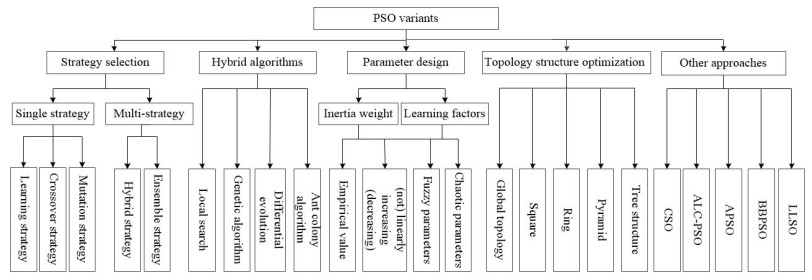
The uses of essential oils in food and traditional medicine have attracted researchers worldwide for applications in the food, agriculture, cosmetics, and health industries. Among the sources of essential oils, the Alpinia genus produces galangal essential oils with great potential for use in many applications. This genus has been used for a long time in traditional medicine in some countries along with for food flavouring and spices. These essential oils from many plant organs of this genus contain several bioactive compounds with the typical phytochemical is 1,8-cineole or eucalyptol. Scientifically, the bioactivity of galangal essential oils has been proven. Some efforts have been conducted efficiently to extract the galangal essential oil, including using novel technologies-This article aims to review the Alpinia species bearing essential oils and their traditional uses, phytochemicals of galangal essential oils and their bioactivity, the methods of extraction, phytochemical profiles, stabilization, uses, and potential applications of these essential oils. This review also compares the bioactivity of galangal essential oils and their traditional uses and potential applications. The results of this review show that Alpinia species has a long history to use as traditional medicines in many countries. There is a closely correlation between traditional uses of galangal with the scientifically proven of health benefits. The studies on galangal essential oil bioactivity have deeply explored the capability as antioxidants, antimicrobial, anti-inflammatory, anticancer, anti-antiparasitic activities and cardiovascular impairment. The uses of galangal essential oils are correlated with their bioactivity. Galangal essential phytochemicals is characterized by the presence of 1,8-cineole. The 1,8-cineole is found in the parts of this plant organ with its concentration varies depending on the galangal species. Some studies indicates that the novel technologies produce higher yield than conventional method for essential oil extraction. The application of galangal essential oil has the constraint of high hydrophobicity, resulting in a poor solubility in the aqueous phase. Various techniques of emulsification are used to improve the its water miscibility. Galangal essential oils are potential to use in many sectors such as agriculture, food, pharmaceutical and personal care industries. The appropriate extraction method is still a challenge mainly to improve the yield along with preserving the bioactive compounds. Another challenge is exploring other Alpinia species, proof their health benefits, and exploring their potential uses in many sectors.
Citation: R. Amilia Destryana, Teti Estiasih, Sukardi, Dodyk Pranowo. The potential uses of Galangal (Alpinia sp.) essential oils as the sources of biologically active compounds[J]. AIMS Agriculture and Food, 2024, 9(4): 1064-1109. doi: 10.3934/agrfood.2024057
[1] | Shangbo Zhou, Yuxiao Han, Long Sha, Shufang Zhu . A multi-sample particle swarm optimization algorithm based on electric field force. Mathematical Biosciences and Engineering, 2021, 18(6): 7464-7489. doi: 10.3934/mbe.2021369 |
[2] | Xin Zhou, Shangbo Zhou, Yuxiao Han, Shufang Zhu . Lévy flight-based inverse adaptive comprehensive learning particle swarm optimization. Mathematical Biosciences and Engineering, 2022, 19(5): 5241-5268. doi: 10.3934/mbe.2022246 |
[3] | Yufeng Wang, BoCheng Wang, Zhuang Li, Chunyu Xu . A novel particle swarm optimization based on hybrid-learning model. Mathematical Biosciences and Engineering, 2023, 20(4): 7056-7087. doi: 10.3934/mbe.2023305 |
[4] | Fei Chen, Yanmin Liu, Jie Yang, Meilan Yang, Qian Zhang, Jun Liu . Multi-objective particle swarm optimization with reverse multi-leaders. Mathematical Biosciences and Engineering, 2023, 20(7): 11732-11762. doi: 10.3934/mbe.2023522 |
[5] | Dongning Chen, Jianchang Liu, Chengyu Yao, Ziwei Zhang, Xinwei Du . Multi-strategy improved salp swarm algorithm and its application in reliability optimization. Mathematical Biosciences and Engineering, 2022, 19(5): 5269-5292. doi: 10.3934/mbe.2022247 |
[6] | Zhiyan Xing, Yanlong Yang, Zuopeng Hu . Nash equilibrium realization of population games based on social learning processes. Mathematical Biosciences and Engineering, 2023, 20(9): 17116-17137. doi: 10.3934/mbe.2023763 |
[7] | Qing Wu, Chunjiang Zhang, Mengya Zhang, Fajun Yang, Liang Gao . A modified comprehensive learning particle swarm optimizer and its application in cylindricity error evaluation problem. Mathematical Biosciences and Engineering, 2019, 16(3): 1190-1209. doi: 10.3934/mbe.2019057 |
[8] | Kefeng Fan, Cun Xu, Xuguang Cao, Kaijie Jiao, Wei Mo . Tri-branch feature pyramid network based on federated particle swarm optimization for polyp segmentation. Mathematical Biosciences and Engineering, 2024, 21(1): 1610-1624. doi: 10.3934/mbe.2024070 |
[9] | Xuepeng Zheng, Bin Nie, Jiandong Chen, Yuwen Du, Yuchao Zhang, Haike Jin . An improved particle swarm optimization combined with double-chaos search. Mathematical Biosciences and Engineering, 2023, 20(9): 15737-15764. doi: 10.3934/mbe.2023701 |
[10] | Ruiping Yuan, Jiangtao Dou, Juntao Li, Wei Wang, Yingfan Jiang . Multi-robot task allocation in e-commerce RMFS based on deep reinforcement learning. Mathematical Biosciences and Engineering, 2023, 20(2): 1903-1918. doi: 10.3934/mbe.2023087 |
The uses of essential oils in food and traditional medicine have attracted researchers worldwide for applications in the food, agriculture, cosmetics, and health industries. Among the sources of essential oils, the Alpinia genus produces galangal essential oils with great potential for use in many applications. This genus has been used for a long time in traditional medicine in some countries along with for food flavouring and spices. These essential oils from many plant organs of this genus contain several bioactive compounds with the typical phytochemical is 1,8-cineole or eucalyptol. Scientifically, the bioactivity of galangal essential oils has been proven. Some efforts have been conducted efficiently to extract the galangal essential oil, including using novel technologies-This article aims to review the Alpinia species bearing essential oils and their traditional uses, phytochemicals of galangal essential oils and their bioactivity, the methods of extraction, phytochemical profiles, stabilization, uses, and potential applications of these essential oils. This review also compares the bioactivity of galangal essential oils and their traditional uses and potential applications. The results of this review show that Alpinia species has a long history to use as traditional medicines in many countries. There is a closely correlation between traditional uses of galangal with the scientifically proven of health benefits. The studies on galangal essential oil bioactivity have deeply explored the capability as antioxidants, antimicrobial, anti-inflammatory, anticancer, anti-antiparasitic activities and cardiovascular impairment. The uses of galangal essential oils are correlated with their bioactivity. Galangal essential phytochemicals is characterized by the presence of 1,8-cineole. The 1,8-cineole is found in the parts of this plant organ with its concentration varies depending on the galangal species. Some studies indicates that the novel technologies produce higher yield than conventional method for essential oil extraction. The application of galangal essential oil has the constraint of high hydrophobicity, resulting in a poor solubility in the aqueous phase. Various techniques of emulsification are used to improve the its water miscibility. Galangal essential oils are potential to use in many sectors such as agriculture, food, pharmaceutical and personal care industries. The appropriate extraction method is still a challenge mainly to improve the yield along with preserving the bioactive compounds. Another challenge is exploring other Alpinia species, proof their health benefits, and exploring their potential uses in many sectors.
With the advancement of computational intelligence technology, many different types of metaheuristic algorithms have emerged. The metaheuristic algorithms can be classified into individual-based metaheuristic algorithms and population-based metaheuristic algorithms. The particle swarm optimization (PSO) algorithm is a representative of population-based intelligence algorithms. PSO is widely used due to its advantages of convergence speed, simplicity and few parameters [1]. The application of PSO appeared in various fields [2,3,4,5]. However, traditional PSO algorithms have difficulty escaping from the local optimum, resulting in low convergence accuracy. Therefore, lots of improved versions of PSO algorithms have blossomed. Specifically, these PSO variants can be roughly divided into five kinds: 1) strategy selection [6,7,8], 2) hybrid algorithms [9,10,11], 3) parameter design [12,13], 4) topology structure optimization [14,15] and 5) other approaches [16,17,18,19,20], as shown in Figure 1. Drawing upon five strands of research into PSO variants, this study attempts to further carry out research on the first aspect, i.e., the influence of multi-strategy selection on the performance of PSO.
As briefly reviewed above, a considerable amount of literature has grown up around the theme of a single strategy, such as the learning strategy, the crossover strategy, the mutation strategy and so on. In terms of learning strategies, Liang et al. [6] proposed a comprehensive learning PSO algorithm (CLPSO), in which the particles could learn from better particles on the same dimension to increase diversity. CLPSO shows good performance for solving multi-modal optimization problems. Similarly, HCLPSO [7] was proposed based on the comprehensive learning (CL) strategy through two-population cooperation, which was integrated learning from personal best particle (pbest) and global best particle (gbest) to balance exploration and exploitation processes. To improve the global search ability and prevent premature convergence, Liang et al. [21] proposed a CL strategy on the basis of crossover. Moreover, Xu et al. [22] proposed a PSO algorithm depending on dimension learning. In respect of crossover strategy, Chen et al. [23] introduced a PSO algorithm relying on two crossover methods (arithmetic crossover and differential evolution crossover) to construct guidance vectors concerning premature convergence of the population. At the later stage of iterations, Zhang et al. [24] developed a crossover operation that is carried out on the current poor pbest in order to enhance the quality of the offspring individuals and promote the convergence speed. From the perspective of mutation strategy, a PSO variant was designed based on the cooperation of large-scale and small-scale mutations, aspiring to balance the global and local search capabilities [25]. Focusing on enhancing the diversity of the population, Li et al. [26] proposed a differential mutation method to increase the global exploration potentiality of the PSO algorithm. Identically, drawing inspiration from the phenomenon of fireworks explosions, Huang et al. [27] designed a mutation operator to enhance the exploration capability of multi-objective optimization algorithms.
Up to now, a proliferation of studies has also grown up around the issue of the multi-strategy PSO algorithm. Wang et al. [28] combined Cauchy mutation and opposition learning to prevent premature convergence. In order to accelerate the convergence speed of the population, Ouyang et al. [29] used the strategies of stochastic learning and opposition learning. Zhang et al. [30] proposed the strategy of learning from excellent individuals and dynamic differential evolution. Wang et al. [31] utilized the CL strategy and non-uniform mutation operator to promote the exploration capability of the algorithm. The method of Gaussian mutation was also used to increase the ability to break away from the local optimum. Likewise, the CL strategy with an archive set and the elite learning strategy were employed to enhance the global search and local exploitation abilities of the algorithm, respectively [32]. In another PSO variant, a multi-strategy learning PSO algorithm was proposed and applied to different stages of evolution for large-scale optimization problems [33]. In addition, the self-adaptive method, which has been extensively studied in relation to the ensemble PSO algorithm, is particularly applicable to multi-strategy selection for PSO variants. For instance, an ensemble learning PSO algorithm (EPSO) was proposed to select the best strategy for generating fine offspring by tracking the success rate of strategy improvement over a given time period [34]. Based on game theory, another multi-strategy selection mechanism, SDPSO [8], was designed. Strategies with high payoffs were selected to generate offspring with a higher probability. Moreover, Li et al. [35] designed different particle velocity formulas from exploitation, the local optimum, exploration and convergence, thus forming a hybrid learning strategy method.
Furthermore, reinforcement learning (RL) has penetrated deeply into evolutionary algorithms (EAs), embracing new areas for RL-guided EAs and forming new hybrid methods [36]. RL, as a sub-field of machine learning, is inspired by behaviorism in psychology. RL is the way that agents learn by "trial and error, " and what they learn is a mapping from environmental states to actions, with the goal of making agents get the maximum reward [37]. RL has been widely adopted in intelligent approach. Liu et al. [38] used the RL technique to guide the parameter optimization of the PSO algorithm. Wang et al. [39] embedded RL into the hierarchical learning PSO algorithm for selecting the number of layers. For the optimization of functions, Li et al. [40] resorted to the RL technique for multi-modal multi-objective optimization problems. Hu et al. [41] employed a similar method to select differential evolution mutation strategies and then solve constrained optimization problems. The RL technique was also adopted to solve dynamic multi-objective optimization problems [42]. Moreover, deep RL was embedded into a multi-objective evolutionary optimization algorithm based on decomposition for the selection of adaptive operators [43]. RL-guided EAs were also given to solve application problems in diverse domains [44,45,46,47,48].
Based on the above description, it can be seen that these studies provide important insights into the relationship between RL techniques and EAs. Although some PSO variants employ the idea of Q-learning, the purpose is only to select appropriate parameters [38] or level numbers [39]. To the best of our knowledge, however, the method of self-adaptive multi-strategy selection combined with the RL technique has not been provided in existing multi-swarm cooperation PSO variants, and the effect of the multi-swarm PSO algorithm using RL as an auxiliary tool is still unknown. It follows that some effective schemes, such as Q-learning, mixed techniques, etc., are still underutilized to improve the performance of EAs. Therefore, under the guidance of the RL technique, a new multi-strategy selection mechanism is designed. In the evolutionary process, the same particle can be assigned different strategies while different particles can be given the same strategy. Each strategy can be evaluated by the Q-table and refreshed automatically during iterations. Hence, a more flexible structure for multi-strategy self-learning is reshaped. The main contributions of this paper are highlighted as follows:
∙ Q-learning, as the classical RL technique, is thoroughly investigated in the multi-strategy self-learning PSO algorithm. A multi-strategy self-learning method is constructed to select appropriate strategies for producing high-quality offspring.
∙ The traditional state division in Q-learning is the uniform division method [38], [40,41]. In the proposed algorithm, a non-uniform division approach is proposed which is more suitable for optimization problems. Meanwhile, experiment results also show that this partition is related to the dimension of the particles and is more favorable for solving high-dimensional problems.
∙ A novel multi-strategy self-learning particle swarm optimizer (MPSORL) is designed to obtain a better balance between exploration and exploitation. MPSORL has faster convergence speed and better global optimization ability than compared approaches in most cases.
∙ Systematic experiments on two popular test suites and a real-world case have demonstrated that the multi-strategy self-learning mechanism is effective, and the proposed MPSORL outperforms other state-of-the-art algorithms.
The remainder of this paper is arranged as follows. The related theory is introduced in Section 2. The MPSORL algorithm in detail is designed in Section 3. Section 4 is the simulation experiment and analysis. Further discussion is presented in Section 5. Section 6 concludes this paper.
PSO simulates the foraging behavior of birds, and a particle is often compared to a bird [49]. PSO focuses on two properties of particles: position and velocity. Each particle searches the space independently, remembers its own past optimal solution and also knows the current optimal solution found by the whole swarm of particles. Generally, Equations (2.1) and (2.2) are used to describe the particles' velocity and position, respectively [50].
vk+1ij=w∗vkij+c1∗r1∗(pbestkij−xkij)+c2∗r2∗(gbestkj−xkij) | (2.1) |
xk+1ij=xkij+vk+1ij | (2.2) |
where w denotes the inertia weight, and c1, c2 denote the learning factors. r1 and r2 are uniformly distributed random numbers in the range of [0, 1]. Let i=1,2,⋯,N, where N is the number of particles. Likewise, let j=1,2,⋯,D, where D is the dimension of a particle. k denotes the number of the iteration. xkij and vkij are the position and velocity components of the ith particle at the kth iteration. For the minimization problem, the optimal position pbestki found by the particle can be expressed as follows:
pbestki={xki,iff(xki)<f(pbestk−1i)pbestk−1i,iff(xki)≥f(pbestk−1i) | (2.3) |
where f(⋅) denotes the fitness value. Furthermore, Equation (2.4) describes the current optimal position for the entire particle space.
gbestk=pbestkg | (2.4) |
where g=argmin1≤i≤N[f(pbestki)].
In RL, there are five essential elements: agent, environment, states, actions and reward. The Q-learning algorithm is a method that uses temporal difference to solve RL control problems [51]. Concretely, at a certain time t, the agent decides the action at; and the environment offers a reward rt+1, generates the next state st+1 according to the agent's action at and then gives feedback to the agent. Here, the state set is denoted by S={s1,s2,⋯,sp}, and p denotes the number of states. The action set is denoted by A={a1,a2,⋯,aq}, and q denotes the number of actions. The characteristic of the Q-learning algorithm is to construct a two-dimensional table, named the Q-table. According to the potential states and actions, the agent looks up the table to obtain the Q-value and then give the optimal action. The details are shown in Figure 2.
In the Q-table, the Q-value is computed as follows:
Q(st,at)=Q(st,at)+α[rt+1+γmaxa∈AQ(st+1,a)−Q(st,at)]. | (2.5) |
Notably, Equation (2.5) has the following attributes:
1) The right-hand side of the formula consists of three parts: the old value Q(st,at), the immediate reward rt+1, and the estimate of the optimal future value Q(st+1,a).
2) The parameter α represents the learning rate, which denotes a trade-off between the previous learning and the current learning.
3) The parameter γ refers to the factor that considers the impact of future rewards on the present, and it is a number in the range [0, 1]. If γ→1, the future state has a greater impact on Q-value. On the contrary, if γ→0, Q-value is more affected by the current state.
The pseudocode for Q-learning is expressed in Algorithm 1.
Algorithm 1 Q-learning algorithm |
1: Initialize Q(s, a), α, γ, r, ε 2: Repeat : 3: Choose the best action at for the current state st using policy (e.g., ε-greedy strategy); 4: Execute action at; 5: Get the immediate reward rt+1; 6: Get the maximum Q-value for the next state st+1; 7: Update the Q-table by Eq (2.5); 8: Update the current state st←st+1; 9: Until the condition is terminal |
When solving global optimization problems with EAs, an important issue is how to balance exploration and exploitation. However, it is hard to maintain such a balance owing to the characteristics of the problems. In order to better match the properties of different functions, it is desirable to find a method to select the appropriate strategy adaptively. In the multi-strategy PSO algorithm, different strategies can greatly affect the performance of the algorithm. In other words, the selection of strategy plays a vital role in improving the performance of the algorithm. In fact, as one of the most well-known RL algorithms, Q-learning can directly learn the optimal strategy [51]. New states and rewards are generated by executing the action, and then the Q-table is updated. The excellence or worseness of a strategy depends on the cumulative reward after playing the strategy for a long time. Based on this idea, a multi-strategy self-learning method based on the RL technique is proposed. Therefore, a new EA based on the framework of PSO, named MPSORL, is designed. The specific steps are described in the next section. Table 1 shows a comparison of terms between PSO and Q-learning.
Q-learning | PSO |
Agents | Particles |
Environment | Optimization problems |
State | Fitness value |
Action | Strategy |
This section will describe the detailed design of the Q-learning algorithm, the related theory of which has been presented briefly in Section 2.2. The purpose is to embed the newly designed Q-learning elements into the multi-strategy PSO algorithm and then form the multi-strategy self-learning method.
For any subject, the distribution of student scores can always be divided into five levels: excellent, good, medium, pass and fail. In most cases, the number of individuals located at each level may not necessarily be equal. Referring to the student scores distribution analogy, it is reasonable that the state adopts a non-uniform division according to the fitness value. As a result, the fitness value is divided into five grades from the smallest to the largest, namely, s1 to s5, as shown in Figure 3. It is not difficult to find from Figure 3 that the number of particles may not be equally distributed. Thus, the number of particles within each level is not the same. To the best of our knowledge, the traditional state division in Q-learning has only focused on the uniform division method [38,40,41]. The two methods are compared, and the advantages of the non-uniform state division are illustrated by the experiment in Section 5.1.
If the opportunity to try is distributed equally among actions, the average reward of each action is eventually taken as an approximation of the expected reward. If the opportunity to try is always given to the action with the highest current average reward, it may not be easy to find the optimal action. It can be seen that these two methods are contradictory. So, a compromise needs to be made between exploration and exploitation. Specifically, the ε-greedy strategy is adopted to execute a compromise between exploration and exploitation based on probability, that is, at each trial, it explores with probability ε and selects an action at random with uniform probability. On the contrary, it exploits with probability 1−ε and chooses the currently best action. Here, ε is a smaller number within [0, 1], and the value is analyzed experimentally in Section 4.2. The ε-greedy strategy is displayed in Eq (3.1).
a={argmaxa∈AQ(s,a),withprobability1−εrandom,otherwise. | (3.1) |
For a sequence (s,a,s′,r), the payoff cannot be obtained until the transition arises from state s to state s′ by executing action a. Each particle has an associated reward r. If the objective function value decreases, the reward is set to 1. If the objective function value cannot be reduced to an acceptable level of state, the value of the reward is set to 0. The rule is presented by Eq (3.2) as follows:
r={1,ifsi−1←si,i=2,⋯,p0,otherwise. | (3.2) |
As described in Section 3.2, under the framework of SDPSO [8], this paper uses a cooperation framework of small (pop1) and large (pop2) subpopulations [8,34]. Unlike the previous work, a new multi-strategy self-learning method is developed in the large population, i.e, the RL technique is adopted to train the behavior of particles. The Q-table is shown in Table 2. Among them, actions refer to the strategies that particles use to generate offspring individuals. Multi-strategy and self-adaptive selection techniques can provide complementary advantages. Here, LIPS [52], UPSO [53], LDWPSO [50] and CLPSO [6] are denoted as actions a1, a2, a3 and a4, respectively. Concretely, LIPS can work well in searching for multiple local optima in multimodal optimization problems, UPSO shows the advantages of dealing with unimodal problems, the convergence speed of LDWPSO is faster than other compared approaches, and CLPSO can preserve the population diversity throughout the iteration process. As a result, a multi-strategy self-adaptive selection scheme is developed by adopting these strategies. According to the fitness value of the individuals, the state of the population is first divided inhomogeneously. Then, the ε-greedy strategy is used to select the action for each particle. Next, the offspring individuals are generated to update pbest and gbest. Thus, the fitness of offspring individuals acts as an input to the environment, and the next state is calculated for each particle. The process of state transition produces the corresponding reward value. Then, the Q-table is updated. This process is repeated until the termination condition is met. From the above statement, the framework of multi-strategy self-learning is displayed in Figure 4.
State | Action | |||
a1 | a2 | a3 | a4 | |
s1 | Q(s1, a1) | Q(s1, a2) | Q(s1, a3) | Q(s1, a4) |
s2 | Q(s2, a1) | Q(s2, a2) | Q(s2, a3) | Q(s2, a4) |
s3 | Q(s3, a1) | Q(s3, a2) | Q(s3, a3) | Q(s3, a4) |
s4 | Q(s4, a1) | Q(s4, a2) | Q(s4, a3) | Q(s4, a4) |
s5 | Q(s5, a1) | Q(s5, a2) | Q(s5, a3) | Q(s5, a4) |
Based on the above description, the pseudocode of MPSORL is described in Algorithm 2. First, some input parameters are defined. Then, when the program enters the loop, pop1 is updated by CLPSO in lines 4–8. For pop2, lines 10–33 are to assign the optimal strategy for each particle adaptively. Specifically, lines 10–11 are to divide state and execute selection action based on the Q-table. Lines 12–17 are to generate offspring individuals by means of various strategies. For every learning period (LP) iteration, the Q-table is updated in lines 19–33. The action is reselected in line 22, which is to enhance exploration abilities. It should be noted that no additional parameters are introduced. The procedures in lines 1–34 are executed iteratively until the termination condition is met.
Algorithm 2 The proposed algorithm |
Input:
Initialize N, D, the maximum number of fitness evaluations (Max_Fes); Initialize the maximum number of iterations (Max_Iter), k=0, kk=0, fit=0; Initialize the inertia weight w, learning factors c1, c2, position, velocity; Evaluate the fitness value, fit=fit+N, record pbest and gbest; Initialize Q-table, state, reward, learning period LP, ε, α, γ. Output: Best solution. 1: while termination condition is not met do 2: k=k+1; 3: /* pop1 */ 4: for i=1:length(pop1) do 5: Update the particle's velocity and position by CLPSO; 6: Evaluate the fitness of the particle, fit=fit+1; 7: Update pbesti and gbest; 8: end for 9: /* pop2 */ 10: Divide state for pop2 according to Section 3.2.1; 11: Select action based on Q-table using ε-greedy strategy according to Eq (3.1); 12: for i=length(pop1)+1:N do 13: Update the particle's velocity by action ai; 14: Update the particle's position; 15: Evaluate the fitness of the particle, fit=fit+1; 16: Update pbesti and gbest; 17: end for 18: /* update Q-table */ 19: if k<Max_Iter then 20: if mod(k, LP) = 0 then 21: Determine next state; 22: Select action using ε-greedy strategy; 23: Calculate reward according to Eq (3.2); 24: Update Q-table using Eq (2.5); 25: end if 26: else 27: if mod(kk, LP) = 0 then 28: Determine next state; 29: Calculate reward according to Eq (3.2); 30: Update Q-table using Eq (2.5); 31: end if 32: kk=kk+1; 33: end if 34: end while |
In MPSORL, the worst-case time complexity of pop1 is O (N1D), where N1 is the population size of pop1. The worst-case time complexity of pop2 is O (N2D), where N2 is the population size of pop2. Compared with the standard PSO, MPSORL requires sorting the particles in the pop2 and then assigning each particle to a specific state. The mean time complexities of the two procedures can be denoted by (N2log(N2)) and O (N2D), respectively. In addition, MPSORL needs to update the Q-table through Q-learning. The worst-case time complexity is O (N2D). According to the above component complexity analysis, MPSORL has the overall computational time complexity O (N1D+3N2D+N2log(N2)).
This paper introduces the RL technique to classify particle states and guide particle movements, and a multi-strategy self-learning mechanism based on the RL technique is proposed to select the optimal strategy to update particles' information. In the same state, particles may adopt different strategies, and particles in different states may also adopt the same strategy. The details are analyzed below:
1) Self-learning method based on the RL technique. The action set is constructed by the strategy pool. The whole process is controlled by the Q-table, which facilitates independent learning and completes the strategy selection. This makes the proposed algorithm significantly different from other multi-strategy selection PSO algorithms. For instance, the limitation of EPSO [34] lies in the fact that the information about fitness improvement is not fully utilized to update the adoption probability of the strategies. In addition, in SDPSO [8], a restart operator might reduce the convergence speed.
