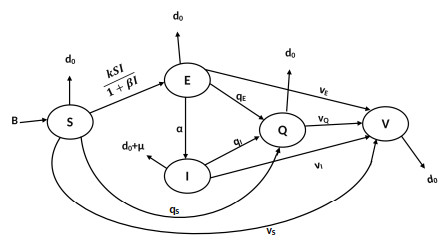
The susceptible, exposed, infected, quarantined and vaccinated (SEIQV) population is accounted for in a mathematical model of COVID-19. This model covers the therapy for diseased people as well as therapeutic measures like immunization for susceptible people to enable understanding of the dynamics of the disease's propagation. Each of the equilibrium points, i.e., disease-free and endemic, has been proven to be globally asymptotically stable under the assumption that R0 is smaller or larger than unity, respectively. Although vaccination coverage is high, the basic reproduction number depends on the vaccine's effectiveness in preventing disease when R0>0. The Jacobian matrix and the Routh-Hurwitz theorem are used to derive the aforementioned analysis techniques. The results are further examined numerically by using the standard second-order Runge-Kutta (RK2) method. In order to visualize the global dynamics of the aforementioned model, the proposed model is expanded to examine some piecewise fractional order derivatives. We may comprehend the crossover behavior in the suggested model's illness dynamics by using the relevant derivative. To numerical present the results, we use RK2 method.
Citation: Mdi Begum Jeelani, Abeer S Alnahdi, Rahim Ud Din, Hussam Alrabaiah, Azeem Sultana. Mathematical model to investigate transmission dynamics of COVID-19 with vaccinated class[J]. AIMS Mathematics, 2023, 8(12): 29932-29955. doi: 10.3934/math.20231531
[1] | C. W. Chukwu, Fatmawati . Modelling fractional-order dynamics of COVID-19 with environmental transmission and vaccination: A case study of Indonesia. AIMS Mathematics, 2022, 7(3): 4416-4438. doi: 10.3934/math.2022246 |
[2] | K. A. Aldwoah, Mohammed A. Almalahi, Mansour A. Abdulwasaa, Kamal Shah, Sunil V. Kawale, Muath Awadalla, Jihan Alahmadi . Mathematical analysis and numerical simulations of the piecewise dynamics model of Malaria transmission: A case study in Yemen. AIMS Mathematics, 2024, 9(2): 4376-4408. doi: 10.3934/math.2024216 |
[3] | Rahat Zarin, Amir Khan, Aurangzeb, Ali Akgül, Esra Karatas Akgül, Usa Wannasingha Humphries . Fractional modeling of COVID-19 pandemic model with real data from Pakistan under the ABC operator. AIMS Mathematics, 2022, 7(9): 15939-15964. doi: 10.3934/math.2022872 |
[4] | Oluwaseun F. Egbelowo, Justin B. Munyakazi, Manh Tuan Hoang . Mathematical study of transmission dynamics of SARS-CoV-2 with waning immunity. AIMS Mathematics, 2022, 7(9): 15917-15938. doi: 10.3934/math.2022871 |
[5] | Sara Salem Alzaid, Badr Saad T. Alkahtani . Real-world validation of fractional-order model for COVID-19 vaccination impact. AIMS Mathematics, 2024, 9(2): 3685-3706. doi: 10.3934/math.2024181 |
[6] | Moh. Mashum Mujur Ihsanjaya, Nanang Susyanto . A mathematical model for policy of vaccinating recovered people in controlling the spread of COVID-19 outbreak. AIMS Mathematics, 2023, 8(6): 14508-14521. doi: 10.3934/math.2023741 |
[7] | Ishtiaq Ali, Sami Ullah Khan . Dynamics and simulations of stochastic COVID-19 epidemic model using Legendre spectral collocation method. AIMS Mathematics, 2023, 8(2): 4220-4236. doi: 10.3934/math.2023210 |
[8] | Nadiyah Hussain Alharthi, Mdi Begum Jeelani . Analyzing a SEIR-Type mathematical model of SARS-COVID-19 using piecewise fractional order operators. AIMS Mathematics, 2023, 8(11): 27009-27032. doi: 10.3934/math.20231382 |
[9] | Chontita Rattanakul, Inthira Chaiya . A mathematical model for predicting and controlling COVID-19 transmission with impulsive vaccination. AIMS Mathematics, 2024, 9(3): 6281-6304. doi: 10.3934/math.2024306 |
[10] | Salah Boulaaras, Ziad Ur Rehman, Farah Aini Abdullah, Rashid Jan, Mohamed Abdalla, Asif Jan . Coronavirus dynamics, infections and preventive interventions using fractional-calculus analysis. AIMS Mathematics, 2023, 8(4): 8680-8701. doi: 10.3934/math.2023436 |
The susceptible, exposed, infected, quarantined and vaccinated (SEIQV) population is accounted for in a mathematical model of COVID-19. This model covers the therapy for diseased people as well as therapeutic measures like immunization for susceptible people to enable understanding of the dynamics of the disease's propagation. Each of the equilibrium points, i.e., disease-free and endemic, has been proven to be globally asymptotically stable under the assumption that R0 is smaller or larger than unity, respectively. Although vaccination coverage is high, the basic reproduction number depends on the vaccine's effectiveness in preventing disease when R0>0. The Jacobian matrix and the Routh-Hurwitz theorem are used to derive the aforementioned analysis techniques. The results are further examined numerically by using the standard second-order Runge-Kutta (RK2) method. In order to visualize the global dynamics of the aforementioned model, the proposed model is expanded to examine some piecewise fractional order derivatives. We may comprehend the crossover behavior in the suggested model's illness dynamics by using the relevant derivative. To numerical present the results, we use RK2 method.
COVID-19 is a contagious disease that can be easily transmitted from one person to another. At the end of 2019, Wuhan, China, was the site of this terrible infection for the first time. Later, this disease gave rise to a worldwide outbreak, which the World Health Organization (WHO) notified in the first quarter of 2020 [1]. Almost all of the countries of the world have experienced widespread transmission of this virus. The aforementioned sickness has currently claimed the lives of almost 50 billion people. COVID-19 has affected more than 500 million people worldwide. We cite [2,3] for a detailed explanation of how diseases are contracted and spread. Because the pandemic has affected the entire world, every nation has implemented its own policies. Some nations have implemented lockdowns, while others have issued laws requiring strict safety measures like wearing face masks and avoiding large gatherings, etc. The countries most impacted by the disease are Brazil, Italy, India, Iran, Spain, USA and the UK. Earlier research suggested that the virus originated in animals, but later research showed that it may also transfer from person to person [4,5].
Numerous infectious diseases are being investigated for potential treatments, controls, cures, etc. Since epidemiology is an important field of medical science, one of the key subfields from this viewpoint is mathematical biology. In contrast to earlier times, researchers are now becoming increasingly interested in biomathematics and bio-math engineering. In order to give a framework for comprehending the dynamics of diverse infectious diseases, mathematical models have been utilized. The most effective tools for studying different diseases are mathematical models [6,7,8]. We can create numerous predictions, regulating strategies, etc [9,10,11], for a disease in a community by using mathematical models. Considering these factors, researchers have shown a strong interest in the aforementioned subject, we refer the reader to [12,13,14,15]. Many mathematical models have recently been developed by researchers for COVID-19 as well; we provide e.g., see [16,17,18,19]. Major public health difficulties and an economic catastrophe are being faced internationally as a result of the aforementioned disease's recent pandemic status and quick global spread.
