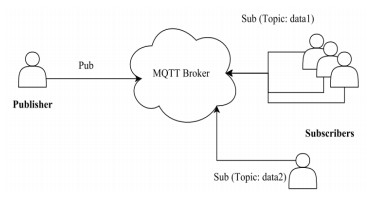
In many applications, complex biological phenomena can be reproduced via structured mathematical models, which depend on numerous biotic and abiotic input parameters, whose effect on model outputs can be of paramount importance. The calibration of model parameters is crucial to obtain the best fit between simulated and experimental data. Sensitivity analysis and uncertainty quantification constitute essential tools in the field of biological systems modeling. Despite the significant number of applications of sensitivity analysis in wet anaerobic digestion, there are no examples of global sensitivity analysis for mathematical models describing the dry anaerobic digestion in plug-flow reactors. For the first time, the present study explores the global sensitivity analysis and uncertainty quantification for a plug-flow reactor model. The investigated model accounts for the mass/volume variation that takes place in these systems as a result of solid waste conversion in gaseous value-added compounds. A preliminary screening based on the Morris' method allowed for the definition of three different groups of parameters. A surrogate model was constructed to investigate the relation between input and output parameters without running demanding simulations from scratch. The obtained Sobol' indices allowed to perform the quantitative global sensitivity analysis. Finally, the uncertainty quantification results led to the definition of the probability density function related to the investigated quantity of interest. The study showed that the net methane production is mostly sensitive to the values of the conversion parameter related to the particulate biodegradable volatile solids in acetic acid k1 and to the kinetic parameter describing the acetic acid uptake k2. The application of these techniques led to helpful information for model calibration and validation.
Citation: Daniele Bernardo Panaro, Andrea Trucchia, Vincenzo Luongo, Maria Rosaria Mattei, Luigi Frunzo. Global sensitivity analysis and uncertainty quantification for a mathematical model of dry anaerobic digestion in plug-flow reactors[J]. Mathematical Biosciences and Engineering, 2024, 21(9): 7139-7164. doi: 10.3934/mbe.2024316
[1] | Yiqin Bao, Qiang Zhao, Jie Sun, Wenbin Xu, Hongbing Lu . An edge cloud and Fibonacci-Diffie-Hellman encryption scheme for secure printer data transmission. Mathematical Biosciences and Engineering, 2024, 21(1): 96-115. doi: 10.3934/mbe.2024005 |
[2] | Pengpeng Wang, Xu Wang, Yumin Shen, Jinlong Wang, Xiaoyun Xiong . PBFT optimization algorithm based on community contributions. Mathematical Biosciences and Engineering, 2023, 20(6): 10200-10222. doi: 10.3934/mbe.2023447 |
[3] | Peng Guan, Lincoln C. Wood, Jason X. Wang, Linh N. K. Duong . Barriers to blockchain adoption in the seaport industry: A fuzzy DEMATEL analysis. Mathematical Biosciences and Engineering, 2023, 20(12): 20995-21031. doi: 10.3934/mbe.2023929 |
[4] | Jiayuan Zhang, Rongxin Guo, Yifan Shi, Wanting Tang . An anti-impersonation attack electronic health record sharing scheme based on proxy re-encryption and blockchain. Mathematical Biosciences and Engineering, 2024, 21(6): 6167-6189. doi: 10.3934/mbe.2024271 |
[5] | Yongjun Ren, Yan Leng, Yaping Cheng, Jin Wang . Secure data storage based on blockchain and coding in edge computing. Mathematical Biosciences and Engineering, 2019, 16(4): 1874-1892. doi: 10.3934/mbe.2019091 |
[6] | Abdullah Lakhan, Mazhar Ali Dootio, Ali Hassan Sodhro, Sandeep Pirbhulal, Tor Morten Groenli, Muhammad Saddam Khokhar, Lei Wang . Cost-efficient service selection and execution and blockchain-enabled serverless network for internet of medical things. Mathematical Biosciences and Engineering, 2021, 18(6): 7344-7362. doi: 10.3934/mbe.2021363 |
[7] | Naila Naz, Muazzam A Khan, Suliman A. Alsuhibany, Muhammad Diyan, Zhiyuan Tan, Muhammad Almas Khan, Jawad Ahmad . Ensemble learning-based IDS for sensors telemetry data in IoT networks. Mathematical Biosciences and Engineering, 2022, 19(10): 10550-10580. doi: 10.3934/mbe.2022493 |
[8] | Jiahe Xu, Yuan Tian, Tinghuai Ma, Najla Al-Nabhan . Intelligent manufacturing security model based on improved blockchain. Mathematical Biosciences and Engineering, 2020, 17(5): 5633-5650. doi: 10.3934/mbe.2020303 |
[9] | Ch. Rupa, Divya Midhunchakkaravarthy, Mohammad Kamrul Hasan, Hesham Alhumyani, Rashid A. Saeed . Industry 5.0: Ethereum blockchain technology based DApp smart contract. Mathematical Biosciences and Engineering, 2021, 18(5): 7010-7027. doi: 10.3934/mbe.2021349 |
[10] | Xiao-Dong Yang, Ze-Fan Liao, Bin Shu, Ai-Jia Chen . Blockchain-based multi-authority revocable data sharing scheme in smart grid. Mathematical Biosciences and Engineering, 2023, 20(7): 11957-11977. doi: 10.3934/mbe.2023531 |
In many applications, complex biological phenomena can be reproduced via structured mathematical models, which depend on numerous biotic and abiotic input parameters, whose effect on model outputs can be of paramount importance. The calibration of model parameters is crucial to obtain the best fit between simulated and experimental data. Sensitivity analysis and uncertainty quantification constitute essential tools in the field of biological systems modeling. Despite the significant number of applications of sensitivity analysis in wet anaerobic digestion, there are no examples of global sensitivity analysis for mathematical models describing the dry anaerobic digestion in plug-flow reactors. For the first time, the present study explores the global sensitivity analysis and uncertainty quantification for a plug-flow reactor model. The investigated model accounts for the mass/volume variation that takes place in these systems as a result of solid waste conversion in gaseous value-added compounds. A preliminary screening based on the Morris' method allowed for the definition of three different groups of parameters. A surrogate model was constructed to investigate the relation between input and output parameters without running demanding simulations from scratch. The obtained Sobol' indices allowed to perform the quantitative global sensitivity analysis. Finally, the uncertainty quantification results led to the definition of the probability density function related to the investigated quantity of interest. The study showed that the net methane production is mostly sensitive to the values of the conversion parameter related to the particulate biodegradable volatile solids in acetic acid k1 and to the kinetic parameter describing the acetic acid uptake k2. The application of these techniques led to helpful information for model calibration and validation.
As rapid development of internet of things (IoTs), a large number of devices in the IoTs and the volume of data generated make it further messy and huge. Further, there are high security risks while establishing an ecosystem containing all devices of the IoTs [1]. The blockchain is a decentralized ledger based on the consensus mechanism, cryptography and distributed storage in the intelligent transportation system. A perfect protocol and architecture design are essential to build a blockchain secure and reliable storage and trading network. In the field of IoT, blockchain can be used to provide security and privacy for user data in the network [2]. In the field of big data, blockchain can be used to improve data security and data quality [3]. In the field of healthcare domain Agbo et al. [4] made blockchain technology in healthcare: A systematic review. In the field of agri-food domain, Mirabelli [5] proposed a blockchain-based solution for agri-food supply chains. In the field of energy domain, Andoni et al. [6] proposed Blockchain technology in the energy sector: A systematic review of challenges and opportunities. In the field of Construction industry domain, Plevris et al. [7] proposed. Blockchain in civil engineering, architecture and construction industry: State of the art, evolution, challenges and opportunities. Bakogiannis et al. [8] proposed leveraging blockchain technology to break the cloud computing market monopoly in the field of cloud computing. In order to obtain data security, blockchain is also used in the field of fog computing and edge computing [9]. Khan et al. [10] pointed out that the scalability problem in the public blockchain hinders the provision of the best solution for enterprises and industries. Key management, encryption algorithm and smart contract are the core features of blockchain security [11]. Blockchain applications pay more attention to the core blockchain technologies and jointly provide a solid security cornerstone for blockchain applications from multiple dimensions such as security, data security, management security and application security [12], Bhat et al. [13] designed the agricultural food supply chain management system based on blockchain and IoT. Huh et al. [14] studied the blockchain consensus algorithm for feasible management of new and renewable energy. Astarita et al. [15] reviewed the application of blockchain in transportation system.
