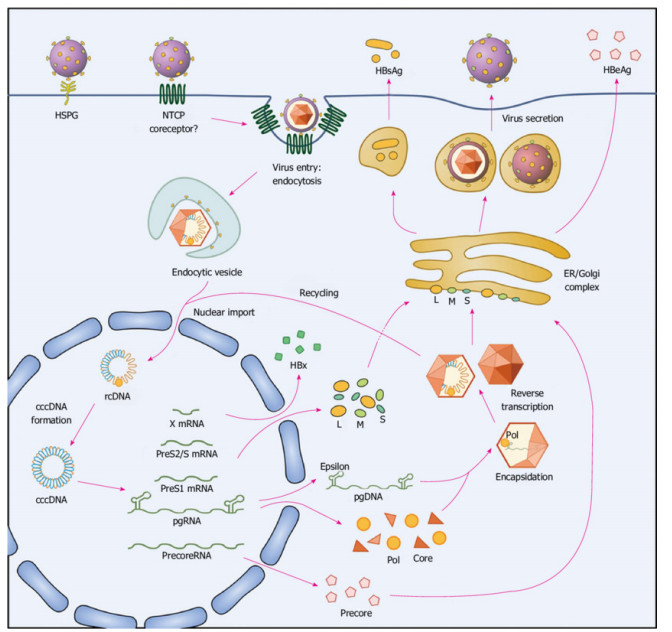
The components of the immune system develop in utero and like a computer, some components are immediately functional (the innate components) but other components must learn the programs and details necessary to function (antigen adaptive components). Like other systems, including military and municipal, the innate and antigen specific components develop into an immune system that helps maintain and surveil the other body processes and systems for aberrations, provide surveillance and protection of the mucoepithelial borders and protection from microbial invasion. Inability, excesses, or errors in these processes cause disease. Aging of the immune system brings immunosenescence, inflammaging, more errors, and decreased surveillance which increases risk for new infections (e.g. COVID-19, influenza), recurrence of latent infections, cancer and autoimmune and inflammatory diseases. With greater understanding of the surveillance, effector and regulatory deficits upon aging, better therapies can be developed.
Citation: Ken S. Rosenthal, Jordan B. Baker. The immune system through the ages[J]. AIMS Allergy and Immunology, 2022, 6(3): 170-187. doi: 10.3934/Allergy.2022013
[1] | Fathalla A. Rihan, Hebatallah J. Alsakaji . Analysis of a stochastic HBV infection model with delayed immune response. Mathematical Biosciences and Engineering, 2021, 18(5): 5194-5220. doi: 10.3934/mbe.2021264 |
[2] | Saima Rashid, Rehana Ashraf, Qurat-Ul-Ain Asif, Fahd Jarad . Novel stochastic dynamics of a fractal-fractional immune effector response to viral infection via latently infectious tissues. Mathematical Biosciences and Engineering, 2022, 19(11): 11563-11594. doi: 10.3934/mbe.2022539 |
[3] | Alia M. Alzubaidi, Hakeem A. Othman, Saif Ullah, Nisar Ahmad, Mohammad Mahtab Alam . Analysis of Monkeypox viral infection with human to animal transmission via a fractional and Fractal-fractional operators with power law kernel. Mathematical Biosciences and Engineering, 2023, 20(4): 6666-6690. doi: 10.3934/mbe.2023287 |
[4] | Jiying Ma, Shasha Ma . Dynamics of a stochastic hepatitis B virus transmission model with media coverage and a case study of China. Mathematical Biosciences and Engineering, 2023, 20(2): 3070-3098. doi: 10.3934/mbe.2023145 |
[5] | Pensiri Yosyingyong, Ratchada Viriyapong . Global dynamics of multiple delays within-host model for a hepatitis B virus infection of hepatocytes with immune response and drug therapy. Mathematical Biosciences and Engineering, 2023, 20(4): 7349-7386. doi: 10.3934/mbe.2023319 |
[6] | Tingting Xue, Long Zhang, Xiaolin Fan . Dynamic modeling and analysis of Hepatitis B epidemic with general incidence. Mathematical Biosciences and Engineering, 2023, 20(6): 10883-10908. doi: 10.3934/mbe.2023483 |
[7] | Noura Laksaci, Ahmed Boudaoui, Seham Mahyoub Al-Mekhlafi, Abdon Atangana . Mathematical analysis and numerical simulation for fractal-fractional cancer model. Mathematical Biosciences and Engineering, 2023, 20(10): 18083-18103. doi: 10.3934/mbe.2023803 |
[8] | Yiping Tan, Yongli Cai, Zhihang Peng, Kaifa Wang, Ruoxia Yao, Weiming Wang . Dynamics of a stochastic HBV infection model with drug therapy and immune response. Mathematical Biosciences and Engineering, 2022, 19(8): 7570-7585. doi: 10.3934/mbe.2022356 |
[9] | Jutarat Kongson, Chatthai Thaiprayoon, Apichat Neamvonk, Jehad Alzabut, Weerawat Sudsutad . Investigation of fractal-fractional HIV infection by evaluating the drug therapy effect in the Atangana-Baleanu sense. Mathematical Biosciences and Engineering, 2022, 19(11): 10762-10808. doi: 10.3934/mbe.2022504 |
[10] | Dong-Me Li, Bing Chai, Qi Wang . A model of hepatitis B virus with random interference infection rate. Mathematical Biosciences and Engineering, 2021, 18(6): 8257-8297. doi: 10.3934/mbe.2021410 |
The components of the immune system develop in utero and like a computer, some components are immediately functional (the innate components) but other components must learn the programs and details necessary to function (antigen adaptive components). Like other systems, including military and municipal, the innate and antigen specific components develop into an immune system that helps maintain and surveil the other body processes and systems for aberrations, provide surveillance and protection of the mucoepithelial borders and protection from microbial invasion. Inability, excesses, or errors in these processes cause disease. Aging of the immune system brings immunosenescence, inflammaging, more errors, and decreased surveillance which increases risk for new infections (e.g. COVID-19, influenza), recurrence of latent infections, cancer and autoimmune and inflammatory diseases. With greater understanding of the surveillance, effector and regulatory deficits upon aging, better therapies can be developed.
Hepatitis B virus causes hepatitis B (HB), an extremely fatal liver disease, and is responsible for more than 2 billion chronic infections that have been discovered globally [1,2]. It is a significant issue for wellbeing promotion. It can lead to prolonged liver damage, persistent inflammation, and a significant chance of fatality through hepatocellular carcinoma and encephalopathy [3]. Hepatitis B infestations can only happen if the pathogen can get into the circulatory system and affect the liver (see Figure 1). Once inside the liver, the infection multiplies and emits a significant quantity of fresh pathogens into the interstitial fluid [4,5].
However, there are two potential stages of this infectious disease: acute and chronic. HB virus that is severe seems to last less than 6 months, typically. If the illness is severe, the defensive mechanism is expected to eradicate the organism of the pathogen, and the patient should fully recover in the next few weeks. The majority of individuals who develop HB have an active virus. The incubation period for persistent HB is 12 weeks or more. Most newborns who contract HBV during infancy, as well as several children between the ages of one and six, develop a degenerative disease. Individuals having persistent HBV disease constitute a sizable 2/3 of chronically transmitted individuals. Despite carrying and spreading germs, these individuals do not exhibit any indications of illness [6]. The surviving one-third experienced acute hepatitis, a liver condition that could potentially be incredibly dangerous (see Figure 2). Over 2.4 billion individuals worldwide suffer from recurrent liver problems. HB's severe or long-term effects cause over 600,000 deaths a year [6]. Cytotoxic T lymphocytes (CTLs) can deliberately threaten contaminated hepatocellular through severe HBV infections and contribute to the pathophysiology of liver failure by coordinating various immunological mechanisms; see [6].
It is crucial to take into account the impact of temporal constraints on the HBV pathway because intercellular propagation and disease penetration are processes that require to be accomplished within a certain environment [7,8]. Additionally, the criteria for development and contact are based on the disease's kind and phase, the defensive system's health, and the milieu of the organism wherein the association works [9]. The participant's general lack of well-being affects the environment of the body. One strategy for investigating the effects of internal environmental parameters on the evolution of HBV transmission could be to modify the determinism account of the bacteriophage association to incorporate the randomized pressure in both an incremental and random manner. Numerous studies, including [10,11,12], have investigated numerical simulations to look into the mechanisms of HBV spreading with sound intensity.
When trying to evaluate the analogous prediction systems, stochastic modelling of highly contagious infection agents has a vital influence and adds a sense of authenticity [13]. In general, various organisms respond in the equivalent habitat to virus-induced designs and highly infectious pathogens, and the consequences can vary. Because the environment is constantly changing, the system's attributes actually oscillate around certain optimum levels [14]. Wang et al. [15] created an insidiously contagious and randomized stochastic HIV infectious framework and studied the results of stationary transmission durability. The researchers [16] addressed how a randomized HBV candida transmission concept with a significant delay in the propagation factor causes regular eruptions and the stagnant distribution and elimination consequences. In addition, the pathogens will be wiped out if the stochastic procreative quantity is below one, and if the stochastic procreative index is significantly higher than one, the infectious disease will be stochastically persistent with a perturbation theory model generated. According to Sun et al. [17], the presence of a solution for the stochastic highly contagious pathogenic framework of CTL feedback and decentralized delay was investigated. Rihan and Alsakaji [18] presented an investigation of an HBV infection framework, including a delayed immune reaction. For further details on epidemic systems; see [19,20,21] and the references cited therein.
Due to their involvement in assisting representatives in exploring the concealed characteristics of the complexities of interactive structures in rheological, permeation, machine design, electromagnetic fields, control, remote sensing, and thermodynamics, implementations of deterministic fractional differential equations (DEs) and fractional stochastic DEs motivated by Brownain motion (BM) have historically attracted the majority of attention in potential implementation. The basic differential and integral operators are unable to capture imperfections, but both fractional derivative/integral operators have been recognized as powerful computing tools [22,23,24]. Furthermore, when simulating physical and experimental events, F-F formulations exhibit diverse and distinct types of variability [25,26,27]. I think it seems that there are undoubtedly many real-world problems that neither fractal nor fractional interpretations are able to accurately reproduce on an individualized dimension. Researchers realized that novel mathematical procedures were required to replicate extremely complicated formations. Despite the assertion that there is hardly anything novel or transformative, it is difficult to believe that the combination of two existing concepts may produce a groundbreaking procedure. For added complexity, a unique differential formula was first implemented in [28]. This logical statement might be understood as the result of the accumulation of the fractal differentiation of a fractional derivative of a specific model. Evidently, there are three possible readings; it all depends on the kernels [29,30,31,32]. The concept was contested and applied to other challenges such as chaotic outgrowths, outbreaks, and diffusion, among others ([33,34,35,36]), and the overwhelming majority of publications provided extremely impressive predicted findings.
In fact, random events are prevalent worldwide. Systems frequently experience random disturbances. Various studies have been conducted on stochastic dynamics; for example, a wide range of scientific theories, including meteorology, accounting, biology, and telecommunication systems, frequently exhibit randomized fluctuations with long-term dependency. In order to analyze fractional stochastic processes, fractional BM employing the Hurst index H(1/2,1) has been proposed as an alternative to classical BM [37,38]. Kerboua et al. [39] investigated the SFDEs with perturbed regulatory frameworks involving fractional BM. Pei and Xu [40] investigated the non-Lipschitz SDEs driven by fractional BM. In 2021, authors [41] presented a novel notion for analyzing and predicting the transmission of COVID-19 throughout Africa and Europe using stochastic and deterministic methods. Alkahtani and Koca [42] contemplated the fractional stochastic SIR system within the fractional calculus technique. Rashid et al. [43] expounded the stochastic F-F tuberculosis model via a nonsingular kernel with random densities.
In the research analysis, we examine the behaviour of fractional stochastic delay DEs of the HBV system involving cell-to-cell propagation and CTLs immunological responsiveness via the fractal-fractional operator based on the exponential decay kernel with random densities, which is inspired by the aforementioned clinical and mathematical concerns. This biological model governed by fractional BM has not yet been demonstrated in the mainstream. As a result of this reality and in an effort to fill this discrepancy, we commence scientific work on one of these formulae in this article. We include the impact of several time delays (TD) and randomness inside a recipient to furnish a highly authentic scenario for the virus's design phase. In the meantime, existence-uniqueness, stochastic basic reproductive number, and the local stability of disease steady states are investigated in the stochastic context. Numerical results are presented by employing the revolutionary technique proposed by Atangana and Araz [41] in the F-F derivative sense. Graphical illustrations are presented with low random densities, incorporating the fractal-dimension and fractional-order. In a nutshell, we presented the simulation findings with and without control.
Before advancing on to the formal description, it is imperative to study certain fundamental F-F operator concepts. Take into account the parameters provided in [28] as well as the functional w(t1), which is continuous and fractal differentiable on [c,d] with fractal-dimension ϖ and fractional-order δ.
