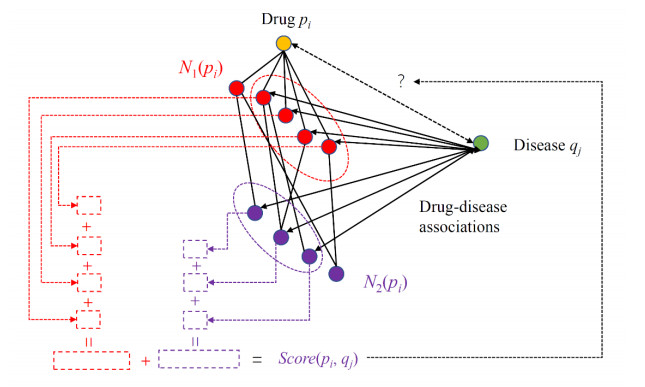
Multiscale modelling is a promising quantitative approach for studying infectious disease dynamics. This approach garners attention from both individuals who model diseases and those who plan for public health because it has great potential to contribute in expanding the understanding necessary for managing, reducing, and potentially exterminating infectious diseases. In this article, we developed a nested multiscale model of hepatitis B virus (HBV) that integrates the within-cell scale and the between-cell scale at cell level of organization of this disease system. The between-cell scale is linked to the within-cell scale by a once off inflow of initial viral infective inoculum dose from the between-cell scale to the within-cell scale through the process of infection; the within-cell scale is linked to the between-cell scale through the outflow of the virus from the within-cell scale to the between-cell scale through the process of viral shedding or excretion. The resulting multiple scales model is bidirectionally coupled in such a way that the within-cell scale and between-cell scale sub-models mutually affect each other, creating a reciprocal relationship. The computed reproductive number from the multiscale model confirms that the within-host scale and the between-host scale influence each other in a reciprocal manner. Numerical simulations are presented that also confirm the theoretical results and support the initial assumption that the within-cell scale and the between-cell scale influence each other in a reciprocal manner. This multiple scales modeling approach serves as a valuable tool for assessing the impact and success of health strategies aimed at controlling hepatitis B virus disease system.
Citation: Huguette Laure Wamba Makeng, Ivric Valaire Yatat-Djeumen, Bothwell Maregere, Rendani Netshikweta, Jean Jules Tewa, Winston Garira. Multiscale modelling of hepatitis B virus at cell level of organization[J]. Mathematical Biosciences and Engineering, 2024, 21(9): 7165-7193. doi: 10.3934/mbe.2024317
[1] | Saranya Muniyappan, Arockia Xavier Annie Rayan, Geetha Thekkumpurath Varrieth . DTiGNN: Learning drug-target embedding from a heterogeneous biological network based on a two-level attention-based graph neural network. Mathematical Biosciences and Engineering, 2023, 20(5): 9530-9571. doi: 10.3934/mbe.2023419 |
[2] | Huiqing Wang, Sen Zhao, Jing Zhao, Zhipeng Feng . A model for predicting drug-disease associations based on dense convolutional attention network. Mathematical Biosciences and Engineering, 2021, 18(6): 7419-7439. doi: 10.3934/mbe.2021367 |
[3] | Chenhao Wu, Lei Chen . A model with deep analysis on a large drug network for drug classification. Mathematical Biosciences and Engineering, 2023, 20(1): 383-401. doi: 10.3934/mbe.2023018 |
[4] | Bo Zhou, Bing Ran, Lei Chen . A GraphSAGE-based model with fingerprints only to predict drug-drug interactions. Mathematical Biosciences and Engineering, 2024, 21(2): 2922-2942. doi: 10.3934/mbe.2024130 |
[5] | Xianfang Wang, Qimeng Li, Yifeng Liu, Zhiyong Du, Ruixia Jin . Drug repositioning of COVID-19 based on mixed graph network and ion channel. Mathematical Biosciences and Engineering, 2022, 19(4): 3269-3284. doi: 10.3934/mbe.2022151 |
[6] | Meng Jiang, Bo Zhou, Lei Chen . Identification of drug side effects with a path-based method. Mathematical Biosciences and Engineering, 2022, 19(6): 5754-5771. doi: 10.3934/mbe.2022269 |
[7] | Peter Hinow, Edward A. Rietman, Sara Ibrahim Omar, Jack A. Tuszyński . Algebraic and topological indices of molecular pathway networks in human cancers. Mathematical Biosciences and Engineering, 2015, 12(6): 1289-1302. doi: 10.3934/mbe.2015.12.1289 |
[8] | Cristian Tomasetti, Doron Levy . An elementary approach to modeling drug resistance in cancer. Mathematical Biosciences and Engineering, 2010, 7(4): 905-918. doi: 10.3934/mbe.2010.7.905 |
[9] | Rishin Haldar, Swathi Jamjala Narayanan . A novel ensemble based recommendation approach using network based analysis for identification of effective drugs for Tuberculosis. Mathematical Biosciences and Engineering, 2022, 19(1): 873-891. doi: 10.3934/mbe.2022040 |
[10] | Lili Jiang, Sirong Chen, Yuanhui Wu, Da Zhou, Lihua Duan . Prediction of coronary heart disease in gout patients using machine learning models. Mathematical Biosciences and Engineering, 2023, 20(3): 4574-4591. doi: 10.3934/mbe.2023212 |
Multiscale modelling is a promising quantitative approach for studying infectious disease dynamics. This approach garners attention from both individuals who model diseases and those who plan for public health because it has great potential to contribute in expanding the understanding necessary for managing, reducing, and potentially exterminating infectious diseases. In this article, we developed a nested multiscale model of hepatitis B virus (HBV) that integrates the within-cell scale and the between-cell scale at cell level of organization of this disease system. The between-cell scale is linked to the within-cell scale by a once off inflow of initial viral infective inoculum dose from the between-cell scale to the within-cell scale through the process of infection; the within-cell scale is linked to the between-cell scale through the outflow of the virus from the within-cell scale to the between-cell scale through the process of viral shedding or excretion. The resulting multiple scales model is bidirectionally coupled in such a way that the within-cell scale and between-cell scale sub-models mutually affect each other, creating a reciprocal relationship. The computed reproductive number from the multiscale model confirms that the within-host scale and the between-host scale influence each other in a reciprocal manner. Numerical simulations are presented that also confirm the theoretical results and support the initial assumption that the within-cell scale and the between-cell scale influence each other in a reciprocal manner. This multiple scales modeling approach serves as a valuable tool for assessing the impact and success of health strategies aimed at controlling hepatitis B virus disease system.
Diseases are a core issue of human health, has and have existed since the emergence of human beings. A large number of people die from various diseases every year. The problem of how to treat different diseases is always a hot topic in medical science. Numerous efforts have been made over the years, especially in the past 100 years. Plenty of schemes have been designed to treat diseases. Among them, drug is considered to be one of the effective ways. However, it is not easy to develop a new drug, which always requires some rigorous and complex steps; drug development is a long procedure and is always very expensive. According to relevant reports, the average time for designing a new drug is about 10-15 years [1] and can cost up to 802 million dollars [2]. Although the investment on drug development has sharply increased in these years, the number of drug approvals each year remains quite low. Designing new techniques for accelerating the procedures of drug development remains quite urgent.
Drug repositioning is deemed as an alternative pipeline, which can promote drug development procedures. For most existing drugs, our cognizance is not very complete, as some latent effects have not been discovered. The purpose of drug repositioning is to discover the latent effects of existing drugs, thereby discovering the new diseases that these drugs can treat. Because numerous clinical tests have been conducted on existing drugs, the launch of these drugs for treating new diseases can be evidently accelerated. However, it is still laborious to find out and confirm the new effects of existing drugs. The use of computational methods is an effective alternative procedure, which has become quite popular [3,4,5,6,7].