Message queue telemetry transmission (MQTT) is one of the common communication protocols for any platform in the IoTs. Whether from the perspective of overall security, equipment compatibility and resource consumption, MQTT protocol has certain advantages and is the most competitive communication protocol in the current IoT. However, the user password login form adopted by MQTT protocol has some security problems [16]. Diffie-Hellman (DH) key exchange algorithm is used to share public key and private key pairing for encryption and decryption in a secured manner. This algorithm is named after its inventor Diffie and Hellman [17], DH is not an encryption algorithm, but a secure key exchange algorithm, which is commonly used in cloud computing and blockchain systems [18]. Fibonacci sequence, also known as golden section sequence, is mathematically defined by recursive method: F(0) = 0, f(1) = 1, f(n) = f(n−1) + F(n−2) (n ≥ 2, n ∈ N) is used in many fields of physics and chemistry [19] and the third-order Fibonacci sequence is also used in the encryption and decryption applications [20].
Aiming at the problems of complex system and difficulty in implementation of the application of blockchain in intelligent transportation system, this paper proposes a lightweight fuzzy decision blockchain scheme through MQTT and DH Fibonacci technologies. Through this, the blockchain service scheme is scalable and easy to implement. DH and Fibonacci transformation are adopted to form lightweight fuzzy decision blockchain (LF-BC), which enhances the security and ensures the sustainable safety of the intelligent transportation system. The main contributions of this paper include three aspects:
● DH and Fibonacci transform are combined to form the fuzzy practical Byzantine fault tolerance (DH-FIB) algorithm which is used to generate LF-BC and to enhance the security.
● Realize scalable blockchain service scale and realize reliable communication of blockchain through MQTT protocol.
● In LF-BC blockchain system, using F-PBFT consensus algorithm, both fault tolerance rate and the transmission efficiency are significantly improved.
The paper is organized as follows. An extensive literature review on related technologies is undertaken in the second part. The third part proposes a lightweight fuzzy decision blockchain scheme. The fourth part compares and analyzes the experimental results. The fifth part summarizes the conclusion.
In this paper, we implement a trusted lightweight blockchain scheme based on MQTT and Fibonacci, discuss the recent existing research on MQTT protocol, Fibonacci matrix, DH algorithm, blockchain technology and propose to apply DH-FIB algorithm to blockchain scheme.
MQTT was originally developed by IBM as a communication protocol for telemedicine services. It is widely used in the IoTs because of its simplicity, specification, low overhead and easy implementation [21]. MQTT-publish based communication protocol, which creates and controls the hierarchy of topics, or subscribes to multiple topics. In March 2013, Oasis announced that MQTT was the preferred standard for the emerging IoTs messaging protocol. The model of MQTT protocol applied to the IoTs [22] is shown in Figure 1.
MQTT protocol frame format is shown in Table 1:
Control header | Packet length | Variable length header | Payload |
1 Byte | 1 to 4 Bytes | 0–Y Bytes | 0–X Bytes |
The type of MQTT protocol package [23], is shown in Table 2.
Packets type | Type value | Details |
CONNECT | 0x01 | Client request to connect to server |
CONNACK | 0x02 | Connect acknowledgment |
PUBLISH | 0x03 | Publish message |
PUBACK | 0x04 | Publish acknowledgment |
PUBREC | 0x05 | Publish received (assured delivery part 1) |
PUBREL | 0x06 | Publish received (assured delivery part 2) |
PUBCOMP | 0x07 | Publish received (assured delivery part 3) |
SUBSCRIBE | 0x08 | Client subscribe request |
UNSUBSCRIBE | 0x0a | Unsubscribe request |
PINGREQ | 0x0c | PING request |
DISCONNECT | 0x0e | Client is disconnecting |
MQTT protocol only specifies the message format and does not limit the load content and format of users. Therefore, we can customize the load format. There are three options for load format: 1) Hexadecimal/Binary: In this, there is no readability, but the flow can be controlled to relatively small extent; 2) String: This is easy to read, but is still not the best choice; 3) JSON-key value pair: The hierarchy is concise and clear, which is easy to read and write. While implementing the blockchain scheme, considering the security of confidentiality, hexadecimal format is adopted along with Modbus to implement the monitoring and control of the terminal.
MQTT protocol is simple, standardized and has certain advantages. It is the most competitive communication protocol in the current IoTs [24].
Fibonacci, an Italian mathematician in the 13th century, introduced an interesting sequence (called as Fibonacci sequence) from the problem of rabbit reproduction, record as {Fn}. It is a second-order recursive sequence, F0 = 0, F1 = 1, Fn+1 = Fn + Fn−1, n = 1, 2, 3…, and we call each term of this sequence as Fibonacci number. Researchers are interested in Fibonacci sequence over years because of its unique properties and are widely using in computational mathematics, optimization theory, operations research and other fields [25,26].
Reference [27] defines Fibonacci matrix T2, which corresponds to the second-order Fibonacci sequence {Fn} and is expressed as:
(Fn+1FnFnFn - 1)=(1110)n=T2n,n=1,2,3… | (1) |
Reference [28] defines the third-order Fibonacci matrix T3, which corresponds to the third-order Fibonacci sequence {Fn}−a third-order recursive sequence F0 = 1, F1 = 1, F2 = 2, Fn+1 = Fn + Fn−1 + Fn−2, n = 2, 3, 4…, and is expressed as:
(FnFn+1−FnFn−1 Fn−1 Fn−Fn−1 Fn−2 Fn−2 Fn−1−Fn−2 Fn−3)=(111100010)n=T3n,n=3,4,5… | (2) |
Since the determinant |T3| = 1 corresponding to the third-order Fibonacci matrix, T3 is reversible.
T3=(111100010)T−13=(0100011−1−1) | (3) |
Similarly, |T3n| = 1, so T3n is also reversible. The formulae for calculating T3n and (T3n)−1 are as follows:
Since:
(111100010)k1+k2=(111100010)k1∗(111100010)k2 | (4) |
(0100011−1−1)k1+k2=(0100011−1−1)k1∗(0100011−1−1)k2 | (5) |
So:
T3n=(111100010)n(T3n)−1=(0100011−1−1)n | (6) |
For example, n = 5, T35 and (T35)−1 are calculated by formula (6):
T35=(13117764432)(T53)−1=(0−122−2−3−351) | (7) |
We make Fn = T3n, Fn−1 = (T3n)−1, use the characteristics of the third-order Fibonacci matrix T3n reversible sum formula (6), take n as a parameter, and apply Fn and Fn−1 to the encryption process of LF-BC blockchain in this paper.
Cryptography is the most important part of the blockchain system and the basic technology to realize the blockchain [29]. DH is an asymmetric encryption algorithm, which is commonly used in blockchain encryption schemes. It is also a public key algorithm [30]. Each communication party generates a private key and a public key, where the private key is not public. After the public key is publicly exchanged, the communication parties can calculate the shared key, and the shared key calculated by both parties is the same. Therefore, both parties can encrypt and decrypt the message [31,32] through the shared key, so as to realize the encrypted transmission of the message. DH negotiates a shared key through unreliable channels. In many industrial security protocols, such as SSL/TLS, SSH, Kerberos, ISO-9798-3, just fast key and PKI key distribution are widely used.
Alice sends the encrypted transmission process of plaintext M to Bob, as shown in Figure 2.
