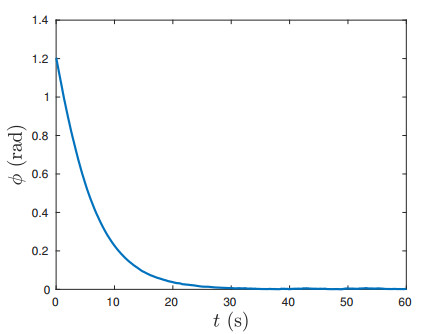
Citation: Mitchell Ratner, Chih-Chieh (Jason) Chiu. Portfolio Effects of VIX Futures Index[J]. Quantitative Finance and Economics, 2017, 1(3): 288-299. doi: 10.3934/QFE.2017.3.288
[1] | Álvaro Rodríguez Abella, Melvin Leok . Discrete Dirac reduction of implicit Lagrangian systems with abelian symmetry groups. Journal of Geometric Mechanics, 2023, 15(1): 319-356. doi: 10.3934/jgm.2023013 |
[2] | Elias Maciel, Inocencio Ortiz, Christian E. Schaerer . A Herglotz-based integrator for nonholonomic mechanical systems. Journal of Geometric Mechanics, 2023, 15(1): 287-318. doi: 10.3934/jgm.2023012 |
[3] | Valentin Duruisseaux, Melvin Leok . Time-adaptive Lagrangian variational integrators for accelerated optimization. Journal of Geometric Mechanics, 2023, 15(1): 224-255. doi: 10.3934/jgm.2023010 |
[4] | Xavier Rivas, Daniel Torres . Lagrangian–Hamiltonian formalism for cocontact systems. Journal of Geometric Mechanics, 2023, 15(1): 1-26. doi: 10.3934/jgm.2023001 |
[5] | Marcin Zając . The dressing field method in gauge theories - geometric approach. Journal of Geometric Mechanics, 2023, 15(1): 128-146. doi: 10.3934/jgm.2023007 |
[6] | Robert I McLachlan, Christian Offen . Backward error analysis for conjugate symplectic methods. Journal of Geometric Mechanics, 2023, 15(1): 98-115. doi: 10.3934/jgm.2023005 |
[7] | Markus Schlarb . A multi-parameter family of metrics on stiefel manifolds and applications. Journal of Geometric Mechanics, 2023, 15(1): 147-187. doi: 10.3934/jgm.2023008 |
[8] | Jacob R. Goodman . Local minimizers for variational obstacle avoidance on Riemannian manifolds. Journal of Geometric Mechanics, 2023, 15(1): 59-72. doi: 10.3934/jgm.2023003 |
[9] | Jordi Gaset, Arnau Mas . A variational derivation of the field equations of an action-dependent Einstein-Hilbert Lagrangian. Journal of Geometric Mechanics, 2023, 15(1): 357-374. doi: 10.3934/jgm.2023014 |
[10] | Alexander Pigazzini, Cenap Özel, Saeid Jafari, Richard Pincak, Andrew DeBenedictis . A family of special case sequential warped-product manifolds. Journal of Geometric Mechanics, 2023, 15(1): 116-127. doi: 10.3934/jgm.2023006 |
Spacecraft, underwater vehicles, aerial vehicles, and mobile robots require accurate knowledge of their orientation with respect to a known inertial frame. Typically, attitude estimators rely on the measurements of angular velocity and known inertial vectors in the body-fixed frame. Therefore, the rigid body attitude estimation problem using angular velocity and inertial vectors measurements in a body-fixed frame has been widely studied in past research. In practice, angular velocity is measured at a higher rate than inertial vectors. In this work*, we address the attitude estimation problem given multi-rate measurements of inertially fixed vectors and angular velocity by minimizing the "energy" stored in the state estimation errors.
*A preliminary arxiv version of this article is available at [1]
One of the earliest solutions to attitude determination from vector measurements is the TRIAD algorithm, used to determine the rotation matrix from two linearly independent inertial vector measurements [2]. In [3], Wahba presented the attitude determination problem as an optimization problem using three or more vector measurements where the cost function is the sum of the squared norms of vector errors. Various methods have been proposed in the literature to solve the Wahba's problem. Davenport was the first to reduce the Wahba's problem to finding the largest eigenvalue and the corresponding eigenvector of the so-called Davenport's K-matrix [4]. In a similar approach, Mortari presented the EStimator of the Optimal Quaternion (ESOQ) algorithm in[5], which provides the closed-form expressions of a 4×4 matrix's eigenvalues and then computes the eigenvector associated with the greatest of them, representing the optimal quaternion. The QUEST algorithm of [6] determines the attitude that achieves the best-weighted overlap of an arbitrary number of reference vectors. A Singular Value Decomposition (SVD) based method of solving the Wahba's problem was proposed by [7]. Markley also devised a Fast Optimal Matrix Algorithm (FOMA) to solve Wahba's problem in [8]. A coordinate-free framework of geometric mechanics was used in [9] to obtain a solution to Wahba's problem with robustness to measurement noise. [10] provides a numerical solution to Wahba's problem.
Attitude estimation methods based on minimizing "energy" stored in state estimation errors can be found in [11,12,13,14]. Prior research that has designed attitude estimation schemes based on geometric mechanics includes [15,16,17,18]. Comprehensive surveys of various attitude estimation methods are available in [19,20]. However, most of the aforementioned schemes for attitude estimation work only in continuous time or measurement rich environments and neglect the sparsity in the inertial vector measurements. Inertial vector measurements are usually obtained with the help of Sun (and star) sensors or magnetometers which are accurate but suffer from lower sampling rates. [21] provides one of the first solutions to rigid body attitude estimation with multi-rate measurements using uncertainty ellipsoids. A recursive method based on the cascade combination of an output predictor and an attitude observer can be found in [22]. An attitude estimation scheme on the Special Orthogonal group using intermittent body-frame vector measurements was presented in [23]. In [13], a filtering scheme in continuous-time is proposed by applying the Lagrange-d'Alembert principle on suitably formulated artificial kinetic and potential energy functions where the authors formulate filter equations assuming that inertial vector measurements and angular velocity measurements are available synchronously and continuously. A discrete-time estimation scheme in the presence of multi-rate measurements is proposed in [14]. This work presents the Lyapunov analysis but does not contain a variational interpretation.