In order to theoretically improve existing mathematical models for the aforementioned disease, it is necessary to evaluate the influence of recently developed vaccinations with known high efficacy that have weak transmission-controlling measures. For example, Pearson et al. [20] studied COVID-19 immunization in low as well as middle-income countries. they found it to be very cost-effective, according to recent research [21], if a vaccination with high efficacy and low cost is available. This will have a big impact on disease eradication. Prior to pharmaceutical interventions like treatment and immunization, non-pharmaceutical precautionary measures like the self-quarantining of confirmed cases, isolation, using of face masks, hand washing, social distancing, lockdowns, avoiding gatherings, and closing schools have been employed. This, however, is not a permanent solution. The best strategy will thus be to develop a suitable immunization and make it broadly accessible in every civilization at a fair price. Investigation of COVID-19, various researchers have developed many models. In order to do this, we have aimed to develop a mathematical model with a class of people who have received vaccinations and qualitatively analyze it while considering the therapeutic choices, immunization of those who are susceptible, and healthcare for infected/hospitalized people. Our model takes into account certain important biological and epidemiological elements of the disease under study, such as the disease's inhibitory effect, mortality rates from infections and natural causes, birth rate, and various vaccination rates.
Here, we build the dynamical model for COVID-19. Additionally, we depict the interactions between several model compartments in flow charts in Figure 1. Consequently, we first explain our model as follows:
{dSdt=B−kSI1+βI−(VS+d0+qS)SdEdt=kSI1+βI−(α+d0+qE+vE)EdIdt=αE−(d0+μ+vI+qI)IdQdt=qSS+qEE+qII−(d0+vQ)QdVdt=vSS+vEE+vII+vQQ−d0V. | (1.1) |
Parameters | Physical meaning and representation |
Susceptible compartment | |
Exposed compartment | |
Infected compartment | |
Quarantine compartment | |
Vaccinated compartment | |
Birth rate | |
COVID death rate | |
Natural death rate | |
inhibitory effect rate | |
Saturation constant | |
Infection rate from exposed class | |
Quarantine from susceptible class | |
Quarantine from exposed class | |
Quarantine from infected class | |
Vaccination rate of susceptible | |
Vaccination rate of exposed | |
Vaccination rate of infected | |
Vaccination rate of quarantine |
In Figure 1, the model is presented as a flowchart. Epidemiological models have been examined using difference equations or classical order derivatives. Some references are cited as [22,23]. The aforementioned operators cannot generate the phenomenon's global dynamical behavior because of their local nature [24,25,26,27]. As a result, academics have now taken the majority of models into account when considering fractional order derivatives [28,29,30,31]. The growth of the aforementioned field is attributable to its numerous applications to practical issues, particularly in the fields of mathematical biology and dynamical analysis [32,33,34,35,36]. As far as we are aware, fractional calculus is being used to describe an increasing number of real-world scenarios; we refer the reader to [37,38,39,40,41,42,43]. Additionally, the idea of fractional calculus has been applied in a number of scientific and technological fields [44,45,46]. Because of its global nature and memory-preserving methodology, the theory of fractional calculus has been widely used in the mathematical modeling of numerous diseases for further information, see [47,48,49]. The conventional analytical and numerical approaches have been upgraded to handle situations with fractional order derivatives (see references [50,51]). To examine diverse dynamical issues involving fractional order derivatives, the classical decomposition, perturbation, and transformation methods, for instance, have been expanded (some references are cited as [52,53]). Traditional numerical techniques, such as RKM tools, Euler and Taylor methods, the Adams-Bashforth method, etc., have been upgraded more and more to cope with fractional order problems (see [54,55]). Recently, a novel idea has been identified that describes the crossover behavior of the dynamics, because the states of multiple evolution processes frequently change suddenly. Due to these effects, typical derivatives, whether classical or fractional, cannot be used to appropriately address the relevant multi-phase behaviors. Therefore, scholars [56,57,58,59,60,61] have suggested this idea, which has the potential to appropriately address the aforementioned behavior to a certain extent. As a result, we also attempt at numerical analysis for our studied mathematical model by looking at multi-phase behaviors under various fractional orders by utilizing some numerical technique based on Newton interpolation polynomials. Discussion and a graphic presentation versus some actual facts are offered.
The provided model (1.1) can be stated as follows in the sense of the new derivative:
PWCDφ+0(S)(t)=B−kSI1+βI−(VS+d0+qS)SPWCDφ+0(E)(t)=kSI1+βI−(α+d0+qE+vE)EPWCDφ+0(I)(t)=αE−(d0+μ+vI+qI)IPWCDφ+0(Q)(t)=qSS+qEE+qII−(d0+vQ)QPWCDφ+0(V)(t)=vSS+vEE+vII+vQQ−d0V, | (1.2) |
where PWCD+0 denotes piecewise classical or Caputo derivative with two sub-intervals in [0,T].
For further clarification, we respectively express the left hand sides of (1.2) as follows:
PWCDφ+0(S)(t)={D+0[S(t)], 0<t≤t1,CDφ+0(S)(t)=1Γ(1−φ)∫t2t1(t−η)−φdS(η)dηdη, t1<t≤T,,PWCDφ+0(E)(t)={D+0[E(t)], 0<t≤t1,CDφ+0(E)(t)=1Γ(1−φ)∫t2t1(t−η)−φdE(η)dηdη, t1<t≤T,,PWCDφ+0(I)(t)={D+0[I(t)], 0<t≤t1,CDφ+0(I)(t)=1Γ(1−δ)∫t2t1(t−η)−δdI(η)dηdη, t1<t≤T,,PWCDφ+0(Q)(t)={D+0[Q(t)], 0<t≤t1,CDφ+0(Q)(t)=1Γ(1−δ)∫t2t1(t−η)−δdQ(η)dηdη, t1<t≤T,,PWCDφ+0(V)(t)={D+0[V(t)], 0<t≤t1,CDφ+0(V)(t)=1Γ(1−δ)∫t2t1(t−η)−δdV(η)dη(η)dη, t1<t≤T,, | (1.3) |
where D+0 and CDφ+0 are classical and Caputo derivatives, respectively.
Our manuscript is structured as follows: In the first section, we give introduction. In the Section 2, we give basic results. Stability results are given in the Section 3. Numerical results are given in the Section 4. In the Section 5, the fractional order form of the proposed model is investigated. In the Section 6, we given conclusion.
We recollect some fundamental results of fractional calculus.
Definition 2.1. [43] Let ϖ>0, then, the non-integer order integral of a function S:[0,∞)→R is defined as
Iφ+0S(t)=1Γ(φ)∫t0S(η)(t−η)1−φdη, |
provided that right-hand side exists. Also, derivative in the Caputo sense is defined as
Dφ0+S(t)={1Γ(1−φ)∫t0(t−η)−φS′(η)dη, 0<φ<1,dSdt, φ=1. |
Lemma 2.2. [43] Let h∈L[0,∞), then, the solution of
Dφ0+S(t)=h(t), φ∈(0,1],S(0)=S0 |
is given by
S(t)=S0+1Γ(φ)∫t0h(η)(t−η)1−φdη. |
Here, we recollect the piecewise definitions of the fractional order derivative and integral.
