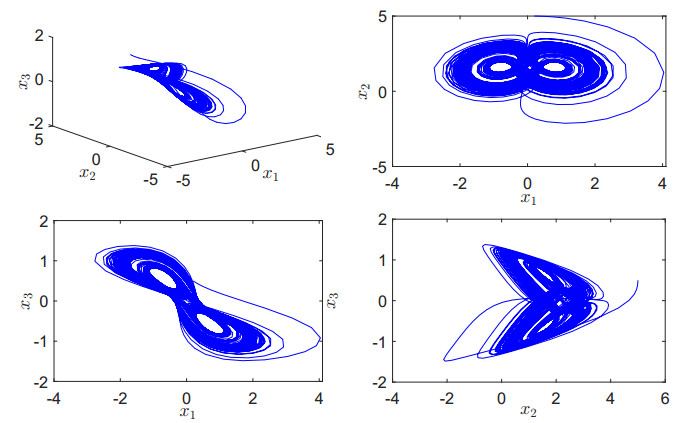
Aiming at the problem that the convergence time of the chaotic finance/economic system cannot be set independently and the continuous macro-control is required, this paper investigates the predefined-time control of the chaotic finance/economic system based on event-triggered mechanism. The predefined-time control approach ensures the chaotic finance system quickly converge to the stable state within a pre-determined time. Moreover, in order to avoid continuous macro-control, an event-trigger mechanism is added into the above predefined-time control approach, which guarantees that the control input is updated only when some predefined event occurs. Rigorous theoretical derivation is presented and concrete simulation experiments are carried out to validate the feasibility and applicability of the proposed control strategy.
Citation: Qiaoping Li, Yu Chen, Lingyuan Ma. Predefined-time control of chaotic finance/economic system based on event-triggered mechanism[J]. AIMS Mathematics, 2023, 8(4): 8000-8017. doi: 10.3934/math.2023404
[1] | Chao Ma, Tianbo Wang, Wenjie You . Master-slave synchronization of Lurie systems with time-delay based on event-triggered control. AIMS Mathematics, 2023, 8(3): 5998-6008. doi: 10.3934/math.2023302 |
[2] | Tao Xie, Xing Xiong . Finite-time synchronization of fractional-order heterogeneous dynamical networks with impulsive interference via aperiodical intermittent control. AIMS Mathematics, 2025, 10(3): 6291-6317. doi: 10.3934/math.2025287 |
[3] | Qiaoping Li, Sanyang Liu . Predefined-time vector-polynomial-based synchronization among a group of chaotic systems and its application in secure information transmission. AIMS Mathematics, 2021, 6(10): 11005-11028. doi: 10.3934/math.2021639 |
[4] | Biwen Li, Yujie Liu . Quasi-synchronization of nonlinear systems with parameter mismatch and time-varying delays via event-triggered impulsive control. AIMS Mathematics, 2025, 10(2): 3759-3778. doi: 10.3934/math.2025174 |
[5] | Le You, Chuandong Li, Xiaoyu Zhang, Zhilong He . Edge event-triggered control and state-constraint impulsive consensus for nonlinear multi-agent systems. AIMS Mathematics, 2020, 5(5): 4151-4167. doi: 10.3934/math.2020266 |
[6] | Tian Xu, Jin-E Zhang . Intermittent control for stabilization of uncertain nonlinear systems via event-triggered mechanism. AIMS Mathematics, 2024, 9(10): 28487-28507. doi: 10.3934/math.20241382 |
[7] | Kairui Chen, Yongping Du, Shuyan Xia . Adaptive state observer event-triggered consensus control for multi-agent systems with actuator failures. AIMS Mathematics, 2024, 9(9): 25752-25775. doi: 10.3934/math.20241258 |
[8] | Jiaojiao Li, Yingying Wang, Jianyu Zhang . Event-triggered sliding mode control for a class of uncertain switching systems. AIMS Mathematics, 2023, 8(12): 29424-29439. doi: 10.3934/math.20231506 |
[9] | Zuo Wang, Hong Xue, Yingnan Pan, Hongjing Liang . Adaptive neural networks event-triggered fault-tolerant consensus control for a class of nonlinear multi-agent systems. AIMS Mathematics, 2020, 5(3): 2780-2800. doi: 10.3934/math.2020179 |
[10] | Jiaqi Liang, Zhanheng Chen, Zhiyong Yu, Haijun Jiang . Fixed-time consensus of second-order multi-agent systems based on event-triggered mechanism under DoS attacks. AIMS Mathematics, 2025, 10(1): 1501-1528. doi: 10.3934/math.2025070 |
Aiming at the problem that the convergence time of the chaotic finance/economic system cannot be set independently and the continuous macro-control is required, this paper investigates the predefined-time control of the chaotic finance/economic system based on event-triggered mechanism. The predefined-time control approach ensures the chaotic finance system quickly converge to the stable state within a pre-determined time. Moreover, in order to avoid continuous macro-control, an event-trigger mechanism is added into the above predefined-time control approach, which guarantees that the control input is updated only when some predefined event occurs. Rigorous theoretical derivation is presented and concrete simulation experiments are carried out to validate the feasibility and applicability of the proposed control strategy.
During the past several years, a novel science branch called economic physics has risen gradually, in which, the research approaches based on mathematics, physics and complex sciences are applied to explain and deal with the financial and economical problems.
