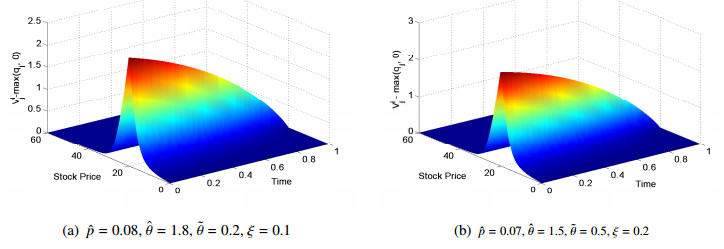
In the case of the KoBoL model with the jump process (KoBoLJ), the pricing problem of American call option is investigated in this paper. The pricing model of this kind of financial derivatives is a free boundary problem with a fractional-partial-integro-differential equation (FPIDE). In fact, it is impossible to obtain the analytical solution of the mathematical model. Hence, the mathematical model with free boundary should be changed as a fixed one and then the numerical scheme is set to solve the transformed model. In the proposed approach, we proved that the American call option values obtained by the current method are not lower than the intrinsic values of this option. Moreover the PCGNR method with the fast Fourier transform (FFT) technique was employed to handle the semi-globalness of the fractional-integro operator. The significant effects of the parameters in our model on the optimal exercise price curve ware analyzed.
Citation: Bing Feng, Congyin Fan. American call option pricing under the KoBoL model with Poisson jumps[J]. Networks and Heterogeneous Media, 2025, 20(1): 143-164. doi: 10.3934/nhm.2025009
[1] | Congyin Fan, Wenting Chen, Bing Feng . Pricing stock loans under the L$ \acute{e} $vy-$ \alpha $-stable process with jumps. Networks and Heterogeneous Media, 2023, 18(1): 191-211. doi: 10.3934/nhm.2023007 |
[2] | Ming-Kai Wang, Cheng Wang, Jun-Feng Yin . A second-order ADI method for pricing options under fractional regime-switching models. Networks and Heterogeneous Media, 2023, 18(2): 647-663. doi: 10.3934/nhm.2023028 |
[3] | Xiongfa Mai, Ciwen Zhu, Libin Liu . An adaptive grid method for a singularly perturbed convection-diffusion equation with a discontinuous convection coefficient. Networks and Heterogeneous Media, 2023, 18(4): 1528-1538. doi: 10.3934/nhm.2023067 |
[4] | Changli Yuan, Mojdeh Delshad, Mary F. Wheeler . Modeling multiphase non-Newtonian polymer flow in IPARS parallel framework. Networks and Heterogeneous Media, 2010, 5(3): 583-602. doi: 10.3934/nhm.2010.5.583 |
[5] | Renata Bunoiu, Claudia Timofte . Homogenization of a thermal problem with flux jump. Networks and Heterogeneous Media, 2016, 11(4): 545-562. doi: 10.3934/nhm.2016009 |
[6] | Min Shen, Gabriel Turinici . Liquidity generated by heterogeneous beliefs and costly estimations. Networks and Heterogeneous Media, 2012, 7(2): 349-361. doi: 10.3934/nhm.2012.7.349 |
[7] | Sergei Yu. Pilyugin, Maria S. Tarasova, Aleksandr S. Tarasov, Grigorii V. Monakov . A model of voting dynamics under bounded confidence with nonstandard norming. Networks and Heterogeneous Media, 2022, 17(6): 917-931. doi: 10.3934/nhm.2022032 |
[8] | Sergei Yu. Pilyugin, M. C. Campi . Opinion formation in voting processes under bounded confidence. Networks and Heterogeneous Media, 2019, 14(3): 617-632. doi: 10.3934/nhm.2019024 |
[9] | Carlo Brugna, Giuseppe Toscani . Boltzmann-type models for price formation in the presence of behavioral aspects. Networks and Heterogeneous Media, 2015, 10(3): 543-557. doi: 10.3934/nhm.2015.10.543 |
[10] | Wen Shen . Traveling waves for conservation laws with nonlocal flux for traffic flow on rough roads. Networks and Heterogeneous Media, 2019, 14(4): 709-732. doi: 10.3934/nhm.2019028 |
In the case of the KoBoL model with the jump process (KoBoLJ), the pricing problem of American call option is investigated in this paper. The pricing model of this kind of financial derivatives is a free boundary problem with a fractional-partial-integro-differential equation (FPIDE). In fact, it is impossible to obtain the analytical solution of the mathematical model. Hence, the mathematical model with free boundary should be changed as a fixed one and then the numerical scheme is set to solve the transformed model. In the proposed approach, we proved that the American call option values obtained by the current method are not lower than the intrinsic values of this option. Moreover the PCGNR method with the fast Fourier transform (FFT) technique was employed to handle the semi-globalness of the fractional-integro operator. The significant effects of the parameters in our model on the optimal exercise price curve ware analyzed.
The American option is an important financial tool and is widely used in real market. However, the pricing model of this financial derivative is a free boundary problem so that it is impossible to obtain the analytic pricing formula of this financial derivative. Thus, in the past two decades, the numerical method becomes a mainstream tool to solve the mathematical model.
More and more authors have studied the American option in the case of Black-Scholes model (BS). For example, Geske and Johson [1] investigated the American put option, and an analytic solution to this option was derived. Moreover, based on the analytical solution, the risk hedging coefficient for American put was obtained. Based on this literature, Zhu et al. [2,3] used the integral transformation method to solve the American Contingent Claims pricing mathematical model, and the price and optimal exercise price of this kind of financial derivatives are obtained. Gyulov and Koleva [4] developed a numerical method based on the penalty for American option in the case of the BS model with the regime-switching process. Xiang and Wang [5] proposed an efficient quasi-Monte Carlo method for estimating American option sensitivities. Wang et al. [6] constructed a high-order deferred correction algorithm combined with penalty iteration for solving American option pricing model. Elettra and Rossella [7] use the Recurrent neural network framework for computing prices and deltas of American options in high dimensions. Under the framework of the Cox-Ingersoll-Ross (CIR), Zhang et al. [8] proposed an efficient numerical method for the American options pricing. Additionally for the perpetual American put option, an analytical solution is proposed under the framework of BS in [9].