2) State divisions of particles unequal in the population. Particles are divided into five grades according to fitness: excellent, good, medium, pass and fail. The better the particle's fitness value is, the higher its state level. The divisions distinguish the MPSORL from the RL-guided PSO variants [38,40,41] which have only focused on the uniform division.
3) Feedback mechanism. Strategy selection in existing multi-strategy PSO variants does not provide feedback information, relying on their performance evaluations [8,34,35]. In Eq (2.5), the Q-value is evaluated by the immediate and future rewards. The future reward has varying degrees of influence on the Q-value. According to the theory of Q-learning and the individual's multi-step evolutionary performance, if one regulates future payoffs and then feeds them back to the agent, the offspring may also be influenced.
In this section, orthogonal experiments are first adopted to determine five vital parameters. Then, the superiority of the multi-strategy PSO algorithm is verified on 30-dimensional problems of the CEC2017 test suite [54]. Next, the performance of the algorithm is further verified on the 50- and 100-dimensional problems of CEC2017, respectively. Subsequently, MPSORL is tested on the latest suite, CEC2019 [55]. The detailed information for CEC2017 and CEC2019 is outlined in Tables 3 and 4. Finally, the algorithm is also applied to a real-world problem.
Function type | No. | Test Functions | F(x∗) | D |
Unimodal Functions | F1 | Shifted and Rotated Bent Cigar Function | 100 | 30/50/100 |
F2 | Shifted and Rotated Sum of Different Power Function* | 200 | 30/50/100 | |
Simple Multimodal Functions | F3 | Shifted and Rotated Zakharov Function | 300 | 30/50/100 |
F4 | Shifted and Rotated Rosenbrock Function | 400 | 30/50/100 | |
F5 | Shifted and Rotated Rastrigin Function | 500 | 30/50/100 | |
F6 | Shifted and Rotated Expanded Schaffer's F6 Function | 600 | 30/50/100 | |
F7 | Shifted and Rotated Lunacek Bi-Rastrigin Function | 700 | 30/50/100 | |
F8 | Shifted and Rotated Non-Continuous Rastrigin Function | 800 | 30/50/100 | |
F9 | Shifted and Rotated Levy Function | 900 | 30/50/100 | |
Hybrid Functions | F10 | Shifted and Rotated Schwefel Function | 1000 | 30/50/100 |
F11 | Hybrid Function 1 (N = 3) | 1100 | 30/50/100 | |
F12 | Hybrid Function 2 (N = 3) | 1200 | 30/50/100 | |
F13 | Hybrid Function 3 (N = 3) | 1300 | 30/50/100 | |
F14 | Hybrid Function 4 (N = 4) | 1400 | 30/50/100 | |
F15 | Hybrid Function 5 (N = 4) | 1500 | 30/50/100 | |
F16 | Hybrid Function 6 (N = 4) | 1600 | 30/50/100 | |
F17 | Hybrid Function 6 (N = 5) | 1700 | 30/50/100 | |
F18 | Hybrid Function 6 (N = 5) | 1800 | 30/50/100 | |
F19 | Hybrid Function 6 (N = 5) | 1900 | 30/50/100 | |
Composition Functions | F20 | Hybrid Function 6 (N = 6) | 2000 | 30/50/100 |
F21 | Composition Function 1 (N = 3) | 2100 | 30/50/100 | |
F22 | Composition Function 2 (N=3) | 2200 | 30/50/100 | |
F23 | Composition Function 3 (N = 4) | 2300 | 30/50/100 | |
F24 | Composition Function 4 (N = 4) | 2400 | 30/50/100 | |
F25 | Composition Function 5 (N = 5) | 2500 | 30/50/100 | |
F26 | Composition Function 6 (N = 5) | 2600 | 30/50/100 | |
F27 | Composition Function 7 (N = 6) | 2700 | 30/50/100 | |
F28 | Composition Function 8 (N = 6) | 2800 | 30/50/100 | |
F29 | Composition Function 9 (N = 3) | 2900 | 30/50/100 | |
F30 | Composition Function 10 (N = 3) | 3000 | 30/50/100 | |
Note: *F2 has been excluded because it shows unstable behavior especially for higher dimensions. |
No. | Functions | F(x∗) | D | Search range |
F31 | Storn's Chebyshev Polynomial Fitting Problem | 1 | 9 | [-8192, 8192] |
F32 | Inverse Hilbert Matrix Problem | 1 | 16 | [-16384, 16384] |
F33 | Lennard-Jones Minimum Energy Cluster | 1 | 18 | [-4, 4] |
F34 | Rastrigin Function | 1 | 10 | [-100,100] |
F35 | Griewank's Function | 1 | 10 | [-100,100] |
F36 | Weierstrass Function | 1 | 10 | [-100,100] |
F37 | Modified Schwefel Function | 1 | 10 | [-100,100] |
F38 | Expanded Schaffer's F6 Function | 1 | 10 | [-100,100] |
F39 | Happy Cat Function | 1 | 10 | [-100,100] |
F40 | Ackley Function | 1 | 10 | [-100,100] |
Experimental environment is Windows 7, Intel(R) Xeon(R) CPU E5-2667 v4@3.30 GHz, and the memory is 16 GB. All the code was tested on the Matlab R2020b platform.
The population size is set to 40, 40 and 80 in 30, 50 and 100 dimensions, respectively. Max_Fes is set to 10,000 × dimension. The results are based on the errors of the optimized values minus the standard optimal value. Each algorithm is evaluated on each test function for 30 independent runs. Moreover, for comparative fairness, the same number of evaluations is used. All test functions are minimization problems.
The performance of the algorithm is measured by two non-parametric tests, namely, the Wilcoxon rank sum test with a significance level 0.05 and the Friedman test [56,57]. The symbols "+/≈/−" mean that the proposed algorithm is better, has no significant difference or is relatively worse than the comparison algorithm, respectively. The parameter settings are presented in Table 5.
Algorithm | Key parameters | Year | Ref. |
LDWPSO | w:0.9−0.2, c1:2.5−0.5, c2:0.5−2.5, Vmax = 0.5*range | 1998 | [50] |
CLPSO | w:0.9−0.2, c:3.0−1.5, Vmax = 0.5*range | 2006 | [6] |
UPSO | w:0.9−0.2, c1:2.5−0.5, c2:0.5−2.5 | 2004 | [53] |
LIPS | χ:0.7298, Vmax = 0.5*range, nsize = 3 | 2013 | [52] |
HCLPSO | w:0.99−0.2, c:3.0−1.5, c1:2.5−0.5, c2:0.5−2.5, Vmax = 0.2*range | 2015 | [7] |
EPSO | w:0.9−0.4, c:3.0−1.5, w1:0.9−0.2, c1:2.5−0.5, c2:0.5−2.5, Vmax = 0.5*range | 2017 | [34] |
SDPSO | w:0.9−0.2, c:3.0−1.5, c1:2.5−0.5, c2:0.5−2.5, Vmax = 0.5*range | 2022 | [8] |
MRFO | S = 2 | 2020 | [58] |
I-GWO | a = 2−0 | 2021 | [59] |
BeSD | K = 5 | 2021 | [60] |
MPSORL | w:0.9−0.2, c:3.0−1.5, c1:2.5−0.5, c2:0.5−2.5, Vmax = 0.5*range | − | − |
In MPSORL, there are five important parameters: the size of pop1 (N1), the learning period (LP), the exploration rate (ε), the learning factor (α), the decay rate (γ). The parameter levels of MPSORL are shown in Table 6. The orthogonal table of L16(45) is shown in Table 7. The algorithm corresponding to each combination of parameters is tested on 30-dimensional problems from CEC2017. From Figure 5 below, it is not difficult to find that the optimal parameters combination is: 0.4, 50, 0.8, 0.6 and 0.8.
Level | Factors | ||||
pop1 (N1) | LP | epsilon (ε) | alpha (α) | gamma (γ) | |
1 | 0.1 | 20 | 0.80 | 0.3 | 0.6 |
2 | 0.2 | 50 | 0.85 | 0.4 | 0.7 |
3 | 0.3 | 100 | 0.90 | 0.5 | 0.8 |
4 | 0.4 | 200 | 0.95 | 0.6 | 0.9 |
pop1 (N1) | LP | epsilon (ε) | alpha (α) | gamma (γ) | average rank | |
1 | 0.1 | 20 | 0.8 | 0.3 | 0.6 | 8.5 |
2 | 0.1 | 50 | 0.85 | 0.4 | 0.7 | 9.345 |
3 | 0.1 | 100 | 0.9 | 0.5 | 0.8 | 10.672 |
4 | 0.1 | 200 | 0.95 | 0.6 | 0.9 | 11.483 |
5 | 0.2 | 20 | 0.85 | 0.5 | 0.9 | 7.81 |
6 | 0.2 | 50 | 0.8 | 0.6 | 0.8 | 6.207 |
7 | 0.2 | 100 | 0.95 | 0.3 | 0.7 | 10.293 |
8 | 0.2 | 200 | 0.9 | 0.4 | 0.6 | 9.207 |
9 | 0.3 | 20 | 0.9 | 0.6 | 0.7 | 7.879 |
10 | 0.3 | 50 | 0.95 | 0.5 | 0.6 | 9.828 |
11 | 0.3 | 100 | 0.8 | 0.4 | 0.9 | 6.379 |
12 | 0.3 | 200 | 0.85 | 0.3 | 0.8 | 7.293 |
13 | 0.4 | 20 | 0.95 | 0.4 | 0.8 | 9.362 |
14 | 0.4 | 50 | 0.9 | 0.3 | 0.9 | 7.931 |
15 | 0.4 | 100 | 0.85 | 0.6 | 0.6 | 6.793 |
16 | 0.4 | 200 | 0.8 | 0.5 | 0.7 | 7.017 |
In this section, in order to verify the superiority of the multi-strategy PSO algorithm, several single-strategy PSO algorithms, namely, LDWPSO, UPSO, CLPSO and LIPS, are selected to be compared with the multi-strategy MPSORL algorithm.
Table 8 summarizes the statistical results by comparing these four single-strategy PSO algorithms with MPSORL via Wilcoxon rank sum test. As shown in Table 8, for unimodal functions (F1, F3), it can be found that the MPSORL algorithm performs best on F3. Except for F4, MPSORL ranks first for all simple multimodal functions (F4−F10). For the hybrid functions (F11−F20), the MPSORL algorithm obtains excellent results on F11, F12, F16−F18 and F20, and there is no significant difference with the first-ranked algorithms on F13 and F14 functions. For the composition functions (F21−F30), the computational results on F21−F24, F26, F29, and F30 are significantly better than those of the other algorithms, and there is no significant difference on the F25 and F28 functions compared with the comparison algorithms that achieved the best results.
IEEE CEC2017 with 30D | LDWPSO | UPSO | CLPSO | LIPS | MPSORL |
mean±std | mean±std | mean±std | mean±std | mean±std | |
F1 | 4.64E+03±5.02E+03+ | 2.03E+03±2.03E+03+ | 8.17E+00±1.07E+01− | 9.24E+02±1.60E+03+ | 1.05E+02±2.10E+02 |
F3 | 3.07E+02±4.39E+02+ | 1.46E+02±2.08E+02+ | 2.84E+04±6.40E+03+ | 2.49E+03±1.59E+03+ | 2.65E−02±7.68E−02 |
F4 | 9.26E+01±2.63E+01+ | 3.58E+01±3.85E+01≈ | 5.90E+01±2.66E+01+ | 3.59E+01±2.95E+01≈ | 4.13E+01±2.72E+01 |
F5 | 6.95E+01±1.83E+01+ | 6.99E+01±1.13E+01+ | 4.76E+01±8.81E+00+ | 1.05E+02±2.29E+01+ | 3.25E+01±1.00E+01 |
F6 | 4.27E−01±5.54E−01+ | 1.20E+00±9.13E−01+ | 4.92E−07±2.49E−07+ | 2.29E+01±8.13E+00+ | 2.35E−13±6.63E−14 |
F7 | 1.18E+02±2.17E+01+ | 9.67E+01±1.34E+01+ | 9.22E+01±1.01E+01+ | 1.13E+02±1.93E+01+ | 8.33E+01±1.15E+01 |
F8 | 6.93E+01±1.74E+01+ | 6.44E+01±1.13E+01+ | 5.33E+01±7.36E+00+ | 9.36E+01±2.08E+01+ | 3.67E+01±1.33E+01 |
F9 | 1.40E+02±1.22E+02+ | 9.18E+01±6.82E+01+ | 1.18E+02±5.19E+01+ | 1.52E+03±9.83E+02+ | 1.27E+00±1.05E+00 |
F10 | 3.21E+03±6.26E+02+ | 2.90E+03±4.36E+02+ | 2.18E+03±2.71E+02+ | 3.11E+03±4.73E+02+ | 1.86E+03±3.64E+02 |
F11 | 8.61E+01±4.17E+01+ | 5.86E+01±2.68E+01+ | 6.69E+01±2.04E+01+ | 1.04E+02±3.48E+01+ | 4.65E+01±3.30E+01 |
F12 | 3.79E+04±1.95E+04+ | 8.47E+04±8.91E+04+ | 4.02E+05±2.22E+05+ | 1.30E+05±1.33E+05+ | 2.34E+04±1.03E+04 |
F13 | 9.64E+03±1.20E+04+ | 7.86E+03±6.06E+03+ | 3.41E+02±1.26E+02≈ | 4.42E+03±5.13E+03+ | 4.90E+02±5.07E+02 |
F14 | 6.14E+03±4.66E+03+ | 3.66E+03±3.79E+03≈ | 2.89E+04±2.86E+04+ | 8.02E+03±5.99E+03+ | 3.94E+03±5.06E+03 |
F15 | 5.33E+03±5.78E+03+ | 2.47E+03±2.83E+03+ | 1.44E+02±1.03E+02− | 1.97E+03±3.02E+03+ | 2.88E+02±3.70E+02 |
F16 | 7.60E+02±2.01E+02+ | 6.57E+02±1.25E+02+ | 5.16E+02±1.21E+02+ | 7.24E+02±2.56E+02+ | 3.97E+02±1.79E+02 |
F17 | 2.26E+02±1.59E+02+ | 1.69E+02±7.73E+01+ | 1.22E+02±5.72E+01+ | 2.88E+02±1.55E+02+ | 9.27E+01±4.66E+01 |
F18 | 9.36E+04±5.84E+04≈ | 1.04E+05±4.07E+04≈ | 1.37E+05±6.93E+04+ | 8.81E+04±7.43E+04≈ | 8.72E+04±5.27E+04 |
F19 | 7.41E+03±1.02E+04+ | 2.04E+03±2.27E+03+ | 5.83E+01±4.54E+01− | 2.50E+03±3.42E+03+ | 1.55E+02±1.42E+02 |
F20 | 2.78E+02±1.26E+02+ | 2.77E+02±7.74E+01+ | 1.56E+02±7.37E+01≈ | 4.76E+02±1.29E+02+ | 1.34E+02±6.92E+01 |
F21 | 2.70E+02±1.43E+01+ | 2.62E+02±3.02E+01+ | 2.40E+02±4.08E+01+ | 2.95E+02±2.04E+01+ | 2.33E+02±9.38E+00 |
F22 | 3.90E+02±1.10E+03+ | 1.01E+02±1.73E+00+ | 1.13E+02±6.95E+00+ | 3.19E+02±8.32E+02≈ | 1.00E+02±1.08E+00 |
F23 | 4.29E+02±2.15E+01+ | 4.32E+02±2.02E+01+ | 4.00E+02±1.20E+01+ | 5.35E+02±3.83E+01+ | 3.86E+02±1.07E+01 |
F24 | 5.15E+02±3.12E+01+ | 4.87E+02±1.20E+01+ | 4.76E+02±9.15E+01+ | 5.70E+02±4.66E+01+ | 4.53E+02±7.86E+00 |
F25 | 3.96E+02±1.37E+01+ | 3.89E+02±5.82E+00+ | 3.87E+02±1.13E+00≈ | 3.87E+02±4.05E+00≈ | 3.87E+02±1.05E+00 |
F26 | 1.43E+03±7.28E+02+ | 6.40E+02±7.57E+02≈ | 4.02E+02±1.94E+02+ | 9.96E+02±1.18E+03≈ | 3.75E+02±3.19E+02 |
F27 | 5.37E+02±1.22E+01+ | 5.48E+02±1.57E+01+ | 5.10E+02±5.27E+00− | 5.84E+02±2.16E+01+ | 5.15E+02±6.39E+00 |
F28 | 4.13E+02±2.81E+01+ | 3.15E+02±3.50E+01≈ | 4.20E+02±6.12E+00+ | 3.10E+02±2.98E+01≈ | 3.36E+02±5.26E+01 |
F29 | 6.76E+02±1.76E+02+ | 7.95E+02±1.28E+02+ | 5.44E+02±6.75E+01+ | 1.03E+03±1.70E+02+ | 4.94E+02±4.09E+01 |
F30 | 5.83E+03±3.07E+03+ | 8.18E+03±6.88E+03+ | 4.82E+03±8.11E+02+ | 6.87E+03±2.12E+03+ | 4.06E+03±1.14E+03 |
+/≈/− | 28/1/0 | 24/5/0 | 22/3/4 | 23/6/0 | / |
Overall, better or comparable results are achieved on 25 of 29 functions, accounting for 86.2%. On F1, F15, F19 and F27, MPSORL is a little worse than CLPSO. Finally, in the last row of Table 8, the statistical results show that the MPSORL algorithm performs appreciably better than the other four comparison algorithms. In addition, Figure 6 illustrates the Friedman test results. The average rank of MPSORL is 1.38, ranking first, which is better than other algorithms. It implies that the proposed multiple-strategy MPSORL algorithm has remarkable advantages compared with the single-strategy PSO algorithms.
For some functions, which are randomly chosen, the convergence process of the algorithm is plotted. From Figure 7, it can be seen that MPSORL has the highest convergence accuracy among all PSO variants on unimodal functions F3 and simple multimodal functions F5, F8–F10. Also, there is a clear rate of convergence on F9. Among compared algorithms, LDWPSO shows a faster convergence speed but easily fails into local optima. On hybrid functions F12, F16 and F20, the convergence accuracy and rate of CLPSO are significantly worse than those of MPSORL on F12; but on the other problems, the convergence trend is similar, while the accuracy is not as good as MPSORL. On composition functions F21–F24 and F26, MPSORL is significantly better than CLPSO in terms of higher convergence precision and faster convergence speed. Furthermore, other PSO variants converge much faster but perform worse in fine tuning solutions. Therefore, the obvious advantage of the multi-strategy algorithm over the single-strategy PSO algorithm is also verified in terms of convergence.
In this section, three PSO variants and three other metaheuristic algorithms are selected, namely, HCLPSO, EPSO, SDPSO, MRFO, I-GWO and BeSD. Concretely, HCLPSO, EPSO and SDPSO are multi-population cooperative algorithms, among which adaptive multi-strategy selection methods are adopted by EPSO and SDPSO. Next, we compare the performances of these algorithms and MPSORL on 50D and 100D, respectively.
Table 9 presents the statistical results of MPSORL and the other six comparison algorithms on 29 50D CEC2017 test functions. Among them, the MPSORL algorithm performs best on F1 for unimodal functions. For simple multimodal functions (F4−F10), MPSORL performs remarkably on F5, F6, F9 and F10. Also, there is no significant difference between MPSORL and the top-ranked algorithms on F7 and F8. In addition, for the hybrid functions (F11−F20), MPSORL performs significantly better than the other six algorithms on F13, F15, F17, and F20. On F11 and F19, there is not much difference between MPSORL and the algorithms that achieved the best results. For the composition functions (F21−F30), MPSORL obtains the top performance on F21, and there is no remarkable difference with the algorithms having the best results on F22, F23, F25, F26 and F28−F30.
IEEE CEC2017 with 50D | HCLPSO | EPSO | SDPSO | MRFO | I-GWO | BeSD | MPSORL |
mean±std | mean±std | mean±std | mean±std | mean±std | mean±std | mean±std | |
F1 | 1.65E+02±2.03E+02≈ | 2.15E+03±2.12E+03+ | 3.46E+02±3.11E+02+ | 4.94E+03±5.51E+03+ | 5.00E+03±6.84E+03+ | 8.66E+02±1.13E+03+ | 1.35E+02±1.34E+02 |
F3 | 1.55E+01±1.61E+01− | 6.38E−04±1.14E−03− | 5.11E+00±2.31E+01− | 6.98E+03±2.44E+03+ | 1.91E+04±4.05E+03+ | 1.22E+03±7.08E+02+ | 5.22E+02±5.03E+02 |
F4 | 7.85E+01±4.42E+01≈ | 4.05E+01±3.72E+01− | 5.97E+01±4.46E+01≈ | 8.48E+01±5.02E+01≈ | 1.01E+02±6.05E+01+ | 9.56E+01±4.32E+01+ | 7.11E+01±3.82E+01 |
F5 | 1.05E+02±1.88E+01+ | 1.12E+02±1.81E+01+ | 8.12E+01±2.15E+01≈ | 3.27E+02±4.73E+01+ | 3.53E+02±2.02E+01+ | 1.78E+02±1.27E+01+ | 7.83E+01±1.77E+01 |
F6 | 6.63E−13±1.43E−13+ | 2.90E−08±9.81E−08+ | 1.15E−05±1.96E−05≈ | 4.38E+01±1.12E+01+ | 3.60E−02±1.10E−01+ | 8.17E−03±4.72E−03+ | 4.55E−13±1.12E−13 |
F7 | 1.77E+02±1.47E+01≈ | 1.62E+02±2.47E+01≈ | 1.89E+02±2.52E+01+ | 7.11E+02±1.49E+02+ | 4.28E+02±2.94E+01+ | 2.72E+02±2.09E+01+ | 1.69E+02±2.47E+01 |
F8 | 1.02E+02±2.03E+01+ | 1.12E+02±1.87E+01+ | 7.77E+01±1.78E+01≈ | 3.44E+02±4.50E+01+ | 3.60E+02±1.62E+01+ | 1.81E+02±1.51E+01+ | 7.85E+01±1.58E+01 |
F9 | 2.32E+02±2.11E+02+ | 2.12E+02±1.18E+02+ | 1.08E+02±1.10E+02+ | 9.67E+03±2.08E+03+ | 5.35E+01±2.02E+02+ | 4.20E+02±9.59E+02+ | 2.62E+01±2.74E+01 |
F10 | 3.94E+03±5.03E+02≈ | 3.88E+03±4.66E+02≈ | 3.84E+03±3.84E+02≈ | 6.71E+03±1.07E+03+ | 1.22E+04±6.62E+02+ | 6.29E+03±4.18E+02+ | 3.78E+03±4.13E+02 |
F11 | 1.49E+02±3.44E+01+ | 1.65E+02±5.18E+01+ | 1.86E+02±4.90E+01+ | 1.42E+02±3.76E+01+ | 2.41E+02±2.99E+01+ | 1.07E+02±2.31E+01≈ | 1.18E+02±4.29E+01 |
F12 | 5.18E+05±2.49E+05+ | 1.42E+05±1.16E+05− | 8.07E+04±4.03E+04− | 5.70E+05±2.96E+05+ | 5.93E+06±3.15E+06+ | 1.84E+05±1.06E+05− | 3.81E+05±2.02E+05 |
F13 | 6.33E+02±2.47E+02+ | 1.50E+03±1.26E+03+ | 6.97E+02±4.59E+02≈ | 2.63E+03±3.33E+03+ | 5.36E+04±2.13E+05+ | 1.30E+03±1.42E+03+ | 5.10E+02±4.38E+02 |
F14 | 2.70E+04±1.69E+04≈ | 1.54E+04±1.17E+04− | 1.03E+04±7.06E+03− | 2.77E+04±2.05E+04≈ | 2.29E+05±9.37E+04+ | 1.11E+02±1.75E+01− | 3.64E+04±2.73E+04 |
F15 | 3.00E+02±2.31E+02+ | 5.40E+02±4.62E+02+ | 3.78E+02±4.07E+02≈ | 6.29E+03±5.46E+03+ | 3.97E+05±8.32E+05+ | 4.77E+03±2.46E+03+ | 1.99E+02±1.25E+02 |
F16 | 1.01E+03±2.61E+02≈ | 1.10E+03±2.75E+02+ | 8.91E+02±2.85E+02≈ | 1.89E+03±3.66E+02+ | 1.38E+03±6.26E+02+ | 7.69E+02±1.58E+02− | 9.49E+02±1.85E+02 |
F17 | 8.33E+02±1.48E+02+ | 8.14E+02±2.37E+02+ | 7.20E+02±1.69E+02≈ | 1.50E+03±3.63E+02+ | 1.25E+03±2.86E+02+ | 7.29E+02±1.12E+02≈ | 6.65E+02±1.77E+02 |
F18 | 1.40E+05±9.18E+04≈ | 1.03E+05±1.93E+05− | 6.48E+04±5.77E+04− | 1.39E+05±6.87E+04≈ | 2.71E+06±1.18E+06+ | 5.24E+03±2.46E+03− | 1.86E+05±1.80E+05 |
F19 | 6.16E+02±8.92E+02≈ | 3.76E+02±3.38E+02≈ | 6.33E+02±1.08E+03≈ | 1.39E+04±1.09E+04+ | 2.06E+04±1.24E+04+ | 7.03E+03±7.90E+03≈ | 7.31E+02±1.36E+03 |
F20 | 5.05E+02±2.01E+02+ | 6.17E+02±1.59E+02+ | 5.00E+02±2.19E+02+ | 1.25E+03±3.68E+02+ | 1.01E+03±3.19E+02+ | 4.80E+02±1.28E+02+ | 3.99E+02±1.64E+02 |
F21 | 3.04E+02±2.13E+01+ | 3.08E+02±2.28E+01+ | 2.72E+02±2.36E+01≈ | 4.77E+02±4.99E+01+ | 5.39E+02±1.84E+01+ | 3.48E+02±1.32E+01+ | 2.70E+02±1.12E+01 |
F22 | 3.17E+03±2.10E+03≈ | 3.96E+03±1.82E+03+ | 2.89E+03±2.18E+03≈ | 7.34E+03±1.02E+03+ | 1.18E+04±3.25E+03+ | 3.27E+03±3.46E+03≈ | 3.19E+03±2.08E+03 |
F23 | 5.46E+02±2.71E+01+ | 5.56E+02±2.92E+01+ | 5.22E+02±3.04E+01≈ | 8.30E+02±1.12E+02+ | 7.92E+02±1.84E+01+ | 5.95E+02±2.53E+01+ | 5.29E+02±2.52E+01 |
F24 | 6.35E+02±3.23E+01+ | 6.17E+02±2.89E+01+ | 5.77E+02±2.50E+01− | 8.99E+02±1.16E+02+ | 8.52E+02±1.54E+01+ | 6.45E+02±2.96E+01+ | 5.92E+02±2.36E+01 |
F25 | 5.08E+02±3.32E+01≈ | 5.15E+02±3.05E+01≈ | 5.21E+02±3.32E+01≈ | 5.59E+02±3.68E+01+ | 5.44E+02±2.80E+01+ | 5.76E+02±1.76E+01+ | 5.20E+02±3.22E+01 |
F26 | 1.42E+03±9.15E+02≈ | 1.59E+03±1.07E+03≈ | 1.70E+03±5.29E+02≈ | 3.89E+03±3.87E+03≈ | 4.48E+03±8.29E+02+ | 2.27E+03±1.62E+03+ | 1.51E+03±8.29E+02 |
F27 | 6.31E+02±2.76E+01≈ | 6.46E+02±3.54E+01≈ | 6.67E+02±3.19E+01≈ | 9.66E+02±1.74E+02+ | 6.46E+02±5.44E+01≈ | 6.21E+02±4.76E+01− | 6.48E+02±4.34E+01 |
F28 | 4.80E+02±2.21E+01≈ | 4.88E+02±2.04E+01≈ | 4.92E+02±2.25E+01≈ | 4.95E+02±2.72E+01≈ | 4.80E+02±2.30E+01≈ | 5.17E+02±2.04E+01+ | 4.93E+02±1.81E+01 |
F29 | 6.94E+02±1.96E+02≈ | 7.62E+02±1.50E+02≈ | 7.21E+02±1.59E+02≈ | 1.53E+03±3.60E+02+ | 1.39E+03±2.72E+02+ | 7.59E+02±8.20E+01≈ | 7.10E+02±1.80E+02 |
F30 | 7.02E+05±5.32E+04≈ | 7.03E+05±6.25E+04≈ | 7.43E+05±7.14E+04≈ | 1.05E+06±3.28E+05+ | 1.52E+07±1.16E+07+ | 8.25E+05±4.54E+04+ | 7.05E+05±5.83E+04 |
+/≈/− | 13/15/1 | 15/9/5 | 5/19/5 | 24/5/0 | 27/2/0 | 19/5/5 | / |
On the whole, 21 of the 29 functions have achieved desirable results, accounting for about 72.4%. Furthermore, the statistical results in the last row from Table 9 also demonstrate that MPSORL has reached better results when compared with the state-of-the-art algorithms. Moreover, Figure 8 shows the Friedman test results, and the MPSORL algorithm ranks first.
