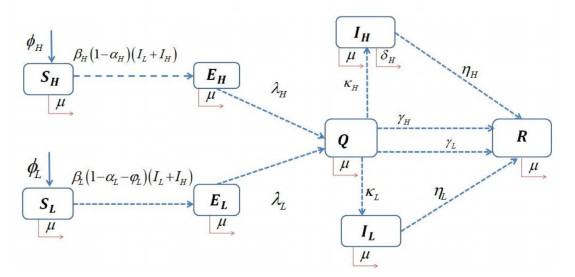
The use of vaccines has always been controversial. Individuals in society may have different opinions about the benefits of vaccines. As a result, some people decide to get vaccinated, while others decide otherwise. The conflicting opinions about vaccinations have a significant impact on the spread of a disease and the dynamics of an epidemic. This study proposes a mathematical model of COVID-19 to understand the interactions of two populations: the low risk population and the high risk population, with two preventive measures. Unvaccinated individuals with chronic diseases are classified as high risk population while the rest are a low risk population. Preventive measures used by low risk group include vaccination (pharmaceutical way), while for the high risk population they include wearing masks, social distancing and regular hand washing (non-pharmaceutical ways). The susceptible and infected sub-populations in both the low risk and the high risk groups were studied in detail through calculations of the effective reproduction number, model analysis, and numerical simulations. Our results show that the introduction of vaccination in the low risk population will significantly reduce infections in both subgroups.
Citation: Siriprapa Ritraksa, Chadaphim Photphanloet, Sherif Eneye Shuaib, Arthit Intarasit, Pakwan Riyapan. Mathematical modeling to study the interactions of two risk populations in COVID-19 spread in Thailand[J]. AIMS Mathematics, 2023, 8(1): 2044-2061. doi: 10.3934/math.2023105
[1] | Moh. Mashum Mujur Ihsanjaya, Nanang Susyanto . A mathematical model for policy of vaccinating recovered people in controlling the spread of COVID-19 outbreak. AIMS Mathematics, 2023, 8(6): 14508-14521. doi: 10.3934/math.2023741 |
[2] | Maria M. Martignoni, Proton Rahman, Amy Hurford . Rotational worker vaccination provides indirect protection to vulnerable groups in regions with low COVID-19 prevalence. AIMS Mathematics, 2022, 7(3): 3988-4003. doi: 10.3934/math.2022220 |
[3] | C. W. Chukwu, Fatmawati . Modelling fractional-order dynamics of COVID-19 with environmental transmission and vaccination: A case study of Indonesia. AIMS Mathematics, 2022, 7(3): 4416-4438. doi: 10.3934/math.2022246 |
[4] | Khalaf M. Alanazi . The asymptotic spreading speeds of COVID-19 with the effect of delay and quarantine. AIMS Mathematics, 2024, 9(7): 19397-19413. doi: 10.3934/math.2024945 |
[5] | Mdi Begum Jeelani, Abeer S Alnahdi, Rahim Ud Din, Hussam Alrabaiah, Azeem Sultana . Mathematical model to investigate transmission dynamics of COVID-19 with vaccinated class. AIMS Mathematics, 2023, 8(12): 29932-29955. doi: 10.3934/math.20231531 |
[6] | Asma Hanif, Azhar Iqbal Kashif Butt, Tariq Ismaeel . Fractional optimal control analysis of Covid-19 and dengue fever co-infection model with Atangana-Baleanu derivative. AIMS Mathematics, 2024, 9(3): 5171-5203. doi: 10.3934/math.2024251 |
[7] | Oluwaseun F. Egbelowo, Justin B. Munyakazi, Manh Tuan Hoang . Mathematical study of transmission dynamics of SARS-CoV-2 with waning immunity. AIMS Mathematics, 2022, 7(9): 15917-15938. doi: 10.3934/math.2022871 |
[8] | Xiaoying Pan, Longkun Tang . A new model for COVID-19 in the post-pandemic era. AIMS Mathematics, 2024, 9(8): 21255-21272. doi: 10.3934/math.20241032 |
[9] | Deniz UÇAR, Elçin ÇELİK . Analysis of Covid 19 disease with SIR model and Taylor matrix method. AIMS Mathematics, 2022, 7(6): 11188-11200. doi: 10.3934/math.2022626 |
[10] | Ali Yousef . A fractional-order model of COVID-19 with a strong Allee effect considering the fear effect spread by social networks to the community and the existence of the silent spreaders during the pandemic stage. AIMS Mathematics, 2022, 7(6): 10052-10078. doi: 10.3934/math.2022560 |
The use of vaccines has always been controversial. Individuals in society may have different opinions about the benefits of vaccines. As a result, some people decide to get vaccinated, while others decide otherwise. The conflicting opinions about vaccinations have a significant impact on the spread of a disease and the dynamics of an epidemic. This study proposes a mathematical model of COVID-19 to understand the interactions of two populations: the low risk population and the high risk population, with two preventive measures. Unvaccinated individuals with chronic diseases are classified as high risk population while the rest are a low risk population. Preventive measures used by low risk group include vaccination (pharmaceutical way), while for the high risk population they include wearing masks, social distancing and regular hand washing (non-pharmaceutical ways). The susceptible and infected sub-populations in both the low risk and the high risk groups were studied in detail through calculations of the effective reproduction number, model analysis, and numerical simulations. Our results show that the introduction of vaccination in the low risk population will significantly reduce infections in both subgroups.
