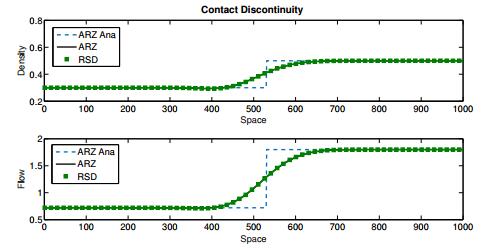
This paper investigates the stability of bifurcating solutions for a prey-predator model with population flux by attractive transition. Applying spectral analysis and the principle of exchange of stability, we obtain that the bifurcating solutions are stable/unstable under some certain conditions.
Citation: Qian Xu, Chunfeng Xing. The stability of bifurcating solutions for a prey-predator model with population flux by attractive transition[J]. AIMS Mathematics, 2021, 6(7): 6948-6960. doi: 10.3934/math.2021407
[1] | Na Min, Hongyang Zhang, Xiaobin Gao, Pengyu Zeng . Impacts of hunting cooperation and prey harvesting in a Leslie-Gower prey-predator system with strong Allee effect. AIMS Mathematics, 2024, 9(12): 34618-34646. doi: 10.3934/math.20241649 |
[2] | Jinting Lin, Changjin Xu, Yiya Xu, Yingyan Zhao, Yicheng Pang, Zixin Liu, Jianwei Shen . Bifurcation and controller design in a 3D delayed predator-prey model. AIMS Mathematics, 2024, 9(12): 33891-33929. doi: 10.3934/math.20241617 |
[3] | Yingyan Zhao, Changjin Xu, Yiya Xu, Jinting Lin, Yicheng Pang, Zixin Liu, Jianwei Shen . Mathematical exploration on control of bifurcation for a 3D predator-prey model with delay. AIMS Mathematics, 2024, 9(11): 29883-29915. doi: 10.3934/math.20241445 |
[4] | Wei Ou, Changjin Xu, Qingyi Cui, Yicheng Pang, Zixin Liu, Jianwei Shen, Muhammad Zafarullah Baber, Muhammad Farman, Shabir Ahmad . Hopf bifurcation exploration and control technique in a predator-prey system incorporating delay. AIMS Mathematics, 2024, 9(1): 1622-1651. doi: 10.3934/math.2024080 |
[5] | Sahabuddin Sarwardi, Hasanur Mollah, Aeshah A. Raezah, Fahad Al Basir . Direction and stability of Hopf bifurcation in an eco-epidemic model with disease in prey and predator gestation delay using Crowley-Martin functional response. AIMS Mathematics, 2024, 9(10): 27930-27954. doi: 10.3934/math.20241356 |
[6] | Vikas Kumar, Nitu Kumari . Controlling chaos in three species food chain model with fear effect. AIMS Mathematics, 2020, 5(2): 828-842. doi: 10.3934/math.2020056 |
[7] | Kwadwo Antwi-Fordjour, Rana D. Parshad, Hannah E. Thompson, Stephanie B. Westaway . Fear-driven extinction and (de)stabilization in a predator-prey model incorporating prey herd behavior and mutual interference. AIMS Mathematics, 2023, 8(2): 3353-3377. doi: 10.3934/math.2023173 |
[8] | Saud Fahad Aldosary, Rizwan Ahmed . Stability and bifurcation analysis of a discrete Leslie predator-prey system via piecewise constant argument method. AIMS Mathematics, 2024, 9(2): 4684-4706. doi: 10.3934/math.2024226 |
[9] | Qinghui Liu, Xin Zhang . Chaos detection in predator-prey dynamics with delayed interactions and Ivlev-type functional response. AIMS Mathematics, 2024, 9(9): 24555-24575. doi: 10.3934/math.20241196 |
[10] | Chenxuan Nie, Dan Jin, Ruizhi Yang . Hopf bifurcation analysis in a delayed diffusive predator-prey system with nonlocal competition and generalist predator. AIMS Mathematics, 2022, 7(7): 13344-13360. doi: 10.3934/math.2022737 |
This paper investigates the stability of bifurcating solutions for a prey-predator model with population flux by attractive transition. Applying spectral analysis and the principle of exchange of stability, we obtain that the bifurcating solutions are stable/unstable under some certain conditions.