However, in the case of the classical BS model, the stock price is assumed to follow the Geometric Brownian motion, which cannot reflect the character of the risk asset in the real market. The conclusions in [10,11,12,13] show that the risk asset price should appear to be "phenomenon of jumps" and "asymmetric distribution". Thus, the more complex stochastic differential equation should be used to capture the characters of the risk asset by many scholars. Prominent examples, including the FMLS equation [14], CGMY equation [15], and KoBoL equation [16]. Moreover, as described in [17], both FMLS and CGMY equations are the special cases of the KoBoL equation, so we consider the American call pricing problem in this paper. Under this framework, the function of the option price value is governed by the fractional partial differential equation free boundary problem, which is proved in [18]. Following this work, Chen and Lin [19] used the integral transformation method to obtain the analytical solution of the European option pricing model. For the European double barrier option pricing model, the numerical method is set, and the convergence rate and stability of this numerical method are proved in [17]. Mohapatra et al. [20] considered the numerical solution for the time fractional Black-Scholes model under jump-diffusion involving a Caputo differential operator, and their schemes are investigated for numerous European option pricing jump-diffusion models. Guo et al. [21] proposed a numerical method for European and American option pricing under the time fractional jump-diffusion model in Caputo scene. Fan et al. [22] considered the values and optimal exercise prices of the American option under the CGMY model with the regime-switching process.
Based on the literature, the American call option pricing problem is investigated in the case of the KoBoL model with the jump process (KoBoLJ) in this paper. Under the framework of the KoBoLJ model, the function of the American call value follows a FPIDE, and the pricing model is a free boundary problem. To obtain a FPIDE boundary value problem over a fixed rectangular domain, a nonlinear penalty term is added to the governing equation. However, it is still impossible to achieve the analytical solution of the new mathematical model. Hence, the finite differential method is essentially considered in this paper. Moreover, a dense coefficient matrix resulted from the fractional derivatives in the final linear system, which requires the computational cost in the order of O(M3), where M is the number of spatial grid nodes. This shows that the computational time of numerical method will increase.
The major contributions of this study can be summarized as follows:
ⅰ) The Poisson jumps is introduced into the KoBoL model due to the need to capture the characters of stock price so that the option pricing models can capture market risk;
ⅱ) The preconditioned conjugate gradient normal residual (PCGNR) method with a Strang's circulant pre-conditioner [23] and the fast Fourier transform (FFT) technique are used, so that the computational cost reduces significantly from O(M3) to O(MlogM);
ⅲ) Based on the numerical scheme, we prove that the American call option value generated by the penalty method cannot fall below the value obtained when the American call option is exercised early, i.e., V(x,t)≥max(ex−K,0).
The rest of this article is outlined below. In the next section, the pricing mathematical model of American call under the framework of the KoBoLJ model is derived in detail. In Section 3, the numerical scheme is proposed, and we prove that the American call option value obtained by our numerical method is bigger than the exercising value. In Section 4, we prove the PCGNR method with a circulant pre-conditioner and the FFT technique to calculate the final system. Moreover, the numerical experiments are presented with some discussions in Section 5. We conclude this paper in the last section.
Take (Ω,Ft,P) as a filtered probability, where t∈[0,T]. The KoBoL model with the jump process is defined on this probability space. Following the assumptions in [24], under this model, the logarithmic price of the underlying, i.e., xt=ln(St), satisfies the following stochastic differential equation
dxt=(r−ν−D−ξς)dt+dLKoBoLt+d(Nt∑i=1Yi), | (2.1) |
with solution
ST=Ste(r−v)(T−t)+∫TtdLKoBoLu, |
where xt is the logarithmic form stock price xt=lnSt, r is the risk-free interest rate, D is dividend, dLKoBoLt is the increment of a Lˊevy process under the equivalent martingale measure, and ν=12σα[p(λ−1)α+q(λ+1)α−λα−αλα−1(q−p)] is convexity adjustment so that the expectation of ST becomes E[ST]=er(T−t)St. Parameter α∈(1,2) determines whether the KoBoLJ stochastic process has finite or infinite variation. The relative frequency and overall upwind and downwind movements KoBoLJ stochastic process are controlled by q>0,p>0(p+q=1). The decay rate of tails of our stochastic process probability density function is controlled by parameter λ>0. Nt is a Poisson process and it is characterized by the jump intensity ξ≥0. {Yi,i=1,2,…} is a sequence of independent and identically distributed hyper-exponential random variables with probability density function
fY(y)=m1∑i=1ˆpiˆθie−ˆθiy1{y≥0}+m2∑j=1˜pj˜θje−˜θjy1{y≤0}. |
Note that ˆpi (i=1,2,...,m1) and ˜pj (j=1,2,...,m2) denote the probabilities of the ith positive and negative jumps, respectively. They satisfy ∑m1i=1^pi+∑m2j=1~pj=1. ˆθi>1 (i=1,...,m1) is the magnitude of the upward jumps and ˜θj>0 (j=1,...,m2) is that of the downward random jumps. The average jump size is given by
ς=EP[exp(Y1)−1]=m1∑i=1ˆpiˆθiˆθi−1+m2∑j=1˜pj˜θj˜θj+1−1, | (2.2) |
where EP is the expectation operator under probability measure P.
Now, we turn to formulate mathematically the pricing of the American option under our model. Financially, the payoff function of American call contract can be written as
Π(xT,T)=max(ex−K,0), | (2.3) |
where K is the strike price. According to the no-arbitrage pricing principle, one can obtain
V(x,t)=e−r(T−t)EP[Π(xT,T)]. | (2.4) |
Then, according to the conclusions in [18], it can be obtained that the American call option value V(x,t) satisfies the following equation
∂V(x,t)∂t+a∂V(x,t)∂x+ξ∫+∞−∞V(x+y,t)fY(y)dy+12σα[peλxxDαxfe−λxV(x,t)+qe−λx−∞DαxeλxV(x,t)]=(b+12σαλα)V(x,t), | (2.5) |
where x∈(−∞,xf],t∈[0,T], a=r−ν−D−ξς−λα−1(q−p),b=r+ξ, and
eλxxDαxfe−λxV(x,t)=eλxΓ(2−α)∂2∂x2∫xfxe−λξV(ξ,t)(ξ−x)α−1dξ, |
e−λx−∞DαxeλxV(x,t)=e−λxΓ(2−α)∂2∂x2∫x−∞eλξV(ξ,t)(x−ξ)α−1dξ. |
In fact, the fractional derivative in Eq (2.5) is closely related to the KoBoLJ model. Moreover, the fractional derivatives in the governing Eq (2.5) are non-local in order to describe the American call option value in the holding region (−∞,xf].
