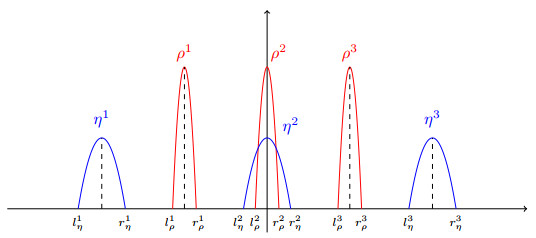
A possible example of a stationary solution to (3) with
The intelligent clonal optimizer (ICO) is a new evolutionary algorithm, which adopts a new cloning and selection mechanism. In order to improve the performance of the algorithm, quasi-opposition-based and quasi-reflection-based learning strategy is applied according to the transition information from exploration to exploitation of ICO to speed up the convergence speed of ICO and enhance the diversity of the population. Furthermore, to avoid the stagnation of the optimal value update, an adaptive parameter method is designed. When the update of the optimal value falls into stagnation, it can adjust the parameter of controlling the exploration and exploitation in ICO to enhance the convergence rate of ICO and accuracy of the solution. At last, an improved intelligent chaotic clonal optimizer (IICO) based on adaptive parameter strategy is proposed. In this paper, twenty-seven benchmark functions, eight CEC 2104 test functions and three engineering optimization problems are used to verify the numerical optimization ability of IICO. Results of the proposed IICO are compared to ten similar meta-heuristic algorithms. The obtained results confirmed that the IICO exhibits competitive performance in convergence rate and accurate convergence.
Citation: Jiahao Zhang, Zhengming Gao, Suruo Li, Juan Zhao, Wenguang Song. Improved intelligent clonal optimizer based on adaptive parameter strategy[J]. Mathematical Biosciences and Engineering, 2022, 19(10): 10275-10315. doi: 10.3934/mbe.2022481
[1] | Simone Fagioli, Yahya Jaafra . Multiple patterns formation for an aggregation/diffusion predator-prey system. Networks and Heterogeneous Media, 2021, 16(3): 377-411. doi: 10.3934/nhm.2021010 |
[2] | Rinaldo M. Colombo, Francesca Marcellini, Elena Rossi . Biological and industrial models motivating nonlocal conservation laws: A review of analytic and numerical results. Networks and Heterogeneous Media, 2016, 11(1): 49-67. doi: 10.3934/nhm.2016.11.49 |
[3] | Mostafa Bendahmane . Analysis of a reaction-diffusion system modeling predator-prey with prey-taxis. Networks and Heterogeneous Media, 2008, 3(4): 863-879. doi: 10.3934/nhm.2008.3.863 |
[4] | Ivano Primi, Angela Stevens, Juan J. L. Velázquez . Pattern forming instabilities driven by non-diffusive interactions. Networks and Heterogeneous Media, 2013, 8(1): 397-432. doi: 10.3934/nhm.2013.8.397 |
[5] | Marie Henry . Singular limit of an activator-inhibitor type model. Networks and Heterogeneous Media, 2012, 7(4): 781-803. doi: 10.3934/nhm.2012.7.781 |
[6] | Stefan Berres, Ricardo Ruiz-Baier, Hartmut Schwandt, Elmer M. Tory . An adaptive finite-volume method for a model of two-phase pedestrian flow. Networks and Heterogeneous Media, 2011, 6(3): 401-423. doi: 10.3934/nhm.2011.6.401 |
[7] | José Antonio Carrillo, Yingping Peng, Aneta Wróblewska-Kamińska . Relative entropy method for the relaxation limit of hydrodynamic models. Networks and Heterogeneous Media, 2020, 15(3): 369-387. doi: 10.3934/nhm.2020023 |
[8] | Panpan Xu, Yongbin Ge, Lin Zhang . High-order finite difference approximation of the Keller-Segel model with additional self- and cross-diffusion terms and a logistic source. Networks and Heterogeneous Media, 2023, 18(4): 1471-1492. doi: 10.3934/nhm.2023065 |
[9] | Julien Barré, Pierre Degond, Diane Peurichard, Ewelina Zatorska . Modelling pattern formation through differential repulsion. Networks and Heterogeneous Media, 2020, 15(3): 307-352. doi: 10.3934/nhm.2020021 |
[10] | Verónica Anaya, Mostafa Bendahmane, Mauricio Sepúlveda . Mathematical and numerical analysis for Predator-prey system in a polluted environment. Networks and Heterogeneous Media, 2010, 5(4): 813-847. doi: 10.3934/nhm.2010.5.813 |
The intelligent clonal optimizer (ICO) is a new evolutionary algorithm, which adopts a new cloning and selection mechanism. In order to improve the performance of the algorithm, quasi-opposition-based and quasi-reflection-based learning strategy is applied according to the transition information from exploration to exploitation of ICO to speed up the convergence speed of ICO and enhance the diversity of the population. Furthermore, to avoid the stagnation of the optimal value update, an adaptive parameter method is designed. When the update of the optimal value falls into stagnation, it can adjust the parameter of controlling the exploration and exploitation in ICO to enhance the convergence rate of ICO and accuracy of the solution. At last, an improved intelligent chaotic clonal optimizer (IICO) based on adaptive parameter strategy is proposed. In this paper, twenty-seven benchmark functions, eight CEC 2104 test functions and three engineering optimization problems are used to verify the numerical optimization ability of IICO. Results of the proposed IICO are compared to ten similar meta-heuristic algorithms. The obtained results confirmed that the IICO exhibits competitive performance in convergence rate and accurate convergence.
The mathematical modelling of the collective motion through aggregation/diffusion phenomena has raised a lot of interest in the recent years and it has been deeply studied for its application in several areas, such as biology [9, 39, 47, 48], ecology [35, 42, 43], animal swarming [3, 4, 40, 46] sociology and economics, [49, 50, 51]. One of the common idea in this modelling approach is that a certain population composed by agents evolves according to long-range attraction and short-range repulsion forces between agents. We are interested in modelling the problem of predator-prey interactions, namely we consider two populations that attract (prey) and repel (predators) each others. The pioneering works for this problem are the ones by Lotka, [36] and Volterra[54], which describe the predator-prey interaction via reaction terms in a set of differential equations, possibly combined with diffusion terms, see [41] and the references therein.
As in [21], in this paper we model predator-prey interactions via a transport terms rather than a reaction ones as follows: consider
{˙Xi(t)=−N∑k=1mkX(∇Sr1(Xi(t)−Xk(t))+∇Sa1(Xi(t)−Xk(t)))−M∑h=1mhY∇K(Xi(t)−Yh(t)),˙Yj(t)=−M∑h=1mhY(∇Sr2(Xi(t)−Xk(t))+∇Sa2(Yj(t)−Yh(t)))+αM∑k=1mkX∇K(Yj(t)−Xk(t)), | (1) |
with
The formal limit when the number of particles tends to infinity leads to the following system of partial differential equations
{∂tρ=div(ρ∇(d1ρ−Sa1∗ρ−K∗η)),∂tη=div(η∇(d2η−Sa2∗η+αK∗ρ)), | (2) |
where
The goal of this paper is to show that the model above catches one of the main features that occur in nature, namely the formation of spatial patterns where the predators are surrounded of empty zones and the prey aggregates around, that is usually observed in fish schools or in flock of sheeps, see [32, 38]. In the fully aggregative case, namely system (2) with
Existence theory for solutions to system of the form (2) can be performed in the spirit of [10, 20]. In particular, system (2) should be framed in the context of non symmetrizable systems, for which the Wasserstein gradient flow theory developed in [1] and adapted to systems in [22] does not work. In [10, 20, 22], the authors consider different choices for the diffusion part (no diffusion in [22], diagonal nonlinear diffusion in [10] and cross-diffusion with dominant diagonal in [20]), and the existence of solutions is proved via an implicit-explicit version of the JKO scheme [33].
In the following, we reduce our analysis to the one-dimensional setting
{∂tρ=∂x(ρ∂x(d1ρ−Sρ∗ρ−K∗η))∂tη=∂x(η∂x(d2η−Sη∗η+αK∗ρ)). | (3) |
We are interested in the existence of stationary solutions to (3), which are solutions to the following system
{0=(ρ(d1ρ−Sρ∗ρ−K∗η)x)x,0=(η(d2η−Sη∗η+αK∗ρ)x)x, | (4) |
as well as their properties, e.g., symmetry, compact support, etc. We shall assume throughout the paper that
{0=(ρ(θρ−˜Sρ∗ρ−˜K∗η)x)x,0=(η(θη−Sη∗η+˜α˜K∗ρ)x)x. |
The stationary equation for the one species case, i.e.,
∂tρ=∂x(ρ∂x(θρ−S∗ρ)) |
is studied several papers, see [2, 7, 11, 16] and therein references. In [7] the Krein-Rutman theorem is used in order to characterise the steady states as eigenvectors of a certain non-local operator. The authors prove that a unique steady state with given mass and centre of mass exists provided that
In this paper we apply the aforementioned approach in order to show that stationary solutions to (3) are composed of multiple Barenblatt profiles. Let us introduce, for fixed
M={(ρ,η)∈(L∞∩L1(R))2:ρ,η≥0,‖ρ‖L1=zρ,‖η‖L1=zη}. |
Definition 1.1. We say that a pair
●
●
supp(ρ)=Nρ⋃i=1Iiρandsupp(η)=Nη⋃i=1Iiη, |
respectively and
where, for
Example 1.1. A possible example of steady states as defined above is a steady state
Remark 1.1. We remark that one should be careful with finding a shape of steady state. More precisely, one should choose the numbers
In order to simplify the notations, in the following we will denote with
(A1)
(A2)
(A3)
Note that assumption (A2) together with the sign in front of the nonlocal terms
The main result of the paper is the following
Theorem 1.1. Assume that
(i)
Bil=Nl∑j=1S′l(cmil−cmjl)zjl+αlNh∑j=1K′(cmil−cmjh)zjh=0, | (5) |
(ii) the following quantities
Dil=−Nl∑j=1S″l(cmil−cmjl)zjl−αlNh∑j=1K″(cmil−cmjh)zjh, | (6) |
are strictly positive, for all
where
where
● each interval
●
● the solutions
zρ=Nρ∑i=1ziρandzη=Nη∑i=1ziη, |
respectively.
The paper is structured as follows. In Section 2 we recall the basics notions on optimal transport and we introduce the
We start collecting preliminary concepts on the Wasserstein distance. Let
Pp(Rn)={μ∈P(Rn):mp(μ)=∫Rn|x|pdμ(x)<∞}. |
For a measure
∫Rkf(y)dT#μ(y)=∫Rnf(T(x))dμ(x)forallfBorelfunctionsonRk. |
We endow the space
Wpp(μ,ν)=infγ∈Γ(μ,ν){∫Rn×Rn|x−y|pdγ(x,y)}, | (7) |
where
Since we are working in a 'multi-species' structure, we consider the product space
\begin{equation*} \mathcal{W}_{p}^{p}(\mathit{\boldsymbol{\mu}},\mathit{\boldsymbol{\nu}}) = W_{p}^{p}(\mu_{1},\nu_{1})+W_{p}^{p}(\mu_{2},\nu_{2}), \end{equation*} |
with
\begin{equation} u_\mu(z) \doteq \inf \bigl\{ x \in \mathbb{R} \colon \mu((-\infty,x]) > z \bigr\}, \quad z\in [0,1], \end{equation} | (8) |
see [14].