Definition 2.3. [61] If h(t) is a differentiable function, then the definition of the classical, and fractional piecewise derivative is
PWC0Dφth(t)={h′(t), 0<t≤t1,Dφ0+h(t) t1<t≤t2, |
such that PWC0Dφt is the classical derivative for 0<t≤t1 and fractional derivative for t1<t≤t2.
Definition 2.4. [61] Let h be a differentiable function, then, the classical, and fractional order piecewise integration is given as
PWIφ0+h(t)={∫t0h(η)dη, 0<t≤t1,1Γ(φ)∫tt1(t−η)φ−1h(η)d(η) t1<t≤t2, |
such that PFI+0φ(t) denotes the classical integration for 0<t≤t1, and Reimann-Liouville integration for t1<t≤t2.
Lemma 2.5. [61] Consider the problem with the piecewise fractional order derivative
PFC0Dφth(t)=F(t,h(t)), 0<φ≤1, |
whose solution is given by
h(t)={h0+∫t0h(η)dη, 0<t≤t1;h(t1)+1Γ(φ)∫tt1(t−η)φ−1h(η)d(η) t1<t≤t2. |
Here, we define the Taylor series from [59]; consider
Dφtx(t)=F(t,x(t)),x(0)=x0, | (2.1) |
where F:[0,∞)×R→R. The generalized Taylor series is thus given by
x(t+h)=x(t)+hφγ(φ+1)Dφtx(t)+h2φγ(2φ+1)D2φtx(t)+... | (2.2) |
The outcomes of fractional calculus are used here. D2φtx=DφtF(t,x(t))+F(t,x(t))DφxF(t,x(t)). By using (2.2), after rearranging the terms, we obtain the general formula shown below.
x(t+h)=x(t)+hφγ(φ+1)F(t,x(t))+hφ2Γ(φ+1)[K1+K2], | (2.3) |
where
K1=F(ti,xi(ti)), K2=F(ti+2hφΓ(φ+1)Γ(2φ+1), x(ti)+2hφΓ(φ+1)Γ(2φ+1)F(ti,xi(ti))). | (2.4) |
Assume that φ=1; the standard method described by (4.2).
We initially examine our model's feasibility in this part. In order to achieve this, we add each equation in the model (1.1) under the condition that
N(t)=S(t)+E(t)+I(t)+Q(t)+V(t), |
one has
dNdt=B−d0N−μI. | (3.1) |
From (3.1), we have
limt→∞supN≤N0. |
Then, limt→∞supN=N0 if and only if limt→∞supI=0.
In the system (1.1), the first equation yields
0≤limt→∞supS≤S0. |
Similarly, the second equation of the model (1.1) yields
0≤limt→∞supE≤E0. |
From above, we conclude that dNdt<0 if N>N0. Additionally, we have
χ={(S,E,I,Q,V)∈R5+:N≤N0≤Bd0}. | (3.2) |
Disease free equilibrium points: We analyze whether the system (1.1) has an equilibrium point. E0=(S0,0,0,0)=(Bdo,0,0,0) is a representation of the model (1.1) disease-free equilibrium.
S0=BvS+d0+qSQ0=S0d0+vQV0=vSS0+vQQ0d0. |
Endemic equilibrium: From (1.1), lets assume that dSdt=0,dEdt=0,dIdt=0,dQdt=0 and dVdt=0; then, by simple calculation one can find the endemic equilibrium to be as follows:
S∗(t)=(α+d0+qE+vE)(d0+μ+vI+qI)(1+βI∗)αkE∗(t)=(d0+μ+vI+qI)I∗αI∗(t)=αkβ−(vS+d0+qS)(α+d0+qE+vE)(d0+μ+vI+qI)(α+d0+qE+vE)(d0+μ+vI+qI)(k+β(vS+d0+qS))Q∗(t)=qSS∗+qEE∗+qII∗d0+vQV∗(t)=vSS∗+vQQ∗+vII∗d0. |
In epidemiology, the idea of the fundamental reproduction number, or R0, outlines how illnesses spread and are treated. Both the frequency of the illness in the populace and the best countermeasures to protect the neighborhood's residents from the deadly virus are disclosed by R0. The most recent method for determining R0 is as follows. If we set χ=(E,I), we have from the system (1.1) the following
dχdt=H−F, |
where
H=(kSI1+βI0) |
and
F=((α+d0+qE+vE)E−αE(t)+(d0+μ+vI+qI)I). |
The Jacobian of H for the disease-free equilibrium is
H=(0kS000), |
and for the disease-free equilibrium, the Jacobian of F is given by
F=(α+d0+qE+vE0−αd0+μ+qI+vI). |
Hence
F−1=1(α+d0+qE+vE)(d0+μ+vI+qI)(d0+μ+vI+qI0αα+d0+qE+vE.). |
We have
HF−1=1(α+d0+qE+vE)(d0+μ+vI+qI)(αkS0000). |
Hence, the required R0 is given by
R0=kαB(α+d0+vE+qE)(vS+d0+qS)(d0+μ+qI+vI). | (3.3) |
If and only if the reproduction number meets the requirement that R0<1, then we may state that the disease-free equilibrium is stable and the population is disease-free. You can halt the spread of an epidemic by exercising prudence. By sitting the numerical values of the variables above, R0=0.98347. This number shows that the spread of COVID-19 in the locality is well managed.
On the basis of R0, we established the following theorem.
Theorem 3.1. (ⅰ) If R0≤1, then positive equilibria for system (1.1) are not possible.
(ⅱ) If R0>1, then there is an endemic equilibrium, often known as a distinct positive (unique) equilibrium E∗=(S∗,I∗,Q∗,V∗).
Some further results on equilibrium point with global and local stability are presented here for system (1.1).
Theorem 3.2. The disease-free equilibrium E0 is locally asymptotically stable, if R0≤1, and unstable if R0>1.
Proof. The Jacobian matrix at E0 is given below:
M0=[−(vS+d0+qS)0−kBvS+d0+qS000−(α+d0+qE+vE)−kBvS+d0+qS000α−(d0+μ+vI+qI)00qSqEqI−(d0+vQ)00vSvEvIvQ−d0]. |
From a simple straightforward calculation M0 has three eigenvalues which are negative; Λ1=−(vS+d0+qS), Λ2=−(d0+vQ) and Λ3=−d0. The next eigenvalues of M0 are derived from
Λ2+(2d0+α+μ+vI+qI+qE+vE)Λ+(α+d0+qE+vE)(d0+μ+vI+qI)−kαBvS+d0+qS=0. | (3.4) |
If R0>1, then,
(α+d0+qE+vE)(d0+μ+vI+qI)−kαBvS+d0+qS<0, |
which means that (3.4) has two roots, i.e., one positive root and one negative root. Therefore, the disease-free equilibrium E0 has an unstable saddle point. When R0=1, to get the result for the disease-free equilibrium E0 that is globally asymptotically stable, we consider the function called the Lyapunov function.