The previous works in attitude estimations are presented in a continuous time domain and then discretized for numerical implementation. This voids the theoretical guarantee of asymptotic stability provided by the continuous-time estimator. Furthermore, most of the previous work in attitude estimation do not consider the realistic scenario when the inertial vectors and angular velocities are measured at a different rate. In the current work, we try to overcome these shortcoming by focusing on developing an asymptotically stable optimal geometric discrete-time attitude estimator based on the minimization of "energy" stored in the errors of the state estimators in the presence of multi-rate measurements. The measurements can be corrupted by noise and we do not assume any specific statistics (like normal distribution) on the measurement noise. However, the noise is assumed to be bounded. We represent the attitude as a rotation matrix which precludes potential singularity issues due to local coordinates (such as Euler angles). The multi-rate discrete-time filtering scheme presented here is obtained by applying the discrete-time Lagrange-D'Alembert principle [24] on a discrete-time lagrangian followed by a discrete-time Lyapunov analysis using a Lyapunov candidate that depends on the state estimation errors. The filtering scheme provided is asymptotically stable with an almost global region of convergence.
This paper is organized as follows. In the section 2, the attitude estimation problem is formulated as Wahba's optimization problem and then some important properties of the Wahba's cost function are presented. In the section 3, continuous-time rigid body attitude kinematics has been discretized and the propagation model for the measurements in the multi-rate measurement case is presented. Section 4 contains the application of variational mechanics to obtain a filter equation for attitude estimation. The filter equations obtained in the 4 are proven to be asymptotically stable with an almost global domain of convergence by deriving an appropriate dissipation torque using the discrete-time Lyapunov method in the 5. Filter equations are numerically verified with realistic measurements (corrupted by bounded noise) in the 6. Finally, 7 presents the concluding remarks with contributions and future work.
We define the trace inner product on Rm×n as
⟨A1,A2⟩:=trace(AT1A2). | (2.1) |
The group of orthogonal frame transformations on R3 is defined by O(3):={Q∈R3×3|det(Q)=±1}. The Special orthogonal group on R3 is denoted as SO(3) defined as SO(3):={R∈R3×3|RTR=RRT=I3}. The corresponding Lie algebra is denoted as so(3):={M∈R3×3|M+MT=0}. Let (⋅)×:R3→so(3)⊂R3×3 be the skew-symmetric matrix cross-product operator and denotes the vector space isomorphism between R3 and so(3):
v×=[v1v2v3]×=[0−v3v2v30−v1−v2v10]. | (2.2) |
Further, let vex(⋅):so(3)→R3 be the inverse of (⋅)×. exp(⋅):so(3)→SO(3) is the map defined as
exp(M)=∞∑i=01k!Mk. | (2.3) |
We define Ad:SO(3)×so(3)→so(3) as
AdRΩ×=RΩ×RT=(RΩ)×. | (2.4) |
In the rest of the article, the text "Consider the time interval [t0,T]⊆R+", indicates that the estimation process will be carried out for the time interval [t0,T] and the time interval is divided into N equal sub-intervals [ti,ti+1] for i=0,1,…,N with tN=T with time step size is denoted as, h:=ti+1−ti; unless specified otherwise. Furthermore, throughout this article we use (⋅)m to denote measured quantities. For example, if Ω is used to denote angular velocity, Ωm denotes measured value of the angular velocity.
For the attitude estimation, we consider k∈N known and linearly independent inertial vectors in the body-fixed frame. Let's denote these vectors in the body-fixed frame by umj∈R3 for j=1,…,k, where k≥2. Note, that k≥2 is necessary for determining the attitude uniquely. When k=2, the cross product of the two measured vectors is used as the third independent measurement. Let ej∈R3 be the corresponding known inertial vectors. We denote the true vectors in the body-fixed frame by uj:=RTej, where R is the rotation matrix of the body-fixed frame with respect to the inertial frame. This rotation matrix provides a coordinate-free global and unique description of the attitude of the rigid body. Define the matrix populated by all k measured vectors expressed in the body-fixed frame as column vectors as
Um=[um1um2um1×um2]∈R3×3whenk=2and,Um=[um1um2…umk]∈R3×kwhenk>2, | (2.5) |
and the corresponding inertial frame vectors as
E=[e1e2e1×e2]∈R3×3whenk=2and,E=[e1e2…ek]∈R3×kwhenk>2. | (2.6) |
The true body vector matrix is as below:
U=RTE=[u1u2u1×u2]∈R3×3whenk=2and,U=RTE=[u1u2…uk]∈R3×kwhenk>2. | (2.7) |
The optimal attitude determination problem using a set of vector measurements is finding an estimated rotation matrix ˆR∈SO(3), such that the weighted sum of squared norms of the vector errors
sj=ej−ˆRumj | (2.8) |
is minimized. This attitude determination problem is known as Wahba's problem and consists of minimizing
U(ˆR,Um)=12k∑j=1wj(ej−ˆRumj)T(ej−ˆRumj) | (2.9) |
with the respect to ˆR∈SO(3), and the weights wj>0 for all j∈{1,2,…,k}. we can express (2.9) as
U(ˆR,Um)=12⟨E−ˆRUm,(E−ˆRUm)W⟩, | (2.10) |
where Um is given by (2.5), E is given by (2.6), and W=diag(wi) is the positive definite diagonal matrix of the weight factors for the measured directions. W in (2.10) can be generalized to be any positive definite matrix. It is to be noted that, having different weights for different vector measurements is helpful as in general we can have some sensors that provide more accurate measurements than others. Therefore, it makes sense to provide more weight to those accurate measurements during attitude estimation.
This is an observer design that, like any other observer, can filter measurement noise from realistic measurements. As observer designs are based on perfect (deterministic) measurements for which their stability properties apply, it is useful to confirm these properties through numerical simulations. We have Um=U=RTE in the absence of measurement errors or noise. Let Q=RˆRT∈SO(3) denote the attitude estimation error. The following lemmas from [13] stated here without proof give the structure and characterization of critical points of the Wahba's cost function.