In the Section 1 we mentioned that the well-posedness of (3) can be stated according to the results in [10, 20] in an arbitrary space dimension
\begin{align*} {\mathcal{F}}_{\left[\mu,\nu\right]}(\rho,\eta) & = \frac{\theta}{2}\int_{ \mathbb{R}^n}\rho^2+\eta^2 dx - \frac{1}{2}\int_{ \mathbb{R}^n}\rho S_\rho\ast \rho dx- \frac{1}{2}\int_{ \mathbb{R}^n}\eta S_\eta\ast \eta dx\\ &- \int_{ \mathbb{R}^n}\rho K \ast \mu dx+ \alpha\int_{ \mathbb{R}^n}\eta K \ast \nu dx, \end{align*} |
for a fixed reference couple of measures
Definition 2.1. A curve
(i)
(ii) for almost every
\begin{align*} \frac{d}{dt}\int_ {\mathbb{R}^{n}}\phi\rho dx & = -\theta\int_ {\mathbb{R}^{n}}\rho\nabla\rho\cdot\nabla\phi\,dx+\int_ {\mathbb{R}^{n}}\rho\left(\nabla S_\rho \ast \rho + \nabla K\ast\eta\right)\nabla \phi \,dx, \\ \frac{d}{dt}\int_ {\mathbb{R}^{n}}\varphi\eta dx & = -\theta\int_ {\mathbb{R}^{n}}\eta\nabla\eta\cdot\nabla\varphi\,dx+\int_ {\mathbb{R}^{n}}\eta\left(\nabla S_\eta \ast \eta -\alpha \nabla K\ast\rho\right)\nabla \phi \,dx. \end{align*} |
Theorem 2.1. Assume that (A1)-(A3) are satisfied. Let
{\mathcal{F}}_{[\mathit{\boldsymbol{\mu}}_0]}\left(\mathit{\boldsymbol{\mu}}_0\right) < +\infty. |
Then, there exists a weak solution to (3) in the sense of Definition 2.1 with
As already mentioned the proof of Theorem 2.1 is a special case of the results in [10, 20] and consists in the following main steps:
1. Let
\begin{equation} \mathit{\boldsymbol{\mu}}_\tau^{k+1}\in\mathop {\arg \min }\limits_{\mathit{\boldsymbol{\mu}}\in {\mathscr{P}_{2}({\mathbb{R}^{n}})}^2}\left\{\frac{1}{2\tau}\mathcal{W}_2^2(\mathit{\boldsymbol{\mu}}_\tau^k,\mathit{\boldsymbol{\mu}})+ {\mathcal{F}}_{[\mathit{\boldsymbol{\mu}}_\tau^k]}(\mathit{\boldsymbol{\mu}})\right\}. \end{equation} | (9) |
For a fixed
\mathit{\boldsymbol{\mu}}_\tau(t) = (\rho_\tau(t),\eta_\tau(t)) = \mathit{\boldsymbol{\mu}}_\tau^k \qquad t\in((k-1)\tau,k\tau],\quad k\in\mathbb{N}, |
with
2. There exists an absolutely continuous curve
3. There exist a constant
\begin{equation} \int_0^T\left[||\rho_\tau(t,\cdot)||_{H^1( {\mathbb{R}^{n}})}^2+||\eta_\tau(t,\cdot)||_{H^1( {\mathbb{R}^{n}})}^2 \right]\,dt\le C(T,\mathit{\boldsymbol{\mu}}_0), \end{equation} | (10) |
and the sequence
L^{2}((0,T)\times \mathbb{R}^n)\times L^{2}((0,T)\times \mathbb{R}^n). |
The estimate in (10) can be deduced by using the so called Flow-interchange Lemma introduced in [37], see also [25]. In order to deduce the strong convergence we use the extended version of the Aubin-Lions Lemma in [44].
4. The approximating sequence
\begin{equation} \mathit{\boldsymbol{\mu}}^\epsilon = (\rho^\epsilon,\eta^\epsilon) = (P_\#^\epsilon\rho_\tau^{k+1},\eta_\tau^k), \end{equation} | (11) |
where
\begin{equation} 0\leq\frac{1}{2\tau}\left[\mathcal{W}_2^2(\mathit{\boldsymbol{\mu}}_\tau^{k+1},\mathit{\boldsymbol{\mu}}^\epsilon)- \mathcal{W}_2^2(\mathit{\boldsymbol{\mu}}_\tau^{k}, \mathit{\boldsymbol{\mu}}_\tau^{k+1})\right]+ {\mathcal{F}}_{[\mathit{\boldsymbol{\mu}}_\tau^{k}]}(\mathit{\boldsymbol{\mu}}^\epsilon)- {\mathcal{F}}_{[\mathit{\boldsymbol{\mu}}_\tau^{k}]}(\mathit{\boldsymbol{\mu}}^{\epsilon}). \end{equation} | (12) |
After some manipulations, sending first
The existence of weak solutions to the purely non-local systems, i.e.,
\begin{equation} \begin{cases} \partial_{t}\rho = {\rm{div}}\big( \rho \nabla\big( S_\rho*\rho+K_\rho*\eta \big)\big), \\ \partial_{t}\eta = {\rm{div}}\big( \eta \nabla\big( S_\eta*\eta+K_\eta*\rho \big)\big), \end{cases} \end{equation} | (13) |
with generic cross-interaction kernels
\begin{equation} \begin{cases} \partial_{t}\rho = {\rm{div}}\big( \rho \nabla\big( S_\rho*\rho+K*\eta \big)\big), \\ \partial_{t}\eta = {\rm{div}}\big( \eta \nabla\big( S_\eta*\eta-\alpha K*\rho \big)\big), \end{cases} \end{equation} | (14) |
and its relation with the particle system
\begin{equation} \begin{cases} \dot{X_i}(t) = -\sum\limits_{k = 1}^N m_X^k \nabla S_\rho(X_i(t)-X_k(t)) -\sum\limits_{k = 1}^M m_Y^k \nabla K(X_i(t)-Y_k(t)), & \\ \dot{Y_j}(t) = -\sum\limits_{k = 1}^M m_Y^k \nabla S_\eta(Y_j(t)-Y_k(t)) +\alpha\sum\limits_{k = 1}^N m_X^k \nabla K(Y_j(t)-X_k(t)). & \end{cases} \end{equation} | (15) |
It is proved that stationary states of system (14) are linear combinations of Dirac's deltas, namely
\begin{equation} \left(\bar{\rho},\bar{\eta}\right) = \left(\sum\limits_{k = 1}^{N}m_X^{k}\delta_{\bar{X}_{k}}(x),\sum\limits_{h = 1}^{M}m_Y^{h}\delta_{\bar{Y}_{h}}(x)\right). \end{equation} | (16) |
where
\begin{equation} \begin{cases} 0 = \sum\limits_{k = 1}^N \nabla S_\rho(\bar{X}_k-\bar{X}_i)m_X^k + \sum\limits_{h = 1}^M \nabla K(\bar{Y}_h-\bar{X}_i)m_Y^h & \\ 0 = \sum\limits_{h = 1}^M \nabla S_\eta(\bar{Y}_h-\bar{Y}_j)m_Y^h -\alpha \sum\limits_{k = 1}^N \nabla K(\bar{X}_k-\bar{Y}_j)m_X^k & \\ \end{cases}. \end{equation} | (17) |
for
\begin{equation} C_\alpha = \alpha\sum\limits_{i = 1}^{N}m_{X}^{i}X_{i}-\sum\limits_{j = 1}^{M}m_{Y}^{j}Y_{j} \end{equation} | (18) |
that is a conserved quantities, and therefore one would like to produce a unique steady state once the quantity
As a preliminary result, we now prove the existence of one possible shape of steady state, that will be a prototype example for the general case. The steady state is what we can call a mixed steady state, that identifies the case in which the predators can catch the prey, see Figure 2.
The proof of the existence of such steady state follows by using the strong version of the Krein-Rutman theorem, see [26].
Theorem 2.2 (Krein-Rutman). Let
(i) the spectral radius
(ii)
As pointed out in [6], using this strategy we can only obtain existence of stationary states for a diffusion coefficient that depends on the support, without providing any one-to-one correspondence between the diffusion constant (eigenvalue) and the support. Even if non completely satisfactory, the following results give a useful insight on the possible conditions we can expect in order to get existence of steady states, see Remark 3.1.
Let us first introduce a proper definition for mixed steady states as in Figure 2.
Definition 2.2. Let
I_\rho: = {\rm{supp}}(\rho) = [-R_\rho,R_\rho],\qquad {\rm{and}}\quad I_\eta: = {\rm{supp}}(\eta) = [-R_\eta,R_\eta]. |
Let us now assume that
\begin{equation} \begin{cases} \theta \rho(x) - S_\rho*\rho(x) - K*\eta(x) = C_{\rho} &\qquad x\in I_\rho \\ \theta\eta(x) - S_\eta*\eta(x)+\alpha K*\rho(x) = C_{\eta} &\qquad x\in I_\eta \end{cases}. \end{equation} | (19) |
where
\begin{equation} \begin{cases} \theta\rho_{x} = \int_{-R_\rho}^{R_\rho}S_\rho(x-y)\rho_{y}(y)dy + \int_{-R_\eta}^{R_\eta}K(x-y)\eta_{y}(y)dy &\quad\quad x\in I_\rho \\[.5cm] \theta\eta_{x} = \int_{-R_\eta}^{R_\eta}S_\eta(x-y)\eta_{y}(y)dy - \alpha\int_{-R_\rho}^{R_\rho}K(x-y)\rho_{y}(y)dy &\quad\quad x\in I_\eta \end{cases}. \end{equation} | (20) |
By symmetry properties of the kernels