In recent years, lots of computational methods have been designed for drug repositioning. Many of these methods focused on the prediction of drug-disease associations (DDAs). The validated DDAs were deeply analyzed and some special patterns were discovered, which can be used to identify latent DDAs. Previous studies have modeled such prediction problems as a recommender system [8,9,10,11,12]. These methods always set up one or more kernels of drugs, diseases, or drugs and diseases. Some complex fusion procedures were applied to these kernels, thereby scoring each pair of drugs and diseases. Network-based methods are another group for predicting DDAs. They always construct multiple networks, which contain not only drugs and diseases but also other related objects, such as long non-coding RNAs (lncRNAs), micro RNAs (miRNAs), target proteins, etc. Among these methods, some can be directly applied to networks to make predictions [13,14,15,16,17,18,19], whereas others can adopt networks to access features of drugs and diseases, and the downstream classification algorithms complete the prediction task [20,21,22,23,24,25]. Recently, deep learning algorithms have been used to construct methods for predicting DDAs, such as convolutional neural networks [26] and graph convolutional networks [27,28]. Several computational methods designed binary classifiers to predict DDAs. The validated DDAs were retrieved from public databases, which were termed as positive samples. However, the selection of negative samples was a problem. Some studies adopted random selection to pick up the same number of negative samples from unlabeled pairs of drugs and diseases [21,26,27]. As latent DDAs may be included, such selection may lead to an unstable decision boundary of the following constructed classifiers. This study gave a contribution in this regard.
In this study, a novel reliable negative selection scheme, named RNSS, was proposed, which can help us select reliable negative samples (i.e., pairs of drugs and diseases with low probabilities of being actual DDAs). Such a scheme employed the k-neighbors of a drug in a drug network and evaluated the relationships between one drug and one disease based on the relationships between k-neighbors and the disease. Three classic classification algorithms were adopted to construct classifiers using negative samples generated by an RNSS: random forest (RF) [29], bayes network (BN), and the nearest neighbor algorithm (NNA) [30]. The results indicated that the classifiers with the RNSS provided a nearly perfect performance and were much better than those with traditional negative selection schemes, indicating that the RNSS can genuinely screen out reliable negative samples.
The validated DDAs were directly accessed from a previous study [21]. These interactions were extracted from chemical-disease interactions collected in the Comparative Toxicogenomics Database (CTD) (http://ctdbase.org) [31,32,33]. In detail, the file "CTD_chemicals_diseases.csv.gz" in CTD was downloaded, from which the chemical-disease associations with "DirectEvidence" were extracted. Then, the chemical-disease associations without DrugBank IDs were discarded. Approximately 63, 472 associations remained, which were deemed as positive samples in this study. Approximately 2, 794 drugs (represented by DrugBank IDs) and 3, 019 diseases (represented by MESH or OMIM identifiers) were involved in the positive samples.
Generally, negative samples were necessary to build the binary classification model. However, the selection of negative samples was a challenging problem as the unlabeled pairs of drugs and diseases may be latent DDAs. Some previous studies adopted random selection to construct negative samples. Here, we proposed a scheme to select reliable negative samples. Based on such a scheme, negative samples that were one, two, and three times as many positive samples were selected and combined with positive samples to constitute datasets.
In this study, we tackled the prediction of DDAs by modeling a binary classification problem. From the CTD, the validated positive samples were obtained as mentioned in Section 2.1. However, there are no public databases that collect the negative samples for DDAs due to a lack of their application values [26]. Several previous studies adopted a random selection scheme to generate negative samples, which may induce an unstable decision boundary of the classifier [34]. Thus, it is necessary to design an efficient scheme to select reliable negative samples. These samples should have a low likelihood of being actual DDAs. This section introduced a novel scheme to select reliable negative samples, named RNSS. Its procedures are described below.
First, a drug network was constructed, which defined 2, 794 drugs as nodes. The associations between drugs should be determined, thereby defining the edges within the network. It is known that the Simplified Molecular Input Line Entry System (SMILES) [35] format is the most widely used representations of drugs, from which the fingerprints of drugs can be extracted. Here, the RDKit (http://www.rdkit.org/) was adopted to extract the extended-connectivity fingerprint (ECFP) of each investigated drug. For drug p, its fingerprints constitute a set, denoted by F(p). The Tanimoto coefficient was applied on the fingerprint sets of two drugs, p1 and p2, to measure their associations, formulated by
Qf(p1,p2)=|F(p1)∩F(p2)||F(p1)∪F(p2)|. | (1) |
Two nodes in the network were connected by an edge if and only if the association between their corresponding drugs was larger than zero. In addition, each edge e was assigned a weight, denoted by w(e), which was the association between two drugs. Such a network is denoted by Wd.
It is known that drugs in similar structures are more likely to treat similar diseases. In view of this, the relationships between a drug, denoted by pi, and a disease, denoted by qj, can be measured by the relationships between similar drugs of pi and qj. In Wd, these drugs are the direct neighbors of pi, (i.e., the 1-neighbors of pi). Furthermore, the drugs with a distance two to pi (2-neighbors of pi) may also provide contributions, also for the k-neighbors of pi (k > 2). In view of this, the k-neighbors of pi was picked up from Wd, denoted by Nk(pi), which consisted of drugs with distance k to pi. For each drug p in N1(pi), the association between it and pi (i.e., the weight on the edge connecting them) can be directly used to measure the relationship between pi and qj. However, it is problematic to utilize drugs in Nk(pi) (k > 1), as these drugs have no direct associations with pi. To settle such a problem, the weight of a path must be defined. For a path P with length l, containing edges e1,e2,⋯,el, its weight, denoted by w(P), was defined as
w(P)=(∏li=1w(ei))Fdecay(P), | (2) |
where w(ei) represents the weight of edge ei, Fdecay(P) is a decay function, which can increase the influence of path length as the long path indicate weak association between two endpoints, computed by
Fdecay(P)=θ⋅l, | (3) |
where θ is a parameter, which was set to 2.26 as suggested in [36,37,38,39,40]. For each drug p in Nk(pi) (k > 1), its linkage to pi can be measured by the weights of the paths connecting them. If multiple paths connecting them, the maximum path weight was selected. Based on the above definitions, the linkage between pi and drug p in Nk(pi) can be synthesized as follows:
L(pi,p)={Qf(pi,p)p∈N1(pi)max{w(Pi)|i=1,2,⋯,m}p∈Nk(pi)(k>1), | (4) |
where P1,P2,⋯,Pm represent all paths connecting pi and p with length k. In Nk(pi), some drugs can constitute DDAs with qj, whereas others cannot. In view of this, we defined an indictor function as follows:
Δ(p,qj)={1ifpandqjcanconstituteaDDA0otherwise. | (5) |
Then, the relationship between pi and qj can be measured by the following level score:
Score(pi,qj)=∑tk=1∑p∈Nk(pi)L(pi,p)⋅Δ(p,qj). | (6) |
Because it is time-consuming to find all paths connecting two nodes with a long distance, a threshold t was employed in Eq (6). Such a setting was also reasonable because paths with long distances play few or even no contributions to measure the linkage between pi and qj. An example is shown in Figure 1, where the threshold t is set to 2.
It is clear that the high outcome of Eq (6) indicated the strong relationships between the drug and disease. On the contrary, it was almost impossible for the pair of drugs and diseases with a low score to be an actual DDA. These pairs can be high-quality negative samples and may be helpful to construct classifiers with a high performance. In theory, the unlabeled pairs of drugs and diseases with low level scores should be selected as negative samples. Such an operation can be conducted by setting a low threshold s to the level score (i.e., the unlabeled pairs of drugs and diseases with level scores no more than s were selected). These selected negative samples comprised a negative sample pool, denoted by NS(s).
In traditional machine learning, it is very important to encode each sample with its essential properties. In this study, we directly adopted the drug and disease features reported in our previous study [21], which were derived from multiple networks. Networks are recently a popular research form as they can overview each object with all other objects as background. As the procedures of network construction and feature extraction have been described in detail in a previous study [21], we only gave a brief introduction on such procedures.
Twelve drug networks were constructed, where eight contained only drugs, and the other four included other objects. For the former eight networks, they were constructed in terms of drug associations collected from two public databases: KEGG (https://www.genome.jp/kegg/) [41,42] and STITCH (http://stitch.embl.de, version 4.0) [43], and that defined by the Anatomical Therapeutic Chemical (ATC) codes of two drugs. As for the later four networks, they indicated the relationships between drug and one of the following objects: proteins, pathways, side effects, and gene ontology (GO) terms. The target proteins and side effects of drugs were retrieved from DrugBank (https://go.drugbank.com/) [44] and SIDER (http://sideeffects.embl.de/) [45], respectively. In CTD, the related GO terms and pathways for chemicals were also collected, which were picked up for building drug networks.
