11356-021-14975-3 doi: 10.1007/s11356-021-14975-3
![]() |
[33] |
McMichael AJ (2013) Globalization, climate change, and human health. N Engl J Med 368: 13351343. https://doi.org/10.1056/NEJMra1109341 doi: 10.1056/NEJMra1109341
![]() |
[34] |
Meierrieks D (2021) Weather shocks, climate change and human health. World Dev 138: 105228. https://doi.org/10.1016/j.worlddev.2020.105228 doi: 10.1016/j.worlddev.2020.105228
![]() |
[35] |
Millimet DL, Roy J (2016) Empirical tests of the pollution haven hypothesis when environmental regulation is endogenous. J Appl Econometrics 31: 652677. https://doi.org/10.1002/jae.2451 doi: 10.1002/jae.2451
![]() |
[36] | Mishra V, Smyth R (2012) Environmental regulation and wages in China. J Environ Plann Manage 55: 10751093. |
[37] | Naso P, Huang Y, Swanson T (2017) The porter hypothesis goes to china: Spatial development, environmental regulation and productivity. Cies Res Pap. |
[38] |
Ouyang X, Shao Q, Zhu X, et al. (2019) Environmental regulation, economic growth and air pollution: Panel threshold analysis for OECD countries. Sci Total Environ 657: 234241. https://doi.org/10.1016/j.scitotenv.2018.12.056 doi: 10.1016/j.scitotenv.2018.12.056
![]() |
[39] |
Qiu S, Wang Z, Geng S (2021) How do environmental regulation and foreign investment behavior affect green productivity growth in the industrial sector? An empirical test based on Chinese provincial panel data. J Environ Manage 287: 112282. https://doi.org/10.1016/j.jenvman.2021.112282 doi: 10.1016/j.jenvman.2021.112282
![]() |
[40] |
Ravindra K, Rattan P, Mor S, et al. (2019) Generalized additive models: Building evidence of air pollution, climate change and human health. Environ Int 132: 104987. https://doi.org/10.1016/j.envint.2019.104987 doi: 10.1016/j.envint.2019.104987
![]() |
[41] |
Reinhardt F (1999) Market failure and the environmental policies of firms: Economic rationales for "beyond compliance" behavior. J Ind Ecol 3: 921. https://doi.org/10.1162/108819899569368 doi: 10.1162/108819899569368
![]() |
[42] |
Ren S, Hao Y, Wu H (2021) How Does Green Investment Affect Environmental Pollution? Evidence from China. Environ Resour Econ 81: 2551. https://doi.org/10.1007/s10640-021-00615-4 doi: 10.1007/s10640-021-00615-4
![]() |
[43] | Ren S, Liu Z, Zhanbayev R, et al. (2022) Does the internet development put pressure on energy-saving potential for environmental sustainability? Evidence from China. J Econ Anal 1: 81101. |
[44] |
Rosa EA, Dietz T (2012) Human drivers of national greenhouse-gas emissions. Nat Clim Change 2: 581586. https://doi.org/10.1038/nclimate1506 doi: 10.1038/nclimate1506
![]() |
[45] |
Shao S, Yang L (2014). Natural resource dependence, human capital accumulation, and economic growth: A combined explanation for the resource curse and the resource blessing. Energy Policy 74: 632642. https://doi.org/10.1016/j.enpol.2014.07.007 doi: 10.1016/j.enpol.2014.07.007
![]() |
[46] |
Shen X, Lin B (2020) Policy incentives, R&D investment, and the energy intensity of China's manufacturing sector. J Clean Prod 255: 120208. https://doi.org/10.1016/j.jclepro.2020.120208 doi: 10.1016/j.jclepro.2020.120208
![]() |
[47] |
Shu K, Lu Y, Yu Kuai, et al. (2021). Influences of environmental regulations on skill premium: mediating effect of industrial structure optimization. Environ Econ Pol Stud 23: 245273. https://doi.org/10.1007/s10018-020-00288-1 doi: 10.1007/s10018-020-00288-1
![]() |
[48] |
Song M, Xie Q, Wang S, et al. (2021). Intensity of environmental regulation and environmentally biased technology in the employment market. Omega 100: 102201. https://doi.org/10.1016/j.omega.2020.102201 doi: 10.1016/j.omega.2020.102201
![]() |
[49] |
Su Y, Li Z, Yang C (2021) Spatial interaction spillover effects between digital financial technology and urban ecological efficiency in China: an empirical study based on spatial simultaneous equations. Int J Environ Res Public Health 18: 8535. https://doi.org/10.3390/ijerph18168535 doi: 10.3390/ijerph18168535
![]() |
[50] |
Wang A, Hu S, Lin B (2021) Can environmental regulation solve pollution problems? Theoretical model and empirical research based on the skill premium. Energy Econ 94: 105068. https://doi.org/10.1016/j.eneco.2020.105068 doi: 10.1016/j.eneco.2020.105068
![]() |
[51] |
Wang X, Sun C, Wang S, et al. (2018) Going green or going away? A spatial empirical examination of the relationship between environmental regulations, biased technological progress, and green total factor productivity. Int J Environ Res Public Health 15: 1917. https://doi.org/10.3390/ijerph15091917 doi: 10.3390/ijerph15091917
![]() |
[52] |
Wang Y, Shen N (2016) Environmental regulation and environmental productivity: The case of China. Renewable Sust Energy Rev 62: 758766. https://doi.org/10.1016/j.rser.2016.05.048 doi: 10.1016/j.rser.2016.05.048
![]() |
[53] |
Wang Y, Yan W, Ma D, et al. (2018) Carbon emissions and optimal scale of China's manufacturing agglomeration under heterogeneous environmental regulation. J Clean Prod 176: 140150. https://doi.org/10.1016/j.jclepro.2017.12.118 doi: 10.1016/j.jclepro.2017.12.118
