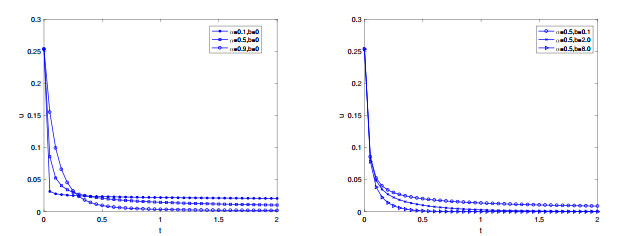
The present work is concerned with the efficient numerical schemes for a time-fractional diffusion equation with tempered memory kernel. The numerical schemes are established by using a L1 difference scheme for generalized Caputo fractional derivative in the temporal variable, and applying the Legendre spectral collocation method for the spatial variable. The sum-of-exponential technique developed in [Jiang et al., Commun. Comput. Phys., 21 (2017), 650-678] is used to discrete generalized fractional derivative with exponential kernel. The stability and convergence of the semi-discrete and fully discrete schemes are strictly proved. Some numerical examples are shown to illustrate the theoretical results and the efficiency of the present methods for two-dimensional problems.
Citation: Zunyuan Hu, Can Li, Shimin Guo. Fast finite difference/Legendre spectral collocation approximations for a tempered time-fractional diffusion equation[J]. AIMS Mathematics, 2024, 9(12): 34647-34673. doi: 10.3934/math.20241650
[1] | Manal Alqhtani, Khaled M. Saad . Numerical solutions of space-fractional diffusion equations via the exponential decay kernel. AIMS Mathematics, 2022, 7(4): 6535-6549. doi: 10.3934/math.2022364 |
[2] | Chuanli Wang, Biyun Chen . An hp-version spectral collocation method for fractional Volterra integro-differential equations with weakly singular kernels. AIMS Mathematics, 2023, 8(8): 19816-19841. doi: 10.3934/math.20231010 |
[3] | Ahmed F. S. Aboubakr, Gamal M. Ismail, Mohamed M. Khader, Mahmoud A. E. Abdelrahman, Ahmed M. T. AbdEl-Bar, Mohamed Adel . Derivation of an approximate formula of the Rabotnov fractional-exponential kernel fractional derivative and applied for numerically solving the blood ethanol concentration system. AIMS Mathematics, 2023, 8(12): 30704-30716. doi: 10.3934/math.20231569 |
[4] | Chuanhua Wu, Ziqiang Wang . The spectral collocation method for solving a fractional integro-differential equation. AIMS Mathematics, 2022, 7(6): 9577-9587. doi: 10.3934/math.2022532 |
[5] | Bin Fan . Efficient numerical method for multi-term time-fractional diffusion equations with Caputo-Fabrizio derivatives. AIMS Mathematics, 2024, 9(3): 7293-7320. doi: 10.3934/math.2024354 |
[6] | Obaid Algahtani, M. A. Abdelkawy, António M. Lopes . A pseudo-spectral scheme for variable order fractional stochastic Volterra integro-differential equations. AIMS Mathematics, 2022, 7(8): 15453-15470. doi: 10.3934/math.2022846 |
[7] | Xiaojun Zhou, Yue Dai . A spectral collocation method for the coupled system of nonlinear fractional differential equations. AIMS Mathematics, 2022, 7(4): 5670-5689. doi: 10.3934/math.2022314 |
[8] | A. H. Tedjani, A. Z. Amin, Abdel-Haleem Abdel-Aty, M. A. Abdelkawy, Mona Mahmoud . Legendre spectral collocation method for solving nonlinear fractional Fredholm integro-differential equations with convergence analysis. AIMS Mathematics, 2024, 9(4): 7973-8000. doi: 10.3934/math.2024388 |
[9] | M. A. Zaky, M. Babatin, M. Hammad, A. Akgül, A. S. Hendy . Efficient spectral collocation method for nonlinear systems of fractional pantograph delay differential equations. AIMS Mathematics, 2024, 9(6): 15246-15262. doi: 10.3934/math.2024740 |
[10] | Khalid K. Ali, Mohamed A. Abd El Salam, Mohamed S. Mohamed . Chebyshev fifth-kind series approximation for generalized space fractional partial differential equations. AIMS Mathematics, 2022, 7(5): 7759-7780. doi: 10.3934/math.2022436 |
The present work is concerned with the efficient numerical schemes for a time-fractional diffusion equation with tempered memory kernel. The numerical schemes are established by using a L1 difference scheme for generalized Caputo fractional derivative in the temporal variable, and applying the Legendre spectral collocation method for the spatial variable. The sum-of-exponential technique developed in [Jiang et al., Commun. Comput. Phys., 21 (2017), 650-678] is used to discrete generalized fractional derivative with exponential kernel. The stability and convergence of the semi-discrete and fully discrete schemes are strictly proved. Some numerical examples are shown to illustrate the theoretical results and the efficiency of the present methods for two-dimensional problems.
Fractional differential equations have attracted extensive attention for their ability to accurately describe complex physical phenomena in both micro and macro perspectives [1,2,3]. The differential equations with fractional derivative in time variable can better describe genetic and memory characteristics of some physical processes. For different physical situations, different memory function arise from various fractional derivative operators, such as the power memory kernel, exponential memory kernel, and Mittag-Leffler memory kernel functions [4].
In this work, we consider the following initial boundary value problem:
{∂α,λ(t)0,tu(x,t)=Δu(x,t)+f(x,t),x∈Ω,t∈(0,T],u(x,t)=0,x∈∂Ω,t∈(0,T],u(x,0)=u0(x),x∈Ω, | (1.1) |
where Δ=∂2∂x21+∂2∂x22 denotes the Laplace operator, Ω=[−1,1]2 denotes the computational domain with boundary ∂Ω, x=(x1,x2)∈Ω stands for the space variables, and ∂α,λ(t)0,t denotes the generalized Caputo time-fractional derivative [5]
∂α,λ(t)0,tu(x,t)=1Γ(1−α)∫t0λ(t−s)(t−s)α∂u∂s(x,s)ds,0<α<1. | (1.2) |
In fractional derivative (1.2), we assume that the kernel function satisfies λ(t)∈C2[0,T], λ(t)>0 and λ′(t)≤0 for all t∈[0,T]. Particularly, if kernel takes λ(t)=e−bt(b>0), fractional derivative (1.2) gives
∂α,e−bt0,tu(x,t)=1Γ(1−α)∫t0e−b(t−s)(t−s)α∂u∂s(x,s)ds, | (1.3) |
provides the tempered fractional derivative and integral
∂α,e−bt0,tu(x,t)=e−btΓ(1−α)∫t0(t−s)−α∂∂s(u(x,s)ebs)ds−bΓ(1−α)∫t0e−b(t−s)(t−s)αu(x,s)ds. | (1.4) |
Obviously, from a mathematical perspective, the Caputo-type fractional derivative (1.2) can be interpreted as a kind of generalized fractional derivative [4,6,7]. From Figure 1, we can see the influence of parameters on the solution. More application (in macroscopic and microscopic scales) of the model given in problem (1.1) with tempered fractional derivative, see references [3,8,9]. There are many research works on the discretization of fractional derivative (1.2). By splitting into two parts, Alikhanov first discussed the L1 formula of fractional derivative (1.2) in the work [5], and proposed a new difference scheme for the time-fractional diffusion equation with the fractional derivatives (1.2). Recently, in view of relation (1.4), Chen et al. [10] established two different L1 discretizations for tempered Caputo fractional derivative on the graded meshes, which developed in reference [11].
The nonlocality of the fractional derivative will make storage and computation very expensive if we adopt a lower-order method. For example, if we use the L1 scheme to calculate (1.1), the required computational and storage amounts are O(NNT) and O(NN2T), respectively, where N is the number of spatial grid points and NT stands for the number of grid points in time. When the time step is small enough, it means that the numerical calculation needs expensive storage costs and computational costs, so it is particularly important to develop fast or high-order numerical schemes. The sum-of-exponential (SOE) technique is one of the effective techniques to approximate the fractional derivatives with the power kernel function [12,13]. In this topic, Jiang et al. [13] used the SOE method to approximate the kernel function t−(α+1)(0<α<1) and solved fractional diffusion equations on unbounded domains. Yan et al. [14] combined the L2-1σ scheme with SOE approximation successfully solved an initial boundary value problem of the time fractional diffusion equation. Combining the SOE approximation, Xu et al. [15] proposed a fast L2 discretization of Caputo fractional derivatives, and they get the error estimates by rearranging the coefficients of the fast L2 difference approximation. With the help of the SOE technique, Gu et al. [16] proposed a fast difference algorithm to solve the generalized time-space fractional diffusion equation with fractional derivative (1.3). It seems that the authors of [16] fail to give any convergence analysis of the fast L1 difference scheme.
As a higher-order numerical method, the spectral method has been widely used for solving many kinds of differential equations [17,18] including fractional differential equations [15]. Lin and Xu [19] solved the time-fractional diffusion equation based on the finite difference method in the time direction and the Legendre spectral method in space. Later, Li and Xu [20] improved their previous results and proposed a time-space spectral method for the time-fractional diffusion equation. Compared with one-dimensional problems, solving high-dimensional problems is more difficult due to the increase in storage and computational cost. Zeng et al. [21] proposed the Legendre Galerkin spectral method for two-dimensional Riesz space fractional nonlinear reaction-diffusion equations and obtained the optimal spatial error estimation. Guo et al. [22] used the Legendre spectral method to solve two-dimensional fractional nonlinear reaction-diffusion wave equations. Recently, combining the fast L2 discretization of Caputo fractional derivatives, Xu et al. [15] proposed a fast difference/spectral method for a time fractional equation in one dimension. In their numerical scheme, the convergence order in the temporal direction is (3−α) and the convergence order in the spatial direction is O(N1−m), where m is the regularity of the exact solution in the spatial direction. This work suggests that the fast difference approximation with high-order discretization schemes is an effective way to solve the time fractional differential equations. Following this line, in this paper, we try to develop a fast finite difference/Legendre spectral collocation method for problem (1.1) with smooth solution and discuss the error estimates for the present numerical schemes. First, the generalized fractional derivative is discretized by L1 discretization, and the time direction convergence order is analyzed. Secondly, by using SOE approximation of exponential kernel function, a fast L1 scheme with exponential memory kernel function is obtained. Therefore, it can be seen that the calculation and storage costs of the fast scheme are significantly reduced. With the similar technique given in [15], we will discuss the error estimates when the fast L1 method is used to solve problem (1.1).
