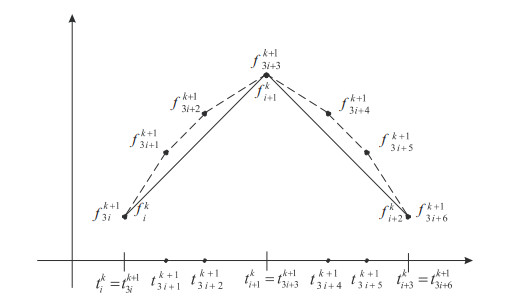
The growth of the Internet of Things makes it possible to share information on risky vehicles openly and freely. How to create dynamic knowledge graphs of continually changing risky vehicles has emerged as a crucial technology for identifying risky vehicles, as well as a research hotspot in both artificial intelligence and field knowledge graphs. The node information of the risky vehicle knowledge graph is not rich, and the graph structure plays a major role in its dynamic changes. The paper presents a fusion algorithm based on relational graph convolutional network (R-GCN) and Long Short-Term Memory (LSTM) to build the dynamic knowledge graph of risky vehicles and conducts a comparative experiment on the link prediction task. The results showed that the fusion algorithm based on R-GCN and LSTM had better performance than the other methods such as GCN, DynGEM, ROLAND, and RE-GCN, with the MAP value of 0.2746 and the MRR value of 0.1075. To further verify the proposed algorithm, classification experiments are carried out on the risky vehicle dataset. Accuracy, precision, recall, and F-values were used as heat-tolerance evaluation indexes in classification experiments, the values were 0.667, 0.034, 0.422, and 0.52 respectively.
Citation: Yongmei Zhang, Zhirong Du, Lei Hu. A construction method of urban road risky vehicles based on dynamic knowledge graph[J]. Electronic Research Archive, 2023, 31(7): 3776-3790. doi: 10.3934/era.2023192
[1] | Shahida Bashir, Rabia Mazhar, Bander Almutairi, Nauman Riaz Chaudhry . A novel approach to study ternary semihypergroups in terms of prime soft hyperideals. AIMS Mathematics, 2023, 8(9): 20269-20282. doi: 10.3934/math.20231033 |
[2] | Shahida Bashir, Ahmad N. Al-Kenani, Maria Arif, Rabia Mazhar . A new method to evaluate regular ternary semigroups in multi-polar fuzzy environment. AIMS Mathematics, 2022, 7(7): 12241-12263. doi: 10.3934/math.2022680 |
[3] | Moquddsa Zahra, Dina Abuzaid, Ghulam Farid, Kamsing Nonlaopon . On Hadamard inequalities for refined convex functions via strictly monotone functions. AIMS Mathematics, 2022, 7(11): 20043-20057. doi: 10.3934/math.20221096 |
[4] | Adnan, Waseem Abbas, Sayed M. Eldin, Mutasem Z. Bani-Fwaz . Numerical investigation of non-transient comparative heat transport mechanism in ternary nanofluid under various physical constraints. AIMS Mathematics, 2023, 8(7): 15932-15949. doi: 10.3934/math.2023813 |
[5] | Tao Yan, Ghulam Farid, Sidra Bibi, Kamsing Nonlaopon . On Caputo fractional derivative inequalities by using strongly $ (\alpha, h-m) $-convexity. AIMS Mathematics, 2022, 7(6): 10165-10179. doi: 10.3934/math.2022565 |
[6] | Tareq Saeed, Muhammad Adil Khan, Hidayat Ullah . Refinements of Jensen's inequality and applications. AIMS Mathematics, 2022, 7(4): 5328-5346. doi: 10.3934/math.2022297 |
[7] | Yang Pan, Yan Liu . New classes of few-weight ternary codes from simplicial complexes. AIMS Mathematics, 2022, 7(3): 4315-4325. doi: 10.3934/math.2022239 |
[8] | M. Zakarya, Ghada AlNemer, A. I. Saied, H. M. Rezk . Novel generalized inequalities involving a general Hardy operator with multiple variables and general kernels on time scales. AIMS Mathematics, 2024, 9(8): 21414-21432. doi: 10.3934/math.20241040 |
[9] | Ling Zhu, Zhengjie Sun . Refinements of Huygens- and Wilker- type inequalities. AIMS Mathematics, 2020, 5(4): 2967-2978. doi: 10.3934/math.2020191 |
[10] | Muhammad Adil Khan, Josip Pečarić, Yu-Ming Chu . Refinements of Jensen’s and McShane’s inequalities with applications. AIMS Mathematics, 2020, 5(5): 4931-4945. doi: 10.3934/math.2020315 |
The growth of the Internet of Things makes it possible to share information on risky vehicles openly and freely. How to create dynamic knowledge graphs of continually changing risky vehicles has emerged as a crucial technology for identifying risky vehicles, as well as a research hotspot in both artificial intelligence and field knowledge graphs. The node information of the risky vehicle knowledge graph is not rich, and the graph structure plays a major role in its dynamic changes. The paper presents a fusion algorithm based on relational graph convolutional network (R-GCN) and Long Short-Term Memory (LSTM) to build the dynamic knowledge graph of risky vehicles and conducts a comparative experiment on the link prediction task. The results showed that the fusion algorithm based on R-GCN and LSTM had better performance than the other methods such as GCN, DynGEM, ROLAND, and RE-GCN, with the MAP value of 0.2746 and the MRR value of 0.1075. To further verify the proposed algorithm, classification experiments are carried out on the risky vehicle dataset. Accuracy, precision, recall, and F-values were used as heat-tolerance evaluation indexes in classification experiments, the values were 0.667, 0.034, 0.422, and 0.52 respectively.
The refinement schemes can be viewed as a class of iterative algorithms. The main feature of these schemes is that they use initially the rough sketch of any curve called polygon to finally produce smooth sketch also called refined sketch. Initially, a refinement scheme was introduced by de Rahm [3] in 1956. Later on, Chaikin [1] introduced the scheme for curve generation in 1974. Deslauriers and Dubuc presented the interpolating schemes for curve modeling in 1989. This triggered off the modern era for the investigation of the refinement schemes. These refinement schemes have been used for curve and surface modeling for a long time by designers, engineers, computer scientists and mathematicians. The details of these applications are presented by Farin [7].
Initially, the binary refinement schemes were introduced. These schemes have two rules to refine each edge of the polygon. These rules are same for each edge of the polygon except the initial and final edges. These rules are classified as even and odd rules. These schemes take initial sketch as an input and return the refined sketch as an output. In the next step, these schemes take previously refined sketch as an input and produce more refined sketch. Repeated application of this procedure give the smooth curve/shape. Nowadays, there are many generalizations of the binary schemes. The class of ternary refinement schemes is one of the generalization of the class of binary schemes. In this class of the schemes, three rules are used instead of two rules to refine each edge of the polygon.
The analysis of the schemes is the crucial issue. Initially, Dyn et al. [4], introduced the divided difference (DD) technique to analyze the schemes in 1987. Later on, Dyn et al. [5], extended this technique for the analysis of the class of binary schemes in 1991. The study of the limiting curves produced by the binary refinement schemes was also presented by Micchelli and Prautzch [11] in 1989. Sabin [15] in 2010, gave further insight in this technique. The further extension of Dyn et al.'s work was done by Mustafa and Zahid [12] in 2013. The cross-differences of directional DD techniques was introduced by Qu [14] in 1991. The further generalization of the DD technique has not been done so for.
Another technique for the smoothness analysis of the schemes was introduced by Dyn [6] in 2002. This technique is known as Laurent polynomial technique. This technique was used by Hassan and Dodgson [8], Hassan et al. [9], Mustafa et al. [13]. Khan and Mustafa [10], Siddiqi and Rehan [16], Zheng et al. [17] and many others to analyze their binary and ternary refinement schemes. Nowadays many authors are also using this technique. However, this technique also has some limitations and need improvement. In this technique, first a sequence of coefficients used in the refinement rules of the schemes is converted into the polynomial. Secondly, the polynomial has been factorized. In this technique, multiplication, division, factorization of the polynomials are involved. Further, the computation of inequalities and the comparison of the terms are also involved. The technique, we are going to introduce is the generalization of DD technique. In this technique, the computation of inequalities and the comparison of three terms are involved. There are three simple algebraic expressions in each inequality. Further these algebraic expressions contain only the coefficients used in the refinement rules of the schemes.