Second order traffic models have been investigated since the early 70's (e.g. [27,33]) and refined with the introduction of the Aw-Rascle-Zhang (ARZ) model [3] and are still subject of current research. Traffic models in general range from individual based microscopic to averaged macroscopic considerations, see [9,15,19,32] and the references therein. The motivation for the consideration of second order traffic models is that on the one hand microscopic models may explode for a large number of cars and, on the other hand, first order models are supposed to be dependent on the traffic density only and hence lack of real-world effects. In particular, macroscopic traffic flow models with delay terms have gained special attention recently, see [30,26]. While second order traffic models without delay terms are well understood on a theoretical and analytical level [3,9,15], models of this type including time delays are less considered so far [26], and if considered, the delay is usually rewritten by a Taylor approximation [30,26]. In contrast, the explicit modeling of reaction times via delays is a well-known approach in microscopic traffic modeling, see e.g. [6], and therefore the intention is that the introduction of an explicit delay can make macroscopic models more reasonable. More precisely, we expect similar effects as in microscopic delayed models such as the developing of stop-and-go waves based on the reaction time.
Starting from car-following type models, our aim is therefore to develop and study second order macroscopic models with an explicit dependence on the delay term. For this purpose, we consider the explicit derivation of delayed second order models from delayed microscopic models in three different ways following [4,22,30]. We will end up with a system of equations for the traffic density
∂tρ+∂x(ρv)=0,∂t(ρw)+∂x(ρvw)=0, | (1) |
where
For the numerical simulation of the delayed macroscopic models, we use finite differences methods in a straightforward way and combine the method of lines with solvers for delayed differential equations. We analyze numerically the delayed models and compare the solutions to the classical the ARZ model. A comparison to real data and the corresponding fit of parameters (similar to [14]) is also presented.
This work is organized as follows: In section 2 we rigorously derive three second order macroscopic models based on the delayed microscopic model description according to [4,22,30]. The connection to the classical non-delayed ARZ model is also shown. In section 3, we introduce and discuss numerical methods for the solution of microscopic models with delay and second order delayed traffic models using ideas from [5]. In section 4, we present simulation results for comparisons of the different model level of descriptions and particularly stress on phenomena of the delayed macroscopic models.
In this section we derive macroscopic traffic models from delayed microscopic models of the car-following or follow-the-leader-type using three different approaches, cf. [4,22,30]. The resulting models are hyperbolic delay partial differential equations, where the time delay is explicitly kept and appears as an additional term in the equation for the velocity. As we will see, this new type of models gives rise to potential new dynamical behavior.
The microscopic model under consideration dates back to the 1950s and belongs to the class of car-following models, see e.g. [6]. In particular, we consider a system of ordinary differential equations for the location
˙xi(t)=vi(t)˙vi(t)=C(vi+1(t−T)−vi(t−T))(xi+1(t−T)−xi(t−T))γ+1,i=1,…,N, | (2) |
with
Apparently, the acceleration at time
˙xi(t)=vi(t)˙vi(t)=vrefΔXγ(vi+1(t−T)−vi(t−T))(xi+1(t−T)−xi(t−T))γ+1,i=1,…,N. | (3) |
The microscopic model equations (3) are the staring point for the macroscopic equations. We focus on three approaches, i.e. reverse spatial discretization [4], coarse graining [22], Taylor method [30], to derive the corresponding macroscopic delayed models. All approaches lead to second order traffic models that which mainly differ in the delay term.
The first approach we are interested in is based on a derivation proposed in [4], where the classical ARZ model is derived from (2). Here, we apply the same technique to the delayed equations (3) by identifying the local density
ρi(t):=ΔXxi+1(t)−xi(t). |
We further define
P(τ):={vrefγτ−γifγ>0−vrefln(τ)ifγ=0. |
Then, the new variables
˙τi(t)=˙xi+1(t)−˙xi(t)ΔX=vi+1(t)−vi(t)ΔX˙wi(t)=˙vi(t)+ddtP(τi(t))=vrefΔX(vi+1(t−T)−vi(t−T))τi(t−T)γ+1+P′(τi(t))˙τi(t)=vrefτi(t−T)γ+1(vi+1(t−T)−vi(t−T))ΔX−vrefτi(t)γ+1vi+1(t)−vi(t)ΔX | (4) |
since
∂tτ(x,t)=∂xv(x,t)∂tw(x,t)=vref(∂xv(x,t−T)ρ(x,t−T)γ+1−∂xv(x,t)ρ(x,t)γ+1), | (5) |
where
∂tτ(x,t)=∂xv(x,t),∂tw(x,t)=0. | (6) |
In fact, a direct computation shows that (5) is equivalent to
∂tτ(x,t)=∂xv(x,t)∂tv(x,t)=vref∂xv(x,t−T)ρ(x,t−T)γ+1 | (7) |
applying the definitions of
Let us take a closer look at the right-hand side of the momentum equation (7). Compared to the classical model, we now get the temporal change in speed dependent on the density and the spatial derivative of speed from an earlier time. For the interpretation of
So far, we have considered the Lagrangian macroscopic equations (5) and (7), respectively. In a next step, we transform these equations into Eulerian coordinates by introducing the new variables
∂xˆx=τ,∂xˆt=0,∂tˆx=v,∂tˆt=1, | (8) |
or, exploiting
∂tτ=∂ˆtτ+(∂ˆxτ)v,∂xv=(∂ˆxv)τ,∂tw=∂ˆtw+(∂ˆxw)v. | (9) |
In the following, we write
∂tρ(ˆx,t)+∂ˆx(ρ(ˆx,t)v(ˆx,t))=0∂t(ρ(ˆx,t)w(ˆx,t))+∂ˆx(ρ(ˆx,t)v(ˆx,t)w(ˆx,t))=vref(∂ˆxv(ˆx,t−T)ρ(ˆx,t−T)γ−∂ˆxv(ˆx,t)ρ(ˆx,t)γ) | (10) |
which are obviously closely related to the undelayed ARZ model.