Lemma 2.1. Let rank(E) = 3 and the singular value decomposition of E be given by
E:=UEΣEVTEwhereUE∈O(3),VE∈SO(m).ΣE∈Diag+(3,m), | (2.11) |
and Diag+(n1,n2) is the vector space of n1×n2 matrices with positive entries along the main diagonal and all the other components zero. Let σ1,σ2,σ3 denote the main diagonal entries of ΣE. Further, Let W from (2.10) be given by
W=VEW0VTEwhereW0∈Diag+(m,m) | (2.12) |
and the first three diagonal entries of W0 are given by
w1=d1σ21,w2=d2σ22,w3=d3σ23whered1,d2,d3>0. | (2.13) |
Then, K=EWET is positive definite and
K=UEΔUTEwhereΔ=diag(d1,d2,d3) | (2.14) |
is its eigen-decomposition. Moreover, if di≠dj for i≠j and i,j∈{1,2,3} then ⟨I−Q,K⟩ is a Morse function whose set of critical points given as the solution of SK(Q):=vex(KQ−QTK)=0:
CQ:={I,Q1,Q2,Q3}whereQi=2UEaiaTiUTE−I | (2.15) |
and ai is the ith column vector of the identity matrix I∈SO(3).
Lemma 2.2. Let K=EWET have the properties given by lemma 2.1. Then the map SO(3)∋Q↦⟨I−Q,K⟩∈R with critical points given by (2.15) has a global minimum at the identity I∈SO(3), a global maximum and two hyperbolic saddle points whose indices depend on the distinct eigenvalues d1,d2, and d3 of K.
Consider the time interval [t0,T]⊆R+. Let the true angular velocity in the body-fixed frame be denoted by Ω∈R3. The true and measured angular velocities at the time instant ti will be denoted by Ωi and Ωmi respectively. Further, let Ui and Umi denote the matrix formed by true and measured inertial vectors in the body-fixed frame at the time instant ti respectively. The assumption is that angular velocity measurements and inertial vectors measurements in the body-fixed frame are coming at a different but constant rate. In general coarse rate gyros have much higher sampling rate than that of a coarse attitude sensor. Therefore, in a realistic scenario, angular velocities are measured at a higher rate than the inertial vector measurements in the body-fixed frame. Therefore, we assume that the measurements of angular velocity (Ωm) are available after each time interval h say, Ωm0,Ωm1,…,ΩmN while, inertial vector measurements in the body-fixed frame are available after time interval nh,n∈N say, Um0,Umn,Um2n,….
We have, U=RTE. Therefore, at time instants ti and ti+1, the following relations will hold true respectively; Ui=RTiEi,Ui+1=RTi+1Ei+1. Here, Ri and Ri+1 are the rotation matrices from body-fixed frame to inertial frame at time instants ti and ti+1 respectively. Ei=Ei+1=E are the corresponding known vectors expressed in the inertial frame. Note that the vectors are fixed in the inertial frame and do not change with the time. The continuous time attitude kinematics are,
˙R=RΩ×. | (3.1) |
We discretize the kinematics in (3.1) as follows,
Ri+1=Riexp(h2(Ωi+1+Ωi)×), | (3.2) |
where, exp(⋅):so(3)↦SO(3) is the map defined as,
exp(M)=∞∑k=01k!Mk. | (3.3) |
Using (2.7) and the discretization from (3.2) we get,
Ui+1=exp(−h2(Ωi+1+Ωi)×)RTiEi=exp(−h2(Ωi+1+Ωi)×)Ui. | (3.4) |
For the instants of time when inertial vector measurements in the body-fixed frame are not available we will use (3.4) to obtain the missing values of Umi. This implies that for the time instants (n−1)h<ti<nh,n∈N, by employing the propagation scheme in (3.4), we propagate direction vector measurements between the instants at which they are measured, using the angular velocity measurements that are obtained at a faster rate. We now formalize the aforementioned inertial vector measurement model as below,
˜Umi:={Umi,ifimodn=0exp(−h2(Ωmi−1+Ωmi)×)˜Umi−1,otherwise. | (3.5) |
Note that in the absence of measurements errors, we have Ωmi=Ωi,∀i∈{0,1,…,N}. Also, Umi=Ui for the time instants when inertial vector measurements are available. Now, at time instant t0, we have ˜Um0=Um0=U0 and Ωm0=Ω0. Using (3.5) at time instant t1, noting that Ωm1=Ω1, we get ˜Um1=exp(−h2(Ω0+Ω1)×)U0. Comparing it with (3.4), we have ˜Um1=U1. Using the relation from (2.7) we have ˜Um1=RT1E1. Similarly, combining (3.4), and (3.5), and using the relation in (2.7) we get the following relation for all i∈{0,1,…,N} in the absence of measurement errors:
˜Umi=RTiEi. | (3.6) |
Consider the time interval [t0,T]⊆R+. Let the true angular velocity in the body-fixed frame be denoted by Ω∈R3. The true and measured angular velocities at the time instant ti will be denoted by Ωi and Ωmi respectively. It is assumed that a measurement Um0 is available at time instant t0. Now the next measurement will be available after a time interval nh where n is an integer variable taking values between n1 and n2 which are user provided. Note that since n is varying, that sampling rate of inertial vector sensor is not fixed but varying over time. Considering the discrete kinematics of R and U from (3.2) and (3.4) we propose the following discrete-time vector measurement model,
˜Umi:={Umi,ifmeasurement availableexp(−h2(Ωmi−1+Ωmi)×)˜Umi−1,otherwise. | (3.7) |
Note that now we have obtained values of Ωmi and ˜Umi for each time instant ti and similar to the previous arguments, it can be proven that in the absence of measurement errors, we have Ωmi=Ωi and ˜Umi=RTiEi.
Again consider the time interval [t0,T]⊆R+. Let the angular velocity measurements Ωm∈R3 be available after each time interval of h. Now suppose the inertial vector measurements are available after a time interval rh where r=n1/n2 is a rational number with n1,n2∈N. Let n be the greatest common factor of n1 and n2. Let h′=nh/n2. Now, consider the time interval [t0,T]⊆R+ divided into N′ equal sub-intervals [t′i,t′i+1] for i=0,1,…,N′ with t′N=T and let t′i+1−t′i=h′. Note that a measurement Ωm will be available after a time interval of n2h′/n and a measurement Um will be available after time interval rh=n1n2×n2nh′=n1h′/n.
We define the measurement model for Ωm as
˜Ωmi:={Ωmi,ifimodn2/n=0Ωmi−1,otherwise. | (3.8) |
for all i=0,1,…,N′. Now we can define the vector measurement model to be,
˜Umi:={Umi,ifimodn1/n=0exp(−h2(Ωmi−1+Ωmi)×)˜Umi−1,otherwise. | (3.9) |
Note that the division of the time interval [t0,T] with the time step size of h′ is finer than that of with the time step size of h with the relation as h=n2nh′. Therefore for each i∈{0,1,…,N} we have j=n2ni∈{0,1,…,n′} such that ti=t′j. Redefining Ωmi:=˜Ωmn2ni, and ˜Umi=˜Umn2ni for all i∈{0,1,…,N}. Note that now we have obtained values of Ωmi and ˜Umi for each time instant ti and similar to previous arguments, it can be proven that in the absence of measurement errors, we have Ωmi=Ωi and ˜Umi=RTiEi.