The rest of this paper is organized as follows: In Section 2, we present time discretizations for problem (1.1) and discuss the stability and convergence of the present semi-discrete scheme. Combing the Legendre spectral collocation method for spatial variables, in Section 3, we discuss the finite difference/spectral collocation method for problem (1.1). The error estimates of the fully discrete scheme are also established in this section. For the generalized fractional derivative with exponential kernel, we develop the sum-of-exponentials technique developed in [13] to accelerate the calculation process. The error estimates of the related numerical schemes are discussed in Section 4. In Section 5, we present two numerical examples to verify the efficiency of our numerical algorithm and theoretical results. Finally, some conclusions are provided in Section 6.
First, we discretize the fractional derivative with a generalized memory kernel, assuming the solution u(t)∈C2[0,T]. The computational domain [0,T] is uniformly divided into NT intervals with the uniform grids tk=kτ, k=0,1,…,NT. For λ(t)∈C2[0,T], the time fractional derivative with a generalized memory kernel is discretized as [5]
∂α,λ(t)0,tu(t)|t=tk+1=1Γ(1−α)∫tk+10λ(tk+1−s)(tk+1−s)αu′(s)ds=τ1−αΓ(2−α)k∑l=0(λk−l+1/2ak−l+(λk−l−λk−l+1)bk−l)ut,l+Rk1+Rk2, | (2.1) |
where
λl=λ(tl), ut,l=u(tl+1)−u(tl)τ, Π1,lu(s)=u(tl+1)s−tlτ+u(tl)tl+1−sτ,al=(l+1)1−α−l1−α, bl=12−α[(l+1)2−α−l2−α]−12[(l+1)1−α+l1−α], l≥0, |
and the error
Rk1=τ1−αΓ(1−α)k∑l=0ut,l∫10λk−l+1−z−λk−l+1/2−(λk−l−λk−l+1)(z−1/2)(k−l+1−z)αdz,Rk2=1Γ(1−α)k∑l=0∫tl+1tlλ(tk+1−s)(u(s)−Π1,lu(s))′(tk+1−s)αds, |
give [5]
|Rk1|≤t1−αk+14Γ(2−α)τ2max0≤t≤tk+1|u′(t)|max0≤t≤tk+1|λ′′(t)|, |Rk2|≤˜cmax0≤t≤tk+1|u′′(t)|λ(0)τ2−α, |
where ˜c depends on α.
We rewrite (2.1) as follows:
∂α,λ(t)0,tu(t)|t=tk+1=α−10[k−1∑j=0(cj+1−cj)u(tk−j)+c0u(tk+1)−cku(t0)]+rk+1τ, |
where α0=ταΓ(2−α),cl=(λl+1/2al+(λl−λl+1)bl)(l≥0), and the truncation error gives rk+1τ=Rk1+Rk2. For the sake of simplification, we define the fractional differential operator Lα,λ(t)tu(x,tk+1) by
Lα,λ(t)tu(x,tk+1)=α−10[k−1∑j=0(cj+1−cj)u(x,tk−j)+c0u(x,tk+1)−cku(x,t0)]. | (2.2) |
Using Lα,λ(t)tu(x,tk+1) to approximate ∂α,λ(t)0,tu(x,t) in problem (1.1) at tk+1, and denoting uk+1 is an approximation of u(x,tk+1), fk+1=f(x,tk+1), we obtain the time semi-discrete scheme of problem (1.1), i.e.,
c0u1−α0Δu1=c0u0+α0f1, | (2.3) |
for k=0.
For 1≤k≤NT−1,
k−1∑j=0(cj−cj+1)uk−j+cku0=c0uk+1−α0Δuk+1−α0fk+1. | (2.4) |
After a simple calculation, the truncation error of the semi-discretized schemes (2.6) and (2.7) give rk+1=α0rk+1τ and satisfies
|rk+1|=α0|rk+1τ|≤˜cmax0≤t≤tj+1|∂2tu(x,t)|λ(0)τ2. | (2.5) |
Considering the variational formulation of (1.1), we define some functional spaces endowed with standard norms and inner products that will be used hereafter [17,18,26]
Hm(Ω)={u|u∈L2(Ω),Dβu∈L2(Ω) for 0≤|β|≤m},H10(Ω)={u|u∈H1(Ω),u|∂Ω=0}, |
where β=(β1,β2), |β|=β1+β2,Dβ=∂|β|∂xβ11∂xβ22, L2(Ω) is the space of measurable functions whose square is Lebesgue integrable in Ω, which equip with the inner products
(u,v)=∫Ωuvdx, (u,v)1=(u,v)+α0c−10(∇u,∇v), |
and the normH1 is defined by
||v||1=(v,v)1/21, ‖v‖1=(‖v‖2+α0c−10‖∇v‖2)1/2. |
The weak formulation of the (2.3) and (2.4) reads: Find uk+1∈H10(Ω), such that
(u1,v)+α0c−10(∇u1,∇v)=(u0,v)+α0c−10(f1,v), | (2.6) |
for k=0, and
(uk+1,v)+α0c−10(∇uk+1,∇v)=c−10k−1∑j=0(cj−cj+1)(uk−j,v)+c−10ck(u0,v)+α0c−10(fk+1,v), | (2.7) |
for all v∈H10(Ω) and 1≤k≤NT−1.
Lemma 1. [5] If λ(t)>0, λ′(t)≤0, and λ(t)∈C2[0,T], the weighted coefficients al, bl and cl(l=0,1,…) are given by (2.1), satisfy
a0>a1>a2>…>al>1−α(l+1)α, b0>b1>…bl>0, |
c0>c1>…>cl>α0λ(tl+1/2)Γ(1−α)tαl+1>α0λ(T)Γ(1−α)Tα. |
Theorem 1. For all τ>0, the semi-discretized schemes (2.6) and (2.7) are unconditionally stable, i.e.,
‖uk+1‖1≤‖u0‖+˜cα0c−10max0≤j≤k+1‖fj‖,k=0,1,…,NT−1. | (2.8) |
Proof. For k=0, substituting v=u1 in (2.6) and using ‖u1‖≤‖u1‖1, we have
‖u1‖21≤‖u0‖‖u1‖+α0c−10‖f1‖‖u1‖≤‖u0‖‖u1‖1+α0c−10max0≤j≤1‖fj‖‖u1‖1. | (2.9) |
From (2.9), we have ‖u1‖1≤‖u0‖+˜cα0c−10max0≤j≤1‖fj‖. For k>1, we prove (2.8) by mathematical induction. Assume that there holds
‖uj‖1≤‖u0‖+˜cα0c−10max0≤l≤j‖fl‖,j=1,2,…,k. | (2.10) |
Now prove (2.8) using inequality (2.10). Substituting v=uk+1 into (2.7), we obtain
‖uk+1‖2+α0c−10‖∇uk+1‖2=c−10k−1∑j=0(cj−cj+1)(uk−j,uk+1)+α0c−10(fk+1,uk+1)+ckc−10(u0,uk+1), | (2.11) |
which implies
‖uk+1‖21≤c−10k−1∑j=0(cj−cj+1)‖uk−j‖‖uk+1‖+α0c−10‖fk+1‖‖uk+1‖+ckc−10‖u0‖‖uk+1‖. |
Using Lemma 1 and the inequality ‖uk+1‖≤‖uk+1‖1, we have
‖uk+1‖1≤c−10k−1∑j=0(cj−cj+1)(‖u0‖+˜cα0c−10max0≤j≤k−j‖fj‖)+ckc−10‖u0‖+α0c−10‖fk+1‖=c−10[k−1∑j=0(cj−cj+1)(‖u0‖+˜cα0c−10max0≤j≤k−j‖fj‖)+ck‖u0‖+α0‖fk+1‖]. |
Note that ‖u0‖+˜cα0c−10max0≤j≤k−j‖fj‖≤‖u0‖+˜cα0c−10max0≤j≤k‖fj‖(j=0,1,…,k−1). Therefore
‖uk+1‖1≤c−10[(k−1∑j=0(cj−cj+1)+ck)(‖u0‖+˜cα0c−10max0≤j≤k‖fj‖)]+α0c−10‖fk+1‖≤‖u0‖+˜cα0c−10max0≤j≤k+1‖fj‖. |
Finally, we obtain (2.8).
Here we provide some lemmas to analyze coefficients cl and al, which will help to study the convergence analysis of the solution. In the following sections, ˜c is used to represent a constant, which may be different under different situations. Here, we employ the technique used in reference [19] to do the error estimates of schemes (2.6) and (2.7).
Theorem 2. Let u(x,tk) be the exact solution of problem (1.1), {uk}NTk=0 be the solution of schemes (2.6) and (2.7), there holds
‖u(x,tk)−uk‖1≤{˜cTαmaxt∈(0,T]‖∂2tu(x,t)‖τ2−α,0<α<1,˜cTmaxt∈(0,T]‖∂2tu(x,t)‖τ,α→1, | (2.12) |
where ˜c is independent of u,τ and T.