Another approach to derive a delayed macroscopic equation is the so-called coarse-graining (CG) proposed in [12,22]. Here, we focus on [22] and highlight the most important steps. Although the method is different from reverse spatial discretization (RSD) from subsection 2.2, we note that the resulting equations are quite similar.
We start by defining the density
ˆρ(x,t):=∑iδ(xi(t)−x),ˆq(x,t):=∑i˙xi(t)δ(xi(t)−x), | (11) |
where
ρ(x,t):=∫Φ(x−x′,t−t′)ˆρ(x′,t′)dx′dt′,q(x,t):=∫Φ(x−x′,t−t′)ˆq(x′,t′)dx′dt′ | (12) |
by applying the coarse graining envelope function
∂∂tρ(x,t)+∂∂xq(x,t)=0∂∂tq(x,t)=ρ(x,t)⟨¨xi(t′)⟩(x,t)−∂∂x[ρ(x,t)⟨˙x2i(t′)⟩(x,t)] | (13) |
with the bracketed average of a quantity
⟨fi(x′,t′)⟩(x,t)=1ρ(x,t)∫Φ(x−x′,t−t′)∑ifi(x′,t′)δ(xi(t′)−x′)dx′dt′. |
By introducing
ρ(x,t)(∂∂tv(x,t)+v(x,t)∂∂xv(x,t))=ρ(x,t)⟨¨xi(t′)⟩(x,t)−∂∂x(ρ(x,t)Θ(x,t)). | (14) |
The first equation for the density is obviously the same as in (10) and the derivation so far is independent on microscopic dynamics. However, the second equation for the momentum involves a term
Let us first consider the general case of a car-following model of the form
¨xi(t)=B(Δxi,Δ˙xi,˙xi). |
Then coarse graining leads to the approximated momentum equation
∂∂tv(x,t)+v(x,t)∂∂xv(x,t)≈B(⟨Δxi⟩,⟨Δ˙xi⟩,v), |
where we further approximate
⟨Δxi⟩≈ρ−1(x,t)+12ρ(x,t)∂∂xρ−1(x,t)+16ρ2(x,t)∂2∂x2ρ−1(x,t)⟨Δ˙xi⟩≈ρ−1(x,t)∂∂xv(x,t)+12ρ2(x,t)∂2∂x2v(x,t). |
Plugging in and expanding around
B(⟨Δxi⟩,⟨Δ˙xi⟩,v(x,t))≈B(ρ−1(x,t),0,v(x,t))+B1(12ρ(x,t)∂∂xρ−1(x,t))+B2(ρ−1(x,t)∂∂xv(x,t)), |
where
∂tv(x,t)+v(x,t)∂xv(x,t)=B(ρ−1(x,t),0,v(x,t))+B12ρ(x,t)∂xρ−1(x,t)+B2ρ(x,t)∂xv(x,t). | (15) |
We remark that it is also possible to include terms of higher order leading to higher order approximations as well as higher order derivatives. Since we are interested in delayed models driven by (3), we can identify
B(Δxi,Δ˙xi,˙xi)=CΔ˙xi(x,t−T)Δxi(x,t−T)γ+1B1=0,B2=Cρ(x,t−T)γ+1,B3=0. | (16) |
The system of equations is then
∂tτ(x,t)=∂xv(x,t)∂tv(x,t)+v(x,t)∂xv(x,t)=vrefρ(x,t−T)γ∂xv(x,t). | (17) |
In conservative form, we may write the system of equations
∂tρ(x,t)+∂x(ρ(x,t)v(x,t))=0∂t(ρ(x,t)w(x,t))+∂x′(ρ(x,t)v(x,t)w(x,t))=vref[∂xv(x,t)ρ(x,t−T)γ−∂xv(x,t)ρ(x,t)γ] | (18) |
which is (up to the term
For completeness, we also state the Lagrangian representation of (18). If we use the inverse transformation from subsection 2.2 and write the equations dependent on
∂tτ(x,t)=∂xv(x,t)∂tv(x,t)=vrefρ(x,t−T)γ+1∂xv(x,t). | (19) |
The last approach we present is based on a Taylor expansion which was originally introduced in [30]. However, in contrast to the original approach, we derive a second order delayed model instead of a first order model only. Furthermore, we keep the explicit delay while the new model is derived from the microscopic level and do not apply a diffusive approximation.