\begin{equation} \begin{aligned} \theta\rho_{x} = \int_{0}^{R_\rho}\Big(S_\rho(x-y) - S_\rho(x+y&)\Big)\rho_{y}(y)dy \\ &+\int_{0}^{R_\eta}\Big(K(x-y) - K(x+y)\Big)\eta_{y}(y)dy, \\ \theta\eta_{x} = \int_{0}^{R_\eta}\Big(S_\eta(x-y) - S_\eta(x+y&)\Big)\eta_{y}(y)dy \\ &- \alpha\int_{0}^{R_\rho}\Big(K(x-y) - K(x+y)\Big)\rho_{y}(y)dy. \end{aligned} \end{equation} | (21) |
In order to simplify the notations, we set
\begin{equation*} \widetilde{G}(x,y): = G(x-y)-G(x+y),\quad{\rm{for}}\quad G = S_\rho,S_\eta,K. \end{equation*} |
Notice that
\begin{equation} \begin{cases} \theta p(x) = \int_{0}^{R_\rho}\widetilde{S}_{\rho}(x,y)p(y)dy + \int_{0}^{R_\eta}\widetilde{K}(x,y)q(y)dy \\[0.2cm] \theta q(x) = \int_{0}^{R_\eta}\widetilde{S}_{\eta}(x,y)q(y)dy - \alpha\int_{0}^{R_\rho}\widetilde{K}(x,y)p(y)dy \end{cases}. \end{equation} | (22) |
Proposition 2.1. Assume that
\begin{equation} C < \frac{\int_{0}^{R_\eta}\widetilde{S}_{\eta}(x,y)h(y)dy}{\int_{0}^{R_\rho}\widetilde{K}(x,y)k(y)dy}, \end{equation} | (23) |
for any
\alpha < \min\bigg\{ C\,,\frac{- S'_\eta(R_\eta)z_\eta}{- R_\eta^2 K''(0) z_\rho} \bigg\}, |
where
Proof. Let us first introduce the following Banach space
\begin{equation*} \mathcal{X}_{R_\rho,R_\eta} = \big\{(p,q)\in C^{1}([0,R_\rho])\times C^{1}([0,R_\eta]):p(0) = q(0) = 0\big\}, \end{equation*} |
endowed with the
\begin{equation*} T_{R_\rho,R_\eta}[P] : = (f,g)\in C^{1}([0,R_\rho])\times C^{1}([0,R_\eta]), \end{equation*} |
where
\begin{equation*} \begin{aligned} &f(x) = \int_{0}^{R_\rho}\widetilde{S}_{\rho}(x,y)p(y)dy + \int_{0}^{R_\eta}\widetilde{K}(x,y)q(y)dy &\quad{\rm{for}}\quad x\in[0,R_\rho], \\ &g(x) = \int_{0}^{R_\eta}\widetilde{S}_{\eta}(x,y)q(y)dy - \alpha\int_{0}^{R_\rho}\widetilde{K}(x,y)p(y)dy &\quad{\rm{for}}\quad x\in[0,R_\eta]. \end{aligned} \end{equation*} |
The assumptions on the kernels ensure that the operator
\begin{equation*} \mathcal{K}_{R_\rho,R_\eta} = \big\{P\in \mathcal{X}_{R_\rho,R_\eta}:p\geq0,q\geq0\big\}. \end{equation*} |
It can be shown that this set is indeed a solid cone in
\begin{equation*} \begin{aligned} \mathcal{H}_{R_\rho,R_\eta} = \big\{P\in \mathcal{K}_{R_\rho,R_\eta} &:p^{\prime}(0) > 0,\; p (x) > 0 \quad {\rm{for \;all}} \quad x\in (0,R_\rho), \quad {\rm{and}} \\ &q^{\prime}(0) > 0,\; q(x) > 0 \quad {\rm{for \;all}} \quad x\in (0,R_\eta) \big\}\subset \overset{\circ}{ \mathcal{K}_{R_\rho,R_\eta}}, \end{aligned} \end{equation*} |
where
\begin{equation} \int_{0}^{R_\eta}\widetilde{S}_{\eta}(x,y)q(y)dy - \alpha\int_{0}^{R_\rho}\widetilde{K}(x,y)p(y)dy > 0, \end{equation} | (24) |
if and only if
\begin{equation*} \begin{aligned} &\frac{d}{dx}\Big|_{x = 0}\Bigg( \int_{0}^{R_\eta}\widetilde{S}_{\eta}(x,y)q(y)dy - \alpha\int_{0}^{R_\rho}\widetilde{K}(x,y)p(y)dy \Bigg) \\ & = \int_{0}^{R_\eta}\widetilde{S}_{\eta,x}(0,y)q(y)dy - \alpha\int_{0}^{R_\rho}\widetilde{K}_{x}(0,y)p(y)dy \\ & = \int_{0}^{R_\eta}\big(S_\eta^{\prime}(-y) - S_\eta^{\prime}(y)\big)q(y)dy - \alpha\int_{0}^{R_\rho}\big(K^{\prime}(-y) - K^{\prime}(y)\big)p(y)dy \\ & = -2\int_{0}^{R_\eta}S_\eta^{\prime}(y)q(y)dy + 2\alpha\int_{0}^{R_\rho}K^{\prime}(y)p(y)dy: = A. \end{aligned} \end{equation*} |
Now, we need to find the condition on
\begin{equation*} \begin{aligned} -\frac{2}{R_\eta}\int_{0}^{R_\eta}S_\eta^{\prime}(y)q(y)dy & = -\frac{2}{R_\eta}\int_{0}^{R_\eta}S''_\eta(y)\eta(y)dy \\ &\geq \left( \frac{1}{R_\eta}\int_{0}^{R_\eta}-S''_\eta(y) dy \right) \left( \frac{2}{R_\eta}\int_{0}^{R_\eta} \eta(y)dy \right) \\ & = \frac{-S'_\eta(R_\eta) z_\eta}{R_\eta^2}. \end{aligned} \end{equation*} |
Using the concavity assumption of
\begin{equation*} -2\int_{0}^{R_\rho}K'(y)p(y)dy = -2\int_{0}^{R_\rho}K''(y)\rho(y)dy < -K''(0) z_\rho. \end{equation*} |
Thus,
\begin{equation} \alpha < \frac{- S'_\eta(R_\eta)z_\eta}{- R_\eta^2 K''(0) z_\rho}. \end{equation} | (25) |
As a consequence,
\begin{equation*} T_{R_\rho,R_\eta}[P] = \theta P, \end{equation*} |
with an eigenspace generated by one given nontrivial element
Remark 2.1. Motivated from the purely aggregative case (13), we actually expect a more rich behavior for the possible steady states configurations, such as the separated stationary state in Figure 3. This is expected as a possible winning strategy for the prey, since it corresponds to prey finding a safe distance from the predators. Unfortunately, the symmetrization argument used in the previous proof seems to fail, since in this case we need to require the symmetry around
In this Section we prove the existence and uniqueness of a multiple bumps steady state in the sense of Definition 1 fixing masses and a small diffusion coefficient. Following the approach in [5, 6], we first formulate the problem in terms of the pseudo-inverse functions and then we use the Implicit Function Theorem (cf. [19, Theorem 15.1]).
We start rewriting our stationary system in terms of pseudo-inverse functions. Let
\begin{equation} \begin{cases} 0 = \big( \rho \big( \theta\rho - S_\rho*\rho - \alpha_{\rho} K*\eta \big)_{x}\big)_{x}, \\ 0 = \big( \eta \big( \theta\eta - S_\eta*\eta - \alpha_{\eta} K*\rho \big)_{x}\big)_{x}. \end{cases} \end{equation} | (26) |
where
\int_ \mathbb{R} x\rho(x)dx = cm_{\rho}, \qquad \int_ \mathbb{R} x\eta(x)dx = cm_{\eta}. |
Remember that the only conserved quantity in the evolution, together with the masses, is the joint centre of mass
\begin{equation} CM_\alpha = \alpha cm_\rho - cm_\eta, \end{equation} | (27) |
that we can consider fixed. Define the cumulative distribution functions of
F_{\rho}(x) = \int_{-\infty}^{x}\rho(x)dx, \qquad F_{\eta}(x) = \int_{-\infty}^{x}\eta(x)dx. |
Let
u_{l}(z) = \inf \{ x\in \mathbb{R} : F_{l}(x)\geq z \}, \quad l \in \{\rho,\eta\}, |
supported on
{\rm{supp}}(u_{l}) = [0,z_{l}] : = J_{l},\qquad l \in \{\rho,\eta\}. |
For
\int\rho_{i}(x)dx = z_{\rho}^i, \quad \int\eta_{j}(x)dx = z_{\eta}^j, \quad i = 1,2,\ldots, N_\rho, \quad j = 1,2,\ldots, N_\eta, |
and the centres of masses accordingly,
\int x\rho_{i}(x)dx = cm_{\rho}^i, \quad \int x\eta_{j}(x)dx = cm_{\eta}^j, \quad \quad i = 1,2,\ldots, N_\rho, \quad j = 1,2,\ldots, N_\eta, |
and we can always assume that the centres of masses are ordered species by species, i.e.
\begin{equation} \begin{cases} \sum\limits_{j = 1}^{N_\rho} S'_\rho( cm_\rho^i - cm_\rho^j )z_\rho^j + \sum\limits_{j = 1}^{N_\eta} K'( cm_\rho^i - cm_\eta^j ) z_\eta^j = 0, \quad i = 1,\ldots,N_\rho,\\ \sum\limits_{j = 1}^{N_\eta} S'_\eta( cm_\eta^i - cm_\eta^j ) z_\eta^j - \alpha\sum\limits_{j = 1}^{N_\rho} K'( cm_\eta^i - cm_\rho^j ) z_\rho^j = 0, \quad i = 1,\ldots,N_\eta, \end{cases} \end{equation} | (28) |
coupled with the conservation of the joint centre of mass
u_l(z) = \sum\limits_{i = 1}^{N_l} u_l^i(z) \mathbb{1}_{J_l^i}(z), \quad l \in \{\rho,\eta\}, |
where
{\rm{supp}}(u_{l}) = [0,z_{l}] = J_{l} = \bigcup\limits_{i = 1}^{N_l}\left[\sum\limits_{k = 1}^{i} z_l^{k-1}, \sum\limits_{k = 1}^{i} z_l^k \right]: = \bigcup\limits_{i = 1}^{N_l}\left[\hat{z}_l^{i}, \tilde{z}_l^i \right]: = \bigcup\limits_{i = 1}^{N_l}J_l^i, l \in \{\rho,\eta\}, |
with