Encryption process (Alice)
Step 1: First agree two parameters g, N with Bob;
Step2: Randomly generates own private key x, and calculate X = gx mod N, X as the public key;
Step3: Select the public key Y = gy mod N, is sent by Bob and calculate the key: K = Yx mod N = gxy mod N, K as the shared key with Bob;
Step 4: Calculate the encrypted data Cm = Ek1(M);
Step5: Discloses sending Cm to Bob.
Decryption process (Bob)
Step1: First agree two parameters g, n with Alice;
Step2: Randomly generates own private key y, and calculates Y = gy mod N, Y as the public key;
Step3: Select the public key X = gx mod N sent by the sender and calculate the key, G = Xy mod N = gx ymod N, G as the shared key with Alice;
Step4: Publicly receives cm and decrypts data, DG(Cm) = DG(EK(M)) = M;
Step5: Finally obtains m from the slave.
DH is the basis of authentication key exchange (AKE) scheme in the post quantum computing era [33]. It combines DH and Fibonacci transformation to form LF-BC blockchain, which is a specific application of DH in vehicle intelligent transportation system.
Blockchain technology was originally applied in the field of Internet finance to solve the trust problem of the third-party central institutions and provide a trust mechanism for decentralized transactions. The central idea of blockchain technology comes from the Bitcoin project released and implemented in 2009. The core technologies involved in the project mainly include cryptography, incentive mechanism, workload proof mechanism, P2P network, distributed database [34] and other technologies. It has four key characteristics.
● Decentralized network
The essence of blockchain is a decentralized distributed database, which is composed of a series of blocks generated by cryptography. The blocks contain data information that cannot be tampered within a certain period of time. Block contains data record, current block root hash, previous block root hash, time stamp and other information. Each user node in the network is both a client and a server. Each node has a complete backup of the whole database, and all nodes insert data through a specific consensus algorithm [35,36].
● Trusted blockchain structure
A blockchain consists of a block header and a block body as shown in Figure 3.
In Figure 3, block hash represents the hash value of the block header, pre-hash represents the hash value of the previous block, Merkel hash represents the Merkel hash value generated by the transaction number Tx_id, transaction number represents the number of transaction orders, time stamp represents the time stamp of the generated block, public key represents the public key of the generated block, and signature represents that the private key encrypts and signs of the user information. Policy header represents the permission control header of the block body [37], Transaction_i (i = 1...n) represents several transaction orders of the block body.
● Smart contract
Smart contracts are programmable protocols that allow the execution of the contract terms and agreements. The concept was first proposed by Szabo, who defined a smart contract as "a computerized transaction protocol that executes the terms of a contract". Thus, smart contracts can be deployed to a blockchain database and users can develop computer codes based on contractual clauses [38].
● Consensus algorithm
Practical byzantine fault tolerance (PBFT) algorithm is a consensus algorithm proposed by Miguel Castro and Arbara Liskov in 1999. PBFT is an algorithm to solve the consistency of state machine replicas in distributed scenarios [39]. The implementation of PBFT is divided into five steps: (1) Request, (2) Pre-prepare, (3) Prepare, (4) Commit, (5) Reply [40].
The process of PBFT algorithm is shown in Figure 4.
PBFT is a state machine replication algorithm. State machines replicate in different nodes of the distributed system. The copy of each state machine not only saves the state of the service, but also realizes the operation of the service. For convenience of description, assuming that n is the total number of cluster nodes, the security and consistency of the consensus cluster can be guaranteed when the failed nodes do not exceed (n−1)/3. Therefore, the fault-tolerance rate of PBFT is:
F1=(n−1)/3 | (8) |
In other words, as long as the nodes exceeding (n−1)−F1 = 2*(n−1)/3 are honest, the validity of blockchain data can be guaranteed.
The lightweight fuzzy decision blockchain scheme mainly includes four parts: client, terminal, blockchain system and cloud plan work. 1) The client is the user's operating software, which can be a web client or APP; 2) The terminal mainly realizes GPS positioning, signal processing and control, and then realizes communication with the cloud platform; 3) Blockchain system is a service system responsible for data security, which refers to MQTT broker; 4) Cloud platform is a network platform responsible for remote communication and data management. The system architecture is shown in Figure 5, the communication between client, terminal, blockchain system and cloud platform is carried out through the publish and subscription (Pub/Sub) topic of MQTT. there is implementation process in Section 3.5.
Based on MQTT and Fibonacci fuzzy decision blockchain scheme, due to the use of LF-BC blockchain in the architecture, the security problem of the traditional centralized architecture has been effectively solved. When the system is implemented, it mainly includes two transactions: 1) User registration and 2) Data transmission, as shown in Figure 6.
In the user registration stage, the user inputs the user information to form a transaction order, and the registration information is encrypted and stored on the nodes of the blockchain through cryptographic technology and consensus algorithm F-PBFT; while in the data transmissionstage, the data message forms a transaction order. Through cryptography and consensus algorithm F-PBFT, the data message is stored in the blockchain and the information is transmitted to the terminal.
The basic principle of generating DH-FIB algorithm from Fibonacci matrix to DH encryption algorithm is as follows: 1) The sender and the receiver generate their own private key vectors, such as x = (x1, x2, x3), and generate their own public key vectors X and Y. 2) After X and y are exchanged, generate shared key vectors K and G, and calculate that the values of K and G are equal. 3) The sender's original m is encrypted into ciphertext Cm through K, and C is multiplied by Fibonacci matrix Fn to generate ciphertext vector Em = C*Fn, Em = {E1, E2, E3}, Em and sends it to the receiver. 4) The receiver multiplies the received em by the Fibonacci inverse matrix Fn−1, Em*Fn−1 = Cm*Fn*Fn−1 = Cm, finally decrypts Cm through the key vector G to obtain the original vector Em = (M1, M2, M3), and finally compares M1, M2, M3 through the voting algorithm to obtain the original M.
DH-FIB is called fuzzy decision encryption algorithm. Since the encryption key is random and Fibonacci transformed, the ciphertext generated each time is different. For example, the ciphertext obtained by the middleman is ambiguous. Secondly, due to the use of key vector, it is necessary to make decision on the result to achieve the purpose of fault tolerance, reliability and sustainability.
The encryption process of user sending plaintext m to Terminal is shown in Figure 7.
● Encryption process (user)
Step 1: First agree three parameters g, N, n of Fn with terminal;
Step 2: Randomly generate private key vector x = (x1, x2, x3) and calculate public key vector: X = (gx1 mod N, gx2mod N, gx3mod N);
Step3: Select terminal's public key vector Y = (gy1 mod N, gy2 mod N, gy3 mod N) and calculate: K = Yx = (gx1*y1mod N, gx2*y2mod N, gx3*y3mod N); K is defined as (K1, K2, K3), and is used as the shared key with terminal;
Step 4: Calculate the encrypted data C, for example, the original file m has 5 bytes;
M = (M1, M2, M3, M4, M5) (even for n bytes, the principle remains the same), calculate encryption matrix: Cm = Ek(M).
Cm=(C11C12C13C21C22C23C31C32C33C41C42C43C51C52C53) | (9) |
Step 5: Select the third-order Fibonacci matrix Fn, see formula (6);
Step 6: Calculate the encryption matrix Em by formulas (9) and (6);
Em=Cm∗F=(E11E12E13E21E22E23E31E32E33E41E42E43E51E52E53) | (10) |
Step 7: Convert Em to encrypted vector;
Em={E1,E2,E3} | (11) |
Step 8: Publicly send Em to terminal.
Decryption process (terminal)
Step 1: First agree three parameters g, N, n of Fn with user;
Step 2: Randomly generate their own private key vector y = (y1, y2, y3) and calculate public key vector: Y = (gy1 mod N, gy2 mod N, gy3 mod N);
Step 3: Select user's public key vector X = (gx1 mod N, gx2mod N, gx3mod N), and calculate: G = Xy = −(gx1*y1mod N, gx2*y2mod N, gx3*y3mod N), G is defined as (G1, G2, G3), and is used as the shared key with user;
Step 4: Publicly accept T, T = {E1, E2, E3};
Step 5: Select the third-order Fibonacci inverse matrixFn−1, see formula (6);
Step6: The decryption transformation matrix Cm is calculated by formulae (10) and (6). Cm = E*Fn−1 mod N;
Since:
Fn∗F−1n=(100010001) | (12) |
So:
Cm=(C11C12C13C21C22C23C31C32C33C41C42C43C51C52C53) | (13) |
Step 7: The decryption matrix is calculated by G and formula (13).