Definition 2.1. ([28]) The F-F operator of w(t1) involving the index kernel in terms of Riemann–Liouville (RL) can be presented as follows for δ∈[0,1]:
FFPDδ,ϖ0,t1(w(t1))=1Γ(s−δ)ddtϖ1t1∫0(t1−u)s−δ−1w(u)du, | (2.1) |
where dw(u)duϖ=limt1↦uw(t1)−w(u)tϖ1−uϖ and s−1<δ,ϖ≤s∈N.
Definition 2.2. ([28]) The F-F operator of w(t1) involving the exponential function kernel in terms of RL can be described as follows for δ∈[0,1]:
FFEDδ,ϖ0,t1(w(t1))=M(δ)1−δddtϖ1t1∫0exp(−δ1−δ(t1−u))w(u)du, | (2.2) |
such that M(0)=M(1)=1 having δ>0,ϖ≤s∈N.
Definition 2.3. ([28]) The corresponding F-F integral operator of (2.1) is stated as:
FFPJδ0,t1(w(t1))=ϖΓ(δ)t1∫0(t1−u)δ−1uϖ−1w(u)du. | (2.3) |
Definition 2.4. ([28]) The corresponding F-F integral operator of (2.2) is stated as:
FFEJδ0,t1(w(t1))=δϖM(δ)t1∫0uϖ−1w(u)du+ϖ(1−δ)tϖ−11w(t1)M(δ). | (2.4) |
In this study, we reveal a revolutionary system of stochastic delay DEs for Hepatitis B virus replication in a single recipient, despite the fact that the intracellular stage of over expression is not entirely appreciated. We surmise that throughout an HBV infestation, balanced (unexposed) metabolic enzymes can become infectious both by interaction with contaminated hepatocytes and by freshly generated complimentary pathogens. We furthermore suppose that the challenge-contaminated lymphocytes can be especially attacked by the cytotoxic T lymphocytes (CTLs). With the infiltration of a membrane and the ejection of retroviruses and the creation of viruses, there is still an unavoidably intracellular TD (significant-delay). Additionally, TD is necessary to reflect the gestation, which is the duration needed for the creation of fresh pathogens. Furthermore, we offer a delay differential framework to integrate the CTL community alongside infections predicated on the underlying framework of Nowak et al. [44]. A model is used to evaluate the structure
{˙˜H(t1)=λ−ϕ1˜H(t1)−γ1˜H(t1)˜V(t1)−γ2˜H(t1)˜I(t1),˙˜I(t1)=γ1˜H(t1−ζ1)˜V(t1−ζ1)+γ2˜H(t1−ζ1)˜I(t1−ζ1)−γ3˜I(t1)˜D(t1)−ϕ2˜I(t1),˙˜V(t1)=ω˜I(t1−ζ2)−ϕ3˜V(t1),˙˜D(t1)=ϑ−ϕ4˜D(t1)+γ4˜I(t1)˜D(t1), | (3.1) |
where ˜H(t1),˜I(t1),˜V(t1),˜D(t1), respectively, indicate the hepatocytes that are pure and productive of pathogens, infectious hepatocellular, HB infection pathogens as well as the CTLs. From the first component of the following formula, TD containing ζ1 is applied to estimate the length of period it takes from the first infections of a tissue and the generation of additional vesicles. The other component also incorporates the response time vital for proper hepatocytes to be invaded by malignant hepatocytes interactions before becoming contaminated hepatocellular, whilst ζ2 denotes the time that is required for recently generated particulates to develop before becoming contagious components. Either uncontrolled pathogens attack normal tissue at a pace of γ1˜H˜V (disease transmission mechanism), or viral proteins communicate directly normal tissues at a speed of γ2˜H˜I (cell-to-cell spread mechanism). As a result, the expression "γ1˜H˜V+γ2˜H˜I" denotes the overall disease incidence of susceptible organisms. CTLs generate at a consistent rate of η2 from the hypothalamus and at a pace of γ4˜I˜D as a consequence of stimulating invading pathogens, and they remove invading pathogens at a rate of γ3˜I˜D, where ω is the rate at which viral proteins producing free radicals infections (see Figure 3).
In addition, the complexities of HBV transmission may be impacted by unpredictable perturbations in the mechanism of transmission within the recipient, including fluctuations in climate, emotion, and other endogenous rhythms. Because of this, many researchers have included randomization to determinism in studies of biologic processes to illustrate the influence of environmental heterogeneity, as seen in [44,45].
By incorporating nonlinear disturbance on the spontaneous mortality rate using white noise into every other component of the scheme, we capture the influence of randomness in the host for a more reasonable position of the virus's progression (3.2). Although the HBV infection model's characteristics are not known in advance, the region to which they correspond can indeed be easily identified. So, we suggest a delayed probabilistic approach of the following:
{d˜H(t1)=(λ−ϕ1˜H(t1)−γ1˜H(t1)˜V(t1)−γ2˜H(t1)˜I(t1))dt1+σ1˜HB1(t1),d˜I(t1)=(γ1˜H(t1−ζ1)˜V(t1−ζ1)+γ2˜H(t1−ζ1)˜I(t1−ζ1)−γ3˜I(t1)˜D(t1)−ϕ3˜I(t1))dt1+σ2˜IB2(t1),d˜V(t1)=(ω˜I(t1−ζ2)−ϕ3˜V(t1))dt1+σ3˜VB3(t1),d˜D(t1)=(ϑ−ϕ4˜D(t1)+γ4˜I(t1)˜D(t1))dt1+σ4˜DB4(t1), | (3.2) |
depending on the ICs ˜H(δ)=μ1(δ),˜I(δ)=μ2(δ),˜V(δ)=μ3(δ),˜D(δ)=μ4(δ).
Also, δ∈[−ζ,0],ζ=max{ζ1,ζ2},μκ(δ)∈C,κ=1,...,4. C([−ζ,0],R4+) is the collection of Lebesgue integrable functions in this case including Bκ,κ=1,...,4 is a real-valued standard BM specified on a complete probability space (ω,A,P) meeting the basic requirements [46] and σκ,κ=1,...,4 denote the concentrations of the white noise.
Symbols | Explanation |
λ | Rate of viral hepatocellular development via bone marrow and various tissues |
ϑ | Rate at which CTLs are produced in the thymus |
ϕ1 | Percentage of uninfectious hepatocytes that naturally die |
ϕ2 | Percentage of infectious hepatocytes that naturally die |
ϕ3 | Frequency of harmless pathogens dying |
ϕ4 | Fatality rate of CTLs |
γ1 | Successful viral interaction incidence with healthy hepatocytes |
γ2 | Efficient proportion of interaction between healthy and diseased hepatocytes |
γ3 | CTLs' efficiency at eradicating infectious hepatocytes |
γ4 | CTL development speed as a result of contaminated cells' activation |
ω | Speed of spontaneous viral activity in affected tissues |
In previous decades, the idea of reproduction has been extensively used in epidemiological modelling since it has been recognized as a valuable mathematical tool for evaluating reproduction in a specific illness. According to the concept proposed by Atangana [48], one will identify two components F and ˜V, then
(F˜V−λ˜I)=0 |
will be analyzed to generate reproductive number [49]. The component F is particularly intriguing because it is derived from the nonlinear part of the infected classes.
∂∂˜˜I(˜IN)=[N−˜I]N2 |
and
∂2∂˜I2((N−˜I)N2)=−2[N−˜I]N3=−2(˜H+˜V+˜D)(˜H+˜I+˜V+˜D)3. |
At disease free equilibrium E0=(λϕ1,0,0,ϑϕ4), we have
∂2∂˜I2((N−˜I)N2)=−2(˜H0+˜D0)(˜H0+˜D0)3. |
Therefore, we have
FA=[−2(γ1˜H0+˜D0)(˜H0+˜D0)30]=[−2γ1(λϕ1+ϑϕ4)(λϕ1+ϑϕ4)30]=[−2γ1(ϕ1ϕ4)2(λϕ4+ϑϕ1)20] |
Then,
det(FA˜V−1−λ˜I)=0 |
gives
A=−2γ1ϕ21ϕ34(λϕ4+ϑϕ1)2(γ3ϑ+ϕ2ϕ4)<0. |
Also, A indicates that the expansion will not repeat and will consequently have a single magnitude and wipe out. A>0 indicates that there is sufficient intensity to initiate the regeneration phase, implying that the dispersion will have more than one cycle. Consequently, researchers will supply a strong insight of the aforesaid number.
In this part, we outline a few prerequisites that will ensure a non-negative solution of the stochastic delay DEs scheme presented in (3.2). This is feasible because a favourable result will exist if the system's coefficients satisfy the growth and Lipschitz assumptions.
Theorem 4.1. Suppose there is a system (3.2) (˜H(t1),˜I(t1),˜V(t1),˜D(t1)) with ICs having t1≥−ζ and the solution will stay in R4+, almost probably.
Proof. By means of the system (3.2), satisfies the Lipschitz continuous, there exists a peculiar solution (˜H(t1),˜I(t1),˜V(t1),˜D(t1)) defined on [−ζ,ζe), where ζe signifies the explosion time. In order to demonstrate the solution, we need to to illustrate that ζe=∞. Assume that Λ0>0 be a large enough, thus, we have (˜H(t1),˜I(t1),˜V(t1),˜D(t1))={(μ1(t1),μ2(t1),μ3(t1),μ4(t1)):t1∈(−ζ,0)}∈C([−ζ,0];R4+) contained in [1Λ0,Λ0]. Introducing the stopping time, so for every Λ≥Λ0, we have
ζΛ:=inf{t1∈[−ζ,ζe):min{˜H(t1),˜I(t1),˜V(t1),˜D(t1)}}≤1Λ |
or equivalently
ζΛ:=inf{t1∈[−ζ,ζe):max{˜H(t1),˜I(t1),˜V(t1),˜D(t1)}}≥Λ. |
Now letting infψ=∞ and increasing function ζΛ on Λ. Also, suppose ζ∞=limΛ↦∞ζΛ, then ζ∞≤ζe and need to prove ζ∞=∞ almost surely. We intend to find that ζe=∞ almost surely. If this claim is factually inaccurate, then ∃ some constants T>0 and ϵ∈(0,1) such that P{ζ∞≤T}>ϵ. Thus, for an integer Λ1≥Λ0 such that P{ζΛ≤T}>ϵ,∀Λ≥Λ1. Introducing a mapping U1:R4+↦R+ as:
U1(˜H(t1),˜I(t1),˜V(t1),˜D(t1)):=(˜H−1−ln˜H)+(˜I−1−ln˜I)+Λ2˜V+Λ2(˜D−1−ln˜D)+t1∫t1−ζ1(γ1˜H(s1)˜V(s1)+γ2˜H(s1)˜I(s1))ds1+ωΛ2t1∫t1−ζ1˜I(s1)ds1, |
where Λ2 refer to be a non-negative constant to be determined. In view of the Itô's formula, we have
dU1(˜H(t1),˜I(t1),˜V(t1),˜D(t1))=(1−1˜H)d˜H+(1−1˜I)d˜I+Λ2(1−1˜D)d˜D+Λ2˜V+121˜H2(d˜H)2+121˜I2(d˜I)2+Λ2121˜D2(d˜D)2+(γ1˜H˜V−γ1˜H(t1−ζ1)˜V(t1−ζ1)+γ2˜H˜I−γ2˜H(t1−ζ1)˜I(t1−ζ1)+ωΛ2˜I−ωΛ2˜I(t1−ζ1))=(˜H−1)σ1˜HdB1+(˜I−1)σ2˜IdB2+Λ2(˜D−1)σ4˜DdB4+Λ2σ3˜VdB3+{(λ−ϕ1˜H−λ˜H+ϕ1+γ1˜V+γ2˜I+12σ21)+(ϕ2+γ3˜D−γ1˜H(t1−ζ1)˜V(t1−ζ1)˜I−γ2˜H(t1−ζ1)˜I(t1−ζ1)˜I+12σ22)+Λ2(ω˜I−ϕ3˜V)+Λ2(ϑ−ϕ4˜D+γ4˜I˜D−ϑ˜D+ϕ4−γ4˜I+12σ24)}dt1. |
Simple computations yield
dU1(t1)=HU1dt1−{σ1dB1(t1)+σ2dB2(t1)+σ3dB3(t1)+σ4dB4(t1)}, |
where HU1=(λ−ϕ1˜H−λ˜H+ϕ1+γ1˜V+γ2˜I+12σ21)+(ϕ2+γ3˜D−γ1˜H(t1−ζ1)˜V(t1−ζ1)˜I−γ2˜H(t1−ζ1)˜I(t1−ζ1)˜I+12σ22)+Λ2(ω˜I−ϕ3˜V)+Λ2(ϑ−ϕ4˜D+γ4˜I˜D−ϑ˜D+ϕ4−γ4˜I+12σ24)=C.