The rest of this paper is structured as follows. In Section 2, a general ternary refinement scheme and its DD schemes are introduced. Their convergence is also presented in this section. In Section 3, the deviation between successive levels of polygons produced by the ternary and its DD refinement schemes are presented. The inequalities for the analysis of ternary schemes are presented in Section 4. Applications of these inequalities are also presented in this section. Summary of the work and comparison are presented in Sections 5 and 6 respectively.
We first introduce the ternary and its first, second and third order divided differences (DD) refinement schemes. Then we present their convergence in this section.
Let fk={(i/3k,fki)∈RN, i∈Z, N≥2, k≥0}, be a polygon at kth refinement level then the (k+1)th polygon fk+1, can be obtained by the following three refinement rules
{fk+13i=m∑j=0ρjfki+j,fk+13i+1=m∑j=0ϱjfki+j,fk+13i+2=m∑j=0σjfki+j. | (2.1) |
whereas m>0 while if tki=i/3k then (tk+13i=tki, fk+13i), (tk+13i+1=13(2tki+tki+1), fk+13i+1)
and (tk+13i+2=13(tki+2tki+1), fk+13i+2) are the points of the polygon fk+1.
The above rules are the affine combinations of fki's so
m∑ν=0ρν=m∑ν=0ϱν=m∑ν=0σν=1. | (2.2) |
The one step of the refinement procedure is shown in Figure 1. The repeated application of these rules is known as ternary refinement scheme.
The first, second and third order divided difference (DD) ternary refinement schemes (TRS) are presented in this section.
Lemma 2.1. Let fk={(i/3k,fki)} be a polygon of TRS at kth refinement level and d[1]kj=3k(fkj+1−fkj) be the first divided difference then the first order DD TRS is defined as
{d[1](k+1)3i=3m−1∑μ=0{μ∑ν=0(ρμ−ν−ϱμ−ν)d[1]ki+μ},d[1](k+1)3i+1=3m−1∑μ=0{μ∑ν=0(ϱμ−ν−σμ−ν)d[1]ki+μ},d[1](k+1)3i+2=3m∑μ=0{μ∑ν=0(σμ−ν−ρμ−ν−1)d[1]ki+μ}. | (2.3) |
Proof. Since the first order DD is defined as
d[1]kν=3k(fkν+1−fkν), | (2.4) |
therefore by (2.1), we get
d[1](k+1)3i=3k+1{(ϱ0−ρ0)fki+(ϱ1−ρ1)fki+1+(ϱ2−ρ2)fki+2+…+(ϱm−1−ρm−1)fki+m−1+(ϱm−ρm)fki+m}. | (2.5) |
Now consider the linear combination
d[1](k+1)3i=y0d[1]ki+y1d[1]ki+1+y2d[1]ki+2+…+ym−1d[1]ki+(m−1). | (2.6) |
The goal is to find the unknown y0,⋯,ym−1. It can be written as
d[1](k+1)3i=3k{−y0fki+(y0−y1)fki+1+(y1−y2)fki+2+…+(ym−3−ym−2)fkm−2+(ym−2−ym−1)fki+(m−1)+ym−1fki+m}. | (2.7) |
Comparing (2.5) and (2.7), we get
y0=3(ρ0−ϱ0),y1=3(ρ0−ϱ0)+3(ρ1−ϱ1),y2=3(ρ0−ϱ0)+3(ρ1−ϱ1)+3(ρ2−ϱ2),⋮ym−1=3(ρ0−ϱ0)+3(ρ1−ϱ1)+⋯+3(ρm−1−ϱm−1). |
Substituting in (2.6), we get
d[1](k+1)3i=3[(ρ0−ϱ0)d[1]ki+{(ρ0−ϱ0)+(ρ1−ϱ1)}d[1]ki+1+{(ρ0−ϱ0)+(ρ1−ϱ1)+(ρ2−ϱ2)}d[1]ki+2+…+{(ρ0−ϱ0)+(ρ1−ϱ1)+…+(ρm−1−ϱm−1)}d[1]ki+(m−1)]. |
This implies
d[1](k+1)3i=3m−1∑μ=0{μ∑ν=0(ρμ−ν−ϱμ−ν)d[1]ki+μ}. |
Similarly, we get
d[1](k+1)3i+1=3m−1∑μ=0{μ∑ν=0(ϱμ−ν−σμ−ν)d[1]ki+μ}, |
and
d[1](k+1)3i+2=3m∑μ=0{μ∑ν=0(σμ−ν−ρμ−ν−1)d[1]ki+μ}. |
This completes the proof. By adopting the similar procedure, we get the following results.
Lemma 2.2. Let fk={(i/3k,fki)} be a polygon of TRS at kth refinement level and
d[2]kj=32k(2!)−1(fkj−1−2fkj+fkj+1) |
be the second order divided difference then the second order DD TRS is defined as
{d[2](k+1)3i=32m−1∑μ=0[μ∑ν=0{(ν+1)σμ−ν+νϱμ−ν−2νρμ−ν}d[2]ki+μ],d[2](k+1)3i+1=32m−2∑μ=0[μ∑ν=0{(ν+1)(ρμ−ν+σμ−ν−2ϱμ−ν)}d[2]ki+μ+1],d[2](k+1)3i+2=32m−1∑μ=0[μ∑ν=0{νρμ−ν+(ν+1)ϱμ−ν−(2ν+2)σμ−ν}d[2]ki+μ+1]. | (2.8) |
Lemma 2.3. Let fk={(i/3k,fki)} be a polygon of TRS at kth refinement level and
d[3]k)j=33k(3!)−1(−fkj−1+3fkj−3fkj+1+fkj+2) |
be the third order divided difference then the third order DD TRS is defined as
{d[3](k+1)3i=33m−2∑μ=0[μ∑ν=0{(ν+1)σμ−ν−3ν(ν+1)2(ρμ−ν−ϱμ−ν)}d[3]ki+μ],d[3](k+1)3i+1=33m−2∑μ=0[μ∑ν=0{(ν+1)ρμ−ν−3(ν+1)(ν+2)2(ϱμ−ν−σμ−ν)}d[3]ki+μ+1],d[3](k+1)3i+2=33m−2∑μ=0[μ∑ν=0{3ν(ν+1)2ρμ−ν+(ν+1)ϱμ−ν−3(ν+1)(ν+2)2σμ−ν}d[3]ki+μ+1]. | (2.9) |
The convergence of first, second and third order divided difference (DD) ternary refinement schemes (TRS) is presented in this section. Throughout the paper, π[0,n] denotes the set of continuous functions on the closed and bounded interval [0,n].
Lemma 2.4. Let fk={(i/3k,fki)} be a polygon of TRS at kth refinement level and d[1]k={(i/3k,d[1]ki)} be a polygon of first order DD scheme. If limk→∞d[1]k=d[1]∈π[0,n], and f is the limiting curve/shape produced by TRS then d[1]=f′.
Proof. Bernstein polynomial for x∈[0,n] with data values fki is
Bk(x)=s∑i=0(si)(xn)i(1−xn)s−ifki. |
Its first derivative is
B′k(x)=s∑i=0(si)in(xn)i−1(1−xn)s−ifki+s∑i=0(si)(xn)i(s−i)(−1n)(1−xn)s−i−1fki. | (2.10) |
This implies
B′k(x)=sns−1∑i=0(s−1i)(xn)i(1−xn)s−i−1(fki+1−fki). |
For s=3kn, we get
B′k(x)=s−1∑i=0(s−1i)(xn)i(1−xn)s−i−13k(fki+1−fki). |
This again implies
B′k(x)=s−1∑i=0(s−1i)(xn)i(1−xn)s−i−1d[1]ki. |
Since the Bernstien polynomials are uniformly convergent therefore limk→∞Bk=f and limk→∞B′k=d[1] on [0,n]. So f′=d[1]∈π[0,n]. Hence the proof.
Lemma 2.5. Let fk={(i/3k,fki)} and d[2]k={(i/3k,d[2]ki)} be the polygons of TRS and its second order DD schemes respectively. If limk→∞d[2]k=d[2]∈π[0,n], and f is the limiting curve produced by TRS then d[2]=f″.