\begin{equation} \begin{cases} \frac{\theta}{2}\partial_{z}\Big(\big(\partial_{z}u_{\rho}(z)\big)^{-2}\Big) = \int_{J_{\rho}}S_{\rho}^{\prime}\big(u_{\rho}(z)-u_{\rho}(\xi)\big)d\xi \\ + \alpha_{\rho}\int_{J_{\eta}}K^{\prime}\big(u_{\rho}(z)-u_{\eta}(\xi)\big)d\xi,\; \; z\in J_{\rho}, \\ \frac{\theta}{2}\partial_{z}\Big(\big(\partial_{z}u_{\eta}(z)\big)^{-2}\Big) = \int_{J_{\eta}}S_{\eta}^{\prime}\big(u_{\eta}(z)-u_{\eta}(\xi)\big)d\xi \\ + \alpha_{\eta}\int_{J_{\rho}}K^{\prime}\big(u_{\eta}(z)-u_{\rho}(\xi)\big)d\xi,\; \; z\in J_{\eta}. \end{cases} \end{equation} | (29) |
The restriction to
\begin{equation} \begin{aligned} \frac{\theta}{2}\partial_{z}\Big(\big(\partial_{z}u_{l}^i(z)\big)^{-2}\Big) = \sum\limits_{j = 1}^{N_l} \int_{J_{l}^j}&S_{l}^{\prime}\big(u_{l}^i(z) - u_{l}^j(\xi)\big)d\xi \\ &+ \alpha_{l}\sum\limits_{j = 1}^{N_h}\int_{J_{h}^j}K^{\prime}\big(u_{l}^i(z) - u_{h}^j(\xi)\big)d\xi, \quad z\in J_l^i. \end{aligned} \end{equation} | (30) |
Similar to [5, 6], we suggest the linearization
u_l^i = cm_l^i + \delta v_l^i \quad i = 1,2,\ldots,N_l, \;{\rm{ and }} \;l \in \{\rho,\eta\}, |
with
\begin{equation} \begin{aligned} \frac{\delta}{2}\partial_{z}\Big(\big(\partial_{z}v_{l}^i(z)\big)^{-2}\Big) = & \sum\limits_{j = 1}^{N_l}\int_{J_{l}^j}S_{l}^{\prime}\Big(cm_l^i - cm_l^j + \delta\big( v_{l}^i(z)-v_{l}^j(\xi)\big) \Big)d\xi \\ & + \alpha_{l}\sum\limits_{j = 1}^{N_h}\int_{J_{h}^j}K^{\prime}\Big(cm_l^i - cm_h^j + \delta\big( v_{l}^i(z)-v_{h}^j(\xi) \big)\Big)d\xi. \end{aligned} \end{equation} | (31) |
Multiplying (31) by
\begin{equation} \begin{aligned} \frac{\delta^2}{\partial_{z}v_{l}^i(z)} = & \sum\limits_{j = 1}^{N_l}\int_{J_{l}^j}S_{l}\Big(cm_l^i - cm_l^j + \delta\big( v_{l}^i(z)-v_{l}^j(\xi)\big) \Big)d\xi \\ & + \alpha_{l}\sum\limits_{j = 1}^{N_h}\int_{J_{h}^j}K\Big(cm_l^i - cm_h^j + \delta\big( v_{l}^i(z)-v_{h}^j(\xi) \big)\Big)d\xi + A_l^i,\quad z\in J_l^i, \end{aligned} \end{equation} | (32) |
where
\begin{equation} \begin{aligned} A_l^i = -\sum\limits_{j = 1}^{N_l}\int_{J_{l}^j}S_{l}\Big(cm_l^i - &cm_l^j + \delta\big( \lambda_l^i - v_{l}^j(\xi)\big) \Big)d\xi \\ & - \alpha_{l}\sum\limits_{j = 1}^{N_h}\int_{J_{h}^j}K\Big(cm_l^i - cm_h^j + \delta\big( \lambda_l^i - v_{h}^j(\xi) \big)\Big)d\xi. \end{aligned} \end{equation} | (33) |
Next, we set
\begin{equation} \begin{aligned} \delta^3 z = & \sum\limits_{j = 1}^{N_l}\int_{J_{l}^j}G_{l}\Big(cm_l^i - cm_l^j + \delta\big( v_{l}^i(z)-v_{l}^j(\xi)\big) \Big)d\xi \\ & + \alpha_{l}\sum\limits_{j = 1}^{N_h}\int_{J_{h}^j}H\Big(cm_l^i - cm_h^j + \delta\big( v_{l}^i(z)-v_{h}^j(\xi) \big)\Big)d\xi + A_l^i\delta v_l^i(z) + \beta_l^i,\quad z\in J_l^i. \end{aligned} \end{equation} | (34) |
Let us denote with
\begin{equation} \begin{aligned} \beta_l^i = \, \delta^3 \bar{z}_l^i & - \sum\limits_{j = 1}^{N_l}\int_{J_{l}^j}G_{l}\big(cm_l^i - cm_l^j - \delta v_{l}^j(\xi) \big)d\xi \\ & \quad - \alpha_{l}\sum\limits_{j = 1}^{N_h}\int_{J_{h}^j}H\big(cm_l^i - cm_h^j - \delta v_{h}^j(\xi)\big)d\xi. \end{aligned} \end{equation} | (35) |
As a consequence of all above computations, we get the following relation for
\begin{equation} \begin{aligned} &\delta^3 (z - \bar{z}_l^i) \\ = & \,\sum\limits_{j = 1}^{N_l}\int_{J_{l}^j}G_{l}\Big(cm_l^i - cm_l^j + \delta\big( v_{l}^i(z)-v_{l}^j(\xi)\big) \Big) - G_l\big(cm_l^i - cm_l^j - \delta v_{l}^j(\xi) \big) \,d\xi \\ & -\delta v_l^i(z) \sum\limits_{j = 1}^{N_l}\int_{J_{l}^j}S_{l}\Big(cm_l^i - cm_l^j + \delta\big( \lambda_l^i - v_{l}^j(\xi)\big) \Big) \,d\xi \\ & + \alpha_{l}\sum\limits_{j = 1}^{N_h}\int_{J_{h}^j}H\Big(cm_l^i - cm_h^j + \delta\big( v_{l}^i(z)-v_{h}^j(\xi) \big)\Big) - H\big(cm_l^i - cm_h^j - \delta v_{h}^j(\xi)\big) \,d\xi \\ & - \delta v_l^i(z) \alpha_{l}\sum\limits_{j = 1}^{N_h}\int_{J_{h}^j}K\Big(cm_l^i - cm_h^j + \delta\big( \lambda_l^i - v_{h}^j(\xi) \big)\Big) \,d\xi . \end{aligned} \end{equation} | (36) |
If we define, for
\begin{equation} \begin{aligned} & \mathcal{F}_l^i[p;\delta](z) \\ & = \, \bar{z}_l^i - z + \, \delta^{-3}\Bigg[ \sum\limits_{j = 1}^{N_l}\int_{J_{l}^j}G_{l}\Big(cm_l^i - cm_l^j + \delta\big( v_{l}^i(z)-v_{l}^j(\xi)\big) \Big) \\ &- G_l\big(cm_l^i - cm_l^j - \delta v_{l}^j(\xi) \big) \,d\xi \\ & -\delta v_l^i(z) \sum\limits_{j = 1}^{N_l}\int_{J_{l}^j}S_{l}\Big(cm_l^i - cm_l^j + \delta\big( \lambda_l^i - v_{l}^j(\xi)\big) \Big) \,d\xi \\ & + \alpha_{l}\sum\limits_{j = 1}^{N_h}\int_{J_{h}^j}H\Big(cm_l^i - cm_h^j + \delta\big( v_{l}^i(z)-v_{h}^j(\xi) \big)\Big) \\ &- H\big(cm_l^i - cm_h^j - \delta v_{h}^j(\xi)\big) \,d\xi \\ & - \delta v_l^i(z) \alpha_{l}\sum\limits_{j = 1}^{N_h}\int_{J_{h}^j}K\Big(cm_l^i - cm_h^j + \delta\big( \lambda_l^i - v_{h}^j(\xi) \big)\Big) \,d\xi \Bigg], \quad z\in J_l^i, \end{aligned} \end{equation} | (37) |
we have that (30) reduces to the equation
\begin{equation} \begin{aligned} & \int_{J_{l}^j}G_{l}\Big(cm_l^i - cm_l^j + \delta\big( v_{l}^i(z)-v_{l}^j(\xi)\big) \Big) - G_l\big(cm_l^i - cm_l^j - \delta v_{l}^j(\xi) \big) \,d\xi \\ & = \Big[ S_l(cm_l^i - cm_l^j) \delta v_{l}^i(z) + \frac{\delta^2}{2} S'_l(cm_l^i - cm_l^j)(v_{l}^i(z))^2 \\ & \quad + \frac{\delta^3}{6} S''_l(cm_l^i - cm_l^j)(v_{l}^i(z))^3 \Big] |J_l^j| \\ & + \int_{J_{l}^j} \frac{\delta^3}{2} S''_l(cm_l^i - cm_l^j)\big( v_{l}^j(\xi) \big)^2 v_l^i(z) \,d\xi +R(S'''_l,\delta^4), \end{aligned} \end{equation} | (38) |
where we used the fact that
\begin{equation} \begin{aligned} & - \delta v_l^i(z) \int_{J_{l}^j}S_{l}\Big(cm_l^i - cm_l^j + \delta\big( \lambda_{l}^i - v_{l}^j(\xi)\big) \Big) \,d\xi \\ & = \Big[ - S_l(cm_l^i - cm_l^j) \delta v_{l}^i(z) - \delta^2 S'_l(cm_l^i - cm_l^j) \lambda_l^i v_{l}^i(z) \\ & \quad - \frac{\delta^3}{2} S''_l(cm_l^i - cm_l^j) (\lambda_l^i)^2 v_{l}^i(z) \Big] |J_l^j| \\ & - \int_{J_{l}^j} \frac{\delta^3}{2} S''_l(cm_l^i - cm_l^j)\big( v_{l}^j(\xi) \big)^2 v_l^i(z) \,d\xi+R(S'''_l,\delta^4). \end{aligned} \end{equation} | (39) |
Summing up the contributions in (38) to (39), we get that the self-interaction part in (37) reduces to
\begin{equation} \begin{aligned} &\delta^3 \Big[ \frac{\delta^{-1}}{2} S'_l( cm_l^i - cm_l^j ) v_l^i(z)(v_l^i(z)-2\lambda_l^i) \\ & + \frac{1}{6} S''_l(cm_l^i - cm_l^j) \big( (v_{l}^i(z))^3 - 3 v_{l}^i(z) (\lambda_l^i)^2 \big) \Big] |J_l^j|+R(S'''_l,\delta^4). \end{aligned} \end{equation} | (40) |
Similarly, for the cross-interaction part we obtain
\begin{equation} \begin{aligned} &\delta^3 \Big[ \frac{\delta^{-1}}{2} K'( cm_l^i - cm_h^j ) v_l^i(z)(v_l^i(z)-2\lambda_l^i) \\ & + \frac{1}{6} K''(cm_l^i - cm_h^j) \big( (v_{l}^i(z))^3 - 3 v_{l}^i(z) (\lambda_l^i)^2 \big) \Big] |J_h^j|+R(K''',\delta^4). \end{aligned} \end{equation} | (41) |
Putting together the contributions of (40) and (41) in the functional equation (37), we get
\begin{equation} \begin{aligned} \mathcal{F}_l^i[p;\delta](z) = & \, (\bar{z}_l^i - z) + \frac{D_{l}^i}{6} \Big( 3 v_{l}^i(z) (\lambda_l^i)^2 - (v_{l}^i(z))^3 \Big) \\ & + \delta^{-1} \frac{B_{l}^i}{2} v_l^i(z)(v_l^i(z)-2\lambda_l^i) +R(S'''_l,K''',\delta^4), \end{aligned} \end{equation} | (42) |
where we used the notations introduced in (6) and (5), namely
D_{l}^i = - \sum\limits_{j = 1}^{N_l} S''_l(cm_l^i - cm_l^j) |J_l^j| -\alpha_l \sum\limits_{j = 1}^{N_h} K''(cm_l^i - cm_h^j ) |J_h^j| , |