Proof. Firstly, we prove that the following inequality
‖u(x,tj)−uj‖1≤˜cc−1j−1maxt∈(0,T]|∂2tu(x,t)|λ(0)τ2,j=1,2,…,NT. | (2.13) |
Let ej=u(x,tj)−uj, for j=1, we obtain
(e1,v)+α0c−10(∇e1,∇v)=(e0,v)+(r1,v). | (2.14) |
If we assume the initial value is exact (i.e., e0=0), let v=e1 in (2.14), using (2.5), we obtain
‖e1‖1≤‖r1‖≤˜cc−10maxt∈(0,T]|∂2tu(x,t)|λ(0)τ2. |
Suppose (2.13) holds for all j=1,2,…,k, we need to prove it also holds for j=k+1. In view of
(ek+1,v)+α0c−10(∇ek+1,∇v)=c−10k−1∑j=0(cj−cj+1)(ek−j,v)+c−10ck(e0,v)+(rk+1,v). | (2.15) |
Let v=ek+1 in (2.15), we have
‖ek+1‖2+α0c−10‖∇ek+1‖2=c−10k−1∑j=0(cj−cj+1)(ek−j,ek+1)+c−10ck(e0,ek+1)+(rk+1,ek+1). | (2.16) |
We rewrite (2.16) as follows:
‖ek+1‖21≤c−10[k−1∑j=0(cj−cj+1)˜cc−1k−jmaxt∈(0,T]|∂2tu(x,t)|λ(0)τ2+˜cmaxt∈(0,T]|∂2tu(x,t)|λ(0)τ2]‖ek+1‖. |
Using Lemma 1, we have c−1k−j<c−1k, it follows that
‖ek+1‖1≤c−10[k−1∑j=0(cj−cj+1)˜cc−1kmaxt∈(0,T]|∂2tu(x,t)|λ(0)τ2+˜cmaxt∈(0,T]|∂2tu(x,t)|λ(0)τ2]≤˜cc−1kmaxt∈(0,T]|∂2tu(x,t)|λ(0)τ2, |
which implies (2.13). Lemma 1 suggests that
k−αc−1k−1λ(tk+1/2)≤k−αΓ(1−α)tαkα0λ(tk−1/2)λ(tk+1/2)≤Γ(1−α)ταα0λ(tk+1/2)λ(tk−1/2)≤Γ(1−α)ταΓ(2−α)τα=11−α. |
Hence, we have
‖u(x,tk)−uk‖1≤˜cc−1k−1maxt∈(0,T]|∂2tu(x,t)|λ(0)τ2=˜ck−αλ(tk+1/2)c−1k−11λ(tk+1/2)kαmaxt∈(0,T]|∂2tu(x,t)|λ(0)τ2≤˜c11−α1λ(tk+1/2)(kτ)αmaxt∈(0,T]|∂2tu(x,t)|λ(0)τ2−α≤˜cTαmaxt∈(0,T]|∂2tu(x,t)|τ2−α. |
Next we consider the case α→1, we firstly prove that
‖u(tj)−uj‖1≤˜cjmaxt∈(0,T]|∂2tu(x,t)|τ2,j=1,2,…,NT. | (2.17) |
Inequality (2.17) obviously holds for j=1. Suppose (2.17) holds for j=1,2,…,k. Now we will prove that (2.17) still holds when j=k+1. In fact, with the similar method given in Theorem 1, we have
‖u(tk+1)−uk+1‖1≤c−10(c0−c1)‖ek‖+c−10k−1∑j=1(cj−cj+1)‖ek−j‖+c−10ck‖e0‖+‖rk+1‖≤c−10[(c0−c1)˜ckmaxt∈(0,T]|∂2tu(x,t)|τ2+k−1∑j=1(cj−cj+1)(k−j)˜cmaxt∈(0,T]|∂2tu(x,t)|τ2]+˜cmaxt∈(0,T]|∂2tu(x,t)|τ2=c−10[(c0−c1)kk+1+k−1∑j=1(cj−cj+1)k−jk+1+c0k+1](k+1)˜cmaxt∈(0,T]|∂2tu(x,t)|τ2. | (2.18) |
Simple calculations, we can check that
(c0−c1)kk+1+k−1∑j=1(cj−cj+1)k−jk+1+c0k+1=(c0−c1)+k−1∑j=1(cj−cj+1)−(c0−c1)1k+1−k−1∑j=1(cj−cj+1)j+1k+1+c0k+1. | (2.19) |
With the help of (2.18), Eq (2.19) provides that
‖ek+1‖1≤c−10[(c0−c1)+k−1∑j=1(cj−cj+1)−(c0−c1)1k+1−k−1∑j=1(cj−cj+1)j+1k+1+c0k+1]˜c(k+1)maxt∈(0,T]|∂2tu(x,t)|τ2. | (2.20) |
Since
(c0−c1)1k+1+k−1∑j=1(cj−cj+1)j+1k+1+ck≥1k+1[(c0−c1)+k−1∑j=1(cj−cj+1)+ck]=c0k+1, |
we have
−(c0−c1)1k+1−k−1∑j=1(cj−cj+1)j+1k+1+c0k+1≤ck. | (2.21) |
Substituting (2.21) into (2.20), we obtain
‖ek+1‖1≤c−10[(c0−c1)+k−1∑j=1(cj−cj+1)+ck](k+1)˜cmaxt∈(0,T]|∂2tu(x,t)|τ2≤˜cTmaxt∈(0,T]|∂2tu(x,t)|τ. |
We finally obtain our conclusion.
Let PN(Ix) and PN(Iy) be the spaces of all polynomials of degree less than or equal to N defined on domains Ix=(−1,1) and Iy=(−1,1), respectively. Let VN(Ω)=PN(Ix)⨂PN(Iy), {ξj}Nj=0, {ξi}Ni=0 are Legendre-Gauss-Labatoo (LGL) points, and {ωj}Nj=0 and {ωi}Ni=0 are the weights, which satisfy the following quadrature formula [25,26]
∫1−1∫1−1φ(x1,x2)dx1x2=N∑j1=0N∑j2=0φ(ξj1,ξj2)ωj1ωj2,∀φ∈V2N−1(Ω). |
Define the inner product and norm as follows [18,25,26]
(ϕ,ψ)N=N∑i=0N∑j=0ϕ(ξi,ξj)ψ(ξi,ξj)ωiωj,‖ψ‖0,N=(ϕ,ϕ)1/2N. |
We will use the H10-orthogonal projection operator πN such that [24]
(∇(u−πNu),∇v)=0,∀v∈V0N(Ω), | (3.1) |
for all u∈H10(Ω). Here V0N(Ω) is defined as
V0N(Ω)=(PN(Ix)⊗PN(Iy))∩H10(Ω). |
For this projection, there have estimates [25,26]
‖u−πNu‖l≤˜cNl−m‖u‖m,u∈Hm(Ω)∩H10(Ω),m≥1,l=0,1. | (3.2) |
Lemma 2. [25] For all φ∈Hm(Ω)( m≥1), vN∈PN(Ω), there holds
(φ,vN)−(φ,vN)N≤˜cN−m‖φ‖m‖vN‖0,N. | (3.3) |
Finite difference/Legendre collocation approximation of problem (1.1) gives: Find ukN∈V0N(Ω),
(uk+1N,vN)N+α0c−10(∇uk+1N,∇vN)N=c−10k−1∑j=0(cj−cj+1)(uk−jN,vN)N+ckc−10(u0N,vN)N+(fk+1,vN)N, | (3.4) |
for all vN∈V0N(Ω). The discrete H1-norm is defined by
‖ψN‖1,N=((ψN,ψN)N+α0c−10(∇ψN,∇ψN)N)1/2,∀ψN∈VN(Ω). |
To do the error estimate, we split the error as u(tk)−ukN=(u(tk)−πNu(tk))−(ukN−πNu(tk)):=ϵkN−ekN. Without loss of generality, we only consider the homogeneous case of problem (1.1), i.e., f=0.
Theorem 3. Let u(x,t) be the exact solution of problem (1.1), and ukN be the solution of fully discrete scheme (3.4) with the initial condition u0N=πNu0. Suppose ∂2tu∈L∞((0,T];Hm(Ω))(m≥1), then
||u(x,tk)−ukN||1,N≤{˜cTα1−α1λ(T)(N−m||∂α,λ(t)tu||L∞(Hm)+τ2−αλ(0)(N−m||∂2tu||L∞(Hm)+‖∂2tu‖L∞(L2)))+˜cN1−m||u||L∞(Hm),0<α<1,˜cTλ(T)(N−m‖∂α,λ(t)tu‖L∞(Hm)+τλ(0)(N−m‖∂2tu‖L∞(Hm)+‖∂2tu‖L∞(L2)))+˜cN1−m‖u‖L∞(Hm),α→1, | (3.5) |
where ‖∂α,λ(t)tu‖L∞(Hm)=supt∈(0,T)‖∂α,λ(t)tu(x,t)‖m, ‖u‖L∞(Hm)=supt∈(0,T)‖u(x,t)‖m, ‖∂2tu‖L∞(Hm)=supt∈(0,T)‖∂2tu(x,t)‖m, and ‖∂2tu‖L∞(L2)=supt∈(0,T)‖∂2tu(x,t)‖.