After more than a year of continuing to impose different travel restrictions to combat the COVID-19 pandemic, Thailand has lifted its pre-arrival and arrival testing requirements for international visitors [1]. For several months only few locally transmitted COVID-19 cases have been reported due to strict quarantine rules for arrivals. As of June 17, 2022, more than 30,000 deaths and over 4 million COVID-19 cases have been recorded, with an average of 2,000 COVID-19 cases per day [2]. Vaccination is an essential tool for primary prevention and the major way for the long-term management strategy of the COVID-19 outbreak [3]. As of June 22, 2022, 66.3 percent of the world's population has been vaccinated against COVID-19 with at least one dose of vaccine. Globally, 12 billion doses have been administered with 6.33 million people vaccinated each day. Only 17.8 percent of people in low-income countries have received at least one dose [4]. In Thailand, about 81 percent of the population have received at least one vaccine dose; 73 percent have received two vaccine doses; and a third dose have been received by 36 percent of the population [5]. The geographic distribution of vaccine coverage in Thailand shows that people who live in Bangkok have been vaccinated with at least one dose, while people living in provinces of the metropolitan area have also received the first dose of vaccination. However, there was significantly less vaccination coverage for people in areas far from Bangkok [6]. The use of vaccines has always been controversial. A large proportion of the population in many countries remains reluctant to get the COVID-19 vaccination [7] because it is believed that the vaccination side effects may be worse than the disease itself. Others cite their religious and political beliefs as reasons [8]. People in a society may have different opinions about the benefits of vaccines and may convince relatives or friends not to get a vaccination [9]. At the inception of COVID-19, individuals from different countries worldwide were skeptical about the COVID-19 shots. The governments of these countries came up with different techniques and mediums to enforce the vaccinations. These include fines (Greece), lockdowns of unvaccinated (Austria), refusal of entry into bars and restaurants (Finland and Lithuania), travel and entry bans (all countries), compulsory vaccination of employees (Hungary), voucher gifts (Slovakia), and self-payment of medical bills (Singapore) [10]. The attitudes towards vaccines have clear consequences on the spread of diseases and their transformation into epidemics [9]. At the time of writing this article, at least 218 countries and territories have administered more than 12 billion doses of a COVID-19 vaccine. Furthermore, several different vaccines have been developed at record speed, in large part due to years of research on related viruses and billions of dollars in investment. Therefore, to measure the progress of different countries is a challenge because many countries are using two-dose vaccines. These inconsistent data make it difficult to determine the total or partial number of people vaccinated [11]. Tourism is a huge driver of the Thai economy earning about 20% of its gross domestic product (GDP). In 2019, Thailand was the 8th largest tourist destination in the world, with China being an important market. It has also welcomed 40 million visitors, with the top three spending categories for inbound visitors being accommodation (28%), shopping (24%), and food and beverage (21%). Unfortunately, the pandemic and related restrictions have affected Thai tourism. This is due to the decline in international travel, with passengers on international flights to Thailand down 95 percent in September 2021 compared to the previous year [12]. Recently, Thailand's Centre of COVID-19 Situation Administration (CCSA) has approved the cancellation of Thailand Pass registration and US$ 10,000 health insurance requirements for foreign tourists visiting Thailand, effective July 1, 2022 [13]. Currently, 32.9% of the world's population has not been vaccinated [14]. Thailand remains one of the most popular tourist destinations in the world. Thus, it is expected that many individuals (vaccinated and unvaccinated) worldwide will look to travel here, especially since most governments and airlines are less strict with travel requirements. Inspired by this situation, this study was done through modeling and simulation techniques in order to understand the interactions that will occur between individuals who are considered low risk (people who have received two or more doses of the vaccine) and individuals at a high risk (people who have not been vaccinated, have underlying diseases, and those under 5 years of age) in Thailand by considering Bangkok and Phuket as case studies. Bangkok is included because that it is the capital city of Thailand. In addition, from January to June 2021, Bangkok generated the highest amount of tourist revenue namely slightly over 41 billion baht (1,154,216,830 USD). Besides Bangkok, Phuket is also a popular tourist destination for foreigners [15].
This paper is organized as follows. In Section 2, we generate a new model with some assumptions which address the COVID-19 situation in Thailand, by presenting a diagram for describing a system of differential equations. In Section 3, we present the model dynamsics and analysis including to compute the effective reproduction number. Results are discussed in Section 4. Finally, we summarize and conclude our work with recommendations in Section 5.
Recently, Asempapa et al. [16] formulated a COVID-19 mathematical model in low- and high-risk populations with pharmaceutical and non-pharmaceutical measures. Brazil and South Africa were the subjects of case studies. In their study, the non-pharmaceutical interventions considered for the low risk population included wearing masks, social distancing, and regular hand washing. On the other hand, the high risk individuals must comply with additional precautions such as telework, and avoiding social gatherings or public places to reduce the spread of infection. Their study also classified people with chronic diseases and the elderly as high risk individuals while the rest are classified as low risk. One research gap in their study was that the effects of vaccination were not considered in either low- or high- risk groups. By using the enormous amount of data available in the studies mentioned above, we have created a model to understand the interactions that will occur between two different population groups in Thailand. The model will be divided into eight compartments, namely high risk susceptible (SH), low risk susceptible (SL), high risk exposed (EH), low risk exposed (EL), high risk infected (IH), low risk infected (IL), quarantined (Q), and recovered (R). In addition, the interventions were considered by Asempapa et al. [16], so our study focuses on vaccination in the low risk compartments.
The assumptions relating to the model are as follows:
● We assumed that the high risk susceptible class becomes exposed after interacting with the high risk infected, low risk infected, or those who are quarantined.
● Similarly, low risk susceptible population becomes exposed after interacting with the high risk infected, low risk infected, or those who are quarantined.
● High risk individuals considered in this study are individuals with underlying chronic diseases and the elderly (ages 60 and above) who have not been vaccinated.
● In low risk infection, we assumed that individuals are fully vaccinated. Thus, deaths can only arise naturally and not due to COVID-19 infection. However, in high risk infection compartment, death can occur naturally and due to COVID-19.
● We assumed that individuals both in the high risk and low risk class first move to quarantine. If they recover, then they move to the recovery compartment. Otherwise, they move to either the high risk infected class or low risk infected class, respectively.
● Also, reinfection in this model is not considered as a result of the current nature of COVID-19.
A diagram of the model based on above assumptions is illustrated in Figure 1.