and
B_{l}^i = \sum\limits_{j = 1}^{N_l} S'_l( cm_l^i - cm_l^j ) |J_l^j| + \alpha_l\sum\limits_{j = 1}^{N_h} K'( cm_l^i - cm_h^j ) |J_h^j|. |
Note that since the values
\begin{equation} (\bar{z}_l^i - z) + \frac{D_{l}^i}{6} \Big( 3 v_{l}^i(z) (\lambda_l^i)^2 - (v_{l}^i(z))^3 \Big) = 0, \quad z\in J_l^i, \end{equation} | (43) |
that gives a unique solution once the value of
\begin{equation} \Lambda_l^i[p;\delta] = \mathcal{F}_l^i[p;\delta](\tilde{z}_l^i). \end{equation} | (44) |
Performing Taylor expansions similar to the ones in (38) and (39) we get that
\begin{equation} \begin{aligned} \Lambda_l^i[p;0] & = \, (\bar{z}_l^i - \tilde{z}_l^i) + \frac{D_{l}^i}{3} (\lambda_l^i)^3, \end{aligned} \end{equation} | (45) |
and we are now in the position to solve
\begin{equation} \begin{cases} (\bar{z}_l^i - z) + \frac{D_{l}^i}{6} \Big( 3 v_{l}^i(z) (\lambda_l^i)^2 - (v_{l}^i(z))^3 \Big) = 0, \\ (\bar{z}_l^i - \tilde{z}_l^i) + \frac{D_{l}^i}{3} (\lambda_l^i)^3 = 0. \end{cases} \end{equation} | (46) |
The second equation in (46) admits a solution once we have that
\begin{equation} \lambda_l^i = \left(\frac{3(\tilde{z}_l^i - \bar{z}_l^i)}{D_l^i} \right)^{1/3}. \end{equation} | (47) |
By construction
\begin{equation} \begin{aligned} \bar{\rho}^i(x)& = \frac{D_\rho^i}{2}\left((\lambda_\rho^i)^2-(x-cm_\rho^i)^2\right) \mathbb{1}_{I_{\rho}^i}(x), \quad i = 1,\ldots,N_\rho, \\ \bar{\eta}^h(x)& = \frac{D_\eta^h}{2}\left((\lambda_\eta^h)^2-(x-cm_\eta^h)^2\right) \mathbb{1}_{I_{\eta}^h}(x),\quad h = 1,\ldots,N_\eta, \end{aligned} \end{equation} | (48) |
where the intervals
l_k^{i_k} = cm_k^{i_k}-\lambda_k^{i_k}, \quad r_k^{i_k} = cm_k^{i_k}+\lambda_k^{i_k}, \quad i_k = 1,\ldots,N_k,\,k = \rho,\eta. |
We are now ready to reformulate (36) as a functional equation on a proper Banach space. Consider the spaces
\begin{equation} \Omega_l^i = \left\{v \in L^\infty\left(\left[\bar{z}_l^i,\tilde{z}_l^i\right)\right)\,|\, v \;{\rm{ increasing}},\, v(\bar{z}_l^i) = 0\right\},\, i = 1,\ldots,N_l,\, l\in\{\rho,\eta\}, \end{equation} | (49) |
endowed with the
\Omega_l = \mathop \times \limits_{i = 1}^{N_l}\Omega_l^i,\,{\rm{ for }}\; l\in\{\rho,\eta\}. |
We now introduce the space
\begin{equation} \Omega = \Omega_\rho \times \mathbb{R}^{N_\rho}\times\Omega_\eta \times \mathbb{R}^{N_\eta}, \end{equation} | (50) |
with elements
\begin{equation} |||\omega ||| = \sum\limits_{i = 1}^{N_\rho}\Big(\|v_{\rho}^i\|_{L^{\infty}}+ |\lambda_{\rho}^i| \Big)+ \sum\limits_{i = 1}^{N_\eta}\Big(\|v_{\eta}^i\|_{L^{\infty}} + |\lambda_{\eta}^i|\Big). \end{equation} | (51) |
For
\begin{equation} ||| \omega|||_{\gamma} = ||| \omega||| + \sum\limits_{l\in \{ \rho,\eta \} }\sum\limits_{i = 1}^{N_l} \sup\limits_{z\in \tilde{J}_l^i}\frac{|\lambda_l^i-v_l^i(z)|}{(\tilde{z}_l^i - z)^{\gamma}}, \end{equation} | (52) |
and set
\begin{equation} \mathcal{T}[\omega;\delta](z) : = \begin{pmatrix} \mathcal{F}_{\rho}[\omega;\delta](z) \\[0.2cm] \Lambda_{\rho}[\omega;\delta] \\[0.2cm] \mathcal{F}_{\eta}[\omega;\delta](z) \\[0.2cm] \Lambda_{\eta}[\omega;\delta] \end{pmatrix}, \end{equation} | (53) |
where for
\begin{equation} \begin{aligned} & {\mathcal{F}}_{l}[\omega;\delta](z) = \left( {\mathcal{F}}_l^1[\omega;\delta](z),\ldots, {\mathcal{F}}_l^{N_l}[\omega;\delta](z) \right), \\ &\Lambda_{l}[\omega;\delta](z) : = \left( \Lambda_l^1[\omega;\delta],\ldots,\Lambda_l^{N_l}[\omega;\delta] \right). \end{aligned} \end{equation} | (54) |
The operator
\begin{equation} D\mathcal{T}[\omega;\delta] = \begin{pmatrix} D_{v_\rho} {\mathcal{F}}_\rho(\delta) & D_{\lambda_\rho} {\mathcal{F}}_\rho(\delta) & D_{v_\eta} {\mathcal{F}}_\rho(\delta) & D_{\lambda_\eta} {\mathcal{F}}_\rho(\delta) \\[0.2 cm] D_{v_\rho}\Lambda_\rho(\delta) & D_{\lambda_\rho}\Lambda_\rho(\delta) & D_{v_\eta}\Lambda_\rho(\delta) & D_{\lambda_\eta}\Lambda_\rho(\delta) \\[0.2 cm] D_{v_\rho} {\mathcal{F}}_\eta(\delta) & D_{\lambda_\rho} {\mathcal{F}}_\eta(\delta) & D_{v_\eta} {\mathcal{F}}_\eta(\delta) & D_{\lambda_\eta} {\mathcal{F}}_\eta(\delta) \\[0.2 cm] D_{v_\rho}\Lambda_\eta(\delta) & D_{\lambda_\rho}\Lambda_\eta(\delta) & D_{v_\eta}\Lambda_\eta(\delta) & D_{\lambda_\eta}\Lambda_\eta(\delta) \end{pmatrix}, \end{equation} | (55) |
where the components are actually matrices defined by
\begin{equation*} \begin{aligned} &D_{v_h} {\mathcal{F}}_l(\delta) = \left(\frac{ \partial {\mathcal{F}}_l^i[\omega;\delta]}{\partial v_h^j }(\nu_h^j) \right)_{i,j = 1}^{N_l,N_h}, \quad D_{\lambda_h} {\mathcal{F}}_l(\delta) = \left(\frac{ \partial {\mathcal{F}}_l^i[\omega;\delta]}{\partial \lambda_h ^j}(a_h^j) \right)_{i,j = 1}^{N_l,N_h} \\ &D_{v_h}\Lambda_l(\delta) = \left(\frac{ \partial\Lambda_l^i[\omega;\delta]}{\partial v_h ^j}(\nu_h^j) \right)_{i,j = 1}^{N_l,N_h}, \quad D_{\lambda_h}\Lambda_l(\delta) = \left(\frac{ \partial\Lambda_l^i[\omega;\delta]}{\partial \lambda_h^j }(a_h^j) \right)_{i,j = 1}^{N_l,N_h}, \end{aligned} \end{equation*} |
where
\begin{equation*} \begin{aligned} & \frac{\partial {\mathcal{F}}_l^i[\omega;\delta]}{\partial v_l^i}(\nu_l^i) \\ & = -\delta ^{-2}\int_{J_{l}^i} \nu_{l}^i(\xi) \Bigg [S_{l}\Big( \delta\big( v_{l}^i(z)-v_{l}^i(\xi)\big) \Big) - \delta v_l^i(z) S'_{l}\Big(\delta\big( \lambda_l^i - v_{l}^i(\xi)\big) \Big) \\ & - S_l\Big( -\delta v_{l}^i(\xi) \Big) \Bigg] \,d\xi \\ & + \delta^{-2}\nu_{l}^i(z) \sum\limits_{j = 1 }^{N_l}\int_{J_{l}^j}\Bigg[S_{l}\Big(cm_l^i - cm_l^j + \delta\big( v_{l}^i(z)-v_{l}^j(\xi)\big) \Big) \\ & - S_{l}\Big(cm_l^i - cm_l^j + \delta\big( \lambda_l^i - v_{l}^j(\xi)\big) \Big) \Bigg] \,d\xi \\ & + \delta^{-2}\nu_{l}^i(z)\alpha_{l}\sum\limits_{j = 1}^{N_h}\int_{J_{h}^j}\Bigg[K\Big(cm_l^i - cm_h^j + \delta\big( v_{l}^i(z)-v_{h}^j(\xi) \big)\Big) \\ & - K\Big(cm_l^i - cm_h^j + \delta\big( \lambda_l^i - v_{h}^j(\xi) \big)\Big)\Bigg]d\xi. \end{aligned} \end{equation*} |
A Taylor expansion around
\begin{equation*} \frac{\partial {\mathcal{F}}_l^i[\omega;0]}{\partial v_l^i}(\nu_l^i) = \, \frac{D_l^i}{2} \Big( (\lambda_l^i)^2 - (v_{l}^i(z))^2 \Big) \nu_{l}^i(z). \end{equation*} |
Concerning the other terms in
\begin{equation*} \begin{aligned} &\frac{\partial {\mathcal{F}}_l^i[\omega;\delta]}{\partial v_l^j}(\nu_l^j) \\ & = -\delta ^{-2}\sum\limits_{j = 1}^{N_l}\int_{J_{l}^j}\nu_{l}^j(\xi)\Bigg[ S_{l}\Big( cm_l^i - cm_l^j+\delta\big( v_{l}^i(z)-v_{l}^j(\xi)\big) \Big) \\ & -S_l\Big( (cm_l^i - cm_l^j-\delta v_{l}^j(\xi) \Big) -\delta v_l^i(z)S'_{l}\Big(cm_l^i - cm_l^j+\delta\big( \lambda_l^i - v_{l}^j(\xi)\big) \Big) \Bigg] \,d\xi, \end{aligned} \end{equation*} |
that all vanish in the limit
\begin{equation*} \begin{aligned} \frac{\partial {\mathcal{F}}_l^i[\omega;\delta]}{\partial \lambda_l^i}(a_l^i) = \, -\delta^{-1}v_l^i(z)a_l^i\Bigg[ & \sum\limits_{j = 1}^{N_l}\int_{J_{l}^j}S'_{l}\Big(cm_l^i - cm_l^j + \delta\big( \lambda_l^i - v_{l}^j(\xi)\big) \Big) \,d\xi \\ & + \alpha_{l}\sum\limits_{j = 1}^{N_h}\int_{J_{h}^j}K'\Big(cm_l^i - cm_h^j + \delta\big( \lambda_l^i - v_{h}^j(\xi) \big)\Big) \,d\xi \Bigg]. \end{aligned} \end{equation*} |
Then, Taylor expansion w.r.t.