Finance/economic system [1,2,3] is an open and irreversible entropy increasing process which is far away from the equilibrium point and is constructed by many factors. Influenced by the changes of various parameters, it is quite common for its motion state to appear chaotic state due to instability. Previous research results show that chaos theory can reasonably explain the internal operation mechanism of economic phenomena and the essential changes in economic prospects. Therefore, nonlinear chaotic finance/economic economics has gradually developed into a research highlight in the field of economic physics, and has obtained fruitful achievement.
Considering that the appearance of chaotic behaviour in the finance/economic system will lead to the uncertainty and unpredictability of macroeconomic control, various control techniques have been proposed to ensure the chaotic finance/economic system stabilises to the equilibrium point, such as feedback control [4], sliding mode control [5], adaptive control [6,7], fuzzy control [8], active control [9,10], H∞ control [11], and so forth.
It is worth noting that, most of the above research results are based on the asymptotic stability control strategy, therefore, the convergence time of the closed-loop system is infinite and the convergence speed is slow. In fact, it is of more practical significance to realize the stability of the chaotic finance/economic system within a finite time. Based on the above purpose, the concept of finite-time stability the chaotic finance/economic system has been investigated. Applying the time-delay feedback control technique, Chen investigated the finite-time H∞ control problem of the chaotic finance system with external disturbance [12]. A finite-time resilient fault-tolerant guaranteed cost control scheme is proposed by Ahmad, to solve the fluctuations in investment policy strategy for the chaotic nonlinear finance system [13]. For the chaotic finance/economic system with perturbance, Ahmad designed a finite-time controller, which can not only eliminate the influence of external perturbance, but also ensure that the perturbed state converges to the equilibrium point quickly in a finite time without oscillation [14]. However, in the finite-time control algorithm, the convergence time of the closed-loop system depends heavily on the initial state and control parameters of the system [15]. Therefore, it will be of more practical significance to design a novel control scheme so that the convergence time of the finance/economic system can be set independently in advance according to the economic market demand, without being affected by the initial state of the system or other control gains. This is the main objective of this paper.
The existing control schemes of chaotic finance/economic system all adopt continuous control strategy, which needs real-time regulation. During the operation process of the financial system, frequent regulations, on the one hand, will increase the operation cost, on the other hand, it is not easy to operate in practice. Therefore, it is essential and urgent for the control of chaotic finance/economic system to reduce the update frequency of control input, without damaging the good convergence performance of the controlled system.
Compared with the traditional control technology, event-triggered control is an emerging discontinuous control strategy [16,17]. It originates from the control problem of network system and aims to reduce the update frequency of network data, thus reducing the load of network communication. Its core idea is to add an event trigger between the sensor and the controller, which can recognize the error between the sampling output at the current time and the sampling output at the previous event-triggered time. The sampled data is transferred to the controller only if the above error violates some pre-set threshold, otherwise, the controller input remains unchanged. Compared with the continuous control strategy, the event-triggered control strategy can effectively reduce the update frequency of the controller input [18,19,20,21]. If the above event-triggered control strategy can be applied to the control process of the chaotic finance/economic system, it will effectively reduce the frequency of macro-control. Therefore, this is an interesting and valuable work. So far, this subject is still open, so it is another motivation of this paper.
Motivated by the above analysis, this paper is dedicated to investigate the predefined-time control of chaotic finance/economic system based on the event-triggered mechanism. The rest of this paper is organized as follows. In Section 2, a novel concept called predefined-time stability and its related properties are introduced. In Section 3, the nonlinear dynamics of the chaotic finance/economic system is established and the object of this work is expounded. In Sections 4 and 5, the predefined-time control strategy and the event-triggered predefined-time control strategy are designed to realize the predefined-time stability of the chaotic finance/economic system, respectively. In Section 6, some simulation experiments are presented to illustrate the validity and superiority of the proposed control scheme. In Section 7, the conclusion and future work are declared.
The main highlights of this paper are summarized as below:
● Firstly, by designing the predefined-time control scheme, the stabilizing time Tp of the chaotic finance/economic system can be preseted off-line by the designer without being affected by the initial state of the system or other control gains, which is superior to the finite-time control technique.
● Secondly, the traditional finite-time control algorithm in the existing literatures needs to design many control parameters. However, the predefined-time control algorithm proposed in this paper only needs to design one control parameter q, which is easy to operate.
● Thirdly, by introducing the event-triggered mechanism into the control strategy, the frequency of macro-control policy of the government is effectively reduced without damaging the good convergence performance of the chaotic finance/economic system, which is of more practical value.
In this section, we will introduced a novel concept called predefined-time stability and some related properties.
Definition 1. [22] (Predefined-time stability.) Let Tp>0 be a constant which can be preset arbitrarily. The origin x(t)=0 of the nonlinear dynamical system
˙x(t)=f(t,x;ϱ),t∈[t0,+∞) | (2.1) |
is said to be globally predefined-time stable, if and only if
{limt→Tp−x(t,x0)=0,t∈[t0,t0+Tp)x(t,x0)≡0,t∈[t0+Tp,+∞) | (2.2) |
holds for arbitrary x0 of system (2.1). If so, the constant Tp is called the predefined-time. Here, x∈Rn denotes the system state, ϱ∈Rm denotes the system parameter which satisfies ˙ϱ=0, t0≥0 represents the initial time and x0=x(t0) stands for the initial state for system (2.1).