Three networks were built for diseases, involving basic information of diseases such as pathway, gene, and phenotype information. This information was also sourced from the CTD. According to the related pathways of two diseases, their association was measured by the Tanimoto coefficient of two pathway sets. The disease associations based on gene and phenotype information can be assessed in the same way. Then, three networks were built with these disease associations.
The drug and disease networks mentioned above contained abundant information of drugs and diseases, respectively. Informative drug and disease features can be extracted from them. As multiple networks were constructed for drug and disease, a powerful network embedding algorithm, Mashup [46], was adopted. Its greatest merit is that it can process more than one network. Two stages are contained in this algorithm. In the first stage, the raw feature vector for each node in each network is extracted in terms of a random walk with restart [47,48]. The feature vectors for the same node that are derived from different networks are fused in the second stage. At the same time, the dimension is reduced. Mashup was applied to the twelve drug networks to generate drug features and the disease features were produced from three disease networks. Various dimensions, changing from 50 to 1000, were produced for drugs and diseases. The optimal dimension for drugs and diseases can be determined by a ten-fold cross-validation [49].
In this study, the RNSS was proposed to select reliable negative samples. Three classic classification algorithms were selected to construct models based on positively validated positive samples and selected reliable negative samples, thereby elaborating the utility of an RNSS. These classification algorithms included RF [29], BN and NNA [30], which were also adopted in the previous study [21]. These algorithms were designed using quite different ideas and principles. Their common results can provide a universal significance. For this investigation, if the classifiers with an RNSS were generally better than those without an RNSS or with other negative sample selection schemes for any of these three algorithms, it can prove that an RNSS is an efficient scheme to select high-quality negative samples. To quickly implement the above algorithms, corresponding tools (RandomForest, BayesNet and IBk) in Weka [50] were employed. Their default parameters were adopted because the purpose of this study was to test whether the employment of an RNSS can improve the performance of models rather than to build models with excellent performance.
In this study, a ten-fold cross-validation was adopted to evaluate the performance of all constructed classifiers [49]. Such a method divides samples into ten parts. Each part is singled out as the test set and the rest of the parts constitute the training set. The classifier based on the training set is applied to the test set. In an RNSS, the calculation of the level score is related to the positive samples in the training set. Thus, we first divided the positive samples into ten parts. When one part of the positive samples was singled out, which was put into the test set, we used the rest of the positive samples to compute the level scores of unlabeled samples and then selected negative samples. In this way, the information of the test samples was completely excluded when training the classifiers. It was a rigorous cross-validation.
For the binary classification, plenty of measurements have been designed to evaluate the performance of various models. The direct way to display the predicted results of one model is a confusion matrix, which contains four entries: true positive (TP), false negative (FN), false positive (FP), and true negative (TN). Several measurements can be calculated according to these entries. In this study, we selected sensitivity (SN), specificity (SP), accuracy (ACC), precision, F1-measure [51,52,53,54,55,56,57], and the Matthews correlation coefficient (MCC) [58], which can be computed by
SN=TPTP+FN | (7) |
SP=TNTN+FP | (8) |
ACC=TP+TNTP+FP+TN+FN | (9) |
Precision=TPTP+FP | (10) |
F1−measure=2×Recall×PrecisionRecall+Precision | (11) |
MCC=TP×TN−FP×FN√(TP+FP)×(TP+FN)×(TN+FP)×(TN+FN) | (12) |
Besides, the receiver operating characteristic (ROC) and precision-recall (PR) curves were used to comprehensively evaluate the models' performance. The ROC curve sets the SN as the Y-axis and the false positive rate (i.e., 1-SP) as the X-axis, which are obtained by setting different thresholds. The PR curve is defined in a similar way, which defines recall (i.e., SN) as the X-axis and precision as the Y-axis. The area under the ROC and PR curves are essential measurements to assess the models' performance, which were denoted by AUROC and AUPR, respectively.
In this study, a computation method was proposed to identify DDAs. To enhance the performance of the method, a novel negative sample selection scheme, RNSS, was designed. The classifiers using samples selected by an RNSS were built and evaluated. The entire procedure is illustrated in Figure 2.
When assessing the level score of one drug and one disease, the threshold t (Eq (6)) determined which k-neighbors of the drug were considered. It is clear that neighbors with a long distance to the drug give few contributions. Here, we set such threshold t as two, that is, the direct neighbors and 2-neighbors of the drug were included to assess its associations to diseases. After obtaining the level scores of all unlabeled pairs using Eq (6) with t = 2, we set two thresholds (0.05 and 0.1) to construct two negative sample pools (i.e., NS(0.05) and NS(0.1)). From each pool, the same number of negative samples to the positive samples were randomly selected and combined with positive samples to constitute a balanced dataset. Each sample was represented by drug and disease features derived from multiple drug and disease networks. The dimensions for drug and disease features were set to various values between 50 and 1, 000. All possible dimension combinations were attempted. Three classification algorithms (RF, BN, and NNA) were applied to construct classifiers on balanced datasets. These classifiers were assessed by a ten-fold cross-validation. The best performance (measured by MCC) of the RF, BN, and NNA classifiers using negative samples selected from two pools is listed in Table 1.
Classification algorithm | Negative sample pool | Dimension | SN | SP | ACC | Precision | F1-measure | MCC | |
Drug feature | Disease feature | ||||||||
Random forest | NS(0.05) | 1000 | 1000 | 1.000 | 0.979 | 0.989 | 0.979 | 0.989 | 0.979 |
NS(0.1) | 850 | 900 | 1.000 | 0.953 | 0.975 | 0.950 | 0.974 | 0.951 | |
Bayes network | NS(0.05) | 550 | 50 | 1.000 | 0.979 | 0.989 | 0.979 | 0.989 | 0.979 |
NS(0.1) | 550 | 50 | 1.000 | 0.952 | 0.975 | 0.950 | 0.974 | 0.950 | |
Nearest neighbor algorithm | NS(0.05) | 1000 | 50 | 0.978 | 0.974 | 0.976 | 0.974 | 0.976 | 0.951 |
NS(0.1) | 1000 | 50 | 0.956 | 0.954 | 0.955 | 0.954 | 0.955 | 0.910 |
For the balanced dataset with negative samples selected from NS(0.05), the RF classifier yielded an MCC of 0.979, which was very high. The other five measurements (SN, SP, ACC, precision, and F1-measure) were 1.000, 0.979, 0.989, 0.979, and 0.989, respectively. Such a performance suggested that the RF classifier can give a nearly perfect prediction. As for the BN and NNA classifiers, they also produced a high performance. The MCC values of these two classifiers were 0.979 and 0.951, respectively. Considering that BN and NNA were not very powerful classification algorithms, such a performance was extreme high for them. The ROC and PR curves of above three classifiers are illustrated in Figure 3. The AUROC values for three classifiers were 0.9962, 0.9893, and 0.9758, respectively, and AUPR values were 0.9969, 0.9893, and 0.9653, respectively. They were all very high, further suggesting the high performance of three classifiers. The above results implied that the negative samples selected from NS(0.05) were quite different from the positive samples, inducing easy classifications. This fact also proves the effectiveness of the RNSS.
For another pool NS(0.1), negative samples were also randomly selected and comprised the balanced dataset with positive samples. The MCC of the RF classifier on such a dataset was 0.951, which was lower than that yielded by the RF classifier using samples selected from NS(0.05). The SP, ACC, precision, and F1-measure all slightly decreased compared with those of the RF classifier using the samples selected from NS(0.05) (Table 1). The ROC and PR curves as alongside the AUROC and AUPR (Figure 3) of the RF classifier on such a balanced dataset were inferior to those yielded by the RF classifier using samples selected from NS(0.05). A similar phenomenon occurred for the BN and NNA classifiers. It was suggested that the level score (Eq (6)) can really indicate the quality of negative samples, that is, low level scores indicated the high quality of negative samples. It was a proper strategy to select negative samples with low level scores.
In Section 3.1, all classifiers were set up on balanced datasets. To give a further test, some classifiers based on imbalanced datasets were constructed and evaluated. We randomly selected negative samples from each negative sample pool that were twice or thrice as many as positive samples to comprise imbalanced datasets. On each imbalanced dataset, the classifiers with different combinations of drug and disease feature dimensions were set up and evaluated by a ten-fold cross-validation. The best performance for three classification algorithms is listed in Tables 2 and 3.