Additionally, the convergence properties of some functions are depicted. The detailed information is displayed in Figure 9. On F1, it can be observed that other algorithms are easily trapped in local optima except for MPSORL and HCLPSO, which have good convergency. MPSORL has high convergence accuracy, but its convergence speed is not as fast as SDSPO on F5. For F9 and F10, HCLPSO, EPSO, SDPSO and MPSORL perform similarly with respect to convergence. However, MPSORL keeps improving its fitness on F10. In addition, MRFO suffers from premature convergence during the optimization process. On F13 and F15, MPSORL has not only fast convergence speed but also high convergence accuracy. Moreover, MPSORL is remarkably better than other comparison algorithms on F17 and F21 in terms of higher convergence precision. After 250,000 evaluations, MPSORL is still improving on F20, whereas other algorithms have not further improved. By comparing these results, it can be concluded that the proposed MPSORL algorithm can outperform these compared algorithms on most of these test examples.
In order to further verify the effectiveness of the proposed MPSORL in solving high-dimensional problems, Table 10 presents the comparison results between MPSORL and the other five algorithms on 100-dimensional problems. Specifically, for simple multimodal functions (F4−F10), MPSORL ranks first on F5 and F7–F10, accounting for about 71.4%. For hybrid functions (F11−F20), MPSORL is significantly better than the other five comparison algorithms on F13, F16, F19 and F20. In parallel, MPSORL and the superior algorithms on F15 and F17 have no significant differences. For composition functions (F21−F30), MPSORL achieves the best results on F22, F24, F26 and F28. Among the remaining six functions, the best results of the other five functions are not significantly different from those of MPSORL except for F25. This implies that the proposed algorithm achieves better or comparable results on 21 of 29 functions. Furthermore, the statistical results in the last row of Table 10 show that MPSORL outperforms the latest compared algorithms.
IEEE CEC2017 with 100D | HCLPSO | SDPSO | MRFO | I-GWO | BeSD | MPSORL |
mean±std | mean±std | mean±std | mean±std | mean±std | mean±std | |
F1 | 6.74E+03±7.22E+03≈ | 7.59E+03±8.88E+03≈ | 5.59E+03±7.18E+03≈ | 1.30E+05±5.51E+04+ | 3.34E+03±2.87E+03≈ | 7.31E+03±7.60E+03 |
F3 | 7.51E+03±3.89E+03− | 2.07E+04±1.75E+04− | 8.58E+04±1.31E+04≈ | 9.01E+04±1.11E+04≈ | 3.42E+04±5.67E+03− | 8.75E+04±1.84E+04 |
F4 | 2.16E+02±3.25E+01≈ | 1.95E+02±4.18E+01− | 2.37E+02±4.09E+01≈ | 3.06E+02±3.91E+01+ | 2.81E+02±4.11E+01+ | 2.28E+02±5.13E+01 |
F5 | 2.91E+02±5.87E+01≈ | 3.05E+02±8.14E+01≈ | 7.98E+02±6.75E+01+ | 9.15E+02±3.20E+01+ | 6.46E+02±3.17E+01+ | 2.81E+02±5.72E+01 |
F6 | 2.56E−05±8.27E−05− | 4.90E−02±3.90E−02+ | 5.13E+01±6.93E+00+ | 1.93E+00±9.54E−01+ | 4.36E−01±1.80E−01+ | 8.82E−04±1.13E−03 |
F7 | 5.79E+02±7.60E+01+ | 5.43E+02±1.46E+02+ | 2.17E+03±2.71E+02+ | 1.10E+03±6.67E+01+ | 7.33E+02±4.82E+01+ | 4.22E+02±8.11E+01 |
F8 | 2.98E+02±5.97E+01≈ | 3.21E+02±6.77E+01+ | 8.83E+02±8.99E+01+ | 9.15E+02±3.05E+01+ | 6.52E+02±3.42E+01+ | 2.88E+02±6.62E+01 |
F9 | 1.55E+03±7.37E+02+ | 4.79E+03±2.78E+03+ | 2.06E+04±1.69E+03+ | 4.15E+03±3.17E+03+ | 1.91E+04±5.45E+03+ | 5.35E+02±3.20E+02 |
F10 | 1.17E+04±1.02E+03+ | 1.20E+04±7.35E+02+ | 1.41E+04±1.21E+03+ | 2.89E+04±7.52E+02+ | 1.84E+04±6.20E+02+ | 1.10E+04±8.17E+02 |
F11 | 7.57E+02±1.78E+02≈ | 9.67E+02±1.92E+02+ | 5.66E+02±1.18E+02− | 5.74E+03±1.66E+03+ | 6.27E+02±1.35E+02− | 7.10E+02±1.19E+02 |
F12 | 7.72E+05±3.83E+05− | 3.04E+05±1.00E+05− | 1.40E+06±5.88E+05+ | 4.01E+07±1.53E+07+ | 2.26E+06±7.87E+05+ | 1.03E+06±4.16E+05 |
F13 | 1.40E+03±8.97E+02+ | 1.64E+03±9.03E+02+ | 6.05E+03±8.30E+03+ | 7.10E+03±7.79E+03+ | 3.27E+03±1.64E+03+ | 9.36E+02±4.44E+02 |
F14 | 1.11E+05±3.95E+04≈ | 5.95E+04±2.98E+04− | 1.33E+05±8.46E+04≈ | 3.85E+06±1.83E+06+ | 4.78E+03±3.89E+03− | 1.43E+05±6.90E+04 |
F15 | 6.80E+02±2.92E+02+ | 5.05E+02±1.33E+02≈ | 2.34E+03±2.69E+03+ | 3.05E+03±3.30E+03+ | 5.44E+02±3.77E+02≈ | 5.34E+02±1.39E+02 |
F16 | 3.20E+03±3.53E+02+ | 2.90E+03±4.68E+02≈ | 3.95E+03±6.79E+02+ | 5.73E+03±1.58E+03+ | 3.33E+03±2.88E+02+ | 2.76E+03±4.80E+02 |
F17 | 2.41E+03±3.39E+02≈ | 2.22E+03±2.87E+02≈ | 2.96E+03±5.02E+02+ | 4.80E+03±4.04E+02+ | 2.15E+03±2.11E+02≈ | 2.23E+03±2.91E+02 |
F18 | 2.09E+05±8.99E+04− | 1.17E+05±3.31E+04− | 3.29E+05±1.21E+05≈ | 1.13E+07±7.10E+06+ | 5.71E+04±1.82E+04− | 4.13E+05±1.83E+05 |
F19 | 4.43E+02±2.87E+02+ | 3.32E+02±2.18E+02≈ | 2.93E+03±3.10E+03+ | 3.16E+03±3.87E+03+ | 9.38E+02±5.61E+02+ | 2.85E+02±1.11E+02 |
F20 | 2.25E+03±2.52E+02+ | 2.08E+03±3.21E+02+ | 3.19E+03±5.90E+02+ | 4.25E+03±3.40E+02+ | 2.43E+03±2.55E+02+ | 1.87E+03±2.71E+02 |
F21 | 5.47E+02±5.29E+01≈ | 5.32E+02±6.67E+01≈ | 9.60E+02±1.28E+02+ | 1.12E+03±3.20E+01+ | 7.73E+02±2.92E+01+ | 5.41E+02±6.73E+01 |
F22 | 1.32E+04±1.15E+03+ | 1.21E+04±4.15E+03+ | 1.68E+04±1.49E+03+ | 2.98E+04±1.01E+03+ | 2.02E+04±4.18E+02+ | 1.17E+04±3.25E+03 |
F23 | 8.03E+02±2.63E+01+ | 7.83E+02±3.24E+01≈ | 1.33E+03±1.38E+02+ | 1.38E+03±3.55E+01+ | 1.06E+03±2.63E+01+ | 7.86E+02±2.07E+01 |
F24 | 1.31E+03±5.20E+01≈ | 1.30E+03±5.71E+01≈ | 2.19E+03±2.78E+02+ | 1.76E+03±3.11E+01+ | 1.32E+03±9.25E+01≈ | 1.29E+03±6.33E+01 |
F25 | 7.38E+02±6.47E+01− | 7.78E+02±6.94E+01≈ | 7.84E+02±5.27E+01≈ | 8.61E+02±4.59E+01+ | 8.62E+02±4.13E+01+ | 7.88E+02±6.23E+01 |
F26 | 7.29E+03±6.01E+02≈ | 7.72E+03±6.71E+02+ | 1.69E+04±7.42E+03+ | 1.22E+04±5.14E+02+ | 1.23E+04±2.30E+03+ | 6.28E+03±2.76E+03 |
F27 | 7.91E+02±3.21E+01≈ | 7.73E+02±3.15E+01≈ | 1.22E+03±1.85E+02+ | 9.53E+02±1.30E+02+ | 8.53E+02±5.71E+01+ | 7.77E+02±2.78E+01 |
F28 | 5.71E+02±3.64E+01≈ | 5.65E+02±2.80E+01≈ | 5.70E+02±3.28E+01≈ | 6.69E+02±3.07E+01+ | 6.69E+02±2.81E+01+ | 5.65E+02±2.94E+01 |
F29 | 2.93E+03±2.89E+02≈ | 3.01E+03±4.44E+02≈ | 3.97E+03±5.99E+02+ | 4.90E+03±4.35E+02+ | 3.49E+03±2.48E+02+ | 3.08E+03±4.84E+02 |
F30 | 5.83E+03±2.82E+03≈ | 5.73E+03±2.78E+03≈ | 7.14E+03±3.85E+03≈ | 2.43E+05±1.07E+05+ | 1.06E+04±2.71E+03+ | 5.80E+03±3.15E+03 |
+/≈/− | 10/14/5 | 10/14/5 | 20/8/1 | 28/1/0 | 21/4/4 | / |
Next, Figure 10 shows the result of the Friedman test. The MPSORL algorithm still ranks first. Moreover, the trends of convergence of some functions are plotted in Figure 11. The detailed analysis is as follows: First, MPSORL has higher accuracy than other algorithms on F7 and F9. For F10, the fitness value is still increasing in the later stages of evolution, indicating good search ability. Second, the results also show that MPSORL could provide a superior convergence rate with fewer evaluations on F13, F16, F24 and F26. In addition, SDPSO and HCLPSO have fast convergence speeds but poor exploitation on F20 and F22 compared to MPSORL. Overall, MPSORL can obtain better solutions with high accuracy and stronger local searching abilities. The conclusions also imply that strategy selection based on RL can improve the performance of MPSORL. Moreover, it can be seen that the performance of the MPSORL algorithm does not decrease with the increase of dimensions, and it is also competitive in higher dimensional problems.
CEC2019, which has 10 functions to optimize, is a more complicated test suite than CEC2017. In this section, seven PSO algorithms (LDWPSO, UPSO, CLPSO, LIPS, HCLPSO, EPSO and SDPSO) and three latest metaheuristic algorithms (MRFO, I-GWO and BeSD) are compared with MPSORL. The results of non-parametric test are shown in Table 11. MPSORL is obviously better than LDWPSO, UPSO, CLPSO, LIPS, HCLPSO, EPSO, MRFO, I-GWO and BeSD and is tied with SDPSO. The only weakness is that the performance of MPSORL is relatively worse than that of MRFO and BeSD on F31 and F32. The "No Free Lunch" Theorem [61], which states that there is no general-purpose optimization algorithm that can solve all types of optimization problems, may explain this phenomenon. At the same time, the Friedman test reveals that the MPSORL algorithm outperforms all of the compared algorithms. The results are plotted in Figure 12, which also validates that the framework of the reinforcement learning technique to guide the strategy selection of the PSO algorithm is effective on a different test suite.
IEEE CEC2019 | LDWPSO | UPSO | CLPSO | LIPS | HCLPSO | MPSORL |
mean±std | mean±std | mean±std | mean±std | mean±std | mean±std | |
F31 | 5.22E+04±8.95E+04≈ | 5.71E+04±3.49E+04− | 8.62E+05±5.36E+05+ | 4.70E+04±4.30E+04≈ | 6.08E+04±1.20E+05≈ | 5.93E+04±6.88E+04 |
F32 | 2.61E+02±1.04E+02≈ | 3.23E+02±1.35E+02≈ | 6.25E+02±1.54E+02+ | 2.73E+02±9.38E+01≈ | 2.43E+02±8.17E+01≈ | 2.44E+02±1.03E+02 |
F33 | 3.95E−01±7.47E−02≈ | 4.80E−01±4.01E−01+ | 9.06E−01±3.06E−01+ | 6.22E−01±8.09E−01≈ | 3.95E−01±7.47E−02+ | 3.66E−01±1.33E−01 |
F34 | 8.52E+00±4.08E+00+ | 9.20E+00±2.93E+00+ | 4.27E+00±1.21E+00≈ | 1.05E+01±4.19E+00+ | 4.41E+00±1.47E+00≈ | 4.55E+00±1.77E+00 |
F35 | 1.05E−01±6.19E−02+ | 3.86E−02±1.90E−02≈ | 6.52E−02±2.62E−02+ | 1.00E−02±8.04E−03− | 5.13E−02±2.18E−02≈ | 3.33E−02±2.24E−02 |
F36 | 3.15E−01±5.71E−01+ | 4.36E−01±5.00E−01+ | 1.59E−01±7.46E−02+ | 4.76E−01±5.95E−01+ | 1.99E−03±2.78E−03+ | 3.89E−04±1.69E−03 |
F37 | 4.41E+02±2.38E+02+ | 4.66E+02±1.71E+02+ | 1.47E+02±7.73E+01≈ | 7.55E+02±2.49E+02+ | 2.49E+02±1.11E+02+ | 1.61E+02±1.30E+02 |
F38 | 1.99E+00±4.61E−01+ | 2.21E+00±4.28E−01+ | 2.03E+00±2.62E−01+ | 3.04E+00±4.87E−01+ | 1.65E+00±3.53E−01+ | 1.31E+00±6.48E−01 |
F39 | 1.32E−01±6.08E−02≈ | 6.99E−02±3.05E−02− | 1.75E−01±3.92E−02+ | 1.23E−01±4.73E−02≈ | 1.01E−01±3.46E−02− | 1.30E−01±3.08E−02 |
F40 | 1.42E+01±9.09E+00≈ | 1.46E+01±8.32E+00≈ | 1.88E+01±3.91E+00+ | 1.93E+01±3.65E+00≈ | 1.54E+01±8.57E+00≈ | 1.21E+01±9.91E+00 |
+/≈/− | 5/5/0 | 5/3/2 | 8/2/0 | 4/5/1 | 4/5/1 | / |
IEEE CEC2019 | EPSO | SDPSO | MRFO | I-GWO | BeSD | MPSORL |
mean±std | mean±std | mean±std | mean±std | mean±std | mean±std | |
F31 | 3.20E+04±2.87E+04≈ | 4.42E+04±5.14E+04≈ | 0.00E+00±0.00E+00− | 7.24E+04±1.35E+05≈ | 0.00E+00±0.00E+00− | 5.93E+04±6.88E+04 |
F32 | 2.30E+02±8.66E+01≈ | 2.58E+02±1.05E+02≈ | 3.70E+00±3.69E−01− | 1.06E+03±3.38E+02+ | 4.84E+00±1.97E+00− | 2.44E+02±1.03E+02 |
F33 | 3.41E−01±1.55E−01≈ | 3.55E−01±1.40E−01≈ | 3.95E−01±7.47E−02≈ | 1.95E+00±1.18E+00+ | 9.35E−01±2.89E−01+ | 3.66E−01±1.33E−01 |
F34 | 5.82E+00±1.98E+00+ | 4.48E+00±1.50E+00≈ | 2.50E+01±1.55E+01+ | 1.98E+01±4.20E+00+ | 8.50E+00±1.96E+00+ | 4.55E+00±1.77E+00 |
F35 | 8.57E−02±4.08E−02+ | 4.81E−02±3.37E−02≈ | 1.11E−01±1.07E−01+ | 5.26E−01±9.12E−02+ | 5.04E−02±1.27E−02≈ | 3.33E−02±2.24E−02 |
F36 | 2.46E−01±2.42E−01+ | 2.72E−02±1.21E−01≈ | 2.13E+00±1.70E+00+ | 1.93E−01±3.60E−01+ | 1.34E−01±1.17E−01+ | 3.89E−04±1.69E−03 |
F37 | 2.40E+02±1.47E+02≈ | 1.80E+02±1.04E+02≈ | 7.29E+02±3.16E+02+ | 7.33E+02±2.42E+02+ | 4.46E+02±1.29E+02+ | 1.61E+02±1.30E+02 |
F38 | 1.65E+00±4.69E−01+ | 1.19E+00±5.05E−01≈ | 2.45E+00±3.70E−01+ | 2.06E+00±2.53E−01+ | 2.50E+00±1.64E−01+ | 1.31E+00±6.48E−01 |
F39 | 1.27E−01±3.64E−02≈ | 1.32E−01±2.78E−02≈ | 2.05E−01±7.63E−02+ | 1.84E−01±4.15E−02+ | 1.11E−01±1.76E−02− | 1.30E−01±3.08E−02 |
F40 | 1.24E+01±9.65E+00+ | 1.22E+01±9.85E+00≈ | 1.50E+01±9.02E+00+ | 1.50E+01±9.03E+00+ | 1.76E+01±4.33E+00+ | 1.21E+01±9.91E+00 |
+/≈/− | 5/5/0 | 0/10/0 | 7/1/2 | 9/1/0 | 6/1/3 | / |
The research background of the problem is the spread spectrum radar polyphase code design, which is a nonlinear non-convex min-max problem with continuous variables having numerous local optima [62]. The mathematical model is expressed as follows:
minx∈Xf(x)=max{ϕ1(x),ϕ2(x),⋯,ϕ2m(x)} | (4.1) |
where
X={(x1,⋯,xn)∈Rn∣0≤xj≤2π(j=1,2,⋯,n)},m=2n−1 | (4.2) |
ϕ2i−1(x)=n∑j=icos(j∑k=|2i−j−1|+1xk),i=1,2,⋯,n | (4.3) |
ϕ2i(x)=0.5+n∑j=i+1cos(j∑k=|2i−j|+1xk),i=1,2,⋯,n−1 | (4.4) |
ϕm+i(x)=−ϕi(x). | (4.5) |
The parameters are set as follows: The dimension is 20, Max_Fes is set to 100,000, and the population size is 30. The boxplot of statistical results after 30 independent runs is shown in Figure 13. It is apparent that the average optimal value of MPSORL is minimum. It indicates that the proposed algorithm has certain advantages in solving the practical application problem.
A multi-strategy self-learning method is constructed by using Q-learning theory, and a novel multi-strategy selection particle swarm optimizer is designed. In order to intuitively show the superior performance of MPSORL, the design method and convergence performance about the proposed algorithm are further discussed from the following three aspects: 1) state division, 2) convergence comparison and 3) diversity analysis.
The state division depends on the transformation of the particle fitness. This section discusses the effect of state partitioning, and here two cases of uniform and non-uniform partitioning are compared and analyzed. The uniform partition state is set to S1 = [20, 40, 60, 80]. The non-uniform partition state is set to S2 = [10, 25, 45, 70].
The other parameters set are the same as Section 4.1. MPSORL runs 30 times independently on CEC2017 with 30, 50, and 100 dimensions, respectively. As can be seen from Table 12, state partitioning has little effect on the 30 dimensional problems. However, MPSORL with non-uniform state division provides higher R+ values than R− values in all cases. Meanwhile, in the 50 and 100 dimensions, it is not difficult to find that the effect of non-uniform state division is better than that of uniform division. The higher the dimension is, the better the non-uniform partition. As a result, we can conclude that the state division has a direct effect on the algorithm's performance with the increased number of decision variables. Therefore, the state of the proposed algorithm is set to non-uniform status division S2.
MPSORL | Non-uniform vs. Uniform status division | |||||
Wins (+) | Losses (−) | Ties | R+ | R− | p-value | |
30D | 14 | 15 | 0 | 220 | 215 | 0.957 |
50D | 19 | 10 | 0 | 278 | 157 | 0.191 |
100D | 20 | 9 | 0 | 297 | 138 | 0.086 |
To compare the difference between the convergence performance of multi-strategy PSO algorithms, Table 13 presents some of the common functions. In the following, these functions are used to compare and analyze the convergence speed of multi-strategy PSO algorithms, including EPSO, SDPSO, and MPSORL. Among them, EPSO selects the optimizer based on the success rate of strategies. SDPSO selects strategies by calculating their payoffs. However, the MPSORL algorithm chooses the strategies based on the Q-table. The parameters are set as follows: The number of runs is 20, the population size is 40, and Max_Iter is 500. On these different types of functions, the proposed MPSORL converges faster than the other two multi-strategy PSO algorithms by looking at the trajectories of particle movement, as shown in Figure 14(b), (d), (f), (h). Therefore, according to the experiments in Section 4 and further verification in this section, the results reveal that the MPSORL algorithm outperforms other state-of-the-art algorithms in terms of accuracy, convergence speed and non-parametric statistical significance. It can be proved that the proposed MPSORL, guided by RL technique, has good robustness, superiority and competitiveness.
Function type | No. | Function | F(x∗) | D | search range |
Bowl-Shaped | F41 | Sphere Function | 0 | 2 | [-100 100] |
Many Local Minima | F42 | Rastrigin Function | 0 | 2 | [-5.12 5.12] |
Many Local Minima | F43 | Ackley Function | 0 | 2 | [-32.768, 32.768] |
Plate-Shaped | F44 | Matyas Function | 0 | 2 | [-10 10] |
In order to further verify the exploration and exploitation capabilities of MPSORL, the diversities of several multi-swarm PSO variants (HCLPSO, EPSO and SDPSO) are compared. The diversity metric of the population is as follows [63]:
Diversity=1NN∑i=1√D∑j=1(xij−ˉxj)2 | (5.1) |
ˉxj=1NN∑i=1xij | (5.2) |
where xij indicates the jth dimension of the ith particle, and ˉxj represents the jth dimension of the mean position of the population. The population size is 40, and Max_Iter is 500. Figure 15(a)–(d) illustrates the diversity curves of the four functions proposed in Section 5.2. On all different types of functions, MPSORL has the greatest diversity at the early stage while preserving an appreciable convergence trend at the later stage, focusing on fine search. Conversely, HCLPSO has the worst diversity compared to other PSO variants. EPSO shows inferior diversity on the Sphere, Rastrigin, and Matyas functions, while facing a weaker exploitation ability on these four functions after 200 iterations. Similarly, SDPSO maintains stronger diversity in most cases but has some defects, such as poor local search on the Rastrigin and Matyas functions. To summarize, owing to the introduction of RL techniques and the effective multi-strategy self-learning mechanism, MPSORL achieves a good trade-off between exploration and exploitation compared with several other multi-swarm PSO variants.
This paper combines the RL technique with the PSO algorithm as the research engine, and then an adaptive multi-strategy self-learning framework based on Q-learning is proposed. During the evolution, each particle matches a strategy. The strategy is selected based on the Q-table. The information associated with the Q-table consists of states, action and reward. Based on the student achievement distribution analogy, a non-uniform state division method is designed to better match the change in dimensions of decision variables. Extensive experimental results show that the multi-strategy self-learning mechanism guided by the RL technique is more flexible and can effectively improve the performance of the proposed algorithm.
The multi-strategy learning framework can also be applied to other intelligent algorithms to solve global optimization problems, such as the differential evolution algorithm, the genetic algorithm, etc. MPSORL has achieved better performance in searching for optimal solutions to problems when compared with some state-of-the-art algorithms. However, as with most swarm intelligent approaches, the proposed algorithm is time-consuming in dealing with large-scale complex optimization problems. In addition, this work simply provides valuable insights into RL-guided PSO algorithms. Therefore, it can be expected in future research that developing more efficient strategies for strategy pools and optimizing the self-adaptive selection mechanism can further improve the efficiency of the algorithms.
This work is supported by the National Natural Science Foundation of China (No.61966030), Key Laboratory of Industrial Internet of Things and Network Control and Ministry of Education (2022-ZJ-Y21).
The authors declare no conflicts of interest.