In this paper, we take American call as the research object, so the function V(x,t) satisfies the following boundary conditions:
{limx→−∞V(x,t)=0,V(xf,t)=exf−K,∂V(xf,t)∂x=Sf=ef,V(x,T)=max(ex−K,0). | (2.6) |
To sum up, a complete pricing mathematical model of American call under the KoBoLJ process can be obtained as Eqs (2.5) and (2.6). Moreover, we remark that the above FPIDE system is much more difficult to solve than the corresponding BS case with jumps, with the main difficulty resulting from the free boundary and the non-localness of the fractional-integro differential operator. In the following section, a new numerical scheme is proposed to solve it efficiently.
According to the unique characteristics of the American call option, the value function V(x,t) of this financial derivative should satisfy the following inequality constraint
V(x,t)≥max(ex−K,0), | (2.7) |
for all t∈[0,T] and x≤xf.
There are two parts in this section. In the first part, the free boundary problem should be changed as one defined on a fixed interval by introducing a nonlinear penalty term. Both the difference scheme and theoretical analysis are displayed in the second part.
Let 0<ϵ≪1 and C>0 be a fixed constant, and we will determine its specific value. We construct the following nonlinear penalty term
εCVε(x,t)+ε−Q(x),andQ(x)=ex−K. | (3.1) |
Then we add it to Eq (2.5)and obtain the following system,
∂Vε(x,t)∂t+a∂Vϵ(x,t)∂x+12σα[peλxxDαxmaxe−λxVε(x,t)+qe−λx−∞DαxeλxVε(x,t)]+ϵCVε(x,t)+ϵ−Q(x)+ξ∫+∞−∞V(x+y,t)fY(y)dy=(b+12σαλα)Vε(x,t), | (3.2) |
where x∈(−∞,xmax],t∈[0,T],1<α<2,
limx→−∞Vε(x,t)=0, | (3.3) |
Vϵ(xmax,t)=exmax−K, | (3.4) |
Vϵ(x,T)=max(ex−K,0). | (3.5) |
Moreover, according to the conclusion in [25], the maximum value of risk asset equal to 4 times of K value. The subscript ε of Vε(x,t) should be omitted for clarity.
Define Δt>0 and Δx>0 as time and spatial step, respectively. Taking N,M as the positive N∗Δt=T and MΔx=xmax. Thus
ti=(i−1)Δt,i=1,2,...,N+1,xj=(j−1)Δx,j=1,2,...,M+1. |
The forward and backward difference schemes are used for the discrete first-order space. For the time derivative, we use the forward and backward difference schemes, respectively. The approximation of the left-sided and right-sided tempered fractional derivatives given in formula [26] can be used to discretize the left-sided and right-sided tempered fractional derivatives as the following:
e−λx−∞Dαx(eλxVji)−λαVji=1(Δx)α∞∑k=0gkVij−k+1+O(Δx),eλx−∞Dαx(e−λxVji)−λαVji=1(Δx)αM−j+1∑k=0gkVij+k−1+O(Δx), |
where Vij is the value of function V(x,t) at grid point (xj,ti). The coefficients gk(k=0,1,2,⋯) are used and satisfy the following two equations
gk={(−1)k(αk)e−(k−1)λΔx,fork≠1−α−eλΔx(1−e−λΔx)α,fork=1, | (3.6) |
In addition, the integral term contained in the governing equation of Eq (3.2) is approximated by the trapezoidal rules [24], i.e.,
∫+∞−∞V(xj+y,ti)fY(y)dy≈M∑ℓ=0ρMℓ−j[Viℓ+Viℓ+1]+Rj, |
where
ρMj=12∫(j+1)ΔxjΔxfY(y)dy={12∑m1ℓ=1ˆpℓ(e−ˆθℓjΔx−e−ˆθℓ(j+1)Δx),j≥0,12∑m2ℓ=1˜pℓ(e˜θℓ(j+1)Δx−e˜θℓjΔx),j≤0, | (3.7) |
and
Rj=∫+∞xM+1−xj(exj+y−K)fY(y)dy,=exjm1∑ℓ=1ˆpℓˆθℓˆθℓ−1e(1−ˆθℓ)(xmax−xj)−Km1∑ℓ=1ˆpℓe−ˆθℓ(xmax−xj),=(exmax−K)m1∑ℓ=1ˆpℓˆθℓe−ˆθℓ(xmax−xj). | (3.8) |
To sum up, the fully implicit difference scheme for Eq (3.2) can be obtained as follows:
Vi+1j−VijΔt+aVij−Vij−1Δx+ξM∑ℓ=0ρMℓ−j[Viℓ+Viℓ+1]+12σα[p(Δx)αM−j+2∑k=0gαk,λVij+k−1+q(Δx)α∞∑k=0gαk,λVij−k+1]+ξRj+ϵCVij+ϵ−qj=bVij, | (3.9) |
and the boundary conditions are approximated as
limj→∞Vij=0, | (3.10) |
ViM+1=exmax−K, | (3.11) |
VN+1j=max(exj−K,0), | (3.12) |
The fact that the values of Vij for all i,j) must satisfy the constraint condition (2.7) should be strictly proven. In order to ensure completion of proof, we first give two lemmas as follows
Lemma 3.11. ([26]) If α∈(1,2), then the coefficients gk in Eq (3.6) satisfy
{g0=eλΔx,g1=−α−eλΔx(1−e−λΔx)α<0,g2>g3>...>0,∑∞k=0gk=0,∑mk=0gk<0, |
where m≥1.
Lemma 3.2. ([27]) Both the coefficients ρMj in Eq (3.7) and Rj in Eq (3.8) are bounded and satisfy
M∑−∞ρMj≤12, |
Rj≤exmax−K. |
Theorem 3.1. If Δt≤1|b+2ξ∑Mℓ=0ρMℓ−j| and the constant C satisfies the following inequality
C≥|a|exmax−1xmax+σα[(λ+1)α+e(λ+2)xmax]+(b+3ξ)K. |
then Vij obtained by Eq (3.9) satisfies the following inequality Vij≥max(exj−K,0). Here, K=exp(xmax)−K.
Proof. We are going to complete the proof in two steps: We first prove Vij≥exj−K and then prove that Vij≥0 for all i,j.