Classification algorithm | Negative sample pool | Dimension | SN | SP | ACC | Precision | F1-measure | MCC | |
Drug feature | Disease feature | ||||||||
Random forest | NS(0.05) | 1000 | 1000 | 1.000 | 0.989 | 0.993 | 0.979 | 0.989 | 0.984 |
NS(0.1) | 850 | 900 | 0.999 | 0.975 | 0.983 | 0.950 | 0.974 | 0.962 | |
Bayes network | NS(0.05) | 550 | 50 | 1.000 | 0.989 | 0.993 | 0.979 | 0.989 | 0.984 |
NS(0.1) | 550 | 50 | 1.000 | 0.975 | 0.983 | 0.950 | 0.974 | 0.963 | |
Nearest neighbor algorithm | NS(0.05) | 1000 | 50 | 0.978 | 0.985 | 0.983 | 0.971 | 0.974 | 0.962 |
NS(0.1) | 1000 | 50 | 0.953 | 0.973 | 0.967 | 0.946 | 0.950 | 0.925 |
Classification algorithm | Negative sample pool | Dimension | SN | SP | ACC | Precision | F1-measure | MCC | |
Drug feature | Disease feature | ||||||||
Random forest | NS(0.05) | 1000 | 1000 | 0.999 | 0.992 | 0.994 | 0.979 | 0.989 | 0.984 |
NS(0.1) | 850 | 900 | 0.999 | 0.984 | 0.987 | 0.950 | 0.974 | 0.966 | |
Bayes network | NS(0.05) | 550 | 50 | 1.000 | 0.992 | 0.994 | 0.979 | 0.989 | 0.985 |
NS(0.1) | 550 | 50 | 1.000 | 0.984 | 0.987 | 0.950 | 0.974 | 0.966 | |
Nearest neighbor algorithm | NS(0.05) | 1000 | 50 | 0.976 | 0.988 | 0.985 | 0.970 | 0.973 | 0.962 |
NS(0.1) | 1000 | 50 | 0.951 | 0.982 | 0.974 | 0.945 | 0.948 | 0.931 |
Of the imbalanced datasets, which contained negative samples twice as many as positive samples, the RF classifier generated MCC values of 0.984 and 0.962 for two negative sample pools (Table 2). The values for the BN classifier were 0.984 and 0.963 (Table 2). For the NNA classifier, it yielded MCC values of 0.962 and 0.925 (Table 2). It was amazing that these three classifiers on such imbalanced datasets provided even better performance than those on the balanced datasets, as described in Section 3.1. Generally, the performance of the classifiers on imbalanced dataset may decrease, especially on the minor class. However, the overall performance of the above classifiers actually increased. As for their performance on the minor class (positive samples), measured by SN, the decrease range was quite limited. The ROC and PR curves of the above classifiers are illustrated in Figure 4(A), (B). Based on AUROC and AUPR, the performance of the classifiers on imbalanced datasets did not clearly decrease, quite coincident with results assessed by other measurements.
For other imbalanced datasets containing negative samples thrice as many as positive samples, the performance of the three classifiers on two pools is shown in Table 3. It can be observed that such a performance was quite similar to that in Tables 1 and 2. The same conclusions can be obtained from the ROC and PR curves (Figure 4(C), (D)) of these classifiers. These results indicate that the classifiers with an RNSS were not very sensitive to the imbalanced problem. As the negative sample pools constructed by the RNSS included negative samples with high quality, the increment on negative samples did not amplify the difficulties for learning an efficient classifier. Furthermore, the classifiers using negative samples selected from NS(0.05) were superior to those using negative samples selected from NS(0.1), suggesting that the level score was a good indicator to select negative samples with higher quality.
In the RNSS, the distance limitation t was an important parameter, which directly influenced the calculation of the level score (see Eq (6)). It was interesting to investigate the effect of such a parameter. The above classifiers were all based on negative samples selected by the RNSS with t = 2. Here, we investigated the classifiers using negative samples selected by an RNSS with t = 1. In fact, an RNSS with t = 1 was the same as a previous negative sample selection method reported in [59], named finding reliable negative samples (FIRE).
When t was set to 1, numerous unlabeled samples were assigned a level score of 0. Thus, we added the threshold 0 for parameter s (i.e., three negative sample pools were considered), including NS(0), NS(0.05) and NS(0.1). From each pool, we first randomly selected as many negative samples as positive samples to constitute balanced datasets. Three classifiers with different parameter combinations mentioned above were constructed on each balanced dataset and evaluated by a ten-fold cross-validation. The best performance for each classification algorithm was picked up and detailed measurements listed in Section 2.6 are illustrated in Figure 5. For easy comparisons, the performance of classifiers with an RNSS (t = 2) is also listed in this figure. Given the same classification algorithm (RF, BN or NNA), classifiers with an RNSS (t = 2) were generally superior to those with an RNSS (t = 1) in terms of all measurements. The improvement for BN and NNA classifiers was very great, whereas that for the RF classifier was slightly enhanced. As the RF classifiers with an RNSS (t = 1) provided a relatively higher performance than BN and NNA classifiers with an RNSS (t = 1), it was difficult to achieve any improvements.
In addition, the imbalanced datasets were constructed by selecting two and three times as many negative samples as positive samples from each of three pools. Classifiers built on these datasets were also evaluated by a ten-fold cross-validation. The best performance for each classification algorithm is shown in Appendix Figures A1 and A2, from which we can conclude the same result (i.e., the classifiers with an RNSS (t = 2) were obviously better than those with an RNSS (t = 1)).
With the above arguments, despite the balanced or imbalanced datasets, classifiers with an RNSS (t = 2) yielded better performance, indicating that the employment of 2-neighbors of drugs can improve the selection of negative samples. As mentioned above, numerous unlabeled samples were assigned a level score of zero by an RNSS (t = 1). The drug in each of these samples did not have direct neighbors that were related to the disease in the same sample. However, such a drug may have some 2-neighbors that were associated with the disease, which made the level score yielded by an RNSS (t = 2) larger than zero. The employment of 2-neighbors can help us further classify these unlabeled samples, thereby screening out negative samples with a higher quality.
In many studies, random selection of negative samples is a widely used scheme to construct binary classifiers [21,26,27,60,61]. Here, several classifiers were built using such scheme, which were compared with classifiers with an RNSS to elaborate the superiority of the RNSS. To give a full comparison, we constructed three datasets containing negative samples one, two, and three times as many as positive samples. Three classification algorithms (RF, BN and NNA) were adopted to build the classifiers. Feature dimensions of drug and disease were the same as classifiers with an RNSS (t = 2, s = 0.05), as listed in Tables 1-3. All classifiers were also assessed by a ten-fold cross-validation. The performance is listed in Table 4.
Classification algorithm | Ratio of positive and negative samples | SN | SP | ACC | Precision | F1-measure | MCC | AUROC | AUPR |
Random forest | 1:1 | 0.739 | 0.782 | 0.761 | 0.773 | 0.756 | 0.522 | 0.818 | 0.803 |
1:2 | 0.570 | 0.884 | 0.779 | 0.711 | 0.632 | 0.483 | 0.818 | 0.690 | |
1:3 | 0.435 | 0.925 | 0.803 | 0.659 | 0.524 | 0.420 | 0.807 | 0.591 | |
Bayes network | 1:1 | 0.667 | 0.837 | 0.752 | 0.804 | 0.729 | 0.512 | 0.751 | 0.703 |
1:2 | 0.673 | 0.836 | 0.782 | 0.672 | 0.672 | 0.509 | 0.751 | 0.558 | |
1:3 | 0.675 | 0.826 | 0.788 | 0.564 | 0.614 | 0.473 | 0.747 | 0.460 | |
Nearest neighbor algorithm | 1:1 | 0.695 | 0.685 | 0.690 | 0.688 | 0.691 | 0.380 | 0.671 | 0.616 |
1:2 | 0.571 | 0.780 | 0.711 | 0.565 | 0.568 | 0.351 | 0.660 | 0.451 | |
1:3 | 0.497 | 0.826 | 0.744 | 0.487 | 0.492 | 0.321 | 0.638 | 0.346 |
For balanced dataset (i.e., the ratio of positive and negative samples was 1:1), the MCC values of RF, BN, and NNA classifiers were only 0.522, 0.512, and 0.380, respectively. Compared with the MCC values of RF, BN, and NNA classifiers with an RNSS (t = 2, s = 0.05), which were 0.979, 0.979, and 0.951 (Table 1), respectively, such a performance was much lower. The same results can be obtained in terms of other measurements. Thus, the classifiers with an RNSS were much stronger than those using randomly selected negative samples. For the imbalanced datasets (the ratio of positive and negative samples = 1:2 or 1:3), we can also conclude that classifiers using randomly selected negative samples was much inferior to those with an RNSS (see Tables 2-4). Above results indicated that the RNSS was effective to help us select negative samples with high quality, thereby improving the classifiers.