[1] |
Zhang WJ, Luo JG, Kong LY (2016) The genus Alpinia: A review of its phytochemistry and pharmacology. World J Tradit Chinese Med 2: 26–41. https://doi.org/10.15806/j.issn.2311-8571.2015.0026 doi: 10.15806/j.issn.2311-8571.2015.0026
![]() |
[2] |
Abubakar IB, Malami I, Yahaya Y, et al. (2018) A review on the ethnomedicinal uses, phytochemistry and pharmacology of Alpinia officinarum Hance. J Ethnopharmacol 224: 45–62. https://doi.org/10.1016/j.jep.2018.05.027 doi: 10.1016/j.jep.2018.05.027
![]() |
[3] | Ravindran PN, Pillai GS, Balachandran I (2012) 15-Galangal. In: Peter KV (Ed.), Handbook of herbs and spices, 2nd ed., Cambridge: Woodhead Publishing, 303–318. https://doi.org/10.1533/9780857095688.303 |
[4] |
Ghosh S, Rangan L (2013) Alpinia: The gold mine of future therapeutics. 3 Biotech 3: 173–185. https://doi.org/10.1007/s13205-012-0089-x doi: 10.1007/s13205-012-0089-x
![]() |
[5] | Criley RA (2014) Alpinia to Zingiber–zingiberales in commercial floriculture. XXIX International Horticultural Congress on Horticulture: Sustaining Lives, Livelihoods and Landscapes (IHC2014): 1104, 435–454. https://doi.org/10.17660/ActaHortic.2015.1104.64 |
[6] |
Eram S, Mujahid M, Bagga P, et al. (2019) A review on phytopharmacological activity of Alpinia galanga. Int J Pharm Pharm Sci 11: 6–11. https://doi.org/10.22159/ijpps.2019v11i3.31352 doi: 10.22159/ijpps.2019v11i3.31352
![]() |
[7] | Kazeminia M, Mehrabi A, Mahmoudi R (2022) Chemical composition, biological activities, and nutritional application of Asteraceae family herbs: A systematic review. Trends Phytochem Res 6: 187–213. |
[8] | Olaoluwa O, Taiwo O, Nahar L, et al. (2022) Ethnopharmacology, phytochemistry and biological activities of selected African species of the genus Ficus. Trends Phytochem Res 6: 46–69. |
[9] |
Basri AM, Taha H, Ahmad N (2017) A review on the pharmacological activities and phytochemicals of Alpinia officinarum (galangal) extracts derived from bioassay-guided fractionation and isolation. Pharmacogn Rev 11: 43. https://doi.org/10.4103/phrev.phrev_55_16 doi: 10.4103/phrev.phrev_55_16
![]() |
[10] | Suja S, Chinnaswamy P (2008) Inhibition of in vitro cytotoxic effect evoked by Alpinia galanga and Alpinia officinarum on PC - 3 cell line. Anc Sci Life 27: 33–40. |
[11] |
da Cunha GH, de Moraes MO, Fechine FV, et al. (2013) Vasorelaxant and antihypertensive effects of methanolic fraction of the essential oil of Alpinia zerumbet. Vascul Pharmacol 58: 337–345. https://doi.org/10.1016/j.vph.2013.04.001 doi: 10.1016/j.vph.2013.04.001
![]() |
[12] |
Ramanunny AK, Wadhwa S, Gulati M, et al. (2022) Journey of Alpinia galanga from kitchen spice to nutraceutical to folk medicine to nanomedicine. J Ethnopharmacol 291: 115144. https://doi.org/10.1016/j.jep.2022.115144 doi: 10.1016/j.jep.2022.115144
![]() |
[13] |
Bitari A, Oualdi I, Touzani R, et al. (2023) Alpinia officinarum Hance: A mini review. Mater Today Proc 72: 3869–3874. https://doi.org/10.1016/j.matpr.2022.10.080 doi: 10.1016/j.matpr.2022.10.080
![]() |
[14] |
Ibrahim H, Aziz AN, Syamsir DR, et al. (2009) Essential oils of Alpinia conchigera Griff. and their antimicrobial activities. Food Chem 113: 575–577. https://doi.org/10.1016/j.foodchem.2008.08.033 doi: 10.1016/j.foodchem.2008.08.033
![]() |
[15] | Khumpirapang N, Suknuntha K, Anuchapreeda S, et al. (2021) Binding effects of Alpinia galanga oil and its nanoemulsion 1 to GABAA receptors in rat cortical membranes. https://doi.org/10.21203/rs.3.rs-816924/v1 |
[16] |
Cavalcanti BC, Ferreira JRO, Cabral IO, et al. (2012) Genetic toxicology evaluation of essential oil of Alpinia zerumbet and its chemoprotective effects against H2O2-induced DNA damage in cultured human leukocytes. Food Chem Toxicol 50: 4051–4061. https://doi.org/10.1016/j.fct.2012.03.038 doi: 10.1016/j.fct.2012.03.038
![]() |
[17] |
Sahoo S, Singh S, Sahoo A, et al. (2020) Molecular and phytochemical stability of long term micropropagated greater galanga (Alpinia galanga) revealed suitable for industrial applications. Ind Crops Prod 148: 112274. https://doi.org/10.1016/j.indcrop.2020.112274 doi: 10.1016/j.indcrop.2020.112274
![]() |
[18] |
Xin M, Guo S, Zhang W, et al. (2017) Chemical constituents of supercritical extracts from Alpinia officinarum and the feeding deterrent activity against Tribolium castaneum. Molecules 22: 647. https://doi.org/10.3390/molecules22040647 doi: 10.3390/molecules22040647
![]() |
[19] |
Zhang L, Liang X, Ou Z, et al. (2020) Screening of chemical composition, anti-arthritis, antitumor and antioxidant capacities of essential oils from four Zingiberaceae herbs. Ind Crops Prod 149: 112342. https://doi.org/10.1016/j.indcrop.2020.112342 doi: 10.1016/j.indcrop.2020.112342
![]() |
[20] |
Zhang L, Pan C, Ou Z, et al. (2020) Chemical profiling and bioactivity of essential oils from Alpinia officinarum Hance from ten localities in China. Ind Crops Prod 153: 112583. https://doi.org/10.1016/j.indcrop.2020.112583 doi: 10.1016/j.indcrop.2020.112583
![]() |
[21] |
de Lira CS, Pontual EV, de Albuquerque LP, et al. (2015) Evaluation of the toxicity of essential oil from Alpinia purpurata inflorescences to Sitophilus zeamais (maize weevil). Crop Prot 71: 95–100. https://doi.org/10.1016/j.cropro.2015.02.004 doi: 10.1016/j.cropro.2015.02.004
![]() |
[22] |
Santos GKN, Dutra KA, Barros RA, et al. (2012) Essential oils from Alpinia purpurata (Zingiberaceae): Chemical composition, oviposition deterrence, larvicidal and antibacterial activity. Ind Crops Prod 40: 254–260. https://doi.org/10.1016/j.indcrop.2012.03.020 doi: 10.1016/j.indcrop.2012.03.020
![]() |
[23] |
Pereira PS, Maia AJ, Duarte AE, et al. (2018) Cytotoxic and anti-kinetoplastid potential of the essential oil of Alpinia speciosa K. Schum. Food Chem Toxicol 119: 387–391. https://doi.org/10.1016/j.fct.2018.01.024 doi: 10.1016/j.fct.2018.01.024
![]() |
[24] |
Santos BA, Roman-Campos D, Carvalho MS, et al. (2011) Cardiodepressive effect elicited by the essential oil of Alpinia speciosa is related to L-type Ca2+ current blockade. Phytomedicine 18: 539–543. https://doi.org/10.1016/j.phymed.2010.10.015 doi: 10.1016/j.phymed.2010.10.015
![]() |
[25] |
da Cruz JD, Mpalantinos MA, Ramos A de S, et al. (2020) Chemical standardization, antioxidant activity and phenolic contents of cultivated Alpinia zerumbet preparations. Ind Crops Prod 151: 112495. https://doi.org/10.1016/j.indcrop.2020.112495 doi: 10.1016/j.indcrop.2020.112495
![]() |
[26] | Sethunga M, Ranaweera K, Gunathilake K, et al. (2022) Recent advances in the extraction methods of essential oils and oleoresins from plant materials and its potential applications: A comprehensive review. J Food Bioprocess Eng 5: 151–167. |
[27] |
Dhifi W, Bellili S, Jazi S, et al. (2016) Essential oils' chemical characterization and investigation of some biological activities: A critical review. Medicines 3: 25. https://doi.org/10.3390/medicines3040025 doi: 10.3390/medicines3040025
![]() |
[28] | Olalere OA, Gan CY, Taiwo AE, et al. (2024) Essential oils: Sustainable extraction techniques and nutraceuticals perspectives. In: Sarkar T, Pati S (Eds.), Bioactive Extraction and Application in Food and Nutraceutical Industries, Springer, 373–389. https://doi.org/10.1007/978-1-0716-3601-5_15 |
[29] |
Van HT, Thang TD, Luu TN, et al. (2021) An overview of the chemical composition and biological activities of essential oils from: Alpinia genus (Zingiberaceae). RSC Adv 11: 37767–37783. https://doi.org/10.1039/D1RA07370B doi: 10.1039/D1RA07370B
![]() |
[30] |
Zhou C, Abdel-Samie MA, Li C, et al. (2020) Active packaging based on swim bladder gelatin/galangal root oil nanofibers: Preparation, properties and antibacterial application. Food Packag Shelf Life 26: 100586. https://doi.org/10.1016/j.fpsl.2020.100586 doi: 10.1016/j.fpsl.2020.100586
![]() |
[31] |
Aziman N, Abdullah N, Noor ZM, et al. (2014) Phytochemical profiles and antimicrobial activity of aromatic Malaysian herb extracts against food-borne pathogenic and food spoilage microorganisms. J Food Sci 79: M583–M592. https://doi.org/10.1111/1750-3841.12419 doi: 10.1111/1750-3841.12419
![]() |
[32] |
Das G, Patra JK, Gonçalves S, et al. (2020) Galangal, the multipotent super spices: A comprehensive review. Trends Food Sci Technol 101: 50–62. https://doi.org/10.1016/j.tifs.2020.04.032 doi: 10.1016/j.tifs.2020.04.032
![]() |
[33] |
Kress WJ, Liu AZ, Newman M, et al. (2005) The molecular phylogeny of Alpinia (Zingiberaceae): A complex and polyphyletic genus of gingers. Am J Bot 92: 167–178. https://doi.org/10.3732/ajb.92.1.167 doi: 10.3732/ajb.92.1.167
![]() |
[34] |
Victório CP (2011) Therapeutic value of the genus Alpinia, Zingiberaceae. Rev Bras Farmacogn 21: 194–201. https://doi.org/10.1590/S0102-695X2011005000025 doi: 10.1590/S0102-695X2011005000025
![]() |
[35] |
Palanirajan A, Kannappan P, Kanniappan GV (2022) Anticancer activity of Alpinia purpurata (Vieill) K. Schum. against MNU and testosterone induced prostate cancer in male Wistar albino rats. Pharmacol Res-Mod Chinese Med 3: 100105. https://doi.org/10.1016/j.prmcm.2022.100105 doi: 10.1016/j.prmcm.2022.100105
![]() |
[36] |
Liang W, Ge X, Lin Q, et al. (2024) Assembly mechanism of glycosylated soybean protein isolate-chitosan nanocomposite particles and its Pickering emulsion construction for stabilization of Alpinia galanga essential oil: A mechanistic investigation. Ind Crops Prod 217: 118869. https://doi.org/10.1016/j.indcrop.2024.118869 doi: 10.1016/j.indcrop.2024.118869
![]() |
[37] |
Liu P, Wu SL, Wang T, et al. (2023) Four new phenolic compounds from the fruits of Alpinia galanga. Phytochem Lett 55: 75–79. https://doi.org/10.1016/j.phytol.2023.04.001 doi: 10.1016/j.phytol.2023.04.001
![]() |
[38] |
Ding P, Yang L, Feng C, et al. (2019) Research and application of Alpinia officinarum in medicinal field. Chinese Herb Med 11: 132–140. https://doi.org/10.1016/j.chmed.2019.04.003 doi: 10.1016/j.chmed.2019.04.003
![]() |
[39] |
Rahman MA, Rahman MM, Ahmed N (2012) Essential oil of Alpinia calcarata Rosc. Rhizome: Heals inflammation and nociception in animal models. J Biol Act Prod Nat 2: 365–376. https://doi.org/10.1080/22311866.2012.10719145 doi: 10.1080/22311866.2012.10719145
![]() |
[40] |
Perveen R, Islam F, Khanum J, et al. (2012) Preventive effect of ethanol extract of Alpinia calcarata Rosc on Ehrlich's ascitic carcinoma cell induced malignant ascites in mice. Asian Pac J Trop Med 5: 121–125. https://doi.org/10.1016/S1995-7645(12)60009-1 doi: 10.1016/S1995-7645(12)60009-1
![]() |
[41] |
Hema PS, Nair MS (2009) Flavonoids and other constituents from the rhizomes of Alpinia calcarata. Biochem Syst Ecol 37: 52–54. https://doi.org/10.1016/j.bse.2009.01.001 doi: 10.1016/j.bse.2009.01.001
![]() |
[42] |
Ramya R, Kalaiselvi M, Narmadha R, et al. (2015) Secondary metabolite credentials and in vitro free radical scavenging activity of Alpinia calcarata. J Acute Med 5: 33–37. https://doi.org/10.1016/j.jacme.2015.02.005 doi: 10.1016/j.jacme.2015.02.005
![]() |
[43] |
Erusappan T, Paramasivam S, Ekambaram SP (2022) Identification of galangin as the bioactive compound from Alpinia calcarata (Haw.) Roscoe rhizomes to inhibit IRAK-1/MAPK/NF-κB p65 and JAK-1 signaling in LPS stimulated RAW 264.7 cells. J Ethnopharmacol 288: 114975. https://doi.org/10.1016/j.jep.2022.114975 doi: 10.1016/j.jep.2022.114975
![]() |
[44] |
Wu Y, Zhang WJ, Huang DY, et al. (2015) Chemical compositions and insecticidal activities of Alpinia kwangsiensis essential oil against Lasioderma serricorne. Molecules 20: 21939–21945. https://doi.org/10.3390/molecules201219818 doi: 10.3390/molecules201219818
![]() |
[45] |
Wang S, Xiang J, Zhang G, et al. (2024) Essential oil from Fructus Alpinia zerumbet ameliorates atherosclerosis by activating PPARγ-LXRα-ABCA1/G1 signaling pathway. Phytomedicine 123: 155227. https://doi.org/10.1016/j.phymed.2023.155227 doi: 10.1016/j.phymed.2023.155227
![]() |
[46] |
Chan EWC, Wong SK (2015) Phytochemistry and pharmacology of ornamental gingers, Hedychium coronarium and Alpinia purpurata: A review. J Integr Med 13: 368–379. https://doi.org/10.1016/S2095-4964(15)60208-4 doi: 10.1016/S2095-4964(15)60208-4
![]() |
[47] |
Raj CA, Ragavendran P, Sophia D, et al. (2012) Evaluation of in vitro antioxidant and anticancer activity of Alpinia purpurata. Chin J Nat Med 10: 263–268. https://doi.org/10.3724/SP.J.1009.2012.00263 doi: 10.3724/SP.J.1009.2012.00263
![]() |
[48] |
He ZH, Ge W, Yue GGL, et al. (2010) Anti-angiogenic effects of the fruit of Alpinia oxyphylla. J Ethnopharmacol 132: 443–449. https://doi.org/10.1016/j.jep.2010.08.024 doi: 10.1016/j.jep.2010.08.024
![]() |
[49] |
Yu SH, Kim HJ, Jeon SY, et al. (2020) Anti-inflammatory and anti-nociceptive activities of Alpinia oxyphylla Miquel extracts in animal models. J Ethnopharmacol 260: 112985. https://doi.org/10.1016/j.jep.2020.112985 doi: 10.1016/j.jep.2020.112985
![]() |
[50] |
Xie J, Sun B, Wang S, et al. (2009) Isolation and purification of nootkatone from the essential oil of fruits of Alpinia oxyphylla Miquel by high-speed counter-current chromatography. Food Chem 117: 375–380. https://doi.org/10.1016/j.foodchem.2009.04.011 doi: 10.1016/j.foodchem.2009.04.011
![]() |
[51] |
Cheng CY, Chiang S, Kao ST, et al. (2021) Alpinia oxyphylla Miq extract reduces cerebral infarction by downregulating JNK-mediated TLR4/T3JAM-and ASK1-related inflammatory signaling in the acute phase of transient focal cerebral ischemia in rats. Chin Med 16: 1–21. https://doi.org/10.1186/s13020-021-00495-2 doi: 10.1186/s13020-021-00495-2
![]() |
[52] |
Qi Y, Cheng X, Jing H, et al. (2019) Effect of Alpinia oxyphylla—Schisandra chinensis herb pair on inflammation and apoptosis in Alzheimer's disease mice model. J Ethnopharmacol 237: 28–38. https://doi.org/10.1016/j.jep.2019.03.029 doi: 10.1016/j.jep.2019.03.029
![]() |
[53] |
Dong J, Zhou M, Qin Q, et al. (2023) Structurally diverse new eudesmane sesquiterpenoids with anti-inflammatory activity from the fruits of Alpinia oxyphylla. Bioorg Chem 134: 106431. https://doi.org/10.1016/j.bioorg.2023.106431 doi: 10.1016/j.bioorg.2023.106431
![]() |
[54] |
Wang M, Bi W, Fan K, et al. (2018) Ameliorating effect of Alpinia oxyphylla—Schisandra chinensis herb pair on cognitive impairment in a mouse model of Alzheimer's disease. Biomed Pharmacother 97: 128–135. https://doi.org/10.1016/j.biopha.2017.10.088 doi: 10.1016/j.biopha.2017.10.088
![]() |
[55] |
Tie Y, Sun Z, Tong X, et al. (2024) Multi-omic analysis revealed the therapeutic mechanisms of Alpinia oxyphylla fructus water extract against bladder overactivity in spontaneously hypertensive rats. Phytomedicine 123: 155154. https://doi.org/10.1016/j.phymed.2023.155154 doi: 10.1016/j.phymed.2023.155154
![]() |
[56] |
Qiu C, Mu L, Wang J, et al. (2023) Sesquiterpenoids from the fruits of Alpinia oxyphylla Miq. and their neuroprotective effect. Phytochemistry 211: 113680. https://doi.org/10.1016/j.phytochem.2023.113680 doi: 10.1016/j.phytochem.2023.113680
![]() |
[57] |
Deng J, He B, He D, et al. (2016) A potential biopreservative: Chemical composition, antibacterial and hemolytic activities of leaves essential oil from Alpinia guinanensis. Ind Crops Prod 94: 281–287. https://doi.org/10.1016/j.indcrop.2016.09.004 doi: 10.1016/j.indcrop.2016.09.004
![]() |
[58] |
Roy B, Swargiary A, Giri BR (2012) Alpinia nigra (Family Zingiberaceae): An anthelmintic medicinal plant of north-east India. Adv Life Sci 2: 39–51. https://doi.org/10.5923/j.als.20120203.01 doi: 10.5923/j.als.20120203.01
![]() |
[59] |
Ghosh S (2014) Chemical composition and bioactivity studies of Alpinia nigra essential oils. Ind Crops Prod 53: 111–119. https://doi.org/10.1016/j.indcrop.2013.12.026 doi: 10.1016/j.indcrop.2013.12.026
![]() |
[60] |
Chen Z, He B, Zhou J, et al. (2016) Chemical compositions and antibacterial activities of essential oils extracted from Alpinia guilinensis against selected foodborne pathogens. Ind Crops Prod 83: 607–613. https://doi.org/10.1016/j.indcrop.2015.12.063 doi: 10.1016/j.indcrop.2015.12.063
![]() |
[61] |
Li QM, Luo JG, Wang XB, et al. (2013) Sesquiterpenes from the rhizomes of Alpinia japonica and their inhibitory effects on nitric oxide production. Fitoterapia 86: 29–34.https://doi.org/10.1016/j.fitote.2013.01.015 doi: 10.1016/j.fitote.2013.01.015
![]() |
[62] |
Wang CY, Song ZM, He CL, et al. (2017) A constituent of Alpinia katsumadai suppresses allergic airway inflammation. Phytochem Lett 22: 149–153. https://doi.org/10.1016/j.phytol.2017.09.009 doi: 10.1016/j.phytol.2017.09.009
![]() |
[63] |
Pogačar MŠ, Klančnik A, Bucar F, et al. (2015) Alpinia katsumadai extracts inhibit adhesion and invasion of Campylobacter jejuni in animal and human foetal small intestine cell lines. Phyther Res 29: 1585–1589. https://doi.org/10.1002/ptr.5396 doi: 10.1002/ptr.5396
![]() |
[64] |
Mahawer S, Kumar R, Prakash O, et al. (2023) A review of phytochemical and pharmacological properties of Alpinia malaccensis (Burm. F.) Roscoe.(Zingiberaceae). Curr Top Med Chem 23: 1964–1972. https://doi.org/10.2174/1568026623666230522104104 doi: 10.2174/1568026623666230522104104
![]() |
[65] |
Hong Y, Liu X, Wang H, et al. (2022) Chemical composition, anticancer activities and related mechanisms of the essential oil from Alpinia coriandriodora rhizome. Ind Crops Prod 176: 114328. https://doi.org/10.1016/j.indcrop.2021.114328 doi: 10.1016/j.indcrop.2021.114328
![]() |
[66] | Wu Y, Zhang WJ, Huang DY, et al. (2015) Chemical Compositions and Insecticidal Activities of Alpinia kwangsiensis Essential Oil against Lasioderma serricorne. Molecules 20: 21939–21945. |
[67] |
Jantan I, Ahmad F, Ahmad AS (2004) Constituents of the rhizome and seed oils of greater galangal Alpinia galangal (L.) Willd. from Malaysia. J Essent Oil Res 16: 174–176. https://doi.org/10.1080/10412905.2004.9698687 doi: 10.1080/10412905.2004.9698687
![]() |
[68] |
Raina AP, Verma SK, Abraham Z (2014) Volatile constituents of essential oils isolated from Alpinia galanga Willd. (L.) and A. officinarum Hance rhizomes from North East India. J Essent Oil Res 26: 24–28. https://doi.org/10.1080/10412905.2013.822430 doi: 10.1080/10412905.2013.822430