Furthermore, the mathematical representation and variable descriptions of the dynamics in Figure 1 are given in (2.1) and Table 1, respectively.
dSLdt=ϕL−βL(1−αL−φL)(IL+IH)SL−μSL,dSHdt=ϕH−βH(1−αH)(IL+IH)SH−μSH,dELdt=βL(1−αL−φL)(IL+IH)SL−(λL+μ)EL,dEHdt=βH(1−αH)(IL+IH)SH−(λH+μ)EH,dQdt=λLEL+λHEH−(γL+γH+κL+κH+μ)Q,dILdt=κLQ−(ηL+μ)IL,dIHdt=κHQ−(ηH+δH+μ)IH,dRdt=ηHIH+ηLIL+γLQ+γHQ−μR. | (2.1) |
Variable/Parameter | Description |
SL | susceptible compartment for low risk individuals |
EL | exposed compartment for low risk individuals |
IL | infected compartment for low risk individuals |
SH | susceptible compartment for high risk individuals |
EH | exposed compartment for high risk individuals |
IH | infected compartment for high risk individuals |
Q | quarantine compartment |
R | recovered compartment |
ϕL | recruitment to the low risk susceptible class |
ϕH | recruitment to the high risk susceptible class |
βL | effective contact rate of low risk individuals |
βH | effective contact rate of high risk individuals |
αL | the use of non-pharmaceutical intervention (face masks) used by |
low risk individuals | |
αH | the use of non-pharmaceutical intervention (face masks) by high risk |
to reduce the transmission | |
φL | the use of pharmaceutical intervention (vaccination) used by |
the low risk individuals to reduce the transmission spread | |
λH | rate of progression by individuals in the high risk exposed compartment |
to the quarantine compartment | |
λL | rate of progression by individuals in the low risk exposed compartment |
to the quarantine compartment | |
κH | rate of progression by individuals in the high risk exposed compartment |
to the infected compartment | |
κL | rate of progression by individuals in the low risk exposed compartment |
to the infected compartment | |
ηH | rate of progression by individuals in the high risk infected compartment |
to the recovered compartment | |
ηL | rate of progression by individuals in the low risk infected compartment |
to the recovered compartment | |
δH | COVID-19 mortality rate for individuals in the high risk |
infected compartment | |
γL | recovery rate for low risk individuals in the quarantine compartment |
γH | recovery rate for high risk individuals in the quarantine compartment |
μ | natural death rate for individuals in all compartments |
In this section, we will present details relating to dynamics and analysis of the formulated model.
The dynamics of the model are carried out by examining if it exists in an invariant region.
In this section, the COVID-19 model given in (2.1) will be analyzed in a suitable feasible region to show that it is biologically relevant (mathematically well-posed and meaningful biologically) when all the variables and parameters in the model are non-negative for all time t≥0.
Lemma 3.1. The region Ω={(SL,SH,EL,EH,Q,IL,IH,R)∈R8+≤ϕL+ϕHμ} is positively-invariant for Model (2.1) with non-negative initial conditions in R8+.
Proof. If (SL,SH,EL,EH,Q,IL,IH,R)∈R8+ denote any solutions of Model (2.1), then the addition of all the model equations yield:
dNdt=ϕL+ϕH−μN−δHIH≤ϕL+ϕH−μN. |
Since (SL,SH,EL,EH,Q,IL,IH,R) are all non-negative, then N(0)≥0. Using integration, this becomes
N(t)≤N(0)e−μt+ϕL+ϕHμ(1−e−μt). |
Thus, if N(t)≤ϕL+ϕHμ, then N(0)≤ϕL+ϕHμ. Hence, it follows from [17] that Ω is positively invariant and initial conditions in Ω will remain in Ω for all time t>0.
The details relating to the analysis of the Model (2.1) are presented in this section. We begin with computing all the equilibrium points of Model (2.1) by setting all derivatives of each compartment to zero. This is represented by
dSLdt=dSHdt=dELdt=dEHdt=dILdt=dIHdt=dQdt=dRdt=0. | (3.1) |
We can find several equilibrium points from Eq (3.1), but in epidemiology, we focus on two equilibrium points: the COVID-19 free equilibrium and the COVID-19 endemic equilibrium.
The COVID-19 free equilibrium point is that there are no COVID-19 infections within the two populations (low risk and high risk) considered in this study. This implies that IL=IH=0. Thus, we denote the COVID-19 free equilibrium point as Ω0 which is
Ω0=(S∗L,E∗L,I∗L,S∗H,E∗H,I∗H,Q∗,R∗)=(ϕLμ,0,0,ϕHμ,0,0,0,0), |
where ϕLμ denotes the initial size of the low risk individuals in the susceptible compartment, and ϕHμ represents the initial size of the high risk individuals in the susceptible compartment.
One of the widely repeated terms during the outbreak of COVID-19 pandemic is the basic reproduction number (BRN). It denotes the average number of secondary infections that arise from a single infected individual. It is often denoted by R0. It is also regarded as a central concept in epidemiology, which indicates infection risk with respect to epidemic spread [16]. If R0>1, then the infected number of individuals is expected to rise. However, if R0<1, transmissions are expected to reduce or die out. In this study, we redefine the BRN as effective reproduction number (ERN) denoted as RHL. Here, RHL denotes the number of secondary cases of COVID-19 infection arising from one individual infected with COVID-19 in the presence of different pharmaceutical and non-pharmaceutical interventions. Using the next-generation matrix approach [18], the computation of RHL of Model (2.1) is given from Eq (3.2) to Eq (3.6).