Lemma 1. [23] Let Tp>0 be a predefined constant. If there exists a radially unbounded Lyapunov function V(t) for the dynamics system (2.1) and it satisfies
˙V(t)≤−πpTp(V1+p2+V1−p2), |
where p∈(0,1) is a real constant.
Then, the origin of system (2.1) will be globally predefined-time stable within the predefined-time Tp.
Proof. For any initial state x0∈Rn of system (2.1), the convergence time T(x0) can be calculated as
T(x0)=∫T(x0)t0dt=∫0V(x0)1˙V(t)dV≤∫0V(x0)−1πpTp(V1+p2+V1−p2)dV=pTpπ∫V(x0)0dVV1−p2(1+Vp)=pTpπ⋅2p⋅∫V(x0)0dVp21+Vα=pTpπ⋅2p⋅arctan(Vp2)|V(x0)0=2Tpπarctan(Vp2(x0)). |
In virtue of V(x0)≥0, we obtain
arctan(Vp2(x0))∈(0,π2]. |
Hence it holds that
Tp=supT(x0). |
Remark 1. From Lemma 1, it can be seen that, the convergence time Tp for predefined-time stability can be pre-specified without being affected by the initial state x0 or other system parameter ϱ, thus it can be set freely and has more practical value.
Definition 2. For the vector ξ=(ξ1,⋯,ξN)⊤∈RN and constant q∈R∖{0}, the functions |⋅|:ξ↦|ξ| and ⌈⋅⌋q:x↦⌈ξ⌋q are defined as
|ξ|=(|ξ1|,⋯,|ξN|)⊤, | (2.3) |
⌈ξ⌋q=(sign(ξ1)|ξ1|q,⋯,sign(ξN)|ξN|q)⊤, | (2.4) |
in which sign(⋅) refers to the sign function.
Specially, for q=1, it holds that
⌈ξ⌋=(sign(ξ1)|ξ1|,⋯,sign(ξN)|ξN|)⊤. | (2.5) |
Lemma 2. [24] Let ξ1,ξ2,⋯,ξN≥0. Then,
i) It holds for m∈(0,1) that
(N∑i=1ξi)m≤N∑i=1ξmi≤N1−m(N∑i=1ξi)m. | (2.6) |
ii) It holds for m∈[1,+∞) that
N1−m(N∑i=1ξi)m≤N∑i=1ξmi≤(N∑i=1ξi)m. | (2.7) |
Lemma 3. [25] Let ξ∈RN. Then
‖ξ‖m≤‖ξ‖n≤N(1n−1m)‖ξ‖m, | (2.8) |
where m,n denote two real constants which satisfy m>n>0. ‖ξ‖m refers to the m−norm of ξ, which is defined as
‖ξ‖m=(N∑i=1|ξi|m)1m. | (2.9) |
Specially, for m=2,n=1, it holds that
‖ξ‖2≤‖ξ‖1≤N12‖ξ‖2. | (2.10) |
Lemma 4. [17] For a constant p∈(0,1] and two vectors x,y∈Rn, it holds that
‖x+y‖p1≤2p−1(‖x‖p1+‖y‖p1). | (2.11) |
Moreover, if ‖x‖1≥‖y‖1, then
−x⊤⌈x+y⌋p≤−21−px⊤⌈x⌋p+2p−1∣x∣⊤∣y∣p. | (2.12) |
Lemma 5. [17] For a constant p∈[1,+∞) and two vectors x,y∈Rn, it holds that
‖x+y‖p1≤‖x‖p1+‖y‖p1. | (2.13) |
Moreover, if ‖x‖1≥‖y‖1, then
−x⊤⌈x+y⌋p≤−x⊤⌈x⌋p+∣x∣⊤∣y∣p. | (2.14) |
Lemma 6. (Young's inequality) Let p,q∈(1,+∞) be two real constants, which satisfy
1p+1q=1. |
Then, it holds for any constants x,y∈R+∪0 that
xy≤xpp+yqq. | (2.15) |
Consider the chaotic finance/economic system proposed in [26], which is composed of four sub-components that include production, stock, money, and labor force. The mathematical model of the above chaotic finance/economic system is described by
{˙x1(t)=x3(t)+x1(t)(x2(t)−a)+u1(t),˙x2(t)=1−bx2(t)−x21(t)+u2(t),˙x3(t)=−x1(t)−cx3(t)+u3(t), | (3.1) |
where the vector
x(t)=(x1(t),x2(t),x3(t))⊤∈R3 |
denotes the system state, whose elements x1,x2,x3∈R stand for the interest rate, the investment demand, and the price index, respectively; the system parameters a,b,c∈R+ stand for the saving amount, the cost per investment, and the elasticity of demand of the commercial markets; the vector u(t)=(u1(t),u2(t),u3(t))⊤∈R3 represents the control input (i.e., macro-control measures of the government). The stability and bifurcation of these balancing points have been discussed detailed [26,27,28]. When we set
(a,b,c)⊤=(0.9,0.2,1.2)⊤,(x1(0),x2(0),x3(0))⊤=(0.2,5,0.5)⊤ |
and
(u1(0),u2(0),u3(0))⊤=(0,0,0)⊤, |
the chaotic attractors of system (3.1) are displayed by Figure 1.