Mn=DG(Cm)=(M11M12M13M21M22M23M31M32M33M41M42M43M51M52M53) | (14) |
Set:
Mn={M1,M2,M3} | (15) |
From formula (14), M1, M2, M3 should be equal;
Step 8: The original text is obtained from the decryption Matrix Mn through the voting algorithm.
The DH-FIB algorithm is applied to the blockchain system, and the generated blockchain is called lightweight fuzzy decision blockchain (LF-BC). Corresponding to a specific application, such as the remote monitoring system, the client in the system monitors the terminal through MQTT. We define the remote terminal as RTU, the control message adopts MQTT frame format, and the domain in MQTT frame format adopts DH-FIB blockchain structure, as shown in Figure 8. A blockchain includes a block header and a block body. The block body includes a policy header and several transaction orders. The transaction order is defined as a Modbus message.
The block header is used to ensure the security and integrity of blockchain. Police header is used for permission control and is the basis of blockchain data structure. The authorizer is a single entity user or multiple user groups [41].
LF-BC blockchain has three main functions:
● Authority management
Authority management completes permission control through police control header, which includes record number (No), authorizer, RTU_id, active and state. RTU authority management function is a very important part of LF-BC blockchain system, which is directly related to the security of application system.
● Storage management
Storage management is mainly used to manage the transactions. The transaction includes: transaction number (Tx_id) and Modbus message. Modbus protocol is the basic protocol commonly used in industrial automation system [42], which is also widely used in intelligent transportation system. The Modbus format is shown in Table 3.
RTU_id | Function | Data_Adress | DATA | CRC |
● DH-FIB encryption and fault tolerance management
LF-BC blockchain adopts encryption fault-tolerant management for transactions. An example used in this paper is the remote monitoring system. Communication messages need to be encrypted to prevent man in the middle attack and eavesdropping.
As shown in Figure 9, Modbus messages generate encryption vectors Em = {E1, E2, E3} through DH-FIB encryption algorithm. See formula (11), E1, E2 and E3 is used as three transactions of the blockchain respectively. After receiving the blockchain, the receiver decrypts it through DH-FIB algorithm to form three new transactions Mn = {M1, M2, M3}, as shown in formula (14), and finally restores it to Modbus message through fault-tolerant voting algorithm.
Ordinary blockchain schemes use PBFT consensus algorithm. A complete PBFT consensus needs to be completed twice communication, the complexity is O(n2), and its fault tolerance rate is F1 = (n−1)/3, see formula (9). This paper defines the consensus algorithm of the LF-BC blockchain scheme as F-PBFT. Through DH-FIB encryption algorithm, a complete message forms a consensus node. The consensus node contains three transaction orders Mn = {M1, M2, m3}. See formula (15). Take {M1, M2, m3} as a group with a fault tolerance of F2 = 1/3. Vote in the group to reach consensus. Therefore, A complete F-PBFT consensus needs to be completed twicecommunication, the complexity is O(n2/3), which greatly reduces the complexity of the communication.
Since one message generates three transaction orders and PBFT voting algorithm is adopted at the same time, the fault tolerance rate of F-PBFT is:
F3=(n−1)−(1−F1)∗(1−F2)=(n−1)−(1−1/3)∗(1−(n−1)/3)=5∗(n−1)/9 | (16) |
The composition of the system is shown in Figure 5, and the subscription topic definition of its components is shown in Table 4:
Component | Subscrip topic |
MQTT-1, MQTT-2, ..., MQTT-n | MQTT_topic |
Cloud Platform | Cloud_topic |
Client | Client_topic |
Terminal | Terminal_topic |
MQTT communication process:
The process that the client sends data to the terminal through MQTT is as follows:
● The client sends information to the cloud platform by publish topic (Cloud_topic);
● The cloud platform receives the information and sends information to MQTT-1, MQTT-2, ..., MQTT-n by publish topic (MQTT_topic);
● After receiving the information, MQTT-1, MQTT-2, ..., MQTT-n performs the consensus algorithm and sends information to the cloud platform by publish topic (Cloud_topic);
● The cloud platform receives three groups of information. The cloud platform sends a group of information to the terminal through the voting algorithm by publish topic (Terminal_topic).
The implementation process of LF-BC blockchain security scheme realizes the security of communication messages through the system without leakage. It mainly involves: encryption method, MQTT transmission, consensus algorithm, blockchain authentication, etc., mainly including: 1) User registration process and 2) data transmission process.
● User registration process
The flow chart of user registration (registered user name and password) in the system is shown in Figure 10.
Step 1: User sets three parameters g, N, n of Fn, randomly generates x = (x1, x2, x3), and calculates X and Fn. X = (gx1 mod N, gx2mod N, gx3mod N);
Step 2: User sends g, N, n, X to cloud platform; U = > P:{g, N, n, X};
Step 3: Cloud platform generates y = (y1, y2, y3) and calculates Y and G; Y = (gy1 mod N, gy2mod N, gy3mod N); G = Xy = (gx1*y1mod N, gx2*y2mod N, gx3*y3mod N);
Step 4: Cloud platform sends Y to user; P = > U:{Y};
Step 5: User calculates K; K = Yx = (gx1*y1mod N, gx2*y2mod N, gx3*y3mod N);
Step 6: User inputs ID and PW, calculates: Em = Ek (ID, PW)* Fn; U = > P:{ID, Em};
Step 7: Cloud platform decrypts Em, calculates:
a) ID, PW = DG(Em*Fn-1);
b) Judge whether the ID and PW are equal to those in the database;
c) Calculate user id: Uid = H(ID, PW);
d) Calculate digital signature: S = sign(id, G).
Step 8: Cloud platform sends Uid to user; P = > U:{Uid};
Step 9: Cloud platform sends Uid, timestamp T, public key; P, S and blockB_hashto blockchain system; P = > B:{Uid, T, Y, P, B_hash};
Step 10: Blockchain system forms a blockchain and vote the transaction through the F-PBFT consensus algorithm and saves it to each blockchain node.
● Data transmission process
The security of data transmission is the most critical part of LF-BC blockchain scheme, which realizes the encryption and integrity of data. The data transmission flow chart is shown in Figure 11.
Step 1: User input Uid, RTU_addr, Modbus packet M_data; U = > P:{Uid, RTU_addr, M_data};
Step 2: Cloud platform checks whether the Uid is equal to the Uid of the database, and makes digital signature: S = sign(Uaddr, G);
Step 3: Cloud platform sends the address Uid, T, P, S and blockB_hash to blockchain system; P = > B:{Uid, T, P, S, B_hash};
Step 4: Blockchain system forms ablockclaim transaction and vote the transaction through the F-PBFT consensus algorithm, saves it to each block node;
Step 5: Blockchain system sends Police_head and transaction to cloud platform; B = > P:{Police_head, Transaction}
Step 6: Cloud platform sends Transaction to RTU; P = > RTU:{Transaction};
Step 7: RTU checks whether the RTU_addr is consistent with its own, parse packet M_data from the transaction;
Step 8: RTU checks whether the CRC is correct and executes Modbus command P_data;
Step 9: RTU sends Ack-data to Cloud platform; RTU = > P:{Ack-data};
Step 10: Cloud platform sends Ack-data to user. P = > U:{Ack-data}.
This section takes the vehicle intelligent transportation system as an example to realize the specific application. First, establish a LF-BC blockchain through MQTT broker. Only authorized nodes can read blocks, execute smart contracts and verify new blocks in this blockchain. Secondly, the pre approved nodes agree and verify the blockchain through F-PBFT. The authorized nodes include data load terminal, cloud platform, MQTT broker and client.