Observe that in any of the aforementioned three cases, the measurement models provide values Ωmi and ˜Umi for all the discrete-time instants ti,i∈{0,1,…,N} in the time interval [t0,T].
The value of the Wahba's cost function at each instant encapsulates the error in the attitude estimation. We can consider the Wahba's cost function as an artificial potential energy-like term. Therefore using (2.10) we have
Ui=U(ˆRi,˜Umi)=12⟨Ei−ˆRi˜Umi,(Ei−ˆRi˜Umi)Wi⟩, | (4.1) |
where ˜Umi is according to the inertial vector propagation model presented in section 3. The term encapsulating the "energy" in the angular velocity estimation error is denoted by the map Tv defined as
Tvi:=Tv(ˆΩi,Ωmi,ˆΩi+1,Ωmi+1):=m2(Ωmi+Ωmi+1−ˆΩi−ˆΩi+1)T(Ωmi+Ωmi+1−ˆΩi−ˆΩi+1), | (4.2) |
where m>0 is a scalar and ˆΩi stands for the value of estimated angular velocity at the discrete time instant ti. We can write (4.2) in terms of the angular velocity estimation error ωi:=Ωmi−ˆΩi as
Tv(ωi,ωi+1)=m2(ωi+ωi+1)T(ωi+ωi+1). | (4.3) |
The discrete time Lagrangian can be written as:
L(ˆRi,˜Umi,ωi,ωi+1)=Tv(ωi,ωi+1)−U(ˆRi,˜Umi)=m2(ωi+ωi+1)T(ωi+ωi+1)−12⟨Ei−ˆRi˜Umi,(Ei−ˆRi˜Umi)Wi⟩. | (4.4) |
We use variational mechanics [25,26] approach to obtain an optimal estimation scheme for the constructed Lagrangian. In the absence of a dissipative term, the variational approach would result in (a generalization to the Lie group of) the Euler-Lagrange equations that we obtain in the context of optimal control. The generalization would be an Euler-Poincare equation on SO(3) [27]. Therefore, if the estimation process is started at time t0, then the discrete-time action functional corresponding to the discrete-time Lagrangian (4.4) over the time interval [t0,T] can be expressed as
sd(L(ˆRi,˜Umi,ωi,ωi+1))=hN∑i=0(L(ˆRi,˜Umi,ωi,ωi+1))=hN∑i=0{m2(ωi+ωi+1)T(ωi+ωi+1)−12⟨Ei−ˆRi˜Umi,(Ei−ˆRi˜Umi)Wi⟩}. | (4.5) |
Consider attitude state estimation in discrete-time in the presence of multirate measurements with noise and initial state estimate errors. Applying the discrete-time Lagrange-d'Alembert principle [24] from variational mechanics to the action functional sd(L(ˆRi,˜Umi,ωi,ωi+1)) given by (4.5), in the presence of a dissipation term on ωi=Ωmi−ˆΩi, leads to the following attitude and angular velocity filtering scheme.
Proposition 1. Consider the time-interval [t0,T]. Consider the multi-rate measurement models from Section 3 such that we have values Ωmi and ˜Umi for all the discrete-time instants ti,i∈{0,1,…,N} in the time interval [t0,T]. Let the Wi be chosen such that Ki=EiWiETi satisfies eigen-decomposition condition (2.14) of lemma 2.1. Also, let τDi∈R3 denote the value of the dissipation torque at the time instant ti. A discrete-time optimal filter obtained by applying the discrete-time Lagrange-d'Alembert principle would be as follows:
{ˆRi+1=ˆRiexp(h2(ˆΩi+1+ˆΩi)×)m(ωi+2+ωi+1)=exp(−h2(ˆΩi+2+ˆΩi+1)×){m(ωi+1+ωi)+h2SLi+1(ˆRi+1)−h2τDi+1}ˆΩi=Ωmi−ωi, | (4.6) |
where SLi(ˆRi)=vex(LTiˆRi−ˆRTiLi)∈R3, Li=EiWi(˜Umi)T, and (ˆR0,ˆΩ0)∈SO(3)×R3×3 are initial estimated states.
Proof. Consider a first variation in the discrete attitude estimate as
δˆRi=ˆRiΣ×i, | (4.7) |
where Σi∈R3 represents a variation for the discrete attitude estimate. For fixed end-point variations, we have Σ0=ΣN=0. A first order approximation is to assume that ˆΩ× and δˆΩ× commute. Taking the first variation of the discrete-time attitude kinematics according to the first equation of (4.6) and comparing with (4.7) we get
δˆRi+1=δˆRiexp(h2(ˆΩi+1+ˆΩi)×)+h2ˆRiexp(h2(ˆΩi+1+ˆΩi)×)δ(ˆΩi+1+ˆΩi)×=ˆRi+1Σ×i+1. | (4.8) |
(4.8) can be rearranged to
ˆRi+1h2δ(ˆΩi+1+ˆΩi)×=ˆRi+1Σ×i+1−ˆRi+1Adexp(−h2(ˆΩi+1+ˆΩi)×)Σ×i⇒h2δ(ˆΩi+1+ˆΩi)×=Σ×i+1−Adexp(−h2(ˆΩi+1+ˆΩi)×)Σ×i. | (4.9) |
(4.9) can be equivalently written as an equation in R3 as follows:
h2δ(ˆΩi+1+ˆΩi)=Σi+1−exp(−h2(ˆΩi+1+ˆΩi)×)Σi. | (4.10) |
Note that, ωi=Ωmi−ˆΩi gives us