L(E,I)=αE+(α+d0+qE+vE)I. |
Taking the derivative of L(E,I) with respect to time t gives
dL(E,I)dt=[kαS1+αI−(α+d0+qE+vE)(d0+μ+vI+qI)]I≤[kαS−(α+d0+qE+vE)(d0+μ+vI+qI)]I≤αkS0R0(R0SS0−1)≤0. | (3.5) |
Suppose that I=0 implies that dL(E,I)dt=0. Furthermore, when R0=1, which implies that E0 is globally asymptotically stable in Φ.
In the next theorem, we study the stability at point E∗.
Theorem 3.3. If R0>1, the endemic equilibrium E∗ is globally asymptotically stable.
Proof. For the system (1.1), the Jacobian matrix is
M=[−kI∗1+βI∗−(vS+d0+vS)0kS∗(1+βI∗)200kI∗1+βI∗−(α+d0+qE+vE)kS∗(1+βI∗)2000α(d0+μ+vI+qI)00qSqEqI−(d0+vQ)0vSvEvIvQ−d0]. |
We see that the eigen values of M' i.e., Λ1=−d0 and Λ2=−(d0+vQ) are negative. Also, we have
Λ3+A1Λ2+A2Λ+A3=0, |
where
A1=kI∗1+βI∗+3d0+vS+vE+μ+vI+qI+qE+qS>0A2=(kI∗1+βI∗+vS+d0+qS)(2d0+α+μ+vI+qI+vE+qE)+(α+d0+qE+vE)(d0+μ+vI+qI)−αkS∗(1+βI∗)2>0>(kI∗1+βI∗+vS+d0+qS)(2d0+α+μ+vI+qI+vE+qE)>0A3=(kI∗1+βI∗+vS+d0+qS)[(α+d0+vE+qE)(d0+μ+vI+qI)−αkS∗(1+βI∗)2]+αkI∗(1+βI∗).αkS∗(1+βI∗)2>0. |
Consider the following equation
αkS∗(1+βI∗)=(α+d0+qE+vI)(d0+μ+qI). |
Using the Routh-Hurwitz theorem, we have that A1.A2−A3>0 by a simple direct calculation which shows that E∗ is locally asymptotically stable. This completes the proof.
Using the parameters from Table 2, we conducted the numerical simulations for model (1.1), we describe them in this section. Here, we describe how we simulate our system (1.1) by using the second-order Runge-Kutta (RK2) method as implemented in [64]. For this, we took into consideration
dx(t)dt=Ψ(t,x(t)). | (4.1) |
Parameters | Physical meaning and representation | Numerical value |
S | Susceptible compartment | 222.6 million[65] |
E | Exposed compartment | 120million [65,66,67] |
I | Infected compartment | 1.30[65] |
Q | Quarantine compartment | 1.30 [65] |
V | Vaccinated compartment | 100 millions [66] |
B | Birth rate | 1000059×365 [67] |
μ | COVID death rate | 0.018 [67] |
d0 | Natural death rate | 159×365 [67] |
β | inhibitory effect rate | 0.0701 [65,67] |
k | Saturation constant | 0.00019 [66,67] |
α | Infection rate from exposed class | 0.0833 [65,67] |
qS | Quarantine from susceptible class | 0.0701 [65,66,67] |
qE | Quarantine from exposed class | 0.13 [65,67,68] |
qI | Quarantine from infected class | 0.0701 [67] |
vS | Vaccination rate of susceptible | 0.0001 [65,67] |
vE | Vaccination rate of exposed | 0.4 [65,67] |
vI | Vaccination rate of infected | 0.00001 [65,67] |
vQ | Vaccination rate of quarantine | 0.0999 [65,67] |
Here x=(S,E,I,Q,V). The suggested approach allows us to express the relevant iterative formula as
xi+1=xi+h2Ψ(ti+h2,xi(ti)+K12). | (4.2) |
Here, K1=hF(ti,xi(ti)), also, h=ti+1−ti. We simulated our results by using the numerical approach described in (4.2) and use the numbers from Table 2 in order to evaluate with our built-in model (1.1). We use the results of the numerical simulations to demonstrate our theoretical results. The parameter values and their descriptions for the numerical simulations are listed in Table 2. However, some parameter values that are unknown have been assumed for the sake of illustration.
We applied 300 days of the actual data from Pakistan. The overall population of the nation is approximately equal to 222.60 million, according to the data in [65,67]. Nearly 47 of the nation's population was fully vaccinated during the past nine months, while 57 was partially vaccinated. In order to analyze the dynamics of disease propagation and the impact of the vaccination on its cure, we simulated the outcomes for 300 days as shown in Figures 2–6, respectively by using the numerical approach described by (4.2) of RK2 type. Here, we describe the model (1.1).
Figures 2 through 6 depict the spread of the disease over a 300-day period at the specified speeds. The exposed class and the susceptible class as shown by the actual data that were employed. The infection spread relatively quickly in less than 50 days. As a result, there were also more persons under quarantine. More people had full or partial vaccinations during this time. We can notice that there were more persons who had received vaccinations.
Here, we develop a numerical scheme based on RK2 method for the model (1.2) as expressed in terms of (1.3). We have developed a numerical method for the two sub-intervals of [0,T]. We express the system (1.2) in terms of 1.3 by using (x)=(S,E,I,Q,V) as follows:
PWCDφ+0(S)(t)={D+0[S(t)]=Ψ1(t,(x)), 0<t≤t1,CDφ+0(S)(t)=Ψ1(t,(x)), t1<t≤T,,PWCDφ+0(E)(t)={D+0[E(t)]=Ψ2(t,(x)),, 0<t≤t1,CDφ+0(E)(t)=Ψ2(t,(x)),, t1<t≤T,,PWCDφ+0(I)(t)={D+0[I(t)]=Ψ3(t,(x)),, 0<t≤t1,CDφ+0(I)(t)=Ψ3(t,(x)),, t1<t≤T,,PWCDφ+0(Q)(t)={D+0[Q(t)]=Ψ4(t,(x)),, 0<t≤t1,CDφ+0(Q)(t)=Ψ4(t,(x)),, t1<t≤T,,PWCDφ+0(V)(t)={D+0[V(t)]=Ψ5(t,(x)),, 0<t≤t1,CDφ+0(V)(t)=Ψ5(t,(x)), t1<t≤T. | (5.1) |
We may write the aforesaid system (5.1) by using (4.2) for classical order derivative when 0<t<t1 and (2.3) with the (2.4) for the fractional order Caputo derivative with t1<t<T. Hence, we can write the first equation of the system (5.1) as follows:
S(ti+1))={Si−1(ti−1)+h2Ψ1[ti−1+h2,xi−1(ti−1)+K12], 0<t≤t1Si(ti)+hφγ(φ+1)Ψ1(ti,xi(ti))+hφ2Γ(φ+1)[K2+K3], t1<t≤T, | (5.2) |
where h=ti+1−ti, and
K1=Ψ1(ti−1,xi−1(ti−1)), K2=Ψ1(ti,xi(ti)),K3=Ψ1(ti+2hφΓ(φ+1)Γ(2φ+1),x(ti)+2hφΓ(φ+1)Γ(2φ+1)Ψ1(ti,xi(ti))). | (5.3) |
Similarly, the equations for the other compartments can be written as follows:
E(ti+1))={Ei−1(ti−1)+h2Ψ2[ti−1+h2,xi−1(ti−1)+K12], 0<t≤t1Ei(ti)+hφγ(φ+1)Ψ2(ti,xi(ti))+hφ2Γ(φ+1)[K2+K3], t1<t≤T, | (5.4) |
I(ti+1))={Ii−1(ti−1)+h2Ψ3[ti−1+h2,xi−1(ti−1)+K12], 0<t≤t1Ii(ti)+hφγ(φ+1)Ψ3(ti,xi(ti))+hφ2Γ(φ+1)[K2+K3], t1<t≤T, | (5.5) |
Q(ti+1))={Qi−1(ti−1)+h2Ψ4[ti−1+h2,xi−1(ti−1)+K12], 0<t≤t1Qi(ti)+hφγ(φ+1)Ψ4(ti,xi(ti))+hφ2Γ(φ+1)[K2+K3], t1<t≤T, | (5.6) |
and
V(ti+1))={Vi−1(ti−1)+h2Ψ5[ti−1+h2,xi−1(ti−1)+K12], 0<t≤t1Vi(ti)+hφγ(φ+1)Ψ5(ti,xi(ti))+hφ2Γ(φ+1)[K2+K3], t1<t≤T. | (5.7) |
Here, we plot the approximated results for two sets of fractional orders in two cases.