Proof. Subtracting ekN=ukN−πNu(tk) on both sides of Eq (3.4), we obtain
(ek+1N,vN)N+α0c−10(∇ek+1N,∇vN)N=c−10k−1∑j=0(cj−cj+1)(uk−jN,vN)N+ckc−10(u0N,vN)N−(πNu(tk+1),vN)N−α0c−10(∇πNu(tk+1),∇vN)N. |
Rewriting the above formula, we obtain
(ek+1N,vN)N+α0c−10(∇ek+1N,∇vN)N=c−10k−1∑j=0(cj−cj+1)(ek−jN,vN)N+ckc−10(e0N,vN)N+(εk+11,vN)N+(εk+12,vN)N, |
where
εk+11=(u(tk+1)−πNu(tk+1))−c−10k−1∑j=0(cj−cj+1)(u(tk−j)−πNu(tk−j))−ckc−10(u(t0)−πNu(t0)), |
and
εk+12=−(u(tk+1)−πNu(tk+1))+c−10k−1∑j=0(cj−cj+1)u(tk−j)+ckc−10u(t0)−πNu(tk+1)+α0c−10ΔπNu(tk+1). |
For term (εk+11,vN)N, we have
(εk+11,vN)N=(Id−πN)(u(tk+1)−c−10k−1∑j=0(cj−cj+1)u(tk−j)−ckc−10u(t0),vN)N=α0c−10((Id−πN)(∂α,λ(t)tu(tk+1)−rk+1τ),vN)N, |
where Id is the identity operator. Using (3.3), we have
|(εk+11,vN)N|≤α0c−10[((Id−πN)(∂α,λ(t)tu(tk+1)−rk+1τ),vN)+˜cN−1‖(Id−πN)(∂αtu(tk+1)−rk+1τ)‖1‖vN‖0,N]≤α0c−10[‖(Id−πN)(∂α,λ(t)tu(tk+1)−rk+1τ)‖0,N+˜cN−1‖(Id−πN)(∂α,λ(t)tu(tk+1)−rk+1τ)‖1]‖vN‖0,N. |
By using of (3.2), we obtain
|(εk1,vN)N|≤˜cα0c−10(N−m‖∂α,λ(t)tu‖L∞(Hm)+τ2−αλ(0)N−m‖∂2tu‖L∞(Hm))‖vN‖0,N. |
Note that
(εk+12,vN)N=−(u(tk+1)−c−10k−1∑j=0(cj−cj+1)u(tk−j)−ckc−10u(t0),vN)N−α0c−10(∇πNu(tk+1),∇vN)N, |
the facts (∇πNu(tk),∇vN)N=(∇πNu(tk),∇vN) and (∂α,λ(t)tu(tk),vN)=−(∇u(tk),∇vN), we obtain
|(εk+12,vN)N|≤α0c−10(Lα,λ(t)tu(tk+1),vN)−α0c−10(Lα,λ(t)tu(tk+1),vN)N+α0c−10(∂α,λ(t)tu(tk+1)−Lα,λ(t)tu(tk+1),vN). |
Furthermore, we obtain
|(εk+12,vN)N|≤˜cα0c−10[N−m‖∂α,λ(t)tu‖L∞(Hm)+λ(0)τ2−α(‖∂2tu‖L∞(L2)+N−m||∂2tu||L∞(Hm))]‖vN‖0,N. |
Combining the estimates of (εk1,vN)N and (εk2,vN)N, we have
‖ek+1N‖1,N≤c−10k−1∑j=0(cj−cj+1)‖ek−jN‖0,N+ckc−10‖e0N‖0,N+˜cα0c−10[N−m‖∂α,λ(t)tu‖L∞(Hm)+λ(0)τ2−α(‖∂2tu‖L∞(L2)+N−m||∂2tu||L∞(Hm))]. | (3.6) |
Moreover, with the same technique used in Theorem 2, using inequality (3.6), we can prove that
‖ekN‖1,N≤c−1k−1˜cα0c−10[N−m‖∂α,λ(t)tu‖L∞(Hm)+λ(0)τ2−α(‖∂2tu‖L∞(L2)+N−m||∂2tu||L∞(Hm))]≤˜cc−1k−1k−αλ(tk+1/2)Γ(2−α)λ(tk+1/2)kατα(N−m||∂α,λ(t)tu||L∞(Hm)+τ2−αλ(0)‖∂2tu‖L∞(L2)+τ2−αλ(0)N−m‖∂2tu‖L∞(Hm))≤˜cTα(1−α)(λ(T))[N−m‖∂α,λ(t)tu‖L∞(Hm)+λ(0)τ2−α(‖∂2tu‖L∞(L2)+N−m||∂2tu||L∞(Hm))]. |
Hence, applying triangle inequality, we obtain
‖u(tk)−ukN‖1,N≤‖u(tk)−πNu(tk)‖1,N+‖ukN−πNu(tk)‖1,N≤˜cN1−m‖u‖L∞(Hm)+˜cTα(1−α)λ(T)×[N−m‖∂α,λ(t)tu‖L∞(Hm)+λ(0)τ2−α(‖∂2tu‖L∞(L2)+N−m||∂2tu||L∞(Hm))]. |
For α→1, similar to the case discussed in Theorem 2, we obtain
‖ekN‖1,N≤˜cTλ(T)(N−m||∂α,λ(t)tu||L∞(Hm)+τλ(0)‖∂2tu‖L∞(L2)+τλ(0)N−m‖∂2tu‖L∞(Hm)). |
Applying triangle inequality once again, we have
‖u(tk)−ukN‖1,N≤‖u(tk)−πNu(tk)‖1,N+‖ukN−πNu(tk)‖1,N,≤˜cN1−m‖u‖L∞(Hm)+˜cTλ(T)(N−m||∂α,λ(t)tu||L∞(Hm)+τλ(0)‖∂2tu‖L∞(L2)+τλ(0)N−m‖∂2tu‖L∞(Hm)), |
which implies the desired result.
Applying the same technique given in reference [13], we use the sum-of-exponentials (SOE) approximation to approximate the power function t−α(0<α<1) in fractional derivative (2.1). And for the approach error, we have the following results:
Lemma 3. [13] For a given absolute error ε, the kernel functions 1tα(α∈(0,1)), there exist positive real numbers {ηαi}Nexpi=1 and {wαi}Nexpi=1 such that
|t−α−Nexp∑i=1wαie−ηαit|≤ε,t∈[τ,T], | (4.1) |
where Nexp=O(log1ε(loglog1ε+logTτ)+log1τ(loglog1ε+log1τ)).
For the details of the proof of Lemma 3, see [13]. Following the same idea given in references [13,16], we split the Caputo fractional derivative (1.3) into a local term L(tk) and a history term H(tk) in discretized fractional derivative (2.1) with kernel λ(t)=e−bt(b≥0), i.e.,
∂α,λ(t)0,tu(t)|t=tk=1Γ(1−α)∫tk−10eb(tk−s)(tk−s)αu′(s)ds+1Γ(1−α)∫tktk−1eb(tk−s)(tk−s)αu′(s)ds:=H(tk)+L(tk). |
For the local term, we discrete it as the follows
L(tk):≈u(tk)−u(tk−1)τΓ(1−α)∫tktk−1e−b(tk−s)(tk−s)αds,=u(tk)−u(tk−1)τΓ(2−α)(e−bττ1−α+b∫τ0e−bθθ1−αdθ). | (4.2) |
The history term, using SOE approximation, we obtain
H(tk):=1Γ(1−α)∫tk−10e−b(tk−s)(tk−s)αu′(s)ds≈1Γ(1−α)∫tk−10Nexp∑i=1wαie−ηαi(tk−s)e−b(tk−s)u′(s)ds=1Γ(1−α)Nexp∑i=1∫tk−10wαie−˜ηαi(tk−s)u′(s)ds, |
where ˜ηαi=ηαi+b. For simplicity, we rewrite H(tk) as
H(tk)≈1Γ(1−α)Nexp∑i=1wαiUαh,i(tk), |
with
Uαh,i(tk)=e−˜ηαiτUαh,i(tk−1)+∫tk−1tk−2e−˜ηαi(tk−s)u′(s)ds. |
Using linear interpolation operators to approximate u′(s), we have
∫tk−1tk−2e−˜ηαi(tk−s)u′(s)ds=∫tk−1tk−2e−˜ηαi(tk−s)(Π1,k−1u(s))′ds=ˆaiu(tk−1)+ˆbiu(tk−2)+O(τ2−α), |
where ˆai=1−e−(ηαi+b)ττ(ηαi+b)e(ηαi+b)τ, ˆbi=−ˆai. Hence, history term has recurrence relation
Uαh,i(tk)=e−˜ηαiτUαh,i(tk−1)+ˆaiu(tk−1)+ˆbiu(tk−2). |
Collecting the above formulas, we obtain the fast finite difference operator of fractional derivative (1.3)
Fα,λ(t)tu1=Lα,λ(t)t(t1),k=1,Fα,λ(t)tuk=u(tk)−u(tk−1)τΓ(2−α)(e−bττ1−α+b∫τ0e−bθθ1−αdθ)+1Γ(1−α)Nexp∑i=1wαiUαh,i(tk),2≤k≤NT, | (4.3) |
where Uαh,i(t1)=0.
If we use the difference operator (4.3) to approximate fractional derivatives (1.3) at time level tk, we get the semi-discrete scheme
Fα,λ(t)tuk−Δuk=f(tk),1≤k≤NT. |
The weak form in L2-inner product gives: Find uk∈H10(Ω)(1≤k≤NT), such that
(Fα,λ(t)tuk,v)+(∇uk,∇v)=(fk,v),∀v∈H10(Ω). | (4.4) |
For the sake of simplification, (2.2) can be rewritten as
Lα,λ(t)tu(tk)=τ−αΓ(2−α)c−10[u(tk)−k∑j=1ρk,αk−ju(tk−j)], |
where ρk,αk−j=cj−1−cjc0 as 1≤j≤k−1, ρk,αk−j=ck−1c0 as j=k. By comparing the two operators Fα,λ(t)t and Lα,λ(t)t, we have
Fα,λ(t)tu(tk)−Lα,λ(t)tu(tk)=1Γ(1−α)k∑j=1∫tjtj−1[(tk−s)−α−Nexp∑i=1wαie−ηαi(tk−s)]e−b(tk−s)(Π1,ju(s))′ds=1α0(1−α)−1k∑j=1[˜ak−ju(tj−1)+˜bk−ju(tj)], |
where Π1,ju(s)=u(tj−1)tj−sτ+u(tj)s−tj−1τ, s∈[tj−1,tj],
˜ak−j=−τα−1∫tjtj−1[(tk−s)−α−Nexp∑i=1wαie−ηαi(tk−s)]e−b(tk−s)ds, |
and
˜bk−j=τα−1∫tjtj−1[(tk−s)−α−Nexp∑i=1wαie−ηαi(tk−s)]e−b(tk−s)ds. |
Simple calculations, we can check that
|˜ak−j|=|˜bk−j|≤ταε. |
For the truncation error of the fast difference operator Fα,λ(t)t gives
Rk,ατ:=∂α,λ(t)tu(tk)−Fα,λ(t)tu(tk),2≤k≤NT. |
Lemma 4. Suppose that u(t)∈C2[0,T], for any 0<α<1, there holds
|Rk,ατ|≤˜cτ2−αmax0≤t≤tk|u′′(t)|+˜cεmax0≤t≤tk|u(t)|,2≤k≤NT. |
Proof. By the triangle inequality
|Rk,ατ|=|∂α,λ(t)tu(tk)−Fαtu(tk)|≤|∂α,λ(t)tu(tk)−Lαtu(tk)|+|Lαtu(tk)−Fαtu(tk)|≤˜cτ2−αmax0≤t≤tk|u′′(t)|+1α0(1−α)−1k∑j=1[˜ak−ju(tj−1)+˜bk−ju(tj)]≤˜cτ2−αmax0≤t≤tk|u′′(t)|+˜cεmax0≤t≤tk|u(t)|. |
Furthermore, we can rewrite the fast difference operator as
Fα,λ(t)tuk=1α0c−10[uk−k∑j=1ρk,αk−juk−j]+1α0(1−α)−1k∑j=1[˜ak−juj−1+˜bk−juj]=1α0c−10[uk−k∑j=1ρk,αk−juk−j]+1α0(1−α)−1k∑j=0ζk,αk−juk−j, |
where
ζ1,α0=˜a0,ζ1,α1=˜b0;ζ2,α0=˜a1,ζ2,α1=˜a0+˜b1,ζ2,α2=˜b0;ζ3,α0=˜a2,ζ3,α1=˜a1+˜b2,ζ3,α2=˜a0+˜b1,ζ3,α3=˜b0;ζk,α0=˜ak−1,ζ3,αk−j=˜aj−1+˜bj,ζk,αk−1=˜b0,k≥4,j=2,3,…,k−1. |
The coefficients ρk,αk−j have the following properties.