We start by expanding the delayed variables position
xi(t−T)=xi(t)−T˙xi(t)+O(T2) |
and equivalently
vi(t−T)=vi(t)−T˙vi(t)+O(T2). |
By using the microscopic description (3) we can characterize the following delayed system:
xi(t−T)=xi(t)−Tvi(t)+O(T2)vi(t−T)=vi(t)−T(C(vi+1(t−T)−vi(t−T))(xi+1(t−T)−xi(t−T))γ+1)+O(T2),i=1,…,N. | (20) |
The definition
Δxi(t−T)≈Δxi(t)−TΔvi(t) |
and
Δvi(t−T)≈Δvi(t)−T(CΔvi+1(t−T)(Δxi+1(t−T))γ+1−CΔvi(t−T)(Δxi(t−T))γ+1). |
To derive macroscopic equations, we use again
∂tτ(x,t)=∂xv(x,t), |
where
∂tvi(t)=CΔvi(t−T)Δxi(t−T)γ+1≈C1(Δxi(t)−TΔvi(t))γ+1×(Δvi(t)−T[CΔvi+1(t−T)(Δxi+1(t−T))γ+1−CΔvi(t−T)(Δxi(t−T))γ+1])≈C11ρi(t)γ+1−(γ+1)1ρi(t)γTΔvi(t)×(Δvi(t)−CT(Δvi+1(t−T)(ρi+1(t−T))γ+1−Δvi(t−T)(ρi(t−T))γ+1)). |
For simplicity, we omit the terms
∂tτ(x,t)=∂xv(x,t)∂tv(x,t)=vrefρ(x,t)γ+11−(γ+1)ρ(x,t)T∂xv(x,t)×∂x(v(x,t)−2vrefT(ρ(x,t−T)γ+1∂xv(x,t−T))) | (21) |
in Lagrangian coordinates. However, the interpretation of the right-hand side is now different compared to (7) and (19).
By the use of Taylor expansion, correction terms enter the model. This can be interpreted as a correction towards the fact that drivers do not react immediately but after some delay. These correction terms are, as in the previous models, dependent on the product of density, the spatial derivative of speed and the (current and past) state of the traffic. Note that in the case we ignore the terms depending on
As already seen, the introduction of time delays lead to a right-hand side in the equation for the velocity that includes different states of traffic. This can be interpreted as an anticipation of the change in traffic based on the current and past state. Hence, we are able to model the drivers ability to 'extrapolate' the traffic situation to change his/her driving behavior.
Similar to the microscopic case, the introduction of explicit delays requires the extension of the initial data time span to ensure well-posedness. For all states
We now aim to discuss some key properties of the delayed macroscopic models. First, we show that for vanishing delays the models coincide with the classical ARZ model. In this context, we also refer to the weak solutions of the ARZ model and investigate the changes caused by the delayed case. Second, we comment on the positivity of solutions. Closely related to this discussion is the question of stability. We inverstigate the system of delayed differential equations (DDEs) resulting from discretizing in space and derive properties to ensure stable solutions.
Lemma 3.1. The macroscopic delayed models (7), (19) and (21) converge to the undelayed ARZ model for
Proof. The first equation of all proposed models is mass conservation and has no explicit dependence on the delay
Therefore, the velocity equation needs to be expressed in terms of
∂tw(x,t)=vref(∂xv(x,t−T)ρ(x,t−T)γ+1−∂xv(x,t)ρ(x,t)γ+1) |
and
∂tw(x,t)=vref(∂xv(x,t)ρ(x,t−T)γ+1−∂xv(x,t)ρ(x,t)γ+1), |
respectively. The velocity equation for the model derived by Taylor expansion (21) reads
∂tw(x,t)=vref1ρ(x,t)γ+1−(γ+1)1ρ(x,t)γT∂xv(x,t)×(∂xv(x,t)−2vrefT∂x[ρ(x,t−T)γ+1∂xv(x,t−T)])−vref∂xv(x,t)ρ(x,t)γ+1. |
Then, in the limit
In this section, we discuss the solutions to Riemann problems for the ARZ model (1) and explain how the delayed models fit into this framework. The ARZ model is a system of conservation laws and we have three basic types of weak solutions to the Riemann problem, i.e. contact discontinuities, shocks and rarefaction waves, see [3,15] for an overview. We define
f=(fρfρw)=(ρvρwv). | (22) |
For further investigations, we consider the ARZ model in the form
∂tu+∇f∂xu=0, |
where
λ1=v−ρP(ρ),r1=(1w)λ2=v,r2=(1w+ρP′(ρ)) | (23) |
with
Now, let us focus on the weak solutions to Riemann problems with left state
u(t,x)=u0(x−vlt). | (24) |
For the delayed models, we do not necessarily get the same solution for
For the shock solution, we consider the Rankine Hugoniot condition
f(ul)−f(ur)=s(ul−ur). |
This gives for every state a curve which can be connected to the latter by a shock. The shock solution belongs to the genuine nonlinear eigenvalue. In the ARZ model, a fixed
u(t,x)=u0(x−st). | (25) |
It remains unclear how the delayed models fit into this framework due to the missing definition of a flux function and hence the evaluation of the Rankine Hugoniot condition.