![]() |
[69] |
Nampoothiri SV, Menon AN, Esakkidurai T, et al. (2016) Essential oil composition of Alpinia calcarata and Alpinia galanga rhizomes-a comparative study. J Essent Oil Bear Plants 19: 82–87. https://doi.org/10.1080/0972060X.2015.1126534 doi: 10.1080/0972060X.2015.1126534
![]() |
[70] |
Khumpirapang N, Pikulkaew S, Anuchapreeda S, et al. (2018) Alpinia galanga oil—A new natural source of fish anaesthetic. Aquac Res 49: 1546–1556. https://doi.org/10.1111/are.13609 doi: 10.1111/are.13609
![]() |
[71] |
Singh S, Sahoo S, Sahoo BC, et al. (2021) Enhancement of bioactivities of rhizome essential oil of Alpinia galanga (Greater galangal) Through Nanoemulsification. J Essent Oil-Bear Plants 24: 648–657. https://doi.org/10.1080/0972060X.2021.1951847 doi: 10.1080/0972060X.2021.1951847
![]() |
[72] |
Lin LY, Shen KH, Yeh XY, et al. (2016) Integrated process for production of galangal acetate, the 'Wasabi-Like' spicy compound, and analysis of essential oils of rhizoma Alpinia officinarum (Hance) Farw. J Food Sci 81: H1565–H1575. https://doi.org/10.1111/1750-3841.13326 doi: 10.1111/1750-3841.13326
![]() |
[73] | Wei Y, Sui D, Xu H, et al. (2017) Atractylodes lancea rhizome water extract reduces triptolide-induced toxicity and enhances anti-inflammatory effects. Chin J Nat Med 15: 905–911. |
[74] |
Nguyen DD, Saensouk S, Nguyen van C, et al. (2023) Taxonomic notes of Alpinia subsect. Catimbium (Zingiberaceae) in Vietnam: The first record of Alpinia nobilis and description of a new species Alpinia hoangviet. Biodiversitas 24: 5293–5301. https://doi.org/10.13057/biodiv/d241010 doi: 10.13057/biodiv/d241010
![]() |
[75] |
Yuan Y, Lin LJ, Huang XB, et al. (2017) Analysis of the essential oils of Alpiniae officinarum Hance in different extraction methods. IOP Conf Ser: Mater Sci Eng 231: 012112. https://doi.org/10.1088/1757-899X/231/1/012112 doi: 10.1088/1757-899X/231/1/012112
![]() |
[76] |
Sukardi S, Pulungan MH, Asmara SNL (2022) Pulsed electric field energy calculation to damage red galangal (Alpinia purpurata K. Scumm) rhizome slices and its essential oil yield and quality with hydrodistillation. Ces a Slov Farm Cas Ces Farm Spol a Slov Farm Spol 71: 103–105. https://doi.org/10.5817/CSF2022-3-103 doi: 10.5817/CSF2022-3-103
![]() |
[77] |
Ge X, Liang Q, Long Y, et al. (2022) Assessment of fresh Alpinia galanga (A. galanga) drying techniques for the chemical composition of essential oil and its antioxidant and biological activity. Food Chem 392: 133314. https://doi.org/10.1016/j.foodchem.2022.133314 doi: 10.1016/j.foodchem.2022.133314
![]() |
[78] |
Kawai H, Kuraya E, Touyama A, et al. (2021) Improved yield and antioxidant activity of essential oil from Alpinia zerumbet (Zingiberaceae) leaves by underwater shockwave pretreatment. Food Bioprod Process 125: 134–140. https://doi.org/10.1016/j.fbp.2020.11.003 doi: 10.1016/j.fbp.2020.11.003
![]() |
[79] | Sethunga M, Ranaweera K, Munaweera I, et al. (2020) Recent advances in the extraction methods of essential oils and oleoresins from plant materials and its potential applications: A comprehensive review. J Food Bioprocess Eng 5: 151–167. |
[80] |
Nguyễn TAT, Huỳnh TĐ, Natthakan R (2019) Extraction and encapsulation of lesser galangal (Alpinia officinarum) essential oil using microwave pretreatment and spray drying. Tạp chí Nô ng nghiệp và Phát triển 18: 57–63. https://doi.org/10.52997/jad.9.03.2019 doi: 10.52997/jad.9.03.2019
![]() |
[81] | Ghasemy-Piranloo F, Kavousi F, Dadashian S (2020) Comparison for the production of essential oil by conventional, novel and biotechnology methods. https://doi.org/10.22541/au.160315281.12110663/v1 |
[82] | Rios JL (2016) Chapter 1—Essential oils: What they are and how the terms are used and defined. In: Preedy VR (Ed.), Essential Oils in Food Preservation, Flavor and Safety, 3–10. https://doi.org/10.1016/B978-0-12-416641-7.00001-8 |
[83] |
Sharmeen JB, Mahomoodally FM, Zengin G, et al. (2021) Essential oils as natural sources of fragrance compounds for cosmetics and cosmeceuticals. Molecules 26: 666. https://doi.org/10.3390/molecules26030666 doi: 10.3390/molecules26030666
![]() |
[84] |
Van HT, Le NT, Huynh NTA, et al. (2022) Chemical composition and antibacterial activities of essential oils from rhizomes and aerial parts of Homalomena cochinchinensis (Araceae). Nat Prod Res 36: 3129–32. https://doi.org/10.1080/14786419.2021.1939333 doi: 10.1080/14786419.2021.1939333
![]() |
[85] |
Liang W, Ge X, Lin Q, et al. (2024) Ternary composite degradable plastics based on Alpinia galanga essential oil Pickering emulsion templates: A potential multifunctional active packaging. Int J Biol Macromol 257: 128580. https://doi.org/10.1016/j.ijbiomac.2023.128580 doi: 10.1016/j.ijbiomac.2023.128580
![]() |
[86] |
Hoch CC, Petry J, Griesbaum L, et al. (2023) 1,8-cineole (eucalyptol): A versatile phytochemical with therapeutic applications across multiple diseases. Biomed Pharmacother 167: 115467. https://doi.org/10.1016/j.biopha.2023.115467 doi: 10.1016/j.biopha.2023.115467
![]() |
[87] | Jirovetz L, Buchbauer G, Shafi MP, et al. (2003) Analysis of the essential oils of the leaves, stems, rhizomes and roots of the medicinal plant Alpinia galanga from southern India. Acta Pharm 53: 73–82. |
[88] |
Raj G, Pradeep DP, Yusufali C, et al. (2013) Chemical profiles of volatiles in four Alpinia species from Kerala, South India. J Essent Oil Res 25: 97–102. https://doi.org/10.1080/10412905.2012.751058 doi: 10.1080/10412905.2012.751058
![]() |
[89] |
Akhtar P, Ali M, Mir SR, et al. (2004) Volatile constituents of rhizomes of Alpinia galanga (Linn.) Willd. J Essent Oil Bear Plants 7: 243–246. https://doi.org/10.1080/0972-060X.2004.10643400 doi: 10.1080/0972-060X.2004.10643400
![]() |
[90] |
Charles DJ, Simon JE, Singh NK (1992) The essential oil of Alpinia galanga Willd. J Essent Oil Res 4: 81–82. https://doi.org/10.1080/10412905.1992.9698016 doi: 10.1080/10412905.1992.9698016
![]() |
[91] | Sripor W, Jinda N (2014) Effect of Alpinia galangal essential oil on bacteria spoilage. The 26 th Meeting of the Thai Society for Biotechnology a International Conference, Thailand, 1–6. |
[92] |
Pripdeevech P, Nuntawong N, Wongpornchai S (2009) Composition of essential oils from the rhizomes of three Alpinia species grown in Thailand. Chem Nat Compd 45: 562–564. https://doi.org/10.1007/s10600-009-9367-1 doi: 10.1007/s10600-009-9367-1
![]() |
[93] | Hamad A, Alifah A, Permadi A, et al. (2016) Chemical constituents and antibacterial activities of crude extract and essential oils of Alpinia galanga and Zingiber officinale. Int Food Res J 23: 837. |
[94] | Wu Y, Wang Y, Li ZH, et al. (2014) Composition of the essential oil from Alpinia galanga rhizomes and its bioactivity on Lasioderma serricorne. Bull Insectol 67: 247–254. |
[95] |
Arambewela LSR, Arawwawala M, Owen NL, et al. (2007) Volatile oil of Alpinia galanga willd. of Sri Lanka. J Essent Oil Res 19: 455–456. https://doi.org/10.1080/10412905.2007.9699950 doi: 10.1080/10412905.2007.9699950
![]() |
[96] |
Rialita T, Radiani H, Alfiah D (2019) Antimicrobial activity of the combination of red galangal (Alpinia purpurata K. Schum) and cinnamon (Cinnamomum burmanii) essential oils on Escherichia coli and Staphylococcus aureus bacteria. J Phys Conf Ser 1217: 012132. https://doi.org/10.1088/1742-6596/1217/1/012132 doi: 10.1088/1742-6596/1217/1/012132
![]() |
[97] |
Sukardi S, Pulungan MH, Asmara SNL (2022) Pulsed electric field energy calculation to damage red galangal (Alpinia purpurata, K. Scumm) rhizome slices and its essential oil yield and quality with hydrodistillation. Ces a Slov Farm Cas Ces Farm Spol a Slov Farm Spol 71: 103–115. https://doi.org/10.5817/CSF2022-3-103 doi: 10.5817/CSF2022-3-103
![]() |
[98] |
Rana VS, Verdeguer M, Blazquez MA (2010) GC and GC/MS analysis of the volatile constituents of the oils of Alpinia galanga (L.) Willd and A. officinarum Hance rhizomes. J Essent Oil Res 22: 521–524. https://doi.org/10.1080/10412905.2010.9700388 doi: 10.1080/10412905.2010.9700388
![]() |
[99] |
Wu J, Lei LW, Mei WL, et al. (2012) High-level 1,8-cineole in the Alpinia officinarum essential oil from Hainan Island of China. Chem Nat Compd 48: 325–326. https://doi.org/10.1007/s10600-012-0238-9 doi: 10.1007/s10600-012-0238-9
![]() |
[100] |
Tram Ngoc L, Yamauchi R, Kato K (2001) Volatile components of the essential oils in galanga (Alpinia officinarum Hance) from Vietnam. Food Sci Technol Res 7: 303–306. https://doi.org/10.3136/fstr.7.303 doi: 10.3136/fstr.7.303
![]() |
[101] |
Chandrakanthan M, Handunnetti SM, Premakumara GSA, et al. (2020) Topical anti-Inflammatory activity of essential oils of Alpinia calcarata Rosc., its main constituents, and possible mechanism of action. Evidence-Based Complementary Altern Med 2020: 2035671. https://doi.org/10.1155/2020/2035671 doi: 10.1155/2020/2035671
![]() |
[102] |
Nampoothiri SV, Venugopalan VV, Joy B, et al. (2012) Comparison of essential oil composition of three ginger cultivars from sub Himalayan region. Asian Pac J Trop Biomed 2: S1347–S1350. https://doi.org/10.1016/S2221-1691(12)60414-6 doi: 10.1016/S2221-1691(12)60414-6
![]() |
[103] | Poonkodi K, Baranika SK V, Udayakumar P, et al. (2017) Chemical composition and Anti-inflammatory potential of essential oil of Alpinia calcarata Rosc.-grown in South India. Int J Pharm Sci Rev Res 42: 207. |
[104] |
Rout PK, Sahoo S, Rath SP, et al. (2005) Analysis of the leaf, rhizome and root oils of two accessions of Alpinia calcarata rosc. cultivated at Bhubaneswar. J Essent Oil Res 17: 398–400. https://doi.org/10.1080/10412905.2005.9698941 doi: 10.1080/10412905.2005.9698941
![]() |
[105] |
Feng YX, Zhang X, Wang Y, et al. (2021) The potential contribution of cymene isomers to insecticidal and repellent activities of the essential oil from Alpinia zerumbet. Int Biodeterior Biodegrad 157: 105138. https://doi.org/10.1016/j.ibiod.2020.105138 doi: 10.1016/j.ibiod.2020.105138
![]() |
[106] |
Saikia J, Sarkar A, Washmin N, et al. (2023) Effect of postharvest drying on physicochemical properties, volatile yield, composition, and sensory attributes of Alpinia zerumbet (shell ginger) rhizome. Ind Crops Prod 198: 116719. https://doi.org/10.1016/j.indcrop.2023.116719 doi: 10.1016/j.indcrop.2023.116719
![]() |
[107] |
Wu B, Gan A, Wang R, et al. (2023) Alpinia oxyphylla Miq. volatile oil ameliorates depressive behaviors and inhibits neuroinflammation in CUMS-exposed mice by inhibiting the TLR4-medicated MyD88/NF-κB signaling pathway. J Chem Neuroanat 130: 102270. https://doi.org/10.1016/j.jchemneu.2023.102270 doi: 10.1016/j.jchemneu.2023.102270
![]() |
[108] |
Trung HT, Giang LD, Thuan VT, et al. (2023) Chemical Composition of Essential Oils Extracted from the Leaves and Rhizomes of Alpinia hongiaoensis Tagane.(Zingiberaceae) growing Wild in Vietnam. J Essent Oil Bear Plants 26: 396–402. https://doi.org/10.1080/0972060X.2023.2187708 doi: 10.1080/0972060X.2023.2187708
![]() |
[109] | Wu Y, Zhang WJ, Huang DY, et al. (2015) Chemical compositions and insecticidal activities of Alpinia kwangsiensis essential oil against Lasioderma serricorne. Molecules 20: 21939–21945. |
[110] |
Yodha AWM, Badia E, Musdalipah, et al. (2023) Essential oils of Alpinia monopleura and their antibacterial and antioxidant activity. Molekul 18: 80–88. https://doi.org/10.20884/1.jm.2023.18.1.6265 doi: 10.20884/1.jm.2023.18.1.6265
![]() |
[111] |
Tra NT, Ha NX, Tuyen NV, et al. (2023) Essential oils of Alpinia vietnamica rhizomes and leaves: Chemical composition, cytotoxicity, α-glucosidase inhibition, and molecular docking approach. Nat Prod Commun 18: 10. https://doi.org/10.1177/1934578X231206280 doi: 10.1177/1934578X231206280
![]() |
[112] |
Jusoh S, Sirat HM, Ahmad F (2013) Essential oils of Alpinia rafflesiana and their antimicrobial activities. Natural Product Communications, 8: 9. https://doi.org/10.1177/1934578X1300800933 doi: 10.1177/1934578X1300800933
![]() |
[113] | Raina AP, Abraham Z (2017) Essential oil profiling of Alpinia species from southern India. Indian J Exp Biol 55: 776–781. |
[114] |
Zhang D, Zou L, Wu DT, et al. (2021) Discovery of 1'-acetoxychavicol acetate (ACA) as a promising antibacterial compound from galangal (Alpinia galanga (Linn.) Willd). Ind Crops Prod 171: 113883. https://doi.org/10.1016/j.indcrop.2021.113883 doi: 10.1016/j.indcrop.2021.113883
![]() |
[115] |
Mallavarapu GR, Rao L, Ramesh S, et al. (2002) Composition of the volatile oils of Alpinia galanga rhizomes and leaves from India. J Essent Oil Res 14: 397–399. https://doi.org/10.1080/10412905.2002.9699900 doi: 10.1080/10412905.2002.9699900
![]() |
[116] |
Tang G, Chen Y, Jiang Y, et al. (2018) Green human resource management practices: scale development and validity. Asia Pac J Hum Resour 56: 31–55. https://doi.org/10.1111/1744-7941.12147 doi: 10.1111/1744-7941.12147
![]() |
[117] |
Tian Y, Jia X, Wang Q, et al. (2022) Antioxidant, antibacterial, enzyme inhibitory, and anticancer activities and chemical composition of Alpinia galanga flower essential oil. Pharmaceuticals 15: 1069. https://doi.org/10.3390/ph15091069 doi: 10.3390/ph15091069
![]() |
[118] |
Feng YX (2020) Contact toxicity and repellent efficacy of Valerianaceae spp. to three stored-product insects and synergistic interactions between two major compounds camphene and bornyl acetate. Ecotoxicol Environ Saf 190: 110106. https://doi.org/10.1016/j.ecoenv.2019.110106 doi: 10.1016/j.ecoenv.2019.110106
![]() |
[119] |
Eumkeb G, Sakdarat S, Siriwong S (2010) Reversing β-lactam antibiotic resistance of Staphylococcus aureus with galangin from Alpinia officinarum Hance and synergism with ceftazidime. Phytomedicine 18: 40–45. https://doi.org/10.1016/j.phymed.2010.09.003 doi: 10.1016/j.phymed.2010.09.003
![]() |
[120] |
Gazal M, Valente MR, Acosta BA, et al. (2014) Neuroprotective and antioxidant effects of curcumin in a ketamine-induced model of mania in rats. Eur J Pharmacol 724: 132–139. https://doi.org/10.1016/j.ejphar.2013.12.028 doi: 10.1016/j.ejphar.2013.12.028
![]() |
[121] |
Reid K, Wright V, Omoregie S (2016) Anticancer properties of Alpinia officinarum (lesser galangal)—A mini review. Int J Adv Res 4: 300–306. https://doi.org/10.21474/IJAR01/380 doi: 10.21474/IJAR01/380
![]() |
[122] |
Lima PR, De Melo TS, Carvalho KMMB, et al. (2013) 1,8-cineole (eucalyptol) ameliorates cerulein-induced acute pancreatitis via modulation of cytokines, oxidative stress and NF-κB activity in mice. Life Sci 92: 1195–1201. https://doi.org/10.1016/j.lfs.2013.05.009 doi: 10.1016/j.lfs.2013.05.009
![]() |
[123] |
Dhakad AK, Pandey V V, Beg S, et al. (2018) Biological, medicinal and toxicological significance of Eucalyptus leaf essential oil: A review. J Sci Food Agric 98: 833–848. https://doi.org/10.1002/jsfa.8600 doi: 10.1002/jsfa.8600
![]() |
[124] |
Cai Z-M, Peng J-Q, Chen Y, et al. (2021) 1, 8-Cineole: A review of source, biological activities, and application. J Asian Nat Prod Res 23: 938–954. https://doi.org/10.1080/10286020.2020.1839432 doi: 10.1080/10286020.2020.1839432
![]() |
[125] |
Raina AP, Abraham Z (2016) Chemical profiling of essential oil of Kaempferia galanga L. germplasm from India. J Essent oil Res 28: 1077165. https://doi.org/10.1080/10412905.2015.1077165 doi: 10.1080/10412905.2015.1077165
![]() |
[126] |
Santos MS, Jezler CN, Oliveira A de, et al. (2012) Harvest time and plant age on the content and chemical composition of the essential oil of Alpinia zerumbet. Horticultura Brasileira, 30: 385–390. https://doi.org/10.1590/S0102-05362012000300005 doi: 10.1590/S0102-05362012000300005
![]() |
[127] |
Chan EWC, Wong SK (2015) Phytochemistry and pharmacology of ornamental gingers, Hedychium coronarium and Alpinia purpurata: A review. J Integr Med 13: 368–379. https://doi.org/10.1016/S2095-4964(15)60208-4 doi: 10.1016/S2095-4964(15)60208-4
![]() |
[128] |
Kanjilal PB, Kotoky R, Couladis M (2010) Essential oil composition of leaf and rhizome oil of Alpinia nigra (Gaertner) BL Burtt. from Northeast India. J Essent Oil Res 22: 358–359. https://doi.org/10.1080/10412905.2010.9700345 doi: 10.1080/10412905.2010.9700345
![]() |
[129] |
Aljobair MO (2022) Chemical composition, antimicrobial properties, and antioxidant activity of galangal rhizome. Food Sci Technol 42: e45622. https://doi.org/10.1590/fst.45622 doi: 10.1590/fst.45622
![]() |
[130] |
Eram S, Mujahid MD, Bagga P, et al. (2019) A review on phytopharmacological activity of Alpinia galanga. Int J Pharm Pharm Sci 11: 6–11. https://doi.org/10.22159/ijpps.2019v11i3.31352 doi: 10.22159/ijpps.2019v11i3.31352
![]() |
[131] | Mahae N, Chaiseri S (2009) Antioxidant activities and antioxidative components in extracts of Alpinia galanga (L.) Sw. Agric Nat Resour 43: 358–369. |
[132] |
Mandal D, Sarkar T, Chakraborty R (2022) Critical review on nutritional, bioactive, and medicinal potential of spices and herbs and their application in food fortification and nanotechnology. Appl Biochem Biotechnol 195: 1319–1513. https://doi.org/10.1007/s12010-022-04132-y doi: 10.1007/s12010-022-04132-y
![]() |
[133] |
Ramanunny AK, Wadhwa S, Gulati M, et al. (2022) Journey of Alpinia galanga from kitchen spice to nutraceutical to folk medicine to nanomedicine. J Ethnopharmacol 291: 115144. https://doi.org/10.1016/j.jep.2022.115144 doi: 10.1016/j.jep.2022.115144
![]() |
[134] |
Sahoo S, Singh S, Sahoo A, et al. (2020) Molecular and phytochemical stability of long term micropropagated greater galanga (Alpinia galanga) revealed suitable for industrial applications. Ind Crops Prod 148: 112274. https://doi.org/10.1016/j.indcrop.2020.112274 doi: 10.1016/j.indcrop.2020.112274
![]() |
[135] |
Zhou C, Li C, Siva S, et al. (2021) Chemical composition, antibacterial activity and study of the interaction mechanisms of the main compounds present in the Alpinia galanga rhizomes essential oil. Ind Crops Prod 165: 113441. https://doi.org/10.1016/j.indcrop.2021.113441 doi: 10.1016/j.indcrop.2021.113441
![]() |
[136] |