δ(ωi+1+ωi)=−δ(ˆΩi+1+ˆΩi). | (4.11) |
Consider artificial potential energy term as expressed in (4.1). Taking its first variation with the respect to estimated attitude ˆR, we get
δUi=12{⟨−δˆRi˜Umi,(Ei−ˆRi˜Umi)Wi⟩+⟨Ei−ˆRi˜Umi,(−δˆRi˜Umi)Wi⟩}=⟨−δˆRi˜Umi,(Ei−ˆRi˜Umi)Wi⟩=⟨−ˆRiΣ×i,(Ei−ˆRi˜Umi)Wi⟩=trace((˜Umi)TΣ×iˆRTi(Ei−ˆRi˜Umi)Wi)=trace((Σ×i)T˜UmiWiETiˆRi)=⟨Σ×i,˜UmiWiETiˆRi⟩=12⟨Σ×,˜UmiWiETiˆRi−ˆRTiEiWi(˜Umi)T⟩=12⟨Σ×i,LTiˆRi−ˆRTiLi⟩=STLi(ˆRi)Σi. | (4.12) |
Consider the first variation in the artificial kinetic energy Tv(ωi,ωi+1) as in (4.3) with the respect to the angular velocity estimation error,
δTvi=m(ωi+ωi+1)Tδ(ωi+ωi+1). | (4.13) |
Using the results in (4.10) and (4.11) we get
δTvi=−m(ωi+ωi+1)Tδ(Ωi+Ωi+1)=2hm(ωi+ωi+1)T(exp(−h2(ˆΩi+1+ˆΩi)×)Σi−Σi+1). | (4.14) |
The first variation of the discrete-time action sum in (4.5) using (4.12) and (4.14) can be written as
δsd=hN∑i=0{δTvi−δUi}=N∑i=0{2m(ωi+ωi+1)Texp(−h2(ˆΩi+1+ˆΩi)×)Σi−2m(ωi+ωi+1)TΣi+1−hSTLi(ˆRi)Σi}. | (4.15) |
Applying the discrete-time Lagrange-d'Alembert principle to the attitude motion, we obtain
δsd+hN−1∑i=0τTDiΣi=0⇒N−1∑i=0{2m(ωi+ωi+1)T[exp(−h2(ˆΩi+1+ˆΩi)×)Σi−Σi+1]−hSTLi(ˆRi)Σi+hτTDiΣi}=0. | (4.16) |
For 0≤i<N, (4.16) leads to
2m(ωi+2+ωi+1)Texp(−h2(ˆΩi+2+ˆΩi+1)×)−2m(ωi+1+ωi)T−hSTLi+1(ˆRi+1)+hτTDi+1=0⇒2mexp(−h2(ˆΩi+2+ˆΩi+1)×)(ωi+2+ωi+1)=2m(ωi+1+ωi)+hSLi+1(ˆRi+1)−hτDi+1, | (4.17) |
which in turn leads to the second filter equation.
Remark 1. Please note that, we introduced a virtual dissipation torque in the Proposition 1 and the performance of the estimator will depend on the value of dissipation torque. In the next section, we determine the value of dissipation torque via discrete-Lyapunov analysis so that the resulting estimator is almost globally asymptotically stable.
For the Lyapunov stability of the filter equations, we need to construct a suitable Lyapunov candidate function. We use the Wahba's cost function expressed in (4.1) as the artificial potential energy which encapsulates the error in the estimation of attitude. A new term encapsulating the "energy" in the angular velocity estimation error can be constructed as the map Tl:R3×R3→R defined as
Tli:=Tl(ˆΩi,Ωmi):=m2(Ωmi−ˆΩi)T(Ωmi−ˆΩi), | (5.1) |
where m>0 is a scalar same as before. Further, (5.1) can be written in terms of angular velocity estimation error, ωi:=Ωmi−ˆΩi as follows:
Tl(ωi)=m2(ωi)T(ωi). | (5.2) |
In the absence of measurement errors, we have ˜Umi=RTiEi. Therefore we can we can write (4.1) in terms of state estimation error Qi=RiˆRTi as
U(ˆRi,˜Umi)=12⟨Ei−ˆRiRTiEi,(Ei−ˆRiRTiEi)Wi⟩=⟨I−RiˆRTi,EiWiETi⟩⇒Ui=U(Qi)=⟨I−Qi,Ki⟩whereKi=EiWiETi. | (5.3) |
The weights Wi's are chosen such that Ki is always positive definite with distinct eigenvalues according to lemma 2.1.
Theorem 5.1. Consider the time-interval [t0,T]. Consider the multi-rate measurement models from Section 3 such that we have values Ωmi and ˜Umi for all the discrete-time instants ti,i∈{0,1,…,N} in the time interval [t0,T]. Then the estimation scheme in Proposition 1 with the following value of the dissipation torque:
τDi+1=1h{2m(ωi+1+ωi)+hSLi+1(ˆRi+1)−2mm+lexp(h2(ˆΩi+2+ˆΩi+1)×)[2mωi+1+kphSLi+1(ˆRi+1)]}, | (5.4) |
leads to the estimation scheme
{ωi+1=1m+l[(m−l)ωi+kphSLi(ˆRi)]ˆΩi=Ωmi−ωiˆRi+1=ˆRiexp(h2(ˆΩi+1+ˆΩi)×), | (5.5) |
where SLi(ˆRi)=vex(LTiˆRi−ˆRTiLi)∈R3, Li=EiWi(˜Umi)T, l>0, l≠m and kp>0, which is asymptotically stable at the estimation error state (Q,ω):=(I,0) (Qi=RiˆRTi) in the absence of measurement errors. Further, the domain of attraction of (I,0) is a dense open subset of SO(3)×R3.
Proof. Using the third equation from (5.5) we have
Qi+1=Ri+1ˆRTi+1=QiˆRiexp(h2(ωi+1+ωi)×)ˆRTi. | (5.6) |
We choose the following discrete-time Lyapunov candidate:
Vi:=V(Qi,ωi):=kpUi+Tli, | (5.7) |
where kp>0 is a constant.
The stability of the attitude and angular velocity estimation error can be shown by analyzing ΔVi=kpΔUi+ΔTli.