Proof. Take the derivative of (2.10)
B″k(x)=1n2{s(s−1)s∑i=2(s−2)!(i−2)!(s−i)! (xn)i−2(1−xn)s−ifki−2s(s−1)s−1∑i=1(s−2)!(i−1)!(s−i−1)! (xn)i−1(1−xn)s−i−1fki +s(s−1)s−2∑i=0(s−2)!i!(s−i−2)!(xn)i(1−xn)s−i−2fki}. | (2.11) |
This implies
B″k(x)=s(s−1)n2{s−2∑i=0(s−2)!(i)!(s−i−2)! (xn)i(1−xn)s−i−2×(fki+2−2fi+1+fki)}. |
Since s=3kn therefore s(s−1)n2=32k2−1, so we get
B″k(x)=32k2−1{s−2∑i=0(s−2)!(i)!(s−i−2)! (xn)i(1−xn)s−i−2×(fki+2−2fi+1+fki)}. |
This implies
B″k(x)=s−2∑i=0{(s−2)!(i)!(s−i−2)! (xn)i(1−xn)s−i−2}d[2]ki+1, |
where d[2]ki+1 = 32k(2!)−1(fki+2−2fki+1+fki).
Again the uniform convergence of the Bernstien polynomials implies limk→∞B″k=d[2] and limk→∞Bk=f. Therefore when k→∞ then d[2] converges uniformly to f″ on [0,n]. This concludes d[2]=f″∈π[0,n]. Hence the proof.
Lemma 2.6. Let fk={(i/3k,fki)} and d[3]k)={(i/3k,d[3]ki)} be the polygons of TRS and its third order DD schemes respectively. If limk→∞d[3]k=d[3]∈π[0,n] then d[3]=f‴.
Proof. Now take the derivative of (2.11), then
B‴k(x)=1n3s∑i=3s(s−1)(s−2)(s−3)!(i−3)!(s−i)!(xn)i−3(1−xn)s−ifki−3n3s−1∑i=2s(s−1)(s−2)(s−3)!(i−2)!(s−i−1)!(xn)i−2(1−xn)s−i−1fki+3n3s−2∑i=1s(s−1)(s−2)(s−3)!(i−1)!(s−i−2)!(xn)i−1(1−xn)s−i−2fki−1n3s−3∑i=0s(s−1)(s−2)(s−3)!i!(s−i−3)!(xn)i(1−xn)s−i−3fki. |
Since, s=3kn, then
B‴k(x)=33k(3!)−1s−3∑i=0(s−3i)(xn)i(1−xn)s−i−3×(−fki+3fki+1−3fki+2+fki+3). |
This implies
B‴k(x)=s−3∑i=0(s−3i)(xn)i(1−xn)s−i−3d[3]ki+1, |
where
d[3]ki+1=33k(3!)−1(−fki+3fki+1−3fki+2+fki+3). |
This implies B‴k→d[3] and Bk→f. This implies that for k→∞ the d[3] converges uniformly to f‴ on [0,n]. This concludes that d[3]=f‴∈π[0,n]. Hence the proof.
We first introduce the inequalities to compute the deviation between two consecutive points at the same refinement level then we introduce the inequalities to compute the deviation between successive levels of polygons produced by ternary and its DD refinement schemes.
Lemma 3.1. If f0={(i/30,f0i)} is the initial polygon and fk+1={(i/3k+1,fk+1i)} is the polygon obtained by TRS at (k+1)th refinement level. If ϖ<1 then the deviation between two consecutive points at (k+1)th level is
maxi‖fk+1i+1−fk+1i‖≤(ϖ)k+1maxi‖f0i+1−f0i‖, | (3.1) |
ϖ=max{ϖ1,ϖ2,ϖ3}<1, | (3.2) |
{ϖ1=m−1∑μ=0|μ∑ν=0(ρμ−ν−ϱμ−ν)|,ϖ2=m−1∑μ=0|μ∑ν=0(ϱμ−ν−σμ−ν)|,ϖ3=m∑μ=0|μ∑ν=0(σμ−ν−ρμ−ν−1)|. | (3.3) |
Proof. From (2.1), we get
fk+13i+1−fk+13i=m∑ν=0ϱνfki+ν−m∑ν=0ρνfki+ν=m∑ν=0(ϱν−ρν)fki+ν. |
This further implies
fk+13i+1−fk+13i=[(ρ0−ϱ0)(fki+1−fki)+{(ρ0−ϱ0)+(ρ1−ϱ1)}(fki+2−fki+1)+{(ρ0−ϱ0)+(ρ1−ϱ1)+(ρ2−ϱ2)}(fki+3−fki+2)+…+{(ρ0−ϱ0)+(ρ1−ϱ1)+…+(ρm−1−ϱm−1)}(fki+m−fki+m−1)+{(ϱ0−a0)+(ϱ1−ρ1)+…+(ϱm−ρm)}fki+m]. |
This leads to
fk+13i+1−fk+13i=m−1∑μ=0{μ∑ν=0(ρμ−ν−ϱμ−ν)}(fki+μ+1−fki+μ)+m∑ν=0(ϱν−ρν)fki+m. |
Since by (2.2), m∑ν=0(ϱν−ρν)=0, then
fk+13i+1−fk+13i=m−1∑μ=0{μ∑ν=0(ρμ−ν−ϱμ−ν)(fki+μ+1−fki+μ)}. |
By taking maximum norm, we get
‖fk+13i+1−fk+13i‖≤ϖ1maxi‖fki+1−fki‖, | (3.4) |
where
ϖ1=m−1∑μ=0|μ∑ν=0(ρμ−ν−ϱμ−ν)|. |
Using (2.1) and similar procedure as above, we get
‖fk+13i+2−fk+13i+1‖≤ϖ2maxi‖fki+1−fki‖, | (3.5) |
where
ϖ2=m−1∑μ=0|μ∑ν=0(ϱμ−ν−σμ−ν)|. |
and
‖fk+13i+3−fk+13i+2‖≤ϖ3maxi‖fki+1−fki‖, | (3.6) |
where
ϖ3=m∑μ=0|μ∑ν=0(σμ−ν−ρμ−ν−1)|. |
Combining (3.4), (3.5) and (3.6), it proceeds as
maxi‖fk+1i+1−fk+1i‖≤ϖmaxi‖fki+1−fki‖, |
where
ϖ=max{ϖ1,ϖ2,ϖ3}. |
This concludes
maxi‖fk+1i+1−fk+1i‖≤(ϖ)k+1maxi‖f0i+1−f0i‖. |
This completes the proof.
Theorem 3.2. If f0={(i/30,f0i)} is the initial polygon and fk+1={(i/3k+1,fk+1i)} is the polygon obtained by TRS at (k+1)th refinement level. If ϖ∗<1 then the deviation between two successive polygons at kth and (k+1)th levels is
‖fk+1−fk‖∞≤ϖ∗maxi‖fki+1−fki‖, | (3.7) |
where
ϖ∗=max{ϖ4,ϖ5,ϖ6}, | (3.8) |
{ϖ4=m−1∑μ=0|1−μ∑ν=0ρμ−ν|,ϖ5=|23−ϱ0|+m−1∑μ=1|1−μ∑ν=0ϱμ−ν|,ϖ6=|13−σ0|+m−1∑μ=1|1−μ∑ν=0σμ−ν|. | (3.9) |
Proof. Since the maximum deviation between fk+1 and fk occurs at the diadic values tk+13i=tki, tk+13i+1=13(2tki+tki+1) and tk+13i+2=13(tki+2tki+1) respectively, therefore
‖fk+1−fk‖∞≤max{Z1k,Z2k,Z3k}, | (3.10) |
where
{Z1k=maxi‖fk+13i−fki‖,Z2k=maxi‖fk+13i+1−13(2fki+fki+1)‖,Z3k=maxi‖fk+13i+2−13(fki+2fki+1)‖. | (3.11) |
From (2.1), we obtain
fk+13i−fki=m∑ν=0ρνfki+ν−fki. |
Since by (2.1), m∑ν=0ρν−1=0, then
fk+13i−fki=m−1∑μ=0(1−μ∑ν=0ρμ−ν)(fki+μ+1−fki+μ). |
Taking norm, we get
‖fk+13i−fki‖≤ϖ4maxi‖fki+1−fki‖, | (3.12) |
where
ϖ4=m−1∑μ=0|1−μ∑ν=0ρμ−ν|. |
Using (2.1) and similar procedure as above, we get
‖fk+13i+1−(23fki+13fki+1)‖≤ϖ5maxi‖fki+1−fki‖, | (3.13) |
where
ϖ5=|23−ϱ0|+m−1∑μ=1|1−μ∑ν=0ϱμ−ν|. |
‖fk+13i+2−(13fki+23fki+1)‖≤ϖ6maxi‖fki+1−fki‖, | (3.14) |
where
ϖ6=|13−σ0|+m−1∑μ=1|1−μ∑ν=0σμ−ν|. |
From (3.10)-(3.14), we get
‖fk+1−fk‖∞≤ϖ∗maxi|fki+1−fki|, |
where
ϖ∗=max{ϖ4,ϖ5,ϖ6}. |
Hence the proof.