ddt[ELILEHIHQ]=[βL(1−αL−φL)(IL+IH)SL0βH(1−αH)(IL+IH)SH00]−[(λL+μ)EL(ηL+μ)IL−κLQ(λH+μ)EH(ηH+δH+μ)IH−κHQ(γL+γH+κL+κH+μ)Q−λLEL−λHEH]. | (3.2) |
In the above, matrix F and matrix V of the transition terms are given by
F=[0βL(1−αL−φL)S∗L0βL(1−αL−φL)S∗L0000000βH(1−αH)S∗H0βH(1−αH)S∗H00000000000], | (3.3) |
and
V=[λL+μ00000ηL+μ00−κL00λH+μ00000ηH+δH+μ−κH−λL0−λH0γL+γH+κL+κH+μ]. | (3.4) |
The effective reproduction number obtained from the Model (2.1) is defined as the spectral radius of the product FV−1 which is given below
RHL=RL+RH, | (3.5) |
where
RL=λLϕLκLβL(1−αL−φL)μ(λL+μ)(ηL+μ)(γL+γH+κL+κH+μ) andRH=λHϕHκHβL(1−αH)μ(λH+μ)(ηH+δH+μ)(γL+γH+κL+κH+μ). |
As earlier stated in Section 3.2, RHL denotes the number of secondary cases of COVID-19 infection arising from one individual infected with COVID-19 in the presence of different pharmaceutical and non-pharmaceutical interventions. In this study, to achieve a low infection rate, then
RHL=λLϕLκLβL(1−αL−φL)K1+λHϕHκHβL(1−αH)K2<1 |
where K1=μ(λL+μ)(ηL+μ)(γL+γH+κL+κH+μ) and K2=μ(λH+μ)(ηH+δH+μ)(γL+γH+κL+κH+μ). Note that the optimal RHL will be computed in the numerical simulation section.
Here, we investigate the existence of endemic equilibrium point in Model (2.1) whenever RHL>1. Suppose we denote Ω0=(S∗∗L,S∗∗H,E∗∗L,E∗∗H,Q∗∗,I∗∗L,I∗∗H,R∗∗) as the endemic equilibrium point of Model (2.1), then Ω0 can be computed by setting the derivatives of each compartments in Model (2.1) to zero as shown below:
ϕL−βL(1−αL−φL)(I∗∗L+I∗∗H)S∗∗L−μS∗∗L=0,ϕH−βH(1−αH)(I∗∗L+I∗∗H)S∗∗H−μS∗∗H=0,βL(1−αL−φL)(I∗∗L+I∗∗H)S∗∗L−(λL+μ)E∗∗L=0,βH(1−αH)(I∗∗L+I∗∗H)S∗∗H−(λH+μ)E∗∗H=0,λLE∗∗L+λHE∗∗H−(γL+γH+κL+κH+μ)Q∗∗=0,κLQ∗∗−(ηL+μ)I∗∗L=0,κHQ∗∗−(ηH+δH+μ)I∗∗H=0,ηHI∗∗H+ηLI∗∗L+γLQ∗∗+γHQ∗∗−μR∗∗=0. | (3.6) |
Simplify Eq (3.6) by calculating S∗∗L,S∗∗H,E∗∗L,E∗∗H,Q∗∗,I∗∗L,I∗∗H and R∗∗ to obtain
S∗∗L=ϕLβL(1−αL−φL)(I∗∗L+I∗∗H)−μ,S∗∗H=ϕHβH(1−αH)(I∗∗L+I∗∗H)−μ,E∗∗L=ϕL(1−αL−φL)(I∗∗L+I∗∗H)(λL+μ)(1−αL−φL)−μ,E∗∗H=ϕH(1−αH)(I∗∗L+I∗∗H)(λH+μ)(1−αH),Q∗∗=λLE∗∗L+λHE∗∗HγL+γH+κL+κH+μ,I∗∗L=κLQ∗∗ηL+μ,I∗∗H=κHQ∗∗ηH+δH+μ,R∗∗=ηHI∗∗H+ηLI∗∗L+γLQ∗∗+γHQ∗∗μ. | (3.7) |
As described in the model formulation, the Model (2.1) was formulated based on two COVID-19 risk conditions: low and high risks. In order to attain a mathematical tractability, the Model (2.1) will be divided into two-sub models as follows: the first sub-model is
dSLdt=ϕL−βL(1−αL−φL)ILSL−μSL,dELdt=βL(1−αL−φL)ILSL−(λL+μ)EL,dQdt=λLEL−(γL+κL+μ)Q,dILdt=κLQ−(ηL+μ)IL,dRdt=ηLIL+γLQ−μR, | (3.8) |
and the second one is
dSHdt=ϕH−βH(1−αH)IHSH−μSH,dEHdt=βH(1−αH)IHSH−(λH+μ)EH,dQdt=λHEH−(γH+κH+μ)Q,dIHdt=κHQ−(ηH+δH+μ)IH,dRdt=ηHIH+γHQ−μR. | (3.9) |
where Eq (3.8) is low risk sub-model and Eq (3.9) is high risk sub-model, respectively. Since the long time behaviour of individuals to COVID-19 is of upmost importance, therefore, the stability analysis will only consider the global stability case. In addition, only the high risk sub-model will be analyzed.
The global stability analysis of Model (3.9) at the COVID-19 free equilibrium point is shown by the following theorem.
Theorem 3.2. For the Model (3.9), if RH<1, then the global asymptotic stability holds for the COVID-19 free equilibrium point when SH=S∗H and if RH>1, the the COVID-19 free equilibrium point is unstable.
Proof. To show the global stability at the COVID-19 free equilibrium point, the following Lyapunov function is considered
U(SH,EH,IH,Q,R)=13[(SH−S∗H)+(EH−E∗H)+(IH−I∗H)+(Q−Q∗)+(R−R∗)]3. | (3.10) |
Equation (3.10) is greater than zero at the COVID-19 free equilibrium point and equal to zero if we set SH=S∗H=ϕHμ,EH=E∗H=0,IH=I∗H=0,Q=Q∗=0,R=R∗=0. Differentiating (3.10), we obtain
dUdt(SH,0,0,0,0)=(SH−S∗H)2×dSHdt. | (3.11) |
The simplification of (3.11) yields
dUdt(SH,0,0,0,0)=−(SH−S∗H)2×(μSH−ϕH). | (3.12) |
Thus dUdt(SH,0,0,0,0)<0 which is globally asymptotic stability for the COVID-19 free equilibrium point is satisfied if and only if SH>ϕHμ.
The global asymptotic stability of the high risk sub-model will be discussed in this subsection using the Lyapunov's direct method and following from the study of De León (2009) [19].
Theorem 3.3. If RH>1, then the COVID-19 endemic equilibrium point of the high risk sub-model denoted as EE is globally asymptotically stable in the interior of region ΩH={(SH,EH,Q,IH,R)∈R5+≤ϕHμ}.