Besides random selection of negative samples, some studies adopted another scheme to select negative samples [62,63]. For unlabeled samples, K-means were adopted to cluster them and negative samples were equally and randomly selected from each cluster. As stated in [64], the clustering effect was best when the unlabeled samples were clustered into 23 clusters. We also adopted such a setting (i.e., unlabeled pairs of drugs and diseases were clustered into 23 clusters). Unlabeled pairs selected from each cluster were combined to constitute the negative sample set, whose size was the same as the positive sample set. Three classification algorithms (RF, BN, and NNA) were adopted to build the classifiers. Feature dimensions of drug and disease were the same as classifiers with an RNSS (t = 2, s = 0.05), as listed in Tables 1. The evaluation results yielded by the ten-fold cross-validation are listed in Table 5. Compared with evaluation results of classifiers with an RNSS (Table 1), these classifiers were very poor. These results further confirmed the utility of the RNSS.
Classification algorithm | Ratio of positive and negative samples | SN | SP | ACC | Precision | F1-measure | MCC | AUROC | AUPR |
Random forest | 1:1 | 0.053 | 0.867 | 0.460 | 0.271 | 0.088 | -0.142 | 0.600 | 0.510 |
Bayes network | 1:1 | 0.662 | 0.809 | 0.736 | 0.776 | 0.714 | 0.477 | 0.734 | 0.684 |
Nearest neighbor algorithm | 1:1 | 0.732 | 0.757 | 0.745 | 0.751 | 0.741 | 0.490 | 0.722 | 0.669 |
To date, several DDA prediction methods have been proposed. Here, some of them were selected to compare with our method with the RNSS. Their performance is listed in Table 6. For easy comparisons, the performance of our method (RF classifier with s = 0.05 and t = 2) is also provided in this table. It can be observed that our method provided best performance for all eight measurements. This result indicated the superiority of our model and the RNSS.
Model | SN | SP | ACC | Precision | F1-measure | MCC | AUROC | AUPR |
Our model | 1.000 | 0.979 | 0.989 | 0.979 | 0.989 | 0.979 | 0.996 | 0.997 |
RepCOOL [24] | 0.930 | - | - | 0.530 | 0.670 | - | 0.670 | - |
RLFDDA [25] | 0.897 | - | 0.901 | 0.904 | 0.900 | - | 0.964 | - |
Li et al.' method [26] | 0.862 | 0.868 | 0.865 | 0.867 | - | 0.730 | 0.936 | 0.935 |
MGP-DDA [23] | 0.842 | - | 0.867 | 0.886 | 0.863 | - | 0.930 | 0.944 |
Yang and Chen's method [21] | 0.872 | 0.843 | 0.858 | 0.847 | 0.860 | 0.716 | 0.928 | 0.919 |
$: Measurements for all methods except our method were directly picked up from their corresponding literature; -: This measurement was not reported. |
The selection of negative samples is a challenging problem in association prediction. Some schemes have been designed in recent years [59,65,66]. The proposed scheme, RNSS, is more similar to the methods in [59,65]. Thus, this section focused on the similarities and differences to the method in [66]. This method was called self-paced negative sampling strategy (SNSS).
SNSS employs a hardness function to indicate the likehood of one unlabeled sample to be an actual negative sample, similar to the level score in our scheme. This function relies on a multilayer perceptron (MLP) classifier, which is very different from the drug network in our scheme. It is defined as the differences of the probability score yielded by an MLP and the ground-truth label. It is hard to say which scoring system is better, as there does not exist any generally accepted dataset to validate whether the score is correct. On the other hand, the selection strategies of the two methods were quite different. In SNSS, it divides unlabeled samples into some categories according to the results of hardness function and selects negative samples from each category with different proportions. The selection scope was all unlabeled samples. Such a selection can fully train the classifier and increase the robustness. In our scheme, we select negative samples from a pool consisting of unlabeled samples with low level scores (i.e., the selection scope was a part of unlabeled samples). Such a selection can improve the performance of the classifier. The different intentions induce different selection strategies. These methods provide alternative ways to select negative samples when building the binary association prediction methods. It is also interesting to fuse the merits of these two methods for designing a new negative sample selection method.
In this study, we proposed a novel scheme to select high-quality negative drug-disease samples. To elaborate its utility, several classifiers were constructed with negative samples selected by such a scheme. The evaluation results suggested that these classifiers had an extremely strong ability to identify DDAs. Additionally, these classifiers were much better than those using some traditional and previous schemes, confirming the positive effects of the proposed scheme in selecting high-quality negative samples. It is hopeful that such a scheme can be applied to other related problems for building classifiers with a high performance.
The authors declare they have not used Artificial Intelligence (AI) tools in the creation of this article.
The authors declare there is no conflict of interest.
[1] | L. Christian, World Health Organization Statistics 2015, 2015. Available from: https://apps.who.int/mediacentre/news/releases/2015/world-health-statistics-2015/fr/index.html. |
[2] |
M. Kane, Global programme for control of hepatitis B infection, Vaccine, 13 (1995), S47–S49. https://doi.org/10.1016/0264-410X(95)80050-N doi: 10.1016/0264-410X(95)80050-N
![]() |
[3] | A. A. Fall, Études de quelques modèles épidémiologiques: application à la transmission du virus de l'hépatite B en Afrique subsaharienne (cas du Sénégal), Ph.D thesis, Paul Verlaine-Metz University/Universite Gaston Berger, 2010. |
[4] | N. Otric, Cameroon Health Among the 17 Countries Most Affected by Hepatitis, 2017. Available from: https://www.cameroon-info.net/article/cameroun-sante-le-cameroun-parmi-les-17-pays-les-plus-touches-par-lhepatite-selon-296589.html. |
[5] |
G. M. Prifti, D. Moianos, E. Giannakopoulou, V. Pardali, J. E. Tavis, G. Zoidis, Recent advances in hepatitis B treatment, Pharmaceuticals, 14 (2021), 417. https://doi.org/10.3390/ph14050417 doi: 10.3390/ph14050417
![]() |
[6] |