Let Qj=exj−K,uij=Vij−Qj, then we have
ui+1j−aΔtΔxuij−1+12σαΔt[p(Δx)αM−j+2∑k=0gkuij+k−1+q(Δx)α∞∑k=0gkuij−k+1]+ξΔtM∑ℓ=0ρMℓ−j+ξΔtRj+[uiℓ+uiℓ+1]+ϵCΔtuij+ϵ−ΔtF=(1−aΔtΔx+bΔt)uij, |
where
F=aΔx(qj−qj−1)−bqj−ξM∑ℓ=0ρMℓ−j[ezℓ+ezℓ+1−2K]−ξRj+12σα[p(Δx)αM−j+2∑k=0gkqj+k−1+q(Δx)α∞∑k=0gkqj−k+1]. |
Since |eΔx−1Δx|≤exmax−1xmax≤1, ∑∞k=0gkexj−k+1=exj+1∑∞k=0gke−kΔx, and when |z|<1
∞∑k=0(−1)k(αk)zk=(1−z)α. |
Hence, we have
∣F∣≤|a|exmax−1xmax+b(exmax−K)+ξ(exmax−K)+12σα[λα+e(λ+1)xmax+(λ+1)α+e(λ+2)xmax]+ξ|M∑ℓ=0ρMℓ−j[exℓ+exℓ+1−2K]|.≤|a|exmax−1xmax+b(exmax−K)+ξ(exmax−K)+σα[(λ+1)α+e(λ+2)xmax]+ξ|M∑ℓ=0ρMℓ−j[exℓ+exℓ+1−2K]|. |
Moreover, let K=[exp(xmax)−K], then
|M∑ℓ=0ρMℓ−j[exℓ+exℓ+1−2K]|≤2|M∑ℓ=0ρMℓ−jK|2≤KM∑ℓ=0ρMℓ−j≤2K. |
Therefore,
|F|≤|a|exmax−1xmax+σα[(λ+1)α+e(λ+2)xmax]+(b+3ξ)K. |
Let uiJ=minjuij and ui+1L=minjui+1j, then
{1−12σαΔt[p(Δx)αM−j+2∑k=0gαk,λ+q(Δx)α∞∑k=0gαk,λ]}uiJ−bΔtuiJ−ϵCΔtuiJ+ϵ−2ξM∑ℓ=0ρMℓ−juiJΔt+ΔtF≥ui+1L. |
Namely,
[1−(−b−2ξM∑ℓ=0ρMℓ−j)Δt]ui−ϵCΔtui+ϵ+ΔtF≥ui+1L. |
On the other hand, according to Lemma 3.2 and Δt≤1|b+2ξ∑Mℓ=0ρMℓ−j|, we can obtain
1−(−b−2ξM∑ℓ=0ρMℓ−j)Δt≥0. |
Let
A=1−(−b−2ξM∑ℓ=0ρMℓ−j)Δt, |
and define a function H(x) as
H(x)=Ax−ϵCΔtx+ϵ+ΔtF. | (3.13) |
Then, H(ui)≥0 if ui+1≥0. Since H′(x)=A+ϵCΔt(x+ϵ)2≥0, H(0)=Δt(F−C)≤0, and uN+1≥0, we obtain ui≥0. Hence, uij≥0, and consequently Vij≥Qj is satisfied.
Next, we prove that Vij≥0. We define Vi=minjVij and let J satisfy ViJ=Vi. Hence, according to Eq (3.9), the following inequality can be obtained,
{1−12σαΔt[p(Δx)αM−j+2∑k=0gαk,λ+q(Δx)α∞∑k=0gαk,λ]}Vi−bΔtVi−ϵCΔtVi+ϵ−Qj−2ξM∑ℓ=0ρMℓ−jViΔt+ΔtF≥Vi+1J. |
Then,
[1−(−b−2ξM∑ℓ=0ρMℓ−j)Δt]Vi≥Vi+1J+ϵCΔtVi+ϵ−Qj. |
In the first step, Vij≥Qj(∀i,j) is proven, so ϵCΔtVi+ϵ−Qj≥0. Thus,
[1−(−b−2ξM∑ℓ=0ρMℓ−j)Δt]Vi≥Vi+1J. |
Since, VN+1j=max[exp(xj)−K,0]≥0, therefore
Vij≥0,∀i,j. |
To sum up, we complete the proof.
In fact, the penalty term should result that the discrete system (3.9) is nonlinear; therefore, the Newton iteration method is employed. However, due to the existence of the fractional-integro differential operator, there is a matrix with a dense form in the final system. Thus, we should enhance the computational efficiency while decreasing the storage space.
In order to facilitate the computer to simulate the algorithm (3.9), the original semi-infinite region (−∞,xmax]×[0,T] must be truncated into a limited region (x,t)∈(xmin,xmax]×[0,T], where xmin=ln(0.01) in the numerical experiments below. Now, the left boundary condition in the original model is changed to V(xmin,t)=0.
We should redefine the spatial step size Δx=(xmax−xmin)/M, then xj=(j−1)Δx+xmin, for j=2,…,M+1. Now, we define
ϑ=aΔtΔx,β=1−aΔtΔx+Δtb,η=−12Δtσα(Δx)α, |
and
WMl=ρMl+ρMl−1,l=0,±1,±2,...,±(M−2). |
Then, system (3.9) can be rewritten as the following matrix form,
[βI+ϑB+η(pA⊤+qA)−ΔtW]Vi−F(Vi)=Vi+1+Zi−ΔtRi, | (4.1) |
where
F(Vi)=(F(Vi2),F(Vi3),…,F(ViM−1),F(ViM)),Vi=(Vi2,Vi3,…,ViM−1,ViM), |
with
F(Vij)=ϵCΔtVij+ϵ−Qj,Ri=(Ri2,Ri2,...,RiM),Zi=(0,0,...,ηqg0+ϑ(ρM0+ρM1+...+ρMM−2))ViM+1. |
I is an identity matrix of order (M−1), and A⊤ means matrix transpose of A. A, B, and W are Toeplitz matrices, i.e.,
W=ξ[WM0WM1WM2⋯WMM−2WM−1WM0WM1⋯WMM−3WM−2WM−1WM0⋯WMM−4⋮⋱⋱⋱⋮WM2−MWM3−MWM4−M⋯WM0], |
A=[g1g00⋯0g2g10⋯0g3g2g0⋯0⋮⋱⋱⋱⋮gM−2gM−3⋯g1g0gM−1gM−2⋯g2g1]andB=[000⋯00100⋯00010⋯00⋮⋱⋱⋱⋱⋮000⋯00000⋯10]. |
In fact, the nonlinear penalty term shows that the system (4.1) cannot be solved directly; therefore, we first use the Newton iteration method to change it as a linear system,
[βI+ξB+η(pA⊤+qA)−JF(ωl−1)−ΔtW]Δwl=Vi+1−Zi+1−[βI+ξB+η(pA⊤+qA)−ΔtW]wl−1+F(wl−1)−ΔtRi, | (4.2) |
wl=wl−1+κΔwl, |
where l=1,2,3,…, JF(wl−1) is the Jacobian matrix of the vector F(wl−1), and 0<κ<1 is the adjustment factor. During the numerical iteration, it is assumed that for the current time layer ti, the information of the previous time layer ti+1 is known. Therefore, Vi+1 can be taken as the initial value of the iterative sequence wl, i.e., w0=Vi+1. We set Vi=wl once the stopping criterion ∥wl−wl−1∥≤tol for some l is reached, where tol is the stopping tolerance of the iterative method. Now, by taking
M=βI+ξB+η(pA⊤+qA)−JF(wl−1)−ΔtW,bl=Vi+1+ηZi+1−ΔtRi−[βI+ξB+η(pA⊤+qA)−ΔtW]wl−1+F(wl−1), |
Eq (4.2) can be rewritten as
[M−JF(wl−1)](δwl)=bl. | (4.3) |
The most challenging part in solving Eq (4.3) is the high computational cost resulting from the fact that both A and W are dense matrices. To overcome this difficulty, we first apply the CGNR method [28], which is to solve [M−JF]⊤Mδwl=[M−JF]⊤bl instead of Eq (4.3).