Budiati T, Suryaningsih W, Umaroh S, et al. (2018) Antimicrobial activity of essential oil from Indonesian medicinal plants against food-borne pathogens. IOP Conf Ser Earth Environ Sci 207: 012036. https://doi.org/10.1088/1755-1315/207/1/012036 doi: 10.1088/1755-1315/207/1/012036
![]() |
[137] |
Budiati T, Wibisono Y, Pambayun RA, et al. (2020) Inhibition of Listeria monocytogenes by natural antimicrobial. IOP Conf Ser Earth Environ Sci 411: 012042. https://doi.org/10.1088/1755-1315/411/1/012042 doi: 10.1088/1755-1315/411/1/012042
![]() |
[138] | Eff ARY, Rahayu ST (2016) The antibacterial effects of essential oil from galangal rhizome Alpinia galanga (Linn.) pierreon rat (Rattus norvegicus L.) were infected by Salmonella typhi. Asian J Pharm Clin Res 9: 189–193. |
[139] |
Xiao T, Huang J, Wang X, Wu L, Zhou X, Jiang F, Shen X, et al. (2020) Alpinia zerumbet and its potential use as an herbal medication for atherosclerosis: Mechanistic insights from cell and rodent studies. Lifestyle Genomics 13: 138–145. https://doi.org/10.1159/000508818 doi: 10.1159/000508818
![]() |
[140] |
Cunha GH da, Moraes MO de, Fechine FV, et al. (2013) Vasorelaxant and antihypertensive effects of methanolic fraction of the essential oil of Alpinia zerumbet. Vascul Pharmacol 58: 337–345. https://doi.org/10.1016/j.vph.2013.04.001 doi: 10.1016/j.vph.2013.04.001
![]() |
[141] |
Cruz JD d., Mpalantinos MA, Ramos A de S, et al. (2020) Chemical standardization, antioxidant activity and phenolic contents of cultivated Alpinia zerumbet preparations. Ind Crops Prod 151: 112495. https://doi.org/10.1016/j.indcrop.2020.112495 doi: 10.1016/j.indcrop.2020.112495
![]() |
[142] |
Pinto N V, Assreuy AMS, Coelho-de-Souza AN, et al. (2009) Endothelium-dependent vasorelaxant effects of the essential oil from aerial parts of Alpinia zerumbet and its main constituent 1,8-cineole in rats. Phytomedicine 16: 1151–1155. https://doi.org/10.1016/j.phymed.2009.04.007 doi: 10.1016/j.phymed.2009.04.007
![]() |
[143] |
Xiao T, Wu A, Wang X, et al. (2024) Anti-hypertensive and composition as well as pharmacokinetics and tissues distribution of active ingredients from Alpinia zerumbet. Fitoterapia 172: 105753. https://doi.org/10.1016/j.fitote.2023.105753 doi: 10.1016/j.fitote.2023.105753
![]() |
[144] |
Ji Y, Shi T, Zhang Y, et al. (2019) Essential oil from Fructus Alpinia zerumbet (fruit of Alpinia zerumbet (Pers.) Burtt. et Smith) protected against aortic endothelial cell injury and inflammation in vitro and in vivo. J Ethnopharmacol 237: 149–158. https://doi.org/10.1016/j.jep.2019.03.011 doi: 10.1016/j.jep.2019.03.011
![]() |
[145] |
Deng J, He B, He D, et al. (2016) A potential biopreservative: Chemical composition, antibacterial and hemolytic activities of leaves essential oil from Alpinia guinanensis. Ind Crops Prod 94: 281–287. https://doi.org/10.1016/j.indcrop.2016.09.004 doi: 10.1016/j.indcrop.2016.09.004
![]() |
[146] |
Van HT, Dam SM, Phan UTX, et al. (2022) Chemical diversity of essential oils of rhizomes of six species of Zingiberaceae family. J Arid Agric 8: 8–13. https://doi.org/10.25081/jaa.2022.v8.7430 doi: 10.25081/jaa.2022.v8.7430
![]() |
[147] |
Zhou W, He Y, Liu F, et al. (2021) Carboxymethyl chitosan-pullulan edible films enriched with galangal essential oil: Characterization and application in mango preservation. Carbohydr Polym 256: 117579. https://doi.org/10.1016/j.carbpol.2020.117579 doi: 10.1016/j.carbpol.2020.117579
![]() |
[148] |
Kubra IR, Rao LJM (2012) Effect of microwave drying on the phytochemical composition of volatiles of ginger. Int J Food Sci Technol 47: 53–60. https://doi.org/10.1111/j.1365-2621.2011.02806.x doi: 10.1111/j.1365-2621.2011.02806.x
![]() |
[149] |
Rao K, Ch B, Narasu LM, et al. (2010) Antibacterial activity of Alpinia galanga (L) Willd crude extracts. Appl Biochem Biotechnol 162: 871–884. https://doi.org/10.1007/s12010-009-8900-9 doi: 10.1007/s12010-009-8900-9
![]() |
[150] |
Veerasophon J, Sripalakit P, Saraphanchotiwitthaya A (2020) Formulation of anti-acne concealer containing cinnamon oil with antimicrobial activity against Propionibacterium acnes. J Adv Pharm Technol Res 11: 53–58. https://doi.org/10.4103/japtr.JAPTR_1_20 doi: 10.4103/japtr.JAPTR_1_20
![]() |
[151] |
Santos GKN, Dutra KA, Barros RA, et al. (2012) Essential oils from Alpinia purpurata (Zingiberaceae): Chemical composition, oviposition deterrence, larvicidal and antibacterial activity. Ind Crops Prod 40: 254–260. https://doi.org/10.1016/j.indcrop.2012.03.020 doi: 10.1016/j.indcrop.2012.03.020
![]() |
[152] | Ravichandra V, Hanumantharayappa B, Papasani V (2014) Evaluation of cardio protective activity of galangin against doxorubicin induced cardiomyopathy. Int J Pharm Pharm Sci 6: 86–90. |
[153] |
Lahlou S, Interaminense LFL, Leal‐Cardoso JH, et al. (2003) Antihypertensive effects of the essential oil of Alpinia zerumbet and its main constituent, terpinen‐4‐ol, in DOCA‐salt hypertensive conscious rats. Fundam Clin Pharmacol 17: 323–330. https://doi.org/10.1046/j.1472-8206.2003.00150.x doi: 10.1046/j.1472-8206.2003.00150.x
![]() |
[154] |
Lahlou S, Galindo CAB, Leal-Cardoso JH, et al. (2002) Cardiovascular effects of the essential oil of Alpinia zerumbet leaves and its main constituent, terpinen-4-ol, in rats: role of the autonomic nervous system. Planta Med 68: 1097–1102. https://doi.org/10.1055/s-2002-36336 doi: 10.1055/s-2002-36336
![]() |
[155] |
Rocha DG, Holanda TM, Braz HLB, et al. (2023) Vasorelaxant effect of Alpinia zerumbet's essential oil on rat resistance artery involves blocking of calcium mobilization. Fitoterapia 169: 105623. https://doi.org/10.1016/j.fitote.2023.105623 doi: 10.1016/j.fitote.2023.105623
![]() |
[156] |
Pereira PS, Maia AJ, Duarte AE, et al. (2018) Cytotoxic and anti-kinetoplastid potential of the essential oil of Alpinia speciosa K. Schum. Food Chem Toxicol 119: 387–391. https://doi.org/10.1016/j.fct.2018.01.024 doi: 10.1016/j.fct.2018.01.024
![]() |
[157] |
Yustica FK, Widiastuti NI, Sapitri N, et al. (2019) Essential oils from Alpinia purpurata (Zingiberaceae): Chemical composition and Formulation of antiacne cream. Indones J Chem Res 4: 14–21. https://doi.org/10.20885/ijcr.vol4.iss1.art3 doi: 10.20885/ijcr.vol4.iss1.art3
![]() |
[158] |
Khumpirapang N, von Gersdorff Jørgensen L, Müllertz A, et al. (2021) Formulation optimization, anesthetic activity, skin permeation, and transportation pathway of Alpinia galanga oil SNEDDS in zebrafish (Danio rerio). Eur J Pharm Biopharm 165: 193–202. https://doi.org/10.1016/j.ejpb.2021.04.022 doi: 10.1016/j.ejpb.2021.04.022
![]() |
[159] |
Wongphan P, Nampanya P, Chakpha W, et al. (2023) Lesser galangal (Alpinia officinarum Hance) essential oil incorporated biodegradable PLA/PBS films as shelf-life extension packaging of cooked rice. Food Packag Shelf Life 37: 101077. https://doi.org/10.1016/j.fpsl.2023.101077 doi: 10.1016/j.fpsl.2023.101077
![]() |
[160] |
Xu J, Jiang Z, Peng J, et al. (2023) Fabrication of a protein-dextran conjugates formed oral nanoemulsion and its application to deliver the essential oil from Alpinia zerumbet Fructus. Int J Biol Macromol 249: 125918. https://doi.org/10.1016/j.ijbiomac.2023.125918 doi: 10.1016/j.ijbiomac.2023.125918
![]() |
[161] |
Liang W, Ge X, Lin Q, et al. (2024) pH-responsive liposomes for controlled release of Alpinia galanga essential oil: Investigating characteristics, stability, control release behavior, and functionality. Ind Crops Prod 209: 117978. https://doi.org/10.1016/j.indcrop.2023.117978 doi: 10.1016/j.indcrop.2023.117978
![]() |
[162] | Damayanti R, Batubara I, Suparto IH (2015) Essential oil of red galangal (Alpinia galanga (L) Willd) rhizomes as slimming aromatherapy. Int J Pharma Bio Sci 6: 283–289. |
[163] |
Nayaka NMDMW, Swari NLP, Yuda PESK, et al. (2022) Essential oil of Alpinia galanga: Effect of aromatherapy inhalation in mice and physicochemical characterization. J Ilm Medicam 8: 87–92. https://doi.org/10.36733/medicamento.v8i2.3791 doi: 10.36733/medicamento.v8i2.3791
![]() |
[164] |
Daning DRA, Yusiati LM, Hanim C, et al. (2022) Dietary supplementation of galangal (Alpinia galangal) essential oil affects rumen fermentation pattern. Adv Anim Vet Sci 10: 323–334. https://doi.org/10.17582/journal.aavs/2022/10.2.323.334 doi: 10.17582/journal.aavs/2022/10.2.323.334
![]() |
[165] |
Daning DRA, Widyobroto BP, Yusiati LM, et al. (2022) Effect of galangal (Alpinia galanga) essential oil supplementation on milk production, composition, and characteristics of fatty acids in dairy cows. Adv Anim Vet Sci 10: 192–202. https://doi.org/10.17582/journal.aavs/2022/10.1.192.202 doi: 10.17582/journal.aavs/2022/10.1.192.202
![]() |
[166] |
Khumpirapang N, Chaichit S, Jiranusornkul S, et al. (2018) In vivo anesthetic effect and mechanism of action of active compounds from Alpinia galanga oil on Cyprinus carpio (koi carp). Aquaculture 496: 176–184. https://doi.org/10.1016/j.aquaculture.2018.07.017 doi: 10.1016/j.aquaculture.2018.07.017
![]() |
[167] |
Zhou W, He Y, Liu F, et al. (2021) Carboxymethyl chitosan-pullulan edible films enriched with galangal essential oil: Characterization and application in mango preservation. Carbohydr Polym 256: 117579. https://doi.org/10.1016/j.carbpol.2020.117579 doi: 10.1016/j.carbpol.2020.117579
![]() |
[168] |
Dong J, Zhu X min, Wu F ye, et al. (2020) Development of galangal essential oil-based microemulsion gel for transdermal delivery of flurbiprofen: simultaneous permeability evaluation of flurbiprofen and 1,8-cineole. Drug Dev Ind Pharm 46: 91–100. https://doi.org/10.1080/03639045.2019.1706548 doi: 10.1080/03639045.2019.1706548
![]() |
[169] |
Wongphan P, Nampanya P, Chakpha W, et al. (2023) Lesser galangal (Alpinia officinarum Hance) essential oil incorporated biodegradable PLA/PBS films as shelf-life extension packaging of cooked rice. Food Packag Shelf Life 37: 101077. https://doi.org/10.1016/j.fpsl.2023.101077 doi: 10.1016/j.fpsl.2023.101077
![]() |
[170] |
de Lira CS, Pontual EV, de Albuquerque LP, et al. (2015) Evaluation of the toxicity of essential oil from Alpinia purpurata inflorescences to Sitophilus zeamais (maize weevil). Crop Prot 71: 95–100. https://doi.org/10.1016/j.cropro.2015.02.004 doi: 10.1016/j.cropro.2015.02.004
![]() |
[171] |
Api AM, Bartlett A, Belsito D, et al. (2024) Update to RIFM fragrance ingredient safety assessment, eucalyptol, CAS Registry Number 470-82-6. Food Chem Toxicol 183: 114439. https://doi.org/10.1016/j.fct.2024.114439 doi: 10.1016/j.fct.2024.114439
![]() |
[172] |
Rocha DG, Holanda TM, Braz HLB, et al. (2023) Vasorelaxant effect of Alpinia zerumbet's essential oil on rat resistance artery involves blocking of calcium mobilization. Fitoterapia 169: 105623. https://doi.org/10.1016/j.fitote.2023.105623 doi: 10.1016/j.fitote.2023.105623
![]() |
[173] |
Zhou W, Sun Y, Zou L, et al. (2021) Effect of galangal essential oil emulsion on quality attributes of cloudy pineapple juice. Front Nutr 8: 1–11. https://doi.org/10.3389/fnut.2021.751405 doi: 10.3389/fnut.2021.751405
![]() |
[174] |
Podshivalov AV, Bronnikov S, Zuev VV, et al. (2013) Synthesis and characterization of polyurethane-urea microcapsules containing galangal essential oil: statistical analysis of encapsulation. J Microencapsul 30: 198–203. https://doi.org/10.3109/02652048.2012.735261 doi: 10.3109/02652048.2012.735261
![]() |
[175] |
Okonogi S, Chaiyana W (2012) Enhancement of anti-cholinesterase activity of Zingiber cassumunar essential oil using a microemulsion technique. Drug Discov Ther 6: 249–255. https://doi.org/10.5582/ddt.2012.v6.5.249 doi: 10.5582/ddt.2012.v6.5.249
![]() |
[176] |
Xu J, Jiang Z, Peng J, et al. (2023) Fabrication of a protein-dextran conjugates formed oral nanoemulsion and its application to deliver the essential oil from Alpinia zerumbet Fructus. Int J Biol Macromol 249: 125918. https://doi.org/10.1016/j.ijbiomac.2023.125918 doi: 10.1016/j.ijbiomac.2023.125918
![]() |
1. | Xiaoding Meng, Hecheng Li, An adaptive co-evolutionary competitive particle swarm optimizer for constrained multi-objective optimization problems, 2024, 91, 22106502, 101746, 10.1016/j.swevo.2024.101746 | |
2. | Özlem TÜLEK, İhsan Hakan SELVİ, Derin Q Ağları Tabanlı Parçacık Sürü Optimizasyonu, 2023, 35, 1308-9072, 855, 10.35234/fumbd.1313906 | |
3. | Kangjian Sun, Ju Huo, Heming Jia, Lin Yue, Reinforcement learning guided Spearman dynamic opposite Gradient-based optimizer for numerical optimization and anchor clustering, 2023, 11, 2288-5048, 12, 10.1093/jcde/qwad109 | |
4. | Xiaoding Meng, Hecheng Li, 2024, A Learnable Competitive Swarm Operator Driven by Reinforcement Learning for Multi-Objective Optimization Problems, 979-8-3503-7784-2, 156, 10.1109/DOCS63458.2024.10704510 | |
5. | Zeyan Hu, Peng Zheng, Jiaxin Cai, Lingling Zhu, Yuqing Cai, Minghui Fang, 2024, Integrated Energy System Load Forecasting Based on GSO-LSTM and Feature Selection, 979-8-3503-5356-3, 288, 10.1109/ICESEP62218.2024.10651922 | |
6. | Qingyang Jia, Kewei Yang, Yajie Dou, Ziyi Chen, Nan Xiang, Lining Xing, A consensus optimization mechanism with Q-learning-based distributed PSO for large-scale group decision-making, 2025, 93, 22106502, 101841, 10.1016/j.swevo.2024.101841 |
Algorithm 1 Q-learning algorithm |
1: Initialize Q(s, a), α, γ, r, ε 2: Repeat : 3: Choose the best action at for the current state st using policy (e.g., ε-greedy strategy); 4: Execute action at; 5: Get the immediate reward rt+1; 6: Get the maximum Q-value for the next state st+1; 7: Update the Q-table by Eq (2.5); 8: Update the current state st←st+1; 9: Until the condition is terminal |
Q-learning | PSO |
Agents | Particles |
Environment | Optimization problems |
State | Fitness value |
Action | Strategy |
State | Action | |||
a1 | a2 | a3 | a4 | |
s1 | Q(s1, a1) | Q(s1, a2) | Q(s1, a3) | Q(s1, a4) |
s2 | Q(s2, a1) | Q(s2, a2) | Q(s2, a3) | Q(s2, a4) |
s3 | Q(s3, a1) | Q(s3, a2) | Q(s3, a3) | Q(s3, a4) |
s4 | Q(s4, a1) | Q(s4, a2) | Q(s4, a3) | Q(s4, a4) |
s5 | Q(s5, a1) | Q(s5, a2) | Q(s5, a3) | Q(s5, a4) |
Algorithm 2 The proposed algorithm |
Input:
Initialize N, D, the maximum number of fitness evaluations (Max_Fes); Initialize the maximum number of iterations (Max_Iter), k=0, kk=0, fit=0; Initialize the inertia weight w, learning factors c1, c2, position, velocity; Evaluate the fitness value, fit=fit+N, record pbest and gbest; Initialize Q-table, state, reward, learning period LP, ε, α, γ. Output: Best solution. 1: while termination condition is not met do 2: k=k+1; 3: /* pop1 */ 4: for i=1:length(pop1) do 5: Update the particle's velocity and position by CLPSO; 6: Evaluate the fitness of the particle, fit=fit+1; 7: Update pbesti and gbest; 8: end for 9: /* pop2 */ 10: Divide state for pop2 according to Section 3.2.1; 11: Select action based on Q-table using ε-greedy strategy according to Eq (3.1); 12: for i=length(pop1)+1:N do 13: Update the particle's velocity by action ai; 14: Update the particle's position; 15: Evaluate the fitness of the particle, fit=fit+1; 16: Update pbesti and gbest; 17: end for 18: /* update Q-table */ 19: if k<Max_Iter then 20: if mod(k, LP) = 0 then 21: Determine next state; 22: Select action using ε-greedy strategy; 23: Calculate reward according to Eq (3.2); 24: Update Q-table using Eq (2.5); 25: end if 26: else 27: if mod(kk, LP) = 0 then 28: Determine next state; 29: Calculate reward according to Eq (3.2); 30: Update Q-table using Eq (2.5); 31: end if 32: kk=kk+1; 33: end if 34: end while |
Function type | No. | Test Functions | F(x∗) | D |
Unimodal Functions | F1 | Shifted and Rotated Bent Cigar Function | 100 | 30/50/100 |
F2 | Shifted and Rotated Sum of Different Power Function* | 200 | 30/50/100 | |
Simple Multimodal Functions | F3 | Shifted and Rotated Zakharov Function | 300 | 30/50/100 |
F4 | Shifted and Rotated Rosenbrock Function | 400 | 30/50/100 | |
F5 | Shifted and Rotated Rastrigin Function | 500 | 30/50/100 | |
F6 | Shifted and Rotated Expanded Schaffer's F6 Function | 600 | 30/50/100 | |
F7 | Shifted and Rotated Lunacek Bi-Rastrigin Function | 700 | 30/50/100 | |
F8 | Shifted and Rotated Non-Continuous Rastrigin Function | 800 | 30/50/100 | |
F9 | Shifted and Rotated Levy Function | 900 | 30/50/100 | |
Hybrid Functions | F10 | Shifted and Rotated Schwefel Function | 1000 | 30/50/100 |
F11 | Hybrid Function 1 (N = 3) | 1100 | 30/50/100 | |
F12 | Hybrid Function 2 (N = 3) | 1200 | 30/50/100 | |
F13 | Hybrid Function 3 (N = 3) | 1300 | 30/50/100 | |
F14 | Hybrid Function 4 (N = 4) | 1400 | 30/50/100 | |
F15 | Hybrid Function 5 (N = 4) | 1500 | 30/50/100 | |
F16 | Hybrid Function 6 (N = 4) | 1600 | 30/50/100 | |
F17 | Hybrid Function 6 (N = 5) | 1700 | 30/50/100 | |
F18 | Hybrid Function 6 (N = 5) | 1800 | 30/50/100 | |
F19 | Hybrid Function 6 (N = 5) | 1900 | 30/50/100 | |
Composition Functions | F20 | Hybrid Function 6 (N = 6) | 2000 | 30/50/100 |
F21 | Composition Function 1 (N = 3) | 2100 | 30/50/100 | |
F22 | Composition Function 2 (N=3) | 2200 | 30/50/100 | |
F23 | Composition Function 3 (N = 4) | 2300 | 30/50/100 | |
F24 | Composition Function 4 (N = 4) | 2400 | 30/50/100 | |
F25 | Composition Function 5 (N = 5) | 2500 | 30/50/100 | |
F26 | Composition Function 6 (N = 5) | 2600 | 30/50/100 | |
F27 | Composition Function 7 (N = 6) | 2700 | 30/50/100 | |
F28 | Composition Function 8 (N = 6) | 2800 | 30/50/100 | |
F29 | Composition Function 9 (N = 3) | 2900 | 30/50/100 | |
F30 | Composition Function 10 (N = 3) | 3000 | 30/50/100 | |
Note: *F2 has been excluded because it shows unstable behavior especially for higher dimensions. |
No. | Functions | F(x∗) | D | Search range |
F31 | Storn's Chebyshev Polynomial Fitting Problem | 1 | 9 | [-8192, 8192] |
F32 | Inverse Hilbert Matrix Problem | 1 | 16 | [-16384, 16384] |
F33 | Lennard-Jones Minimum Energy Cluster | 1 | 18 | [-4, 4] |
F34 | Rastrigin Function | 1 | 10 | [-100,100] |
F35 | Griewank's Function | 1 | 10 | [-100,100] |
F36 | Weierstrass Function | 1 | 10 | [-100,100] |
F37 | Modified Schwefel Function | 1 | 10 | [-100,100] |
F38 | Expanded Schaffer's F6 Function | 1 | 10 | [-100,100] |
F39 | Happy Cat Function | 1 | 10 | [-100,100] |
F40 | Ackley Function | 1 | 10 | [-100,100] |
Algorithm | Key parameters | Year | Ref. |