C. W. Shepard, E. P. Simard, L. Finelli, A. E. Fiore, B. P. Bell, Hepatitis B virus infection: epidemiology and vaccination, Epidemiol. Rev., 28 (2006), 112–125. https://doi.org/10.1093/epirev/mxj009 doi: 10.1093/epirev/mxj009
![]() |
[7] | M. Matshidiso, Cameroon–The Government Aims to Lower to 53 the Prevalence Rate of Hepatitis B, 2020. Available from: https://actucameroun.com/2020/09/04/cameroun-le-gouvernement-ambitionne-de-baisser-a-53-le-taux-de-prevalence-de-lhepatite-b/. |
[8] | B. A. Collins, D. Meenakshi, O. Sakuya, 91 million Africans infected with hepatitis B or C, 2022. Available from: https://www.afro.who.int/fr/news/91-millions-dafricains-infectes-par-lhepatite-b-ou-c. |
[9] |
W. Garira, B. Maregere, The transmission mechanism theory of disease dynamics: Its aims, assumptions and limitations, Infect. Dis. Model., 8 (2023), 122–144. https://doi.org/10.1016/j.idm.2022.12.001 doi: 10.1016/j.idm.2022.12.001
![]() |
[10] |
W. Garira, K. Muzhinji, Application of the replication–transmission relativity theory in the development of multiscale models of infectious disease dynamics, J. Biol. Dyn., 17 (2023), 2255066. https://doi.org/10.1080/17513758.2023.2255066 doi: 10.1080/17513758.2023.2255066
![]() |
[11] |
W. Garira, The replication-transmission relativity theory for multiscale modelling of infectious disease systems, Sci. Rep., 9 (2019), 16353. https://doi.org/10.1038/s41598-019-52820-3 doi: 10.1038/s41598-019-52820-3
![]() |
[12] |
W. Garira, The research and development process for multiscale models of infectious disease systems, PLoS Comput. Biol., 16 (2020), e1007734. https://doi.org/10.1371/journal.pcbi.1007734 doi: 10.1371/journal.pcbi.1007734
![]() |
[13] |
M. A. Novak, S. Bonhoeffer, A. M. Hill, R. Boehme, H. C. Thomas, H. McDade, Viral dynamics in hepatitis B virus infection, Proc. Natl. Acad. Sci. USA, 93 (1996), 4398–4402. https://doi.org/10.1073/pnas.93.9.4398 doi: 10.1073/pnas.93.9.4398
![]() |
[14] |
A. V. Herz, S. Bonhoeffer, R. M. Anderson, R. M. May, M. A. Nowak, Viral dynamics in vivo: limitations on estimates of intracellular delay and virus decay, Proc. Natl. Acad. Sci. USA, 93 (1996), 7247–7251. https://doi.org/10.1073/pnas.93.14.7247 doi: 10.1073/pnas.93.14.7247
![]() |
[15] |
S. Zeuzem, A. Robert, P. Honkoop, W. K. Roth, S. W. Schalm, J. M. Schmidt, Dynamics of hepatitis B virus infection in vivo, J. Hepatol., 27 (1997), 431–436. https://doi.org/10.1016/S0168-8278(97)80345-5 doi: 10.1016/S0168-8278(97)80345-5
![]() |
[16] |
G. K. Lau, M. Tsiang, J. Hou, S. T. Yuen, W. F. Carman, L. Zhang, et al., Combination therapy with lamivudine and famciclovir for chronic hepatitis B–infected Chinese patients: a viral dynamics study, Hepatology, 32 (2000), 394–399. https://doi.org/10.1053/jhep.2000.9143 doi: 10.1053/jhep.2000.9143
![]() |
[17] |
S. R. Lewin, R. M. Ribeiro, T. Walters, G. K. Lau, S. Bowden, S. Locarnini, et al., Analysis of hepatitis B viral load decline under potent therapy: complex decay profiles observed, Hepatology, 34 (2001), 1012–1020. https://doi.org/10.1053/jhep.2001.28509 doi: 10.1053/jhep.2001.28509
![]() |
[18] |
N. Moolla, M. Kew, P. Arbuthnot, Regulatory elements of hepatitis B virus transcription, J. Viral Hepatitis, 9 (2002), 323–331. https://doi.org/10.1046/j.1365-2893.2002.00381.x doi: 10.1046/j.1365-2893.2002.00381.x
![]() |
[19] | V. Bruss, Hepatitis B virus morphogenesis, World J. Gastroenterol., 13 (2007), 65. |
[20] |
J. Nakabayashi, A. Sasaki, A mathematical model of the intracellular replication and within host evolution of hepatitis type B virus: Understanding the long time course of chronic hepatitis, J. Theor. Biol., 269 (2011), 318–329. https://doi.org/10.1016/j.jtbi.2010.10.024 doi: 10.1016/j.jtbi.2010.10.024
![]() |
[21] |
J. Nakabayashi, The intracellular dynamics of hepatitis B virus (HBV) replication with reproduced virion "re-cycling", J. Theor. Biol., 396 (2016), 154–162. http://dx.doi.org/10.1016/j.jtbi.2016.02.008 doi: 10.1016/j.jtbi.2016.02.008
![]() |
[22] |
W. Garira, A complete categorization of multiscale models of infectious disease systems, J. Biol. Dyn., 11 (2017), 378–435. https://doi.org/10.1080/17513758.2017.1367849 doi: 10.1080/17513758.2017.1367849
![]() |
[23] |
W. Garira, A primer on multiscale modelling of infectious disease systems, Infect. Dis. Model., 3 (2018), 176–191. https://doi.org/10.1016/j.idm.2018.09.005 doi: 10.1016/j.idm.2018.09.005
![]() |
[24] |
W. Garira, D. Mathebula, A coupled multiscale model to guide malaria control and elimination, J. Theor. Biol., 475 (2019), 34–59. https://doi.org/10.1016/j.jtbi.2019.05.011 doi: 10.1016/j.jtbi.2019.05.011
![]() |
[25] |
W. Garira, M. C. Mafunda, From individual health to community health: towards multiscale modeling of directly transmitted infectious disease systems, J. Biol. Syst., 27 (2019), 131–166. https://doi.org/10.1142/S0218339019500074 doi: 10.1142/S0218339019500074
![]() |
[26] |
D. C. Krakauer, N. L. Komarova, Levels of selection in positive-strand virus dynamics, J. Evolution. Biol., 16 (2003), 64–73. https://doi.org/10.1046/j.1420-9101.2003.00481.x doi: 10.1046/j.1420-9101.2003.00481.x
![]() |
[27] |
R. Netshikweta, W. Garira, A nested multiscale model to study paratuberculosis in ruminants, Front. Appl. Math. Stat., 8 (2022), 817060. https://doi.org/10.3389/fams.2022.817060 doi: 10.3389/fams.2022.817060
![]() |
[28] |
R. Netshikweta, W. Garira, An embedded multiscale modelling to guide control and elimination of paratuberculosis in ruminants, Comput. Math. Methods M., 2021 (2021), 9919700. https://doi.org/10.1155/2021/9919700 doi: 10.1155/2021/9919700
![]() |
[29] |
L. Rong, J. Guedj, H. Dahari, D. J. Coffield Jr, M. Levi, P. Smith, et al., Analysis of hepatitis C virus decline during treatment with the protease inhibitor danoprevir using a multiscale model, PLoS Comput. Biol., 9 (2013), e1002959. https://doi.org/10.1371/journal.pcbi.1002959 doi: 10.1371/journal.pcbi.1002959
![]() |
[30] |
I. Hosseini, F. Mac Gabhann, Multi-scale modeling of HIV infection in vitro and APOBEC3G-based anti-retroviral therapy, PLoS Comput. Biol., 8 (2012), e1002371. https://doi.org/10.1371/journal.pcbi.1002371 doi: 10.1371/journal.pcbi.1002371
![]() |
[31] |
G. W. Suryawanshi, A. Hoffmann, A multi-scale mathematical modeling framework to investigate anti-viral therapeutic opportunities in targeting HIV-1 accessory proteins, J. Theor. Biol., 386 (2015), 89–104. https://doi.org/10.1016/j.jtbi.2015.08.032 doi: 10.1016/j.jtbi.2015.08.032
![]() |