However, by noticing that the convergence rate of the CGNR method is still quite low due to the fact that the conditional number of the matrix M⊤M is large, a pre-conditioner technique is applied to accelerate the convergence rate of the CGNR method. It is straightforward to find that the matrix JF is not the Toeplitz matrix, and we should approximate this matrix as a0I, where a0 is the average value of main diagonal elements of matrix JF. Thus, we structure a Toeplitz matrix as follows
T=M−a0I. |
Next, the Strang's circulant preconditioner s(T)=[sj−k]0≤j,k<M for matrix T is structured as
sj={Tj,0≤j<M/2,0,j=M/2if M is even,andj=(M+1)/2 if M is odd,Tj−M,M/2<j<M,Tj+M,0<−j<M. |
Let P denote the Strang's circulant preconditioner s(T)=[sj−k]0≤j,k<M to simplify the expression.
Mathematically, after the PCGNR method with a pre-conditioner P is applied, Eq (4.3) becomes
[(P)−1(M−JF)]⊤[(P)−1(M−JF)]δwl=[(P)−1(M−JF)]⊤(P)−1bl. |
The pseudo-code of the PCGNR method is displayed in Algorithm 1. The matrix-vector multiplication needs only O(MlogM) operations via the fast Fourier transform (FFT) method.
Algorithm 1. PCGNR method for solving (M−JF)(δwl)=bl with a pre-conditioner P. |
Given the initial guess x0, and a stopping tolerance tol. |
Compute r0=[P−1(bl−(M−JF))x0], |
z0=[(P)−1(M−JF)]⊤r0,p0=z0,mr=||r0||22. |
For i=0,1,..., |
wi=[(P)−1(M−JF)]⊤pi, |
αi=||zi||22/||wi||22, |
xi+1=xi+αipi, |
ri+1=ri−αiwi, |
zi+1=[(P)−1(M−JF)]⊤ri+1, |
βi=||zi+1||22/||zi||22, |
pi+1=zi+1+βipi, |
res=||ri+1||22. |
If res/mr<tol, stop; |
otherwise, set δwl=xi+1. |
End for |
Several numerical examples are given to show the computational efficiency of our numerical method in this part. Moreover, the impacts of the key parameter in our model to the option value and optimal exercise boundary are also discussed. All simulations are implemented using MATLAB2014a on a Lenovo T14 laptop with configuration: Intel(R) Core(TM) i7-1260P 2.10 GHz. The CPU time (in seconds) is estimated by using the timing functions tic/toc.
First, we should examine whether or not the numerical solution preserves the basic properties of American call. This could be viewed as a necessary condition for the reliability of the proposed approach. Mathematically, the current numerical solution must satisfy the inequality Vij≥max(qj−K,0). Depicted in Figure 1 are the surfaces of Vij−max(qj−K,0) with different parameter settings, which implies that the inequality is preserved.
Figure 2(a), (b) display the American call value surface and option values and payoff function, respectively. First, the curves in the two figures indicate that the American call option price is an increasing function with respect to an underlying asset price, and the 'high contact' condition for American call is also confirmed by such surfaces in Figure 2(a), which shows KoBoLJ model is indeed reasonable. It can be observed from the two figures that the numerical method based on the penalty term produces the smooth and stable approximation solutions. To sum up, both our model and the numerical scheme are reasonable.
To further investigate the performance of the method, we compare the computational efficiency of the Gaussian elimination (GE), the CGNR method, and the PCGNR method, as shown in Table 1. The parameters adopted for computing this table are K=20, r=0.05, σ=0.24, D=0.06, p=0.6, ˆp=0.07, ˆθ=1.5, ˜θ=0.5, , α=1.52, ξ=0.2, ˆp=0.08, ˆθ=1.8, ˜θ=0.2, ξ=0.1. Moreover, in this table, Ite−In denotes the average iteration number required in each time step. ORGE, ORCGNR and ORPCGNR refer the convergence order in x direction of three different method, respectively. The convergence order is defined as
ORi+1=ln(Erri)−ln(Erri+1)ln(Mi+1)−ln(Mi), |
where Mi is the number of spatial grid nodes employed and
Err=‖Vj,ik−V(k;x,t)ref‖2, |
where ∥⋅∥2 is the L2 norm for matrix, and V(x,t)ref is the benchmark solution determined directly through matrix operation 'A∖b' in Matlab with (M,N)=(212,1000).