Lemma 5. For k≥1, the coefficients ρk,αk−j and c−10 satisfy
(1) ρk,αk−j>0,j=1,2…,k−1; (2) k∑j=1ρk,αk−j=1,j=1,2…,k−1;
(3) ρk,α0>2(1−α)λk−1/23kα; (4) 0<ρk,αk−1<1; (5) 1<c−10<ebτ2.
Proof. See the Appendix A.
To do the error estimates of the fast difference scheme, we follow the technique used in reference [24]. Introducing a parameter σ, then uk−∑kj=1ρk,αk−juk−j can be rewritten as following
uk−k∑j=1ρk,αk−juk−j=(uk−σuk−1)−(ρk,αk−1−σ)(uk−1−σuk−2)−⋯−(ρk,α2+σρk,α3+⋯+σk−3ρk,αk−1−σk−2)(u2−σu1)−(ρk,α1+σρk,α2+⋯+σk−2ρk,αk−1−σk−1)(u1−σu0)−(ρk,α0+σρk,α1+⋯+σk−1ρk,αk−1−σk)u0=ˉuk−k∑j=1ˉρk,αk−jˉuk−j, |
where σ=12ρk,αk−1, σj means power of σ, and
ˉu0=u0,ˉuj=uj−σuj−1,ˉρk,αk−j=j∑l=1σj−lρk,αk−l−σj,j=1,2,…,k. |
Similarly, the sum-of-exponentials part can be rewritten as
1α0(1−α)−1k∑j=0ζk,αk−juk−j=1α0(1−α)−1k∑j=0ˉζk,αk−jˉuk−j, |
where ˉζk,αk−j=∑jl=0σj−lζk,αk−l, j=0,1,…,k. The above statements show that the fast difference operator can be rewritten as
ˉFα,λ(t)tˉuk=Fα,λ(t)tuk=1α0c−10[ˉuk−k∑j=1ˉρk,αk−jˉuk−j]+1α0(1−α)−1k∑j=1ˉζk,αk−jˉuk−j. |
Thus, the semi-discrete scheme (4.4) can be rewritten as
(ˉFα,λ(t)tˉuk,v)+(∇uk,∇v)=(fk,v),∀v∈H10(Ω). | (4.5) |
For the weighted coefficients ˉρk,αk−j and ˉζk,αk−j, there holds
Lemma 6. For k≥2, the coefficients ˉρk,αk−j and ˉζk,αk−j satisfy
(1) ˉρk,αk−j>0, j=1,2…,k; (2) 1ˉρk,α0<1ρk,α0<3kα2(1−α)λk−1/2;
(3) k∑j=1ˉρk,αk−j≤1−2σ(1−α)λk−1/23(1−σ)kα; (4) |ˉζk,αk−j|≤4ετα, j=1,2…,k; (5) 0<σ<12.
Proof. See the Appendix B.
Theorem 4. Assume that the permissible error ε satisfies ε≤min{14√Γ(1−α)σλ(T)3ˆc(1−σ)TαNTebτ/2,Γ(1−α)4ˆc}, then the semi-discrete scheme (4.4) is stable, and its solution satisfies
‖uk‖1≤(c−10+˜c(α0c0)−1/2)(‖u0‖+max0≤j≤k‖fj‖),1≤k≤NT. | (4.6) |
Proof. For k=1, taking v=u1 in (4.4), there holds
(u1,u1)+α0(∇u1,∇u1)=(u0,u1)+(f1,u1). |
Using Schwarz inequality, we arrive at ‖u1‖21≤‖u1‖‖u0‖+‖f1‖‖u1‖, which produces ‖u1‖1≤‖u0‖+‖f1‖. Using (5) of Lemma 5, we have
‖u1‖1≤c−10(‖u0‖+‖f1‖)≤c−10(1+˜c(α0c0)−1/2)(‖u0‖+max0≤j≤1‖fj‖). |
For k≥2, using mathematical induction on the index k, we will prove the following result:
α0c−10‖∇uk‖2≤c−20(‖u0‖2+max0≤j≤k‖fj‖),2≤k≤NT. | (4.7) |
Firstly, substituting v=2ˉuk into semi-discrete scheme (4.5), it yields
2(ˉuk,ˉuk)+2α0c−10(∇uk,∇ˉuk)+2c−10(1−α)k∑j=0ˉζk,αk−j(ˉuk−j,ˉuk)=2k∑j=1ˉρk,αk−j(ˉuk−j,ˉuk)+2(fk,ˉuk). |
Using the identity 2(∇uk,∇ˉuk)=‖∇uk‖2+‖∇ˉuk‖2−σ2‖∇uk−1‖2 and (3) of Lemma 6, we have
‖ˉuk‖2+α0c−10‖∇uk‖2+α0c−10‖∇ˉuk‖2≤σ‖ˉuk−1‖2+α0c−10σ‖∇uk−1‖2+k∑j=2ˉρk,αk−j‖uk−j‖2−2c−10(1−α)k∑j=0ˉζk,αk−j(ˉuk−j,ˉuk)+‖fk‖2+‖ˉuk‖2. | (4.8) |
Furthermore, using Poincaré inequality [26]
‖ˉuk‖≤ˆc‖∇ˉuk‖, | (4.9) |
and (5) of Lemma 5, if ε≤Γ(1−α)4ˆc, we obtain
|−2c−10(1−α)ˉζk,αk(ˉuk,ˉuk)|≤2ταεc−10(1−α)‖ˉuk‖2≤α0c−102‖∇ˉuk‖2, |
where we used the relation ˉζk,αk=ζk,αk=˜b0≤ταε in above derivation. Setting θk=1−k∑j=1ˉρk,αk−j and using Young inequality, we obtain
|−2c−10(1−α)k∑j=1ˉζk,αk−j(ˉuk−j,ˉuk)|≤θkkk∑j=1‖ˉuk−j‖2+kc−20(1−α)2θkk∑j=1(ˉζk,αk−j)2‖ˉuk‖2. |
According to (3) and (4) of Lemma 6, we obtain θk=1−k∑j=1ˉρk,αk−j≥2σ(1−α)λk−1/23(1−σ)kα. Moreover, with the help of Poincaré inequality, we obtain
|−2c−10(1−α)k∑j=1ˉζk,αk−j(ˉuk−j,ˉuk)|≤θkkk∑j=1‖ˉuk−j‖2+3ˆcc−20(1−α)2(1−σ)kα+12σ(1−α)λ(T)(4ταε)2‖∇ˉuk‖2. |
If ε≤14√Γ(1−α)σλ(T)3ˆc(1−σ)TαNTebτ/2, there holds
|−2c−10(1−α)k∑j=1ˉζk,αk−j(ˉuk−j,ˉuk)|≤θkkk∑j=1‖ˉuk−j‖2+3ˆcc−20(1−α)2(1−σ)kα+12σ(1−α)λ(T)(4ταε)2‖∇ˉuk‖2≤θkkk∑j=1‖ˉuk−j‖2+α0c−102‖∇ˉuk‖2. |
Rewriting (4.8) as follows:
α0c−10‖∇uk‖2≤(σ+θkk)‖uk−1‖2+α0c−10σ‖∇uk−1‖2+k∑j=2(ˉρk,αk−j+θkk)‖ˉuk−j‖2+‖fk‖2. |
In view of relation σ+θ2+ˉρ2,α0=1, we obtain (4.7) for k=2. Assuming that (4.7) is already correct for all k=2,3,…,n−1, we can check that
α0c−10‖∇un‖2≤c−20(σ+θnn+k∑j=2ˉρk,αk−j+θnn(n−1))‖u0‖2+‖fn‖2=c−20(‖u0‖2+‖fn‖2)≤c−20(‖u0‖+max0≤j≤n‖fj‖)2,∀n=2,…,NT, |
which implies
√α0c−10‖∇un‖≤c−10(‖u0‖+max0≤j≤n‖fj‖). | (4.10) |
Furthermore, using (4.10) and Poincaré inequality (4.9), we obtain
‖un‖2≤ˆc‖∇un‖2≤˜c(α0c0)−1/2(‖u0‖+max0≤j≤n‖fj‖). | (4.11) |
Combining (4.10) and (4.11), we obtain the desired results.