Similar to the shock solution, the rarefaction wave also belongs to the genuine nonlinear eigenvalue
u(t,x)={ulx<λ1(ul)tv(xt)λ1(ul)<x<λ1(ur)urx>λ1(ur)t, | (26) |
where
To illustrate the behavior of approximate solutions to the delayed model, we refer to our numerical study in section 4, in particular figures 5, 6 and 7.
The early proposed second order models, i.e. the Payne-Whitham-model, suffered from the problem that in some Riemann problems the density and velocity could become negative. This was first pointed out by Daganzo [10] and the ARZ model has been developed to overcome this drawback. Unfortunately, the delayed models we have derived, converge certainly to the classical ARZ model, but might lose the positivity of
For the first approach (reverse spatial discretization) we have to analyze equation (7). We investigate the case that we have non-negative initial data and need to ensure that the solution remains non-negative. However, the solution would become negative in the case
vref∂xv(x,t−T)ρ(x,t−T)γ+1<0 |
with
For the coarse-graining approach (19) we have a different situation:
∂tv(x,t)=vrefρ(x,t−T)γ+1∂xv(x,t). |
Since the term
The last approach, the Taylor model (21), would become negative if
vref1ρ(y,t)γ+1−(γ+1)1ρ(x,t)γT∂xv(x,t)(∂xv(x,t)−2vrefT∂x[ρ(x,t−T)γ+1∂xv(x,t−T)]) |
is smaller than
−ρ(x,t)γ+1∂x[ρ(x,t−T)γ+1∂xv(x,t−T)]<0 |
and can conclude that the speed can become negative.
Remark 1. Negative velocities also appear in the microscopic model (2). This is the case when a driver is quite close to the vehicle in front and the driver in front suddenly reduces the velocity within the given delay time, see e.g. [21] where this effect is seen for a similar microscopic model. The non-physical solutions obtained in this way are avoided by the choice of our numerical experiments.
From theory we know the concept of linear stability for DDEs, see [25]. To apply this idea to the PDE-type delayed models we have derived, we need to discretize the equations in space. For the model based on reverse spatial discretization (7), we get for
∂tτ(xi,t)=v(xi+1,t)−v(xi,t)Δx∂tv(xi,t)=vref1τ(xi,t−T)v(xi+1,t−T)−v(xi,t−T)Δx |
exploiting
∂tτ(xi,t)=−v(xi,t)Δx∂tv(xi,t)=vref1τ∗−v(xi,t−T)Δx. |
Inserting the disturbed steady state, we get
∂tη1(xi,t)=−η2(xi,t)Δx∂tη2(xi,t)=vref1τ∗−η2(xi,t−T)Δx. |
Since
η0,2λ2eλ2t=vref1τ∗−η0,2eλ2(t−T)Δx. |
This equation is equivalent to
λ2=vref1τ∗−e−λ2TΔx. |
This type of equation can be solved for
λ2=1TW(−Tvrefτ∗Δx), |
and hence the solution for
η1(t)=−η0,2Δxλ2eλ2t+C. |
This means, we have stability in a linear sense if
First, we have to introduce suitable discretization schemes for the microscopic and macroscopic delayed models. We make use of already well-established numerical methods for undelayed models and discuss how the delay term can be treated within this framework.
The microscopic model (2) is a system of DDEs. The numerical discretization of the delay term can be done with method of steps, cf. [5]. The concept behind this method is to separate the original initial history problem into several initial value problems stated by ordinary differential equations (ODE) and then apply standard schemes for ODEs.
To do so, we need to split our foreseen time interval into sub-intervals of smaller size such that the delay is still included. If we look at the first interval, we have all delayed values given by the initial history since the interval is smaller than the delay. Therefore, we can plug in the history and get a classical ODE for this first interval that needs to be solved numerically. For the second interval, we can plug in the history and the solution of the first interval for the delayed parts and get again an ODE. Iteratively, we use this strategy to solve the problem on the whole time interval. A good interpolation of the solution is necessary since we might need values in later iterations which do not correspond to the fixed time grid points.