Proof. Suppose W:{(SH,EH,Q,IH,R)∈ΩH:SH,EH,Q,IH,R>0}→R. Constructing a common quadratic function using the high risk sub-model, we obtain:
W(SH,EH,Q,IH,R)=12[(SH−S∗∗H)+(EH−E∗∗H)+(Q−Q∗∗)+(IH−I∗∗H)+(R−R∗∗)]2. | (3.13) |
W is C1 on the interior of ΩH where EE is the global minimum of W on ΩH, and W(S∗∗H,E∗∗H,Q∗∗,I∗∗H,R∗∗)=0. Differentiating W along the solutions of high risk sub-Model (3.9), we obtain
∂W∂t=[(SH−S∗∗H)+(EH−E∗∗H)+(Q−Q∗∗)+(IH−I∗∗H)+(R−R∗∗)]×ddt(SH+EH+Q+IH+R), |
where
ddt(SH+EH+Q+IH+R)=ϕH−μ(SH+EH+Q+IH+R)−δHIH. |
Thus, (3.5) becomes:
∂W∂t=[(SH−S∗∗H)+(EH−E∗∗H)+(Q−Q∗∗)+(IH−I∗∗H)+(R−R∗∗)]×(ϕH−μ(SH+EH+Q+IH+R)−δHIH). | (3.14) |
Using ϕH=μ(S∗∗H+E∗∗H+Q∗∗+I∗∗H+R∗∗)−δHI∗∗H, (3.14) becomes:
∂W∂t=[(SH−S∗∗H)+(EH−E∗∗H)+(Q−Q∗∗)+(IH−I∗∗H)+(R−R∗∗)]×(μ(S∗∗H+E∗∗H+Q∗∗+I∗∗H+R∗∗)−μ(SH+EH+Q+IH+R)−δHI∗∗H−δHIH). | (3.15) |
From (3.15) we obtain:
∂W∂t=[(SH−S∗∗H)+(EH−E∗∗H)+(Q−Q∗∗)+(IH−I∗∗H)+(R−R∗∗)]×(−μ[(SH−S∗∗H)+(EH−E∗∗H)+(Q−Q∗∗)+(IH−I∗∗H)+(R−R∗∗)]−δH(IH−I∗∗H)). | (3.16) |
Let A1=SH−S∗∗H, A2=EH−E∗∗H, A3=Q−Q∗∗, A4=IH−I∗∗H A5=R−R∗∗, and A6=A1+A2+A3+A4+A5.
Thus, (3.16) becomes
∂W∂t=A6(−μA6−δHA4). | (3.17) |
From (3.17), we obtain
∂W∂t=−(μA26+δHA4A6). | (3.18) |
Hence
∂W∂t=−(μA26+δHA4A6)≤0. | (3.19) |
Also, ∂W∂t=0 if SH=S∗∗H,EH=E∗∗H,Q=Q∗∗, IH=I∗∗H and R=R∗∗ in (3.16). Hence, the largest compact invariant set in {(SH,EH,Q,IH,R)∈ΩH:∂W∂t=0} is the singleton EE, where EE is the COVID-19 endemic equilibrium point. Therefore by Lasalle's invariance principle, EE is globally asymptotically stable in the interior of ΩH.
The numerical simulations carried out in this study are presented in this section. The simulation largely focused on the effect of vaccination in the two populations. As stated earlier in the Introduction and Model formulation sections, an important assumption guiding the model is that only non-pharmaceutical intervention (wearing face masks) was employed by individuals in the high risk population. However, in addition to the non-pharmaceutical intervention, individuals in the low risk population have also employed pharmaceutical intervention (vaccination). To get a proper understanding of the effect and importance of vaccination used by individuals in the low risk compartment, a comparison of the infected population in the two groups was conducted by considering four scenarios as presented in Table 2.
Scenarios | Low risk population | High risk population |
Scenario 1 | wearing masks | wearing masks |
Scenario 2 | wearing masks and taking one vaccine dose | wearing masks |
Scenario 3 | wearing masks and taking two vaccine doses | wearing masks |
Scenario 4 | wearing masks and taking two vaccine doses | wearing masks |
with booster shot |
The parameter values used for the numerical simulation and their respective source are provided in Table 3.
Parameter | Value | Source |
ϕL | assumed | – |
ϕH | assumed | – |
βL | assumed | – |
βH | assumed | – |
αL=αH | 1/10 day−1 | [20]-[21] |
φL | 0.51 – 0.95 day−1 | [22] |
λL=λH | 1/7 day−1 | [23]-[24] |
κL=κH | 1/6 day−1 | [25] |
ηL=ηH | 1/14 day−1 | [23]-[24] |
δH | 0.015 day−1 | [26] |
γH | 1/10 day−1 | [20]-[21] |
γL | 0.05 day−1 | [20]-[21] |
μ | 3.625×10−5 day−1 | [27] |
Since the approval of COVID-19 vaccines, Thailand has administered and combined different vaccines (Sinovac, AstraZeneca, Pfizer, and Moderna). The vaccine most administered is Astra Zeneca [28]. For the simulation relating to the dynamics of the four scenarios, three parameters, φL (use of vaccination), αL (effect of face mask in low risk population) and αH (effect of face mask in high risk population) were of utmost importance. For scenario 1, φL=0. For Scenarios 2–4, respectively, φL=0.51,0.879 and 0.95. The values mentioned induce the expected decrease in infections as a result of one vaccine dose, two vaccine doses, and the combination of two vaccine doses with a booster dose, respectively. Plots obtained from the simulations are presented in Figures 2–5.
In addition to the plots obtained for each scenario, the effective reproduction number, associated with each scenario is presented in Table 4.
Scenarios | Effective reproduction number (RL) | Effective reproduction number (RH) |
Scenario 1 | RL=2.745>1 | RH=3.369>1 |
Scenario 2 | RL=1.345>1 | RH=3.369>1 |
Scenario 3 | RL=0.737<1 | RH=3.369>1 |
Scenario 4 | RL=0.485<1 | RH=3.369>1 |
As expected, the tabular results of the ERN associated with the four scenarios show that the vaccination plays a vital role in reducing the number of infections that can occur within the population.