In order to independently control the convergence time Tp of the closed-loop system, we propose the following predefined-time controller.
u(t)=ˉx(t)−κ1⌈x(t)⌋1−q−κ2⌈x(t)⌋1+q, | (4.1) |
where Tp>0 denotes the predetermined convergence time, q∈(0,1) stands for the control gain, and
ˉx(t)=(ax1(t),bx2(t)−1,cx3(t))⊤,κ1=n1−qπ21−q2qTp,κ2=n1+qπ21+q2qTp,n=3. |
Theorem 1. For the chaotic finance/economic system (3.1), the state trajectory x(t) will stabilize to the origin (0,0,0)⊤ within in any predefined-time Tp>0 under the action of the control law (4.1).
Proof. Choose the following Lyapunov function
V(t)=12x⊤(t)x(t). | (4.2) |
It is easy to calculate
˙V(t)=x⊤(t)˙x(t)=n∑i=1xi(t)˙xi(t)=n∑i=1xi(t)⋅(−κ1sign(xi(t))|xi(t)|1−q−κ2sign(xi(t))|xi(t)|1+q)=n∑i=1(−κ1|xi(t)|2−q−κ2|xi(t)|2+q)=−κ1n∑i=1|xi(t)|2−q−κ2n∑i=1|xi(t)|2+q. | (4.3) |
Since 2−q>1, 2+q>1, then, applying Lemmas 2 and 3, we obtain
˙V(t)≤−κ1⋅n−1+q‖xi(t)‖2−q1−κ2⋅n−1−q‖xi(t)‖2+q1≤−κ1⋅n−1+q‖xi(t)‖2−q2−κ2⋅n−1−q‖xi(t)‖2+q2=−πqTp(12‖xi(t)‖22)1−q2−πqTp(12‖xi(t)‖22)1+q2=−πqTp(V1−q2(t)+V1+q2(t)). | (4.4) |
Therefore, according to Lemma 1, the state trajectory x(t) of the chaotic finance/economic system (3.1) will converge to the original point (0,0,0)⊤ within the predefined time Tp.
The framework of the event-triggered control scheme is shown by Figure 2 and the event-triggered mechanism is described as below.
Let t0=0 be the first event-triggered time, and tk denote the latest event-triggered time for the current time t, then, the next event-triggered time tk+1 is defined as
tk+1=min{t>tk:‖x(t)‖1>ϵand‖δ(t)‖1>σ‖x(tk)‖1}, | (5.1) |
where h>0, δ(t)=x(tk)−x(t), t∈[tk,tk+1). The constants σ∈[0,1) and ϵ≥0 are the threshold parameters which determine the transmission frequency of the output-sample x(t). It is obvious that limk→+∞tk=+∞.
Remark 2. In the event-triggered transmission scheme, once the current sample-data x(t) violates the following event-triggered condition
‖x(t)‖1≤ϵor(‖x(t)‖1>ϵand‖δ(t)‖1≤σ‖x(t)‖1),t∈[tk,tk+1) | (5.2) |
an event will be generated by the event trigger, which means the current sample-data x(t) will be transmitted to the controller and the control input u(t) will be updated. Otherwise, the current sample-data x(t) will be discarded and the control input u(t) will be kept by a zero-order hold (ZOH) with the holding time [tk,tk+1).
Remark 3. It can be shown from (5.2) that, the condition ‖x(t)‖1≤ϵ determines the requirement of finance/ecnomics system managers for control accuracy, while the condition ‖δ(t)‖1≤σ‖x(t)‖1 characterizes the tolerance of the system to the relative error
‖δ(t)‖1‖x(t)‖1=‖x(tk)−x(t)‖1‖x(t)‖1. |
Obviously, the smaller the threshold parameters ϵ and σ, the higher the control performance of the system, and the higher the update frequency of control input. The larger the threshold parameters ϵ and σ, the lower the control performance requirements of the system, and the lower the update frequency of control input. In particular, when the threshold parameters ϵ=σ=0, the event-triggered control degenerates into the traditional continuous control.
To ensure that the chaotic finance/ecnomic system stabilizes to the equilibrium point (0,0,0)⊤ within the predetermined time Tp, the following event-triggered control law is designed.
u(t)=ˉx(tk)−β1α1⌈x(tk)⌋μ1−β2α2⌈x(tk)⌋μ2−β3α3⌈x(tk)⌋,t∈[tk,tk+1) | (5.3) |
where 0<q<1 and
ˉx(tk)=(ax1(tk),bx2(tk)−1,cx3(tk))⊤,μ1=1−q,μ2=1+q,β1=π21−q2qTp,β2=π21+q2qTp,β3=Mσn,α1=1μ11+μ1(1−σ1+μ1n1+μ12),α2=1(21−μ2−2μ2−11+μ2)n1−μ22−2μ2−1μ21+μ2σ1+μ2n1+μ22,α3=112(1−σ2n),M=max{a,b,c},n=3. |
Theorem 2. For the chaotic finance/economic system (3.1), if the event-triggered mechanism (5.1) and the control law (5.3) are employed, then the state trajectory x(t) will stabilize to the origin (0,0,0)⊤ within in any predefined-time Tp>0.
Proof. Construct the Lyapunov function candidate as below,
V(t)=12x⊤(t)x(t). | (5.4) |
Based on the event-triggered condition (5.2), we obtain, for any t∈[tk,tk+1), it holds that ‖x(t)‖1≤ϵ or (‖x(t)‖1>ϵ and ‖δ(t)‖1≤σ‖x(t)‖1), which implies ‖δ(t)‖1≤‖x(t)‖1.
Now we discuss it in two cases:
Case 1: ‖x(t)‖1≤ϵ.