Case Ⅰ: When 0<φ≤0.6, the corresponding solutions exhibiting crossover behavior were obtained as in Figures 7–11 for different fractional orders.
Case Ⅱ: Now we present the numerical results corresponding to different fractional orders such that 0.6<φ<1 in Figures 12–16, respectively which show the crossover behaviors of different compartments.
Here, we discuss the results of modeling of the piecewise derivative shown in Figures 7–16. We observed a the sudden change after 150 days. So, for various fractional orders in [0,50) and [50,300], we have plotted the numerical findings for systems (1.3) and (5.1). It is possible to see the declines and rises in several compartments with obvious crossover behavior. This novel idea makes a significant contribution to the understanding of how a system that experiences sudden changes in its dynamics behaves. Additionally, the fractional order derivative is a global operator that furthers our comprehension of the phenomenon. In Figure 17, we have compared some real data of infected cases with the simulated results at given fractional orders. Data applied here were taken from Pakistan from January 1, 2021 to January 30, 2021 (see [65]). We see that the simulated results were very similar to the real data which confirm the authentication of the fractional order model that we have considered.
Also, we have compared the simulated data for the vaccinated class for the period of 11 days i.e., March 1, 2022 to March 11, 2022 (see [67]) in Figure 18.
Here, a dynamical system for the COVID-19 disease has been examined. The traditional method for determining the conditions for both local and global stability, as well as for computing R0 at equilibrium locations, is to employ classical analysis. Additionally, the classical model scenario was numerically simulated. We then developed a strong algorithm to carry out the numerical analysis of the suggested model under the conditions of the new concept, extending the RK2 approach to piece-wise derivative applications. Using actual data from Pakistan for the first 300 days by splitting into two sub intervals, we have carried out a number of numerical simulations for various fractional orders. We have found that fractional calculus that involves applying in terms of piecewise derivative is more effective at clearly indicating the rapid changes in various model compartments. Crossover behavior is what we call such behavior. In the aforementioned process, a system experiences a rapid change in its state of dynamics and runs its crossover behavior is terminated. The aforementioned idea can be further refined to encompass more complex dynamical systems with various types of fractional differential operators. Also, in the future, we will combine stochastic type and fractals-fractional differential type operators in piecewise form for various infectious disease models.
The authors declare they have not used artificial intelligence (AI) tools in the creation of this article.
This work was supported and funded by the Deanship of Scientific Research at Imam Mohammad Ibn Saud Islamic University (IMSIU) (Grant Number IMSIU- RG23124).
The authors declare that they have no competing interest.
[1] | Naming the coronavirus disease (COVID-19) and the virus that causes it, Available from: World Health Organization (WHO), 2020, https://www.who.int/emergencies/diseases/novel-coronavirus-2019/technical-guidance/naming-the-coronavirus-disease-(covid-2019)-and-the-virus-that-causes-it. |
[2] |
S. Zhao, S. S. Musa, Q. Lin, J. Ran, G. Yang, W. Wang, et al., Estimating the unreported number of novel coronavirus (2019-nCoV) cases in China in the first half of January 2020, a data-driven modelling analysis of the early outbreak, J. Clin. Med., 9 (2020), 388. https://doi.org/10.3390/jcm9020388 doi: 10.3390/jcm9020388
![]() |
[3] |
I. Nesteruk, Statistics based predictions of coronavirus 2019-nCoV spreading in mainland China, MedRxiv, 4 (2020), 1988–1989. https://doi.org/10.1101/2020.02.12.20021931 doi: 10.1101/2020.02.12.20021931
![]() |
[4] |
D. S. Hui, E. I. Azhar, T. A. Madani, F. Ntoumi, R. Kock, O. Dar, et al., The continuing 2019-nCoV epidemic threat of novel coronaviruses to global health–The latest 2019 novel coronavirus outbreak in Wuhan, China, Int. J. Infect. Dis., 91 (2020), 264–266. https://doi.org/10.1016/j.ijid.2020.01.009 doi: 10.1016/j.ijid.2020.01.009
![]() |
[5] |
S. Zhao, Q. Lin, J. Ran, S. S. Musa, G. Yang, W. Wang, et al., Preliminary estimation of the basic reproduction number of novel coronavirus (2019-nCoV) in China, Int. J. Infect. Dis., 92 (2020), 214–217. https://doi.org/10.1016/j.ijid.2020.01.050 doi: 10.1016/j.ijid.2020.01.050
![]() |
[6] |
K. Shah, R. Din, W. Deebani, P. Kumam, Z. Shah, On nonlinear classical and fractional order dynamical system addressing COVID-19, Results Phys., 24 (2021), 104069. https://doi.org/10.1016/j.rinp.2021.104069 doi: 10.1016/j.rinp.2021.104069
![]() |
[7] |
A. J. Lotka, Contribution to the theory of periodic reactions, J. Phys. Chem., 14 (1910), 271–274. https://doi.org/10.1021/j150111a004 doi: 10.1021/j150111a004
![]() |
[8] |
N. S. Goel, S. C. MAITRA, E. W. MONTROLL, On the Volterra and other nonlinear models of interacting populations, Rev. Mod. Phys., 43 (1971), 231. https://doi.org/10.1103/RevModPhys.43.231 doi: 10.1103/RevModPhys.43.231
![]() |
[9] |
P. Zhou, X. L. Yang, X. G. Wang, B. Hu, L. Zhang, W. Zhang, et al., A pneumonia outbreak associated with a new coronavirus of probable bat origin, Nature, 579 (2020), 270–273. https://doi.org/10.1038/s41586-020-2012-7 doi: 10.1038/s41586-020-2012-7
![]() |
[10] |
Q. Li, X. Guan, P. Wu, X. Wang, L. Zhou, Y. Tong, et al., Early transmission dynamics in Wuhan, China, of novel coronavirus infected pneumonia, N. Engl. J. Med., 382 (2020), 1199–1207. https://doi.org/10.1056/NEJMoa2001316 doi: 10.1056/NEJMoa2001316