In order to obtain the optimal ERN number when the two populations (RL and RH) are combined, we consider the application of Scenario 4 in two different cases. We recall that Scenario 4 is when the preventive measure for the low risk population is by "face masks, two vaccine doses and a booster vaccine shot". In case 1, we assume that individuals in the low risk population believe that since they have taken 3 vaccine shots, then there is no need to use face mask. For the high risk population, we assume that 50% of the population were constantly using face masks. In case 2, we assume that half of the low risk populations (50%) irrespective of their vaccine status continues to use face masks. In case 2, 90% of the high risk population used face masks. These assumptions are made based on the fact that so many countries worldwide are beginning to drop or lessen their mask mandates. The simulations of both cases were implemented using the values in Table 3 and the resulting graphical plots are presented in Figures 6 and 7, respectively.
The plot in Figure 6 indicates that even though everyone in the low risk population has received 3 vaccine shot doses, there are still infections arising in the population with ERN as RHL=0.985<1. However, these infections will likely not lead to hospitalizations. There is no vaccine that provides a 100% protection for any disease. This does not imply that the COVID-19 vaccines are not effective, it simply indicates that not everyone who received the vaccines in the low risk population has 100 percent protection. In Figure 7, the plot shows that there are fewer infections which will arise compared to Figure 6 with ERN as RHL=0.275<1. The reason for fewer infections is the fact that even though mask mandate has been dropped, half of the population are not just ready yet to stop wearing masks because they feel safer and secured when they use masks.
To examine the effects for introducing vaccination into a high risk population, different vaccination intakes were tested. Our assumption for this simulation is that vaccination replaces face mask (αH). Thus φL = αH. As earlier defined in the Table 1, φL denotes the use of vaccination and the values for different vaccination intake is provided in Section 4.1. For this simulation, the first, second and third vaccination intakes, respectively, were used together with other parameter values in Table 3 for the simulation. The obtained result from the simulation is presented in Figure 8.
Overall, three doses of vaccine intakes will result into less infected individual compared to two and one doses, respectively. The obtained result also reaffirms the idea that getting two or more vaccination doses contributes favourable in reducing the number of COVID-19 cases and deaths. Lastly, though this study has mostly shown the benefits of vaccination, according to the New York times (2022) [29] only 70.3% of the global population have received at least one dose as of October 10, 2022. Thus, there is still a need for the Thai government to continuously monitor the number of cases to build a strong system of prevention and control in Thailand to keep the tourism sector working.
In this study, we formulate a model to understand the interactions that exists within two populations: low risk and high risk populations and the preventive measures adopted by the respective populations. The formulated model was modified from Asempapa et al. [16] by adding vaccination as a preventive measure for the low risk population. The results have shown that vaccination is vital in reducing COVID-19 pandemic in Thailand. The Thai government has recently intensified efforts to achieve herd immunity through an efficient vaccination program. Initial shortage of vaccine supply together with a lack of vaccine options have slowed down this process. However, this has been improved. Thailand has opened its borders to foreign tourists on the 1st of July, 2022. Thus, it is important for individuals in Thailand to complete their full immunization to achieve herd immunity for COVID-19. Attaining herd immunity will go a long way in protecting individuals classified as having high risk and other individuals who are susceptible or vulnerable to the infection.
The authors are thankful to the Faculty of Science and Technology, Prince of Songkla University for the financial support with the contract number SAT64041735.
There are no conflicts of interest as declared by the authors.
[1] | Travel Week, "More room and less crowds" for visitors to reopened Thailand right now, 2022. Available from: https://www.travelweek.ca/news/more-room-and-less-crowds-for-visitors-to-reopened-thailand-right-now-tats-santi-sawangcharoen/. |
[2] | CNN Travel, Unlocking the world: traveling to Thailand during COVID-19: What you need to know before you go, 2022. Available from: https://edition.cnn.com/travel/article/thailand-travel-covid-19/index.html. |
[3] |
M. Piraveenan, S. Sawleshwarkar, M. Walsh, I. Zablotska, S. Bhattacharyya, H. H. Farooqui, et al., Optimal governance and implementation of vaccination programmes to contain the COVID-19 pandemic, R. Soc. Open Sci., 8 (2021), 210429. https://doi.org/10.1098/rsos.210429 doi: 10.1098/rsos.210429
![]() |
[4] |
M. Piraveenan, S. Sawleshwarkar, M. Walsh, I. Zablotska, S. Bhattacharyya, H. H. Farooqui, et al., Optimal governance and implementation of vaccination programmes to contain the COVID-19 pandemic, R. Soc. Open Sci., 8 (2021), 210429. https://doi.org/10.1098/rsos.210429 doi: 10.1098/rsos.210429
![]() |
[5] | Department of Disease Control, Thailand, COVID-19 vaccine progress, 2022. Available from: https://ddc.moph.go.th/vaccine-covid19/. |