In this case, the system state x(t) is fully close to the equilibrium point (0,0,0)⊤ thus meets the requirement of finance/economic system managers for control accuracy.
Case 2: ‖x(t)‖1>ϵ and ‖δ(t)‖1≤σ‖x(t)‖1.
Use the fact δ(t)=x(tk)−x(t), t∈[tk,tk+1), then, the time-derivative of (5.4) along the trajectory of chaotic finance/economic system (3.1) can be calculated as
˙V(t)=x⊤(t)˙x(t)=n∑i=1xi(t)˙xi(t)=x⊤(t)(−β1α1⌈x(tk)⌋μ1−β2α2⌈x(tk)⌋μ2−β3α3⌈x(tk)⌋+ˉx(tk)−ˉx(t))=−β1α1x⊤(t)⌈x(tk)⌋μ1−β2α2x⊤(t)⌈x(tk)⌋μ2−β3α3x⊤(t)⌈x(tk)⌋+x⊤(t)(ˉx(tk)−ˉx(t))=−β1α1x⊤(t)⌈x(t)+δ(t)⌋μ1−β2α2x⊤(t)⌈x(t)+δ(t)⌋μ2−β3α3x⊤(t)⌈x(t)+δ(t)⌋+x⊤(t)(ˉx(tk)−ˉx(t)). | (5.5) |
Since σ∈[0,1), it is easy to get ‖δ(t)‖1≤‖x(t)‖1.
Notice μ1=1−q and q∈(0,1), we get 0<μ1<1, 1<1+μ1<2 and 0<1+μ12<1. Applying Lemma 4, we have
−x⊤(t)⌈x(t)+δ(t)⌋μ1≤−x⊤(t)⌈x(t)⌋μ1+|x(t)|⊤|δ(t)|μ1=−n∑i=1∣xi(t)∣1+μ1+n∑i=1|xi(t)|⋅|δi(t)|μ1. | (5.6) |
Using Lemma 6, we derive
|xi(t)|⋅|δi(t)|μ1≤|xi(t)|1+μ11+μ1+μ1|δi(t)|1+μ11+μ1. | (5.7) |
Substituting (5.7) into (5.6), we obtain
−x⊤(t)⌈x(t)+δ(t)⌋μ1≤−n∑i=1|xi(t)|1+μ1+n∑i=1(|xi(t)|1+μ11+μ1+μ1|δi(t)|1+μ11+μ1)=μ11+μ1(−n∑i=1|xi(t)|1+μ1+n∑i=1|δi(t)|1+μ1). | (5.8) |
Based on Lemmas 2 and 3, we get
−n∑i=1|xi(t)|1+μ1≤−(n∑i=1|xi(t)|2)1+μ12=−‖x(t)‖1+μ12. | (5.9) |
n∑i=1|δi(t)|1+μ1≤‖δ(t)‖1+μ11≤σ1+μ1‖x(t)‖1+μ11≤σ1+μ1n1+μ12‖x(t)‖1+μ12. | (5.10) |
Combing (5.8) with (5.9) and (5.10), we have
−x⊤(t)⌈x(t)+δ(t)⌋μ1≤−μ11+μ1(1−σ1+μ1n1+μ12)‖x(t)‖1+μ12=−1α1‖x(t)‖1+μ12. | (5.11) |
Since μ2=1+q and q∈(0,1), it yields 1<μ2<2, 2<1+μ2<4 and 1<1+μ22<2. With the help of Lemmas 5 and 6, we derive that
−x⊤⌈x(t)+δ(t)⌋μ2≤−21−μ2x⊤(t)⌈x(t)⌋μ2+2μ2−1∣x(t)∣⊤∣δ(t)∣μ2=−21−μ2n∑i=1|xi(t)|1+μ2+2μ2−1n∑i=1|xi(t)|⋅|δi(t)|μ2. | (5.12) |
|xi(t)|⋅|δi(t)|μ2≤|xi(t)|1+μ21+μ2+μ2|δi(t)|1+μ211+μ2. | (5.13) |
Substituting (5.13) into (5.12), we deduce that
−x⊤(t)⌈x(t)+δ(t)⌋μ2≤−21−μ2n∑i=1∣xi(t)∣1+μ2+2μ2−1n∑i=1(|xi(t)|1+μ21+μ2+μ2|δi(t)|1+μ211+μ2)=−(21−μ2−2μ2−11+μ2)n∑i=1|xi(t)|1+μ2+2μ2−1μ21+μ2n∑i=1|δi(t)|1+μ2. | (5.14) |
In view of μ2=1+q and q∈(0,1), we obtain 1<μ2<2 and 1<1+μ22<2. According to Lemmas 2 and 3, we further get
−n∑i=1|xi(t)|1+μ2≤−n1−μ22(n∑i=1|xi(t)|2)1+μ22=−n1−μ22‖x(t)‖1+μ22. | (5.15) |
n∑i=1|δi(t)|1+μ2≤‖δ(t)‖1+μ21≤σ1+μ2‖x(t)‖1+μ21≤σ1+μ2n1+μ22‖x(t)‖1+μ22. | (5.16) |
Substituting (5.15) and (5.16) into (5.14), we obtain
−x⊤(t)⌈x(t)+δ(t)⌋μ2≤−((21−μ2−2μ2−11+μ2)n1−μ22−2μ2−1μ21+μ2σ1+μ2n1+μ22)‖x(t)‖1+μ22=−1α2‖x(t)‖1+μ22. | (5.17) |
Similary, it can be derived that
−x⊤(t)⌈x(t)+δ(t)⌋≤−12(1−σ2n)‖x(t)‖22=−1α3‖x(t)‖22. | (5.18) |
Let M=max{a,b,c} and employ Lemma 3, it yields
x⊤(t)(ˉx(tk)−ˉx(t))=ax1(t)(x1(tk)−x1(t))+bx2(t)(x2(tk)−x2(t))+cx3(t)(x3(tk)−x3(t))=ax1(t)δ1(t)+bx2(t)δ2(t)+cx3(t)δ3(t)≤M|x(t)|⊤⋅|δ(t)|≤M‖x(t)‖1⋅‖δ(t)‖1≤Mσ‖x(t)‖21≤Mσn‖x(t)‖22=β3‖x(t)‖22. | (5.19) |
Substituting (5.11) and (5.17)–(5.19) into (5.5), we obtain
˙V(t)≤−β1‖x(t)‖1+μ12−β2‖x(t)‖1+μ22−β3‖x(t)‖22+β3‖x(t)‖22=−β1‖x(t)‖1+μ12−β2‖x(t)‖1+μ22. | (5.20) |
Since μ1=1−q, μ2=1+q, we get
˙V(t)≤−β1(‖x(t)‖22)1−q2−β2(‖x(t)‖22)1+q2=−πqTp(12‖x(t)‖22)1−q2−πqTp(12‖x(t)‖22)1+q2=−πqTp(V1−q2(t)+V1+q2(t)). | (5.21) |
Therefore, it follows from Lemma 1 that the state trajectory x(t) of the chaotic finance/economic system (3.1) will converge to the original point (0,0,0)⊤ within the predefined time Tp.
Remark 4. It can be seen from the proof process of Theorem 2 that, the selection of threshold parameter σ should ensure α1>0,α2>0, and α3>0. Therefore, we stipulate σ∈[0,σmax) with
σmax=max{σ<1:α1>0,α2>0,andα3>0}, |
Similary, the control gain q∈(0,1) should comply
21−μ2−2μ2−11+μ2>0, |
which implies 2+q>4q.