![]() |
[54] |
Wei Y, Liu X (2006) Productivity spillovers from R&D, exports and FDI in China's manufacturing sector. J Int Bus Stud 37: 544557. https://doi.org/10.1057/palgrave.jibs.8400209 doi: 10.1057/palgrave.jibs.8400209
![]() |
[55] |
Wu H, Hao Y, Ren S (2020a) How do environmental regulation and environmental decentralization affect green total factor energy efficiency: Evidence from China. Energy Econ 91: 104880. https://doi.org/10.1016/j.eneco.2020.104880 doi: 10.1016/j.eneco.2020.104880
![]() |
[56] |
Wu H, Xu L, Ren S, et al. (2020b) How do energy consumption and environmental regulation affect carbon emissions in China? New evidence from a dynamic threshold panel model. Resour Policy 67: 101678. https://doi.org/10.1016/j.resourpol.2020.101678 doi: 10.1016/j.resourpol.2020.101678
![]() |
[57] |
Wu H, Xue Y, Hao Y, et al. (2021) How does internet development affect energy-saving and emission reduction? Evidence from China. Energy Econ 103: 105577. https://doi.org/10.1016/j.eneco.2021.105577 doi: 10.1016/j.eneco.2021.105577
![]() |
[58] |
Xu X, Hou P, Liu Y (2022) The impact of heterogeneous environmental regulations on the technology innovation of urban green energy: a study based on the panel threshold model. Green Financ 1: 115136. https://doi.org/10.3934/GF.2022006 doi: 10.3934/GF.2022006
![]() |
[59] |
Yang J, Guo H, Liu B, et al. (2018) Environmental regulation and the Pollution Haven Hypothesis: do environmental regulation measures matter? J Clean Prod 202: 9931000. https://doi.org/10.1016/j.jclepro.2018.08.144 doi: 10.1016/j.jclepro.2018.08.144
![]() |
[60] |
Yang X, Wang J, Cao J, et al. (2021b) The spatial spillover effect of urban sprawl and fiscal decentralization on air pollution: Evidence from 269 cities in China. Empirical Econ 63: 129. https://doi.org/10.1007/s00181-021-02151-y doi: 10.1007/s00181-021-02151-y
![]() |
[61] |
Yang X, Wu H, Ren S, et al. (2021a) Does the development of the internet contribute to air pollution control in China? Mechanism discussion and empirical test. Struct Change Econ Dyn 56: 207224. https://doi.org/10.1016/j.strueco.2020.12.001 doi: 10.1016/j.strueco.2020.12.001
![]() |
[62] |
Yao Y, Hu D, Yang C, et al. (2021) The impact and mechanism of fintech on green total factor productivity. Green Financ 3: 198221. https://doi.org/10.3934/GF.2021011 doi: 10.3934/GF.2021011
![]() |
[63] | Yu DH, Sun T (2017) Environmental regulation, skill premium and international competitiveness of manufacturing industry. China Ind Econ 5: 3553. |
[64] |
Yuan B, Xiang Q (2018) Environmental regulation, industrial innovation and green development of Chinese manufacturing: Based on an extended CDM model. J Clean Prod 176: 895908. https://doi.org/10.1016/j.jclepro.2017.12.034 doi: 10.1016/j.jclepro.2017.12.034
![]() |
[65] |
Zhang G, Liu W, Duan H (2020a) Environmental regulation policies, local government enforcement and pollution-intensive industry transfer in China. Comput Ind Eng 148: 106748. https://doi.org/10.1016/j.cie.2020.106748 doi: 10.1016/j.cie.2020.106748
![]() |
[66] |
Zhang H (2016) Exploring the impact of environmental regulation on economic growth, energy use, and CO2 emissions nexus in China. Nat Hazards 84: 213231. https://doi.org/10.1007/s11069-016-2417-7 doi: 10.1007/s11069-016-2417-7
![]() |
[67] |
Zhang K, Xu D, Li S (2019) The impact of environmental regulation on environmental pollution in China: An empirical study based on the synergistic effect of industrial agglomeration. Environ sci pollut res 26: 2577525788. https://doi.org/10.1007/s11356-019-05854-z doi: 10.1007/s11356-019-05854-z
![]() |
[68] |
Zhang W, Li G, Uddin MK, et al. (2020b) Environmental regulation, foreign investment behavior, and carbon emissions for 30 provinces in China. J Clean Prod 248: 119208. https://doi.org/10.1016/j.jclepro.2019.119208 doi: 10.1016/j.jclepro.2019.119208
![]() |
[69] | Zheng C, Deng F, Zhuo C, et al. (2022) Green Credit Policy, Institution Supply and Enterprise Green Innovation. J Econ Anal 1: 2851. |
[70] |
Zheng J, He J, Shao X, et al. (2022) The employment effects of environmental regulation: Evidence from eleventh five-year plan in China. J Environ Manage 316: 115197. https://doi.org/10.1016/j.jenvman.2022.115197 doi: 10.1016/j.jenvman.2022.115197
![]() |
[71] |
Zhou H, Qu S, Wu Z, et al. (2020) A study of environmental regulation, technological innovation, and energy consumption in China based on spatial econometric models and panel threshold models. Environ Sci Pollut Res 27: 3789437910. https://doi.org/10.1007/s11356-020-09793-y doi: 10.1007/s11356-020-09793-y
![]() |
[72] |
Zhu S, He C, Liu Y (2014) Going green or going away: Environmental regulation, economic geography and firms' strategies in China's pollution-intensive industries. Geoforum 55: 5365. https://doi.org/10.1016/j.geoforum.2014.05.004 doi: 10.1016/j.geoforum.2014.05.004
![]() |