[32] |
J. Guedj, A. U. Neumann, Understanding hepatitis C viral dynamics with direct-acting antiviral agents due to the interplay between intracellular replication and cellular infection dynamics, J. Theor. Biol., 267 (2010), 330–340. https://doi.org/10.1016/j.jtbi.2010.08.036 doi: 10.1016/j.jtbi.2010.08.036
![]() |
[33] |
E. L. Haseltine, J. B. Rawlings, J. Yin, Dynamics of viral infections: incorporating both the intracellular and extracellular levels, Comput. Chem. Eng., 29 (2005), 675–686. https://doi.org/10.1016/j.compchemeng.2004.08.022 doi: 10.1016/j.compchemeng.2004.08.022
![]() |
[34] | P. Van den Driessche, J. Watmough, Reproduction numbers and sub-threshold endemic equilibria for compartmental models of disease transmission, Math. Biosci., 180 (2002), 29–48. https://doi.org/10.1016/S0025-5564(02)00108-6 |
[35] | P. Van den Driessche, J. Watmough, Further notes on the basic reproduction number, in Mathematical Epidemiology, Springer, (2008), 159–178. https://doi.org/10.1007/978-3-540-78911-6_6 |
[36] | M. M. Ojo, F. O. Akinpelu, Lyapunov functions and global properties of seir epidemic model, Int. J. Chem. Math. Phys., 1 (2017), 11–16. |
[37] |
W. Garira, K. Muzhinji, The universal theory for multiscale modelling of infectious disease dynamics, Mathematics, 11 (2023), 3874. https://doi.org/10.3390/math11183874 doi: 10.3390/math11183874
![]() |
1. | Xiandong Lin, QingLan Ma, Lei Chen, Wei Guo, Zhiyi Huang, Tao Huang, Yu-Dong Cai, Identifying genes associated with resistance to KRAS G12C inhibitors via machine learning methods, 2023, 1867, 03044165, 130484, 10.1016/j.bbagen.2023.130484 | |
2. | Bo Zhou, Bing Ran, Lei Chen, A GraphSAGE-based model with fingerprints only to predict drug-drug interactions, 2024, 21, 1551-0018, 2922, 10.3934/mbe.2024130 | |
3. | QingLan Ma, Lei Chen, KaiYan Feng, Wei Guo, Tao Huang, Yu-Dong Cai, Exploring Prognostic Gene Factors in Breast Cancer via Machine Learning, 2024, 0006-2928, 10.1007/s10528-024-10712-w | |
4. | Yong Yang, Yuhang Zhang, Jingxin Ren, Kaiyan Feng, Zhandong Li, Tao Huang, Yudong Cai, Identification of Colon Immune Cell Marker Genes Using Machine Learning Methods, 2023, 13, 2075-1729, 1876, 10.3390/life13091876 | |
5. | Lei Chen, Ruyun Qu, Xintong Liu, Improved multi-label classifiers for predicting protein subcellular localization, 2023, 21, 1551-0018, 214, 10.3934/mbe.2024010 | |
6. | Qinglan Ma, Yulong Shen, Wei Guo, Kaiyan Feng, Tao Huang, Yudong Cai, Machine Learning Reveals Impacts of Smoking on Gene Profiles of Different Cell Types in Lung, 2024, 14, 2075-1729, 502, 10.3390/life14040502 | |
7. | Lei Chen, Chenyu Zhang, Jing Xu, PredictEFC: a fast and efficient multi-label classifier for predicting enzyme family classes, 2024, 25, 1471-2105, 10.1186/s12859-024-05665-1 | |
8. | Wenwei Wu, Tao He, Xiaying Hao, Kaiwei Xu, Ji Zeng, Jiahui Gu, Lei Chen, Zhengmao Li, Machine learning based method for analyzing vibration and noise in large cruise ships, 2024, 19, 1932-6203, e0307835, 10.1371/journal.pone.0307835 | |
9. | JingXin Ren, Qian Gao, XianChao Zhou, Lei Chen, Wei Guo, KaiYan Feng, Tao Huang, Yu-Dong Cai, Identification of key gene expression associated with quality of life after recovery from COVID-19, 2024, 62, 0140-0118, 1031, 10.1007/s11517-023-02988-8 | |
10. | Jing Xin Ren, Lei Chen, Wei Guo, Kai Yan Feng, Yu-Dong Cai, Tao Huang, Patterns of Gene Expression Profiles Associated with Colorectal Cancer in Colorectal Mucosa by Using Machine Learning Methods, 2024, 27, 13862073, 2921, 10.2174/0113862073266300231026103844 | |
11. | Jiyu Zhang, Tao Huang, Qiao Sun, Jian Zhang, Identifying Pathological Myopia Associated Genes with A Random Walk-Based Method in Protein-Protein Interaction Network, 2024, 19, 15748936, 375, 10.2174/0115748936268218231114070754 | |
12. | Jingxin Ren, XianChao Zhou, Ke Huang, Lei Chen, Wei Guo, KaiYan Feng, Tao Huang, Yu-Dong Cai, Identification of key genes associated with persistent immune changes and secondary immune activation responses induced by influenza vaccination after COVID-19 recovery by machine learning methods, 2024, 169, 00104825, 107883, 10.1016/j.compbiomed.2023.107883 | |
13. | Lei Chen, Xiaoyu Zhao, PCDA-HNMP: Predicting circRNA-disease association using heterogeneous network and meta-path, 2023, 20, 1551-0018, 20553, 10.3934/mbe.2023909 | |
14. | Lei Chen, Linyang Li, Prediction of Drug Pathway-based Disease Classes using Multiple Properties of Drugs, 2024, 19, 15748936, 859, 10.2174/0115748936284973240105115444 | |
15. | Yaowen Gu, Si Zheng, Bowen Zhang, Hongyu Kang, Rui Jiang, Jiao Li, Deep multiple instance learning on heterogeneous graph for drug–disease association prediction, 2025, 184, 00104825, 109403, 10.1016/j.compbiomed.2024.109403 | |
16. | Lei Chen, Shiyi Zhang, Bo Zhou, Herb-disease association prediction model based on network consistency projection, 2025, 15, 2045-2322, 10.1038/s41598-025-87521-7 | |
17. | QingLan Ma, Jingxin Ren, Lei Chen, Wei Guo, KaiYan Feng, Tao Huang, Yu-Dong Cai, Identifying Key Clinical Indicators Associated with the Risk of Death in Hospitalized COVID-19 Patients, 2025, 20, 15748936, 359, 10.2174/0115748936306893240720192301 |
Classification algorithm | Negative sample pool | Dimension | SN | SP | ACC | Precision | F1-measure | MCC | |
Drug feature | Disease feature | ||||||||
Random forest | NS(0.05) | 1000 | 1000 | 1.000 | 0.979 | 0.989 | 0.979 | 0.989 | 0.979 |
NS(0.1) | 850 | 900 | 1.000 | 0.953 | 0.975 | 0.950 | 0.974 | 0.951 | |
Bayes network | NS(0.05) | 550 | 50 | 1.000 | 0.979 | 0.989 | 0.979 | 0.989 | 0.979 |
NS(0.1) | 550 | 50 | 1.000 | 0.952 | 0.975 | 0.950 | 0.974 | 0.950 | |
Nearest neighbor algorithm | NS(0.05) | 1000 | 50 | 0.978 | 0.974 | 0.976 | 0.974 | 0.976 | 0.951 |
NS(0.1) | 1000 | 50 | 0.956 | 0.954 | 0.955 | 0.954 | 0.955 | 0.910 |
Classification algorithm | Negative sample pool | Dimension | SN | SP | ACC | Precision | F1-measure | MCC | |
Drug feature | Disease feature | ||||||||
Random forest | NS(0.05) | 1000 | 1000 | 1.000 | 0.989 | 0.993 | 0.979 | 0.989 | 0.984 |
NS(0.1) | 850 | 900 | 0.999 | 0.975 | 0.983 | 0.950 | 0.974 | 0.962 | |
Bayes network | NS(0.05) | 550 | 50 | 1.000 | 0.989 | 0.993 | 0.979 | 0.989 | 0.984 |
NS(0.1) | 550 | 50 | 1.000 | 0.975 | 0.983 | 0.950 | 0.974 | 0.963 | |
Nearest neighbor algorithm | NS(0.05) | 1000 | 50 | 0.978 | 0.985 | 0.983 | 0.971 | 0.974 | 0.962 |