The application block diagram of vehicle intelligent transportation system is shown in Figure 12. After the load terminal completes the monitoring, it encapsulates the monitoring data to be uploaded (such as equipment type, position, voltage, power, switch, monitoring time, etc.), then digitally signs these data in blockchain, and sends the signed data to cloud platform and blockchain system through MQTT. Finally, The client remotely monitors and controls the device through MQTT.
The following two sections emphasize the performance index analysis to illustrate the implementation effect of the system and verify the feasibility of this scheme.
The lightweight blockchain scheme suggested in this paper is significantly different from the existing centralized monitoring system and analyzes the security of the blockchain scheme from the following three aspects.
● Decentralization: The decentralized network architecture is adopted in which the transaction information is verified by all nodes, and finally a trusted blockchain is formed. Even if some nodes fail or are attacked by hackers, the failed nodes can still return to normal. The centralized system architecture however, is vulnerable to attacks (such as DDoS attacks, SQL injection attacks, etc.).
● Non tamperability: Digital signature can resist forgery attack under random key, so it can not be easily tampered. The fault tolerance performance is improved and the success rate of tampering and modification will be very low in the adoption of DH-FIB encryption algorithm and F-PBFT consensu.
● Traceability: The consensus node will verify the authenticity of the transaction. Each F-PBFT block has the hash value of the previous block, which can be traced back to the source Modbus message. When a malicious node deletes or modifies the content of a block, the system can easily restore and trace all transaction data.
The collection was verified using three methods: the first was manual checking (MAC); the second one was common checking system (CMC)-referred as reading data through a single meter reading software and then checking data through a single image recognition software (such as Baidu cloud recognition) when the data could not be read; and the third one was the Petri parallel system, which was a parallel system of data acquisition and image recognition based on PN.
The higher the fault tolerance rate of the system, the higher is the transmission efficiency and the lower the fault tolerance rate, the lower is the transmission efficiency. The fault tolerance rates of the three schemes are compared below:
● Fault tolerance rate of the data redundancy: Centralized architecture often collects data. For example, a message is transmitted redundantly for three times, and the minority obeys the majority voting is adopted, so the fault tolerance rate is 1/3.
● PBFT fault tolerance rate: The common blockchain architecture adopts that if malicious nodes are evenly distributed on each blockchain, when the number of nodes does not exceed 1/3 of the total number of nodes, the consensus result is correct and has no impact on the system output result. The fault tolerance ability of the system is (n−1)/ 3, so the fault tolerance rate is 1/3.
● F-PBFT fault tolerance rate: The lightweight blockchain architecture in this paper adopts the F-PBFT consensus algorithm. One message generates three transaction orders, as shown in formula 16. The total fault tolerance is 5*(n−1)/9. Therefore, the fault tolerance is 5/9.
On comparing the fault tolerance rates of the three schemes in the histogram in Figure 13, it is observed that the fault tolerance rate of F-PBFT has increased by 22.3%.
Three architectures are compared by testing the data transmission time overhead on the load equipment monitoring system with wireless interference namely, 1) Centralized Architecture (CT-A), 2) Ordinary blockchain Architecture (BC-A); 3) Lightweight fuzzy decision blockchain Architecture (LF-BC-A). In the experiment, each Modbus message data contains 20 bytes, and the error code of one byte is randomly set in every three messages. The transmission time is calculated for the number of messages. B10 represents 10 messages, B20 represents 20 messages, and so on. B100 represents 100 messages. Communication parameters: 9600: 8: n: 1 (rate 9600 bps, 8-bit data bits, no parity check, 1-bit stop bit). Test under the same environment. The results are shown in Table 5.
Architecture | B10 | B20 | B30 | B40 | B50 | B60 | B70 | B80 | B90 | B100 |
CT-A | 1.2 | 2.2 | 3.5 | 4.5 | 5.6 | 7.2 | 9.0 | 10.0 | 12.1 | 14.2 |
BC-A | 1.1 | 2.5 | 3.8 | 4.8 | 5.9 | 8.0 | 9.5 | 11.2 | 13.4 | 15.0 |
LF-BC-A | 1.1 | 2.0 | 3.1 | 4.2 | 5.1 | 6.3 | 7.2 | 8.3 | 9.1 | 10.5 |
The comparison curve based on Table 5 is shown in Figure 14, which intuitively reflects the time overhead of encrypted data transmission. X-axis represents the number of messages to be transmitted, and y-axis represents the time of message transmission.
Following observations are made from Figure 14. 1) In the centralized architecture, when the message is found to have error code (the error code can be judged by the check code in the message), the message needs to be retransmitted, so the transmission time of encrypted data is lower than that of the ordinary blockchain and lightweight blockchain in this paper; 2) On the other hand, in case of the PBFT fault tolerance algorithm, the ordinary blockchain architecture is more efficient than the centralized architecture; 3) whereas, in the F-PBFT fault tolerance algorithm, the LF-BC blockchain architecture has lower data transmission time overhead and the highest efficiency. Both theory and experiment prove that the LF-BC blockchain scheme in this paper has high transmission efficiency in the case of wireless interference.
This sub-section compares the performance of the three architectures by testing on the vehicle intelligent transportation system, combined with six performance indexes: 1) Data flow, 2) Complexity, 3) Fault tolerance, 4) Security, 5) Reliability, 6) Maintainability. The analysis results are shown in Table 6. The inferences are as follows:
Performance index | Centralized architecture (CT-A) | Blockchain architecture (BC-A) | Lightweight blockchain architecture (LF-BC-A) |
Data flow | Small | Big | Medium |
Complexity | Small | Big | Medium |
Fault tolerance | Bad | Good | Best |
Security | Bad | Good | Good |
Reliability | Bad | Good | Good |
Maintainability | Easy | Not easy | Easy |
● Compared with the ordinary blockchain architecture, the LF-BC blockchain architecture in this paper has smaller scale, easier implementation and less complexity, but the data flow is obviously larger than the centralized architecture.
● Further due to the addition of Fibonacci transform on the basis of HD, its security and encryption performance are better.
● The above analysis infers that the fault tolerance and reliability of this scheme have significantly improved.
● Τhe scheme of this paper is lightweight blockchain, which has small scale and is easier to maintain.
Based on the above characteristics, the LF-BC blockchain architecture has certain scalability and can be applied to similar industries, such as agricultural food supply chain system, smart grid system, etc.
This paper proposes a lightweight fuzzy decision blockchain LF-BC scheme based on MQTT and Fibonacci. Through this scheme, the major problem of complex and difficult deployment of blockchain is solved. The reliable communication of the blockchain is realized through MQTT, the LF-BC blockchain is formed by HD algorithm and Fibonacci matrix, which enhances both the security and the fault-tolerance rate by F-PBFT consensus algorithm. Moreover, from the realization of the case under consideration and its comparative analysis of the performance demonstrate the feasibility of this scheme and ensures the sustainable safety of the intelligent transportation system.
This blockchain LF-BC scheme is a lightweight scheme, which has not been verified in a large-scale system. It has only been applied in an urban on-board intelligent transportation system, and has certain limitations. It is envisaged in future research to expand the application of blockchain based on the combination of HD algorithm and Fibonacci matrix, which not only can be applied to the blockchain scheme of intelligent transportation system, but also useful in other aspects.
This study was supported by the Natural Science Foundation Project of China (61976118), the Natural Science Foundation Project of Jiangsu Province (BK20180142), and the key topics of the "13th five-year plan" for Education Science in Jiangsu Province (B-b /2020/01/18).
All authors declare no conflicts of interest in this paper.