Assuming Ki to be constant and letting K=Ki=Ki+1 we obtain
ΔUi=Ui+1−Ui=⟨I−Qi+1,K⟩−⟨I−Qi,K⟩ΔUi=⟨Qi−Qi+1,K⟩=−⟨ΔQi,K⟩, | (5.8) |
where ΔQi=Qi+1−Qi. Therefore,
ΔQi=Qi+1−Qi=Qi[ˆRiexp(h2(ωi+1+ωi)×)ˆRTi−I]. | (5.9) |
Considering the first order expansion of exp(h2(ˆωi+1+ˆωi)×) as
exp(h2(ωi+1+ωi)×)≈I+h2(ωi+1+ωi)×, | (5.10) |
we have
ΔQi=Qi[ˆRi(I+h2(ωi+1+ωi)×)ˆRTi−I]=h2Qi(ˆRi(ωi+1+ωi)׈RTi)=h2Qi(ˆRi(ωi+1+ωi))×. | (5.11) |
It has to be noted that approximation in (5.10) is accurate for small values of h and may affect the stability results for very high values of h. In the absence of measurement errors, we have ˜Umi=RTiEi. Therefore, it follows that
ΔUi=−h2⟨Qi(ˆRi(ωi+1+ωi))×,K⟩=−h2⟨Ri(ωi+1+ωi)׈RTi,EiWiETi⟩=−h2⟨(ωi+1+ωi)׈RTi,RTiEiWiETi⟩=−h2⟨(ωi+1+ωi)׈RTi,˜UmiWiETi⟩, | (5.12) |
and noting that Li=EiWi(˜Umi)T, we get
ΔUi=−h2⟨(ωi+1+ωi)×,LTiˆRi⟩=−h4⟨(ωi+1+ωi)×,LTiˆRi−ˆRTiLi⟩=−h2(ωi+1+ωi)TSLi(ˆRi). | (5.13) |
Similarly we can compute the change in the kinetic energy as follows:
ΔTli=Tl(ωi+1)−Tl(ωi)=(ωi+1+ωi)Tm2(ωi+1−ωi)ΔTli=(ωi+1+ωi)Tm2(ωi+1−ωi). | (5.14) |
The change in the value of the candidate Lyapunov function can be computed as,
ΔVi=Vi+1−Vi=ΔTi+kpΔUi=12(ωi+1+ωi)T(m(ωi+1−ωi)−kphSLi(ˆRi)). | (5.15) |
Similarly, we obtain
ΔVi+1=12(ωi+2+ωi+1)T(m(ωi+2−ωi+1)−kphSLi+1(ˆRi+1)). | (5.16) |
Substituting the value of ωi+2 from the filtering scheme presented in Proposition 1 we get
ΔVi+1=12(ωi+2+ωi+1)T{exp(−h2(ˆΩi+2+ˆΩi+1)×){m(ωi+1+ωi)+hSLi+1(ˆRi+1)−hτDi+1}−2mωi+1−kphSLi+1(ˆRi+1)}. | (5.17) |
Now, for ΔV to be negative definite for all i we require
exp(−h2(ˆΩi+2+ˆΩi+1)×){m(ωi+1+ωi)−h2τDi+1+h2SLi+1(ˆRi+1)}−2mωi+1−kphSLi+1(ˆRi+1)=−l(ωi+2+ωi+1), | (5.18) |
where l>0,l≠m, and ΔVi+1 simplifies to
ΔVi+1=−l2(ωi+2+ωi+1)T(ωi+2+ωi+1). | (5.19) |
Substituting ωi+2 from the third equation presented in Proposition 1 into (5.18),
exp(−h2(ˆΩi+2+ˆΩi+1)×){m(ωi+1+ωi)−h2τDi+1+h2SLi+1(ˆRi+1)}−2mωi+1−kphSLi+1(ˆRi+1)=−lmexp(−h2(ˆΩi+1+ˆΩi)×){m(ωi+1+ωi)−h2τDi+1+h2SLi+1(ˆRi+1)}, | (5.20) |
which further simplifies to,
m+lmexp(−h2(ˆΩi+2+ˆΩi+1)×){m(ωi+1+ωi)−h2τDi+1+h2SLi+1(ˆRi+1)}=2mωi+1+kphSLi+1(ˆRi+1), | (5.21) |
which upon simple manipulations yields (5.4). It can be seen that after substituting (5.4) into Proposition 1, we obtain
ωi+2=1m+l[(m−l)ωi+1+kphSLi+1(ˆRi+1)]. | (5.22) |
(5.22) can also be rewritten as
ωi+1=1m+l[(m−l)ωi+kphSLi(ˆRi)], | (5.23) |
in terms of ωi,ωi+1 and SLi(ˆRi). From (5.19), ΔVi can be written as
ΔVi=−l2(ωi+1+ωi)T(ωi+1+ωi). | (5.24) |
We employ the discrete-time La-Salle invariance principle from [28] considering our domain (SO(3)×R3) to be a subset of R12. We use Theorem 6.3 and Theorem 7.9 from Chapter-1 of [28]. For this we first compute E:={(Qi,ωi)∈SO(3)×R3|ΔVi(Qi,ωi)=0}={(Qi,ωi)∈SO(3)×R3|ωi+1+ωi=0}. From (5.6), ωi+1+ωi=0 implies that
Qi+1=Qi. | (5.25) |
Also, from (5.13) we have ΔU=0 whenever ωi+1+ωi=0. This implies that the potential function, which is a Morse function according to lemma 2.1, is not changing and therefore has converged to one of its stationary points. Stationary points of the Morse function ⟨I−Q,K⟩ are characterized as the solutions of
SK(Qi)=0⇒vex(KQi−QTiK)=0⇒KQi=QTiK. | (5.26) |
Now, Li=EiWi(˜Umi)T=EiWi(RTiEi)T=(EiWiETi)Ri=KRi, which further gives us
(SLi(ˆRi))×=LTiˆRi−ˆRTiLi=RTiKˆRi−ˆRTiKRi. | (5.27) |
Multiplying (5.27) from the right hand side by ˆRTi and from the left hand side by ˆRi,
ˆRi(SLi(ˆRi))׈RTi=ˆRiRTiK−KRiˆRTi=QTiK−KQi. | (5.28) |
At the critical points from (5.26), the right side of the above expression vanishes. Therefore, as ˆRi is an orthogonal matrix, the following holds true at the critical points:
(SLi(ˆRi))×=0⇒SLi(ˆRi)=0. | (5.29) |
Substituting this information in (5.23) yields,
ωi+1=1m+l(m−l)(ωi). | (5.30) |
Now if, ωi+1+ωi=0, we have
2mm+lωi=0⇒ωi=ωi+1=0. | (5.31) |
This leads to the conclusion that the set of estimation errors, E={(Qi,ωi)∈SO(3)×R3|Qi∈CQ,ωi=0}, is the largest invariant set for the estimation error dynamics, and we obtain M=E={(Qi,ωi)∈SO(3)×R3|Qi∈CQ,ωi=0}. Therefore, we obtain the positive limit set as the set,
I:=M∩V−1i(0)={(Q,ω)∈SO(3)×R3|Q∈CQ,ω=0}. | (5.32) |
In the absence of measurement errors, all the solutions of this filter converge asymptotically to the set I. More specifically, the attitude estimation error converges to the set of critical points of ⟨I−Q,K⟩. The unique global minimum of this function is at (Q,ω)=(I,0) from lemma 2.2. Therefore, (Q,ω)=(I,0) is locally asymptotically stable. The remainder of this proof is similar to the last part of the proof of stability of the variational attitude estimator in [13].