To verify the effectiveness of the proposed control strategy, the following illustrative numerical example is carried out. The chaotic finance/economic system involved in this example is formulated by (3.1) and the system parameters and initial conditions are introduced in Section 3. The 2D and 3D phase portraits of system (3.1) without input are illustrated by Figure 1. The time response of each state variable without input is displayed by Figure 3. From Figures 1 and 3, it is appreciable that, the chaotic finance/economic system (3.1) has obvious chaotic characteristics.
Now we appoint the predefined convergence time as Tp=1, and carry out the simulation by applying the event-triggered mechanism (5.1) and the event-triggered control law (5.3), in which, the control gain q is taken as q=0.2. Based on Remark 4, we can calculate the upper bound of the threshold parameter σ as σmax=0.4206. So the threshold parameters of event-triggered mechanism in this simulation are chosen as ϵ=0.01 and σ=0.35. If the simulation step is fixed as h=0.001 and the simulation time is taken as 3, then the simulation results will be shown by Figures 4–8.
From Figure 4 it can be seen that, under the proposed control scheme, the trajectory of state variable xi(t) of chaotic finance/economic system (3.1) stabilizes to zero within the predefined-time Tp=1. Moreover, as shown in Figure 5, the state trajectory x(t) in 3D space converges to the origin (0,0,0)⊤ smoothly without chatting, which shows the effectiveness of the proposed control technique.
The event-triggered instants and event-holding times based on the proposed event-triggered mechanism are displayed by Figure 6, in which, the abscissa corresponding to each match stick represents an event-triggered instant tk, and the height of the match stick represents the holding time tk+1−tk of the k-th event. Figure 6 shows that the distribution of matchsticks is sparse, which means the event-triggered frequency is low, which further indicates that the introduction of the event-triggered mechanism can effectively reduce the update frequency of the controller and thus to save the control cost. This further reveals the applicability of the proposed control strategy.
Next, we carry out another two experiments to explore the role of threshold parameters in the event-triggered mechanism (5.1). The initial state and system parameters remain unchanged. First, we fix threshold parameter ϵ=0.01 and let σ choose different values, then the event-triggered frequencies are shown by Figure 7, from which one can see, compared with the continuous control technique (ϵ=σ=0), the event-triggered control technique can reduce the update frequency of the controller by at least 94.1%. What is more, the update frequency of the controller decreases with the increase of the value of σ.
Similarly, we fix threshold parameter σ=0.35 and let ϵ choose different values, then the event-triggered frequencies are shown by Figure 8, from which one can see, compared with the continuous control technique (ϵ=σ=0), the event-triggered control technique still can reduce the update frequency of the controller by at least 88.9% and the update frequency of the controller decreases with the increase of the value of ϵ.
To further demonstrate the superiority of the predefined-time control technology designed in this paper, we compare it with the famous finite-time control technology [12]. From the comparison of Figures 4 and 9, we can see that, the predefined-time control technology has faster convergence speed and shorter convergence time.