GE | CGNR | PCGNR | ||||||||||||
M | Time(s) | Err | ORGE | Ite−In | Time(s) | Err | ORCGNR | Ite−In | Time(s) | Err | ORPCGNR | |||
25 | 37.5201 | 0.0743 | - | 51.2422 | 1.0240 | 0.0793 | - | 5.2846 | 0.6513 | 0.0945 | - | |||
26 | 150.2406 | 0.0303 | 1.2940 | 52.7299 | 1.9356 | 0.0322 | 1.3003 | 6.4601 | 0.7291 | 0.0402 | 1.2331 | |||
27 | 628.7839 | 0.0114 | 1.4103 | 50.7812 | 3.5031 | 0.0130 | 1.3085 | 7.0381 | 1.3810 | 0.0164 | 1.2935 | |||
28 | 2494.7124 | 0.0042 | 1.4406 | 52.3963 | 9.5677 | 0.0055 | 1.2410 | 6.8341 | 7.1290 | 0.0068 | 1.2701 | |||
29 | 18290.4211 | 0.0017 | 1.3049 | 52.1256 | 49.9873 | 0.0023 | 1.2578 | 6.8930 | 19.0211 | 0.0026 | 1.3870 | |||
210 | ** | ** | ** | 52.6767 | 250.1262 | 0.0010 | 1.2016 | 7.0025 | 21.4600 | 0.0011 | 1.2410 |
We can observe from Table 1 that for a fixed number of nodal points, the total CPU times required by the CGNR and PCGNR to produce the same level of error are significantly less than that of the GE. Furthermore, the average inner iteration numbers required by the PCGNR method are the lowest. These suggest the superiority of the PCGNR method in computational efficiency over the GE and CGNR methods. Moreover, it is clear that the ORGE, ORCGNR, and ORPCGNR are close to 1, which indicates that the three schemes are of first-order convergence in the spatial direction.
Similarly, the convergence order and error in the t direction of the PCGNR method is also examined. First, the V(x,t)ref is the benchmark solution that can be determined directly through matrix operation 'A∖b' in Matlab with (M,N)=(212+1,1000). We increase the grid number in the t direction from 100 to 800. In Table 2, both the Err and OR denote the error and convergence order in the t direction of PCGNR method, respectively. The results are displayed in Table 2. From this table, it is clear that our scheme is first-order convergent.
Number of time steps | Err | OR |
100 | 0.0701 | – |
200 | 0.0334 | 1.0696 |
400 | 0.0156 | 1.0983 |
600 | 0.0103 | 1.0238 |
800 | 0.0046 | 1.1629 |
We first consider the value of parameter α, which affects the optimal exercise boundary of American call. Tow sets of optimal exercise boundary with different α are computed and displayed in Figure 3. From the curves in this figure, one can find that a bigger value of α should show a higher optimal exercise boundary. Financially, the α controls the tail of the distribution of the returns of risk asset, and both tails will be fatter when α becomes bigger. Thus, as α becomes bigger, the possibility of smaller stock price increases, and so does the optimal exercise price.
Next, we consider how the discrete jumps influence the optimal exercise price of American call. As shown in Figure 4 is the optimal exercise price as a function of the time to expiry with different jump intensity ξ. One can observe from this figure that the optimal exercise price increases with respect to ξ. Financially, a larger jump intensity indicates that the risk asset would change more often so that the American call option contract should be more valuable as it contains more risks. Hence, according to the smooth pasty condition across the free boundary, the monotonicity of Sf with respect to ξ holds automatically.
In Figure 5, the optimal exericse price is plotted against the time to expiry with different probabilities of positive jumps ˆp. From the curves in this figure, it is straightforward to find that a larger ˆp results in a lower optimal exercise boundary curve. In fact, the logarithmic return of the risk asset is decreasing with respect to ˆp, because the return decreases with respect to ξ from Eq (2.1) and ξ increases with respect to ˆp from Eq (2.2). Therefore, an increasing ˆp would lower the risk asset value, and thus makes the intermediate American call option less valuable. Therefore, the optimal exercise boundary exf of the intermediate American call decreases with respect to ˆp. Similarly, one could explain the monotonicity of the optimal exercise price with respect to ˆθ and ˜θ. For the length of the paper, we provide those curves in Figure 6 with no detailed explanations.
Next, we should investigate the impacts of parameters p and q. In fact, the upward movement frequency of our stochastic process is controlled by parameter p. If the value of parameter p becomes bigger, which means that our stochastic process should have increased upward movement, then the American call option price should become bigger. As a rational investor, a higher price should be used to exercise the option. Hence, a bigger value of p should result in a higher optimal exercise price as shown in Figure 7. Similarly, we can analyze the impacts of parameter q on the optimal exercise boundary.
The optimal exercise boundary curves under different parameter λ are displayed in Figure 8. As described in Section 3.2, the decay rate of tails of our stochastic process probability density function is controlled by parameter λ>0. Thus, a bigger value of this parameter should result in a thinner tail of the stochastic process density function, and the investor should want to gain a bigger price to exercise the American option.
In this subsection, we consider the stock loans based on the finite moment log-stable process (FMLS). Under this framework of FMLS, the stock loans pricing model is [25].
{∂V(x,t)∂t−(r−D−ν)∂V(x,t)∂x+ν−∞DαxV(x,t)=−rV(x,t),limx→−∞V(x,t)=0,V(x,T)=max(ex−KeγT,0),V(xf,t)=exf−Keγt,∂V(xf,t)∂x=exf, | (5.1) |
where V(x,t) denotes the price of stock loans, r, D, and t are the risk free interest rate, the dividend and the current time, respectively, σ is a constant, and ν=−σαsecαπ2 is a convexity adjustment. t∈[0,T],x∈(−∞,xf),1<α<2,exf is the optimal redemption price of stock loans. Thus,
−∞DαxV(x,t;α)=1Γ(2−α)∂2∂x2∫x−∞V(z,t;α)(x−z)α−1dz. |
In fact, the FMLS model is a special case of the KoBoLJ model. Hence, the method is used to solve model (5.1).
We choose the spatial step size Δx=xmax−xmin211+1 and temporal step size Δt=21000. Thus, we can obtain the following three figures:
The curved surface in Figure 9 and the curve in Figures 10 and 11 show that our numerical method is effective.
In this paper, we consider the American call option pricing based on the KoBoLJ model. The pricing model is a free boundary problem, and the governing equation is a FPIDE. Thus, a numerical scheme based on the penalty function is set. Both the pricing mathematical model and current scheme are very reliable, which is verified by our numerical results. In order to improve computational efficiency, both the PCGNR and fast Fourier transform technique are used to solve the final linear system. Moreover, the impacts of key parameters α,p,ˆp,ˆθ,˜θ, and λ on optimal exercise price are also analyzed.
At the end of this section, we point out that several issues are not discussed in this paper but the future studies will be implemented for them. First, a risk-free interest rate is a constant in our model. In fact, the constant interest rate cannot describe how the interest rate evolves with respect to the time, especially for the option contracts that have a long time horizon. Second, our numerical results show that the KoBoLJ model is a more comprehensive model than the KoBoJ model; therefore, it should be used to investigate other financial derivative pricing and hedging problems, such as the CDS and Stock Loans. Finally, for the jump processes without the consideration of diffusion processes, we should discuss whether their approaches can be extended to models with jumps and diffusion, such as the stochastic volatility and stochastic liquidity [29,30,31].