Thus, we have
dU1(t1)≤Cdt1−{σ1dB1(t1)+σ2dB2(t1)+Λ2σ3dB3(t1)+Λ2σ4dB4(t1)}. |
Performing integration from 0toζΛ∧Ψ, it can be deduced that
∫ζΛ∧Ψ0dU1(χ(t1))≤∫ζΛ∧Ψ0Cdt1−{∫ζΛ∧Ψ0σ1dB1(t1)+∫ζΛ∧Ψ0σ2dB2(t1)+Λ2∫ζΛ∧Ψ0σ3dB3(t1)+Λ2∫ζΛ∧Ψ0σ4dB4(t1)}, |
using the fact that ζΛ∧T=min{ζn,t1}. Implementing the expectation on the aforesaid variants gives
U1(χ(ζΛ∧T))≤U1(χ(0))+C∫ζΛ∧T0dt1−{∫ζΛ∧T0σ1dB1(t1)+∫ζΛ∧T0σ2dB2(t1)+Λ2∫ζΛ∧T0σ3dB3(t1)+Λ2∫ζΛ∧T0σ4dB4(t1)}. |
This implies that
EU1(χ(ζΛ∧T))≤U1(χ(0))+CE≤U1(χ(0))+CT. | (4.1) |
As U1(χ(ζΛ∧T))>0, then
EU1(χ(ζΛ∧T))=E[U1(χ(ζΛ∧T))x(ζΛ≤T)]+E[U1(χ(ζΛ∧T))x(ζΛ>⊺)]≥E[U1(χ(ζΛ∧T))x(ζΛ≤T)]. | (4.2) |
Further, for ζΛ, since certain factors of χ(ζΛ),say(˜H(ζΛ)) including 0<˜H(ζΛ)≤1Λ<1.
Thus, U1(χ(ζΛ))≥−ln(1Λ), this allow us to write U1(χ(ζΛ))=ln(˜H(ζΛ))≤ln(1Λ).
As a result, from (4.2) and the previous expression, we have
EU1(χ(ζΛ∧T))≥E[U1(χ(ζΛ∧T))x(ζΛ≤T)]≥{−ln(1Λ)}. | (4.3) |
Combining (4.1)-(4.3), we have
EU1(χ(ζΛ∧T))≥−ln(1Λ)P(ζΛ∧T). | (4.4) |
It follows that
P(ζΛ∧T)≤EU1(χ(ζΛ∧T))lnΛ≤U1(χ(0))+CTlnΛ. |
Applying limit sup Λ↦∞ on (4.4), ∀T>0, we find
P(ζΛ∧T)≤0⟹limt1↦∞P(ζΛ∧T)=0. |
This is the desired result.
The average number of subsequent viral infections that a contagious individual causes while they are still dangerous is the primary reproductive component in this scenario. We also want to show that stochastic reproduction (Rs0) is a special kind of basic reproduction number.
Initially, considering the system's (3.2) second cohort, that is
d˜I(t1)=(γ1˜H(t1−ζ1)˜V(t1−ζ1)+γ2˜H(t1−ζ1)˜I(t1−ζ1)−γ3˜I(t1)˜D(t1)−ϕ3˜I(t1))dt1+σ2˜IdB2(t1). | (4.5) |
Considering the Itô's technique for twice differentiation mapping f1(˜I)=ln(I), the Taylor series representation is
df1(t1,˜I(t1))=∂f1∂t1dt1+∂f1∂˜Id˜I+12∂2f1∂˜I2(d˜I)2+∂2f1∂˜I∂t1dt1d˜I+12∂2f1∂t21(dt1)2. |
This implies that
df1(t1,˜I(t1))=1˜I(t1){(γ1˜H(t1−ζ1)˜V(t1−ζ1)+γ2˜H(t1−ζ1)˜I(t1−ζ1)−γ3˜I(t1)˜D(t1)−ϕ3˜I(t1))dt1+σ2˜IdB2(t1)}−12˜I2(t1){(γ1˜H(t1−ζ1)˜V(t1−ζ1)+γ2˜H(t1−ζ1)˜I(t1−ζ1)−γ3˜I(t1)˜D(t1)−ϕ3˜I(t1))dt1+σ2˜IdB2(t1)}2. |
It follows that
df1(t1,˜I(t1))={(γ1˜H(t1−ζ1)˜V(t1−ζ1)˜I(t1)+γ2˜H(t1−ζ1)−γ3˜D(t1)−ϕ3)dt1+σ2dB2(t1)}−12˜I2(t1){A21(dt1)2+2A1A2dt1dB2(t1)+A22(dB2(t1))2}, |
where A1=γ1˜H(t1−ζ1)˜V(t1−ζ1)+γ2˜H(t1−ζ1)˜I(t1−ζ1)−γ3˜I(t1)˜D(t1)−ϕ3˜I(t1) and A2=σ2˜I, then (4.6) can be written as
df1(t1,˜I(t1))={(γ1˜H(t1−ζ1)˜V(t1−ζ1)˜I(t1)+γ2˜H(t1−ζ1)−γ3˜D(t1)−ϕ3)dt1+σ2dB2(t1)}−12˜I2(t1){A22(dB2(t1))2}={(γ1˜H(t1−ζ1)˜V(t1−ζ1)˜I(t1)+γ2˜H(t1−ζ1)−γ3˜D(t1)−ϕ3)dt1+σ2dB2(t1)}−12˜I2(t1){(σ2˜I)2}dt1. | (4.6) |
As dt1↦0,(dt1)2,dt1dB2(t1)↦0 and (dB2(t1))2 can be converted to dt1 (By the variance of Wiener technique), we have
df1(t1,˜I(t1))={(γ1˜H(t1−ζ1)˜V(t1−ζ1)˜I(t1)+γ2˜H(t1−ζ1)−γ3˜D(t1)−ϕ3)dt1+σ2dB2(t1)}−12(σ2)2dt1={(γ1˜H(t1−ζ1)˜V(t1−ζ1)˜I(t1)+γ2˜H(t1−ζ1)−γ3˜D(t1)−ϕ3)dt1−12σ22}dt1+σ2dB2(t1). | (4.7) |
Taking into consideration the next generation matrices [47] are as follows
F=[γ2λϕ1γ1λϕ100]and˜V=[γ3ϑϕ4+ϕ20−ωϕ3]. |
Therefore, F and ˜V at disease-free equilibrium E0=(λϕ1,0,0,ϑϕ4), we find
˜V−1=[ϕ4γ3ϑ+ϕ2ϕ40ωϕ4ϕ3(ϕ2ϕ4+γ3ϑ)1/ϕ3]. |
Thus, the basic reproduction number is Rs0=ρ(F˜V−1) is
Rs0=λϕ4(ωγ1+ϕ3γ2)ϕ1ϕ3(ϑγ3+ϕ2ϕ4), |
which is the required stochastic fundamental reproduction number.
Theorem 4.2. For a community's infection to be eradicated, then Rs0<1.
If Rs0<1, then for any provided ICs (˜H(0),˜V(0),˜I(0),˜D(0))=(˜H0,˜V0,˜I0,R0)∈R4+. Therefore, ˜I(t1) admits limt1↦∞supln(˜I(t1))t1≤ϕ3(Rs0−1) almost surely.
Proof. Taking into consideration (4.5), we have
df1(t1,˜I(t1))={(γ1˜H˜V+γ2˜H−γ3˜D−ϕ3−12σ22)dt1+σ2dB2(t1)}. |
It follows that
dln(˜I)=(γ1˜H˜V+γ2˜H−γ3˜D−ϕ3−12σ22)dt1+σ2dB2(t1). |
After performing integration, we have
ln(˜I)=ln(˜I0)+t1∫0(γ1˜H˜V+γ2˜H−γ3˜D−ϕ3−12σ22)dt1+σ2t1∫0dB2(t1)≤ln(˜I0)+(γ2λϕ1−γ3ϑϕ4−12σ22−ϕ3)t1⏟atDFEPE0+σ2t1∫0dB2(t1)≤ln(˜I0)+(γ2λϕ1−γ3ϑϕ4−12σ22−ϕ3)t1+Υ(t1), | (4.8) |
where Υ(t1)=σ3t1∫0dB3(t1) is the martingale. therefore, by the strong principal of large values for Υ(t1), see [50], we get limt1↦∞supΥ(t1)t1=0 almost probably.
After dividing by t1 and applying limit t1↦∞, then (4.8) reduces to
limt1↦∞supln(˜I)t1≤γ2λϕ1−γ3ϑϕ4−12σ22−ϕ3=ϕ3(λϕ4(ωγ1+ϕ3γ2)ϕ1ϕ3(ϑγ3+ϕ2ϕ4)−12ϕ3σ22)=ϕ3(Rs0−1)<0. |
This indicates that Rs0<1.
Finally, Rs0 should be smaller than 1 for virus elimination in a population.
Remark 4.1. Under some settings, the solution of framework (3.2) oscillates all around endemic equilibrium of the undisturbed system (3.1) if Rs0>1. This indicates that as long as the concentrations of white noise are low enough, the sickness will endure.
In what follows, the framework is further expanded to F-F derivative operators.
Firstly, we present the Caputo-Fabrizio F-F derivative for the classical derivative formulation. As a result, the stochastic F-F framework will be quantitatively determined employing the numerical technique described previously. This type of system is presented by
{FFE0Dδ,ϖt1˜H(t1)=(λ−ϕ1˜H(t1)−γ1˜H(t1)˜V(t1)−γ1˜H(t1)˜I(t1))+σ1G1(t1,˜H)B1(t1),FFE0Dδ,ϖt1˜I(t1)=(γ1˜H(t1−ζ1)˜V(t1−ζ1)+γ2˜H(t1−ζ1)˜I(t1−ζ1)−γ3˜I(t1)˜D(t1)−ϕ3˜I(t1))+σ2G2(t1,˜I)B2(t1),FFE0Dδ,ϖt1˜V(t1)=(ω˜I(t1−ζ2)−ϕ3˜V(t1))+σ3G3(t1,˜V)B3(t1),FFE0Dδ,ϖt1˜D(t1)=(ϑ−ϕ4˜D(t1)+γ4˜I(t1)˜D(t1))+σ4G4(t1,˜D)B4(t1). | (5.1) |