[1] |
G. Esposito, L. Frunzo, F. Liotta, A. Panico, F. Pirozzi, Bio-methane potential tests to measure the biogas production from the digestion and co-digestion of complex organic substrates, Open Environ. Eng. J., 5 (2012), 1–8. https://doi.org/10.2174/1874829501205010001 doi: 10.2174/1874829501205010001
![]() |
[2] |
G. Esposito, L. Frunzo, A. Giordano, F. Liotta, A. Panico, F. Pirozzi, Anaerobic co-digestion of organic wastes, Rev. Environ. Sci. Bio/Technol., 11 (2012), 325–341. https://doi.org/10.1007/s11157-012-9277-8 doi: 10.1007/s11157-012-9277-8
![]() |
[3] |
V. Luongo, M. R. Mattei, L. Frunzo, B. D'Acunto, K. Gupta, S. Chellam, et al., A transient biological fouling model for constant flux microfiltration, Math. Biosci. Eng., 20 (2023), 1274–1296. https://doi.org/10.3934/mbe.2023058 doi: 10.3934/mbe.2023058
![]() |
[4] |
Y. Li, S. Y. Park, J. Zhu, Solid-state anaerobic digestion for methane production from organic waste, Renewable Sustainable Energy Rev., 15 (2011), 821–826. https://doi.org/10.1016/j.rser.2010.07.042 doi: 10.1016/j.rser.2010.07.042
![]() |
[5] |
O. Karthikeyan, C. Visvanathan, Bio-energy recovery from high-solid organic substrates by dry anaerobic bio-conversion processes: A review, Rev. Environ. Sci. Bio/Technol., 12 (2013), 257–284. https://doi.org/10.1007/s11157-012-9304-9 doi: 10.1007/s11157-012-9304-9
![]() |
[6] |
P. Vandevivere, New and broader applications of anaerobic digestion, Critical Rev. Env. Sci. Technol., 29 (1999), 151–173. https://doi.org/10.1080/10643389991259191 doi: 10.1080/10643389991259191
![]() |
[7] |
G. Policastro, V. Luongo, L. Frunzo, N. Cogan, M. Fabbricino, A mechanistic mathematical model for photo fermentative hydrogen and polyhydroxybutyrate production, Math. Biosci. Eng., 20 (2023), 7407–7428. https://doi.org/10.3934/mbe.2023321 doi: 10.3934/mbe.2023321
![]() |
[8] |
A. Donoso-Bravo, C. Sadino-Riquelme, D. Gómez, C. Segura, E. Valdebenito, F. Hansen, Modelling of an anaerobic plug-flow reactor. process analysis and evaluation approaches with non-ideal mixing considerations, Bioresour. Technol., 260 (2018), 95–104. https://doi.org/10.1016/j.biortech.2018.03.082 doi: 10.1016/j.biortech.2018.03.082
![]() |
[9] |
I. Białobrzewski, K. Waszkielis, K. Bułkowska, The application of Anaerobic Digestion Model No. 1 for the optimization of biogas production from maize silage, pig manure, cattle manure, and digestate in a full-scale biogas plant, Fuel, 357 (2024), 129789. https://doi.org/10.1016/j.fuel.2023.129789 doi: 10.1016/j.fuel.2023.129789
![]() |
[10] |
R. Kothari, A. Pandey, S. Kumar, V. Tyagi, S. Tyagi, Different aspects of dry anaerobic digestion for bio-energy: An overview, Renewable Sustainable Energy Rev., 39 (2014), 174–195. https://doi.org/10.1016/j.rser.2014.07.011 doi: 10.1016/j.rser.2014.07.011
![]() |
[11] |
D. J. Batstone, J. Keller, I. Angelidaki, S. Kalyuzhnyi, S. Pavlostathis, A. Rozzi, et al., The iwa anaerobic digestion model no 1 (adm1), Water Sci. Technol., 45 (2002), 65–73. https://doi.org/10.2166/wst.2002.0292 doi: 10.2166/wst.2002.0292
![]() |
[12] |
C. De Crescenzo, A. Marzocchella, D. Karatza, S. Chianese, D. Musmarra, Autogenerative high-pressure anaerobic digestion modelling of volatile fatty acids: Effect of pressure variation and substrate composition on volumetric mass transfer coefficients, kinetic parameters, and process performance, Fuel, 358 (2024), 130144, https://doi.org/10.1016/j.fuel.2023.130144 doi: 10.1016/j.fuel.2023.130144
![]() |
[13] | C. De Crescenzo, A. Marzocchella, D. Karatza, A. Molino, P. Ceron-Chafla, R. E. Lindeboom, et al., Modelling of autogenerative high-pressure anaerobic digestion in a batch reactor for the production of pressurised biogas, Biotechnol. Biofuels Bioprod., 15 (2022). https://doi.org/10.1186/s13068-022-02117-x |
[14] |
C. Fall, J. Loaiza-Navía, Design of a tracer test experience and dynamic calibration of the hydraulic model for a full-scale wastewater treatment plant by use of AQUASIM, Water Environ. Res., 79 (2007), 893–900. https://doi.org/10.2175/106143007X176068 doi: 10.2175/106143007X176068
![]() |
[15] | Y. Muslu, Numerical approach to plug-flow activated sludge reactor kinetics, Comput. Biol. Med., 30, (2000), 207–223. https://doi.org/10.1016/S0010-4825(00)00009-3 |
[16] |
V. Vavilin, L. Lokshina, X. Flotats, I. Angelidaki, Anaerobic digestion of solid material: Multidimensional modeling of continuous-flow reactor with non-uniform influent concentration distributions, Biotechnol. Bioeng., 97 (2007), 354–366. https://doi.org/10.1002/bit.21239 doi: 10.1002/bit.21239
![]() |
[17] |
B. Wu, Integration of mixing, heat transfer, and biochemical reaction kinetics in anaerobic methane fermentation, Biotechnol. Bioeng., 109 (2012), 2864–2874. https://doi.org/10.1002/bit.24551 doi: 10.1002/bit.24551
![]() |
[18] |
D. B. Panaro, M. R. Mattei, G. Esposito, J. P. Steyer, F. Capone, L. Frunzo, A modelling and simulation study of anaerobic digestion in plug-flow reactors, Commun. Nonlinear Sci. Numer. Simul., 105 (2022), 106062. https://doi.org/10.1016/j.cnsns.2021.106062 doi: 10.1016/j.cnsns.2021.106062
![]() |
[19] |
Y. Han, Z. Du, X. Hu, Y. Li, D. Cai, J. Fan, et al., Production prediction modeling of food waste anaerobic digestion for resources saving based on SMOTE-LSTM, Appl. Energy, 352 (2023), 122024. https://doi.org/10.1016/j.apenergy.2023.122024 doi: 10.1016/j.apenergy.2023.122024
![]() |
[20] |
A. Saltelli, K. Aleksankina, W. Becker, P. Fennell, F. Ferretti, N. Holst, et al., Why so many published sensitivity analyses are false: A systematic review of sensitivity analysis practices, Environ. Modell. Software, 114 (2019), 29–39. https://doi.org/10.1016/j.envsoft.2019.01.012 doi: 10.1016/j.envsoft.2019.01.012
![]() |
[21] |
A. Donoso-Bravo, J. Mailier, C. Martin, J. Rodríguez, C. A. Aceves-Lara, A. V. Wouwer, Model selection, identification and validation in anaerobic digestion: A review, Water Res., 45 (2011), 5347–5364. https://doi.org/10.1016/j.watres.2011.08.059 doi: 10.1016/j.watres.2011.08.059
![]() |
[22] |
B. Tartakovsky, S. Mu, Y. Zeng, S. Lou, S. Guiot, P. Wu, Anaerobic digestion model No. 1-based distributed parameter model of an anaerobic reactor: II. Model validation, Bioresour. Technol., 99 (2008), 3676–3684. https://doi.org/10.1016/j.biortech.2007.07.061 doi: 10.1016/j.biortech.2007.07.061