NS(0.1) | 1000 | 50 | 0.953 | 0.973 | 0.967 | 0.946 | 0.950 | 0.925 |
Classification algorithm | Negative sample pool | Dimension | SN | SP | ACC | Precision | F1-measure | MCC | |
Drug feature | Disease feature | ||||||||
Random forest | NS(0.05) | 1000 | 1000 | 0.999 | 0.992 | 0.994 | 0.979 | 0.989 | 0.984 |
NS(0.1) | 850 | 900 | 0.999 | 0.984 | 0.987 | 0.950 | 0.974 | 0.966 | |
Bayes network | NS(0.05) | 550 | 50 | 1.000 | 0.992 | 0.994 | 0.979 | 0.989 | 0.985 |
NS(0.1) | 550 | 50 | 1.000 | 0.984 | 0.987 | 0.950 | 0.974 | 0.966 | |
Nearest neighbor algorithm | NS(0.05) | 1000 | 50 | 0.976 | 0.988 | 0.985 | 0.970 | 0.973 | 0.962 |
NS(0.1) | 1000 | 50 | 0.951 | 0.982 | 0.974 | 0.945 | 0.948 | 0.931 |
Classification algorithm | Ratio of positive and negative samples | SN | SP | ACC | Precision | F1-measure | MCC | AUROC | AUPR |
Random forest | 1:1 | 0.739 | 0.782 | 0.761 | 0.773 | 0.756 | 0.522 | 0.818 | 0.803 |
1:2 | 0.570 | 0.884 | 0.779 | 0.711 | 0.632 | 0.483 | 0.818 | 0.690 | |
1:3 | 0.435 | 0.925 | 0.803 | 0.659 | 0.524 | 0.420 | 0.807 | 0.591 | |
Bayes network | 1:1 | 0.667 | 0.837 | 0.752 | 0.804 | 0.729 | 0.512 | 0.751 | 0.703 |
1:2 | 0.673 | 0.836 | 0.782 | 0.672 | 0.672 | 0.509 | 0.751 | 0.558 | |
1:3 | 0.675 | 0.826 | 0.788 | 0.564 | 0.614 | 0.473 | 0.747 | 0.460 | |
Nearest neighbor algorithm | 1:1 | 0.695 | 0.685 | 0.690 | 0.688 | 0.691 | 0.380 | 0.671 | 0.616 |
1:2 | 0.571 | 0.780 | 0.711 | 0.565 | 0.568 | 0.351 | 0.660 | 0.451 | |
1:3 | 0.497 | 0.826 | 0.744 | 0.487 | 0.492 | 0.321 | 0.638 | 0.346 |
Classification algorithm | Ratio of positive and negative samples | SN | SP | ACC | Precision | F1-measure | MCC | AUROC | AUPR |
Random forest | 1:1 | 0.053 | 0.867 | 0.460 | 0.271 | 0.088 | -0.142 | 0.600 | 0.510 |
Bayes network | 1:1 | 0.662 | 0.809 | 0.736 | 0.776 | 0.714 | 0.477 | 0.734 | 0.684 |
Nearest neighbor algorithm | 1:1 | 0.732 | 0.757 | 0.745 | 0.751 | 0.741 | 0.490 | 0.722 | 0.669 |
Model | SN | SP | ACC | Precision | F1-measure | MCC | AUROC | AUPR |
Our model | 1.000 | 0.979 | 0.989 | 0.979 | 0.989 | 0.979 | 0.996 | 0.997 |
RepCOOL [24] | 0.930 | - | - | 0.530 | 0.670 | - | 0.670 | - |
RLFDDA [25] | 0.897 | - | 0.901 | 0.904 | 0.900 | - | 0.964 | - |
Li et al.' method [26] | 0.862 | 0.868 | 0.865 | 0.867 | - | 0.730 | 0.936 | 0.935 |
MGP-DDA [23] | 0.842 | - | 0.867 | 0.886 | 0.863 | - | 0.930 | 0.944 |
Yang and Chen's method [21] | 0.872 | 0.843 | 0.858 | 0.847 | 0.860 | 0.716 | 0.928 | 0.919 |
$: Measurements for all methods except our method were directly picked up from their corresponding literature; -: This measurement was not reported. |
Classification algorithm | Negative sample pool | Dimension | SN | SP | ACC | Precision | F1-measure | MCC | |
Drug feature | Disease feature | ||||||||
Random forest | NS(0.05) | 1000 | 1000 | 1.000 | 0.979 | 0.989 | 0.979 | 0.989 | 0.979 |
NS(0.1) | 850 | 900 | 1.000 | 0.953 | 0.975 | 0.950 | 0.974 | 0.951 | |
Bayes network | NS(0.05) | 550 | 50 | 1.000 | 0.979 | 0.989 | 0.979 | 0.989 | 0.979 |
NS(0.1) | 550 | 50 | 1.000 | 0.952 | 0.975 | 0.950 | 0.974 | 0.950 | |
Nearest neighbor algorithm | NS(0.05) | 1000 | 50 | 0.978 | 0.974 | 0.976 | 0.974 | 0.976 | 0.951 |
NS(0.1) | 1000 | 50 | 0.956 | 0.954 | 0.955 | 0.954 | 0.955 | 0.910 |
Classification algorithm | Negative sample pool | Dimension | SN | SP | ACC | Precision | F1-measure | MCC | |
Drug feature | Disease feature | ||||||||
Random forest | NS(0.05) | 1000 | 1000 | 1.000 | 0.989 | 0.993 | 0.979 | 0.989 | 0.984 |
NS(0.1) | 850 | 900 | 0.999 | 0.975 | 0.983 | 0.950 | 0.974 | 0.962 | |
Bayes network | NS(0.05) | 550 | 50 | 1.000 | 0.989 | 0.993 | 0.979 | 0.989 | 0.984 |
NS(0.1) | 550 | 50 | 1.000 | 0.975 | 0.983 | 0.950 | 0.974 | 0.963 | |
Nearest neighbor algorithm | NS(0.05) | 1000 | 50 | 0.978 | 0.985 | 0.983 | 0.971 | 0.974 | 0.962 |
NS(0.1) | 1000 | 50 | 0.953 | 0.973 | 0.967 | 0.946 | 0.950 | 0.925 |
Classification algorithm | Negative sample pool | Dimension | SN | SP | ACC | Precision | F1-measure | MCC | |
Drug feature | Disease feature | ||||||||
Random forest | NS(0.05) | 1000 | 1000 | 0.999 | 0.992 | 0.994 | 0.979 | 0.989 | 0.984 |
NS(0.1) | 850 | 900 | 0.999 | 0.984 | 0.987 | 0.950 | 0.974 | 0.966 | |
Bayes network | NS(0.05) | 550 | 50 | 1.000 | 0.992 | 0.994 | 0.979 | 0.989 | 0.985 |
NS(0.1) | 550 | 50 | 1.000 | 0.984 | 0.987 | 0.950 | 0.974 | 0.966 | |
Nearest neighbor algorithm | NS(0.05) | 1000 | 50 | 0.976 | 0.988 | 0.985 | 0.970 | 0.973 | 0.962 |
NS(0.1) | 1000 | 50 | 0.951 | 0.982 | 0.974 | 0.945 | 0.948 | 0.931 |
Classification algorithm | Ratio of positive and negative samples | SN | SP | ACC | Precision | F1-measure | MCC | AUROC | AUPR |
Random forest | 1:1 | 0.739 | 0.782 | 0.761 | 0.773 | 0.756 | 0.522 | 0.818 | 0.803 |
1:2 | 0.570 | 0.884 | 0.779 | 0.711 | 0.632 | 0.483 | 0.818 | 0.690 | |
1:3 | 0.435 | 0.925 | 0.803 | 0.659 | 0.524 | 0.420 | 0.807 | 0.591 | |
Bayes network | 1:1 | 0.667 | 0.837 | 0.752 | 0.804 | 0.729 | 0.512 | 0.751 | 0.703 |
1:2 | 0.673 | 0.836 | 0.782 | 0.672 | 0.672 | 0.509 | 0.751 | 0.558 | |
1:3 | 0.675 | 0.826 | 0.788 | 0.564 | 0.614 | 0.473 | 0.747 | 0.460 | |
Nearest neighbor algorithm | 1:1 | 0.695 | 0.685 | 0.690 | 0.688 | 0.691 | 0.380 | 0.671 | 0.616 |
1:2 | 0.571 | 0.780 | 0.711 | 0.565 | 0.568 | 0.351 | 0.660 | 0.451 | |
1:3 | 0.497 | 0.826 | 0.744 | 0.487 | 0.492 | 0.321 | 0.638 | 0.346 |
Classification algorithm | Ratio of positive and negative samples | SN | SP | ACC | Precision | F1-measure | MCC | AUROC | AUPR |
Random forest | 1:1 | 0.053 | 0.867 | 0.460 | 0.271 | 0.088 | -0.142 | 0.600 | 0.510 |
Bayes network | 1:1 | 0.662 | 0.809 | 0.736 | 0.776 | 0.714 | 0.477 | 0.734 | 0.684 |
Nearest neighbor algorithm | 1:1 | 0.732 | 0.757 | 0.745 | 0.751 | 0.741 | 0.490 | 0.722 | 0.669 |
Model | SN | SP | ACC | Precision | F1-measure | MCC | AUROC | AUPR |
Our model | 1.000 | 0.979 | 0.989 | 0.979 | 0.989 | 0.979 | 0.996 | 0.997 |
RepCOOL [24] | 0.930 | - | - | 0.530 | 0.670 | - | 0.670 | - |
RLFDDA [25] | 0.897 | - | 0.901 | 0.904 | 0.900 | - | 0.964 | - |
Li et al.' method [26] | 0.862 | 0.868 | 0.865 | 0.867 | - | 0.730 | 0.936 | 0.935 |
MGP-DDA [23] | 0.842 | - | 0.867 | 0.886 | 0.863 | - | 0.930 | 0.944 |
Yang and Chen's method [21] | 0.872 | 0.843 | 0.858 | 0.847 | 0.860 | 0.716 | 0.928 | 0.919 |
$: Measurements for all methods except our method were directly picked up from their corresponding literature; -: This measurement was not reported. |