Lemma 3.3. If d[1]0={(i/30,d[1]0i)} is the initial polygon and d[1](k+1)=
{(i/3k+1,d[1](k+1)i)} is the polygon obtained by first order DD TRS at (k+1)th refinement level. If ϖ∗∗<1 and
{c1=m−1∑ν=0(m−ν)(2ϱν−σν−ρν)=0,c2=m∑ν=0{(2ν+1)σm−ν−(m−ν)(ρν+ϱν)}=0,c3=m∑ν=0{2νρm−ν−νϱm−ν−(ν+1)σm−ν}=0, | (3.15) |
then the deviation between two consecutive points at (k+1)th level is
maxi‖d[1](k+1)i+1−d[1](k+1)i‖≤(ϖ∗∗)k+1maxi‖d[1]0i+1−d[1]0i‖, | (3.16) |
where
ϖ∗∗=max{ϖ∗1,ϖ∗2,ϖ∗3}, | (3.17) |
{ϖ∗1=3m−2∑μ=0|μ∑ν=0(ν+1)(ρμ−ν−2ϱμ−ν+σμ−ν)|,ϖ∗2=3m−1∑μ=0|μ∑ν=0{(ν+1)ϱμ−ν−(2ν+2)σμ−ν+νρμ−ν}|,ϖ∗3=3m−1∑μ=0|μ∑ν=0{(ν+1)σμ−ν−2νρμ−ν+νϱμ−ν}|. | (3.18) |
Proof. Using (2.3) and proceeding similarly as in Lemma 3.1, we get
If, c1=m−1∑ν=0(m−ν)(2ϱν−σν−ρν)=0, then
‖d[1](k+1)3i+1−d[1](k+1)3i‖≤ϖ∗1maxi‖d[1]ki+1−d[1]ki‖, | (3.19) |
where
ϖ∗1=3m−2∑μ=0|μ∑ν=0(ν+1)(ρμ−ν−2ϱμ−ν+σμ−ν)|. |
Using (2.3) and similar procedure as above, we get
c2=m∑ν=0{(2ν+1)σm−ν−(m−ν)(ρν+ϱν)}=0,
‖d[1](k+1)3i+2−d[1](k+1)3i+1‖≤ϖ∗2maxi‖d[1]ki+1−d[1]ki‖. | (3.20) |
where
ϖ∗2=3m−1∑μ=0|μ∑ν=0{(ν+1)ϱμ−ν−(2ν+2)σμ−ν+νρμ−ν}|. |
And
c3=m∑ν=0{2νρm−ν−νϱm−ν−(ν+1)σm−ν}=0,
‖d[1](k+1)3i+3−d[1](k+1)3i+2‖≤ϖ∗3maxi‖d[1]ki+1−d[1]ki‖, | (3.21) |
where
ϖ∗3=3m−1∑μ=0|μ∑ν=0{(ν+1)σμ−ν−2νρμ−ν+νϱμ−ν}|. |
Combining Eqs (3.19), (3.20) and (3.21), we get
maxi‖d[1](k+1)i+1−d[1](k+1)i‖≤ϖ∗∗maxi‖d[1]ki+1−d[1]ki‖, |
whereas
ϖ∗∗=max{ϖ∗1,ϖ∗2,ϖ∗3}. |
This implies
maxi‖d[1](k+1)i+1−d[1](k+1)i‖≤(ϖ∗∗)k+1maxi‖d[1]0i+1−d[1]0i‖. |
This completes the proof.
Theorem 3.4. If d[1]0={(i/30,d[1]0i)} is the initial polygon and d[1](k+1)=
{(i/3k+1,d[1](k+1)i)} is the polygon obtained by first order DD TRS at (k+1)th refinement level. If ϖ∗∗∗<1 and
{c4=−13+m∑ν=1ν(ρm−ν−ϱm−ν)=0,c5=−13+m∑ν=1ν(ϱm−ν−σm−ν)=0,c6=−13+m∑ν=0{(ν+1)σm−ν−νρm−ν}=0, | (3.22) |
then the deviation between two successive polygons at kth and (k+1)th levels is
‖d[1](k+1)−d[1]k‖∞≤ϖ∗∗∗maxi‖d[1]ki+1−d[1]ki‖, | (3.23) |
where
ϖ∗∗∗=max{ϖ∗4,ϖ∗5,ϖ∗6}, | (3.24) |
{ϖ∗4=3m−2∑μ=0|13+μ∑ν=0{(ν+1)(ϱμ−ν−ρμ−ν)}|,ϖ∗5=3|232−ϱ0+σ0|+3m−2∑μ=1|13+μ∑ν=0{(ν+1)(σμ−ν−ϱμ−ν)}|,ϖ∗6=3|132−σ0|+3m−1∑μ=1|13+μ∑ν=0(νρμ−ν−(ν+1)σμ−ν)|. | (3.25) |
Proof. Since the maximum deviation between d[1](k+1) and d[1]k occurs at the diadic values tk+13i=tki, tk+13i+1=13(2tki+tki+1) and tk+13i+2=13(tki+2tki+1) respectively, therefore
‖d[1](k+1)−d[1]k‖∞≤max{Z4k,Z5k,Z6k}, | (3.26) |
where
{Z4k=maxi‖d[1](k+1)3i−d[1]ki‖,Z5k=maxi‖d[1](k+1)3i+1−13(2d[1]ki+d[1]ki+1)‖,Z6k=maxi‖d[1](k+1)3i+2−13(d[1]ki+2d[1]ki+1)‖. | (3.27) |
By (2.3), we get
d[1](k+1)3i−d[1]ki=3m−1∑μ=0{μ∑ν=0(ρμ−ν−ϱμ−ν)d[1]ki+μ}−d[1]ki. |
This leads to
d[1](k+1)3i−d[1]ki=3[m−2∑μ=0{13+μ∑ν=0(ν+1)(ϱμ−ν−ρμ−ν)(d[1]ki+μ+1−d[1]ki+μ+1)}+{−13+m∑ν=1ν(ρm−ν−ϱm−ν)}d[1]ki+m−1]. |
If, c4=−13+m∑ν=1ν(ρm−ν−ϱm−ν)=0, taking norm we get
‖d[1](k+1)3i−d[1]ki‖≤ϖ∗4maxi‖d[1]ki+1−d[1]ki‖, | (3.28) |
where,
ϖ∗4=3m−2∑μ=0|13+μ∑ν=0{(ν+1)(ϱμ−ν−ρμ−ν)}|. |
Using (2.3) and similar procedure as above, we get
c5=−13+m∑ν=1ν(ϱm−ν−σm−ν)=0,
‖d[1](k+1)3i+1−(23d[1]ki+13d[1]ki+1)‖≤ϖ∗5maxi‖d[1]ki+1−d[1]ki‖, | (3.29) |
where
ϖ∗5=3|232−ϱ0+σ0|+3m−2∑μ=1|13+μ∑ν=0{(ν+1)(σμ−ν−ϱμ−ν)}|. |
And
c6=−13+m∑ν=0{(ν+1)σm−ν−νρm−ν}=0,
maxi‖ˆdk+13i+2−(13ˆdki+23ˆdki+1)‖≤ϖ∗6maxi‖d[1]ki+1−d[1]ki‖, | (3.30) |
where
ϖ∗6=3|132−σ0|+3m−1∑μ=1|13+μ∑ν=0(νρμ−ν−(ν+1)σμ−ν)|. |
From (3.26)-(3.30)
‖d[1](k+1)−d[1]k‖∞≤ϖ∗∗∗maxi‖d[1]ki+1−d[1]ki‖, |
whereas
ϖ∗∗∗=max{ϖ∗4,ϖ∗5,ϖ∗6}. |
Hence the proof.