We use the Matlab solver dde23 to simulate (2). This solver uses an explicit Runge-Kutta (2, 3) pair combined with the method of steps.
0https://de.mathworks.com/help/matlab/ref/dde23.html
For the comparison with the microscopic model, we use a method-of-lines like approach for the discretization of the delayed macroscopic model. We discretize the model in space to get a system of DDEs and apply a forward finite differences discretization to (7), (19) and (21) in Lagrangian coordinates as follows:
Discretization of (7):
∂tτ(xi,t)=v(xi+1,t)−v(xi,t)Δx∂tv(xi,t)=vrefv(xi+1,t−T)−v(xi,t−T)Δxρ(xi,t−T)γ+1. |
Discretization of (19):
∂tτ(xi,t)=v(xi+1,t)−v(xi,t)Δx∂tv(xi,t)=vrefv(xi+1,t)−v(xi,t)Δxρ(xi,t−T)γ+1. |
Discretization of (21):
∂tτ(xi,t)=v(xi+1,t)−v(xi,t)Δx∂tv(xi,t)=vrefρ(xi,t)γ+11−(γ+1)ρ(x,t)Tv(xi+1,t)−v(xi,t)Δx×(v(xi+1,t)−2vrefTρ(xi+1,t−T)v(xi+2,t−T)−v(xi+1,t−T)ΔxΔx−v(xi,t)+2vrefTρ(xi,t−T)v(xi+1,t−T)−v(xi,t−T)ΔxΔx). |
The resulting system can be solved with the method of steps again using the Matlab solver dde23. All discretization procedures need an initial history function. For simplicity, we choose a constant history function with the value of the states at
We remark that the different numerical schemes are used because they showed to be better suited for the respective equation. Moreover, due to the potential instabilities, we can not decrease
The first numerical experiment we focus on is the numerical comparison between the microscopic model (2) and the different derivations of delayed macroscopic models called reverse spatial discretization (RSD) - eq. (7), coarse-graining (CG) and Taylor expansion (TE). Therefore, we present the following scenario: We consider a street with constant initial density in the macroscopic case which means initially uniformly distributed cars in the microscopic situation, i.e.
We simulate a time interval of 100s and the delay is
We see that the models resolve the vacuum solution well and are close to each other.
If we now zoom in space and consider different values for
5 | 1 | 0.5 | 0.1 | ||
RSD | 0.074 | 0.0759 | 0.0706 | 0.0335 | |
RSD | 0.038 | 0.0337 | 0.027 | 0.0288 | |
CG | 0.0727 | 0.0746 | 0.0692 | 0.0308 | |
CG | 0.036 | 0.0305 | 0.0236 | 0.0288 | |
TE | 0.0651 | 0.0683 | 0.0657 | 0.0486 | |
TE | 0.0223 | 0.0181 | 0.0236 | 0.0288 |
In section 3.2 we have seen that weak solutions to the classical ARZ model are hard to interpret for the delayed models. However, from a numerical point of view, a first idea of weak solutions to the delayed model can be given. Here, we focus on the numerical solutions to the RSD model and make comparisons to the three different types of Riemann solutions, see (24) - (26), on the same grid sizes. The results are given in figures 5, 6 and 7.
In a second experiment, we compare the delayed macroscopic models to the classical ARZ model for a delay of
Due to lemma 3.1, all delayed macroscopic models converge to the classical ARZ model, cf. Figure 8. However, the situation changes for a larger delay, e.g.
The third experiment compares our TE approach (21) to the first order convection-diffusion flow model (TCHS) derived in [30]. Since the TE approach is inspired by the derivation introduced in [30], we aim to point out how the two approaches differ regarding the explicit tracking of the delay, cf. discussion in subsection 2.4.
The model in [30] is given by
∂tρ+∂x(ρV(ρ))=−T∂x((ρV(ρ))2∂xρ) |
with
∂tρ−ρV(ρ)∂xρ+ρ∂x(ρV(ρ))=−Tρ∂x((ρV(ρ))2ρ∂xρ) |
This model is solved by a finite difference based method of lines discretization, where the ODE solver is a forward Euler method.
For our comparison, we consider a street with a smooth initial density profile such that we have a relatively high density in the beginning, i.e.