For tm+1=(m+1)Δt1, then we the aforementioned system can be integrated as follows
˜Hm+1=˜H0+(1−δ)δϖM(δ)ϖt1ϖ−1m+1[{˜H∗(t1m+1,˜Hqm+1,˜Iqm+1,˜Vqm+1,˜Dqm+1)+σ1G1(t1m+1,˜Hqm+1)(B1(t1m+1)−B1(t1m))]+δϖM(δ)m∑ℓ=0[t1ℓ+1∫t1ℓϱδ−1˜H∗(ϱ,˜H,˜I,˜V,˜D)dϱ+t1ℓ+1∫t1ℓϱδ−1σ1G1(ϱ,˜H)dB1ϱ], |
˜Im+1=˜I0+(1−δ)δϖM(δ)ϖt1ϖ−1m+1[{˜I∗(t1m+1,˜Hqm+1,˜Iqm+1,˜Vqm+1,˜Dqm+1)+σ2G2(t1m+1,˜Iqm+1)(B2(t1m+1)−B2(t1m))]+δϖM(δ)m∑ℓ=0[t1ℓ+1∫t1ℓϱδ−1˜I∗(ϱ,˜H,˜I,˜V,˜D)dϱ+t1ℓ+1∫t1ℓϱδ−1σ2G2(ϱ,˜I)dB2ϱ], |
˜Vm+1=˜V0+(1−δ)δϖM(δ)ϖt1ϖ−1m+1[{˜V∗(t1m+1,˜Iqm+1,˜Vqm+1)+σ3G3(t1m+1,˜Vqm+1)(B3(t1m+1)−B3(t1m))]+δϖM(δ)m∑ℓ=0[t1ℓ+1∫t1ℓϱδ−1˜V∗(ϱ,˜I,˜V)dϱ+t1ℓ+1∫t1ℓϱδ−1σ3G3(ϱ,˜V)dB3ϱ], |
˜Dm+1=˜D0+(1−δ)δϖM(δ)ϖt1ϖ−1m+1[{˜D∗(t1m+1,˜Iqm+1,˜Dqm+1)+σ4G4(t1m+1,˜Dqm+1)(B4(t1m+1)−B4(t1m))]+δϖM(δ)m∑ℓ=0[t1ℓ+1∫t1ℓϱδ−1˜D∗(ϱ,˜I,˜D)dϱ+t1ℓ+1∫t1ℓϱδ−1σ4G4(ϱ,˜D)dB4ϱ]. |
Utilizing the fact of polynomials in the aforesaid system, we find
˜Hm+1=˜H0+(1−δ)δϖM(δ)ϖt1ϖ−1m+1[{˜H∗(t1m+1,˜Hqm+1,˜Iqm+1,˜Vqm+1,˜Dqm+1)+σ1G1(t1m+1,˜Hqm+1)(B1(t1m+1)−B1(t1m))]+δϖM(δ)m−1∑ℓ=0[{˜H∗(t1ℓ+1,˜Hℓ+1,˜Iℓ+1,˜Vℓ+1,˜Dℓ+1)hϖϖ((ℓ+1)ϖ−ℓϖ)+˜H∗(t1ℓ+1,˜Hℓ+1,˜Iℓ+1,˜Vℓ+1,˜Dℓ+1)−˜H∗(t1ℓ,˜Hℓ,˜Vℓ,˜Iℓ,˜Dℓ)h×(hϖ+1(ℓϖ(ℓ+1+ϖ)−(ℓ+1)ϖ+1)ϖ(ϖ+1))+˜H∗(t1ℓ+1,˜Hℓ+1,˜Iℓ+1,˜Vℓ+1,˜Dℓ+1)−2˜H∗(t1ℓ,˜Hℓ,˜Vℓ,˜Iℓ,˜Dℓ)+˜H∗(t1ℓ−1,˜Hℓ−1,˜Iℓ−1,˜Vℓ−1,˜Dℓ−1)2h2×(2hϖ+2((ℓ−ϖ2)(ℓ+1)ϖ+1−ℓϖ+1(ℓ+1+ϖ2))ϖ(ϖ+1)(ϖ+2))]+σ1δϖM(δ)m−1∑ℓ=0[{G1(t1ℓ+1,˜Hℓ+1)(B1(t1ℓ+1)−B1(t1ℓ))hϖϖ((ℓ+1)ϖ−ℓϖ)+{G1(t1ℓ+1,˜Hℓ+1)(B1(t1ℓ+1)−B1(t1ℓ))−G1(t1ℓ,˜Hℓ)(B1(t1ℓ)−B1(t1ℓ−1))}×(hϖ+1(ℓϖ(ℓ+1+ϖ)−(ℓ+1)ϖ+1)ϖ(ϖ+1))+{G1(t1ℓ+1,˜Hℓ+1)(B1(t1ℓ+1)−B1(t1ℓ))−2G1(t1ℓ,˜Hℓ)(B1(t1ℓ+1)−B1(t1ℓ))2h+G1(t1ℓ−1,˜Hℓ−1)(B1(t1ℓ)−B1(t1ℓ−1))2h}(2hϖ+2((ℓ−ϖ2)(ℓ+1)ϖ+1−ℓϖ+1(ℓ+1+ϖ2))ϖ(ϖ+1)(ϖ+2))], |
˜Im+1=˜I0+(1−δ)δϖM(δ)ϖt1ϖ−1m+1[{˜I∗(t1m+1,˜Hqm+1,˜Iqm+1,˜Vqm+1,˜Dqm+1)+σ2G2(t1m+1,˜Iqm+1)(B2(t1m+1)−B2(t1m))]+δϖM(δ)m−1∑ℓ=0[{˜I∗(t1ℓ+1,˜Hℓ+1,˜Iℓ+1,˜Vℓ+1,Rℓ+1)hϖϖ((ℓ+1)ϖ−ℓϖ)+˜I∗(t1ℓ+1,˜Hℓ+1,˜Iℓ+1,˜Vℓ+1,˜Dℓ+1)−˜I∗(t1ℓ,˜Hℓ,˜Iℓ,˜Vℓ,˜Dℓ)h×(hϖ+1(ℓϖ(ℓ+1+ϖ)−(ℓ+1)ϖ+1)ϖ(ϖ+1))+˜I∗(t1ℓ+1,˜Hℓ+1,˜Iℓ+1,˜Vℓ+1,˜Dℓ+1)−2˜I∗(t1ℓ,˜Hℓ,˜Iℓ,˜Vℓ,˜Dℓ)+˜I∗(t1ℓ−1,˜Hℓ−1,˜Iℓ−1,˜Vℓ−1,˜Dℓ−1)2h2×(2hϖ+2((ℓ−ϖ2)(ℓ+1)ϖ+1−ℓϖ+1(ℓ+1+ϖ2))ϖ(ϖ+1)(ϖ+2))]+σ2δϖM(δ)m−1∑ℓ=0[{G2(t1ℓ+1,˜Iℓ+1)(B2(t1ℓ+1)−B2(t1ℓ))hϖϖ((ℓ+1)ϖ−ℓϖ)+{G2(t1ℓ+1,˜Iℓ+1)(B2(t1ℓ+1)−B2(t1ℓ))−G2(t1ℓ,˜Iℓ)(B2(t1ℓ)−B2(t1ℓ−1))}×(hϖ+1(ℓϖ(ℓ+1+ϖ)−(ℓ+1)ϖ+1)ϖ(ϖ+1))+{G2(t1ℓ+1,˜Iℓ+1)(B2(t1ℓ+1)−B2(t1ℓ))−2G2(t1ℓ,˜Iℓ)(B2(t1ℓ+1)−B2(t1ℓ))2h+G2(t1ℓ−1,˜Iℓ−1)(B2(t1ℓ)−B2(t1ℓ−1))2h}(2hϖ+2((ℓ−ϖ2)(ℓ+1)ϖ+1−ℓϖ+1(ℓ+1+ϖ2))ϖ(ϖ+1)(ϖ+2))], |
˜Vm+1=˜V0+(1−δ)δϖM(δ)ϖt1ϖ−1m+1[{˜V∗(t1m+1,˜Iqm+1,˜Vqm+1)+σ2G2(t1m+1,˜Vqm+1)(B3(t1m+1)−B3(t1m))]+δϖM(δ)m−1∑ℓ=0[{˜V∗(t1ℓ+1,˜Iℓ+1,˜Vℓ+1)hϖϖ((ℓ+1)ϖ−ℓϖ)+˜V∗(t1ℓ+1,˜Iℓ+1,˜Vℓ+1)−˜V∗(t1ℓ,˜Iℓ,˜Vℓ)h×(hϖ+1(ℓϖ(ℓ+1+ϖ)−(ℓ+1)ϖ+1)ϖ(ϖ+1))+˜V∗(t1ℓ+1,˜Iℓ+1,˜Vℓ+1)−2˜V∗(t1ℓ,˜Iℓ,˜Vℓ)+˜V∗(t1ℓ−1,˜Iℓ−1,˜Vℓ−1)2h2×(2hϖ+2((ℓ−ϖ2)(ℓ+1)ϖ+1−ℓϖ+1(ℓ+1+ϖ2))ϖ(ϖ+1)(ϖ+2))]+σ2δϖM(δ)m−1∑ℓ=0[{G2(t1ℓ+1,˜Vℓ+1)(B3(t1ℓ+1)−B3(t1ℓ))hϖϖ((ℓ+1)ϖ−ℓϖ)+{G3(t1ℓ+1,˜Vℓ+1)(B3(t1ℓ+1)−B3(t1ℓ))−G3(t1ℓ,˜Vℓ)(B3(t1ℓ)−B3(t1ℓ−1))}×(hϖ+1(ℓϖ(ℓ+1+ϖ)−(ℓ+1)ϖ+1)ϖ(ϖ+1))+{G3(t1ℓ+1,˜Vℓ+1)(B3(t1ℓ+1)−B3(t1ℓ))−2G3(t1ℓ,˜Vℓ)(B3(t1ℓ+1)−B3(t1ℓ))2h+G3(t1ℓ−1,˜Vℓ−1)(B3(t1ℓ)−B3(t1ℓ−1))2h}(2hϖ+2((ℓ−ϖ2)(ℓ+1)ϖ+1−ℓϖ+1(ℓ+1+ϖ2))ϖ(ϖ+1)(ϖ+2))], |
˜Dm+1=˜D0+(1−δ)δϖM(δ)ϖt1ϖ−1m+1[{˜D∗(t1m+1,˜Iqm+1,˜Dqm+1)+σ4G4(t1m+1,˜Dqm+1)(B4(t1m+1)−B4(t1m))]+δϖM(δ)m−1∑ℓ=0[{˜D∗(t1ℓ+1,˜Iℓ+1,˜Dℓ+1)hϖϖ((ℓ+1)ϖ−ℓϖ)+˜D∗(t1ℓ+1,˜Iℓ+1,˜Dℓ+1)−˜D∗(t1ℓ,˜Iℓ,˜Dℓ)h×(hϖ+1(ℓϖ(ℓ+1+ϖ)−(ℓ+1)ϖ+1)ϖ(ϖ+1))+˜D∗(t1ℓ+1,˜Iℓ+1,˜Dℓ+1)−2˜D∗(t1ℓ,˜Iℓ,˜Dℓ)+˜D∗(t1ℓ−1,˜Iℓ−1,˜Dℓ−1)2h2×(2hϖ+2((ℓ−ϖ2)(ℓ+1)ϖ+1−ℓϖ+1(ℓ+1+ϖ2))ϖ(ϖ+1)(ϖ+2))]+σ1δϖM(δ)m−1∑ℓ=0[{G4(t1ℓ+1,˜Dℓ+1)(B4(t1ℓ+1)−B4(t1ℓ))hϖϖ((ℓ+1)ϖ−ℓϖ)+{G4(t1ℓ+1,˜Dℓ+1)(B4(t1ℓ+1)−B4(t1ℓ))−G4(t1ℓ,˜Dℓ)(B4(t1ℓ)−B4(t1ℓ−1))}×(hϖ+1(ℓϖ(ℓ+1+ϖ)−(ℓ+1)ϖ+1)ϖ(ϖ+1))+{G4(t1ℓ+1,˜Dℓ+1)(B4(t1ℓ+1)−B4(t1ℓ))−2G4(t1ℓ,˜Dℓ)(B4(t1ℓ+1)−B4(t1ℓ))2h+G4(t1ℓ−1,˜Dℓ−1)(B4(t1ℓ)−B4(t1ℓ−1))2h}(2hϖ+2((ℓ−ϖ2)(ℓ+1)ϖ+1−ℓϖ+1(ℓ+1+ϖ2))ϖ(ϖ+1)(ϖ+2))]. |
Furthermore, we can write
˜Hm+1=˜H0+(1−δ)δϖM(δ)ϖt1ϖ−1m+1[{˜H∗(t1m+1,˜Hqm+1,˜Iqm+1,˜Vqm+1,˜Dqm+1)+σ1G1(t1m+1,˜Hqm+1)(B1(t1m+1)−B1(t1m))]+δϖM(δ)m−1∑ℓ=0[{˜H∗(t1ℓ+1,˜Hℓ+1,˜Iℓ+1,˜Vℓ+1,˜Dℓ+1)hϖϖ((ℓ+1)ϖ−ℓϖ)+˜H∗(t1ℓ+1,˜Hℓ+1,˜Iℓ+1,˜Vℓ+1,˜Dℓ+1)−˜H∗(t1ℓ,˜Hℓ,˜Iℓ,˜Vℓ,˜Dℓ)h×(hϖ+1(ℓϖ(ℓ+1+ϖ)−(ℓ+1)ϖ+1)ϖ(ϖ+1))+˜H∗(t1ℓ+1,˜Hℓ+1,˜Iℓ+1,˜Vℓ+1,˜Dℓ+1)−2˜H∗(t1ℓ,˜Hℓ,˜Iℓ,˜Vℓ,˜Dℓ)+˜H∗(t1ℓ−1,˜Hℓ−1,˜Iℓ−1,˜Vℓ−1,˜Dℓ−1)2h2×(2hϖ+2((ℓ−ϖ2)(ℓ+1)ϖ+1−ℓϖ+1(ℓ+1+ϖ2))ϖ(ϖ+1)(ϖ+2))]+σ1δϖM(δ)m−1∑ℓ=0[{G1(t1ℓ+1,˜Hℓ+1)(B1(t1ℓ+1)−B1(t1ℓ))hϖϖ((ℓ+1)ϖ−ℓϖ)+{G1(t1ℓ+1,˜Hℓ+1)(B1(t1ℓ+1)−B1(t1ℓ))−G1(t1ℓ,˜Hℓ)(B1(t1ℓ)−B1(t1ℓ−1))}×(hϖ+1(ℓϖ(ℓ+1+ϖ)−(ℓ+1)ϖ+1)ϖ(ϖ+1))+{G1(t1ℓ+1,˜Hℓ+1)(B1(t1ℓ+1)−B1(t1ℓ))−2G1(t1ℓ,˜Hℓ)(B1(t1ℓ+1)−B1(t1ℓ))2h+G1(t1ℓ−1,˜Hℓ−1)(B1(t1ℓ)−B1(t1ℓ−1))2h}(2hϖ+2((ℓ−ϖ2)(ℓ+1)ϖ+1−ℓϖ+1(ℓ+1+ϖ2))ϖ(ϖ+1)(ϖ+2))] |
+δϖM(δ)[{{˜H∗(t1m+1,˜Hqm+1,˜Iqm+1,˜Vqm+1,˜Dqm+1)+G1(t1m+1,˜Hqm+1)(B1(t1m+1)−B1(t1m))}hϖϖ((m+1)ϖ−mϖ)+˜H∗(t1m+1,˜Hm+1,˜Im+1,˜Vm+1,˜Dm+1)−˜H∗(t1m,˜Hm,˜Im,˜Vm,˜Dm)h+{G1(t1m+1,˜Hm+1)(B1(t1m+1)−B1(t1m))−G1(t1m,˜Hm)(B1(t1m)−B1(t1m−1))}×(hϖ+1(ℓϖ(ℓ+1+ϖ)−(ℓ+1)ϖ+1)ϖ(ϖ+1))+˜H∗(t1m+1,˜Hm+1,˜Im+1,˜Vm+1,˜Dm+1)−2˜H∗(t1m,˜Hm,˜Im,˜Vm,˜Dm)+˜H∗(t1m−1,˜Hm−1,˜Im−1,˜Vm−1,˜Dm−1)2h2+{G1(t1m+1,˜Hm+1)(B1(t1m+1)−B1(t1m))−2G1(t1m,˜Hm)(B1(t1m)−B1(t1m−1))2h+G1(t1m−1,˜Hm−1)(B1(t1m−1)−B1(t1m−2))2h}(2hϖ+2((ℓ−ϖ2)(ℓ+1)ϖ+1−ℓϖ+1(ℓ+1+ϖ2))ϖ(ϖ+1)(ϖ+2))], |