Lemma 3.5. If d[2]0={(i/30,d[2]0i)} is the initial polygon and d[2](k+1)=
{(i/3k+1,d[2](k+1)i)} is the polygon obtained by second order DD TRS at (k+1)th refinement level. If ϑ<1 and
{χ1=m−1∑ν=0{3ν(ν+1)2(ρm−ν−1−ϱm−ν−1)−(ν+1)σm−ν−1}=0,χ2=m−1∑ν=0{(ν+1)(3ν+2)2ϱm−1−ν−(ν+1)(3ν+4)2σm−1−ν}=0,χ3=m−1∑ν=0{3(ν+1)(ν+2)2σm−1−ν−(ν+1)ϱm−1−ν−3ν(ν+1)2ρm−1−ν}=0, | (3.31) |
then the deviation between two consecutive points at (k+1)th level is
maxi‖d[2](k+1)i+1−d[2](k+1)i‖≤(ϑ)k+1maxi‖d[2]0i+1−d[2]0i‖, | (3.32) |
where
ϑ=max{ϑ1,ϑ2,ϑ3}, | (3.33) |
{ϑ1=32m−2∑μ=0|μ∑ν=0{(ν+1)σμ−ν+3ν(ν+1)2(ϱμ−ν−ρμ−ν)}|,ϑ2=32m−2∑μ=0|μ∑ν=0{(ν+1)ρμ−ν+3(ν+1)(ν+2)2(σμ−ν−ϱμ−ν)}|,ϑ3=32m−2∑μ=0|μ∑ν=0{3ν(ν+1)2ρμ−ν+(ν+1)ϱμ−ν−3(ν+1)(ν+2)2σμ−ν}|. | (3.34) |
Theorem 3.6. If d[2]0={(i/30,d[2]0i)} is the initial polygon and d[2](k+1)=
{(i/3k+1,d[2](k+1)i)} is the polygon obtained by second order DD TRS at (k+1)th refinement level. If ϑ∗<1 and
{χ4=−132+m−1∑ν=0[(ν+1)2{νϱm−1−ν+(ν+2)σm−1−ν−2νρm−1−ν}]=0,χ5=−132+m−1∑ν=1[ν(ν+1)2{ρm−ν−1+σm−ν−1−2ϱm−ν−1}]=0,χ6=−132+m∑ν=1{ν(ν+1)2ϱm−ν−ν(ν+1)σm−ν+ν(ν−1)2ρm−ν}=0, | (3.35) |
then the deviation between two successive polygons at kth and (k+1)th levels is
‖d[2](k+1)−d[2]k‖∞≤ϑ∗maxi‖d[2]ki+1−d[2]ki‖, | (3.36) |
where
ϑ∗=max{ϑ4,ϑ5,ϑ6}, | (3.37) |
{ϑ4=32m−2∑μ=0|132+μ∑ν=0{ν(ν+1)ρμ−ν−ν(ν+1)2ϱμ−ν−(ν+1)(ν+2)2σμ−ν}|,ϑ5=32|233|+32m−2∑μ=1|19+μ∑ν=1{ν(ν+1)2(2ϱμ−ν−ρμ−ν−σμ−ν)}|,ϑ6=32|133|+32m−1∑μ=1|132+μ∑ν=1{ν(ν+1)σμ−ν−ν(ν+1)2ϱμ−ν−ν(ν−1)2ρμ−ν}|. | (3.38) |
Lemma 3.7. If d[3]0={(i/30,d[3]0i)} is the initial polygon and d[3](k+1)=
{(i/3k+1,d[3](k+1)i)} is the polygon obtained by third order DD TRS at (k+1)th refinement level. If ϑ∗∗<1 and
{χ7=m−2∑ν=0[(ν+1)(ν+2)2{(ν+1)ρm−ν−2−(2ν+3)ϱm−ν−2+(ν+2)σm−ν−2}]=0,χ8=m−2∑ν=0[(ν+1)(ν+2){(ν−1)2ρm−ν−2+(ν+4)2ϱm−ν−2−(ν+3)σm−ν−2}]=0,χ9=m−2∑ν=0[(ν+1)(ν+2){−νρm−ν−2+(ν−1)2ϱm−ν−2+(ν+4)2σm−ν−2}]=0, | (3.39) |
then the deviation between two consecutive points at (k+1)th level is
maxi‖d[3](k+1)i+1−d[3](k+1)i‖≤(ϑ∗∗)k+1maxi‖d[3]0i+1−d[3]0i‖, | (3.40) |
where
ϑ∗∗=max{ϑ7,ϑ8,ϑ9}, | (3.41) |
{ϑ7=33m−2∑μ=0|μ∑ν=0{ν(ν+1)(ν+2)ϱμ−ν−ν(ν+1)(ν+3)2ρμ−ν−(ν−1)(ν+1)(ν+2)2σμ−ν}|,ϑ8=33m−3∑μ=0|μ∑ν=0{−(ν−1)(ν+1)(ν+2)2ρμ−ν−(ν+1)(ν+2)(ν+4)2ϱμ−ν+(ν+1)(ν+2)(ν+3)σμ−ν}|,ϑ9=33m−3∑μ=0|μ∑ν=0{ν(ν+1)(ν+2)ρμ−ν−(ν−1)(ν+1)(ν+2)2ϱμ−ν−(ν+1)(ν+2)(ν+4)2σμ−ν}|. | (3.42) |
Theorem 3.8. If d[3]0={(i/30,d[3]0i)} is the initial polygon and d[3](k+1)={(i/3k+1,d[3](k+1)i)} is the polygon obtained by third order DD TRS at (k+1)th refinement level. If ϑ∗∗∗<1 and
{χ10=−133+m−2∑ν=0[(ν+1)(ν+2)2{σm−2−ν+νϱm−2−ν−νρm−2−ν}]=0,χ11=−133+m−2∑ν=0[(ν+1)(ν+2)2{ρm−ν−2−(ν+3)ϱm−ν−2+(ν+3)σm−ν−2}]=0,χ12=−133+m−2∑ν=0[(ν+1)(ν+2)2{ϱm−ν−2−(ν+3)σm−ν−2+νρm−ν−2}]=0, | (3.43) |
then the deviation between two successive polygons at kth and (k+1)th levels is
‖d[3](k+1)−d[3]k‖∞≤ϑ∗∗∗maxi‖d[3]ki+1−d[3]ki‖, | (3.44) |
where
ϑ∗∗∗=max{ϑ10,ϑ11,ϑ12}, | (3.45) |
{ϑ10=33m−3∑μ=0|133+μ∑ν=0{(ν+1)(ν+2)2(νρμ−ν−νϱμ−ν−σμ−ν)}|,ϑ11=33|234|+33m−2∑μ=1|133+μ∑ν=1{(ν+1)2{−νρμ−ν+(ν+2)ϱμ−ν−(ν+2)σμ−ν}}|,ϑ12=33|134|+33m−2∑μ=1|{133+μ∑ν=1ν(ν+1)2{(ν+2)σμ−ν−ϱμ−ν−(ν−1)ρμ−ν}}|. | (3.46) |
Now we present the analysis of the ternary refinement schemes. A function is called Cm-continuous if its mth order derivative is continuous. The common class of continuous function is C=C0. It is natural to think of a Cm function as being a little bit rough, but the graph of a C3 function looks smooth. So we discuss the analysis of the schemes up to C3-continuity. The results can be extended similarly. Let {πm[0,n], m=0,1,2,3} denotes the set of Cm-continuous functions on the closed and bounded interval [0,n].
Theorem 4.1. If f0={(i/30,f0i)} is the initial polygon and fk+1={(i/3k+1,fk+1i)} is the polygon obtained by TRS at (k+1)th refinement level. If ϖ, ϖ∗<1 then limk→∞fk=f∈π[0,n]=π0[0,n].
Proof. The (3.7), gives
‖fk+1−fk‖∞≤ϖ∗maxi‖fki+1−fki‖, |
and (3.1), gives
‖fk+1−fk‖∞≤ϖ∗(ϖ)kmaxi‖f0i+1−f0i‖, |
Since ϖ, ϖ∗<1 therefore {fk}∞k=0 is a Cauchy sequence on closed and bounded interval [0,n]. Hence it is convergent. That is
limk→∞fk=f∈π[0,n]=π0[0,n]. |
Hence the proof.