For the convergence of time semi-discrete scheme (4.4), we have
Theorem 5. Let u(x,t) be the exact solution of problem (1.1), and {uk}NTk=1 be the semi-discrete solution of scheme (4.4) with the initial u0=u(0). Under the assumption given in Theorem 4 and ∂2tu(x,t)∈L∞((0,T];L2(Ω)), there holds
‖u(tk)−uk‖1≤˜cα,T(τ2−α‖∂2tu‖L∞(L2)+ε‖u‖L∞(L2)),2≤k≤NT, | (4.12) |
where ˜cα,T depends only on α and T.
Proof. Let ek=u(tk)−uk. Subtracting (4.4) from problem (1.1) at time level tk, it follows that
(Fα,λ(t)tek,v)+(∇ek,∇v)=(Rk,ατ,v),∀v∈H10(Ω). |
With the similar technique in Theorem 4, we obtain
‖ek‖2+α0c−10‖∇ek‖2≤˜cα,Tmax2≤i≤NT‖Rk,ατ‖2,k≥2. |
Applying Lemma 4, we obtain (4.12).
We consider the spectral collocation method in space as follows: Find ukN∈V0N(Ω), such that
(Fα,λ(t)tukN,vN)N+(∇ukN,∇vN)N=0,∀vN∈V0N(Ω). | (4.13) |
Theorem 6. Let u(x,t) be the exact solution of (1.1), and ukN be the solution of scheme (4.13) with the initial condition u0N=πNu0. Suppose ∂2tu(x,t)∈L∞((0,T];Hm(Ω))(m≥1), for k=2,…,NT, there holds
‖u(tk)−ukN‖1≤˜cα,T(τ2−α‖∂2tu‖L∞(L2)+N−mτ2−α‖∂2tu‖L∞(Hm)+N−m‖∂α,λ(t)tu‖L∞(Hm)+N1−m‖u‖L∞(Hm)+ε‖u‖L∞(L2)+εN−m‖u‖L∞(Hm)). | (4.14) |
Proof. Let ekN=ukN−πNu(tk), using (4.13), we have
(Fα,λ(t)tekN,vN)N+(∇ekN,∇vN)N=(Fα,λ(t)tukN,vN)N+(∇ukN,∇vN)N−(Fα,λ(t)tπNu(tk),vN)N−(∇πNu(tk),∇vN)N=(Fα,λ(t)tukN−Fα,λ(t)tπNu(tk),vN)N−(Fα,λ(t)tu(tk),vN)N−(∇πNu(tk),∇vN)N:=εk1(vN)+εk2(vN),∀vN∈V0N(Ω), | (4.15) |
where
εk1(vN)=(Fα,λ(t)tukN−Fα,λ(t)tπNu(tk),vN)N,εk2(vN)=−(Fα,λ(t)tu(tk),vN)N−(∇πNu(tk),∇vN)N. | (4.16) |
Using equality ˉFα,λ(t)tˉuk=Fα,λ(t)tuk, scheme (4.15) can be rewritten as
(ˉFα,λ(t)tˉekN,vN)N+(∇ekN,∇vN)N=εk1(vN)+εk2(vN),∀vN∈V0N(Ω), |
where ˉekN=ekN−σek−1N. Moreover, using Lemma 2, we have
εk1(vN)=(Fα,λ(t)tukN−Fα,λ(t)tπNu(tk),vN)N=((Id−πN)(∂α,λ(t)tu(tk)−Rk,ατ),vN)N≤|((Id−πN)(∂α,λ(t)tu(tk)−Rk,ατ),vN)|+˜cN−1‖(Id−πN)(∂α,λ(t)tu(tk)−Rk,ατ)‖1‖vN‖0,N≤[‖(Id−πN)(∂α,λ(t)tu(tk)−Rk,ατ)‖0,N+˜cN−1‖(Id−πN)(∂α,λ(t)tu(tk)−Rk,ατ)‖1]‖vN‖0,N. | (4.17) |
According to Lemma 4, for l=0,1, we have
‖(Id−πN)Rk,ατ‖l≤˜cα,Tmax0≤t≤tk‖(Id−πN)∂2tu(t)‖lτ2−α+˜cα,Tεmax0≤t≤tk‖(Id−πN)∂2tu(t)‖l. |
Combing (3.2), Eq (4.17) produces
|εk1(vN)|≤˜c(N−m‖∂α,λ(t)tu‖L∞(Hm)+N−mτ2−α‖∂2tu‖L∞(Hm)+εN−m‖u‖L∞(Hm))‖vN‖0,N. |
According to the definition of the ‖⋅‖0,N and projection operator πN, we have
(∇πNu(tk),∇vN)N=(∇πNu(tk),∇vN)=(∇u(tk),∇vN),∀vN∈V0N(Ω). | (4.18) |
Applying (1.1), (4.16), and (4.18), we obtain
εk2(vN)=−(Fα,λ(t)tu(tk),vN)N−(∇πNu(tk),∇vN)N=(Fα,λ(t)tu(tk),vN)−(Fα,λ(t)tu(tk),vN)N+(Rk,ατ,vN). |
Furthermore, using Lemmas 2 and 4, we obtain
|εk2(vN)|≤(˜cN−m‖∂α,λ(t)tu(tk)−Rk,ατ‖m+‖Rk,ατ‖0,N)‖vN‖0,N≤˜cα,T(N−m‖∂α,λ(t)tu‖L∞(Hm)+N−mτ2−α‖∂2tu‖L∞(Hm)+εN−m‖u‖L∞(Hm)+τ2−α‖∂2tu‖L∞(L2)+ε‖u‖L∞(L2))‖vN‖0,N. |
Combining the estimates of |εk1(vN)|, |εk2(vN)| and the Poincaré inequality, we have
|εk1(vN)|+|εk2(vN)|≤˜cα,T(N−m‖∂α,λ(t)tu‖L∞(Hm)+N−mτ2−α‖∂2tu‖L∞(Hm)+εN−m‖u‖L∞(Hm)+τ2−α‖∂2tu‖L∞(L2)+ε‖u‖L∞(L2))‖∇vN‖0,N. |
With the similar argument given in Theorem 4, we obtain
‖ekN‖+√α0c−10‖∇ekN‖≤˜cα,T(N−m‖∂α,λ(t)tu‖L∞(Hm)+N−mτ2−α‖∂2tu‖L∞(Hm)+εN−m‖u‖L∞(Hm)+τ2−α‖∂2tu‖L∞(L2)+ε‖u‖L∞(L2)). |
By the triangle inequality ‖u(tk)−ukN‖1,N≤‖u(tk)−πNu(tk)‖1,N+‖ukN−πNu(tk)‖1,N, we finally obtain our conclusion.
In this section, we will test our theory results given in previous sections. The error in time direction at terminal time T=1 is measured by the point-wise maximum norm. In the following numerical experiments, we used two examples with the exponential kernel function λ(t)=e−t, which modify the numerical examples given in reference [5]. In our numerical experiments, all the algorithms are implemented by MATLAB R2022b, which were run on a 2.90 GHz PC with 32 GB of RAM and the Windows 11 operating system.
Example 1. First, we consider problem (1.1) with exact solution u(x,y,t)=(1+(6−(6+6t+3t2+t3)e−t)sin(πx)sin(πy). The source term and initial values are given by the exact solution.
In Table 1, we list the errors and convergence orders of the present numerical scheme (3.4). Here, we fix the degree of polynomial Nx=Ny=20. It can be observed that the time direction convergence order is O(τ2−α), which is consistent with our theoretical analysis. To test the convergence order in spatial direction, we set τ=1/1000 for α=0.3,0.5, and 0.9. The relationship between the errors and polynomial degree N is shown in the semi-log graph in Figure 2. We find that the numerical solution has the exponential accuracy.
α=0.3 | α=0.5 | α=0.9 | ||||||
τ | Error | Order | τ | Error | Order | τ | Error | Order |
1/10 | 9.3238e-05 | * | 1/10 | 2.3796e-04 | * | 1/10 | 1.1903e-03 | * |
1/20 | 2.9882e-05 | 1.6416 | 1/20 | 8.6493e-05 | 1.4601 | 1/20 | 5.6183e-04 | 1.0831 |
1/40 | 9.4754e-06 | 1.6570 | 1/40 | 3.1118e-05 | 1.4748 | 1/40 | 2.6352e-04 | 1.0922 |
1/80 | 2.9837e-06 | 1.6671 | 1/80 | 1.1129e-05 | 1.4834 | 1/80 | 1.2326e-04 | 1.0962 |
Example 2. To test the effectiveness of the fast difference/spectral collocation scheme (4.13), we consider problem (1.1) with exact solution u(x,y,t)=(3−(2+2t+t2)e−t)sin(πx)sin(2πy), and the source term and initial values are calculated by the exact solution.
The numerical results of schemes (3.4) and (4.13) are listed in Tables 2 and 3. In these tables, we compared the errors, convergence orders, and CPU times of the two schemes in Tables 2 and 3 for α=0.5 and α=0.7, respectively. In the implementation of fast difference/spectral collocation, we take ε=1e−9, and it can be seen that the time convergence order of fast solver is O(τ2−α), which is consistent with our theoretical results. In this example, the CPU(s) are measured, and the total times of numerical errors for the time steps τ vary in [1/40,1/80,1/160,1/320,1/640,1/1280]. We also observe that the fast L1 scheme/spectral collocation has lower complexity and achieves the same accuracy as the L1 scheme ones.