![]() |
[23] |
N. Noykova, M. Gyllenberg, Sensitivity analysis and parameter estimation in a model of anaerobic waste water treatment processes with substrate inhibition, Bioprocess. Eng., 23 (2000), 343–349. https://doi.org/10.1007/s004499900169 doi: 10.1007/s004499900169
![]() |
[24] |
O. Bernard, Z. Hadj-Sadok, D. Dochain, A. Genovesi, J. P. Steyer, Dynamical model development and parameter identification for an anaerobic wastewater treatment process, Biotechnol. Bioeng., 75 (2001), 424–438. https://doi.org/10.1002/bit.10036 doi: 10.1002/bit.10036
![]() |
[25] |
V. Vavilin, S. Rytov, S. Pavlostathis, J. Jokela, J. Rintala, A distributed model of solid waste anaerobic digestion: Sensitivity analysis, Water Sci. Technol., 48 (2003), 147–154. https://doi.org/10.2166/wst.2003.0241 doi: 10.2166/wst.2003.0241
![]() |
[26] |
Y. Lin, C. Wu, Sensitivity analysis of phenol degradation with sulfate reduction under anaerobic conditions, Environ. Model. Assess., 16 (2011), 213–225. https://doi.org/10.1007/s10666-010-9243-1 doi: 10.1007/s10666-010-9243-1
![]() |
[27] |
K. Solon, X. Flores-Alsina, K. V. Gernaey, U. Jeppsson, Effects of influent fractionation, kinetics, stoichiometry and mass transfer on CH4, H2 and CO2 production for (plant-wide) modeling of anaerobic digesters, Water Sci. Technol., 71 (2015), 870–877. https://doi.org/10.2166/wst.2015.029 doi: 10.2166/wst.2015.029
![]() |
[28] | L. Benedetti, D. J. Batstone, B. De Baets, I. Nopens, P. A. Vanrolleghem, Global sensitivity analysis of biochemical, design and operational parameters of the Benchmark Simulation Model no. 2, in Proceedings of the 4th International Congress on Environmental Modelling and Software (iEMSs 2008), (2008), 1322–1330. |
[29] |
Ž. Zonta, M. Alves, X. Flotats, J. Palatsi, Modelling inhibitory effects of long chain fatty acids in the anaerobic digestion process, Water Res., 47 (2013), 1369–1380. https://doi.org/10.1016/j.watres.2012.12.007 doi: 10.1016/j.watres.2012.12.007
![]() |
[30] |
F. Carrera-Chapela, A. Donoso-Bravo, D. Jeison, I. D´ıaz, J. Gonzalez, G. Ruiz-Filippi, Development, identification and validation of a mathematical model of anaerobic digestion of sewage sludge focusing on H2S formation and transfer, Biochem. Eng. J., 112 (2016), 13–19. https://doi.org/10.1016/j.bej.2016.03.008 doi: 10.1016/j.bej.2016.03.008
![]() |
[31] |
I. M. Nasir, T. I. Mohd Ghazi, R. Omar, Anaerobic digestion technology in livestock manure treatment for biogas production: A review, Eng. Life Sci., 12 (2012), 258–269. https://doi.org/10.1002/elsc.201100150 doi: 10.1002/elsc.201100150
![]() |
[32] |
M. D. Morris, Factorial sampling plans for preliminary computational experiments, Technometrics, 33 (1991), 161–174. https://doi.org/10.1080/00401706.1991.10484804 doi: 10.1080/00401706.1991.10484804
![]() |
[33] | M. Baudin, A. Dutfoy, B. Iooss, A. L. Popelin, Open TURNS: An industrial software for uncertainty quantification in simulation, preprint, arXiv: 1501.05242. https://doi.org/10.48550/arXiv.1501.05242 |
[34] |
F. Campolongo, A. Saltelli, Sensitivity analysis of an environmental model: An application of different analysis methods, Reliab. Eng. Syst. Saf., 57 (1997), 49–69. https://doi.org/10.1016/S0951-8320(97)00021-5 doi: 10.1016/S0951-8320(97)00021-5
![]() |
[35] |
A. Trucchia, M. R. Mattei, V. Luongo, L. Frunzo, M. C. Rochoux, Surrogate-based uncertainty and sensitivity analysis for bacterial invasion in multi-species biofilm modeling, Commun. Nonlinear Sci. Numer. Simul., 73 (2019), 403–424. https://doi.org/10.1016/j.cnsns.2019.02.024 doi: 10.1016/j.cnsns.2019.02.024
![]() |
[36] |
A. Trucchia, V. Egorova, G. Pagnini, M. C. Rochoux, On the merits of sparse surrogates for global sensitivity analysis of multi-scale nonlinear problems: Application to turbulence and fire-spotting model in wildland fire simulators, Commun. Nonlinear Sci. Numer. Simul., 73 (2019), 120–145. https://doi.org/10.1016/j.cnsns.2019.02.002 doi: 10.1016/j.cnsns.2019.02.002
![]() |
[37] |
P. T. Roy, N. El Moçayd, S. Ricci, J. C. Jouhaud, N. Goutal, M. De Lozzo, et al., Comparison of polynomial chaos and Gaussian process surrogates for uncertainty quantification and correlation estimation of spatially distributed open-channel steady flows, Stochastic Environ. Res. Risk Assess., 32 (2018), 1723–1741. https://doi.org/10.1007/s00477-017-1470-4 doi: 10.1007/s00477-017-1470-4
![]() |
[38] |
D. Xiu, G. E. Karniadakis, The Wiener–Askey polynomial chaos for stochastic differential equations, SIAM J. Sci. Comput., 24 (2002), 619–644. https://doi.org/10.1137/S1064827501387826 doi: 10.1137/S1064827501387826
![]() |
[39] |
G. Blatman, B. Sudret, Efficient computation of global sensitivity indices using sparse polynomial chaos expansions, Reliab. Eng. Syst. Saf., 95 (2010), 1216–1229. https://doi.org/10.1016/j.ress.2010.06.015 doi: 10.1016/j.ress.2010.06.015
![]() |
[40] | C. K. Williams, C. E. Rasmussen, Gaussian Processes for Machine Learning, MIT press Cambridge, MA, 2 (2006). |
[41] |
A. Trucchia, L. Frunzo, Surrogate based global sensitivity analysis of ADM1-based anaerobic digestion model, J. Environ. Manage., 282 (2021), 111456. https://doi.org/10.1016/j.jenvman.2020.111456 doi: 10.1016/j.jenvman.2020.111456
![]() |
[42] |
F. Charte, I. Romero, M. D. Pérez-Godoy, A. J. Rivera, E. Castro, Comparative analysis of data mining and response surface methodology predictive models for enzymatic hydrolysis of pretreated olive tree biomass, Comput. Chem. Eng., 101 (2017), 23–30. https://doi.org/10.1016/j.compchemeng.2017.02.008 doi: 10.1016/j.compchemeng.2017.02.008
![]() |
[43] |
E. Ficara, S. Hassam, A. Allegrini, A. Leva, F. Malpei, G. Ferretti, Anaerobic digestion models: A comparative study, IFAC Proc. Vol., 45 (2012), 1052–1057. https://doi.org/10.3182/20120215-3-AT-3016.00186 doi: 10.3182/20120215-3-AT-3016.00186
![]() |
[44] | D. Panaro, L. Frunzo, M. Mattei, V. Luongo, G. Esposito, Calibration, validation and sensitivity analysis of a surface-based ADM1 model, Ecol. Modell., 460 (2021), 109726. |
[45] |
C. Veluchamy, A. S. Kalamdhad, A mass diffusion model on the effect of moisture content for solid-state anaerobic digestion, J. Cleaner Prod., 162 (2017), 371–379 https://doi.org/10.1016/j.jclepro.2017.06.099 doi: 10.1016/j.jclepro.2017.06.099