˜Im+1=˜I0+(1−δ)δϖM(δ)ϖt1ϖ−1m+1[{˜I∗(t1m+1,˜Hqm+1,˜Iqm+1,˜Vqm+1,˜Dqm+1)+σ2G2(t1m+1,˜Iqm+1)(B3(t1m+1)−B2(t1m))]+δϖM(δ)m−1∑ℓ=0[{˜I∗(t1ℓ+1,˜Hℓ+1,˜Iℓ+1,˜Vℓ+1,˜Dℓ+1)hϖϖ((ℓ+1)ϖ−ℓϖ)+˜I∗(t1ℓ+1,˜Hℓ+1,˜Iℓ+1,˜Vℓ+1,˜Dℓ+1)−˜I∗(t1ℓ,˜Hℓ,˜Iℓ,˜Vℓ,˜Dℓ)h×(hϖ+1(ℓϖ(ℓ+1+ϖ)−(ℓ+1)ϖ+1)ϖ(ϖ+1))+˜I∗(t1ℓ+1,˜Hℓ+1,˜Iℓ+1,˜Vℓ+1,˜Dℓ+1)−2˜V∗(t1ℓ,˜Hℓ,˜Iℓ,˜Vℓ,˜Dℓ)+˜V∗(t1ℓ−1,˜Hℓ−1,˜Iℓ−1,˜Vℓ−1,˜Dℓ−1)2h2×(2hϖ+2((ℓ−ϖ2)(ℓ+1)ϖ+1−ℓϖ+1(ℓ+1+ϖ2))ϖ(ϖ+1)(ϖ+2))]+σ3δϖM(δ)m−1∑ℓ=0[{G2(t1ℓ+1,˜Iℓ+1)(B2(t1ℓ+1)−B2(t1ℓ))hϖϖ((ℓ+1)ϖ−ℓϖ)+{G2(t1ℓ+1,˜Iℓ+1)(B2(t1ℓ+1)−B2(t1ℓ))−G2(t1ℓ,˜Iℓ)(B2(t1ℓ)−B2(t1ℓ−1))}×(hϖ+1(ℓϖ(ℓ+1+ϖ)−(ℓ+1)ϖ+1)ϖ(ϖ+1))+{G2(t1ℓ+1,˜Iℓ+1)(B2(t1ℓ+1)−B2(t1ℓ))−2G2(t1ℓ,˜Iℓ)(B2(t1ℓ+1)−B2(t1ℓ))2h+G2(t1ℓ−1,˜Iℓ−1)(B2(t1ℓ)−B2(t1ℓ−1))2h}(2hϖ+2((ℓ−ϖ2)(ℓ+1)ϖ+1−ℓϖ+1(ℓ+1+ϖ2))ϖ(ϖ+1)(ϖ+2))] |
+δϖM(δ)[{{˜I∗(t1m+1,˜Hqm+1,˜Iqm+1,˜Vqm+1,˜Dqm+1)+G2(t1m+1,˜Iqm+1)(B2(t1m+1)−B2(t1m))}hϖϖ((m+1)ϖ−mϖ)+˜I∗(t1m+1,˜Hm+1,˜Im+1,˜Vm+1,˜Dm+1)−˜I∗(t1m,˜Hm,˜Im,˜Vm,dm)h+{G2(t1m+1,˜Im+1)(B2(t1m+1)−B2(t1m))−G2(t1m,˜Im)(B2(t1m)−B2(t1m−1))}×(hϖ+1(ℓϖ(ℓ+1+ϖ)−(ℓ+1)ϖ+1)ϖ(ϖ+1))+˜I∗(t1m+1,˜Hm+1,˜Im+1,˜Vm+1,˜Dm+1)−2˜I∗(t1m,˜Hm,˜Im,˜Vm,˜Dm)+˜I∗(t1m−1,˜Hm−1,˜Im−1,˜Vm−1,˜Dm−1)2h2+{G2(t1m+1,˜Im+1)(B2(t1m+1)−B2(t1m))−2G2(t1m,˜Im)(B2(t1m)−B2(t1m−1))2h+G2(t1m−1,˜Im−1)(B2(t1m−1)−B2(t1m−2))2h}(2hϖ+2((ℓ−ϖ2)(ℓ+1)ϖ+1−ℓϖ+1(ℓ+1+ϖ2))ϖ(ϖ+1)(ϖ+2))], |
˜Vm+1=˜V0+(1−δ)δϖM(δ)ϖt1ϖ−1m+1[{˜V∗(t1m+1,˜Iqm+1,˜Vqm+1)+σ3G3(t1m+1,˜Vqm+1)(B3(t1m+1)−B3(t1m))]+δϖM(δ)m−1∑ℓ=0[{˜V∗(t1ℓ+1,˜Iℓ+1,˜Vℓ+1)hϖϖ((ℓ+1)ϖ−ℓϖ)+˜V∗(t1ℓ+1,˜Iℓ+1,˜Vℓ+1)−˜V∗(t1ℓ,˜Iℓ,˜Vℓ)h×(hϖ+1(ℓϖ(ℓ+1+ϖ)−(ℓ+1)ϖ+1)ϖ(ϖ+1))+˜V∗(t1ℓ+1,˜Iℓ+1,˜Vℓ+1)−2˜V∗(t1ℓ,˜Iℓ,˜Vℓ)+˜V∗(t1ℓ−1,˜Iℓ−1,˜Vℓ−1)2h2×(2hϖ+2((ℓ−ϖ2)(ℓ+1)ϖ+1−ℓϖ+1(ℓ+1+ϖ2))ϖ(ϖ+1)(ϖ+2))]+σ1δϖM(δ)m−1∑ℓ=0[{G3(t1ℓ+1,˜Vℓ+1)(B3(t1ℓ+1)−B3(t1ℓ))hϖϖ((ℓ+1)ϖ−ℓϖ)+{G3(t1ℓ+1,˜Vℓ+1)(B1(t1ℓ+1)−B3(t1ℓ))−G3(t1ℓ,˜Vℓ)(B3(t1ℓ)−B3(t1ℓ−1))}×(hϖ+1(ℓϖ(ℓ+1+ϖ)−(ℓ+1)ϖ+1)ϖ(ϖ+1))+{G3(t1ℓ+1,˜Vℓ+1)(B3(t1ℓ+1)−B3(t1ℓ))−2G3(t1ℓ,˜Vℓ)(B3(t1ℓ+1)−B3(t1ℓ))2h+G3(t1ℓ−1,˜Vℓ−1)(B3(t1ℓ)−B3(t1ℓ−1))2h}(2hϖ+2((ℓ−ϖ2)(ℓ+1)ϖ+1−ℓϖ+1(ℓ+1+ϖ2))ϖ(ϖ+1)(ϖ+2))] |
+δϖM(δ)[{{˜V∗(t1m+1,˜Iqm+1,˜Vqm+1)+G3(t1m+1,˜Vqm+1)(B3(t1m+1)−B3(t1m))}hϖϖ((m+1)ϖ−mϖ)+˜V∗(t1m+1,˜Im+1,˜Vm+1)−˜V∗(t1m,˜Im,˜Vm)h+{G3(t1m+1,˜Vm+1)(B3(t1m+1)−B3(t1m))−G3(t1m,˜Vm)(B3(t1m)−B3(t1m−1))}×(hϖ+1(ℓϖ(ℓ+1+ϖ)−(ℓ+1)ϖ+1)ϖ(ϖ+1))+˜V∗(t1m+1,˜Im+1,˜Vm+1)−2˜V∗(t1m,˜Im,˜Vm)+˜V∗(t1m−1,˜Im−1,˜Vm−1)2h2+{G3(t1m+1,˜Vm+1)(B3(t1m+1)−B3(t1m))−2G3(t1m,˜Vm)(B3(t1m)−B3(t1m−1))2h+G3(t1m−1,˜Vm−1)(B3(t1m−1)−B3(t1m−2))2h}(2hϖ+2((ℓ−ϖ2)(ℓ+1)ϖ+1−ℓϖ+1(ℓ+1+ϖ2))ϖ(ϖ+1)(ϖ+2))], |
˜Dm+1=˜D0+(1−δ)δϖM(δ)ϖt1ϖ−1m+1[{˜D∗(t1m+1,˜Iqm+1,˜Dqm+1)+σ4G4(t1m+1,˜Dqm+1)(B4(t1m+1)−B4(t1m))]+δϖM(δ)m−1∑ℓ=0[{˜D∗(t1ℓ+1,˜Iℓ+1,˜Dℓ+1)hϖϖ((ℓ+1)ϖ−ℓϖ)+˜D∗(t1ℓ+1,˜Iℓ+1,˜Dℓ+1)−˜D∗(t1ℓ,˜Iℓ,˜Dℓ)h×(hϖ+1(ℓϖ(ℓ+1+ϖ)−(ℓ+1)ϖ+1)ϖ(ϖ+1))+˜D∗(t1ℓ+1,˜Iℓ+1,˜Dℓ+1)−2˜D∗(t1ℓ,˜Iℓ,˜Dℓ)+˜D∗(t1ℓ−1,˜Iℓ−1,˜Dℓ−1)2h2×(2hϖ+2((ℓ−ϖ2)(ℓ+1)ϖ+1−ℓϖ+1(ℓ+1+ϖ2))ϖ(ϖ+1)(ϖ+2))]+σ4δϖM(δ)m−1∑ℓ=0[{G4(t1ℓ+1,˜Dℓ+1)(B4(t1ℓ+1)−B4(t1ℓ))hϖϖ((ℓ+1)ϖ−ℓϖ)+{G4(t1ℓ+1,˜Dℓ+1)(B4(t1ℓ+1)−B4(t1ℓ))−G4(t1ℓ,˜Dℓ)(B4(t1ℓ)−B4(t1ℓ−1))}×(hϖ+1(ℓϖ(ℓ+1+ϖ)−(ℓ+1)ϖ+1)ϖ(ϖ+1))+{G4(t1ℓ+1,˜Dℓ+1)(B4(t1ℓ+1)−B4(t1ℓ))−2G4(t1ℓ,˜Dℓ)(B4(t1ℓ+1)−B4(t1ℓ))2h+G4(t1ℓ−1,˜Dℓ−1)(B4(t1ℓ)−B4(t1ℓ−1))2h}(2hϖ+2((ℓ−ϖ2)(ℓ+1)ϖ+1−ℓϖ+1(ℓ+1+ϖ2))ϖ(ϖ+1)(ϖ+2))] |
+δϖM(δ)[{{˜D∗(t1m+1,˜Iqm+1,˜Dqm+1)+G4(t1m+1,˜Dqm+1)(B4(t1m+1)−B4(t1m))}hϖϖ((m+1)ϖ−mϖ)+˜D∗(t1m+1,˜Im+1,˜Dm+1)−˜D∗(t1m,˜Im,˜Dm)h+{G4(t1m+1,˜Dm+1)(B4(t1m+1)−B4(t1m))−G4(t1m,˜Dm)(B4(t1m)−B4(t1m−1))}×(hϖ+1(ℓϖ(ℓ+1+ϖ)−(ℓ+1)ϖ+1)ϖ(ϖ+1))+˜D∗(t1m+1,˜Im+1,˜Dm+1)−2˜D∗(t1m,˜Im,˜Dm)+˜D∗(t1m−1,˜Im−1,˜Dm−1)2h2+{G4(t1m+1,˜Dm+1)(B4(t1m+1)−B4(t1m))−2G4(t1m,˜Dm)(B4(t1m)−B4(t1m−1))2h+G4(t1m−1,˜Dm−1)(B4(t1m−1)−B4(t1m−2))2h}(2hϖ+2((ℓ−ϖ2)(ℓ+1)ϖ+1−ℓϖ+1(ℓ+1+ϖ2))ϖ(ϖ+1)(ϖ+2))], |
where
˜Hqm+1=˜H0+(1−δ)δϖM(δ)t1ϖ−1m+1[{˜H∗(t1m,˜Hm,˜Im,˜Vm,˜Dm)+ϖσ1G1(t1m+1,˜Hm+1)(B1(t1m+1)−B1(t1m))]+δϖM(δ)m−1∑ℓ=0[{˜H∗(t1m,˜Hm,˜Im,˜Vm,˜Dm)hϖϖ((ℓ+1)ϖ−ℓϖ)+σ1G1(t1m,˜Hm)(B1(t1m+1)−B1(t1m))hϖϖ((ℓ+1)ϖ−ℓϖ)], |
˜Iqm+1=˜I0+(1−δ)δϖM(δ)t1ϖ−1m+1[{˜I∗(t1m,˜Hm,˜Im,˜Vm,˜Dm)+ϖσ2G2(t1m+1,˜Im+1)(B2(t1m+1)−B2(t1m))]+δϖM(δ)m−1∑ℓ=0[{˜I∗(t1m,˜Hm,˜Im,˜Vm,˜Dm)hϖϖ((ℓ+1)ϖ−ℓϖ)+σ2G2(t1m,˜Im)(B2(t1m+1)−B2(t1m))hϖϖ((ℓ+1)ϖ−ℓϖ)], |
˜Vqm+1=˜V0+(1−δ)δϖM(δ)t1ϖ−1m+1[{˜V∗(t1m,˜Im,˜Vm)+ϖσ3G3(t1m+1,˜Vm+1)(B3(t1m+1)−B3(t1m))]+δϖM(δ)m−1∑ℓ=0[{˜V∗(t1m,˜Im,˜Vm)hϖϖ((ℓ+1)ϖ−ℓϖ)+σ3G3(t1m,˜Vm)(B3(t1m+1)−B3(t1m))hϖϖ((ℓ+1)ϖ−ℓϖ)], |
˜Dqm+1=˜D0+(1−δ)δϖM(δ)t1ϖ−1m+1[{˜D∗(t1m,˜Im,˜Dm)+ϖσ4G4(t1m+1,˜Dm+1)(B4(t1m+1)−B4(t1m))]+δϖM(δ)m−1∑ℓ=0[{˜D∗(t1m,˜Im,˜Dm)hϖϖ((ℓ+1)ϖ−ℓϖ)+σ4G4(t1m,˜Dm)(B4(t1m+1)−B4(t1m))hϖϖ((ℓ+1)ϖ−ℓϖ)]. |
To illustrate the aforementioned mathematical findings, we will provide a few simulation studies in this part. We find the system's stochastic F-F derivative in the Caputo-Fabrizio context using a revolutionary numerical approach introduced in [41]. An evolutionary algorithm was developed to numerically predict outcomes since state formulas possess ICs. Table 2 is an overview of the primary attribute settings. The outcomes with no controls, just vaccine restrictions, just therapy regulation, and both vaccination and medication regulations are compared.