Direct scheme (3.4) | Fast scheme (4.13) | ||||
τ | Error | Order | τ | Error | Order |
1/40 | 6.7179e-06 | * | 1/40 | 6.5977e-06 | * |
1/80 | 2.3550e-06 | 1.5123 | 1/80 | 2.3258e-06 | 1.5042 |
1/160 | 8.2788e-07 | 1.5082 | 1/160 | 8.2074e-07 | 1.5027 |
1/320 | 2.9156e-07 | 1.5056 | 1/320 | 2.8981e-07 | 1.5018 |
1/640 | 1.0281e-07 | 1.5038 | 1/640 | 1.0237e-07 | 1.5013 |
1/1280 | 3.6276e-08 | 1.5029 | 1/1280 | 3.6269e-08 | 1.5010 |
CPU(s) | 657.5 | CPU(s) | 42.6 |
Direct scheme (3.4) | Fast scheme (4.13) | ||||
τ | Error | Order1 | τ | Error | Order1 |
1/40 | 1.9589e-05 | * | 1/40 | 1.9405e-05 | * |
1/80 | 7.8911e-06 | 1.3117 | 1/80 | 7.8473e-06 | 1.3061 |
1/160 | 3.1894e-06 | 1.3069 | 1/160 | 3.1789e-06 | 1.3036 |
1/320 | 1.2916e-06 | 1.3041 | 1/320 | 1.2891e-06 | 1.3021 |
1/640 | 5.2369e-07 | 1.3023 | 1/640 | 5.2313e-07 | 1.3011 |
1/1280 | 2.1246e-07 | 1.3015 | 1/1280 | 2.1237e-07 | 1.3005 |
CPU(s) | 659.0 | CPU(s) | 61.7 |
In this paper, the two-dimensional fractional diffusion equation with generalized memory kernel is analyzed and approximated in time and space. We used the spectral collocation method in the spatial direction, and the L1 formula and the fast L1 formula are used in time to approximate the fractional derivative. Finally, we obtained estimates of the temporal and spatial errors of the present schemes, the time and space error estimates are O(τ2−α+O(N1−m). By comparing the L1 scheme with the fast L1 scheme, we found that the fast L1 scheme can significantly reduce storage costs and computational costs. There were also many improvements in this article, such as the use of graded grids instead of uniform grids (e.g., Stynes et al. [11]) for time grids when the initial singularity of solution is considered. Our future work will focus on this issue with the help of the existing techniques developed in references [10,27,28].
Zunyuan Hu: Methodology, software, writing-original draft, writing-review & editing; Can Li: Conceptualization, methodology, writing-original draft, writing-review & editing; Shimin Guo: Methodology, writing-review & editing. All authors have read and approved the final version of the manuscript for publication.
The authors declare they have not used Artificial Intelligence (AI) tools in the creation of this article.
In this research, Can Li was partially supported by the Science Basic Research Plan in Shaanxi Province of China under Grant No. 2023-JC-YB-045. Shimin Guo was partially supported by the National Natural Science Foundation of China under Grant No. 12271427.
It is declared that none of the authors have any competing interests in this manuscript.
(1) According to the definitions of ρk,αk−j and c0, we obtain
ρk,αk−j=(cj−1−cj)c−10,ρk,α0=ck−1c−10,c−10=1λ1/2a0+(λ0−λ1)b0=1e−bτ/2+(1−e−bτ)(12−α−12)>0. |
By simple calculation, and using Lemma 1, we have
cj−1−cj=λj−1/2aj−1−λj+1/2aj+(λj−1−λj)bj−1−(λj−λj+1)bj>0. |
(2) After calculating term by term, we obtain
k∑j=1ρk,αk−j=ρk,α0+ρk,α1+ρk,α2+…+ρk,αk−1=c−10[(c0−c1)+(c1−c2)+(c2−c3)+…+ck−1]=c−10c0=1. |
(3) Using Lemma 1 once again, we have
ρk,α0=ck−1c−10=λk−1/2ak−1+(λk−1−λk)bk−1λ1/2a0+(λ0−λ1)b0>ταΓ(2−α)λk−1/2Γ(1−α)tαk⋅1λ1/2a0+(λ0−λ1)b0>λk−1/2(1−α)kα⋅1λ1/2a0+(λ0−λ1)b0>2(1−α)λk−1/23kα. |
(4) Since (1) provides that ρk,αk−1>0, then ρk,αk−1=(c0−c1)c−10=1−c1c0<1.
(5) Note that
c0=λ1/2a0+(λ0−λ1)b0=e−12bτ[1−e−12bτ(12−α−12)]+(12−α−12)=e−12bτ[1−e−12bτZ]+Z, |
where Z=12−α−12, 0≤Z≤12. We may rewrite c0 as follows
c0=e−12bτ[1−e−12bτZ]+Z:=g(τ,Z). |
By checking the partial derivatives of g with respect to variable Z, we obtain ∂g(τ,Z)∂Z=1−e−bτ≥0, which means g(τ,Z) is monotone increasing function with respect to variable Z, thus we get c0≤e−12bτ[1−12e−12bτ]+12, and c0≥e−12bτ. Furthermore, we define ˜g(τ)=e−12bτ[1−12e−12bτ]+12 and take the derivative of ˜g(τ), we obtain
∂˜g(τ)∂τ=−12be−12bτ[1−12e−12bτ]+14be−bτ=14be−bτ−12be−12bτ+14be−bτ=be−12bτ[12e−12bτ−12]≤0. |
Hence, ˜g(τ)≤1, 0<c0=g(τ,Z)≤˜g(τ)≤1, it provides that c−10=1g(τ,Z)≥1˜g(τ)≥1. Finally we get 1≤c−10≤e12bτ.
(1) From Lemma 5, for j=1,2,...,k, we have ρk,αk−j>0, thus σ=12ρk,αk−1>0. By using ˉρk,αk−j=∑jl=1σj−lˉρk,αk−l−σj, we further have
ˉρk,αk−j=j∑l=1σj−lˉρk,αk−l−σj=(2σj+σj−2ˉρk,αk−2+σj−3ˉρk,αk−3+...+σˉρk,αk−j+l+ˉρk,αk−j)−σj=σj+σj−2ˉρk,αk−2+σj−3ˉρk,αk−3+...+σˉρk,αk−j+l+ˉρk,αk−j>0. |
(2) According to Lemma 5, we have 1ρk,α0<3kα2(1−α)λk−1/2. Moreover, note that ρk,α0<ˉρk,α0, we have 1ˉρk,α0<1ρk,α0<3kα2(1−α)λk−1/2.
(3) Note that 1ˉρk,α0<3kα2(1−α)λk−1/2, we may check that
k∑j=1ˉρk,αk−j=ρk,αk−1(1+σ+σ2+σ3…+σk−1)+ρk,αk−2(1+σ+σ2+σ3…+σk−2)+…+ρk,α2(1+σ+σ2)+ρk,α1(1+σ)+ρk,α0−(σ+σ2+σ3…+σk)=11−σ[(ρk,αk−1+ρk,αk−2+ρk,αk−3+…+ρk,α1+ρk,α0)−σ(1−σk)−σ(ρk,αk−1σk−1+ρk,αk−2σk−2+…+ρk,α1σ+ρk,α0)]=1−σ1−σˉρk,α0≤1−2σ(1−α)λk−1/23(1−σ)kα. |
(4) In view of |ζk,αk−j|≤2ταε, we obtain |ˉζk,αk−j|=|∑jl=0σj−lζk,αk−j|≤2ταε∑jl=0σl≤4ταε.
(5) From Lemma 5, we have σ=12ρk,αk−1<12.
[1] |
G. M. Zaslavsky, Chaos, fractional kinetics, and anomalous transport, Phys. Rep., 371 (2022), 461–580. https://doi.org/10.1016/S0370-1573(02)00331-9 doi: 10.1016/S0370-1573(02)00331-9
![]() |
[2] |
R. L. Magin, Fractional calculus in bioengineering, Crit. Rev. Biomed. Eng., 32 (2004), 1–104. https://doi.org/10.1615/critrevbiomedeng.v32.i1.10 doi: 10.1615/critrevbiomedeng.v32.i1.10
![]() |
[3] | W. H. Deng, R. Hou, W. L. Wang, P. B. Xu, Modeling anomalous diffusion: From statistics to mathematics, Singapore: World Scientific, 2020. https://doi.org/10.1142/11630 |
[4] |
T. Sandev, A. Chechkin, H. Kantz, R. Metzler, Diffusion and Fokker-Planck-Smoluchowski equations with generalized memory kernel, Fract. Calc. Appl. Anal., 18 (2015), 1006–1038. https://doi.org/10.1515/fca-2015-0059 doi: 10.1515/fca-2015-0059
![]() |
[5] |
A. A. Alikhanov, A time-fractional diffusion equation with generalized memory kernel in differential and difference settings with smooth solutions, Comput. Meth. Appl. Mat., 17 (2017), 1–14. https://doi.org/10.1515/cmam-2017-0035 doi: 10.1515/cmam-2017-0035
![]() |
[6] |
Y. Luchko, M. Yamamoto, The general fractional derivative and related fractional differential equations, Mathematics, 8 (2020), 2115. https://doi.org/10.3390/math8122115 doi: 10.3390/math8122115
![]() |
[7] |
J. G. Liu, F. Z. Geng, An explanation on four new definitions of fractional operators, Acta Math. Sci., 44 (2024), 1271–1279. https://doi.org/10.1007/s10473-024-0405-7 doi: 10.1007/s10473-024-0405-7
![]() |
[8] |
B. I. Henry, T. A. M. Langlands, S. L. Wearne, Anomalous diffusion with linear reaction dynamics: From continuous time random walks to fractional reaction-diffusion equations, Phys. Rev. E, 74 (2006), 031116. https://doi.org/10.1103/PhysRevE.74.031116 doi: 10.1103/PhysRevE.74.031116
![]() |
[9] |
L. Zhao, C. Li, F. Q. Zhao, Efficient diference schemes for the Caputo-tempered fractional difusion equations based on polynomial interpolation, Com. Appl. Math. Comput., 3 (2021), 1–40. https://doi.org/10.1007/s42967-020-00067-5 doi: 10.1007/s42967-020-00067-5
![]() |
[10] |