![]() |
[11] |
I. I. Bogoch, A. Watts, A. Thomas-Bachli, C. Huber, M. U. G. Kraemer, K. Khan, Pneumonia of unknown aetiology in Wuhan, China: potential for international spread via commercial air travel, J. Travel Med., 27 (2020), taaa008. https://doi.org/10.1093/jtm/taaa008 doi: 10.1093/jtm/taaa008
![]() |
[12] |
C. Lu, H. Liu, D. Zhang, Dynamics and simulations of a second order stochastically perturbed SEIQV epidemic model with saturated incidence rate, Chaos Soliton. Fract., 152 (2021), 111312. https://doi.org/10.1016/j.chaos.2021.111312 doi: 10.1016/j.chaos.2021.111312
![]() |
[13] |
X. Liu, L. Yang, Stability analysis of a SEIQV epidemic model with saturated incidence rate, Nonlinear Anal. Real, 13 (2012), 2671–2679. https://doi.org/10.1016/j.nonrwa.2012.03.010 doi: 10.1016/j.nonrwa.2012.03.010
![]() |
[14] |
A. B. Gumel, S. Ruan, T. Day, J. Watmough, F. Brauer, P. van den Driessche, et al., Modelling strategies for controlling SARS out breaks, Proc. R. Soc. Lond. B, 271 (2004), 2223–2232. https://doi.org/10.1098/rspb.2004.2800 doi: 10.1098/rspb.2004.2800
![]() |
[15] |
A. Atangana, S. I. Araz, Modeling third waves of Covid-19 spread with piecewise differential and integral operators: Turkey, Spain and Czechia, Results Phys., 29 (2021), 104694. https://doi.org/10.1016/j.rinp.2021.104694 doi: 10.1016/j.rinp.2021.104694
![]() |
[16] |
Z. Zhang, A. Zeb, S. Hussain, E. Alzahrani, Dynamics of COVID-19 mathematical model with stochastic perturbation, Adv. Differ. Equ., 2020 (2020), 451. https://doi.org/10.1186/s13662-020-02909-1 doi: 10.1186/s13662-020-02909-1
![]() |
[17] |
A. Atangana, S. I. Araz, Mathematical model of COVID-19 spread in Turkey and South Africa: theory, methods, and applications, Adv. Differ. Equ., 2020 (2020), 659. https://doi.org/10.1186/s13662-020-03095-w doi: 10.1186/s13662-020-03095-w
![]() |
[18] |
N. H. Alharthi, M. B. Jeelani, A Fractional model of COVID-19 in the frame of environmental transformation with caputo fractional derivative, Adv. Appl. Stat., 88 (2023), 225–244. https://doi.org/10.17654/0972361723047 doi: 10.17654/0972361723047
![]() |
[19] |
M. B. Jeelani, Stability and computational analysis of COVID-19 using a higher order galerkin time discretization scheme, Adv. Appl. Stat., 86 (2023), 167–206. https://doi.org/10.17654/0972361723022 doi: 10.17654/0972361723022
![]() |
[20] |
C. A. B. Pearson, F. Bozzani, S. R. Procter, N. G. Davies, M. Huda, H. T. Jensen, et al., COVID-19 vaccination in Sindh Province, Pakistan: A modelling study of health impact and cost-effectiveness, PLoS Med., 18 (2021), e1003815. https://doi.org/10.1371/journal.pmed.1003815 doi: 10.1371/journal.pmed.1003815
![]() |
[21] |
R. P. Curiel, H. G. Ramírez, Vaccination strategies against COVID-19 and the diffusion of anti-vaccination views, Sci. Rep., 11 (2021), 6626. https://doi.org/10.1038/s41598-021-85555-1 doi: 10.1038/s41598-021-85555-1
![]() |
[22] |
J. T. Wu, K. Leung, G. M. Leung, Nowcasting and forecasting the potential domestic and international spread of the 2019-nCoV outbreak originating in Wuhan, China: a modelling study, The Lancet, 395 (2020), 689–697. https://doi.org/10.1016/S0140-6736(20)30260-9 doi: 10.1016/S0140-6736(20)30260-9
![]() |
[23] |
M. S. Arshad, D. Baleanu, M. B. Riaz, M. Abbas, A novel 2-stage fractional Runge-kutta method for a time-fractional logistic growth model, Discrete Dyn. Nat. Soc., 2000 (2000), 1020472. https://doi.org/10.1155/2020/1020472 doi: 10.1155/2020/1020472
![]() |
[24] |
T. Abdeljawad, Q. M. Al-Mdallal, F. Jarad, Fractional logistic models in the frame of fractional operators generated by conformable derivatives, Chaos Soliton. Fract., 119 (2019), 94–101. https://doi.org/10.1016/j.chaos.2018.12.015 doi: 10.1016/j.chaos.2018.12.015
![]() |
[25] |
O. A. Omar, R. A. Elbarkouky, H. M. Ahmed, Fractional stochastic modelling of COVID-19 under wide spread of vaccinations: Egyptian case study, Alex. Eng. J., 61 (2022), 8595–8609. https://doi.org/10.1016/j.aej.2022.02.002 doi: 10.1016/j.aej.2022.02.002
![]() |
[26] |
S. Saha, A. K. Saha, Modeling the dynamics of COVID-19 in the presence of Delta and Omicron variants with vaccination and non-pharmaceutical interventions, Heliyon, 9 (2023), e17900. https://doi.org/10.1016/j.heliyon.2023.e17900 doi: 10.1016/j.heliyon.2023.e17900
![]() |
[27] |
H. M. Ahmed, R. A. Elbarkouky, O. A. M. Omar, M. A. Ragusa, Models for COVID-19 daily confirmed cases in different countries, Mathematics, 9 (2021), 659. https://doi.org/10.3390/math9060659 doi: 10.3390/math9060659
![]() |
[28] |
F. Liu, K. Burrage, Novel techniques in parameter estimition for fractinal dynamical models arising from biological systems, Comput. Math. Appl., 62 (2011), 822–833. https://doi.org/10.1016/j.camwa.2011.03.002 doi: 10.1016/j.camwa.2011.03.002
![]() |
[29] | M. T. Hoang, O. F. Egbelowo, Dynamics of a fractional-order hepatitis b epidemic model and its solutions by nonstandard numerical schemes, In: Mathematical modelling and analysis of infectious diseases, Cham: Springer, 2020,127–153. https://doi.org/10.1007/978-3-030-49896-2_5 |
[30] |
A. Zeb, E. Alzahrani, V. S. Erturk, G. Zaman, Mathematical model for coronavirus disease 2019 (COVID-19) containing isolation class, BioMed Res. Int., 2020 (2020), 3452402. https://doi.org/10.1155/2020/3452402 doi: 10.1155/2020/3452402
![]() |
[31] |
K. Shah, A. Ali, S. Zeb, A. Khan, M. A. Alqudah, T. Abdeljawad, Study of fractional order dynamics of nonlinear mathematical model, Alex. Eng. J., 61 (2022), 11211–11224. https://doi.org/10.1016/j.aej.2022.04.039 doi: 10.1016/j.aej.2022.04.039
![]() |
[32] |
S. Boccaletti, W. Ditto, G. Mindlin, A. Atangana, Modeling and forecasting of epidemic spreading: The case of Covid-19 and beyond, Chaos Soliton. Fract., 135 (2020), 109794. https://doi.org/10.1016/j.chaos.2020.109794 doi: 10.1016/j.chaos.2020.109794