Symbols | Values | References | Units |
λ | 6 | [18] | cell ml−1day−1 |
ϑ | 0.2 | [9] | cell ml−1day−1 |
ϕ1 | 0.01 | [55] | day−1 |
ϕ2 | 0.1 | [51] | day−1 |
ϕ3 | 0.1 | [18] | day−1 |
ϕ4 | 0.3 | [9] | day−1 |
γ1 | 0.01 | [57] | virions−1day−1 |
γ2 | 0.1 | [18] | cell−1day−1 |
γ3 | 0.2 | [56] | cell−1day−1 |
γ4 | 0.015 | [18] | cell−1day−1 |
ω | 0.4 | [56] | cell−1day−1 |
Figures 4–5 presents the dynamics of the F-F HBV model (5.1) with attributed values of Table 2 involving TDs ζ1=1,ζ2=2, assuming σ1=0.003,σ2=0.004,σ3=0.006 and σ4=0.004. Straightforward computations result in Rs0<1, satisfying the requirements of Theorem 4.2. Figures 4-5 demonstrate that there are fewer individuals who have been treated by untreated adults and that the proportion of acutely and chronically contaminated youngsters is declining, respectively. Therefore, we observe that controlling the preponderance of individuals who are HBV-positive may significantly lower or reduce the amount of contaminated neonates who are released during the distribution process when fractional-order δ decreasing and fractal-dimension ϖ remains fixed. It is concluded that the F-F HBV model (5.1) generated in this research is perfectly accurate. It is thought that it can highlight several important characteristics that are also true in more simulation designs of HBV infection.
Figures 6–7 define the complexities of the F-F HBV model (5.1) with aforementioned parametric descriptions considering TDs ζ1=1,ζ2=2, assuming σ1=0.003,σ2=0.004,σ3=0.006 and σ4=0.004. This also predicts that, through cytolytic and non-cytolytic processes, CTL cells are essential for the prevention and treatment of HBV infection. Infectious colonies are killed by cytolytic control by manipulating, while noncytolytic regulatory processes "treat" the intracellular pathogens [51]. According to F-F investigations, the infectious equilibrium state is robust and the pace at which pathogens produce free viral infection is low. If a medicine with a profound impact is discovered, the value of the rate of the viral bacteria's item will decrease, and other immunization collaborators should step up their efforts to reduce interaction rates to a meaningful scale when δ is fixed and ϖ declines. The HBV infection can be treated.
Figures 8–9 illustrate the complexities of the F-F HBV model, (5.1) with aforementioned parametric descriptions, considering TDs ζ1=1,ζ2=2, assuming σ1=0.1,σ2=0.2,σ3=0.3 and σ4=0.4. The likelihood that the sickness will disappear is, therefore, one. It has been demonstrated that a white noise setting with a higher intensity may aid in curing the illness more quickly than a simulation sans noise when δ decreases and ϖ increased. Our analysis's clear and fundamental goal is to minimize the harm inflicted by HBV by reducing the population's infection rate while increasing the population's rate of recovery. The authorities have a mission to ensure that people are notified of the proliferation of the HBV.
Figures 10–11 show the intricacies of the F-F HBV model, (5.1) utilizing the parameterized representations stated above and TDs ζ1=1,ζ2=2, assuming σ1=0.1,σ2=0.2,σ3=0.3 and σ4=0.4. The aforementioned configurations 10-11 makes it evident that the basic reproduction ratio Rs0 in framework (3.1) does not incorporate the index of CTL cells, which means that Rs0 is unable to accurately represent the function of immunogenicity. However, formula (5.1) includes the δ and ϖ, which helps illustrate the function of the CTL cells. As a result, the model (5.1) ought to be more feasible.
Figures 12–13 presents the solution for low densities of σκ,κ=1,2,3,4 using the Matlab package. Therefore, if a treatment with a huge influence can be discovered, the HBV infection can be treated provided that the value of ϑ is sufficiently diminished. According to the above mechanism, since many people are uneducated and unaware of viral diseases associated with them, particularly HBV, it indicates that the current regime should encourage good hygiene precautions in the general populace. Both the recycling of syringes on minors in rural areas and inadequate sanitary standards in public health sectors, which are major causes of HBV, should be prohibited. Blood donations must follow a specified protocol and must first be tested and approved by an officially recognized lab.
In a nutshell, the presence of an F-F derivative operator with stochastic disturbance (noise) and intracellular TDs in the framework (3.1) is hypothesized to deliver a better awareness in the presentation of the presented data, which has serious repercussions for treating alternatives and targeted therapies.
In the current study, we examined the effects of high-order stochastic disturbances on the complexities of the delay differential framework of HBV disease, which includes internalized latency, immunotherapy, disease-to-organ and their transmissions via the F-F derivative within the exponential decay kernel. We also presented the non-negative solution pertaining to the unit probability, stochastic reproduction number, and local stability, which is calculated for the steady states. Under certain assumptions, the sickness may eventually go away under certain circumstances, with probability 1. A revolutionary approach is used in a handful of simulated findings to demonstrate the viability of the outcomes. In the therapy of HBV and other viral disorders, the volume of Gaussian white noise is crucial. The presence of random disturbances (noise) and biochemical TDs in the framework is predicated to provide a greater understanding of the quantitative results, which has significant consequences for antibiotic compounds and regenerative medicine. Several additional intriguing subjects need to be looked into more thoroughly. Other types of environmental noise, including Lévy noise, may be taken into consideration [52]. Additionally, the deterministic system can be expanded to incorporate fractional derivatives in the framework to be able to take into account long-run memory of the bacteria's behavior, which is suggested by the research in [53,54].
All sources of funding of the study must be disclosed
The authors declare there is no conflict of interest.
[1] | Simon AK, Hollander GA, McMichael A (2015) Evolution of the immune system in humans from infancy to old age. P Roy Soc B-Biol Sci 282: 20143085. https://doi.org/10.1098/rspb.2014.3085 |
[2] |
Nikolich-Žugich J (2018) The twilight of immunity: emerging concepts in aging of the immune system. Nat Immunol 19: 10-19. https://doi.org/10.1038/s41590-017-0006-x ![]() |
[3] |
Weiskopf D, Weinberger B, Grubeck-Loebenstein B (2009) The aging of the immune system. Transplant Int 22: 1041-1050. https://doi.org/10.1111/j.1432-2277.2009.00927.x ![]() |
[4] |
Weyand CM, Goronzy JJ (2016) Aging of the immune system. Mechanisms and therapeutic targets. Ann Am Thorac Soc 13: S422-S428. https://doi.org/10.1513/AnnalsATS.201602-095AW ![]() |
[5] |
Vasto S, Caruso C (2004) Immunity & ageing: a new journal looking at ageing from an immunological point of view. Immun Ageing 1: 1-4. https://doi.org/10.1186/1742-4933-1-1 ![]() |
[6] |
Shaw AC, Joshi S, Greenwood H, et al. (2010) Aging of the innate immune system. Curr Opin Immunol 22: 507-513. https://doi.org/10.1016/j.coi.2010.05.003 ![]() |
[7] | Hossain Z, Reza AHMM, Qasem WA, et al. (2022) Development of the immune system in the human embryo. Pediatr Res 2022: 1-5. https://doi.org/10.1038/s41390-022-01940-0 |
[8] |
Márquez EJ, Chung CH, Marches R, et al. (2020) Sexual-dimorphism in human immune system aging. Nat Commun 11: 751. https://doi.org/10.1038/s41467-020-14396-9 ![]() |
[9] |
Rosenthal KS (2018) Immune monitoring of the body's borders. AIMS Allergy Immunol 2: 148-164. https://doi.org/10.3934/Allergy.2018.3.148 ![]() |
[10] |
Rosenthal KS (2017) Dealing with garbage is the immune system's main job. MOJ Immunol 5: 00174. https://doi.org/10.15406/moji.2017.05.00174 ![]() |
[11] |
Melville JM, Moss TJM (2013) The immune consequences of preterm birth. Front Neurosci 7: 79. https://doi.org/10.3389/fnins.2013.00079 ![]() |
[12] |
Herzenberg LA, Tung JW (2006) B cell lineages: documented at last!. Nat Immunol 7: 225-226. https://doi.org/10.1038/ni0306-225 ![]() |
[13] |
Hornef MW, Torow N (2020) ‘Layered immunity’ and the ‘neonatal window of opportunity’—timed succession of non-redundant phases to establish mucosal host–microbial homeostasis after birth. Immunology 159: 15-25. https://doi.org/10.1111/imm.13149 ![]() |
[14] |
Filias A, Theodorou GL, Mouzopoulou S, et al. (2011) Phagocytic ability of neutrophils and monocytes in neonates. BMC Pediatr 11: 29. https://doi.org/10.1186/1471-2431-11-29 ![]() |
[15] |
Hebel K, Weinert S, Kuropka B, et al. (2014) CD4+ T cells from human neonates and infants are poised spontaneously to run a nonclassical IL-4 program. J Immunol 192: 5160-5170. https://doi.org/10.4049/jimmunol.1302539 ![]() |
[16] |
Leeansyah E, Loh L, Nixon DF, et al. (2014) Acquisition of innate-like microbial reactivity in mucosal tissues during human fetal MAIT-cell development. Nat Commun 5: 3143. https://doi.org/10.1038/ncomms4143 ![]() |
[17] |
Silva-Santos B, Schamel WW, Fisch P, et al. (2012) γδ T-cell conference 2012: close encounters for the fifth time. Eur J Immunol 42: 3101-3105. https://doi.org/10.1002/eji.201270101 ![]() |
[18] |
Gibbons D, Fleming P, Virasami A, et al. (2014) Interleukin-8 (CXCL8) production is a signatory T cell effector function of human newborn infants. Nat Med 20: 1206-1210. https://doi.org/10.1038/nm.3670 ![]() |
[19] |
Gibbons DL, Haque SF, Silberzahn T, et al. (2009) Neonates harbor highly active γδ T cells with selective impairments in preterm infants. Eur J Immunol 39: 1794-1806. https://doi.org/10.1002/eji.200939222 ![]() |
[20] |
Pou C, Nkulikiyimfura D, Henckel E, et al. (2019) The repertoire of maternal anti-viral antibodies in human newborns. Nat Med 25: 591-596. https://doi.org/10.1038/s41591-019-0392-8 ![]() |
[21] |
Sarvas H, Seppälä I, Kurikka S, et al. (1993) Half-life of the maternal IgG1 allotype in infants. J Clin Immunol 13: 145-151. https://doi.org/10.1007/BF00919271 ![]() |
[22] |
Fouda GG, Martinez DR, Swamy GK, et al. (2018) The Impact of IgG transplacental transfer on early life immunity. Immunohorizons 2: 14-25. https://doi.org/10.4049/immunohorizons.1700057 ![]() |
[23] |
Katherine Z, Sanidad KZ, Amir M, et al. (2022) Maternal gut microbiome-induced IgG regulates neonatal gut microbiome and immunity. Sci Immunol 7: eabh3816. https://doi.org/10.1126/sciimmunol.abh3816 ![]() |
[24] |