M. H. Chen, Z. S. Jiang, W. P. Bu, Two L1 schemes on graded meshes for fractional Feynman-Kac equation, J. Sci. Comput., 88 (2021), 1–24. https://doi.org/10.1007/s10915-021-01581-1 doi: 10.1007/s10915-021-01581-1
![]() |
[11] |
M. Stynes, E. O'Riordan, J. L. Gracia, Error analysis of a finite difference method on graded meshes for a time-fractional diffusion equation, SIAM. J. Numer. Anal., 55 (2017), 1057–1079. https://doi.org/10.1137/16M1082329 doi: 10.1137/16M1082329
![]() |
[12] |
L. Greengard, P. Lin, Spectral approximation of the free-space heat kernel, Appl. Comput. Harmon. A., 9 (2000), 83–97. https://doi.org/10.1006/acha.2000.0310 doi: 10.1006/acha.2000.0310
![]() |
[13] |
S. D. Jiang, J. W. Zhang, Q. Zhang, Z. M. Zhang, Fast evaluation of the Caputo fractional derivative and its applications to fractional diffusion equations, Commun. Comput. Phys., 21 (2017), 650–678. https://doi.org/10.4208/cicp.OA-2016-0136 doi: 10.4208/cicp.OA-2016-0136
![]() |
[14] |
Y. G. Yan, Z. Z. Sun, J. W. Zhang, Fast evaluation of the Caputo fractional derivative and its applications to fractional diffusion equations: A second-order scheme, Commun. Comput. Phys., 22 (2017), 1028–1048. https://doi.org/10.4208/cicp.OA-2016-0136 doi: 10.4208/cicp.OA-2016-0136
![]() |
[15] |
H. Y. Zhu, C. J. Xu, A fast high order method for the time-fractional diffusion equation, SIAM. J. Numer. Anal., 57 (2019), 2829–2849. https://doi.org/10.1137/18M1231225 doi: 10.1137/18M1231225
![]() |
[16] |
X. M. Gu, T. Z. Huang, Y. L. Zhao, P. Lyu, B. Carpentieri, A fast implicit difference scheme for solving the generalized time-space fractional diffusion equations with variable coefficients, Numer. Meth. Part. D. E., 37 (2020), 1136–1162. https://doi.org/10.1002/num.22571 doi: 10.1002/num.22571
![]() |
[17] | B. Y. Guo, Spectral methods and their applications, Singapore: World Scientific, 1998. https://doi.org/10.1142/3662 |
[18] | J. Shen, T. Tang, L. L. Wang, Spectral methods: Algorithms, analysis and applications, Berlin: Springer-Verlag, 2011. https://doi.org/10.1007/978-3-540-71041-7 |
[19] |
Y. M. Lin, C. J. Xu, Finite difference/spectral approximations for the time-fractional diffusion equation, J. Comput. Phys., 225 (2007), 1533–1552. https://doi.org/10.1016/j.jcp.2007.02.001 doi: 10.1016/j.jcp.2007.02.001
![]() |
[20] |
X. J. Li, C. J. Xu, A space-time spectral method for the time fractional diffusion equation, SIAM J. Numer. Anal., 47 (2009), 2108–2131. https://doi.org/10.1137/080718942 doi: 10.1137/080718942
![]() |
[21] |
F. H. Zeng, F. W. Liu, C. P. Li, K. Burrage, I. Turner, V. Anh, A Crank-Nicolson ADI spectral method for a two-dimensional Riesz space fractional nonlinear reaction-diffusion equation, SIAM J. Numer. Anal., 52 (2014), 2599–2622. https://doi.org/10.1137/130934192 doi: 10.1137/130934192
![]() |
[22] |
S. M. Guo, L. Q. Mei, Y. Li, An efficient Galerkin spectral method for two-dimensional fractional nonlinear reaction-diffusion-wave equation, Comput. Math. Appl., 74 (2017), 2449–2465. https://doi.org/10.1016/j.camwa.2017.07.022 doi: 10.1016/j.camwa.2017.07.022
![]() |
[23] |
Y. Maday, B. Meétivet, Chebyshev spectral approximation of Navier-Stokes equations in a two dimensional domain, ESAIM-Math. Model. Num., 21 (1987), 93–123. https://doi.org/10.1051/m2an/1987210100931 doi: 10.1051/m2an/1987210100931
![]() |
[24] | C. W. Lv, C. J. Xu, Improved error estimates of a finite difference/spectral method for time-fractional diffusion equations, Int. J. Numer. Anal. Mod., 12 (2015), 384–400. |
[25] | C. Bernardi, Y. Maday, Approximations spectrales de problemes aux limites elliptiques, Berlin: Springer-Verlag, 1992. |
[26] | A. Quarteroni, A. Valli, Numerical approximation of partial differential equations, Berlin: Springer-Verlag, 1994. https://doi.org/10.1007/978-3-540-85268-1 |
[27] |
Y. L. Jing, C. Li, Block-centered finite difference method for a tempered subdiffusion model with time dependent coefficients, Comput. Math. Appl., 145 (2023), 202–223. https://doi.org/10.1016/j.camwa.2023.06.014 doi: 10.1016/j.camwa.2023.06.014
![]() |
[28] |
J. F. Zhou, X. M. Gu, Y. L. Zhao, H. Li, A fast compact difference scheme with unequal time-steps for the tempered time-fractional Black-Scholes model, Int. J. Comput. Math., 101 (2024), 989–1011. https://doi.org/10.1080/00207160.2023.2254412 doi: 10.1080/00207160.2023.2254412
![]() |
α=0.3 | α=0.5 | α=0.9 | ||||||
τ | Error | Order | τ | Error | Order | τ | Error | Order |
1/10 | 9.3238e-05 | * | 1/10 | 2.3796e-04 | * | 1/10 | 1.1903e-03 | * |
1/20 | 2.9882e-05 | 1.6416 | 1/20 | 8.6493e-05 | 1.4601 | 1/20 | 5.6183e-04 | 1.0831 |
1/40 | 9.4754e-06 | 1.6570 | 1/40 | 3.1118e-05 | 1.4748 | 1/40 | 2.6352e-04 | 1.0922 |
1/80 | 2.9837e-06 | 1.6671 | 1/80 | 1.1129e-05 | 1.4834 | 1/80 | 1.2326e-04 | 1.0962 |
Direct scheme (3.4) | Fast scheme (4.13) | ||||
τ | Error | Order | τ | Error | Order |
1/40 | 6.7179e-06 | * | 1/40 | 6.5977e-06 | * |
1/80 | 2.3550e-06 | 1.5123 | 1/80 | 2.3258e-06 | 1.5042 |
1/160 | 8.2788e-07 | 1.5082 | 1/160 | 8.2074e-07 | 1.5027 |
1/320 | 2.9156e-07 | 1.5056 | 1/320 | 2.8981e-07 | 1.5018 |
1/640 | 1.0281e-07 | 1.5038 | 1/640 | 1.0237e-07 | 1.5013 |
1/1280 | 3.6276e-08 | 1.5029 | 1/1280 | 3.6269e-08 | 1.5010 |
CPU(s) | 657.5 | CPU(s) | 42.6 |
Direct scheme (3.4) | Fast scheme (4.13) | ||||
τ | Error | Order1 | τ | Error | Order1 |
1/40 | 1.9589e-05 | * | 1/40 | 1.9405e-05 | * |
1/80 | 7.8911e-06 | 1.3117 | 1/80 | 7.8473e-06 | 1.3061 |
1/160 | 3.1894e-06 | 1.3069 | 1/160 | 3.1789e-06 | 1.3036 |
1/320 | 1.2916e-06 | 1.3041 | 1/320 | 1.2891e-06 | 1.3021 |
1/640 | 5.2369e-07 | 1.3023 | 1/640 | 5.2313e-07 | 1.3011 |
1/1280 | 2.1246e-07 | 1.3015 | 1/1280 | 2.1237e-07 | 1.3005 |
CPU(s) | 659.0 | CPU(s) | 61.7 |
α=0.3 | α=0.5 | α=0.9 | ||||||
τ | Error | Order | τ | Error | Order | τ | Error | Order |
1/10 | 9.3238e-05 | * | 1/10 | 2.3796e-04 | * | 1/10 | 1.1903e-03 | * |
1/20 | 2.9882e-05 | 1.6416 | 1/20 | 8.6493e-05 | 1.4601 | 1/20 | 5.6183e-04 | 1.0831 |
1/40 | 9.4754e-06 | 1.6570 | 1/40 | 3.1118e-05 | 1.4748 | 1/40 | 2.6352e-04 | 1.0922 |
1/80 | 2.9837e-06 | 1.6671 | 1/80 | 1.1129e-05 | 1.4834 | 1/80 | 1.2326e-04 | 1.0962 |
Direct scheme (3.4) | Fast scheme (4.13) | ||||
τ | Error | Order | τ | Error | Order |
1/40 | 6.7179e-06 | * | 1/40 | 6.5977e-06 | * |
1/80 | 2.3550e-06 | 1.5123 | 1/80 | 2.3258e-06 | 1.5042 |
1/160 | 8.2788e-07 | 1.5082 | 1/160 | 8.2074e-07 | 1.5027 |
1/320 | 2.9156e-07 | 1.5056 | 1/320 | 2.8981e-07 | 1.5018 |
1/640 | 1.0281e-07 | 1.5038 | 1/640 | 1.0237e-07 | 1.5013 |
1/1280 | 3.6276e-08 | 1.5029 | 1/1280 | 3.6269e-08 | 1.5010 |
CPU(s) | 657.5 | CPU(s) | 42.6 |
Direct scheme (3.4) | Fast scheme (4.13) | ||||
τ | Error | Order1 | τ | Error | Order1 |
1/40 | 1.9589e-05 | * | 1/40 | 1.9405e-05 | * |
1/80 | 7.8911e-06 | 1.3117 | 1/80 | 7.8473e-06 | 1.3061 |
1/160 | 3.1894e-06 | 1.3069 | 1/160 | 3.1789e-06 | 1.3036 |
1/320 | 1.2916e-06 | 1.3041 | 1/320 | 1.2891e-06 | 1.3021 |
1/640 | 5.2369e-07 | 1.3023 | 1/640 | 5.2313e-07 | 1.3011 |
1/1280 | 2.1246e-07 | 1.3015 | 1/1280 | 2.1237e-07 | 1.3005 |
CPU(s) | 659.0 | CPU(s) | 61.7 |