Lemma 4.2. If d[1]0={(i/30,d[1]0i)} is the initial polygon and d[1](k+1)=
{(i/3k+1,d[1](k+1)i)} is the polygon obtained by first order DD TRS at (k+1)th refinement level. If ϖ∗∗, ϖ∗∗∗<1 and c1,c2,c3,c4,c5,c6=0, then limk→∞d[1]k=d[1]∈π[0,n]=π0[0,n].
Proof. By (3.23), we have
‖d[1](k+1)−d[1]k‖∞≤ϖ∗∗∗maxi‖d[1]ki+1−d[1]ki‖, |
Using (3.16), we have
‖d[1](k+1)−d[1]k‖∞≤ϖ∗∗∗(ϖ∗∗)kmaxi‖d[1]0i+1−d[1]0i‖, |
Since ϖ∗∗, ϖ∗∗<1, therefore {d[1]k}∞k=0 defines a Cauchy sequence on [0,n] and
limk→∞d[1]k=d[1]∈π[0,n]=π0[0,n]. |
This completes the proof.
Theorem 4.3. Let f∈π0[0,n] be the limiting curve produced by the ternary refinement scheme. If ϖ∗∗, ϖ∗∗∗<1 and c1,c2,c3,c4,c5,c6=0 then f∈π1[0,n].
Proof. Lemma 4.2 leads to limk→∞d[1]k=d[1]∈π0[0,n]. Lemma 2.4 gives d[1]=f′. Therefore, we have the result, f∈π1[0,n].
Lemma 4.4. If d[2]0={(i/30,d[2]0i)} is the initial polygon and d[2](k+1)=
{(i/3k+1,d[2](k+1)i)} is the polygon obtained by second order DD TRS at (k+1)th refinement level. If ϑ, ϑ∗<1 and χ1,χ2,χ3,χ4,χ5,χ6=0 then limk→∞d[2]k=d[2]∈π0[0,n].
Proof. By (3.36), we have
‖d[2](k+1)−d[2]k‖∞≤ϑ∗maxi‖d[2]ki+1−d[2]ki‖, |
Using (3.32), we have
‖d[2](k+1)−d[2]k‖∞≤ϑ∗(ϑ)kmaxi‖d[2]0i+1−d[2]0i‖, |
Since ϑ, ϑ∗<1 therefore {d[2]k}∞k=0 is a Cauchy convergent sequence. So
limk→∞d[2]k=d[2]∈π0[0,n]. |
Hence the proof.
Theorem 4.5. Let f∈π0[0,n] be the limiting curve produced by the ternary refinement scheme. If ϑ, ϑ∗<1 and φ1,φ2,φ3,φ4,φ5,φ6=0 then f∈π2[0,n].
Proof. Lemma 4.4 gives limk→∞d[2]k=d[2]∈π0[0,n]. Lemma 2.5 leads to d[2]=f″. This implies f∈π2[0,n].
Lemma 4.6. If d[3]0={(i/30,d[3]0i)} is the initial polygon and d[3](k+1)=
{(i/3k+1,d[3](k+1)i)} is the polygon obtained by third order DD TRS at (k+1)th refinement level. If ϑ∗∗, ϑ∗∗∗<1 and φ7,φ8,φ9,φ10,φ11,φ12=0 then limk→∞d[3]k)=d[3]∈π0[0,n].
Proof. By (3.44), we have
‖d[3](k+1)−d[3]k‖∞≤ϑ∗∗∗maxi‖d[3]ki+1−d[3]ki‖, |
Using (3.40), we have
‖d[3](k+1)−d[3]k‖∞≤ϑ∗∗∗(ϑ∗∗)kmaxi‖d[3]0i+1−d[3]0i‖, |
Since ϑ∗∗, ϑ∗∗∗<1 therefore {d[3]k}∞k=0 is a Cauchy convergent sequence. Thus
limk→∞d[3]k=d[3]∈π0[0,n]. |
Hence the proof.
Theorem 4.7. Let f∈π0[0,n] be the limiting curve produced by the ternary refinement scheme. If ϑ, ϑ∗<1 and φ7,φ8,φ9,φ10,φ11,φ12=0 then f∈π3[0,n].
Proof. Lemma 4.6, implies that limk→∞d[3]k)=d[3]∈π0[0,n] while Lemma 2.6 leads to d[3]=f‴. This implies f∈π3[0,n].
Application and authenticity of the results
We discuss the analysis of the ternary refinement schemes introduced by [8,9,10,16,17]. We conclude that the results obtained by our methods are always equivalent to the one returned by the Laurent polynomial method. We add the Figure 2 for the interest of general readers by the direction of anonymous reviewer of this paper. The Figure 2(d) depicts the smoothness of the limiting curve produced by the ternary scheme. The Figure 2(a), (b) and (c) represent the initial, first and second refinement level of the ternary scheme.
Example 4.1. If the curve is produced by the following 4-point ternary refinement scheme [17]
{fk+13i=ρ0fki−1+ρ1fki+ρ2fki+1+ρ3fki+2,fk+13i+1=ϱ0fki−1+ϱ1fki+ϱ2fki+2+ϱ3fki+3,fk+13i+2=σ0fki−1+σ1fki+σ2fki+2+σ3fki+3, |
where
{ρ0=(581+53μ), ρ1=(1927−3μ), ρ2=(1354+μ), ρ3=(−1162+13μ),ϱ0=μ, ϱ1=(12−μ), ϱ2=(12−μ), ϱ3=μ,σ0=(−1162+13μ), σ1=(1354+μ), σ2=(1927−3μ), σ3=(581+53μ), |
then by Laurent polynomial method the scheme produces C3-continuous curve for μ∈(−127,227). By Theorems 4.1, 4.3, 4.5 and 4.7, we also get the same results.
Example 4.2. If the curve is produced by the following 4-point ternary refinement scheme [9]
{fk+13i=ρ1fki,fk+13i+1=ϱ0fki−1+ϱ1fki+ϱ2fki+2+ϱ3fki+3,fk+13i+2=σ0fki−1+σ1fki+σ2fki+2+σ3fki+3, |
where
{ρ0=0,ρ1=1,ρ2=0,ρ3=0,ϱ0=−118−16μ, ϱ1=1318+12μ, ϱ2=718−12μ, ϱ3=−118+16μ,σ0=−118+16μ,σ1=718−12μ, σ2=1318+12μ, σ3=−118−16μ, |
then by Laurent polynomial method the scheme produces C2-continuous curve over the interval μ∈(115,19). By Theorems 4.1, 4.3, and 4.5, we also get the same results.
Example 4.3. If the curve is produced by the following 3-point ternary refinement scheme [8]
{fk+13i=ρ0fki−1+ρ1fki+ρ2fki+1,fk+13i+1=ϱ1fki,fk+13i+2=σ0fki−1+σ1fki+σ2fki+1, |
where
{ρ0=(ρ+13), ρ1=(23−2ρ),ρ2=ρ,ϱ0=0,ϱ1=1, ϱ2=0,σ0=ρ, σ1=(23−2ρ),σ2=(ρ+13), |
then by both methods the scheme produces C1-continuous curve for w∈(−19,0).
Example 4.4. If the curve is produced by the following 6-point ternary refinement scheme [10]
{fk+13i=ρ2fki,fk+13i+1=ϱ0fki−2+ϱ1fki−1+ϱ2fki+ϱ3fki+1+ϱ4fki+2+ϱ5fki+3,fk+13i+2=σ0fki−2+σ1fki−1+σ2fki+σ3fki+1+σ4fki+2+σ5fki+3, | (4.1) |
where
{ρ0=0,ρ1=0,ρ2=1,ρ3=0,ρ4=0,ρ5=0,ϱ0=(−1181+13ω), ϱ1=(1327−51ω),ϱ2=(−227+74ω),ϱ3=(7481−46ω),ϱ4=(−527+9ω), ϱ5=ω,σ0=ω, σ1=(−527+9ω), σ2=(7481−46ω),σ3=(−227+74ω),σ4=(1327−51ω), σ5=(−1181+13ω), |
then by both methods the scheme produces C2-continuous curve over the interval w∈(141215,231944).