In this work, a novel event-triggered predefined-time control approach has been proposed to deal with the stability problem of chaotic finance/economic system. Both the theoretical and experimental results have illustrated that, the proposed control strategy can not only ensure that the system converges to the stable state quickly and smoothly within a predefined time, but also significantly reduce the update frequency of the control input, thereby saving the control cost. Recently, it is found that the fractional derivative can provide a favorable tool to describe the memory and hereditary properties of the dynamical behaviors of the financie/economic system [6,8,29,30]. Thereby, the control of the fractional-order financie/economic system is becoming increasingly attractive. Therefore, in our future works, we will dedicate to study the event-triggered predefined-time control of fractional-order financie/economic system.
The research is supported by the National Natural Science Foundation of China with Grant No. 61877046 and the Key Science and Technology Projects of Henan Province with Grant No. 222102210100, and No. 212102210552. Thanks for the editor and reviewers.
The authors declare that they have no competing interests.
[1] |
C. R. B. Moutsinga, E. Pindza, E. Maré, A robust spectral integral method for solving chaotic finance systems, Alex. Eng. J., 59 (2020), 601–611. https://doi.org/10.1016/j.aej.2020.01.016 doi: 10.1016/j.aej.2020.01.016
![]() |
[2] |
L. Shen, G. He, Threshold effect of financial system on high-quality economic development, J. Math., 2022 (2022), 9108130. https://doi.org/10.1155/2022/9108130 doi: 10.1155/2022/9108130
![]() |
[3] |
C. R. B. Moutsinga, E. Pindza, E. Mare, Comparative performance of time spectral methods for solving hyperchaotic finance and cryptocurrency systems, Chaos Solitons Fract., 145 (2021), 110770. https://doi.org/10.1016/j.chaos.2021.110770 doi: 10.1016/j.chaos.2021.110770
![]() |
[4] |
L. Chen, G. Chen, Controlling chaos in an economic model, Phys. A, 374 (2007), 349–358. https://doi.org/10.1016/j.physa.2006.07.022 doi: 10.1016/j.physa.2006.07.022
![]() |
[5] |
S. Dadras, H. R. Momeni, Control of a fractional-order economical system via sliding mode, Phys. A, 389 (2010), 2434–2442. https://doi.org/10.1016/j.physa.2010.02.025 doi: 10.1016/j.physa.2010.02.025
![]() |
[6] |
H. Liu, S. Li, J. Cao, G. Li, A. Alsaedi, F. E. Alsaadi, Adaptive fuzzy prescribed performance controller design for a class of uncertain fractional-order nonlinear systems with external disturbances, Neurocomputing, 219 (2017), 422–430. https://doi.org/10.1016/j.neucom.2016.09.050 doi: 10.1016/j.neucom.2016.09.050
![]() |
[7] |
S. Zheng, G. Dong, Q. Bi, Adaptive modified function projective synchronization of hyperchaotic systems with unknown parameters, Commun. Nonlinear Sci. Numer. Simul., 15 (2010), 3547–3556. https://doi.org/10.1016/j.cnsns.2009.12.010 doi: 10.1016/j.cnsns.2009.12.010
![]() |
[8] |
C. Wang, Adaptive fuzzy control for uncertain fractional-order financial chaotic systems subjected to input saturation, PLoS ONE, 11 (2016), 0164791. https://doi.org/10.1371/journal.pone.0164791 doi: 10.1371/journal.pone.0164791
![]() |
[9] |
H. M. Baskonus, T. Mekkaoui, Z. Hammouch, H. Bulut, Active control of a chaotic fractional order economic system, Entropy, 17 (2015), 5771–5783. https://doi.org/10.3390/e17085771 doi: 10.3390/e17085771
![]() |
[10] |
I. Pan, S. Das, S. Das, Multi-objective active control policy design for commensurate and incommensurate fractional order chaotic financial systems, Appl. Math. Model., 39 (2015), 500–514. https://doi.org/10.1016/j.apm.2014.06.005 doi: 10.1016/j.apm.2014.06.005
![]() |
[11] |
M. Zhao, J. Wang, H∞ control of a chaotic finance system in the presence of external disturbance and input time-delay, Appl. Math. Comput., 233 (2014), 320–327. https://doi.org/10.1016/j.amc.2013.12.085 doi: 10.1016/j.amc.2013.12.085
![]() |
[12] |
E. Xu, Y. Chen, J. Yang, Finite-time H∞ control for a chaotic finance system via delayed feedback, Syst. Sci. Control Eng., 6 (2018), 467–476. https://doi.org/10.1080/21642583.2018.1537863 doi: 10.1080/21642583.2018.1537863
![]() |
[13] |
I. Ahmad, A. Ouannas, M. Shafiq, V. T. Pham, D. Baleanu, Finite-time stabilization of a perturbed chaotic finance model, J. Adv. Res., 32 (2021), 1–14. https://doi.org/10.1016/j.jare.2021.06.013 doi: 10.1016/j.jare.2021.06.013
![]() |
[14] |
S. Harshavarthini, R. Sakthivel, Y. K. Ma, M. Muslimd, Finite-time resilient fault-tolerant investment policy scheme for chaotic nonlinear finance system, Chaos Solitons Fract., 132 (2020), 109567. https://doi.org/10.1016/j.chaos.2019.109567 doi: 10.1016/j.chaos.2019.109567