\begin{equation*} \begin{aligned} \frac{\partial {\mathcal{F}}_l^i[\omega;0]}{\partial \lambda_l^i}(a_l^i) = \, D_l^i \lambda_l^i v_{l}^i(z) a_l^i. \end{aligned} \end{equation*} |
Since all the entrances in the matrix
\begin{equation*} \begin{aligned} & \frac{\partial {\mathcal{F}}_l^i[\omega;\delta]}{\partial v_h^j}(\nu_h^j) \\ & = -\delta ^{-2}\alpha_l\sum\limits_{j = 1}^{N_h}\int_{J_{h}^j}\nu_{h}^j(\xi)\Bigg[ K\Big( cm_l^i - cm_h^j+\delta\big( v_{l}^i(z)-v_{h}^j(\xi)\big) \Big) \\ &-K\Big( cm_l^i - cm_h^j-\delta v_{h}^j(\xi) \Big) - \delta v_l^i(z)K'\Big(cm_l^i - cm_h^j+\delta\big( \lambda_l^i - v_{h}^j(\xi)\big) \Big) \Bigg] \,d\xi, \end{aligned} \end{equation*} |
that vanish in the limit
\begin{equation*} \begin{aligned} &\frac{\partial\Lambda_l^i[\omega;\delta]}{\partial v_l^j}(\nu_l^j) \\ & = -\delta ^{-2}\sum\limits_{j = 1}^{N_l}\int_{J_{l}^j}\nu_{l}^j(\xi)\Bigg[ S_{l}\Big( cm_l^i - cm_l^j+\delta\big( \lambda_{l}^i(z)-v_{l}^j(\xi)\big) \Big) \\ &-S_l\Big( (cm_l^i - cm_l^j-\delta v_{l}^j(\xi) \Big) -\delta\lambda_l^i S'_{l}\Big(cm_l^i - cm_l^j+\delta\big( \lambda_l^i - v_{l}^j(\xi)\big) \Big) \Bigg] \,d\xi. \end{aligned} \end{equation*} |
The terms in
\begin{equation*} \begin{aligned} & \frac{\partial\Lambda_l^i[\omega;\delta]}{\partial v_h^j}(\nu_h^j) \\ & = -\delta ^{-2}\alpha_l\sum\limits_{j = 1}^{N_h}\int_{J_{h}^j}\nu_{h}^j(\xi)\Bigg[ K\Big( cm_l^i - cm_h^j+\delta\big( \lambda_{l}^i-v_{h}^j(\xi)\big) \Big) \\ &-K\Big( cm_l^i - cm_h^j-\delta v_{h}^j(\xi) \Big) -\delta\lambda_l^i K'\Big(cm_l^i - cm_h^j+\delta\big( \lambda_l^i - v_{h}^j(\xi)\big) \Big) \Bigg] \,d\xi, \end{aligned} \end{equation*} |
and the usual Taylor expansions around
\begin{equation*} \begin{aligned} \frac{\partial\Lambda_l^i[\omega;\delta]}{\partial \lambda_l^i}(a_l^i)& = - \delta^{-1}a_l^i \sum\limits_{j = 1}^{N_l}\int_{J_{l}^j} \lambda_l^i S'_{l}\Big(cm_l^i - cm_l^j + \delta\big( \lambda_l^i - v_{l}^j(\xi)\big) \Big) \,d\xi \\ & - \delta^{-1}a_l^i\alpha_l \sum\limits_{j = 1}^{N_h}\int_{J_{h}^j} \lambda_l^i K'_{l}\Big(cm_l^i - cm_h^j + \delta\big( \lambda_l^i - v_{h}^j(\xi)\big) \Big) \,d\xi. \end{aligned} \end{equation*} |
The last Taylor expansion gives
\begin{equation*} \frac{\partial\Lambda_l^i[\omega;0]}{\partial \lambda_l^i}(a_l^i) = D_l^i (\lambda_l^i)^2 a_l^i. \end{equation*} |
We have proved that
\begin{equation} D\mathcal{T}[\omega;0] = \begin{pmatrix} El_1 & \mathrm{dg} \left(D_{\rho}^i \lambda_{\rho}^i v_{\rho}^i a_{\rho}^i \right) & 0 & 0 \\[0.3cm] 0 & \mathrm{dg} \left(D_{\rho}^i (\lambda_{\rho}^i)^2 a_{\rho}^i \right) & 0 & 0 \\[0.3cm] 0 & 0 & El_2 & \mathrm{dg} \left(D_{\eta}^i \lambda_{\eta}^i v_{\eta}^i a_{\eta}^i \right) \\[0.3cm] 0 & 0 & 0 & \mathrm{dg} \left(D_{\eta}^i (\lambda_{\eta}^i)^2 a_{\eta}^i \right) \end{pmatrix}, \end{equation} | (56) |
where
El_1 = \mathrm{dg}\left(\frac{D_{\rho}^i}{2} \Big( (\lambda_{\rho}^i)^2 - (v_{\rho}^i)^2 \Big) \nu_{\rho}^i \right) \,, \\ El_2 = \mathrm{dg} \left(\frac{D_{\eta}^i}{2} \Big( (\lambda_{\eta}^i)^2 - (v_{\eta}^i)^2 \Big) \nu_{\eta}^i \right)\,, |
and
Lemma 3.1. For
Proof. Thanks to the previous computations it is easy to see that
\begin{equation} \begin{aligned} \sup\limits_{|||\omega|||_{1/2}\leq 1}&\frac{1}{(\tilde{z}_l^i - z)}\bigg| \frac{\partial \mathcal{F}_l^i}{\partial v_l^i}[\cdot,\delta](\nu_l^i) -\frac{\partial \Lambda_l^i}{\partial v_l^i}[\cdot;\delta](\nu_l^i) -\bigg( \frac{\partial \mathcal{F}_l^i}{\partial v_l^i}[\cdot;0](\nu_l^i) -\frac{\partial \Lambda_l^i}{\partial v_l^i}[\cdot;0](\nu_l^i) \bigg) \\ &+\frac{\partial \mathcal{F}_l^i}{\partial \lambda_l^i}[\cdot,\delta](a_l^i) -\frac{\partial \Lambda_l^i}{\partial \lambda_l^i}[\cdot;\delta](a_l^i) -\bigg( \frac{\partial \mathcal{F}_l^i}{\partial \lambda_l^i}[\cdot;0](a_l^i) -\frac{\partial \Lambda_l^i}{\partial \lambda_l^i}[\cdot;0](a_l^i) \bigg) \\ &+\frac{\partial \mathcal{F}_l^i}{\partial v_h^j}[\cdot,\delta](\nu_h^j) -\frac{\partial \Lambda_l^i}{\partial v_h^j}[\cdot;\delta](\nu_h^j) -\bigg( \frac{\partial \mathcal{F}_l^i}{\partial v_h^j}[\cdot;0](\nu_h^j) -\frac{\partial \Lambda_l^i}{\partial v_h^j}[\cdot;0](\nu_h^j) \bigg) \\ &+\frac{\partial \mathcal{F}_l^i}{\partial \lambda_h^j}[\cdot,\delta](a_h^j) -\frac{\partial \Lambda_l^i}{\partial \lambda_h^j}[\cdot;\delta](a_h^j) -\bigg( \frac{\partial \mathcal{F}_l^i}{\partial \lambda_h^j}[\cdot;0](a_h^j) -\frac{\partial \Lambda_l^i}{\partial \lambda_h^j}[\cdot;0](a_h^j) \bigg) \bigg| \searrow 0, \end{aligned} \end{equation} | (57) |
as
\begin{equation*} \begin{aligned} & \frac{1}{(\tilde{z}_l^i - z)}\Bigg[ \frac{\partial \mathcal{F}_l^i}{\partial v_h^j}[\cdot,\delta](\nu_h^j) -\frac{\partial \Lambda_l^i}{\partial v_h^j}[\cdot;\delta](\nu_h^j) -\bigg( \frac{\partial \mathcal{F}_l^i}{\partial v_h^j}[\cdot;0](\nu_h^j) -\frac{\partial \Lambda_l^i}{\partial v_h^j}[\cdot;0](\nu_h^j) \bigg)\Bigg]\\ = & -\alpha_l \frac{(v_l^i(z)-\lambda_l^i)^2}{\tilde{z}_l^i-z}\sum\limits_{j = 1}^{N_h}\int_{J_h^j}\frac{K''\big(cm_l^i - cm_h^j + \delta(\lambda_l^i - v_h^j(\xi))\big)\nu_h^j(\xi)}{2} \, d\xi \\ &- \delta\alpha_l \frac{(v_l^i(z)-\lambda_l^i)^3}{\tilde{z}_l^i-z}\sum\limits_{j = 1}^{N_h}\int_{J_h^j}\frac{K'''(\tilde{x}(\xi))\nu_h^j(\xi)}{6} \, d\xi \\ = & -\delta\alpha_l \frac{(v_l^i(z)-\lambda_l^i)^2}{\tilde{z}_l^i-z}\sum\limits_{j = 1}^{N_h}\int_{J_h^j}\frac{K'''(\bar{x}(\xi))(\lambda_l^i-v_h^j(\xi))\nu_h^j(\xi)}{2} \, d\xi \\ &-\delta\alpha_l \frac{(v_l^i(z)-\lambda_l^i)^3}{\tilde{z}_l^i-z}\sum\limits_{j = 1}^{N_h}\int_{J_h^j}\frac{K'''(\tilde{x}(\xi))\nu_h^j(\xi)}{6} \, d\xi. \\ = &-\alpha_l \left( \frac{(v_l^i(z)-\lambda_l^i)^2}{\tilde{z}_l^i-z} +\frac{(v_l^i(z)-\lambda_l^i)^3}{\tilde{z}_l^i-z} \right) O(\delta), \end{aligned} \end{equation*} |
where in the first equality we did a Taylor expansion around the point
\begin{equation*} \begin{aligned} & \frac{1}{(\tilde{z}_l^i - z)}\Bigg[ \frac{\partial \mathcal{F}_l^i}{\partial \lambda_h^j}[\cdot,\delta](a_h^j) -\frac{\partial \Lambda_l^i}{\partial \lambda_h^j}[\cdot;\delta](a_h^j) -\bigg( \frac{\partial \mathcal{F}_l^i}{\partial \lambda_h^j}[\cdot;0](a_h^j) -\frac{\partial \Lambda_l^i}{\partial \lambda_h^j}[\cdot;0](a_h^j) \bigg)\Bigg] = 0. \end{aligned} \end{equation*} |
The first two rows in (57) can be treated as follows,
\begin{equation*} \begin{aligned} & \frac{1}{(\tilde{z}_l^i - z)} \Bigg[ \frac{\partial \mathcal{F}_l^i}{\partial v_l^i}[\cdot,\delta](\nu_l^i) -\frac{\partial \Lambda_l^i}{\partial v_l^i}[\cdot;\delta](\nu_l^i) -\bigg( \frac{\partial \mathcal{F}_l^i}{\partial v_l^i}[\cdot;0](\nu_l^i) -\frac{\partial \Lambda_l^i}{\partial v_l^i}[\cdot;0](\nu_l^i) \bigg) \\ &+\frac{\partial \mathcal{F}_l^i}{\partial \lambda_l^i}[\cdot,\delta](a_l^i) -\frac{\partial \Lambda_l^i}{\partial \lambda_l^i}[\cdot;\delta](a_l^i) -\bigg( \frac{\partial \mathcal{F}_l^i}{\partial \lambda_l^i}[\cdot;0](a_l^i) -\frac{\partial \Lambda_l^i}{\partial \lambda_l^i}[\cdot;0](a_l^i) \bigg) \Bigg] \\ & = \, \frac{\delta}{(\tilde{z}_l^i - z)} \Bigg[ \sum\limits_{j = 1}^{N_l} \int_{J_l^j} \delta^{-2} (v_l^i(z) - \lambda_l^i)(\nu_l^i(z) - a_l^i) S'_l\big(cm_l^i - cm_l^j + \delta(\lambda_l^i - v_l^j(\xi))\big) \\ &\qquad + \delta^{-1}\frac{1}{2} (v_l^i(z) - \lambda_l^i)^2(\nu_l^i(z) - \nu_l^j(\xi)) S''_l\big(cm_l^i - cm_l^j + \delta(\lambda_l^i - v_l^j(\xi))\big) \\ & \qquad + \frac{1}{6} (v_l^i(z) - \lambda_l^i)^3(\nu_l^i(z) - \nu_l^j(\xi)) S'''_l\big(\tilde{x}_1(\xi)\big) \, d\xi \\ &+\alpha_l\sum\limits_{j = 1}^{N_h} \int_{J_h^j} \delta^{-2} (v_l^i(z) - \lambda_l^i)(\nu_l^i(z) - a_l^i) K'\big(cm_l^i - cm_h^j + \delta(\lambda_l^i - v_h^j(\xi))\big) \\ &\qquad + \delta^{-1}\frac{1}{2} (v_l^i(z) - \lambda_l^i)^2(\nu_l^i(z) - \nu_l^j(\xi)) K''\big(cm_l^i - cm_h^j + \delta(\lambda_l^i - v_h^j(\xi))\big) \\ & \qquad + \frac{1}{6} (v_l^i(z) - \lambda_l^i)^3(\nu_l^i(z) - \nu_l^j(\xi)) K'''\big(\tilde{x}_2(\xi)\big) \, d\xi \Bigg] \\ & = \, \Bigg( 2 \frac{(\nu_l^i(z) - a_l^i) (v_l^i(z) - \lambda_l^i)}{(\tilde{z}_l^i - z)} \\ & + (2\nu_l^i(z) - 1) \bigg( \frac{(v_l^i(z) - \lambda_l^i)^3}{(\tilde{z}_l^i - z)} + \frac{(v_l^i(z) - \lambda_l^i)^2}{(\tilde{z}_l^i - z)} \bigg) \Bigg) O(\delta). \end{aligned} \end{equation*} |
Since the functions
\frac{\lambda_l^i-v_l^i(z)}{(\tilde{z}_l^i - z)^{1/2}}, |
are uniformly bounded in
Lemma 3.2. For any
Proof. Given
\begin{equation} D\mathcal{T}[\omega_0;0] \omega = w, \end{equation} | (58) |
admits a unique solution
\begin{equation*} ||\omega||_{1/2}\leq C ||w||_{1}. \end{equation*} |
The determinant of the matrix in (56) is given by
\begin{equation} \det D\mathcal{T} = \left(\prod\limits_{i = 1}^{N_{\rho}}\frac{(D_{\rho}^i)^2}{2} \Big( (\lambda_{\rho}^i)^2 - (v_{\rho}^i)^2 \Big) (\lambda_{\rho}^i)^2 \right) \cdot \left(\prod\limits_{i = 1}^{N_{\eta}}\frac{(D_{\eta}^i)^2}{2} \Big( (\lambda_{\eta}^i)^2 - (v_{\eta}^i)^2 \Big) (\lambda_{\eta}^i)^2 \right) , \end{equation} | (59) |
that is always different from zero under the condition
\begin{equation*} \begin{aligned} \nu_l^i(z) & = \frac{- 2 \sigma_l^i(z)}{D_l^i \big( (v_l^i(z))^2 - (\lambda_l^i)^2 \big)} + \frac{2 \lambda_l^i v_l^i(z) a_l^i}{ (v_l^i(z))^2 - (\lambda_l^i)^2 }, \\ a_l^i & = \frac{k_l^i}{D_l^i(\lambda_l^i)^2}, \end{aligned} \end{equation*} |
that implies
\begin{equation*} \frac{a_l^i-\nu_l^i(z)}{(\tilde{z}_l^i - z)^{1/2}} , \end{equation*} |
is uniformly bounded since
We are now in the position of proving the main result of the paper, namely Theorem 1.1, that we recall below for convenience.