For the next numerical studies and applications, we introduce an alternative numerical discretization of the macroscopic models based on the Eulerian coordinates representation. This is useful since the scenarios we investigate are on a fixed road segment and so Eulerian coordinates are better suited to tackle the boundary conditions. To set up a suitable numerical scheme, we use known methods for hyperbolic partial differential (PDE) equations coupled to a splitting technique, cf. [23]. This means, considering for example the reverse spatial discretization (RSD), we split the model (10) into the classical ARZ model solved in a first step and an additional delayed part solved in a second step with a forward differences approach. We solve the hyperbolic PDE part with the Lax-Friedrichs method using the index
ρ(xj,t+Δt)=12(ρ(xj+1,t)+ρ(xj−1,t))−Δt2Δx(fρ(ρ(xj+1,t),ρw(xj+1,t))−fρ(ρ(xj−1,t),ρw(xj−1,t)))ρw∗(xj,t)12(ρw(xj+1,t)+ρw(xj−1,t))−Δt2Δx(fρw(ρ(xj+1,t),ρw(xj+1,t))−fρw(ρ(xj−1,t),ρw(xj−1,t)))ρw(xj,t+Δt)=vref×(ρw(xj+1,t−T)ρ(xj+1,t−T)−P(1ρ(xj+1,t−T))−ρw(xj−1,t−T)ρ(xj−1,t−T)+P(1ρ(xj−1,t−T))2Δxρ(xj,t−T)−ρw∗(xj+1,t)ρ(xj+1,t)−P(1ρ(xj+1,t))−ρw∗(xj−1,t)ρ(xj−1,t)+P(1ρ(xj−1,t))2Δxρ(xj,t)), |
where
Since the Lax-Friedrichs method is consistent, stable, monotone and
In the following, we introduce two examples to emphasize the impact of delayed macroscopic models in the case of real-world applications.
The first application we have in mind is a frequent traffic light switching. We intend to compare the model (10) to the classical ARZ model (1). The experimental setting is introduced in Figure 11, where at the end of the street, i.e. at
Initially, the density and the velocity are
We see that the presence of the delay term leads to phenomena which can not be observed in the classical ARZ model as for example the broader density plateaus to resolve the stop-and-go behavior. The difference of the two models can be also recognized for the speed
As a second application, we analyze the performance of the models regarding real-measured data. Therefore, we fit the classical (1) and delayed (10) model to real-life data and compare the numerical results.
We start to investigate the fundamental diagram, i.e. the relation between traffic density and traffic flow, for real data taken from the Minnesota Department of Transportation. The data shows a reverse lambda shape, see Figure 15. At low densities, there is a clear linear relation between flow and density. At medium densities however, we get a wide spread of flows, making a functional relation hard or even impossible to identify. We remark that in the classical ARZ model (1) the artificial variable
0http://data.dot.state.mn.us/datatools/
In a next step, we follow the approach in [14] to fit the classical and RSD model to this data. We use data collected by the Regional Transportation Management Center, a division of the Minnesota Department of Transportation (RTMC data), in the same way as described in [14] and approximate the relation between density
Q(ρ)=α[√1+(λp)2+(√1+(λ(1−p))2−√1+(λp)2)ρρmax−√1+λ2(ρρmax−p)2] |
and the resulting solution is plotted in Figure 15.
From
∂t1ρ(x,t)=∂xv(x,t)∂tv(x,t)=ρ(x,t−T)2P′(ρ(x,t−T))∂xv(x,t−T). | (27) |
Now, we test the data-fitted model (27) with RTMC data to check the performance. We follow again [14] and take a segment of street with no on- or off-ramps and three measurement stations. The first and the third station deliver the boundary values for our simulation, while the station in the middle is the reference point, i.e. we will compare the simulation results with the measured data of the second station. So we simulate the segment of road, use the real data as boundary values and get a simulated traffic profile. This profile we compare at the point of the second station with the real data. For a time interval of 600 seconds, i.e. 16:00-16:10 on a workday, the results are plotted in Figure 16 for the fitted delayed and undelayed ARZ model. The images represent the whole time sequence at the reference station in the middle of the segment.
It seems that the undelayed ARZ model tends to avoid deviations while the delayed model has stronger peaks. Beyond that, both models seem to fit the flow better than the density, but this might be due to the specific example. For further discussions on the data-fitted classical ARZ model, which might be useful for this kind of experiment, we refer to [14] and [13].
Instead, let us look at the error between the real data and the simulated the models. We therefore make use of the error defined in [14] which is
E(x,t)=|ρmodel(x,t)−ρdata(x,t)|Δρ+|vmodel(x,t)−vdata(x,t)|Δv |
for every position
E=1T∫T0E(x,t)dt. |
The normalization factors
ϵ=4Eundel−Edel(Eundel+Edel)2 |
where
Summarizing, we have derived a new type of macroscopic second order traffic models including a delay from a microscopic follow-the-leader model in three different ways. The proposed approaches are adapted from well-established models known for the derivation of undelayed macroscopic traffic flow models, i.e. reverse spatial discretization, coarse graining and Taylor expansion. We have also suggested different methods to numerically tackle the resulting equations. The numerical results show that the delayed macroscopic equations lead to reasonable results, in particular in comparison with the classical ARZ model.