Kalliomäki M, Ouwehand A, Arvilommi H, et al. (1999) Transforming growth factor-β in breast milk: a potential regulator of atopic disease at an early age. J Allergy Clin Immunol 104: 1251-1257. https://doi.org/10.1016/S0091-6749(99)70021-7 ![]() |
[25] |
Brodin P (2022) Immune-microbe interactions early in life: A determinant of health and disease long term. Science 376: 945-950. https://doi.org/10.1126/science.abk2189 ![]() |
[26] |
Olin A, Henckel E, Chen Y, et al. (2018) Stereotypic immune system development in newborn children. Cell 174: 1277-1292. https://doi.org/10.1016/j.cell.2018.06.045 ![]() |
[27] |
Johnson JL, Jones MB, Cobb BA (2015) Polysaccharide A from the capsule of Bacteroides fragilis induces clonal CD4+ T cell expansion. J Biol Chem 290: 5007-5014. https://doi.org/10.1074/jbc.M114.621771 ![]() |
[28] |
Kelchtermans H, Billiau A, Matthys P (2008) How interferon-γ keeps autoimmune diseases in check. Trends Immunol 29: 479-486. https://doi.org/10.1016/j.it.2008.07.002 ![]() |
[29] |
Stiemsma LT, Reynolds LA, Turvey SE, et al. (2015) The hygiene hypothesis: current perspectives and future therapies. Immunotargets Ther 4: 143-157. https://doi.org/10.2147/ITT.S61528 ![]() |
[30] |
Okada H, Kuhn C, Feillet H, et al. (2010) The ‘hygiene hypothesis’ for autoimmune and allergic diseases: an update. Clin Exp Immunol 160: 1-9. https://doi.org/10.1111/j.1365-2249.2010.04139.x ![]() |
[31] |
Hill DA, Spergel JM (2018) The atopic march: Critical evidence and clinical relevance. Ann Allerg Asthma Im 120: 131-137. https://doi.org/10.1016/j.anai.2017.10.037 ![]() |
[32] |
Neu N, Duchon J, Zachariah P (2015) TORCH Infections. Clin Perinatol 42: 77-103. https://doi.org/10.1016/j.clp.2014.11.001 ![]() |
[33] |
Menzies D (2000) What does tuberculin reactivity after bacille Calmette–Guérin vaccination tell us?. Clin Infect Dis 31: S71-S74. https://doi.org/10.1086/314075 ![]() |
[34] |
Sherwood ER, Burelbach KR, McBride MA, et al. (2022) Innate immune memory and the host response to infection. J Immunol 208: 785-792. https://doi.org/10.4049/jimmunol.2101058 ![]() |
[35] |
Loske J, Röhmel J, Lukassen S, et al. (2022) Pre-activated antiviral innate immunity in the upper airways controls early SARS-CoV-2 infection in children. Nat Biotechnol 40: 319-324. https://doi.org/10.1038/s41587-021-01037-9 ![]() |
[36] |
Azzi T, Lünemann A, Murer A, et al. (2014) Role for early-differentiated natural killer cells in infectious mononucleosis. Blood 124: 2533-2543. https://doi.org/10.1182/blood-2014-01-553024 ![]() |
[37] |
Takahashi T, Iwasaki A (2021) Sex differences in immune responses. Science 371: 347-348. https://doi.org/10.1126/science.abe7199 ![]() |
[38] |
Klein SL, Flanagan KL (2016) Sex differences in immune responses. Nat Rev Immunol 16: 626-638. https://doi.org/10.1038/nri.2016.90 ![]() |
[39] |
Klein SL, Jedlicka A, Pekosz A (2010) The Xs and Y of immune responses to viral vaccines. Lancet Infect Dis 10: 338-349. https://doi.org/10.1016/S1473-3099(10)70049-9 ![]() |
[40] |
Pinheiro I, Dejager L, Libert C (2011) X-chromosome-located microRNAs in immunity: Might they explain male/female differences?. Bioessays 33: 791-802. https://doi.org/10.1002/bies.201100047 ![]() |
[41] |
Griesbeck M, Ziegler S, Laffont S, et al. (2015) Sex differences in plasmacytoid dendritic cell levels of IRF5 drive higher IFN-α production in women. J Immunol 195: 5327-5336. https://doi.org/10.4049/jimmunol.1501684 ![]() |
[42] |
Haitao T, Vermunt JV, Abeykoon J, et al. (2020) COVID-19 and sex differences: mechanisms and biomarkers. Mayo Clin Proc 95: 2189-2203. https://doi.org/10.1016/j.mayocp.2020.07.024 ![]() |
[43] |
Asano T, Boisson B, Onodi F, et al. (2021) X-linked recessive TLR7 deficiency in ~1% of men under 60 years old with life-threatening COVID-19. Sci Immunol 6: eabl4348. https://doi.org/10.1126/sciimmunol.abl4348 ![]() |
[44] |
Aomatsu M, Kato T, Kasahara E, et al. (2013) Gender difference in tumor necrosis factor-α production in human neutrophils stimulated by lipopolysaccharide and interferon-γ. Biochem Bioph Res Co 441: 220-225. https://doi.org/10.1016/j.bbrc.2013.10.042 ![]() |
[45] |
Brunsing RL, Owens KS, Prossnitz ER (2013) The G protein-coupled estrogen receptor (GPER) agonist G-1 expands the regulatory T-cell population under TH17-polarizing conditions. J Immunother 36: 190-196. https://doi.org/10.1097/CJI.0b013e31828d8e3b ![]() |
[46] |
Jaillon S, Berthenet K, Garlanda C (2019) Sexual dimorphism in innate immunity. Clin Rev Allerg Immu 56: 308-321. https://doi.org/10.1007/s12016-017-8648-x ![]() |
[47] |
Arruvito L, Sanz M, Banham AH, et al. (2007) Expansion of CD4+CD25+ and FOXP3+ regulatory T cells during the follicular phase of the menstrual cycle: implications for human reproduction. J Immunol 178: 2572-2578. https://doi.org/10.4049/jimmunol.178.4.2572 ![]() |
[48] |
Mor G, Aldo P, Alvero A (2017) The unique immunological and microbial aspects of pregnancy. Nat Rev Immunol 17: 469-482. https://doi.org/10.1038/nri.2017.64 ![]() |
[49] |
Krop J, Heidt S, Claas FHJ, et al. (2020) Regulatory T cells in pregnancy: it is not all about FoxP3. Front Immunol 11: 1182. https://doi.org/10.3389/fimmu.2020.01182 ![]() |
[50] |
Hanna J, Goldman-Wohl D, Hamani Y, et al. (2006) Decidual NK cells regulate key developmental processes at the human fetal-maternal interface. Nat Med 12: 1065-1074. https://doi.org/10.1038/nm1452 ![]() |
[51] |
Le Bouteiller P (2013) Human decidual NK cells: unique and tightly regulated effector functions in healthy and pathogen-infected pregnancies. Front Immunol 4: 404. https://doi.org/10.3389/fimmu.2013.00404 ![]() |
[52] |
Sojka DK, Yang L, Yokoyama WM (2019) Uterine natural killer cells. Front Immunol 10: 960. https://doi.org/10.3389/fimmu.2019.00960 ![]() |
[53] |
Yang SL, Wang HY, Li DJ, et al. (2019) Role of decidual natural killer cells at the maternal–fetal interface during pregnancy. Reprod Dev Med 3: 165-169. https://doi.org/10.4103/2096-2924.268161 ![]() |
[54] |
Piccinni MP, Lombardelli L, Logiodice F, et al. (2016) How pregnancy can affect autoimmune diseases progression?. Clin Mol Allergy 14: 11. https://doi.org/10.1186/s12948-016-0048-x ![]() |
[55] |
Bupp MRG, Jorgensen TN (2018) Androgen-induced immunosuppression. Front Immunol 9: 794. https://doi.org/10.3389/fimmu.2018.00794 ![]() |
[56] |
Trigunaite A, Dimo J, Jørgensen TN (2015) Suppressive effects of androgens on the immune system. Cellular Immunol 294: 87-94. https://doi.org/10.1016/j.cellimm.2015.02.004 ![]() |
[57] |
Angele MK, Pratschke S, Hubbard WJ, et al. (2014) Gender differences in sepsis. Virulence 5: 12-19. https://doi.org/10.4161/viru.26982 ![]() |
[58] |
Meier A, Chang J, Chan E, et al. (2009) Sex differences in the Toll-like receptor-mediated response of plasmacytoid dendritic cells to HIV-1. Nat Med 15: 955-959. https://doi.org/10.1038/nm.2004 ![]() |
[59] |
Meester I, Manilla-Muñoz E, León-Cachón, RBR, et al. (2020) SeXY chromosomes and the immune system: reflections after a comparative study. Biol Sex Differ 11: 1-13. https://doi.org/10.1186/s13293-019-0278-y ![]() |
[60] |
Russi AE, Walker-Caulfield ME, Ebel ME, et al. (2015) Cutting edge: c-Kit signaling differentially regulates type 2 innate lymphoid cell accumulation and susceptibility to central nervous system demyelination in male and female SJL mice. J Immunol 194: 5609-5613. https://doi.org/10.4049/jimmunol.1500068 ![]() |
[61] |
Zhang MA, Rego D, Moshkova M, et al. (2012) Peroxisome proliferator-activated receptor (PPAR)α and -γ regulate IFNγ and IL-17A production by human T cells in a sex-specific way. P Natl Acad Sci USA 109: 9505-9510. https://doi.org/10.1073/pnas.1118458109 ![]() |
[62] |
Tadount F, Doyon-Plourde P, Rafferty E, et al. (2020) Is there a difference in the immune response, efficacy, effectiveness and safety of seasonal influenza vaccine in males and females?—A systematic review. Vaccine 38: 444-459. https://doi.org/10.1016/j.vaccine.2019.10.091 ![]() |
[63] |
Gee J, Marquez P, Su J, et al. (2021) First month of COVID-19 vaccine safety monitoring—United States, December 14, 2020-January 13. MMWR Morb Mortal Wkly Rep 70: 283-288. https://doi.org/10.15585/mmwr.mm7008e3 ![]() |
[64] |
López-Otín C, Blasco MA, Partridge L, et al. (2013) The hallmarks of aging. Cell 153: 1194-1217. https://doi.org/10.1016/j.cell.2013.05.039 ![]() |
[65] |
Cuervo AM, Macian F (2014) Autophagy and the immune function in aging. Curr Opin Immunol 29: 97-104. https://doi.org/10.1016/j.coi.2014.05.006 ![]() |
[66] |
Gustafson CE, Kim C, Weyand CM, et al. (2020) Influence of immune aging on vaccine responses. J Allergy Clin Immunol 145: 1309-1321. https://doi.org/10.1016/j.jaci.2020.03.017 ![]() |
[67] |
Ciabattini A, Nardini C, Santoro F, et al. (2018) Vaccination in the elderly: The challenge of immune changes with aging. Semin Immunol 40: 83-94. https://doi.org/10.1016/j.smim.2018.10.010 ![]() |
[68] | Fülöp T, Foris G, Worum I, et al. (1985) Age-dependent alterations of Fcγ receptor-mediated effector functions of human polymorphonuclear leucocytes. Clin Exp Immunol 61: 425-432. |
[69] |
Butcher S, Chahel H, Lord JM (2000) Ageing and the neutrophil: No appetite for killing?. Immunology 100: 411-416. https://doi.org/10.1046/j.1365-2567.2000.00079.x ![]() |
[70] |
Solana R, Tarazona R, Gayoso I, et al. (2012) Innate immunosenescence: Effect of aging on cells and receptors of the innate immune system in humans. Semin Immunol 24: 331-341. https://doi.org/10.1016/j.smim.2012.04.008 ![]() |
[71] |
Mylotte JM, Naughton B, Saludades C, et al. (1998) Validation and application of the pneumonia prognosis index to nursing home residents with pneumonia. J Am Geriatr Soc 46: 1538-1544. https://doi.org/10.1111/j.1532-5415.1998.tb01539.x ![]() |
[72] |
Hasegawa T, Feng Z, Yan Z, et al. (2020) Reduction in human epidermal Langerhans cells with age is associated with decline in CXCL14-mediated recruitment of CD14+ monocytes. J Invest Dermatol 140: 1327-1334. https://doi.org/10.1016/j.jid.2019.11.017 ![]() |
[73] |
Zegarska B, Pietkun K, Giemza-Kucharska P, et al. (2017) Changes of Langerhans cells during skin ageing. Postepy Dermatol Alergol 34: 260-267. https://doi.org/10.5114/ada.2017.67849 ![]() |
[74] |
Salimi S, Hamlyn JM (2020) COVID-19 and crosstalk with the hallmarks of aging. J Gerontol A-Biol 75: e34-e41. https://doi.org/10.1093/gerona/glaa149 ![]() |
[75] |
Becklund B, Purton J, Ramsey C, et al. (2016) The aged lymphoid tissue environment fails to support naïve T cell homeostasis. Sci Rep 6: 30842. https://doi.org/10.1038/srep30842 ![]() |
[76] |
Thompson HL, Smithey MJ, Surh CD, et al. (2017) Functional and homeostatic impact of age-related changes in lymph node stroma. Front Immunol 8: 706. https://doi.org/10.3389/fimmu.2017.00706 ![]() |
[77] |