Theorem 3.1. Assume that
(i)
\begin{equation} B_{l}^i = \sum\limits_{j = 1}^{N_l} S'_l( cm_l^i - cm_l^j ) z_l^j+ \alpha_l\sum\limits_{j = 1}^{N_h} K'( cm_l^i - cm_h^j ) z_h^j = 0, \end{equation} | (60) |
(ii) the following quantities
\begin{equation} D_{l}^i = - \sum\limits_{j = 1}^{N_l} S''_l(cm_l^i - cm_l^j) z_l^j -\alpha_l \sum\limits_{j = 1}^{N_h} K''(cm_l^i - cm_h^j ) z_h^j, \end{equation} | (61) |
are strictly positive, for all
where
where
● each interval
●
● the solutions
z_\rho = \sum\limits_{i = 1}^{N_\rho} z_\rho^i \;\mathit{and}\; z_\eta = \sum\limits_{i = 1}^{N_\eta} z_\eta^i, |
respectively.
Proof. Consider
\mathcal{T}[\omega;\delta](z) = 0, |
admits a unique solution
\omega = ( v_\rho^1(z),\ldots,v_\rho^{N_\rho}(z),\lambda_\rho^1,\ldots,\lambda_\rho^{N_\rho},v_\eta^1(z),\ldots,v_\eta^{N_\eta}(z),\lambda_\eta^1,\ldots,\lambda_\eta^{N_\eta} )\,, |
for
Remark 3.1 Note that conditions (ii) in Theorem 3.1 turn to be conditions on the positions of the centres of masses and on the value of
\alpha < \min\limits_{i = 1,\ldots,N_\eta}\frac{ \sum\limits_{j = 1}^{N_\eta} S''_\eta(cm_\eta^i - cm_\eta^j) |J_\eta^j|}{ \sum\limits_{j = 1}^{N_\rho} K''(cm_\eta^i - cm_\rho^j ) |J_\rho^j| }. |
Note that the above conditions are comparable to the one we got in the proof of Section 2 using the Krein-Rutman approach.
In this section, we study numerically solutions to system (3) using two different methods, the finite volume method introduced in [12, 13] and the particles method studied in [23, 28]. We validate the results about the existence of the mixed steady state and the multiple bumps steady states. Moreover, we perform some examples to show the variation in the behaviour of the solution to system (3) under different choices of initial data and the parameter
\begin{equation} \begin{cases} \partial_t u_{\rho}(z) = &-\frac{\theta}{2}\partial_{z}\Big(\big(\partial_{z}u_{\rho}(z)\big)^{-2}\Big) + \int_{J_{\rho}}S_{\rho}^{\prime}\big(u_{\rho}(z)-u_{\rho}(\xi)\big)d\xi \\ &+ \alpha_{\rho}\int_{J_{\eta}}K^{\prime}\big(u_{\rho}(z)-u_{\eta}(\xi)\big)d\xi,\; \; z\in J_{\rho} \\ \partial_t u_{\eta}(z) = &-\frac{\theta}{2}\partial_{z}\Big(\big(\partial_{z}u_{\eta}(z)\big)^{-2}\Big) + \int_{J_{\eta}}S_{\eta}^{\prime}\big(u_{\eta}(z)-u_{\eta}(\xi)\big)d\xi \\ &+ \alpha_{\eta}\int_{J_{\rho}}K^{\prime}\big(u_{\eta}(z)-u_{\rho}(\xi)\big)d\xi,\; \; z\in J_{\eta}, \end{cases} \end{equation} | (62) |
as the following: Let
\begin{equation} \begin{cases} \partial_t X_{\rho}^i(t) = & \frac{\theta}{2N} \Big(\big(\rho^{i-1}(t)\big)^{-2} - \big(\rho^{i}(t)\big)^{-2} \Big) + \frac{1}{N}\sum\limits_{j = 1}^N S_{\rho}^{\prime}\big(X_{\rho}^i(t)-X_{\rho}^j(t)\big)d\xi \\ & + \frac{\alpha_{\rho}}{N}\sum\limits_{j = 1}^N K^{\prime}\big(X_{\rho}^i(t)-X_{\eta}^j(t)\big)d\xi, \qquad i = 1,\cdots N, \\ \partial_t X_{\eta}^i(t) = & \frac{\theta}{2N} \Big(\big(\eta^{i-1}(t)\big)^{-2} - \big(\eta^{i}(t)\big)^{-2} \Big) + \frac{1}{N}\sum\limits_{j = 1}^N S_{\eta}^{\prime}\big(X_{\eta}^i(t)-X_{\eta}^j(t)\big)d\xi \\ & + \frac{\alpha_{\eta}}{N}\sum\limits_{j = 1}^N K^{\prime}\big(X_{\eta}^i(t)-X_{\rho}^j(t)\big)d\xi, \qquad i = 1,\cdots N, \end{cases} \end{equation} | (63) |
where the densities are reconstructed as
\begin{equation} \begin{cases} \rho^i(t) = \frac{1}{N ( X_l^{i+1}(t) - X_l^i(t) )}, \qquad i = 1,\cdots, N-1, \\ \rho^0(t) = 0, \\ \rho^N(t) = 0, \end{cases} \end{equation} | (64) |
and the same for the
\int_{X_{\rho,0}^i}^{X_{\rho,0}^{i+1}} \rho(t = 0) dX = \frac{1}{N-1}, \qquad i = 1,\cdots,N-1. |
The second method we use is the finite volume method which introduced in [12] and extended to systems in [13], that consists in a
\begin{equation*} \widetilde{\rho}_{i}(t) : = \frac{1}{\Delta x}\int_{U_{i}}\rho(x,t)dx,\qquad \widetilde{\eta}_{i}(t) : = \frac{1}{\Delta x}\int_{U_{i}}\eta(x,t)dx, \end{equation*} |
the averages of the solutions
\begin{equation} \begin{cases} \frac{d\widetilde{\rho}_{i}(t)}{dt} = -\frac{F_{i+\frac{1}{2}}^{\rho}(t) - F_{i-\frac{1}{2}}^{\rho}(t) }{\Delta x}, \\ \frac{d\widetilde{\eta}_{i}(t)}{dt} = -\frac{F_{i+\frac{1}{2}}^{\eta}(t) - F_{i-\frac{1}{2}}^{\eta}(t) }{\Delta x}, \end{cases} \end{equation} | (65) |
where the numerical flux
\begin{equation} F_{i+\frac{1}{2}}^{\rho} = \max (\vartheta_{\rho}^{i+1},0)\Big[ \widetilde{\rho}_{i} + \frac{\Delta x}{2}(\rho_{x})_{i} \Big] + \min (\vartheta_{\rho}^{i+1},0)\Big[ \widetilde{\rho}_{i} - \frac{\Delta x}{2}(\rho_{x})^{i} \Big], \end{equation} | (66) |
where
\begin{equation} \begin{aligned} \vartheta_{\rho}^{i+1} = &- \frac{\theta}{\Delta x} \big( \widetilde{\rho}_{i+1} - \widetilde{\rho}_{i} \big) - \sum\limits_{j}\widetilde{\rho}_{j}\big( S_{\rho}(x_{i+1} - x_{j}) - S_{\rho}(x_{i} - x_{j}) \big) \\ &- \alpha_{\rho} \sum\limits_{j}\widetilde{\eta}_{j}\big( K(x_{i+1} - x_{j}) - K(x_{i} - x_{j}) \big), \end{aligned} \end{equation} | (67) |
and
\begin{equation} (\rho_{x})^{i} = {\rm{minmod}} \Bigg( 2\frac{\widetilde{\rho}_{i+1} - \widetilde{\rho}_{i}}{\Delta x},\; \frac{\widetilde{\rho}_{i+1} - \widetilde{\rho}_{i-1}}{2\Delta x},\; 2\frac{\widetilde{\rho}_{i} - \widetilde{\rho}_{i-1}}{\Delta x} \Bigg). \end{equation} | (68) |
The minmod limiter in (68) has the following definition
\begin{equation} {\rm{minmod}}(a_{1}, a_{2},\; \dots) : = \begin{cases} \min (a_{1}, a_{2},\; \dots), \quad {\rm{if}} \; a_{i} > 0\quad \forall i \\ \max (a_{1}, a_{2},\; \dots), \quad {\rm{if}} \; a_{i} < 0\quad \forall i \\ 0, \qquad\qquad\qquad{\rm{otherwise.}} \end{cases} \end{equation} | (69) |
We have the same as the above expresions for
In all the simulations below, we will fix the kernels as a normalised Gaussian potentials
S_{\rho}(x) = S_{\eta}(x) = K(x) = \frac{1}{\sqrt{\pi}} e^{-x^2}, |
that are under the assumptions on the kernels (A1), (A2) and (A3). This choice helps us in better understanding the variation in the behavior of the solutions w.r.t. the change in the initial data and the parameter
This figure shows how from the initial densities
● in the first row steady states are plotted at the level of density, on the l.h.s. we compare the two methods illustrated above, while on the r.h.s. we show the evolution by the finite volume method,
● in the second row we plot the particles paths for both species obtained with the particles method,
● in the last row we show the pseudo inverse functions corresponding to the steady state densities.
The last example we present shows an interesting traveling waves-type evolution.