[6] | J. Manatee, T. Chirasatienpon, R. Tiamjan, K. Amnatsatsue, R. Nguanjairak, A. V. Miranda, et al., Can Thailand achieve COVID‐19 herd immunity, Public Health Chall., 1 (2022). https://doi.org/10.1002/puh2.7 |
[7] |
F. Jana, K. Annac, F. Erdsiek, Y. Yilmaz-Aslan, P. Brzoska, What are the reasons for refusing a COVID-19 vaccine? A qualitative analysis of social media in Germany, BMC Public Health, 22 (2022), 1–8. https://doi.org/10.1186/s12889-022-13265-y doi: 10.1186/s12889-022-13265-y
![]() |
[8] |
A. Zuzek, G. Lucila, E. Cristian. L. Rocca, J. R. Iglesias, A. L. Braunstein, Epidemic spreading in multiplex networks influenced by opinion exchanges on vaccination, PLoS One, 12 (2017). https://doi.org/10.1371/journal.pone.0186492 doi: 10.1371/journal.pone.0186492
![]() |
[9] |
D. Graeber, C. Schmidt-Petri, C. Schröder, Attitudes on voluntary and mandatory vaccination against COVID-19: evidence from Germany, PLoS One, 16 (2017). https://doi.org/10.1371/journal.pone.0248372 doi: 10.1371/journal.pone.0248372
![]() |
[10] | Bloomberg News, These countries are slapping the unvaccinated with fines and bans, 2022. Available from: https://www.bloomberg.com/news/articles/2021-12-01/these-countries-are-slapping-the-unvaccinated-with-fines-bans. |
[11] | CNN Health, Tracking COVID-19 vaccinations worldwide, 2022. Available from: https://www.cnn.com/interactive/2021/health/global-covid-vaccinations/. |
[12] | Mckinsey Company, Reimagining travel: Thailand tourism after the COVID-19 pandemic, 2022. Available from: https://www.mckinsey.com/industries/travel-logistics-and-infrastructure/our-insights/reimagining-travel-thailand-tourism-after-the-covid-19-pandemic. |
[13] | TAT News, Thailand pass' registration scheme to be lifted from 1 July 2022, 2022. Available from: https://www.tatnews.org/2022/06/thailand-pass-registration-scheme-to-be-lifted-from-1-july-2022/. |
[14] | The New York Times, Tracking coronavirus vaccinations around the world, 2022. Available from: https://www.nytimes.com/interactive/2021/world/covid-vaccinations-tracker.html. |
[15] | Statista, Tourism industry in Thailand-statistics and facts, 2022. Available from: https://www.statista.com/topics/6845/tourism-industry-in-thailand/. |
[16] |
R. Asempapa, B. Oduro, O. O. Apenteng, V. M. Magagula, A COVID-19 mathematical model of at-risk populations with non-pharmaceutical preventive measures: the case of Brazil and South Africa, Infec. Dis. Model., 7 (2022), 45–61. https://doi.org/10.1016/j.idm.2021.11.005 doi: 10.1016/j.idm.2021.11.005
![]() |
[17] |
H. W. Hethcote, The mathematics of infectious diseases, SIAM Rev., 42 (2000), 599–653. https://doi.org/10.1137/S0036144500371907 doi: 10.1137/S0036144500371907
![]() |
[18] |
O. Diekmann, J. A. P. Heesterbeek, J. A. Metz, On the definition and the computation of the basic reproduction ratio R0 in models for infectious diseases in heterogeneous populations, J. Math. Biol., 28 (1990), 365–382. https://doi.org/10.1007/BF00178324 doi: 10.1007/BF00178324
![]() |
[19] | C. V. D. León, Constructions of lyapunov functions for classics SIS, SIR and SIRS epidemic model with variable population size, Foro. Red. Mat., 26 (2009), 1–12. |
[20] |
S. Eikenberry, M. Mancuso, E. Iboi, T. Phan, K. Eikenberry, Y. Kuang, et al., To mask or not to mask: Modeling the potential for face mask use by the general public to curtail the COVID-19 pandemic, Infec. Dis. Model., 5 (2020), 293–308. https://doi.org/10.1016/j.idm.2020.04.001 doi: 10.1016/j.idm.2020.04.001
![]() |
[21] | Imperial College London, Report 9: Impact of Non-pharmaceutical interventions (Npis) to reduce COVID19 mortality and healthcare demand, 2020. Available from: http://hdl.handle.net/10044/1/77482. |
[22] |
J. Bernal, N. Andrews, C. Gower, E. Gallagher, R. Simmons, S. Thelwall, et al., Effectiveness of COVID-19 vaccines against the B.1.617.2 (Delta) variant, N. Engl. J. Med., 385 (2021), 585–594. https://doi.org/10.1056/NEJMoa2108891 doi: 10.1056/NEJMoa2108891
![]() |
[23] |
B. Tang, X. Wang, Q. Li, N. L. Bragazzi, S. Tang, Y. Xiao, et al., Estimation of the transmission risk of the 2019-nCoV and its implication for public health interventions, J. Clin. Med., 9 (2020), 462. https://doi.org/10.3390/jcm9020462 doi: 10.3390/jcm9020462
![]() |
[24] |
F. Zhou, T. Yu, R. Du, G. Fan, Y. Liu, Z. Liu, et al., Clinical course and risk factors for mortality of adult inpatients with COVID-19 in Wuhan, China: A retrospective cohort study, Lancet, 395 (2020), 1054–1062. https://doi.org/10.1016/S0140-6736(20)30566-3 doi: 10.1016/S0140-6736(20)30566-3
![]() |
[25] |
H. A. Rothan, S. N. Byrareddy, The epidemiology and pathogenesis of coronavirus disease (COVID-19) outbreak, J. Autoimmun., 109 (2020), 102433. https://doi.org/10.1016/j.jaut.2020.102433 doi: 10.1016/j.jaut.2020.102433
![]() |
[26] | Imperial College COVID-19 Response Team, Impact of Non-Pharmaceutical Interventions (NPIs) to reduce COVID-19 mortality and healthcare demand, 2020. Available from: http://hdl.handle.net/10044/1/77482. |
[27] | DDC Open Date, Center of disease control, Thailand, 2021. Available from: https://covid19.ddc.moph.go.th/en. |
[28] | Live COVID-19 Vaccination Tracker, Number of people who received the COVID-19 vaccine, 2021. Available from: https://www.mhesi.go.th/index.php/content-page/item/3547-share-of-people-who-received-at-least-one-dose-of-covid-19-vaccine.html. |
[29] | The New York Times, Tracking coronavirus vaccinations around the world, 2022. Available from: https://www.nytimes.com/interactive/2021/world/covid-vaccinations-tracker.html. |
1. | Thitiya Theparod, Pannathon Kreabkhontho, Watchara Teparos, Booster Dose Vaccination and Dynamics of COVID-19 Pandemic in the Fifth Wave: An Efficient and Simple Mathematical Model for Disease Progression, 2023, 11, 2076-393X, 589, 10.3390/vaccines11030589 |
Variable/Parameter | Description |