The first author is mainly responsible for formula derivation and programming. The second author is primarily responsible for writing the paper and conducting theoretical analysis.
The authors declare they have not used Artificial Intelligence (AI) tools in the creation of this article.
The work on this paper is partially supported by the Guizhou University of Commerce Natural Science Projects (No. [2022]ZKZD003). Guizhou University of Commerce Social Science Projects (No. 2024XJSDZD07). Guizhou Provincial Department of Education Natural Science Research Project (No. QJJ[2024]179). And the Disaster Remote Sensing Prevention Workstation of the Academician Innovation Team in Guizhou Province (No. KXJZ[2024]006).
The authors declare there is no conflict of interest.
[1] |
R. Geske, H. E. Johnson, The American put option valued analytically, J. Finance, 5 (1984), 1511–1524. http://dx.doi.org/10.1111/j.1540-6261.1984.tb04921.x doi: 10.1111/j.1540-6261.1984.tb04921.x
![]() |
[2] |
N. T. Le, S. P. Zhu, X. Lu, An integral equation approach for the valuation of American-style down and out calls with rebates, Comput. Math. Appl., 71 (2016), 544–564. https://doi.org/10.1016/j.camwa.2015.12.013 doi: 10.1016/j.camwa.2015.12.013
![]() |
[3] |
S. P. Zhu, X. J. He, X. P. Lu, A new integral equation formulation for American put options, Quant. Finance, 18 (2018), 483–490. https://doi.org/10.1080/14697688.2017.1348617 doi: 10.1080/14697688.2017.1348617
![]() |
[4] |
T. B. Gyulov, M. N. Koleva, Penalty method for indifference pricing of American option in a liquidity switching market, Appl. Numer. Math., 172 (2022), 525–545. https://doi.org/10.1016/j.apnum.2021.11.002 doi: 10.1016/j.apnum.2021.11.002
![]() |
[5] |
J. Xiang, X. Wang, Quasi-Monte Carlo simulation for American option sensitivities, J. Comput. Appl. Math., 413 (2022), 114268. https://doi.org/10.1016/j.cam.2022.114268 doi: 10.1016/j.cam.2022.114268
![]() |
[6] |
D. W. Wang, S. Kirill, C. Christina, A high-order deferred correction method for the solution of free boundary problems using penalty iteration, with an application to American option pricing, J. Comput. Appl. Math., 432 (2023), 115272. https://doi.org/10.1016/j.cam.2023.115272 doi: 10.1016/j.cam.2023.115272
![]() |
[7] |
A. Elettra, A. Rossella, Pricing multidimensional American options, Int. J. Financ. Stud., 11 (2023), 51. http://doi.org/10.3390/IJFS11010051 doi: 10.3390/IJFS11010051
![]() |
[8] |
Q. Zhang, Q. Wang, H. M. Song, Y. L. Hao, Primal-dual active set method for evaluating American put options on zero-coupon bonds, Comput. Appl. Math., 43 (2024), 213–230. http://doi.org/10.1007/S40314-024-02729-Z doi: 10.1007/S40314-024-02729-Z
![]() |
[9] |
L. B. Li, Z. S. Wu, Defaultable perpetual American put option in a last passage time model, Stat. Probab. Lett., 209 (2024), 110018. https://doi.org/10.1016/j.spl.2023.110018 doi: 10.1016/j.spl.2023.110018
![]() |
[10] |
D. S. Bates, Dollar jump fears, 1984–1992: Distributional abnormalities implicit in currency futures options, J. Int. Money Finance, 15 (1996), 65–93. https://doi.org/10.1016/0261-5606(95)00039-9 doi: 10.1016/0261-5606(95)00039-9
![]() |
[11] |
P. Jorion, On jump processes in the foreign exchange and stock markets, Rev. Financ. Stud., 1 (1988), 427–445. https://doi.org/10.1093/rfs/1.4.427 doi: 10.1093/rfs/1.4.427
![]() |
[12] | E. F. Fama, The behavior of stock market prices, J. Business, 38 (1965), 34–105. https://www.jstor.org/stable/2350752 |
[13] | B. B. Mandelbrot, Fractals and Scaling in Finance: Discontinuity, Concentration, Risk: Selecta Volume E, Springer-Verlag, New York, 1997. |
[14] |
P. Carr, L. Wu, The finite moment log stable process and option pricing, J. Finance, 58 (2003), 753–777. https://doi.org/10.1111/1540-6261.00544 doi: 10.1111/1540-6261.00544
![]() |
[15] |
C. Fan, C. H. Zhou, Pricing stock loans with the CGMY model, Discrete. Dyn. Nat. Soc., (2019), 1–11. https://doi.org/10.1155/2019/6903019 doi: 10.1155/2019/6903019
![]() |
[16] |
I. Koponen, Analytic approach to the problem of convergence of truncated Lˇevy flights towards the Gaussian stochastic process, Phys. Rev. E, 52 (1995), 1197. http://doi.org/10.1103/PhysRevE.52.1197 doi: 10.1103/PhysRevE.52.1197
![]() |
[17] |
H. Zhang, F. Liu, I. Turner, S. Chen, The numerical simulation of the tempered fractional Black-Scholes equation for European double barrier option, Appl. Math. Modell., 40 (2016), 5819–5834. https://doi.org/10.1016/j.apm.2016.01.027 doi: 10.1016/j.apm.2016.01.027
![]() |
[18] | A. Cartea, Dynamic Hedging of Financial Instruments when the Underlying Follows a Non-Gaussian Process, Birkbeck College, University of London, London, 2005. http://dx.doi.org/10.2139/ssrn.934812 |
[19] |
W. Chen, S. Lin, Option pricing under the KoBoL model, ANZIAM J., 60 (2018), 175–190. http://dx.doi.org/10.1017/S1446181118000196 doi: 10.1017/S1446181118000196
![]() |
[20] |