Future work includes further theoretical investigations for the delayed PDEs that have been already established for related problems, see for example [1,2] and [31]. Furthermore, deeper investigations on suitable numerical methods for this kind of delayed equations are necessary. From an application point of view, an extension to traffic networks would be interesting.
[1] |
K. Oeda, K. Kuto, Positive steady states for a prey-predator model with population flux by attractive transition, Nonlinear Anal.: Real World Appl., 44 (2018), 589–615. doi: 10.1016/j.nonrwa.2018.06.006
![]() |
[2] | A. Okubo, S. A. Levin, Diffusion and Ecological Problems: Modern Perspectives, New York: Springer-Verlag, 2001. |
[3] |
L. Li, Coexistence theorems of steady states for predator-prey interacting system, Trans. Am. Math. Soc., 305 (1988), 143–166. doi: 10.1090/S0002-9947-1988-0920151-1
![]() |
[4] |
L. Li, On positive solutions of a nonlinear equilibrium boundary value problem, J. Math. Anal. Appl., 138 (1989), 537–549. doi: 10.1016/0022-247X(89)90308-9
![]() |
[5] | J. López-Gómez, Nonlinear eigenvalues and global bifurcation to the search of positive solutions for general Lotka-Volterra reaction diffusion systems with two species, Differ. Integr. Equations, 7 (1994), 1427–1452. |
[6] |
J. López-Gómez, R. Pardo, Coexistence regions in Lotka-Volterra models with diffusion, Nonlinear Anal., 19 (1992), 11–28. doi: 10.1016/0362-546X(92)90027-C
![]() |
[7] |
Y. Yamada, Stability of steady states for prey-predator diffusion equations with homogeneous Dirichlet conditions, SIAM J. Math. Anal., 21 (1990), 327–345. doi: 10.1137/0521018
![]() |
[8] |
T. Kadota, K. Kuto, Positive steady-states for a prey-predator model with some nonlinear diffusion terms, J. Math. Anal. Appl., 323 (2006), 1387–1401. doi: 10.1016/j.jmaa.2005.11.065
![]() |
[9] | K. Oeda, K. Kuto, Characterization of coexistence states for a prey-predator model with large population flux by attractive transition, preprint. |
[10] |
Q. Xu, Y. Guo, The existence and stability of steady states for a prey-predator system with cross diffusion of quasilinear fractional type, Acta Math. Appl. Sin. Engl. Ser., 30 (2014), 257–270. doi: 10.1007/s10255-014-0281-3
![]() |
[11] |
K. Kuto, Bifurcation branch of stationary solutions for a Lotka-Volterra cross-diffusion system in a spatially heterogeneous environment, Nonlinear Anal.: Real World Appl., 10 (2009), 943–965. doi: 10.1016/j.nonrwa.2007.11.015
![]() |
[12] |
S. Djilali, Pattern formation of a diffusive predator-prey model with herd behavior and nonlocal prey competition, Math. Methods Appl. Sci., 43 (2020), 2233–2250. doi: 10.1002/mma.6036
![]() |
[13] |
S. Djilali, Herd behavior in a predator-prey model with spatial diffusion: Bifurcation analysis and Turing instability, J. Appl. Math. Comput., 58 (2018), 125–149. doi: 10.1007/s12190-017-1137-9
![]() |
[14] |
S. Djilali, S. Bentout, Spatiotemporal patterns in a diffusive predator-prey model with prey social behavior, Acta Appl. Math., 169 (2020), 125–143. doi: 10.1007/s10440-019-00291-z
![]() |
[15] | H. Kielhöfer, Bifurcation Theory: An Introduction with Applications to PDEs, Springer, 2004. |
[16] |
J. P. Shi, Persistence and bifurcation of degenerate solutions, J. Funct. Anal., 169 (1999), 494–531. doi: 10.1006/jfan.1999.3483
![]() |
[17] |
J. Blat, K. J. Brown, Global bifurcation of positive solutions in some systems of elliptic equations, SIAM J. Math. Anal., 17 (1986), 1339–1353. doi: 10.1137/0517094
![]() |
1. | Nishith Mohan, Seshadev Padhi, Global bifurcation in a diffusive Beddington-DeAngelis predator–prey model with population flux by attractive transition, 2024, 99, 0031-8949, 075221, 10.1088/1402-4896/ad4fee |
5 | 1 | 0.5 | 0.1 | ||
RSD | 0.074 | 0.0759 | 0.0706 | 0.0335 | |
RSD | 0.038 | 0.0337 | 0.027 | 0.0288 | |
CG | 0.0727 | 0.0746 | 0.0692 | 0.0308 | |
CG | 0.036 | 0.0305 | 0.0236 | 0.0288 | |
TE | 0.0651 | 0.0683 | 0.0657 | 0.0486 | |
TE | 0.0223 | 0.0181 | 0.0236 | 0.0288 |