SL | susceptible compartment for low risk individuals |
EL | exposed compartment for low risk individuals |
IL | infected compartment for low risk individuals |
SH | susceptible compartment for high risk individuals |
EH | exposed compartment for high risk individuals |
IH | infected compartment for high risk individuals |
Q | quarantine compartment |
R | recovered compartment |
ϕL | recruitment to the low risk susceptible class |
ϕH | recruitment to the high risk susceptible class |
βL | effective contact rate of low risk individuals |
βH | effective contact rate of high risk individuals |
αL | the use of non-pharmaceutical intervention (face masks) used by |
low risk individuals | |
αH | the use of non-pharmaceutical intervention (face masks) by high risk |
to reduce the transmission | |
φL | the use of pharmaceutical intervention (vaccination) used by |
the low risk individuals to reduce the transmission spread | |
λH | rate of progression by individuals in the high risk exposed compartment |
to the quarantine compartment | |
λL | rate of progression by individuals in the low risk exposed compartment |
to the quarantine compartment | |
κH | rate of progression by individuals in the high risk exposed compartment |
to the infected compartment | |
κL | rate of progression by individuals in the low risk exposed compartment |
to the infected compartment | |
ηH | rate of progression by individuals in the high risk infected compartment |
to the recovered compartment | |
ηL | rate of progression by individuals in the low risk infected compartment |
to the recovered compartment | |
δH | COVID-19 mortality rate for individuals in the high risk |
infected compartment | |
γL | recovery rate for low risk individuals in the quarantine compartment |
γH | recovery rate for high risk individuals in the quarantine compartment |
μ | natural death rate for individuals in all compartments |
Scenarios | Low risk population | High risk population |
Scenario 1 | wearing masks | wearing masks |
Scenario 2 | wearing masks and taking one vaccine dose | wearing masks |
Scenario 3 | wearing masks and taking two vaccine doses | wearing masks |
Scenario 4 | wearing masks and taking two vaccine doses | wearing masks |
with booster shot |
Parameter | Value | Source |
ϕL | assumed | – |
ϕH | assumed | – |
βL | assumed | – |
βH | assumed | – |
αL=αH | 1/10 day−1 | [20]-[21] |
φL | 0.51 – 0.95 day−1 | [22] |
λL=λH | 1/7 day−1 | [23]-[24] |
κL=κH | 1/6 day−1 | [25] |
ηL=ηH | 1/14 day−1 | [23]-[24] |
δH | 0.015 day−1 | [26] |
γH | 1/10 day−1 | [20]-[21] |
γL | 0.05 day−1 | [20]-[21] |
μ | 3.625×10−5 day−1 | [27] |
Scenarios | Effective reproduction number (RL) | Effective reproduction number (RH) |
Scenario 1 | RL=2.745>1 | RH=3.369>1 |
Scenario 2 | RL=1.345>1 | RH=3.369>1 |
Scenario 3 | RL=0.737<1 | RH=3.369>1 |
Scenario 4 | RL=0.485<1 | RH=3.369>1 |
Variable/Parameter | Description |
SL | susceptible compartment for low risk individuals |
EL | exposed compartment for low risk individuals |
IL | infected compartment for low risk individuals |
SH | susceptible compartment for high risk individuals |
EH | exposed compartment for high risk individuals |
IH | infected compartment for high risk individuals |
Q | quarantine compartment |
R | recovered compartment |
ϕL | recruitment to the low risk susceptible class |
ϕH | recruitment to the high risk susceptible class |
βL | effective contact rate of low risk individuals |
βH | effective contact rate of high risk individuals |
αL | the use of non-pharmaceutical intervention (face masks) used by |
low risk individuals | |
αH | the use of non-pharmaceutical intervention (face masks) by high risk |
to reduce the transmission | |
φL | the use of pharmaceutical intervention (vaccination) used by |
the low risk individuals to reduce the transmission spread | |
λH | rate of progression by individuals in the high risk exposed compartment |
to the quarantine compartment | |
λL | rate of progression by individuals in the low risk exposed compartment |
to the quarantine compartment | |
κH | rate of progression by individuals in the high risk exposed compartment |
to the infected compartment | |
κL | rate of progression by individuals in the low risk exposed compartment |
to the infected compartment | |
ηH | rate of progression by individuals in the high risk infected compartment |
to the recovered compartment | |
ηL | rate of progression by individuals in the low risk infected compartment |
to the recovered compartment | |
δH | COVID-19 mortality rate for individuals in the high risk |
infected compartment | |
γL | recovery rate for low risk individuals in the quarantine compartment |
γH | recovery rate for high risk individuals in the quarantine compartment |
μ | natural death rate for individuals in all compartments |
Scenarios | Low risk population | High risk population |
Scenario 1 | wearing masks | wearing masks |
Scenario 2 | wearing masks and taking one vaccine dose | wearing masks |
Scenario 3 | wearing masks and taking two vaccine doses | wearing masks |
Scenario 4 | wearing masks and taking two vaccine doses | wearing masks |
with booster shot |
Parameter | Value | Source |
ϕL | assumed | – |
ϕH | assumed | – |
βL | assumed | – |
βH | assumed | – |
αL=αH | 1/10 day−1 | [20]-[21] |
φL | 0.51 – 0.95 day−1 | [22] |
λL=λH | 1/7 day−1 | [23]-[24] |
κL=κH | 1/6 day−1 | [25] |
ηL=ηH | 1/14 day−1 | [23]-[24] |
δH | 0.015 day−1 | [26] |
γH | 1/10 day−1 | [20]-[21] |
γL | 0.05 day−1 | [20]-[21] |
μ | 3.625×10−5 day−1 | [27] |
Scenarios | Effective reproduction number (RL) | Effective reproduction number (RH) |
Scenario 1 | RL=2.745>1 | RH=3.369>1 |
Scenario 2 | RL=1.345>1 | RH=3.369>1 |
Scenario 3 | RL=0.737<1 | RH=3.369>1 |
Scenario 4 | RL=0.485<1 | RH=3.369>1 |