![]() |
[33] |
E. Atangana, A. Atangana, Facemasks simple but powerful weapons to protect against COVID-19 spread: Can they have sides effects, Results Phys., 19 (2020), 103425. https://doi.org/10.1016/j.rinp.2020.103425 doi: 10.1016/j.rinp.2020.103425
![]() |
[34] |
S. T. M. Thabet, M. S. Abdo, K. Shah, T. Abdeljawad, Study of transmission dynamics of COVID-19 mathematical model under ABC fractional order derivative, Results Phys., 19 (2020), 103507. https://doi.org/10.1016/j.rinp.2020.103507 doi: 10.1016/j.rinp.2020.103507
![]() |
[35] |
A. Al Elaiw, F. Hafeez, M. B. Jeelani, M. Awadalla, K. Abuasbeh, Existence and uniqueness results for mixed derivative involving fractional operators, AIMS Mathematics, 8 (2023), 7377–7393. https://doi.org/10.3934/math.2023371 doi: 10.3934/math.2023371
![]() |
[36] |
A. Moumen, R. Shafqat, A. Alsinai, H. Boulares, M. Cancan, M. B. Jeelani, Analysis of fractional stochastic evolution equations by using Hilfer derivative of finite approximate controllability, AIMS Mathematics, 8 (2023), 16094–16114. https://doi.org/10.3934/math.2023821 doi: 10.3934/math.2023821
![]() |
[37] |
J. T. Machado, V. Kiryakova, F. Mainardi, Recent history of fractional calculus, Commun. Nonlinear Sci., 16 (2011), 1140–1153. https://doi.org/10.1016/j.cnsns.2010.05.027 doi: 10.1016/j.cnsns.2010.05.027
![]() |
[38] |
F. C. Meral, T. J. Royston, R. Magin, Fractional calculus in viscoelasticity: an experimental study, Commun. Nonlinear Sci., 15 (2010), 939–945. https://doi.org/10.1016/j.cnsns.2009.05.004 doi: 10.1016/j.cnsns.2009.05.004
![]() |
[39] |
L. M. Richard, Fractional calculus in bioengineering, part 1, Critical Reviews in Biomedical Engineering, 32 (2004), 104. https://doi.org/10.1615/CritRevBiomedEng.v32.i1.10 doi: 10.1615/CritRevBiomedEng.v32.i1.10
![]() |
[40] | M. Dalir, M. Bashour, Applications of fractional calculus, Appl. Math. Sci., 4 (2010), 1021–1032. |
[41] |
A. S. Alnahdi, M. B. Jeelani, H. A. Wahash, M. A. Abdulwasaa, A Detailed Mathematical Analysis of the Vaccination Model for COVID-19, Computer Modeling in Engineering Sciences, 135 (2022), 1315–1343. https://doi.org/10.32604/cmes.2022.023694 doi: 10.32604/cmes.2022.023694
![]() |
[42] | K. Dehingia, M. B. Jeelani, A. Das, Artificial intelligence and machine learning: A smart science approach for cancer control, In: Advances in deep learning for medical image analysis, Boca Raton: CRC Press, 2022. https://doi.org/10.1201/9781003230540 |
[43] |
M. B. Jeelani, A. S. Alnahdi, M. A. Almalahi, M. S. Abdo, H. A. Wahash, N. H. Alharthi, Qualitative analyses of fractional integro-differential equations with a variable order under the Mittag-Leffler power law, J. Funct. Space., 2022 (2022), 6387351. https://doi.org/10.1155/2022/6387351 doi: 10.1155/2022/6387351
![]() |
[44] | R. L. Magin, Fractional calculus in bioengineering: A tool to model complex dynamics, In: Proceedings of the 13th International Carpathian Control Conference (ICCC), High Tatras, Slovakia, 2012,464–469. https://doi.org/10.1109/CarpathianCC.2012.6228688 |
[45] | Y. A. Rossikhin, M. V. Shitikova, Applications of fractional calculus to dynamic problems of linear and nonlinear hereditary mechanics of solids, 50 (1997), 15–67. https://doi.org/10.1115/1.3101682 |
[46] | A. Carpinteri, F. Mainardi, Fractals and fractional calculus in continuum mechanics, Vienna: Springer, 1997. https://doi.org/10.1007/978-3-7091-2664-6 |
[47] |
L. M. Richard, Fractional calculus models of complex dynamics in biological tissues, Comput. Math. Appl., 59 (2010), 1586–1593. https://doi.org/10.1016/j.camwa.2009.08.039 doi: 10.1016/j.camwa.2009.08.039
![]() |
[48] |
M. Shimizu, W. Zhang, Fractional calculus approach to dynamic problems of viscoelastic materials, JSME International Journal Series C Mechanical Systems, Machine Elements and Manufacturing, 42 (1999), 825–837. https://doi.org/10.1299/jsmec.42.825 doi: 10.1299/jsmec.42.825
![]() |
[49] |
F. Mainardi, An historical perspective on fractional calculus in linear viscoelasticity, Fract. Calc. Appl. Anal., 15 (2012), 712–717. https://doi.org/10.2478/s13540-012-0048-6 doi: 10.2478/s13540-012-0048-6
![]() |
[50] |
Z. Dai, Y. Peng, H. A. Mansy, R. H. Sandler, T. J. Royston, A model of lung parenchyma stress relaxation using fractional viscoelasticity, Med. Eng. Phys., 37 (2015), 752–758. https://doi.org/10.1016/j.medengphy.2015.05.003 doi: 10.1016/j.medengphy.2015.05.003
![]() |
[51] |
M. A. Matlob, Y. Jamali, The concepts and applications of fractional order differential calculus in modeling of viscoelastic systems, Critical Reviews in Biomedical Engineering, 47 (2019), 249–276. https://doi.org/10.1615/CritRevBiomedEng.2018028368 doi: 10.1615/CritRevBiomedEng.2018028368
![]() |
[52] |
W. Grzesikiewicz, A. Wakulicz, A. Zbiciak, Non-linear problems of fractional calculus in modeling of mechanical systems, Int. J. Mech. Sci., 70 (2013), 90–98. https://doi.org/10.1016/j.ijmecsci.2013.02.007 doi: 10.1016/j.ijmecsci.2013.02.007
![]() |
[53] |
C. Celauro, C. Fecarotti, A. Pirrotta, A. C. Collop, Experimental validation of a fractional model for creep/recovery testing of asphalt mixtures, Constr. Build. Mater., 36 (2012), 458–466. https://doi.org/10.1016/j.conbuildmat.2012.04.028 doi: 10.1016/j.conbuildmat.2012.04.028
![]() |
[54] |
W. Adel, A. Elsonbaty, A. Aldurayhim, A. El-Mesady, Investigating the dynamics of a novel fractional-order monkeypox epidemic model with optimal control, Alex. Eng. J., 73 (2023), 519–542. https://doi.org/10.1016/j.aej.2023.04.051 doi: 10.1016/j.aej.2023.04.051
![]() |
[55] |
A. El-Mesady, A. Elsonbaty, W. Adel, On nonlinear dynamics of a fractional order monkeypox virus model, Chaos Soliton. Fract., 164 (2022), 112716. https://doi.org/10.1016/j.chaos.2022.112716 doi: 10.1016/j.chaos.2022.112716