Example 4.5. If the curve is produced by the following 3-point ternary refinement scheme [16]
{fk+13i=ρ0fki−1+ρ1fki+ρ2fki+1,fk+13i+1=ϱ0fki−1+ϱ1fki+ϱ2fki+2,fk+13i+2=σ0fki−1+σ1fki+σ2fki+2, | (4.2) |
where
{ρ0=(2572+μ),ρ1=(2336−2μ),ρ2=(172+μ),ϱ0=(18+μ), ϱ1=(34−2μ), ϱ2=(18+μ),σ0=(172+μ),σ1=(2336−2μ), σ2=(2572+μ), |
then by both methods the scheme produces C2-continuous curve for μ∈(−172,772).
Here we present the brief summary of the work done so for in this paper.
● If max{ϖ1,ϖ2,ϖ3}<1 where ϖ1,ϖ2,ϖ3 are defined in (3.3) then TRS will generate C0 continuous curve.
● If c1, c2, c3, c4, c5, c6 defined in (3.15) and (3.22) are equal to zero and max{ϖ∗1,ϖ∗2,ϖ∗3}<1, where ϖ∗1,ϖ∗2,ϖ∗3 are defined in (3.18) then TRS will generate C1 continuous curve.
● If χ1, χ2, χ3, χ4, χ5, χ6 defined in (3.31) and (3.35) are equal to zero and max{ϑ1,ϑ2,ϑ3}<1, where ϑ1,ϑ2,ϑ3 are defined in (3.34) then TRS will generate C2 continuous curve.
● If χ7, χ8, χ9 χ10, χ11, χ12 defined in (3.39) and (3.43) are equal to zero and max{ϑ7,ϑ8,ϑ9}<1, where ϑ7,ϑ8,ϑ9 are defined in (3.42) then TRS will generate C3 continuous curve.
There are two major techniques to analyze the refinement schemes. These are called Laurent polynomial and divided difference (DD) techniques.
● Our technique is the generalization of the DD technique for ternary refinement schemes.
● We have presented the explicit form of the general inequalities for the analysis of the ternary refinement schemes. These inequalities contain simple algebraic expressions. In these inequalities the polynomial factorization, division and summation are not involved. Simple arithmetic operations such as subtraction and multiplication are involved to evaluate the inequalities.
● While in Laurent polynomial technique, the polynomial factorization, division and summation are involved to evaluate the inequalities. In this technique, the explicit form of the general inequalities are also not available.
● So it is obvious that the computational complexity of our techniques is less than the complexity of Laurent polynomial technique.
In this paper, we have introduced an alternative technique to analyze a class of ternary refinement schemes. A comparative study of the proposed technique with other existing technique has been presented to prove the effectiveness of the proposed technique. It has been observed that the alternative technique has less computational cost comparative to the existing Laurent polynomial technique.
The second author is grate full to HEC Pakistan for awarding the fellowship under the Indigenous Ph.D. Scholarship Scheme. The author Yu-Ming Chu is supported by National Natural Science Foundation of China (Grant Nos. 61673169, 11971142).
Conceptualization, Ghulam Mustafa and Dumitru Baleanu; Formal analysis, Dumitru Baleanu and Yu-Ming Chu; Methodology, Ghulam Mustafa and Syeda Tehmina Ejaz; Supervision, Ghulam Mustafa; Writing original draft, Syeda Tehmina Ejaz and Ghulam Mustafa; Writing, review and editing, Syeda Tehmina Ejaz and Yu-Ming Chu.
The authors declare no conflict of interest.
[1] | Made in China 2025, Issued by the State Council. |
[2] | Y. C. Zou, Construction and Application of Traffic Knowledge Graph Based on Multi-source Data Fusion (in Chinese), Master's thesis, Dalian University of Technology, 2020. Available from: https://cdmd.cnki.com.cn/Article/CDMD-10141-1020653436.htm. |
[3] | L. Wang, Research on Intelligent Knowledge Support of Urban Rail Transit Construction Safety Management Based on Knowledge Graph (in Chinese), Ph.D thesis, China University of Mining and Technology, 2019. https://doi.org/10.27623/d.cnki.gzkyu.2019.000408 |
[4] | G. L. Zhou, Research on Prediction of Urban Traffic Congestion Area Based on Knowledge Graph and Deep Learning (in Chinese), Master's thesis, University of Science and Techno-logy of China, 2019. Available from: https://kns.cnki.net/kcms2/article/abstract?v = 3uoqIhG8C475KOm_zrgu4lQARvep2SAkOsSuGHvNoCRcTRpJSuXuqaqG2zp1ftApp1d23kvjOO2oSeVFvORibKCV9PhY0Iws & uniplatform = NZKPT & src = copy. |
[5] | M. Zhou, Study on Vehicle Lane Change Risk Situation Based on Driving Behavior and Driving Style Inclination (in Chinese), Chang'an University, 2022. https://doi.org/10.26976/d.cnki.gchau.2022.000496 |
[6] |
S. Pantangi, G. Fountas, P. Anastasopoulos, J. Pierowicz, K. Majka, A. Blatt, Do High Visibility Enforcement programs affect aggressive driving behavior? An empirical analysis using Naturalistic Driving Study data, Accid. Anal. Prev., 138 (2020), 105361. https://doi.org/10.1016/j.aap.2019.105361 doi: 10.1016/j.aap.2019.105361
![]() |
[7] |
P. Sârbescu, A. Rusu, Personality predictors of speeding: anger-aggression and impulsive-Sensation Seeking. A systematic review and meta-analysis, J. Saf. Res., 77 (2021), 86−98. https://doi.org/10.1016/j.jsr.2021.02.004 doi: 10.1016/j.jsr.2021.02.004
![]() |
[8] |
J. Kovaceva, I. Isaksson-Hellman, N. Murgovski, Identification of aggressive driving from naturalistic data in car-following situations, J. Saf. Res., 73 (2020), 225−234. https://doi.org/10.1016/j.jsr.2020.03.003 doi: 10.1016/j.jsr.2020.03.003
![]() |
[9] |
Y. Xu, S. Bao, A. K. Pradhan, Modeling drivers' reaction when being tailgated: a random forests method, J. Saf. Res., 78 (2021), 28−35. https://doi.org/10.1016/j.jsr.2021.05.004 doi: 10.1016/j.jsr.2021.05.004
![]() |
[10] | Y. Dai, Research and Application of Risk Assessment of Dangerous Goods Road Transport Vehicles Based on Hierarchical Fuzzy Network Model (in Chinese), Chang'an University, 2022. https://doi.org/10.26976/d.cnki.gchau.2022.002093 |
[11] | Q. Yang, Research on the Risk Analysis Method of Lane Change on Expressway Vehicles (in Chinese), The People's Public Security University of China, 2022. https://doi.org/10.27634/d.cnki.gzrgu.2022.000006 |
[12] | K. Han, Research on the Video Detection Method for Violations of not Driving in the Guide Lane (in Chinese), Nanjing University of Science and Technology, 2019. https://doi.org/10.27241/d.cnki.gnjgu.2019.001552 |
[13] |
Y. N. Cui, J. Li, L. Shen, Y. Shen, L. Qiao, J. Bo, Duration-HyTE: A Time-aware knowledge representation learning method based on duration modeling, J. Comput. Res. Dev., 57 (2020), 1239–1251. https://doi.org/10.7544/issn1000-1239.2020.20190253 doi: 10.7544/issn1000-1239.2020.20190253
![]() |
[14] | M. Belkin, P. Niyogi, Laplacian eigenmaps and spectral techniques for embedding and clustering, in Advances in Neural Information Processing Systems, 2002. Available from: https://papers.nips.cc/paper/2001/file/f106b7f99d2cb30c3db1c3cc0fde9ccb-Paper.pdf. |
[15] |
S. T. Roweis, L. K. Saul, Nonlinear dimensionality reduction by locally linear embedding, Science, 290 (2000), 2323–2326. https://doi.org/10.1126/science.290.5500.2323 doi: 10.1126/science.290.5500.2323
![]() |