Consider the set,
C=I∖(I,0) | (5.33) |
which consists of all the stationary states that the estimation errors may converge to, besides the desired estimation error state (I,0). Note that all states in the stable manifold of a stationary state in C will converge to this stationary state. From the properties of the critical points Qi∈CQ∖(I) of Φ(⟨K,I−Q⟩) given in lemma 2.2. we see that the stationary points in I∖(I,0)={(Qi,0):Qi∈CQ∖(I)} have stable manifolds whose dimensions depend on the index of Qi. Since the angular velocity estimate error ω converges globally to the zero vector, the dimension of the stable manifold MSi of (Qi,0)∈SO(3)×R3 is
dim(MSi)=3+(3−index of Qi)=6−index of Qi. | (5.34) |
therefore, the stable manifolds of (Q,ω)=(Qi,0) are three-dimensional, four dimensional, or five-dimensional, depending on the index of Qi∈CQ∖(I) according to (5.34). Moreover, the value of the Lyapunov function V(Qi,ωi) is non decreasing (increasing when (Qi,ωi)∉I) for trajectories on these manifolds when going backwards in time. This implies that the metric distance between error states (Q,ω) along these trajectories on the stable manifolds MSi grows with the time separation between these states, and this property does not depend on the choice of the metric on SO(3)×R3. Therefore, these stable manifolds are embedded (closed) sub-manifolds of SO(3)×R3 and so is their union. Clearly, all states starting in the complement of this union, converge to the stable equilibrium (Q,ω)=(I,0); therefore the domain of attraction of this equilibrium is,
DOA(I,0)=SO(3)×R3∖{∪3i=1MSi} | (5.35) |
which is a dense open subset of SO(3)×R3.
This section presents numerical simulation results of the discrete-time estimator presented in the section 5. The rigid body is assumed to have an initial attitude and angular velocity given by,
R0=expmSO(3)((π4×[47,27,57]T)×), |
andΩ0=π60×[−1.2,2.1,−1.9]Trad/s. |
W is selected based on the measured set of inertial vectors E at each instant such that it satisfies lemma 2.1. Initially estimated states have the following initial estimation errors:
Q0=expmSO(3)((π2.5×[47,27,57]T)×), |
andω0=[0.001,−0.002,0.003]Trad/s. |
It has been assumed that there are at most 9 inertially known directions that are being measured by the sensors attached to the rigid body. The number of observed direction can vary randomly between 2 to 9 at each time instant. In the case where the number of observed directions is 2, the cross product of the two measurements is used as the third measurement. The standard rigid body dynamics are used to produce true states of the rigid body by applying sinusoidal forces. The observed directions in the body-fixed frame are simulated with the help of the aforementioned true states. The true quantities are disturbed by bounded, random noise with zero mean to simulate realistic measurements. Based on coarse attitude sensors like sun sensors and magnetometers, a random noise bounded in magnitude by 2.4∘ is added to the matrix U=RTE to generate measured Um. Similarly, a random noise bounded in magnitude by 0.97∘/s, which is close to real noise levels of coarse rate gyros, is added Ω to generate measured Ωm.
The estimator is simulated over a time interval of T = 60s, with a step-size of h=0.01s. The inertial scalar gain is m=1.5 and the dissipation term is chosen to be l=0.3. The difference of sampling rate between measurements of angular velocity and measurements inertial vectors in body-fixed frame is taken to be n=10. Furthermore, the value of gain kp is chosen to be kp=1. The principle angle ϕ of the rigid body's attitude estimation error Q is shown in the Figure 1. Components of estimation error ω in the rigid body's angular velocity are shown in Figure 2.
The estimator is simulated over a time interval of T = 60s, with a step-size of h=0.01s. The inertial scalar gain is m=1.5 and the dissipation term is chosen to be l=0.3. The difference of sampling rate between measurements of angular velocity and measurements inertial vectors in body-fixed frame is to varying randomly but bounded between 10 and 30. Furthermore, the value of gain kp is chosen to be kp=1. The principle angle ϕ of the rigid body's attitude estimation error Q is shown in the Figure 3. Components of estimation error ω in the rigid body's angular velocity are shown in Figure 4.
The estimator is simulated over a time interval of T = 60s, with a step-size of h=0.008s. The inertial scalar gain is m=2.5 and the dissipation term is chosen to be l=0.5. The assumption is that the angular velocity measurements are available after each time interval of 0.008s and inertial vector measurements are available after each time interval of 0.05s. Furthermore, the value of gain kp is chosen to be kp=10. The principle angle ϕ of the rigid body's attitude estimation error Q is shown in the Figure 5. Components of estimation error ω in the rigid body's angular velocity are shown in Figure 6.
We have discussed the performance of the estimator in various scenarios but with the fixed values of initial estimation errors. It remains to see whether the estimator perform good in case of large initial estimation errors. For this part, we restrict our attention to the Case-2 i.e., when the difference of sampling rate between measurement of angular velocity and measurement of inertial vectors is time varying and bounded between 10 and 30. We check the performance of the estimator for three different values of initial estimation errors in angular velocity and attitude. The results of the same are plotted in Figure 7.
On comparing Figures 7a and 7b, when the initial estimation error in principle angle of attitude estimation is increased to about 2.5 times and estimation error in angular velocity is increased to 10 times, convergence takes about 10s more time. However, on comparing Figure 7a and 7c, it can be see that if the initial attitude estimation error is fixed then change in initial angular velocity estimation error(100 times the original values) has no effect on the convergence time. Therefore, it can be concluded that increasing the initial attitude estimation error can increase the convergence time but increase in initial angular velocity estimation error has no effect on the convergence time.
In this section, we discuss how convergence time can be decreased by increasing the value of gain. Same as before, we restrict our attention to the Case-2 i.e., when the difference of sampling rate between measurement of angular velocity and measurement of inertial vectors is time varying and bounded between 10 and 30. We consider very high errors in initial estimation errors and plot the performance of the estimator for three different values of gain kp in Figure 8. As it can be seen, increasing the value of gain significantly improves the convergence time.