![]() |
[46] |
F. Xu, Z. W. Wang, L. Tang, Y. Li, A mass diffusion-based interpretation of the effect of total solids content on solid-state anaerobic digestion of cellulosic biomass, Bioresour. Technol., 167 (2014), 178–185. https://doi.org/10.1016/j.biortech.2014.05.114 doi: 10.1016/j.biortech.2014.05.114
![]() |
[47] |
F. Liotta, P. Chatellier, G. Esposito, M. Fabbricino, E. D. Van Hullebusch, P. N. Lens, Hydrodynamic mathematical modelling of aerobic plug flow and nonideal flow reactors: A critical and historical review, Crit. Rev. Environ. Sci. Technol., 44 (2014), 2642–2673. https://doi.org/10.1080/10643389.2013.829768 doi: 10.1080/10643389.2013.829768
![]() |
[48] |
A. Marrel, B. Iooss, B. Laurent, O. Roustant, Calculations of Sobol indices for the Gaussian process metamodel, Reliab. Eng. Syst. Saf., 94 (2009), 742–751. https://doi.org/10.1016/j.ress.2008.07.008 doi: 10.1016/j.ress.2008.07.008
![]() |
[49] | I. Sobolprime, Sensitivity analysis for nonlinear mathematical models, Math. Model. Comput. Exp., 1 (1993), 407–414. |
[50] | A. Saltelli, M. Ratto, T. Andres, F. Campolongo, J. Cariboni, D. Gatelli, et al., Global Sensitivity Analysis: The Primer, John Wiley & Sons, 2008. |
[51] | M. Baudin, K. Boumhaout, T. Delage, B. Iooss, J. M. Martinez, Numerical stability of Sobol'indices estimation formula, in Proceedings of the 8th International Conference on Sensitivity Analysis of Model Output (SAMO 2016), 30 (2016), 50–51. |
[52] |
J. Yang, L. Lu, W. Ouyang, Y. Gou, Y. Chen, H. Ma, et al., Estimation of kinetic parameters of an anaerobic digestion model using particle swarm optimization, Biochem. Eng. J., 120 (2017), 25–32. https://doi.org/10.1016/j.bej.2016.12.022 doi: 10.1016/j.bej.2016.12.022
![]() |
[53] |
N. Kythreotou, G. Florides, S. A. Tassou, A review of simple to scientific models for anaerobic digestion, Renewable Energy, 71 (2014), 701–714. https://doi.org/10.1016/j.renene.2014.05.055 doi: 10.1016/j.renene.2014.05.055
![]() |
[54] |
J. Y. X. Ling, Y. J. Chan, J. W. Chen, D. J. S. Chong, A. L. L. Tan, S. K. Arumugasamy, et al., Machine learning methods for the modelling and optimisation of biogas production from anaerobic digestion: A review, Environ. Sci. Pollut. Res., 31 (2024), 19085–19104. https://doi.org/10.1007/s11356-024-32435-6 doi: 10.1007/s11356-024-32435-6
![]() |
1. | B. Sathish, T. P. Anithaashri, 2024, 3193, 0094-243X, 020195, 10.1063/5.0233252 | |
2. | Ali Mohammad Norouzzadeh Gilmolk, Mohammad Reza Aref, Lightweight Image Encryption Using a Novel Chaotic Technique for the Safe Internet of Things, 2024, 17, 1875-6883, 10.1007/s44196-024-00535-3 | |
3. | Jong Hyuk Park, Editorial: Artificial Intelligence-based Security Applications and Services for Smart Cities, 2024, 21, 1551-0018, 7012, 10.3934/mbe.2024307 | |
4. | Yiqin Bao, Qiang Zhao, Jie Sun, Wenbin Xu, Hongbing Lu, An edge cloud and Fibonacci-Diffie-Hellman encryption scheme for secure printer data transmission, 2023, 21, 1551-0018, 96, 10.3934/mbe.2024005 | |
5. | P.S. Akshatha, S.M. Dilip Kumar, MQTT and blockchain sharding: An approach to user-controlled data access with improved security and efficiency, 2023, 4, 20967209, 100158, 10.1016/j.bcra.2023.100158 |
Control header | Packet length | Variable length header | Payload |
1 Byte | 1 to 4 Bytes | 0–Y Bytes | 0–X Bytes |
Packets type | Type value | Details |
CONNECT | 0x01 | Client request to connect to server |
CONNACK | 0x02 | Connect acknowledgment |
PUBLISH | 0x03 | Publish message |
PUBACK | 0x04 | Publish acknowledgment |
PUBREC | 0x05 | Publish received (assured delivery part 1) |
PUBREL | 0x06 | Publish received (assured delivery part 2) |
PUBCOMP | 0x07 | Publish received (assured delivery part 3) |
SUBSCRIBE | 0x08 | Client subscribe request |
UNSUBSCRIBE | 0x0a | Unsubscribe request |
PINGREQ | 0x0c | PING request |
DISCONNECT | 0x0e | Client is disconnecting |
RTU_id | Function | Data_Adress | DATA | CRC |
Component | Subscrip topic |
MQTT-1, MQTT-2, ..., MQTT-n | MQTT_topic |
Cloud Platform | Cloud_topic |
Client | Client_topic |
Terminal | Terminal_topic |
Architecture | B10 | B20 | B30 | B40 | B50 | B60 | B70 | B80 | B90 | B100 |
CT-A | 1.2 | 2.2 | 3.5 | 4.5 | 5.6 | 7.2 | 9.0 | 10.0 | 12.1 | 14.2 |
BC-A | 1.1 | 2.5 | 3.8 | 4.8 | 5.9 | 8.0 | 9.5 | 11.2 | 13.4 | 15.0 |
LF-BC-A | 1.1 | 2.0 | 3.1 | 4.2 | 5.1 | 6.3 | 7.2 | 8.3 | 9.1 | 10.5 |
Performance index | Centralized architecture (CT-A) | Blockchain architecture (BC-A) | Lightweight blockchain architecture (LF-BC-A) |
Data flow | Small | Big | Medium |
Complexity | Small | Big | Medium |
Fault tolerance | Bad | Good | Best |
Security | Bad | Good | Good |
Reliability | Bad | Good | Good |
Maintainability | Easy | Not easy | Easy |
Control header | Packet length | Variable length header | Payload |
1 Byte | 1 to 4 Bytes | 0–Y Bytes | 0–X Bytes |
Packets type | Type value | Details |
CONNECT | 0x01 | Client request to connect to server |
CONNACK | 0x02 | Connect acknowledgment |
PUBLISH | 0x03 | Publish message |
PUBACK | 0x04 | Publish acknowledgment |
PUBREC | 0x05 | Publish received (assured delivery part 1) |
PUBREL | 0x06 | Publish received (assured delivery part 2) |
PUBCOMP | 0x07 | Publish received (assured delivery part 3) |
SUBSCRIBE | 0x08 | Client subscribe request |
UNSUBSCRIBE | 0x0a | Unsubscribe request |
PINGREQ | 0x0c | PING request |
DISCONNECT | 0x0e | Client is disconnecting |
RTU_id | Function | Data_Adress | DATA | CRC |
Component | Subscrip topic |
MQTT-1, MQTT-2, ..., MQTT-n | MQTT_topic |
Cloud Platform | Cloud_topic |
Client | Client_topic |
Terminal | Terminal_topic |
Architecture | B10 | B20 | B30 | B40 | B50 | B60 | B70 | B80 | B90 | B100 |
CT-A | 1.2 | 2.2 | 3.5 | 4.5 | 5.6 | 7.2 | 9.0 | 10.0 | 12.1 | 14.2 |
BC-A | 1.1 | 2.5 | 3.8 | 4.8 | 5.9 | 8.0 | 9.5 | 11.2 | 13.4 | 15.0 |
LF-BC-A | 1.1 | 2.0 | 3.1 | 4.2 | 5.1 | 6.3 | 7.2 | 8.3 | 9.1 | 10.5 |
Performance index | Centralized architecture (CT-A) | Blockchain architecture (BC-A) | Lightweight blockchain architecture (LF-BC-A) |
Data flow | Small | Big | Medium |
Complexity | Small | Big | Medium |
Fault tolerance | Bad | Good | Best |
Security | Bad | Good | Good |
Reliability | Bad | Good | Good |
Maintainability | Easy | Not easy | Easy |