Naylor K, Li G, Vallejo AN, et al. (2005) The influence of age on T cell generation and TCR diversity. J Immunol 174: 7446-7452. https://doi.org/10.4049/jimmunol.174.11.7446 ![]() |
[78] |
Goronzy JJ, Lee WW, Weyand CM (2007) Aging and T-cell diversity. Exp Gerontol 42: 400-406. https://doi.org/10.1016/j.exger.2006.11.016 ![]() |
[79] |
Moro-García MA, Alonso-Arias R, López-Vázquez A, et al. (2012) Relationship between functional ability in older people, immune system status, and intensity of response to CMV. Age 34: 479-495. https://doi.org/10.1007/s11357-011-9240-6 ![]() |
[80] |
Pangrazzi L, Weinberger BZ (2020) T cells, aging and senescence. Exp Gerontol 134: 110887. https://doi.org/10.1016/j.exger.2020.110887 ![]() |
[81] |
Solana R, Tarazona R, Aiello AE, et al. (2012) CMV and Immunosenescence: from basics to clinics. Immun Ageing 9: 23. https://doi.org/10.1186/1742-4933-9-23 ![]() |
[82] |
Frasca D, Blomberg BB, Fuldner R, et al. (2018) “Aging and immunity” symposium: Meeting report. Exp Gerontol 105: 1-3. https://doi.org/10.1016/j.exger.2017.12.004 ![]() |
[83] |
Goronzy J, Weyand C (2013) Understanding immunosenescence to improve responses to vaccines. Nat Immunol 14: 428-436. https://doi.org/10.1038/ni.2588 ![]() |
[84] |
Feehan J, Tripodi N, Apostolopoulos V (2021) The twilight of the immune system: The impact of immunosenescence in aging. Maturitas 147: 7-13. https://doi.org/10.1016/j.maturitas.2021.02.006 ![]() |
[85] |
Raynor J, Lages CS, Shehata H, et al. (2012) Homeostasis and function of regulatory T cells in aging. Curr Opin Immunol 24: 482-487. https://doi.org/10.1016/j.coi.2012.04.005 ![]() |
[86] |
Neiman M, Hellström C, Just D, et al. (2019) Individual and stable autoantibody repertoires in healthy individuals. Autoimmunity 52: 1-11. https://doi.org/10.1080/08916934.2019.1581774 ![]() |
[87] |
Khan MWA, Al Otaibi A, Sherwani S, et al. (2020) Glycation and oxidative stress increase autoantibodies in the elderly. Molecules 25: 3675. https://doi.org/10.3390/molecules25163675 ![]() |
[88] |
Barcenas-Morales G, Cortes-Acevedo P, Doffinger R (2019) Anticytokine autoantibodies leading to infection: early recognition, diagnosis and treatment options. Curr Opin Infect Dis 32: 330-336. https://doi.org/10.1097/QCO.0000000000000561 ![]() |
[89] |
Merkel PA, Lebo T, Knight V (2019) Functional analysis of anti-cytokine autoantibodies using flow cytometry. Front Immunol 10: 1517. https://doi.org/10.3389/fimmu.2019.01517 ![]() |
[90] |
Bastard P, Gervais A, Le Voyeret T, et al. (2021) Autoantibodies neutralizing type I IFNs are present in ~4% of uninfected individuals over 70 years old and account for ~20% of COVID-19 deaths. Sci Immunol 6: eabl4340. https://doi.org/10.1126/sciimmunol.abl4340 ![]() |
[91] |
Franceschi C, Salvioli S, Garagnani P, et al. (2017) Immunobiography and the heterogeneity of immune responses in the elderly: a focus on inflammaging and trained immunity. Front Immunol 8: 982. https://doi.org/10.3389/fimmu.2017.00982 ![]() |
[92] | Fulop T, Larbi A, Pawelec G, et al. (2021) Immunology of aging: the birth of inflammaging. Clin Rev Allerg Immu 2021: 1-14. https://doi.org/10.1007/s12016-021-08899-6 |
[93] |
Cai D, Liu T (2012) Inflammatory cause of metabolic syndrome via brain stress and NF-κB. Aging 4: 98-115. https://doi.org/10.18632/aging.100431 ![]() |
[94] |
Reed RG (2019) Stress and immunological aging. Curr Opin Behav Sci 28: 38-43. https://doi.org/10.1016/j.cobeha.2019.01.012 ![]() |
[95] |
Klopack ET, Crimmins EM, Cole SW, et al. (2022) Social stressors associated with age-related T lymphocyte percentages in older US adults: Evidence from the US Health and Retirement Study. P Natl Acad Sci USA 119: e2202780119. https://doi.org/10.1073/pnas.2202780119 ![]() |
[96] |
Gouin JP, Hantsoo L, Kiecolt-Glaser JK (2008) Immune dysregulation and chronic stress among older adults: A review. Neuroimmunomodulat 15: 251-259. https://doi.org/10.1159/000156468 ![]() |
[97] |
Minciullo PL, Catalano A, Mandraffino G, et al. (2015) Inflammaging and anti-inflammaging: the role of cytokines in extreme longevity. Arch Immunol Ther Ex 64: 111-126. https://doi.org/10.1007/s00005-015-0377-3 ![]() |
[98] |
Tajbakhsh A, Farahani N, Gheibihayat SM, et al. (2021) Autoantigen-specific immune tolerance in pathological and physiological cell death: Nanotechnology comes into view. Int Immunopharmacol 90: 107177. https://doi.org/10.1016/j.intimp.2020.107177 ![]() |
[99] |
Giefing-Kröll C, Berger P, Lepperdinger G, et al. (2015) How sex and age affect immune responses, susceptibility to infections, and response to vaccination. Aging Cell 14: 309-321. https://doi.org/10.1111/acel.12326 ![]() |
[100] |
Kauffman CA, Yoshikawa TT (2001) Fungal infections in older adults. Clin Infect Dis 33: 550-555. https://doi.org/10.1086/322685 ![]() |
[101] |
Shea KM, Kammerer JS, Winston CA, et al. (2014) Estimated rate of reactivation of latent tuberculosis infection in the United States, overall and by population subgroup. Am J Epidemiol 179: 216-225. https://doi.org/10.1093/aje/kwt246 ![]() |
[102] |
Nikolich-Zugich J, Knox KS, Rios CT, et al. (2020) SARS-CoV-2 and COVID-19 in older adults: what we may expect regarding pathogenesis, immune responses, and outcomes. Geroscience 42: 505-514. https://doi.org/10.1007/s11357-020-00186-0 ![]() |
[103] |
Boraschi D, Italiani P (2014) Immunosenescence and vaccine failure in the elderly: Strategies for improving response. Immunol Lett 162: 346-353. https://doi.org/10.1016/j.imlet.2014.06.006 ![]() |
[104] |
Kogut I, Scholz JL, Cancro MP, et al. (2012) B cell maintenance and function in aging. Semin Immunol 24: 342-349. https://doi.org/10.1016/j.smim.2012.04.004 ![]() |
[105] |
Franceschi C, Capri M, Monti D, et al. (2007) Inflammaging and anti-inflammaging: A systemic perspective on aging and longevity emerged from studies in humans. Mech Ageing Dev 128: 92-105. https://doi.org/10.1016/j.mad.2006.11.016 ![]() |
1. | Maysaa Al-Qureshi, Saima Rashid, Fahd Jarad, Mohammed Shaaf Alharthi, Dynamical behavior of a stochastic highly pathogenic avian influenza A (HPAI) epidemic model via piecewise fractional differential technique, 2023, 8, 2473-6988, 1737, 10.3934/math.2023089 | |
2. | Abdon Atangana, Saima Rashid, Analysis of a deterministic-stochastic oncolytic M1 model involving immune response via crossover behaviour: ergodic stationary distribution and extinction, 2023, 8, 2473-6988, 3236, 10.3934/math.2023167 | |
3. | Saowaluck Chasreechai, Muhammad Aamir Ali, Surapol Naowarat, Thanin Sitthiwirattham, Kamsing Nonlaopon, On some Simpson's and Newton's type of inequalities in multiplicative calculus with applications, 2023, 8, 2473-6988, 3885, 10.3934/math.2023193 | |
4. | Maysaa Al Qurashi, Saima Rashid, Ahmed M. Alshehri, Fahd Jarad, Farhat Safdar, New numerical dynamics of the fractional monkeypox virus model transmission pertaining to nonsingular kernels, 2022, 20, 1551-0018, 402, 10.3934/mbe.2023019 | |
5. | Farhat Safdar, Muhammad Attique, Extended weighted Simpson-like type inequalities for preinvex functions and their use in physical system, 2022, 1016-2526, 621, 10.52280/pujm.2022.541001 | |
6. | Hanan S. Gafel, Saima Rashid, Sayed K. Elagan, Novel codynamics of the HIV-1/HTLV-Ⅰ model involving humoral immune response and cellular outbreak: A new approach to probability density functions and fractional operators, 2023, 8, 2473-6988, 28246, 10.3934/math.20231446 | |
7. | Fabio Grizzi, Marco Spadaccini, Maurizio Chiriva-Internati, Mohamed A A A Hegazi, Robert S Bresalier, Cesare Hassan, Alessandro Repici, Silvia Carrara, Fractal nature of human gastrointestinal system: Exploring a new era, 2023, 29, 1007-9327, 4036, 10.3748/wjg.v29.i25.4036 | |
8. | XUEJUAN LI, RUI ZHAO, PHYSICS-INFORMED DEEP AI SIMULATION FOR FRACTAL INTEGRO-DIFFERENTIAL EQUATION, 2024, 32, 0218-348X, 10.1142/S0218348X24500221 | |
9. | Saima Rashid, Sher Zaman Hamidi, Saima Akram, Muhammad Aon Raza, S. K. Elagan, Beida Mohsen Tami Alsubei, Theoretical and mathematical codynamics of nonlinear tuberculosis and COVID-19 model pertaining to fractional calculus and probabilistic approach, 2024, 14, 2045-2322, 10.1038/s41598-024-59261-7 | |
10. | Saima Rashid, Sher Zaman Hamidi, Muhammad Aon Raza, Rafia Shafique, Assayel Sultan Alsubaie, Sayed K. Elagan, Robustness and exploration between the interplay of the nonlinear co-dynamics HIV/AIDS and pneumonia model via fractional differential operators and a probabilistic approach, 2024, 14, 2045-2322, 10.1038/s41598-024-65329-1 |
Symbols | Explanation |
λ | Rate of viral hepatocellular development via bone marrow and various tissues |
ϑ | Rate at which CTLs are produced in the thymus |
ϕ1 | Percentage of uninfectious hepatocytes that naturally die |
ϕ2 | Percentage of infectious hepatocytes that naturally die |
ϕ3 | Frequency of harmless pathogens dying |
ϕ4 | Fatality rate of CTLs |
γ1 | Successful viral interaction incidence with healthy hepatocytes |
γ2 | Efficient proportion of interaction between healthy and diseased hepatocytes |
γ3 | CTLs' efficiency at eradicating infectious hepatocytes |
γ4 | CTL development speed as a result of contaminated cells' activation |
ω | Speed of spontaneous viral activity in affected tissues |
Symbols | Values | References | Units |
λ | 6 | [18] | cell ml−1day−1 |
ϑ | 0.2 | [9] | cell ml−1day−1 |
ϕ1 | 0.01 | [55] | day−1 |
ϕ2 | 0.1 | [51] | day−1 |
ϕ3 | 0.1 | [18] | day−1 |
ϕ4 | 0.3 | [9] | day−1 |
γ1 | 0.01 | [57] | virions−1day−1 |
γ2 | 0.1 | [18] | cell−1day−1 |
γ3 | 0.2 | [56] | cell−1day−1 |
γ4 | 0.015 | [18] | cell−1day−1 |
ω | 0.4 | [56] | cell−1day−1 |
Symbols | Explanation |
λ | Rate of viral hepatocellular development via bone marrow and various tissues |
ϑ | Rate at which CTLs are produced in the thymus |
ϕ1 | Percentage of uninfectious hepatocytes that naturally die |
ϕ2 | Percentage of infectious hepatocytes that naturally die |
ϕ3 | Frequency of harmless pathogens dying |
ϕ4 | Fatality rate of CTLs |
γ1 | Successful viral interaction incidence with healthy hepatocytes |
γ2 | Efficient proportion of interaction between healthy and diseased hepatocytes |
γ3 | CTLs' efficiency at eradicating infectious hepatocytes |
γ4 | CTL development speed as a result of contaminated cells' activation |
ω | Speed of spontaneous viral activity in affected tissues |
Symbols | Values | References | Units |
λ | 6 | [18] | cell ml−1day−1 |
ϑ | 0.2 | [9] | cell ml−1day−1 |
ϕ1 | 0.01 | [55] | day−1 |
ϕ2 | 0.1 | [51] | day−1 |
ϕ3 | 0.1 | [18] | day−1 |
ϕ4 | 0.3 | [9] | day−1 |
γ1 | 0.01 | [57] | virions−1day−1 |
γ2 | 0.1 | [18] | cell−1day−1 |
γ3 | 0.2 | [56] | cell−1day−1 |
γ4 | 0.015 | [18] | cell−1day−1 |
ω | 0.4 | [56] | cell−1day−1 |