The first example is devoted to validating existence of mixed steady state and separated steady state. By choosing the initial data
(70) |
and fixing
(71) |
with the same
We then, test two cases where we validate the existence of steady states of more bumps. Let us fix
(72) |
as initial data and we take
(73) |
and
Finally, in this example, we detect existence of traveling waves. Indeed, by choosing initial data as
(74) |
[1] | J. H. Holland, Genetic Algorithms, Sci. Am., 267 (1992), 66-73. https://doi.org/10.1038/scientificamerican0792-66 |
[2] |
S. Kirkpatrick, C. D. Gelatt, M. P. Vecchi, Optimization by simulated annealing, Science, 220 (1983), 671-680. https://doi.org/10.1126/science.220.4598.671 doi: 10.1126/science.220.4598.671
![]() |
[3] |
A. Faramarzi, M. Heidarinejad, B. Stephens, S. Mirjalili, Equilibrium optimizer: a novel optimization algorithm, Knowledge Based Syst., 191 (2020), 105190. https://doi.org/10.1016/j.knosys.2019.105190 doi: 10.1016/j.knosys.2019.105190
![]() |
[4] |
R. V. Rao, V. J. Savsani, D. P. Vakharia, Teaching-learning-based optimization: a novel method for constrained mechanical design optimization problems, Comput.-Aided Des., 43 (2011), 303-315. https://doi.org/10.1016/j.cad.2010.12.015 doi: 10.1016/j.cad.2010.12.015
![]() |
[5] | E. Atashpaz-Gargari, C. Lucas, Imperialist competitive algorithm: an algorithm for optimization inspired by imperialistic competition, in 2007 IEEE Congress on Evolutionary Computation, (2007), 4661-4667. https://doi.org/10.1109/CEC.2007.4425083 |
[6] |
Q. Zhang, R. Wang, J. Yang, K. Ding, Y. Li, J. Hu, Collective decision optimization algorithm: a new heuristic optimization method, Neurocomputing, 221 (2017), 123-137. https://doi.org/10.1016/j.neucom.2016.09.068 doi: 10.1016/j.neucom.2016.09.068
![]() |
[7] | J. Kennedy, R. Eberhart, Particle swarm optimization, in Proceedings of ICNN'95 - International Conference on Neural Networks, 4 (1995), 1942-1948. https://doi.org/10.1109/ICNN.1995.488968 |
[8] |
J. Tu, H. Chen, M. Wang, A. H. Gandomi, The colony predation algorithm, J. Bionic Eng., 18 (2021), 674-710. https://doi.org/10.1007/s42235-021-0050-y doi: 10.1007/s42235-021-0050-y
![]() |
[9] |
G. G. Wang, Moth search algorithm: a bio-inspired metaheuristic algorithm for global optimization problems, Memetic Comput., 10 (2018), 151-164. https://doi.org/10.1007/s12293-016-0212-3 doi: 10.1007/s12293-016-0212-3
![]() |
[10] |
M. Dorigo, M. Birattari, T. Stutzle, Ant colony optimization, IEEE Comput. Intell. Mag., 1 (2006), 28-39. https://doi.org/10.1109/MCI.2006.329691 doi: 10.1109/MCI.2006.329691
![]() |
[11] |
D. Karaboga, B. Basturk, A powerful and efficient algorithm for numerical function optimization: artificial bee colony (ABC) algorithm, J. Global Optim., 39 (2007), 459-471. https://doi.org/10.1007/s10898-007-9149-x doi: 10.1007/s10898-007-9149-x
![]() |
[12] |
S. Mirjalili, S. M. Mirjalili, A. Lewis, Grey wolf optimizer, Adv. Eng. Software, 69 (2014), 46-61. https://doi.org/10.1016/j.advengsoft.2013.12.007 doi: 10.1016/j.advengsoft.2013.12.007
![]() |
[13] |
A. Faramarzi, M. Heidarinejad, S. Mirjalili, A. H. Gandomi, Marine predators algorithm: a nature-inspired metaheuristic, Expert Syst. Appl., 152 (2020), 113377. https://doi.org/10.1016/j.eswa.2020.113377 doi: 10.1016/j.eswa.2020.113377
![]() |
[14] |
S. Li, H. Chen, M. Wang, A. A. Heidari, S. Mirjalili, Slime mould algorithm: a new method for stochastic optimization, Future Gener. Comput. Syst., 111 (2020), 300-323. https://doi.org/10.1016/j.future.2020.03.055 doi: 10.1016/j.future.2020.03.055
![]() |
[15] |
K. Zervoudakis, S. Tsafarakis, A mayfly optimization algorithm, Comput. Ind. Eng., 145 (2020), 106559. https://doi.org/10.1016/j.cie.2020.106559 doi: 10.1016/j.cie.2020.106559
![]() |
[16] |
S. Mirjalili, SCA: a Sine Cosine Algorithm for solving optimization problems, Knowledge Based Syst., 96 (2016), 120-133. https://doi.org/10.1016/j.knosys.2015.12.022 doi: 10.1016/j.knosys.2015.12.022
![]() |
[17] |
A. A. Heidari, S. Mirjalili, H. Faris, I. Aljarah, M. Mafarja, H. Chen, Harris hawks optimization: algorithm and applications, Future Gener. Comput. Syst., 97 (2019), 849-872. https://doi.org/10.1016/j.future.2019.02.028 doi: 10.1016/j.future.2019.02.028
![]() |
[18] |
S. Mirjalili, A. Lewis, The whale optimization algorithm, Adv. Eng. Software, 95 (2016), 51-67. https://doi.org/10.1016/j.advengsoft.2016.01.008 doi: 10.1016/j.advengsoft.2016.01.008
![]() |
[19] |
D. Whitley, A genetic algorithm tutorial, Stat. Comput., 4 (1994), 65-85. https://doi.org/10.1007/BF00175354 doi: 10.1007/BF00175354
![]() |
[20] |
A. Cheraghalipour, M. Hajiaghaei-Keshteli, M. M. Paydar, Tree Growth Algorithm (TGA): a novel approach for solving optimization problems, Eng. Appl. Artif. Intell., 72 (2018), 393-414. https://doi.org/10.1016/j.engappai.2018.04.021 doi: 10.1016/j.engappai.2018.04.021
![]() |
[21] | I. Rechenberg, Evolution strategy: nature's way of optimization, in Optimization: Methods and Applications, Possibilities and Limitations, (1989), 106-126. https://doi.org/10.1007/978-3-642-83814-9_6 |
[22] |
R. Storn, K. Price, Differential evolution - A simple and efficient heuristic for global Optimization over continuous spaces, J. Global Optim., 11 (1997), 341-359. https://doi.org/10.1023/A:1008202821328 doi: 10.1023/A:1008202821328
![]() |
[23] |
L. Abualigah, A. Diabat, S. Mirjalili, M. A. Elaziz, A. H. Gandomi, The arithmetic optimization algorithm, Comput. Methods Appl. Mech. Eng., 376 (2021), 113609. https://doi.org/10.1016/j.cma.2020.113609 doi: 10.1016/j.cma.2020.113609
![]() |
[24] | H. R. Tizhoosh, Opposition-based learning: a new scheme for machine intelligence, in International Conference on Computational Intelligence for Modelling, Control and Automation and International Conference on Intelligent Agents, Web Technologies and Internet Commerce (CIMCA-IAWTIC'06), (2005), 695-701. https://doi.org/10.1109/CIMCA.2005.1631345 |
[25] |
W. Guo, P. Xu, F. Dai, F. Zhao, M. Wu, Improved Harris hawks optimization algorithm based on random unscented sigma point mutation strategy, Appl. Soft Comput., 113 (2021), 108012. https://doi.org/10.1016/j.asoc.2021.108012 doi: 10.1016/j.asoc.2021.108012
![]() |
[26] |
T. Si, P. B. C. Miranda, D. Bhattacharya, Novel enhanced Salp Swarm Algorithms using opposition-based learning schemes for global optimization problems, Expert Syst. Appl., 207 (2022), 117961. https://doi.org/10.1016/j.eswa.2022.117961 doi: 10.1016/j.eswa.2022.117961
![]() |
[27] |
A. G. Hussien, An enhanced opposition-based Salp Swarm Algorithm for global optimization and engineering problems, J. Ambient Intell. Hum. Comput., 13 (2022), 129-150. https://doi.org/10.1007/s12652-021-02892-9 doi: 10.1007/s12652-021-02892-9
![]() |
[28] |
W. Wang, L. Xu, K. Chau, Y. Zhao, D. Xu, An orthogonal opposition-based-learning Yin-Yang-pair optimization algorithm for engineering optimization, Eng. Comput., 38 (2022), 1149-1183. https://doi.org/10.1007/s00366-020-01248-9 doi: 10.1007/s00366-020-01248-9
![]() |
[29] |
A. Aleti, I. Moser, A systematic literature review of adaptive parameter control methods for evolutionary algorithms, ACM Comput. Surv., 49 (2017), 1-35. https://doi.org/10.1145/2996355 doi: 10.1145/2996355
![]() |
[30] |
Z. Lei, S. Gao, S. Gupta, J. Chen, G. Y ang, An aggregative learning gravitational search algorithm with self-adaptive gravitational constants, Expert Syst. Appl., 152 (2020), 113396. https://doi.org/10.1016/j.eswa.2020.113396 doi: 10.1016/j.eswa.2020.113396
![]() |
[31] |
V. Sahargahi, V. Majidnezhad, S. T. Afshord, Y. Jafari, An intelligent chaotic clonal optimizer, Appl. Soft Comput., 115 (2022), 108126. https://doi.org/10.1016/j.asoc.2021.108126 doi: 10.1016/j.asoc.2021.108126
![]() |
[32] | S. Rahnamayan, H. R. Tizhoosh, M. M. A. Salama, Quasi-oppositional differential evolution, in 2007 IEEE Congress on Evolutionary Computation, (2007), 2229-2236. https://doi.org/10.1109/CEC.2007.4424748 |
[33] |
A. A. Ewees, M. A. Elaziz, E. H. Houssein, Improved grasshopper optimization algorithm using opposition-based learning, Expert Syst. Appl., 112 (2018), 156-172. https://doi.org/10.1016/j.eswa.2018.06.023 doi: 10.1016/j.eswa.2018.06.023
![]() |
[34] | R. Tanabe, A. S. Fukunaga, Improving the search performance of SHADE using linear population size reduction, in 2014 IEEE Congress on Evolutionary Computation (CEC), (2014), 1658-1665. https://doi.org/10.1109/CEC.2014.6900380 |
[35] | A. W. Mohamed, A. A. Hadi, A. M. Fattouh, K. M. Jambi, LSHADE with semi-parameter adaptation hybrid with CMA-ES for solving CEC2017 benchmark problems, in 2017 IEEE Congress on Evolutionary Computation (CEC), (2017), 145-152. https://doi.org/10.1109/CEC.2017.7969307 |
[36] |
K. Deb, An efficient constraint handling method for genetic algorithms, Comput. Methods Appl. Mech. Eng., 186 (2000), 311-338. https://doi.org/10.1016/S0045-7825(99)00389-8 doi: 10.1016/S0045-7825(99)00389-8
![]() |
[37] | S. Das, P. N. Suganthan, Problem definitions and evaluation criteria for CEC 2011 competition on testing evolutionary algorithms on real world optimization problems, 2010. Available from: https://al-roomi.org/multimedia/CEC_Database/CEC2011/CEC2011_TechnicalReport.pdf. |
[38] |
C. A. C. Coello, Use of a self-adaptive penalty approach for engineering optimization problems, Comput. Ind., 41 (2000), 113-127. https://doi.org/10.1016/S0166-3615(99)00046-9 doi: 10.1016/S0166-3615(99)00046-9
![]() |
[39] |
K. S. Lee, Z. W. Geem, A new meta-heuristic algorithm for continuous engineering optimization: harmony search theory and practice, Comput. Methods Appl. Mech. Eng., 194 (2005), 3902-3933. https://doi.org/10.1016/j.cma.2004.09.007 doi: 10.1016/j.cma.2004.09.007
![]() |
[40] |
Q. He, L. Wang, An effective co-evolutionary particle swarm optimization for constrained engineering design problems, Eng. Appl. Artif. Intell., 20 (2007), 89-99. https://doi.org/10.1016/j.engappai.2006.03.003 doi: 10.1016/j.engappai.2006.03.003
![]() |
1. | Erin Ellefsen, Nancy Rodríguez, Nonlocal Mechanistic Models in Ecology: Numerical Methods and Parameter Inferences, 2023, 13, 2076-3417, 10598, 10.3390/app131910598 | |
2. | Swadesh Pal, Roderick Melnik, Nonlocal Models in Biology and Life Sciences: Sources, Developments, and Applications, 2025, 15710645, 10.1016/j.plrev.2025.02.005 |