J. Mohapatra, S. Sudarshan, R. Higinio, Analytical and numerical solution for the time fractional Black-Scholes model under jump-diffusion, Comput. Econ., 63 (2023), 1853–1878. https://doi.org/10.1007/s10614-023-10386-3 doi: 10.1007/s10614-023-10386-3
![]() |
[21] |
W. Gong, Z. Xu, Y. Sun, An RBF method for time fractional jump-diffusion option pricing model under temporal graded meshes, Axioms, 13 (2024), 674. https://doi.org/10.3390/axioms13100674 doi: 10.3390/axioms13100674
![]() |
[22] |
C. Y. Fan, X. M. Gu, S. H. Dong, H. Yuan, American option valuation under the framework of CGMY model with regime-switching process, Comput. Econ. 6 (2024), 1–25. https://doi.org/10.1007/s10614-024-10734-x doi: 10.1007/s10614-024-10734-x
![]() |
[23] |
X. Chen, D. Ding, S. L. Lei, W. Wang, A fast preconditioned iterative method for two-dimensional options pricing under fractional differential models, Comput. Math. Appl., 79 (2020), 440–456. https://doi.org/10.1016/j.camwa.2019.07.010 doi: 10.1016/j.camwa.2019.07.010
![]() |
[24] |
Z. Zhou, J. Ma, X. Gao, Convergence of iterative Laplace transform methods for a system of fractional PDEs and PIDEs arising in option pricing, East Asian. J. Appl. Math., 8 (2018), 782–808. http://dx.doi.org/10.4208/eajam.130218.290618 doi: 10.4208/eajam.130218.290618
![]() |
[25] |
W. Chen, X. Xu, S. P. Zhu, A predictor-corrector approach for pricing American options under the finite moment log-stable model, Appl. Numer. Math., 97 (2015), 15–29. https://doi.org/10.1016/j.apnum.2015.06.004 doi: 10.1016/j.apnum.2015.06.004
![]() |
[26] |
X. Chen, W. Wang, D. Ding, S. Lei. A fast preconditioned policy iteration method for solving the tempered fractional HJB equation governing American options valuation, Comput. Math. Appl., 73 (2017), 1932–1944. https://doi.org/10.1016/j.camwa.2017.02.040 doi: 10.1016/j.camwa.2017.02.040
![]() |
[27] |
C. Y. Fan, W. T. Chen, B. Feng, Pricing stock loans under the Lˊevy-α-stable process with jumps, Networks Heterogen. Media, 18 (2022), 191–211. https://doi.org/10.3934/nhm.2023007 doi: 10.3934/nhm.2023007
![]() |
[28] |
S. L. Lei, H. W. Sun, A circulant preconditioner for fractional diffusion equations, J. Comput. Phys., 242 (2013), 715–725. https://doi.org/10.1016/j.jcp.2013.02.025 doi: 10.1016/j.jcp.2013.02.025
![]() |
[29] |
X. J. He, S. Lin, A probabilistic approach for the valuation of variance swaps under stochastic volatility with jump clustering and regime switching, Financ. Innovation, 10 (2024), 114. http://dx.doi.org/10.1186/S40854-024-00640-4 doi: 10.1186/S40854-024-00640-4
![]() |
[30] |
X. J. He, S. Lin, A stochastic liquidity risk model with stochastic volatility and its applications to option pricing, Stochastic Models, 10 (2024), 1–20. https://doi.org/10.1080/15326349.2024.2332326 doi: 10.1080/15326349.2024.2332326
![]() |
[31] |
X. J. He, S. Lin, Analytical formulae for variance and volatility swaps with stochastic volatility, stochastic equilibrium level and regime switching, AIMS Math., 9 (2024), 22225–22238. https://doi.org/10.3934/math.20241081 doi: 10.3934/math.20241081
![]() |
GE | CGNR | PCGNR | ||||||||||||
M | Time(s) | Err | ORGE | Ite−In | Time(s) | Err | ORCGNR | Ite−In | Time(s) | Err | ORPCGNR | |||
25 | 37.5201 | 0.0743 | - | 51.2422 | 1.0240 | 0.0793 | - | 5.2846 | 0.6513 | 0.0945 | - | |||
26 | 150.2406 | 0.0303 | 1.2940 | 52.7299 | 1.9356 | 0.0322 | 1.3003 | 6.4601 | 0.7291 | 0.0402 | 1.2331 | |||
27 | 628.7839 | 0.0114 | 1.4103 | 50.7812 | 3.5031 | 0.0130 | 1.3085 | 7.0381 | 1.3810 | 0.0164 | 1.2935 | |||
28 | 2494.7124 | 0.0042 | 1.4406 | 52.3963 | 9.5677 | 0.0055 | 1.2410 | 6.8341 | 7.1290 | 0.0068 | 1.2701 | |||
29 | 18290.4211 | 0.0017 | 1.3049 | 52.1256 | 49.9873 | 0.0023 | 1.2578 | 6.8930 | 19.0211 | 0.0026 | 1.3870 | |||
210 | ** | ** | ** | 52.6767 | 250.1262 | 0.0010 | 1.2016 | 7.0025 | 21.4600 | 0.0011 | 1.2410 |
Number of time steps | Err | OR |
100 | 0.0701 | – |
200 | 0.0334 | 1.0696 |
400 | 0.0156 | 1.0983 |
600 | 0.0103 | 1.0238 |
800 | 0.0046 | 1.1629 |
GE | CGNR | PCGNR | ||||||||||||
M | Time(s) | Err | ORGE | Ite−In | Time(s) | Err | ORCGNR | Ite−In | Time(s) | Err | ORPCGNR | |||
25 | 37.5201 | 0.0743 | - | 51.2422 | 1.0240 | 0.0793 | - | 5.2846 | 0.6513 | 0.0945 | - | |||
26 | 150.2406 | 0.0303 | 1.2940 | 52.7299 | 1.9356 | 0.0322 | 1.3003 | 6.4601 | 0.7291 | 0.0402 | 1.2331 | |||
27 | 628.7839 | 0.0114 | 1.4103 | 50.7812 | 3.5031 | 0.0130 | 1.3085 | 7.0381 | 1.3810 | 0.0164 | 1.2935 | |||
28 | 2494.7124 | 0.0042 | 1.4406 | 52.3963 | 9.5677 | 0.0055 | 1.2410 | 6.8341 | 7.1290 | 0.0068 | 1.2701 | |||
29 | 18290.4211 | 0.0017 | 1.3049 | 52.1256 | 49.9873 | 0.0023 | 1.2578 | 6.8930 | 19.0211 | 0.0026 | 1.3870 | |||
210 | ** | ** | ** | 52.6767 | 250.1262 | 0.0010 | 1.2016 | 7.0025 | 21.4600 | 0.0011 | 1.2410 |
Number of time steps | Err | OR |
100 | 0.0701 | – |
200 | 0.0334 | 1.0696 |
400 | 0.0156 | 1.0983 |
600 | 0.0103 | 1.0238 |
800 | 0.0046 | 1.1629 |