We develop a geometric attitude and angular velocity estimation scheme using the discrete-time Lagrange-D'Alembert principle followed by discrete-time Lyapunov stability analysis in the presence of multi-rate measurements. The attitude determination problem from two or more vector measurements in the body-fixed frame is formulated as Wahba's optimization problem. To overcome the multi-rate challenge, a discrete-time model for attitude kinematics is used to propagate the inertial vector measurements forward in time. The filtering scheme is obtained with the aid of appropriate discrete-time Lagrangian and Lyapunov functions consisting of Wahba's cost function as an artificial potential term and a kinetic energy-like term that is quadratic in the angular velocity estimation error. As it can be observed, the Lyapunov function is not constructed from the same artificial potential and kinetic energy terms that are used for constructing the Lagrangian. There are mainly two reasons behind this; 1) the filtering scheme obtained by applying the discrete Lagrange-d'Alembert principle is implicit in nature and therefore it can increase computational load and runtime making it difficult to use for real-time applications. Therefore, an explicit filtering scheme is more desirable, 2) we also need the filtering scheme to be asymptotically stable. A Lyapunov function, different from system energy constructed appropriately, helps us meet both the requirements. The explicit filtering scheme obtained after the Lyapunov analysis was proven to be asymptotically stable in the absence of measurement noise and the domain of convergence is proven to be almost global. Numerical simulations were carried out with realistic measurement data corrupted by bounded noise. Numerical simulations verified that the estimated states converge to a bounded neighborhood of (I,0). Furthermore, the rate of convergence of the estimated states to the real state can be controlled by choosing appropriate gains. Future endeavors are towards obtaining a discrete-time optimal attitude estimator in the presence of multi-rate measurements when there a constant or slowly time-varying bias in the measurements of angular velocity while also obtaining a bound on the state estimation errors when there is measurement noise in the inertial vector measurements and the angular velocity measurements.
All authors hereby declare that there are no conflicts of interest in this paper.
[1] | Baur D, Lucey B (2010) Is Gold a Hedge or a Safe Haven? An Analysis of Stocks, Bonds and Gold. Financ Rev 45: 217–229. |
[2] | Eun C, Lee J (2010) Mean-variance convergence around the world. J Bank Financ 34: 856–870. |
[3] |
Forbes K, Rigobon R (2002) No contagion, only interdependence. J Financ 57: 2223–2261. doi: 10.1111/0022-1082.00494
![]() |
[4] |
Markwat T, Kole E, Van Dijk D (2009) Contagion as a domino effect in global stock markets. J Bank Financ 33: 1996–2012. doi: 10.1016/j.jbankfin.2009.05.008
![]() |
[5] | Brenner M, Galai D (1989) New Financial Instruments for Hedging Changes in Volatility. Financ Anal J 45: 61–65. |
[6] |
Whaley R (1993) Derivatives on Market Volatility: Hedging Tools Long Overdue. J Deriv 1: 71–84. doi: 10.3905/jod.1993.407868
![]() |
[7] | Whaley R (2009) Understanding the VIX. J Portf Manag 35: 98–105. |
[8] | Daigler R, Rossi L (2006) A Portfolio of Stocks and Volatility. J Invest 15: 99–106. |
[9] | Moran M, Dash S (2007) VIX Futures and Options: Pricing and Using Volatility Products to Manage Downside Risk and Improve Efficiency in Equity Portfolios. J Trading 2: 965–105. |
[10] |
Szado E (2009) VIX Futures and Options-A Case Study of Portfolio Diversification During the 2008 Financial Crisis. J Altern Invest 12: 68–85. doi: 10.3905/JAI.2009.12.2.068
![]() |
[11] |
Chen H, Chung S, Ho K (2011) The diversification effects of volatility-related assets. J Bank Financ 35: 1179–1189. doi: 10.1016/j.jbankfin.2010.09.024
![]() |
[12] |
Warren G (2012) Can Investing in Volatility Help Meet Your Portfolio Objectives? J Portf Manag 38: 82–98. doi: 10.3905/jpm.2012.38.2.082
![]() |
[13] | Deng G, McCann C, Wang O (2012) Are VIX futures ETPs effective hedges? J Index Invest 3: 35–48. |
[14] |
Goltz F, Stoyanov S (2013) The risks of volatility ETNs: a recent incident and underlying issues. J Index Invest 4: 73–81. doi: 10.3905/jii.2013.4.2.073
![]() |
[15] | Hill J (2013) The Different Faces of Volatility Exposure in Portfolio Management. J Altern Invest 15: 9–31. |
[16] |
Whaley R (2013) Trading volatility: At what cost? J Portf Manag 40: 95–108. doi: 10.3905/jpm.2013.40.1.095
![]() |
[17] | Alexander C, Korovilas D (2012) Understanding ETNs on VIX Futures. SSRN research paper: http://dx.doi.org/10.2139/ssrn.2043061. |
[18] | Campasano J, Linn M (2017) Understanding and Trading the Term Structure of Volatility. SSRN research paper: http://dx.doi.org/10.2139/ssrn.2871616. |
[19] | Johnson T (2017) Risk Premia and the VIX Term Structure. J Financ Quant Anal, forthcom. |
[20] | Bahaji H, Aberkane S (2016) How rational could VIX investing be? Econ Model 58: 556–568. |
[21] |
Jung Y (2016) A portfolio insurance strategy for volatility index (VIX) futures. Q Rev Econ Financ 60: 189–200. doi: 10.1016/j.qref.2015.09.001
![]() |
[22] |
Alexander C, Korovilas D, Kapraun J (2016) Diversification with volatility products. J Int Money Financ 65: 213–235. doi: 10.1016/j.jimonfin.2016.03.002
![]() |
[23] |
Bordonado C, Molnár P, Samdal S (2017) VIX Exchange Traded Products: Price Discovery, Hedging, and Trading Strategy. J Futures Mark 37:164–183. doi: 10.1002/fut.21786
![]() |
[24] |
Eraker B, Wu Y (2017) Explaining the negative returns to volatility claims: An equilibrium approach. J Financ Econ 125: 72–98. doi: 10.1016/j.jfineco.2017.04.007
![]() |
[25] |
Engle R (2002) Dynamic Conditional Correlation - A Simple Class of Multivariate GARCH. J Bus Econ Stat 20: 339–50. doi: 10.1198/073500102288618487
![]() |
[26] |
Glosten L, Jagannathan R, Runkle D (1993) On the Relation between the Expected Value and the Volatility of the Nominal Excess Return on Stocks. J Financ 48: 1779–1801. doi: 10.1111/j.1540-6261.1993.tb05128.x
![]() |