![]() |
[56] |
N. Ahmed, A. Elsonbaty, A. Raza, M. Rafiq, W. Adel, Numerical simulation and stability analysis of a novel reaction-diffusion COVID-19 model, Nonlinear Dyn., 106 (2021), 1293–1310. https://doi.org/10.1007/s11071-021-06623-9 doi: 10.1007/s11071-021-06623-9
![]() |
[57] |
A. M. R. Elsonbaty, Z. Sabir, R. Ramaswamy, W. Adel, Dynamical analysis of a novel discrete fractional SITRS model for COVID-19, Fractals, 29 (2021), 2140035. https://doi.org/10.1142/S0218348X21400351 doi: 10.1142/S0218348X21400351
![]() |
[58] |
A. El-Mesady, A. Waleed Adel, A. A. Elsadany, A. Elsonbaty, Stability analysis and optimal control strategies of a fractional-order Monkeypox virus infection model, Phys. Scr., 98 (2023), 095256. https://doi.org/10.1088/1402-4896/acf16f doi: 10.1088/1402-4896/acf16f
![]() |
[59] |
M. M. Khalsaraei, An improvement on the positivity results for 2-stage explicit Runge-Kutta methods, J. Comput. Appl. Math., 235 (2010), 137–143. https://doi.org/10.1016/j.cam.2010.05.020 doi: 10.1016/j.cam.2010.05.020
![]() |
[60] |
Z. J. Fu, Z. C. Tang, H. T. Zhao, P. W. Li, T. Rabczuk, Numerical solutions of the coupled unsteady nonlinear convection-diffusion equations based on generalized finite difference method, Eur. Phys. J. Plus, 134 (2019), 272. https://doi.org/10.1140/epjp/i2019-12786-7 doi: 10.1140/epjp/i2019-12786-7
![]() |
[61] |
A. Atangana, S. I. Araz, New concept in calculus: piecewise differential and integral operators, Chaos Soliton. Fract., 145 (2021), 110638. https://doi.org/10.1016/j.chaos.2020.110638 doi: 10.1016/j.chaos.2020.110638
![]() |
[62] | Current information about COVID-19 in Pakistan, 2021, Available from: https://www.worldometers.info. |
[63] | Pakistan COVID-19 Corona tracker, 2021, Available from: https://www.coronatracker.com/country/pakistan/. |
[64] |
F. Chamchod, N. F. Britton, On the dynamics of a two-strain influenza model with isolation, Math. Model. Nat. Phenom., 7 (2012), 49–61. https://doi.org/10.1051/mmnp/20127305 doi: 10.1051/mmnp/20127305
![]() |
[65] | Pakistan population, Available from: Worldometer, https://www.worldometers.info/world-population/pakistan-population/. |
[66] |
S. Ahmad, A. Ullah, Q. M. Al-Mdallal, H. Khan, K. Shah, A. Khan, Fractional order mathematical modeling of COVID-19 transmission, Chaos Soliton. Fract., 139 (2020), 110256. https://doi.org/10.1016/j.chaos.2020.110256 doi: 10.1016/j.chaos.2020.110256
![]() |
[67] | Vaccines, Available from: UNICEF Pakistan, https://www.unicef.org/pakistan/topics/vaccines |
[68] |
S. Mwalili, M. Kimathi, V. Ojiambo, D. Gathungu, R. Mbogo, SEIR model for COVID-19 dynamics incorporating the environment and social distancing, BMC Res. Notes, 13 (2020), 352. https://doi.org/10.1186/s13104-020-05192-1 doi: 10.1186/s13104-020-05192-1
![]() |
Parameters | Physical meaning and representation |
Susceptible compartment | |
Exposed compartment | |
Infected compartment | |
Quarantine compartment | |
Vaccinated compartment | |
Birth rate | |
COVID death rate | |
Natural death rate | |
inhibitory effect rate | |
Saturation constant | |
Infection rate from exposed class | |
Quarantine from susceptible class | |
Quarantine from exposed class | |
Quarantine from infected class | |
Vaccination rate of susceptible | |
Vaccination rate of exposed | |
Vaccination rate of infected | |
Vaccination rate of quarantine |
Parameters | Physical meaning and representation | Numerical value |
S | Susceptible compartment | 222.6 million[65] |
E | Exposed compartment | 120million [65,66,67] |
I | Infected compartment | 1.30[65] |
Q | Quarantine compartment | 1.30 [65] |
V | Vaccinated compartment | 100 millions [66] |
B | Birth rate | 1000059×365 [67] |
μ | COVID death rate | 0.018 [67] |
d0 | Natural death rate | 159×365 [67] |
β | inhibitory effect rate | 0.0701 [65,67] |
k | Saturation constant | 0.00019 [66,67] |
α | Infection rate from exposed class | 0.0833 [65,67] |
qS | Quarantine from susceptible class | 0.0701 [65,66,67] |
qE | Quarantine from exposed class | 0.13 [65,67,68] |
qI | Quarantine from infected class | 0.0701 [67] |
vS | Vaccination rate of susceptible | 0.0001 [65,67] |
vE | Vaccination rate of exposed | 0.4 [65,67] |
vI | Vaccination rate of infected | 0.00001 [65,67] |
vQ | Vaccination rate of quarantine | 0.0999 [65,67] |
Parameters | Physical meaning and representation |
Susceptible compartment | |
Exposed compartment | |
Infected compartment | |
Quarantine compartment | |
Vaccinated compartment | |
Birth rate | |
COVID death rate | |
Natural death rate | |
inhibitory effect rate | |
Saturation constant | |
Infection rate from exposed class | |
Quarantine from susceptible class | |
Quarantine from exposed class | |
Quarantine from infected class | |
Vaccination rate of susceptible | |
Vaccination rate of exposed | |
Vaccination rate of infected | |
Vaccination rate of quarantine |
Parameters | Physical meaning and representation | Numerical value |
S | Susceptible compartment | 222.6 million[65] |
E | Exposed compartment | 120million [65,66,67] |
I | Infected compartment | 1.30[65] |
Q | Quarantine compartment | 1.30 [65] |
V | Vaccinated compartment | 100 millions [66] |
B | Birth rate | 1000059×365 [67] |
μ | COVID death rate | 0.018 [67] |
d0 | Natural death rate | 159×365 [67] |
β | inhibitory effect rate | 0.0701 [65,67] |
k | Saturation constant | 0.00019 [66,67] |
α | Infection rate from exposed class | 0.0833 [65,67] |
qS | Quarantine from susceptible class | 0.0701 [65,66,67] |
qE | Quarantine from exposed class | 0.13 [65,67,68] |
qI | Quarantine from infected class | 0.0701 [67] |
vS | Vaccination rate of susceptible | 0.0001 [65,67] |
vE | Vaccination rate of exposed | 0.4 [65,67] |
vI | Vaccination rate of infected | 0.00001 [65,67] |
vQ | Vaccination rate of quarantine | 0.0999 [65,67] |