![]() |
[15] |
Q. Li, S. Liu, Predefined-time vector-polynomial-based synchronization among a group of chaotic systems and its application in secure information transmission, AIMS Math., 6 (2021), 11005–11028. https://doi.org/10.3934/math.2021639 doi: 10.3934/math.2021639
![]() |
[16] |
Q. Li, S. Liu, Switching event-triggered network-synchronization for chaotic systems with different dimensions, Neurocomputing, 311 (2018), 32–40. https://doi.org/10.1016/j.neucom.2018.05.039 doi: 10.1016/j.neucom.2018.05.039
![]() |
[17] |
J. Wang, B. Tian, Fixed-time distributed event-triggered formation control with state-dependent threshold, Int. J. Robust Nonlinear, 32 (2022), 1209–1228. https://doi.org/10.1002/rnc.5878 doi: 10.1002/rnc.5878
![]() |
[18] |
Q. Li, S. Liu, Y. Chen, Combination event-triggered adaptive networked synchronization communication for nonlinear uncertain fractional-order chaotic systems, Appl. Math. Comput., 333 (2018), 521–535. https://doi.org/10.1016/j.amc.2018.03.094 doi: 10.1016/j.amc.2018.03.094
![]() |
[19] |
S. Liu, L. Zhou, Network synchronization and application of chaotic lur'e systems based on event-triggered mechanism, Nonlinear Dyn., 83 (2016), 2497–2507. https://doi.org/10.1007/s11071-015-2498-y doi: 10.1007/s11071-015-2498-y
![]() |
[20] |
C. M. Kaneba, X. Mu, X. Li, X. Wu, Event triggered control for fault tolerant control system with actuator failure and randomly occurring parameter uncertainty, Appl. Math. Comput., 415 (2022), 126714. https://doi.org/10.1016/j.amc.2021.126714 doi: 10.1016/j.amc.2021.126714
![]() |
[21] |
S. Hu, J. Qiu, X. Chen, F. Zhao, X. Jiang, Dynamic event-triggered control for leader-following consensus of multiagent systems with the estimator, IET Control Theory Appl., 16 (2022), 475–484. https://doi.org/10.1049/cth2.12245 doi: 10.1049/cth2.12245
![]() |
[22] |
J. D. Sánchez-Torres, D. Gómez-Gutiérrez, E. López, A. G. Loukianov, A class of predefined-time stable dynamical systems, Int. J. Robust Nonlinear, 35 (2018), i1–i29. https://doi.org/10.1093/imamci/dnx004 doi: 10.1093/imamci/dnx004
![]() |
[23] |
C. A. Anguiano-Gijón, A. J. Muñoz-Vázquez, J. D. Sánchez-Torres, G. Romero-Galván, F. Martínez-Reyes, On predefined-time synchronisation of chaotic systems, Chaos Solitons Fract., 122 (2019), 172–178. https://doi.org/10.1016/j.chaos.2019.03.015 doi: 10.1016/j.chaos.2019.03.015
![]() |
[24] |
Z. Zuo, Nonsingular fixed-time consensus tracking for second-order multi-agent networks, Automatica, 54 (2015), 305–309. https://doi.org/10.1016/j.automatica.2015.01.021 doi: 10.1016/j.automatica.2015.01.021
![]() |
[25] | S. Fan, Y. Guo, Y. Ji, Fixed-time consensus for leader-following multi-groups of multi-agent systems with unknown non-linear inherent dynamics, 2019 Chinese Control Conference (CCC), Guangzhou, China, 2019, 5903–5908. https://doi.org/10.23919/ChiCC.2019.8865766 |
[26] |
H. Ma, Y. Chen, Study of the bifurcation topological structure and the global complicated character of a kind of nonlinear finance system (ⅰ), Appl. Math. Mech., 22 (2001), 1240–1251. https://doi.org/10.1023/A:1016313804297 doi: 10.1023/A:1016313804297
![]() |
[27] | J. Jian, X. Deng, J. Wang, Globally exponentially attractive set and synchronization of a class of chaotic finance system, In: W. Yu, H. He, N. Zhang, Advances in neural networks-ISNN 2009, Springer, 5551 (2009), 253–261. https://doi.org/10.1007/978-3-642-01507-6_30 |
[28] |
Q. Gao, J. Ma, Chaos and hopf bifurcation of a finance system, Nonlinear Dyn., 58 (2009), 209. https://doi.org/10.1007/s11071-009-9472-5 doi: 10.1007/s11071-009-9472-5
![]() |
[29] |
H. Jahanshahi, S. S. Sajjadi, S. Bekiros, A. A. Aly, On the development of variable-order fractional hyperchaotic economic system with a nonlinear model predictive controller, Chaos Solitons Fract., 144 (2021), 110698. https://doi.org/10.1016/j.chaos.2021.110698 doi: 10.1016/j.chaos.2021.110698
![]() |
[30] |
A. Yousefpour, H. Jahanshahi, J. M. Munoz-Pacheco, S. Bekiros, Z. Wei, A fractional-order hyper-chaotic economic system with transient chaos, Chaos Solitons Fract., 130 (2020), 109400. https://doi.org/10.1016/j.chaos.2019.109400 doi: 10.1016/j.chaos.2019.109400
![]() |
1. | Erfeng Xu, Wenxing Xiao, Yonggang Chen, Local stabilization for a hyperchaotic finance system via time-delayed feedback based on discrete-time observations, 2023, 8, 2473-6988, 20510, 10.3934/math.20231045 | |
2. | Liping Luo, Yonggang Chen, Jishen Jia, Kaixin Zhao, Jinze Jia, Event-triggered anti-windup strategy for time-delay systems subject to saturating actuators, 2024, 9, 2473-6988, 27721, 10.3934/math.20241346 |