[16] | J. Li, H. Dani, X. Hu, J. Tang, Y. Chang, H. Liu, Attributed network embedding for learning in a dynamic environment, in Proceedings of the 2017 ACM Conference on Information and Knowledge Management, (2017), 387−396. https://doi.org/10.1145/3132847.3132919 |
[17] | G. H. Nguyen, J. B. Lee, R. A. Rossi, N. K. Ahmed, E. Koh, S. Kim, Continuous-time dynamic network embeddings, in Companion Proceedings of the Web Conference 2018, (2018), 969−976. https://doi.org/10.1145/3184558.3191526 |
[18] | W. Yu, W. Cheng, C. Aggarwal, K. Zhang, H. Chen, W. Wang, NetWalk: A flexible deep embedding approach for anomaly detection in dynamic networks, in Proceedings of the 24th ACM SIGKDD International Conference on Knowledge Discovery & Data Mining, (2018), 2672−2681. https://doi.org/10.1145/3219819.3220024 |
[19] | R. Trivedi, M. Farajtabar, P. Biswal, H. Zha, Representation learning over dynamic graphs, preprint, arXiv: 1803.04051. |
[20] | Y. Zuo, G. Liu, H. Lin, J. Guo, X. Hu, J. Wu, Embedding temporal network via neighborhood formation, in Proceedings of the 24th ACM SIGKDD International Conference on Knowledge Discovery & Data Mining, (2018), 2857–2866. https://doi.org/10.1145/3219819.3220054 |
[21] |
B. Lyu, Y. Yang, S. Wen, T. Huang, K. Li, Neural architecture search for portrait parsing, IEEE Trans. Neural Networks Learn. Syst., 34 (2023), 1112−1121. https://doi.org/10.1109/TNNLS.2021.3104872 doi: 10.1109/TNNLS.2021.3104872
![]() |
[22] |
W. Li, S. Wen, K. Shi, Y. Yang, T. Huang, Neural architecture search with a lightweight transformer for text-to-image synthesis, IEEE Trans. Network Sci. Eng., 9(2022), 1567−1576. https://doi.org/10.1109/TNSE.2022.3147787 doi: 10.1109/TNSE.2022.3147787
![]() |
[23] | W. Kipf, M. Welling, Semi-supervised classification with graph convolutional networks, preprint, arXiv: 1609.02907. |
[24] |
B. Lyu, M. Hamdi, Y. Yang, Y. Cao, Z. Yan, K. Li, et al., Efficient spectral graph convolutional network deployment on memristive crossbars, IEEE Trans. Emerging Top. Comput. Intell., 7 (2022), 415−425. https://doi.org/10.1109/TETCI.2022.3210998 doi: 10.1109/TETCI.2022.3210998
![]() |
[25] |
B. Lyu, S. Wen, K. Shi, T. Huang, Multiobjective reinforcement learning-based neural architecture search for efficient portrait parsing, IEEE Trans. Cybern. , 53 (2021), 1158−1169. https://doi.org/10.1109/TCYB.2021.3104866 doi: 10.1109/TCYB.2021.3104866
![]() |
[26] |
J. Zhu, X. Han, H. Deng, C. Tao, L. Zhao, P. Wang, et al., KST-GCN: A knowledge-driven spatial-temporal graph convolutional network for traffic forecasting, IEEE Trans. Intell. Transp. Syst., 23 (2022), 15055–15065. https://doi.org/10.1109/TITS.2021.3136287 doi: 10.1109/TITS.2021.3136287
![]() |
[27] | Y. Fu, S. Okada, L. Wang, L. Guo, Y. Song, J. Liu, et al., CONSK-GCN: Conversational semantic- and knowledge-oriented graph convolutional network for multimodal emotion recognition, in 2021 IEEE International Conference on Multimedia and Expo (ICME), (2021), 1–6. https://doi.org/10.1109/ICME51207.2021.9428438 |
[28] | J. Yang, W. Zhou, L. Wei, J. Lin, J. Han, S. Hu, RE-GCN: Relation enhanced graph convolutional network for entity alignment in heterogeneous knowledge graphs, in Database Systems for Advanced Applications, 12113 (2020), 432–447. https://doi.org/10.1007/978-3-030-59416-9_26 |
[29] | Z. Liu, Z. D. Jiang, F. Wei, OD-GCN object detection by knowledge graph with GCN, preprint, arXiv: 1908.04385v1. |
[30] |
L. Zhao, Y. Song, C. Zhang, Y. Liu, P. Wang, T. Lin, et al., T-GCN: A temporal graph convolutional network for traffic prediction, IEEE Trans. Intell. Transp. Syst., 21 (2020), 3848–3858. https://doi.org/10.1109/TITS.2019.2935152. doi: 10.1109/TITS.2019.2935152
![]() |
[31] | S. Abu-El-Haija, A. Kapoor, B. Perozzi, J. Lee, N-GCN: Multi-scale graph convolution for semi-supervised node classification, in Proceedings of the 35th Uncertainty in Artificial Intelligence Conference, 115 (2020), 841–851. Available from: http://proceedings.mlr.press/v115/abu-el-haija20a.html. |
[32] | A. Pareja, G. Domeniconi, J. Chen, T. Ma, T. Suzumura, H. Kanezashi, et al., EvolveGCN: Evolving graph convolutional networks for dynamic graphs, in Proceedings of the AAAI Conference on Artificial Intelligence, 34 (2020), 5363−5370. https://doi.org/10.1609/aaai.v34i04.5984 |
[33] | P. Goyal, N. Kamra, X. He, Y. Liu, DynGEM: Deep embedding method for dynamic graphs, preprint, arXiv: 1805.11273. |
[34] | Z. Li, X. Jin, W. Li, S. Guan, J. Guo, H. Shen, et al., Temporal knowledge graph reasoning based on evolutional representation learning, in Proceedings of the 44th International ACM SIGIR Conference on Research and Development in Information Retrieval, (2021), 408–417. https://doi.org/10.1145/3404835.3462963 |
[35] | J. Li, Z. Han, H. Cheng, J. Su, Predicting path failure in time-evolving graphs, in the 25th ACM SIGKDD International Conference on Knowledge Discovery & Data Mining, (2019), 1279–1289. https://doi.org/10.1145/3292500.3330847 |
[36] |
H. Peng, H. Wang, B. Du, M. Z. A. Bhuiyan, H. Ma, J. Liu, et al., Spatial temporal incidence dynamic graph neural networks for traffic flow forecasting, Inf. Sci., 521 (2020), 277–290. https://doi.org/10.1016/j.ins.2020.01.043 doi: 10.1016/j.ins.2020.01.043
![]() |
[37] | A. Taheri, K. Gimpel, T. Berger-Wolf, Learning to represent the evolution of dynamic graphs with recurrent models, in Companion Proceedings of the 2019 World Wide Web Conference, (2019), 301−307. https://doi.org/10.1145/3308560.3316581 |
[38] | X. Wang, Y. Ma, Y. Wang, W. Jin, X. Wang, J. Tang, et al., Traffic flow prediction via spatial temporal graph neural network, in Proceedings of the Web Conference 2020, (2020), 1082−1092. https://doi.org/10.1145/3366423.3380186 |
[39] | B. Yu, H. Yin, Z. Zhu, Spatio-temporal graph convolutional networks: A deep learning framework for traffic forecasting, in International Joint Conference on Artificial Intelligence (IJCAI), 2018. Available from: https://www.ijcai.org/Proceedings/2018/0505.pdf. |
[40] | J. You, T. Du, J. Leskovec, ROLAND: Graph learning framework for dynamic graphs, in Proceedings of the 28th ACM SIGKDD Conference on Knowledge Discovery and Data Mining, (2022), 2358–2366. https://doi.org/10.1145/3534678.3539300 |
[41] | Road Traffic Safety Law of the People's Republic of China, The fifth meeting of the Standing Committee of the Tenth National People's Congress. |
[42] | M. S. S. Thomas, N. Kipf, P. Bloem, R. van den Berg, I. Titov, M. Welling, Modeling relational data with graph convolutional networks, in The Semantic Web, 10843(2018), 593–607. https://doi.org/10.1007/978-3-319-93417-4_38 |
1. | Humaira Mustanira Tariq, Rabia Hameed, Ghulam Mustafa, A Study on the Class of Non-Symmetric 3-Point Relaxed Quaternary Subdivision Schemes, 2022, 10, 2169-3536, 132164, 10.1109/ACCESS.2022.3230281 |