LDWPSO | w:0.9−0.2, c1:2.5−0.5, c2:0.5−2.5, Vmax = 0.5*range | 1998 | [50] |
CLPSO | w:0.9−0.2, c:3.0−1.5, Vmax = 0.5*range | 2006 | [6] |
UPSO | w:0.9−0.2, c1:2.5−0.5, c2:0.5−2.5 | 2004 | [53] |
LIPS | χ:0.7298, Vmax = 0.5*range, nsize = 3 | 2013 | [52] |
HCLPSO | w:0.99−0.2, c:3.0−1.5, c1:2.5−0.5, c2:0.5−2.5, Vmax = 0.2*range | 2015 | [7] |
EPSO | w:0.9−0.4, c:3.0−1.5, w1:0.9−0.2, c1:2.5−0.5, c2:0.5−2.5, Vmax = 0.5*range | 2017 | [34] |
SDPSO | w:0.9−0.2, c:3.0−1.5, c1:2.5−0.5, c2:0.5−2.5, Vmax = 0.5*range | 2022 | [8] |
MRFO | S = 2 | 2020 | [58] |
I-GWO | a = 2−0 | 2021 | [59] |
BeSD | K = 5 | 2021 | [60] |
MPSORL | w:0.9−0.2, c:3.0−1.5, c1:2.5−0.5, c2:0.5−2.5, Vmax = 0.5*range | − | − |
Level | Factors | ||||
pop1 (N1) | LP | epsilon (ε) | alpha (α) | gamma (γ) | |
1 | 0.1 | 20 | 0.80 | 0.3 | 0.6 |
2 | 0.2 | 50 | 0.85 | 0.4 | 0.7 |
3 | 0.3 | 100 | 0.90 | 0.5 | 0.8 |
4 | 0.4 | 200 | 0.95 | 0.6 | 0.9 |
pop1 (N1) | LP | epsilon (ε) | alpha (α) | gamma (γ) | average rank | |
1 | 0.1 | 20 | 0.8 | 0.3 | 0.6 | 8.5 |
2 | 0.1 | 50 | 0.85 | 0.4 | 0.7 | 9.345 |
3 | 0.1 | 100 | 0.9 | 0.5 | 0.8 | 10.672 |
4 | 0.1 | 200 | 0.95 | 0.6 | 0.9 | 11.483 |
5 | 0.2 | 20 | 0.85 | 0.5 | 0.9 | 7.81 |
6 | 0.2 | 50 | 0.8 | 0.6 | 0.8 | 6.207 |
7 | 0.2 | 100 | 0.95 | 0.3 | 0.7 | 10.293 |
8 | 0.2 | 200 | 0.9 | 0.4 | 0.6 | 9.207 |
9 | 0.3 | 20 | 0.9 | 0.6 | 0.7 | 7.879 |
10 | 0.3 | 50 | 0.95 | 0.5 | 0.6 | 9.828 |
11 | 0.3 | 100 | 0.8 | 0.4 | 0.9 | 6.379 |
12 | 0.3 | 200 | 0.85 | 0.3 | 0.8 | 7.293 |
13 | 0.4 | 20 | 0.95 | 0.4 | 0.8 | 9.362 |
14 | 0.4 | 50 | 0.9 | 0.3 | 0.9 | 7.931 |
15 | 0.4 | 100 | 0.85 | 0.6 | 0.6 | 6.793 |
16 | 0.4 | 200 | 0.8 | 0.5 | 0.7 | 7.017 |
IEEE CEC2017 with 30D | LDWPSO | UPSO | CLPSO | LIPS | MPSORL |
mean±std | mean±std | mean±std | mean±std | mean±std | |
F1 | 4.64E+03±5.02E+03+ | 2.03E+03±2.03E+03+ | 8.17E+00±1.07E+01− | 9.24E+02±1.60E+03+ | 1.05E+02±2.10E+02 |
F3 | 3.07E+02±4.39E+02+ | 1.46E+02±2.08E+02+ | 2.84E+04±6.40E+03+ | 2.49E+03±1.59E+03+ | 2.65E−02±7.68E−02 |
F4 | 9.26E+01±2.63E+01+ | 3.58E+01±3.85E+01≈ | 5.90E+01±2.66E+01+ | 3.59E+01±2.95E+01≈ | 4.13E+01±2.72E+01 |
F5 | 6.95E+01±1.83E+01+ | 6.99E+01±1.13E+01+ | 4.76E+01±8.81E+00+ | 1.05E+02±2.29E+01+ | 3.25E+01±1.00E+01 |
F6 | 4.27E−01±5.54E−01+ | 1.20E+00±9.13E−01+ | 4.92E−07±2.49E−07+ | 2.29E+01±8.13E+00+ | 2.35E−13±6.63E−14 |
F7 | 1.18E+02±2.17E+01+ | 9.67E+01±1.34E+01+ | 9.22E+01±1.01E+01+ | 1.13E+02±1.93E+01+ | 8.33E+01±1.15E+01 |
F8 | 6.93E+01±1.74E+01+ | 6.44E+01±1.13E+01+ | 5.33E+01±7.36E+00+ | 9.36E+01±2.08E+01+ | 3.67E+01±1.33E+01 |
F9 | 1.40E+02±1.22E+02+ | 9.18E+01±6.82E+01+ | 1.18E+02±5.19E+01+ | 1.52E+03±9.83E+02+ | 1.27E+00±1.05E+00 |
F10 | 3.21E+03±6.26E+02+ | 2.90E+03±4.36E+02+ | 2.18E+03±2.71E+02+ | 3.11E+03±4.73E+02+ | 1.86E+03±3.64E+02 |
F11 | 8.61E+01±4.17E+01+ | 5.86E+01±2.68E+01+ | 6.69E+01±2.04E+01+ | 1.04E+02±3.48E+01+ | 4.65E+01±3.30E+01 |
F12 | 3.79E+04±1.95E+04+ | 8.47E+04±8.91E+04+ | 4.02E+05±2.22E+05+ | 1.30E+05±1.33E+05+ | 2.34E+04±1.03E+04 |
F13 | 9.64E+03±1.20E+04+ | 7.86E+03±6.06E+03+ | 3.41E+02±1.26E+02≈ | 4.42E+03±5.13E+03+ | 4.90E+02±5.07E+02 |
F14 | 6.14E+03±4.66E+03+ | 3.66E+03±3.79E+03≈ | 2.89E+04±2.86E+04+ | 8.02E+03±5.99E+03+ | 3.94E+03±5.06E+03 |
F15 | 5.33E+03±5.78E+03+ | 2.47E+03±2.83E+03+ | 1.44E+02±1.03E+02− | 1.97E+03±3.02E+03+ | 2.88E+02±3.70E+02 |
F16 | 7.60E+02±2.01E+02+ | 6.57E+02±1.25E+02+ | 5.16E+02±1.21E+02+ | 7.24E+02±2.56E+02+ | 3.97E+02±1.79E+02 |
F17 | 2.26E+02±1.59E+02+ | 1.69E+02±7.73E+01+ | 1.22E+02±5.72E+01+ | 2.88E+02±1.55E+02+ | 9.27E+01±4.66E+01 |
F18 | 9.36E+04±5.84E+04≈ | 1.04E+05±4.07E+04≈ | 1.37E+05±6.93E+04+ | 8.81E+04±7.43E+04≈ | 8.72E+04±5.27E+04 |
F19 | 7.41E+03±1.02E+04+ | 2.04E+03±2.27E+03+ | 5.83E+01±4.54E+01− | 2.50E+03±3.42E+03+ | 1.55E+02±1.42E+02 |
F20 | 2.78E+02±1.26E+02+ | 2.77E+02±7.74E+01+ | 1.56E+02±7.37E+01≈ | 4.76E+02±1.29E+02+ | 1.34E+02±6.92E+01 |
F21 | 2.70E+02±1.43E+01+ | 2.62E+02±3.02E+01+ | 2.40E+02±4.08E+01+ | 2.95E+02±2.04E+01+ | 2.33E+02±9.38E+00 |
F22 | 3.90E+02±1.10E+03+ | 1.01E+02±1.73E+00+ | 1.13E+02±6.95E+00+ | 3.19E+02±8.32E+02≈ | 1.00E+02±1.08E+00 |
F23 | 4.29E+02±2.15E+01+ | 4.32E+02±2.02E+01+ | 4.00E+02±1.20E+01+ | 5.35E+02±3.83E+01+ | 3.86E+02±1.07E+01 |
F24 | 5.15E+02±3.12E+01+ | 4.87E+02±1.20E+01+ | 4.76E+02±9.15E+01+ | 5.70E+02±4.66E+01+ | 4.53E+02±7.86E+00 |
F25 | 3.96E+02±1.37E+01+ | 3.89E+02±5.82E+00+ | 3.87E+02±1.13E+00≈ | 3.87E+02±4.05E+00≈ | 3.87E+02±1.05E+00 |
F26 | 1.43E+03±7.28E+02+ | 6.40E+02±7.57E+02≈ | 4.02E+02±1.94E+02+ | 9.96E+02±1.18E+03≈ | 3.75E+02±3.19E+02 |
F27 | 5.37E+02±1.22E+01+ | 5.48E+02±1.57E+01+ | 5.10E+02±5.27E+00− | 5.84E+02±2.16E+01+ | 5.15E+02±6.39E+00 |
F28 | 4.13E+02±2.81E+01+ | 3.15E+02±3.50E+01≈ | 4.20E+02±6.12E+00+ | 3.10E+02±2.98E+01≈ | 3.36E+02±5.26E+01 |
F29 | 6.76E+02±1.76E+02+ | 7.95E+02±1.28E+02+ | 5.44E+02±6.75E+01+ | 1.03E+03±1.70E+02+ | 4.94E+02±4.09E+01 |
F30 | 5.83E+03±3.07E+03+ | 8.18E+03±6.88E+03+ | 4.82E+03±8.11E+02+ | 6.87E+03±2.12E+03+ | 4.06E+03±1.14E+03 |
+/≈/− | 28/1/0 | 24/5/0 | 22/3/4 | 23/6/0 | / |
IEEE CEC2017 with 50D | HCLPSO | EPSO | SDPSO | MRFO | I-GWO | BeSD | MPSORL |
mean±std | mean±std | mean±std | mean±std | mean±std | mean±std | mean±std | |
F1 | 1.65E+02±2.03E+02≈ | 2.15E+03±2.12E+03+ | 3.46E+02±3.11E+02+ | 4.94E+03±5.51E+03+ | 5.00E+03±6.84E+03+ | 8.66E+02±1.13E+03+ | 1.35E+02±1.34E+02 |
F3 | 1.55E+01±1.61E+01− | 6.38E−04±1.14E−03− | 5.11E+00±2.31E+01− | 6.98E+03±2.44E+03+ | 1.91E+04±4.05E+03+ | 1.22E+03±7.08E+02+ | 5.22E+02±5.03E+02 |
F4 | 7.85E+01±4.42E+01≈ | 4.05E+01±3.72E+01− | 5.97E+01±4.46E+01≈ | 8.48E+01±5.02E+01≈ | 1.01E+02±6.05E+01+ | 9.56E+01±4.32E+01+ | 7.11E+01±3.82E+01 |
F5 | 1.05E+02±1.88E+01+ | 1.12E+02±1.81E+01+ | 8.12E+01±2.15E+01≈ | 3.27E+02±4.73E+01+ | 3.53E+02±2.02E+01+ | 1.78E+02±1.27E+01+ | 7.83E+01±1.77E+01 |
F6 | 6.63E−13±1.43E−13+ | 2.90E−08±9.81E−08+ | 1.15E−05±1.96E−05≈ | 4.38E+01±1.12E+01+ | 3.60E−02±1.10E−01+ | 8.17E−03±4.72E−03+ | 4.55E−13±1.12E−13 |
F7 | 1.77E+02±1.47E+01≈ | 1.62E+02±2.47E+01≈ | 1.89E+02±2.52E+01+ | 7.11E+02±1.49E+02+ | 4.28E+02±2.94E+01+ | 2.72E+02±2.09E+01+ | 1.69E+02±2.47E+01 |
F8 | 1.02E+02±2.03E+01+ | 1.12E+02±1.87E+01+ | 7.77E+01±1.78E+01≈ | 3.44E+02±4.50E+01+ | 3.60E+02±1.62E+01+ | 1.81E+02±1.51E+01+ | 7.85E+01±1.58E+01 |
F9 | 2.32E+02±2.11E+02+ | 2.12E+02±1.18E+02+ | 1.08E+02±1.10E+02+ | 9.67E+03±2.08E+03+ | 5.35E+01±2.02E+02+ | 4.20E+02±9.59E+02+ | 2.62E+01±2.74E+01 |
F10 | 3.94E+03±5.03E+02≈ | 3.88E+03±4.66E+02≈ | 3.84E+03±3.84E+02≈ | 6.71E+03±1.07E+03+ | 1.22E+04±6.62E+02+ | 6.29E+03±4.18E+02+ | 3.78E+03±4.13E+02 |
F11 | 1.49E+02±3.44E+01+ | 1.65E+02±5.18E+01+ | 1.86E+02±4.90E+01+ | 1.42E+02±3.76E+01+ | 2.41E+02±2.99E+01+ | 1.07E+02±2.31E+01≈ | 1.18E+02±4.29E+01 |
F12 | 5.18E+05±2.49E+05+ | 1.42E+05±1.16E+05− | 8.07E+04±4.03E+04− | 5.70E+05±2.96E+05+ | 5.93E+06±3.15E+06+ | 1.84E+05±1.06E+05− | 3.81E+05±2.02E+05 |
F13 | 6.33E+02±2.47E+02+ | 1.50E+03±1.26E+03+ | 6.97E+02±4.59E+02≈ | 2.63E+03±3.33E+03+ | 5.36E+04±2.13E+05+ | 1.30E+03±1.42E+03+ | 5.10E+02±4.38E+02 |
F14 | 2.70E+04±1.69E+04≈ | 1.54E+04±1.17E+04− | 1.03E+04±7.06E+03− | 2.77E+04±2.05E+04≈ | 2.29E+05±9.37E+04+ | 1.11E+02±1.75E+01− | 3.64E+04±2.73E+04 |
F15 | 3.00E+02±2.31E+02+ | 5.40E+02±4.62E+02+ | 3.78E+02±4.07E+02≈ | 6.29E+03±5.46E+03+ | 3.97E+05±8.32E+05+ | 4.77E+03±2.46E+03+ | 1.99E+02±1.25E+02 |
F16 | 1.01E+03±2.61E+02≈ | 1.10E+03±2.75E+02+ | 8.91E+02±2.85E+02≈ | 1.89E+03±3.66E+02+ | 1.38E+03±6.26E+02+ | 7.69E+02±1.58E+02− | 9.49E+02±1.85E+02 |
F17 | 8.33E+02±1.48E+02+ | 8.14E+02±2.37E+02+ | 7.20E+02±1.69E+02≈ | 1.50E+03±3.63E+02+ | 1.25E+03±2.86E+02+ | 7.29E+02±1.12E+02≈ | 6.65E+02±1.77E+02 |
F18 | 1.40E+05±9.18E+04≈ | 1.03E+05±1.93E+05− | 6.48E+04±5.77E+04− | 1.39E+05±6.87E+04≈ | 2.71E+06±1.18E+06+ | 5.24E+03±2.46E+03− | 1.86E+05±1.80E+05 |
F19 | 6.16E+02±8.92E+02≈ | 3.76E+02±3.38E+02≈ | 6.33E+02±1.08E+03≈ | 1.39E+04±1.09E+04+ | 2.06E+04±1.24E+04+ | 7.03E+03±7.90E+03≈ | 7.31E+02±1.36E+03 |
F20 | 5.05E+02±2.01E+02+ | 6.17E+02±1.59E+02+ | 5.00E+02±2.19E+02+ | 1.25E+03±3.68E+02+ | 1.01E+03±3.19E+02+ | 4.80E+02±1.28E+02+ | 3.99E+02±1.64E+02 |
F21 | 3.04E+02±2.13E+01+ | 3.08E+02±2.28E+01+ | 2.72E+02±2.36E+01≈ | 4.77E+02±4.99E+01+ | 5.39E+02±1.84E+01+ | 3.48E+02±1.32E+01+ | 2.70E+02±1.12E+01 |
F22 | 3.17E+03±2.10E+03≈ | 3.96E+03±1.82E+03+ | 2.89E+03±2.18E+03≈ | 7.34E+03±1.02E+03+ | 1.18E+04±3.25E+03+ | 3.27E+03±3.46E+03≈ | 3.19E+03±2.08E+03 |
F23 | 5.46E+02±2.71E+01+ | 5.56E+02±2.92E+01+ | 5.22E+02±3.04E+01≈ | 8.30E+02±1.12E+02+ | 7.92E+02±1.84E+01+ | 5.95E+02±2.53E+01+ | 5.29E+02±2.52E+01 |
F24 | 6.35E+02±3.23E+01+ | 6.17E+02±2.89E+01+ | 5.77E+02±2.50E+01− | 8.99E+02±1.16E+02+ | 8.52E+02±1.54E+01+ | 6.45E+02±2.96E+01+ | 5.92E+02±2.36E+01 |
F25 | 5.08E+02±3.32E+01≈ | 5.15E+02±3.05E+01≈ | 5.21E+02±3.32E+01≈ | 5.59E+02±3.68E+01+ | 5.44E+02±2.80E+01+ | 5.76E+02±1.76E+01+ | 5.20E+02±3.22E+01 |
F26 | 1.42E+03±9.15E+02≈ | 1.59E+03±1.07E+03≈ | 1.70E+03±5.29E+02≈ | 3.89E+03±3.87E+03≈ | 4.48E+03±8.29E+02+ | 2.27E+03±1.62E+03+ | 1.51E+03±8.29E+02 |
F27 | 6.31E+02±2.76E+01≈ | 6.46E+02±3.54E+01≈ | 6.67E+02±3.19E+01≈ | 9.66E+02±1.74E+02+ | 6.46E+02±5.44E+01≈ | 6.21E+02±4.76E+01− | 6.48E+02±4.34E+01 |
F28 | 4.80E+02±2.21E+01≈ | 4.88E+02±2.04E+01≈ | 4.92E+02±2.25E+01≈ | 4.95E+02±2.72E+01≈ | 4.80E+02±2.30E+01≈ | 5.17E+02±2.04E+01+ | 4.93E+02±1.81E+01 |
F29 | 6.94E+02±1.96E+02≈ | 7.62E+02±1.50E+02≈ | 7.21E+02±1.59E+02≈ | 1.53E+03±3.60E+02+ | 1.39E+03±2.72E+02+ | 7.59E+02±8.20E+01≈ | 7.10E+02±1.80E+02 |
F30 | 7.02E+05±5.32E+04≈ | 7.03E+05±6.25E+04≈ | 7.43E+05±7.14E+04≈ | 1.05E+06±3.28E+05+ | 1.52E+07±1.16E+07+ | 8.25E+05±4.54E+04+ | 7.05E+05±5.83E+04 |
+/≈/− | 13/15/1 | 15/9/5 | 5/19/5 | 24/5/0 | 27/2/0 | 19/5/5 | / |
IEEE CEC2017 with 100D | HCLPSO | SDPSO | MRFO | I-GWO | BeSD | MPSORL |
mean±std | mean±std | mean±std | mean±std | mean±std | mean±std | |
F1 | 6.74E+03±7.22E+03≈ | 7.59E+03±8.88E+03≈ | 5.59E+03±7.18E+03≈ | 1.30E+05±5.51E+04+ | 3.34E+03±2.87E+03≈ | 7.31E+03±7.60E+03 |
F3 | 7.51E+03±3.89E+03− | 2.07E+04±1.75E+04− | 8.58E+04±1.31E+04≈ | 9.01E+04±1.11E+04≈ | 3.42E+04±5.67E+03− | 8.75E+04±1.84E+04 |
F4 | 2.16E+02±3.25E+01≈ | 1.95E+02±4.18E+01− | 2.37E+02±4.09E+01≈ | 3.06E+02±3.91E+01+ | 2.81E+02±4.11E+01+ | 2.28E+02±5.13E+01 |
F5 | 2.91E+02±5.87E+01≈ | 3.05E+02±8.14E+01≈ | 7.98E+02±6.75E+01+ | 9.15E+02±3.20E+01+ | 6.46E+02±3.17E+01+ | 2.81E+02±5.72E+01 |
F6 | 2.56E−05±8.27E−05− | 4.90E−02±3.90E−02+ | 5.13E+01±6.93E+00+ | 1.93E+00±9.54E−01+ | 4.36E−01±1.80E−01+ | 8.82E−04±1.13E−03 |
F7 | 5.79E+02±7.60E+01+ | 5.43E+02±1.46E+02+ | 2.17E+03±2.71E+02+ | 1.10E+03±6.67E+01+ | 7.33E+02±4.82E+01+ | 4.22E+02±8.11E+01 |
F8 | 2.98E+02±5.97E+01≈ | 3.21E+02±6.77E+01+ | 8.83E+02±8.99E+01+ | 9.15E+02±3.05E+01+ | 6.52E+02±3.42E+01+ | 2.88E+02±6.62E+01 |
F9 | 1.55E+03±7.37E+02+ | 4.79E+03±2.78E+03+ | 2.06E+04±1.69E+03+ | 4.15E+03±3.17E+03+ | 1.91E+04±5.45E+03+ | 5.35E+02±3.20E+02 |
F10 | 1.17E+04±1.02E+03+ | 1.20E+04±7.35E+02+ | 1.41E+04±1.21E+03+ | 2.89E+04±7.52E+02+ | 1.84E+04±6.20E+02+ | 1.10E+04±8.17E+02 |
F11 | 7.57E+02±1.78E+02≈ | 9.67E+02±1.92E+02+ | 5.66E+02±1.18E+02− | 5.74E+03±1.66E+03+ | 6.27E+02±1.35E+02− | 7.10E+02±1.19E+02 |
F12 | 7.72E+05±3.83E+05− | 3.04E+05±1.00E+05− | 1.40E+06±5.88E+05+ | 4.01E+07±1.53E+07+ | 2.26E+06±7.87E+05+ | 1.03E+06±4.16E+05 |
F13 | 1.40E+03±8.97E+02+ | 1.64E+03±9.03E+02+ | 6.05E+03±8.30E+03+ | 7.10E+03±7.79E+03+ | 3.27E+03±1.64E+03+ | 9.36E+02±4.44E+02 |
F14 | 1.11E+05±3.95E+04≈ | 5.95E+04±2.98E+04− | 1.33E+05±8.46E+04≈ | 3.85E+06±1.83E+06+ | 4.78E+03±3.89E+03− | 1.43E+05±6.90E+04 |
F15 | 6.80E+02±2.92E+02+ | 5.05E+02±1.33E+02≈ | 2.34E+03±2.69E+03+ | 3.05E+03±3.30E+03+ | 5.44E+02±3.77E+02≈ | 5.34E+02±1.39E+02 |
F16 | 3.20E+03±3.53E+02+ | 2.90E+03±4.68E+02≈ | 3.95E+03±6.79E+02+ | 5.73E+03±1.58E+03+ | 3.33E+03±2.88E+02+ | 2.76E+03±4.80E+02 |
F17 | 2.41E+03±3.39E+02≈ | 2.22E+03±2.87E+02≈ | 2.96E+03±5.02E+02+ | 4.80E+03±4.04E+02+ | 2.15E+03±2.11E+02≈ | 2.23E+03±2.91E+02 |
F18 | 2.09E+05±8.99E+04− | 1.17E+05±3.31E+04− | 3.29E+05±1.21E+05≈ | 1.13E+07±7.10E+06+ | 5.71E+04±1.82E+04− | 4.13E+05±1.83E+05 |
F19 | 4.43E+02±2.87E+02+ | 3.32E+02±2.18E+02≈ | 2.93E+03±3.10E+03+ | 3.16E+03±3.87E+03+ | 9.38E+02±5.61E+02+ | 2.85E+02±1.11E+02 |
F20 | 2.25E+03±2.52E+02+ | 2.08E+03±3.21E+02+ | 3.19E+03±5.90E+02+ | 4.25E+03±3.40E+02+ | 2.43E+03±2.55E+02+ | 1.87E+03±2.71E+02 |
F21 | 5.47E+02±5.29E+01≈ | 5.32E+02±6.67E+01≈ | 9.60E+02±1.28E+02+ | 1.12E+03±3.20E+01+ | 7.73E+02±2.92E+01+ | 5.41E+02±6.73E+01 |
F22 | 1.32E+04±1.15E+03+ | 1.21E+04±4.15E+03+ | 1.68E+04±1.49E+03+ | 2.98E+04±1.01E+03+ | 2.02E+04±4.18E+02+ | 1.17E+04±3.25E+03 |
F23 | 8.03E+02±2.63E+01+ | 7.83E+02±3.24E+01≈ | 1.33E+03±1.38E+02+ | 1.38E+03±3.55E+01+ | 1.06E+03±2.63E+01+ | 7.86E+02±2.07E+01 |
F24 | 1.31E+03±5.20E+01≈ | 1.30E+03±5.71E+01≈ | 2.19E+03±2.78E+02+ | 1.76E+03±3.11E+01+ | 1.32E+03±9.25E+01≈ | 1.29E+03±6.33E+01 |
F25 | 7.38E+02±6.47E+01− | 7.78E+02±6.94E+01≈ | 7.84E+02±5.27E+01≈ | 8.61E+02±4.59E+01+ | 8.62E+02±4.13E+01+ | 7.88E+02±6.23E+01 |
F26 | 7.29E+03±6.01E+02≈ | 7.72E+03±6.71E+02+ | 1.69E+04±7.42E+03+ | 1.22E+04±5.14E+02+ | 1.23E+04±2.30E+03+ | 6.28E+03±2.76E+03 |
F27 | 7.91E+02±3.21E+01≈ | 7.73E+02±3.15E+01≈ | 1.22E+03±1.85E+02+ | 9.53E+02±1.30E+02+ | 8.53E+02±5.71E+01+ | 7.77E+02±2.78E+01 |
F28 | 5.71E+02±3.64E+01≈ | 5.65E+02±2.80E+01≈ | 5.70E+02±3.28E+01≈ | 6.69E+02±3.07E+01+ | 6.69E+02±2.81E+01+ | 5.65E+02±2.94E+01 |
F29 | 2.93E+03±2.89E+02≈ | 3.01E+03±4.44E+02≈ | 3.97E+03±5.99E+02+ | 4.90E+03±4.35E+02+ | 3.49E+03±2.48E+02+ | 3.08E+03±4.84E+02 |
F30 | 5.83E+03±2.82E+03≈ | 5.73E+03±2.78E+03≈ | 7.14E+03±3.85E+03≈ | 2.43E+05±1.07E+05+ | 1.06E+04±2.71E+03+ | 5.80E+03±3.15E+03 |
+/≈/− | 10/14/5 | 10/14/5 | 20/8/1 | 28/1/0 | 21/4/4 | / |
IEEE CEC2019 | LDWPSO | UPSO | CLPSO | LIPS | HCLPSO | MPSORL |
mean±std | mean±std | mean±std | mean±std | mean±std | mean±std | |
F31 | 5.22E+04±8.95E+04≈ | 5.71E+04±3.49E+04− | 8.62E+05±5.36E+05+ | 4.70E+04±4.30E+04≈ | 6.08E+04±1.20E+05≈ | 5.93E+04±6.88E+04 |
F32 | 2.61E+02±1.04E+02≈ | 3.23E+02±1.35E+02≈ | 6.25E+02±1.54E+02+ | 2.73E+02±9.38E+01≈ | 2.43E+02±8.17E+01≈ | 2.44E+02±1.03E+02 |
F33 | 3.95E−01±7.47E−02≈ | 4.80E−01±4.01E−01+ | 9.06E−01±3.06E−01+ | 6.22E−01±8.09E−01≈ | 3.95E−01±7.47E−02+ | 3.66E−01±1.33E−01 |
F34 | 8.52E+00±4.08E+00+ | 9.20E+00±2.93E+00+ | 4.27E+00±1.21E+00≈ | 1.05E+01±4.19E+00+ | 4.41E+00±1.47E+00≈ | 4.55E+00±1.77E+00 |
F35 | 1.05E−01±6.19E−02+ | 3.86E−02±1.90E−02≈ | 6.52E−02±2.62E−02+ | 1.00E−02±8.04E−03− | 5.13E−02±2.18E−02≈ | 3.33E−02±2.24E−02 |
F36 | 3.15E−01±5.71E−01+ | 4.36E−01±5.00E−01+ | 1.59E−01±7.46E−02+ | 4.76E−01±5.95E−01+ | 1.99E−03±2.78E−03+ | 3.89E−04±1.69E−03 |
F37 | 4.41E+02±2.38E+02+ | 4.66E+02±1.71E+02+ | 1.47E+02±7.73E+01≈ | 7.55E+02±2.49E+02+ | 2.49E+02±1.11E+02+ | 1.61E+02±1.30E+02 |
F38 | 1.99E+00±4.61E−01+ | 2.21E+00±4.28E−01+ | 2.03E+00±2.62E−01+ | 3.04E+00±4.87E−01+ | 1.65E+00±3.53E−01+ | 1.31E+00±6.48E−01 |
F39 | 1.32E−01±6.08E−02≈ | 6.99E−02±3.05E−02− | 1.75E−01±3.92E−02+ | 1.23E−01±4.73E−02≈ | 1.01E−01±3.46E−02− | 1.30E−01±3.08E−02 |
F40 | 1.42E+01±9.09E+00≈ | 1.46E+01±8.32E+00≈ | 1.88E+01±3.91E+00+ | 1.93E+01±3.65E+00≈ | 1.54E+01±8.57E+00≈ | 1.21E+01±9.91E+00 |
+/≈/− | 5/5/0 | 5/3/2 | 8/2/0 | 4/5/1 | 4/5/1 | / |
IEEE CEC2019 | EPSO | SDPSO | MRFO | I-GWO | BeSD | MPSORL |
mean±std | mean±std | mean±std | mean±std | mean±std | mean±std | |
F31 | 3.20E+04±2.87E+04≈ | 4.42E+04±5.14E+04≈ | 0.00E+00±0.00E+00− | 7.24E+04±1.35E+05≈ | 0.00E+00±0.00E+00− | 5.93E+04±6.88E+04 |
F32 | 2.30E+02±8.66E+01≈ | 2.58E+02±1.05E+02≈ | 3.70E+00±3.69E−01− | 1.06E+03±3.38E+02+ | 4.84E+00±1.97E+00− | 2.44E+02±1.03E+02 |
F33 | 3.41E−01±1.55E−01≈ | 3.55E−01±1.40E−01≈ | 3.95E−01±7.47E−02≈ | 1.95E+00±1.18E+00+ | 9.35E−01±2.89E−01+ | 3.66E−01±1.33E−01 |
F34 | 5.82E+00±1.98E+00+ | 4.48E+00±1.50E+00≈ | 2.50E+01±1.55E+01+ | 1.98E+01±4.20E+00+ | 8.50E+00±1.96E+00+ | 4.55E+00±1.77E+00 |
F35 | 8.57E−02±4.08E−02+ | 4.81E−02±3.37E−02≈ | 1.11E−01±1.07E−01+ | 5.26E−01±9.12E−02+ | 5.04E−02±1.27E−02≈ | 3.33E−02±2.24E−02 |
F36 | 2.46E−01±2.42E−01+ | 2.72E−02±1.21E−01≈ | 2.13E+00±1.70E+00+ | 1.93E−01±3.60E−01+ | 1.34E−01±1.17E−01+ | 3.89E−04±1.69E−03 |
F37 | 2.40E+02±1.47E+02≈ | 1.80E+02±1.04E+02≈ | 7.29E+02±3.16E+02+ | 7.33E+02±2.42E+02+ | 4.46E+02±1.29E+02+ | 1.61E+02±1.30E+02 |
F38 | 1.65E+00±4.69E−01+ | 1.19E+00±5.05E−01≈ | 2.45E+00±3.70E−01+ | 2.06E+00±2.53E−01+ | 2.50E+00±1.64E−01+ | 1.31E+00±6.48E−01 |
F39 | 1.27E−01±3.64E−02≈ | 1.32E−01±2.78E−02≈ | 2.05E−01±7.63E−02+ | 1.84E−01±4.15E−02+ | 1.11E−01±1.76E−02− | 1.30E−01±3.08E−02 |
F40 | 1.24E+01±9.65E+00+ | 1.22E+01±9.85E+00≈ | 1.50E+01±9.02E+00+ | 1.50E+01±9.03E+00+ | 1.76E+01±4.33E+00+ | 1.21E+01±9.91E+00 |
+/≈/− | 5/5/0 | 0/10/0 | 7/1/2 | 9/1/0 | 6/1/3 | / |
MPSORL | Non-uniform vs. Uniform status division | |||||
Wins (+) | Losses (−) | Ties | R+ | R− | p-value | |
30D | 14 | 15 | 0 | 220 | 215 | 0.957 |
50D | 19 | 10 | 0 | 278 | 157 | 0.191 |
100D | 20 | 9 | 0 | 297 | 138 | 0.086 |
Function type | No. | Function | F(x∗) | D | search range |
Bowl-Shaped | F41 | Sphere Function | 0 | 2 | [-100 100] |
Many Local Minima | F42 | Rastrigin Function | 0 | 2 | [-5.12 5.12] |
Many Local Minima | F43 | Ackley Function | 0 | 2 | [-32.768, 32.768] |
Plate-Shaped | F44 | Matyas Function | 0 | 2 | [-10 10] |
Algorithm 1 Q-learning algorithm |
1: Initialize Q(s, a), α, γ, r, ε 2: Repeat : 3: Choose the best action at for the current state st using policy (e.g., ε-greedy strategy); 4: Execute action at; 5: Get the immediate reward rt+1; 6: Get the maximum Q-value for the next state st+1; 7: Update the Q-table by Eq (2.5); 8: Update the current state st←st+1; 9: Until the condition is terminal |
Q-learning | PSO |
Agents | Particles |
Environment | Optimization problems |
State | Fitness value |
Action | Strategy |
State | Action | |||
a1 | a2 | a3 | a4 | |
s1 | Q(s1, a1) | Q(s1, a2) | Q(s1, a3) | Q(s1, a4) |
s2 | Q(s2, a1) | Q(s2, a2) | Q(s2, a3) | Q(s2, a4) |
s3 | Q(s3, a1) | Q(s3, a2) | Q(s3, a3) | Q(s3, a4) |
s4 | Q(s4, a1) | Q(s4, a2) | Q(s4, a3) | Q(s4, a4) |
s5 | Q(s5, a1) | Q(s5, a2) | Q(s5, a3) | Q(s5, a4) |
Algorithm 2 The proposed algorithm |
Input:
Initialize N, D, the maximum number of fitness evaluations (Max_Fes); Initialize the maximum number of iterations (Max_Iter), k=0, kk=0, fit=0; Initialize the inertia weight w, learning factors c1, c2, position, velocity; Evaluate the fitness value, fit=fit+N, record pbest and gbest; Initialize Q-table, state, reward, learning period LP, ε, α, γ. Output: Best solution. 1: while termination condition is not met do 2: k=k+1; 3: /* pop1 */ 4: for i=1:length(pop1) do 5: Update the particle's velocity and position by CLPSO; 6: Evaluate the fitness of the particle, fit=fit+1; 7: Update pbesti and gbest; 8: end for 9: /* pop2 */ 10: Divide state for pop2 according to Section 3.2.1; 11: Select action based on Q-table using ε-greedy strategy according to Eq (3.1); 12: for i=length(pop1)+1:N do 13: Update the particle's velocity by action ai; 14: Update the particle's position; 15: Evaluate the fitness of the particle, fit=fit+1; 16: Update pbesti and gbest; 17: end for 18: /* update Q-table */ 19: if k<Max_Iter then 20: if mod(k, LP) = 0 then 21: Determine next state; 22: Select action using ε-greedy strategy; 23: Calculate reward according to Eq (3.2); 24: Update Q-table using Eq (2.5); 25: end if 26: else 27: if mod(kk, LP) = 0 then 28: Determine next state; 29: Calculate reward according to Eq (3.2); 30: Update Q-table using Eq (2.5); 31: end if 32: kk=kk+1; 33: end if 34: end while |
Function type | No. | Test Functions | F(x∗) | D |
Unimodal Functions | F1 | Shifted and Rotated Bent Cigar Function | 100 | 30/50/100 |
F2 | Shifted and Rotated Sum of Different Power Function* | 200 | 30/50/100 | |
Simple Multimodal Functions | F3 | Shifted and Rotated Zakharov Function | 300 | 30/50/100 |
F4 | Shifted and Rotated Rosenbrock Function | 400 | 30/50/100 | |
F5 | Shifted and Rotated Rastrigin Function | 500 | 30/50/100 | |
F6 | Shifted and Rotated Expanded Schaffer's F6 Function | 600 | 30/50/100 | |
F7 | Shifted and Rotated Lunacek Bi-Rastrigin Function | 700 | 30/50/100 | |
F8 | Shifted and Rotated Non-Continuous Rastrigin Function | 800 | 30/50/100 | |
F9 | Shifted and Rotated Levy Function | 900 | 30/50/100 | |
Hybrid Functions | F10 | Shifted and Rotated Schwefel Function | 1000 | 30/50/100 |
F11 | Hybrid Function 1 (N = 3) | 1100 | 30/50/100 | |
F12 | Hybrid Function 2 (N = 3) | 1200 | 30/50/100 | |
F13 | Hybrid Function 3 (N = 3) | 1300 | 30/50/100 | |
F14 | Hybrid Function 4 (N = 4) | 1400 | 30/50/100 | |
F15 | Hybrid Function 5 (N = 4) | 1500 | 30/50/100 | |
F16 | Hybrid Function 6 (N = 4) | 1600 | 30/50/100 | |
F17 | Hybrid Function 6 (N = 5) | 1700 | 30/50/100 | |
F18 | Hybrid Function 6 (N = 5) | 1800 | 30/50/100 | |
F19 | Hybrid Function 6 (N = 5) | 1900 | 30/50/100 | |
Composition Functions | F20 | Hybrid Function 6 (N = 6) | 2000 | 30/50/100 |
F21 | Composition Function 1 (N = 3) | 2100 | 30/50/100 | |
F22 | Composition Function 2 (N=3) | 2200 | 30/50/100 | |
F23 | Composition Function 3 (N = 4) | 2300 | 30/50/100 | |
F24 | Composition Function 4 (N = 4) | 2400 | 30/50/100 | |
F25 | Composition Function 5 (N = 5) | 2500 | 30/50/100 | |
F26 | Composition Function 6 (N = 5) | 2600 | 30/50/100 | |
F27 | Composition Function 7 (N = 6) | 2700 | 30/50/100 | |
F28 | Composition Function 8 (N = 6) | 2800 | 30/50/100 | |
F29 | Composition Function 9 (N = 3) | 2900 | 30/50/100 | |
F30 | Composition Function 10 (N = 3) | 3000 | 30/50/100 | |
Note: *F2 has been excluded because it shows unstable behavior especially for higher dimensions. |
No. | Functions | F(x∗) | D | Search range |
F31 | Storn's Chebyshev Polynomial Fitting Problem | 1 | 9 | [-8192, 8192] |
F32 | Inverse Hilbert Matrix Problem | 1 | 16 | [-16384, 16384] |
F33 | Lennard-Jones Minimum Energy Cluster | 1 | 18 | [-4, 4] |
F34 | Rastrigin Function | 1 | 10 | [-100,100] |
F35 | Griewank's Function | 1 | 10 | [-100,100] |
F36 | Weierstrass Function | 1 | 10 | [-100,100] |
F37 | Modified Schwefel Function | 1 | 10 | [-100,100] |
F38 | Expanded Schaffer's F6 Function | 1 | 10 | [-100,100] |
F39 | Happy Cat Function | 1 | 10 | [-100,100] |
F40 | Ackley Function | 1 | 10 | [-100,100] |
Algorithm | Key parameters | Year | Ref. |
LDWPSO | w:0.9−0.2, c1:2.5−0.5, c2:0.5−2.5, Vmax = 0.5*range | 1998 | [50] |
CLPSO | w:0.9−0.2, c:3.0−1.5, Vmax = 0.5*range | 2006 | [6] |
UPSO | w:0.9−0.2, c1:2.5−0.5, c2:0.5−2.5 | 2004 | [53] |
LIPS | χ:0.7298, Vmax = 0.5*range, nsize = 3 | 2013 | [52] |
HCLPSO | w:0.99−0.2, c:3.0−1.5, c1:2.5−0.5, c2:0.5−2.5, Vmax = 0.2*range | 2015 | [7] |
EPSO | w:0.9−0.4, c:3.0−1.5, w1:0.9−0.2, c1:2.5−0.5, c2:0.5−2.5, Vmax = 0.5*range | 2017 | [34] |
SDPSO | w:0.9−0.2, c:3.0−1.5, c1:2.5−0.5, c2:0.5−2.5, Vmax = 0.5*range | 2022 | [8] |
MRFO | S = 2 | 2020 | [58] |
I-GWO | a = 2−0 | 2021 | [59] |
BeSD | K = 5 | 2021 | [60] |
MPSORL | w:0.9−0.2, c:3.0−1.5, c1:2.5−0.5, c2:0.5−2.5, Vmax = 0.5*range | − | − |
Level | Factors | ||||
pop1 (N1) | LP | epsilon (ε) | alpha (α) | gamma (γ) | |
1 | 0.1 | 20 | 0.80 | 0.3 | 0.6 |
2 | 0.2 | 50 | 0.85 | 0.4 | 0.7 |
3 | 0.3 | 100 | 0.90 | 0.5 | 0.8 |
4 | 0.4 | 200 | 0.95 | 0.6 | 0.9 |
pop1 (N1) | LP | epsilon (ε) | alpha (α) | gamma (γ) | average rank | |
1 | 0.1 | 20 | 0.8 | 0.3 | 0.6 | 8.5 |
2 | 0.1 | 50 | 0.85 | 0.4 | 0.7 | 9.345 |
3 | 0.1 | 100 | 0.9 | 0.5 | 0.8 | 10.672 |
4 | 0.1 | 200 | 0.95 | 0.6 | 0.9 | 11.483 |
5 | 0.2 | 20 | 0.85 | 0.5 | 0.9 | 7.81 |
6 | 0.2 | 50 | 0.8 | 0.6 | 0.8 | 6.207 |
7 | 0.2 | 100 | 0.95 | 0.3 | 0.7 | 10.293 |
8 | 0.2 | 200 | 0.9 | 0.4 | 0.6 | 9.207 |
9 | 0.3 | 20 | 0.9 | 0.6 | 0.7 | 7.879 |
10 | 0.3 | 50 | 0.95 | 0.5 | 0.6 | 9.828 |
11 | 0.3 | 100 | 0.8 | 0.4 | 0.9 | 6.379 |
12 | 0.3 | 200 | 0.85 | 0.3 | 0.8 | 7.293 |
13 | 0.4 | 20 | 0.95 | 0.4 | 0.8 | 9.362 |
14 | 0.4 | 50 | 0.9 | 0.3 | 0.9 | 7.931 |
15 | 0.4 | 100 | 0.85 | 0.6 | 0.6 | 6.793 |
16 | 0.4 | 200 | 0.8 | 0.5 | 0.7 | 7.017 |
IEEE CEC2017 with 30D | LDWPSO | UPSO | CLPSO | LIPS | MPSORL |
mean±std | mean±std | mean±std | mean±std | mean±std | |
F1 | 4.64E+03±5.02E+03+ | 2.03E+03±2.03E+03+ | 8.17E+00±1.07E+01− | 9.24E+02±1.60E+03+ | 1.05E+02±2.10E+02 |
F3 | 3.07E+02±4.39E+02+ | 1.46E+02±2.08E+02+ | 2.84E+04±6.40E+03+ | 2.49E+03±1.59E+03+ | 2.65E−02±7.68E−02 |
F4 | 9.26E+01±2.63E+01+ | 3.58E+01±3.85E+01≈ | 5.90E+01±2.66E+01+ | 3.59E+01±2.95E+01≈ | 4.13E+01±2.72E+01 |
F5 | 6.95E+01±1.83E+01+ | 6.99E+01±1.13E+01+ | 4.76E+01±8.81E+00+ | 1.05E+02±2.29E+01+ | 3.25E+01±1.00E+01 |
F6 | 4.27E−01±5.54E−01+ | 1.20E+00±9.13E−01+ | 4.92E−07±2.49E−07+ | 2.29E+01±8.13E+00+ | 2.35E−13±6.63E−14 |
F7 | 1.18E+02±2.17E+01+ | 9.67E+01±1.34E+01+ | 9.22E+01±1.01E+01+ | 1.13E+02±1.93E+01+ | 8.33E+01±1.15E+01 |
F8 | 6.93E+01±1.74E+01+ | 6.44E+01±1.13E+01+ | 5.33E+01±7.36E+00+ | 9.36E+01±2.08E+01+ | 3.67E+01±1.33E+01 |
F9 | 1.40E+02±1.22E+02+ | 9.18E+01±6.82E+01+ | 1.18E+02±5.19E+01+ | 1.52E+03±9.83E+02+ | 1.27E+00±1.05E+00 |
F10 | 3.21E+03±6.26E+02+ | 2.90E+03±4.36E+02+ | 2.18E+03±2.71E+02+ | 3.11E+03±4.73E+02+ | 1.86E+03±3.64E+02 |
F11 | 8.61E+01±4.17E+01+ | 5.86E+01±2.68E+01+ | 6.69E+01±2.04E+01+ | 1.04E+02±3.48E+01+ | 4.65E+01±3.30E+01 |
F12 | 3.79E+04±1.95E+04+ | 8.47E+04±8.91E+04+ | 4.02E+05±2.22E+05+ | 1.30E+05±1.33E+05+ | 2.34E+04±1.03E+04 |
F13 | 9.64E+03±1.20E+04+ | 7.86E+03±6.06E+03+ | 3.41E+02±1.26E+02≈ | 4.42E+03±5.13E+03+ | 4.90E+02±5.07E+02 |
F14 | 6.14E+03±4.66E+03+ | 3.66E+03±3.79E+03≈ | 2.89E+04±2.86E+04+ | 8.02E+03±5.99E+03+ | 3.94E+03±5.06E+03 |
F15 | 5.33E+03±5.78E+03+ | 2.47E+03±2.83E+03+ | 1.44E+02±1.03E+02− | 1.97E+03±3.02E+03+ | 2.88E+02±3.70E+02 |
F16 | 7.60E+02±2.01E+02+ | 6.57E+02±1.25E+02+ | 5.16E+02±1.21E+02+ | 7.24E+02±2.56E+02+ | 3.97E+02±1.79E+02 |
F17 | 2.26E+02±1.59E+02+ | 1.69E+02±7.73E+01+ | 1.22E+02±5.72E+01+ | 2.88E+02±1.55E+02+ | 9.27E+01±4.66E+01 |
F18 | 9.36E+04±5.84E+04≈ | 1.04E+05±4.07E+04≈ | 1.37E+05±6.93E+04+ | 8.81E+04±7.43E+04≈ | 8.72E+04±5.27E+04 |
F19 | 7.41E+03±1.02E+04+ | 2.04E+03±2.27E+03+ | 5.83E+01±4.54E+01− | 2.50E+03±3.42E+03+ | 1.55E+02±1.42E+02 |
F20 | 2.78E+02±1.26E+02+ | 2.77E+02±7.74E+01+ | 1.56E+02±7.37E+01≈ | 4.76E+02±1.29E+02+ | 1.34E+02±6.92E+01 |
F21 | 2.70E+02±1.43E+01+ | 2.62E+02±3.02E+01+ | 2.40E+02±4.08E+01+ | 2.95E+02±2.04E+01+ | 2.33E+02±9.38E+00 |
F22 | 3.90E+02±1.10E+03+ | 1.01E+02±1.73E+00+ | 1.13E+02±6.95E+00+ | 3.19E+02±8.32E+02≈ | 1.00E+02±1.08E+00 |
F23 | 4.29E+02±2.15E+01+ | 4.32E+02±2.02E+01+ | 4.00E+02±1.20E+01+ | 5.35E+02±3.83E+01+ | 3.86E+02±1.07E+01 |
F24 | 5.15E+02±3.12E+01+ | 4.87E+02±1.20E+01+ | 4.76E+02±9.15E+01+ | 5.70E+02±4.66E+01+ | 4.53E+02±7.86E+00 |
F25 | 3.96E+02±1.37E+01+ | 3.89E+02±5.82E+00+ | 3.87E+02±1.13E+00≈ | 3.87E+02±4.05E+00≈ | 3.87E+02±1.05E+00 |
F26 | 1.43E+03±7.28E+02+ | 6.40E+02±7.57E+02≈ | 4.02E+02±1.94E+02+ | 9.96E+02±1.18E+03≈ | 3.75E+02±3.19E+02 |
F27 | 5.37E+02±1.22E+01+ | 5.48E+02±1.57E+01+ | 5.10E+02±5.27E+00− | 5.84E+02±2.16E+01+ | 5.15E+02±6.39E+00 |
F28 | 4.13E+02±2.81E+01+ | 3.15E+02±3.50E+01≈ | 4.20E+02±6.12E+00+ | 3.10E+02±2.98E+01≈ | 3.36E+02±5.26E+01 |
F29 | 6.76E+02±1.76E+02+ | 7.95E+02±1.28E+02+ | 5.44E+02±6.75E+01+ | 1.03E+03±1.70E+02+ | 4.94E+02±4.09E+01 |
F30 | 5.83E+03±3.07E+03+ | 8.18E+03±6.88E+03+ | 4.82E+03±8.11E+02+ | 6.87E+03±2.12E+03+ | 4.06E+03±1.14E+03 |
+/≈/− | 28/1/0 | 24/5/0 | 22/3/4 | 23/6/0 | / |
IEEE CEC2017 with 50D | HCLPSO | EPSO | SDPSO | MRFO | I-GWO | BeSD | MPSORL |
mean±std | mean±std | mean±std | mean±std | mean±std | mean±std | mean±std | |
F1 | 1.65E+02±2.03E+02≈ | 2.15E+03±2.12E+03+ | 3.46E+02±3.11E+02+ | 4.94E+03±5.51E+03+ | 5.00E+03±6.84E+03+ | 8.66E+02±1.13E+03+ | 1.35E+02±1.34E+02 |
F3 | 1.55E+01±1.61E+01− | 6.38E−04±1.14E−03− | 5.11E+00±2.31E+01− | 6.98E+03±2.44E+03+ | 1.91E+04±4.05E+03+ | 1.22E+03±7.08E+02+ | 5.22E+02±5.03E+02 |
F4 | 7.85E+01±4.42E+01≈ | 4.05E+01±3.72E+01− | 5.97E+01±4.46E+01≈ | 8.48E+01±5.02E+01≈ | 1.01E+02±6.05E+01+ | 9.56E+01±4.32E+01+ | 7.11E+01±3.82E+01 |
F5 | 1.05E+02±1.88E+01+ | 1.12E+02±1.81E+01+ | 8.12E+01±2.15E+01≈ | 3.27E+02±4.73E+01+ | 3.53E+02±2.02E+01+ | 1.78E+02±1.27E+01+ | 7.83E+01±1.77E+01 |
F6 | 6.63E−13±1.43E−13+ | 2.90E−08±9.81E−08+ | 1.15E−05±1.96E−05≈ | 4.38E+01±1.12E+01+ | 3.60E−02±1.10E−01+ | 8.17E−03±4.72E−03+ | 4.55E−13±1.12E−13 |
F7 | 1.77E+02±1.47E+01≈ | 1.62E+02±2.47E+01≈ | 1.89E+02±2.52E+01+ | 7.11E+02±1.49E+02+ | 4.28E+02±2.94E+01+ | 2.72E+02±2.09E+01+ | 1.69E+02±2.47E+01 |
F8 | 1.02E+02±2.03E+01+ | 1.12E+02±1.87E+01+ | 7.77E+01±1.78E+01≈ | 3.44E+02±4.50E+01+ | 3.60E+02±1.62E+01+ | 1.81E+02±1.51E+01+ | 7.85E+01±1.58E+01 |
F9 | 2.32E+02±2.11E+02+ | 2.12E+02±1.18E+02+ | 1.08E+02±1.10E+02+ | 9.67E+03±2.08E+03+ | 5.35E+01±2.02E+02+ | 4.20E+02±9.59E+02+ | 2.62E+01±2.74E+01 |
F10 | 3.94E+03±5.03E+02≈ | 3.88E+03±4.66E+02≈ | 3.84E+03±3.84E+02≈ | 6.71E+03±1.07E+03+ | 1.22E+04±6.62E+02+ | 6.29E+03±4.18E+02+ | 3.78E+03±4.13E+02 |
F11 | 1.49E+02±3.44E+01+ | 1.65E+02±5.18E+01+ | 1.86E+02±4.90E+01+ | 1.42E+02±3.76E+01+ | 2.41E+02±2.99E+01+ | 1.07E+02±2.31E+01≈ | 1.18E+02±4.29E+01 |
F12 | 5.18E+05±2.49E+05+ | 1.42E+05±1.16E+05− | 8.07E+04±4.03E+04− | 5.70E+05±2.96E+05+ | 5.93E+06±3.15E+06+ | 1.84E+05±1.06E+05− | 3.81E+05±2.02E+05 |
F13 | 6.33E+02±2.47E+02+ | 1.50E+03±1.26E+03+ | 6.97E+02±4.59E+02≈ | 2.63E+03±3.33E+03+ | 5.36E+04±2.13E+05+ | 1.30E+03±1.42E+03+ | 5.10E+02±4.38E+02 |
F14 | 2.70E+04±1.69E+04≈ | 1.54E+04±1.17E+04− | 1.03E+04±7.06E+03− | 2.77E+04±2.05E+04≈ | 2.29E+05±9.37E+04+ | 1.11E+02±1.75E+01− | 3.64E+04±2.73E+04 |
F15 | 3.00E+02±2.31E+02+ | 5.40E+02±4.62E+02+ | 3.78E+02±4.07E+02≈ | 6.29E+03±5.46E+03+ | 3.97E+05±8.32E+05+ | 4.77E+03±2.46E+03+ | 1.99E+02±1.25E+02 |
F16 | 1.01E+03±2.61E+02≈ | 1.10E+03±2.75E+02+ | 8.91E+02±2.85E+02≈ | 1.89E+03±3.66E+02+ | 1.38E+03±6.26E+02+ | 7.69E+02±1.58E+02− | 9.49E+02±1.85E+02 |
F17 | 8.33E+02±1.48E+02+ | 8.14E+02±2.37E+02+ | 7.20E+02±1.69E+02≈ | 1.50E+03±3.63E+02+ | 1.25E+03±2.86E+02+ | 7.29E+02±1.12E+02≈ | 6.65E+02±1.77E+02 |
F18 | 1.40E+05±9.18E+04≈ | 1.03E+05±1.93E+05− | 6.48E+04±5.77E+04− | 1.39E+05±6.87E+04≈ | 2.71E+06±1.18E+06+ | 5.24E+03±2.46E+03− | 1.86E+05±1.80E+05 |
F19 | 6.16E+02±8.92E+02≈ | 3.76E+02±3.38E+02≈ | 6.33E+02±1.08E+03≈ | 1.39E+04±1.09E+04+ | 2.06E+04±1.24E+04+ | 7.03E+03±7.90E+03≈ | 7.31E+02±1.36E+03 |
F20 | 5.05E+02±2.01E+02+ | 6.17E+02±1.59E+02+ | 5.00E+02±2.19E+02+ | 1.25E+03±3.68E+02+ | 1.01E+03±3.19E+02+ | 4.80E+02±1.28E+02+ | 3.99E+02±1.64E+02 |
F21 | 3.04E+02±2.13E+01+ | 3.08E+02±2.28E+01+ | 2.72E+02±2.36E+01≈ | 4.77E+02±4.99E+01+ | 5.39E+02±1.84E+01+ | 3.48E+02±1.32E+01+ | 2.70E+02±1.12E+01 |
F22 | 3.17E+03±2.10E+03≈ | 3.96E+03±1.82E+03+ | 2.89E+03±2.18E+03≈ | 7.34E+03±1.02E+03+ | 1.18E+04±3.25E+03+ | 3.27E+03±3.46E+03≈ | 3.19E+03±2.08E+03 |
F23 | 5.46E+02±2.71E+01+ | 5.56E+02±2.92E+01+ | 5.22E+02±3.04E+01≈ | 8.30E+02±1.12E+02+ | 7.92E+02±1.84E+01+ | 5.95E+02±2.53E+01+ | 5.29E+02±2.52E+01 |
F24 | 6.35E+02±3.23E+01+ | 6.17E+02±2.89E+01+ | 5.77E+02±2.50E+01− | 8.99E+02±1.16E+02+ | 8.52E+02±1.54E+01+ | 6.45E+02±2.96E+01+ | 5.92E+02±2.36E+01 |
F25 | 5.08E+02±3.32E+01≈ | 5.15E+02±3.05E+01≈ | 5.21E+02±3.32E+01≈ | 5.59E+02±3.68E+01+ | 5.44E+02±2.80E+01+ | 5.76E+02±1.76E+01+ | 5.20E+02±3.22E+01 |
F26 | 1.42E+03±9.15E+02≈ | 1.59E+03±1.07E+03≈ | 1.70E+03±5.29E+02≈ | 3.89E+03±3.87E+03≈ | 4.48E+03±8.29E+02+ | 2.27E+03±1.62E+03+ | 1.51E+03±8.29E+02 |
F27 | 6.31E+02±2.76E+01≈ | 6.46E+02±3.54E+01≈ | 6.67E+02±3.19E+01≈ | 9.66E+02±1.74E+02+ | 6.46E+02±5.44E+01≈ | 6.21E+02±4.76E+01− | 6.48E+02±4.34E+01 |
F28 | 4.80E+02±2.21E+01≈ | 4.88E+02±2.04E+01≈ | 4.92E+02±2.25E+01≈ | 4.95E+02±2.72E+01≈ | 4.80E+02±2.30E+01≈ | 5.17E+02±2.04E+01+ | 4.93E+02±1.81E+01 |
F29 | 6.94E+02±1.96E+02≈ | 7.62E+02±1.50E+02≈ | 7.21E+02±1.59E+02≈ | 1.53E+03±3.60E+02+ | 1.39E+03±2.72E+02+ | 7.59E+02±8.20E+01≈ | 7.10E+02±1.80E+02 |
F30 | 7.02E+05±5.32E+04≈ | 7.03E+05±6.25E+04≈ | 7.43E+05±7.14E+04≈ | 1.05E+06±3.28E+05+ | 1.52E+07±1.16E+07+ | 8.25E+05±4.54E+04+ | 7.05E+05±5.83E+04 |
+/≈/− | 13/15/1 | 15/9/5 | 5/19/5 | 24/5/0 | 27/2/0 | 19/5/5 | / |
IEEE CEC2017 with 100D | HCLPSO | SDPSO | MRFO | I-GWO | BeSD | MPSORL |
mean±std | mean±std | mean±std | mean±std | mean±std | mean±std | |
F1 | 6.74E+03±7.22E+03≈ | 7.59E+03±8.88E+03≈ | 5.59E+03±7.18E+03≈ | 1.30E+05±5.51E+04+ | 3.34E+03±2.87E+03≈ | 7.31E+03±7.60E+03 |
F3 | 7.51E+03±3.89E+03− | 2.07E+04±1.75E+04− | 8.58E+04±1.31E+04≈ | 9.01E+04±1.11E+04≈ | 3.42E+04±5.67E+03− | 8.75E+04±1.84E+04 |
F4 | 2.16E+02±3.25E+01≈ | 1.95E+02±4.18E+01− | 2.37E+02±4.09E+01≈ | 3.06E+02±3.91E+01+ | 2.81E+02±4.11E+01+ | 2.28E+02±5.13E+01 |
F5 | 2.91E+02±5.87E+01≈ | 3.05E+02±8.14E+01≈ | 7.98E+02±6.75E+01+ | 9.15E+02±3.20E+01+ | 6.46E+02±3.17E+01+ | 2.81E+02±5.72E+01 |
F6 | 2.56E−05±8.27E−05− | 4.90E−02±3.90E−02+ | 5.13E+01±6.93E+00+ | 1.93E+00±9.54E−01+ | 4.36E−01±1.80E−01+ | 8.82E−04±1.13E−03 |
F7 | 5.79E+02±7.60E+01+ | 5.43E+02±1.46E+02+ | 2.17E+03±2.71E+02+ | 1.10E+03±6.67E+01+ | 7.33E+02±4.82E+01+ | 4.22E+02±8.11E+01 |
F8 | 2.98E+02±5.97E+01≈ | 3.21E+02±6.77E+01+ | 8.83E+02±8.99E+01+ | 9.15E+02±3.05E+01+ | 6.52E+02±3.42E+01+ | 2.88E+02±6.62E+01 |
F9 | 1.55E+03±7.37E+02+ | 4.79E+03±2.78E+03+ | 2.06E+04±1.69E+03+ | 4.15E+03±3.17E+03+ | 1.91E+04±5.45E+03+ | 5.35E+02±3.20E+02 |
F10 | 1.17E+04±1.02E+03+ | 1.20E+04±7.35E+02+ | 1.41E+04±1.21E+03+ | 2.89E+04±7.52E+02+ | 1.84E+04±6.20E+02+ | 1.10E+04±8.17E+02 |
F11 | 7.57E+02±1.78E+02≈ | 9.67E+02±1.92E+02+ | 5.66E+02±1.18E+02− | 5.74E+03±1.66E+03+ | 6.27E+02±1.35E+02− | 7.10E+02±1.19E+02 |
F12 | 7.72E+05±3.83E+05− | 3.04E+05±1.00E+05− | 1.40E+06±5.88E+05+ | 4.01E+07±1.53E+07+ | 2.26E+06±7.87E+05+ | 1.03E+06±4.16E+05 |
F13 | 1.40E+03±8.97E+02+ | 1.64E+03±9.03E+02+ | 6.05E+03±8.30E+03+ | 7.10E+03±7.79E+03+ | 3.27E+03±1.64E+03+ | 9.36E+02±4.44E+02 |
F14 | 1.11E+05±3.95E+04≈ | 5.95E+04±2.98E+04− | 1.33E+05±8.46E+04≈ | 3.85E+06±1.83E+06+ | 4.78E+03±3.89E+03− | 1.43E+05±6.90E+04 |
F15 | 6.80E+02±2.92E+02+ | 5.05E+02±1.33E+02≈ | 2.34E+03±2.69E+03+ | 3.05E+03±3.30E+03+ | 5.44E+02±3.77E+02≈ | 5.34E+02±1.39E+02 |
F16 | 3.20E+03±3.53E+02+ | 2.90E+03±4.68E+02≈ | 3.95E+03±6.79E+02+ | 5.73E+03±1.58E+03+ | 3.33E+03±2.88E+02+ | 2.76E+03±4.80E+02 |
F17 | 2.41E+03±3.39E+02≈ | 2.22E+03±2.87E+02≈ | 2.96E+03±5.02E+02+ | 4.80E+03±4.04E+02+ | 2.15E+03±2.11E+02≈ | 2.23E+03±2.91E+02 |
F18 | 2.09E+05±8.99E+04− | 1.17E+05±3.31E+04− | 3.29E+05±1.21E+05≈ | 1.13E+07±7.10E+06+ | 5.71E+04±1.82E+04− | 4.13E+05±1.83E+05 |
F19 | 4.43E+02±2.87E+02+ | 3.32E+02±2.18E+02≈ | 2.93E+03±3.10E+03+ | 3.16E+03±3.87E+03+ | 9.38E+02±5.61E+02+ | 2.85E+02±1.11E+02 |
F20 | 2.25E+03±2.52E+02+ | 2.08E+03±3.21E+02+ | 3.19E+03±5.90E+02+ | 4.25E+03±3.40E+02+ | 2.43E+03±2.55E+02+ | 1.87E+03±2.71E+02 |
F21 | 5.47E+02±5.29E+01≈ | 5.32E+02±6.67E+01≈ | 9.60E+02±1.28E+02+ | 1.12E+03±3.20E+01+ | 7.73E+02±2.92E+01+ | 5.41E+02±6.73E+01 |
F22 | 1.32E+04±1.15E+03+ | 1.21E+04±4.15E+03+ | 1.68E+04±1.49E+03+ | 2.98E+04±1.01E+03+ | 2.02E+04±4.18E+02+ | 1.17E+04±3.25E+03 |
F23 | 8.03E+02±2.63E+01+ | 7.83E+02±3.24E+01≈ | 1.33E+03±1.38E+02+ | 1.38E+03±3.55E+01+ | 1.06E+03±2.63E+01+ | 7.86E+02±2.07E+01 |
F24 | 1.31E+03±5.20E+01≈ | 1.30E+03±5.71E+01≈ | 2.19E+03±2.78E+02+ | 1.76E+03±3.11E+01+ | 1.32E+03±9.25E+01≈ | 1.29E+03±6.33E+01 |
F25 | 7.38E+02±6.47E+01− | 7.78E+02±6.94E+01≈ | 7.84E+02±5.27E+01≈ | 8.61E+02±4.59E+01+ | 8.62E+02±4.13E+01+ | 7.88E+02±6.23E+01 |
F26 | 7.29E+03±6.01E+02≈ | 7.72E+03±6.71E+02+ | 1.69E+04±7.42E+03+ | 1.22E+04±5.14E+02+ | 1.23E+04±2.30E+03+ | 6.28E+03±2.76E+03 |
F27 | 7.91E+02±3.21E+01≈ | 7.73E+02±3.15E+01≈ | 1.22E+03±1.85E+02+ | 9.53E+02±1.30E+02+ | 8.53E+02±5.71E+01+ | 7.77E+02±2.78E+01 |
F28 | 5.71E+02±3.64E+01≈ | 5.65E+02±2.80E+01≈ | 5.70E+02±3.28E+01≈ | 6.69E+02±3.07E+01+ | 6.69E+02±2.81E+01+ | 5.65E+02±2.94E+01 |
F29 | 2.93E+03±2.89E+02≈ | 3.01E+03±4.44E+02≈ | 3.97E+03±5.99E+02+ | 4.90E+03±4.35E+02+ | 3.49E+03±2.48E+02+ | 3.08E+03±4.84E+02 |
F30 | 5.83E+03±2.82E+03≈ | 5.73E+03±2.78E+03≈ | 7.14E+03±3.85E+03≈ | 2.43E+05±1.07E+05+ | 1.06E+04±2.71E+03+ | 5.80E+03±3.15E+03 |
+/≈/− | 10/14/5 | 10/14/5 | 20/8/1 | 28/1/0 | 21/4/4 | / |
IEEE CEC2019 | LDWPSO | UPSO | CLPSO | LIPS | HCLPSO | MPSORL |
mean±std | mean±std | mean±std | mean±std | mean±std | mean±std | |
F31 | 5.22E+04±8.95E+04≈ | 5.71E+04±3.49E+04− | 8.62E+05±5.36E+05+ | 4.70E+04±4.30E+04≈ | 6.08E+04±1.20E+05≈ | 5.93E+04±6.88E+04 |
F32 | 2.61E+02±1.04E+02≈ | 3.23E+02±1.35E+02≈ | 6.25E+02±1.54E+02+ | 2.73E+02±9.38E+01≈ | 2.43E+02±8.17E+01≈ | 2.44E+02±1.03E+02 |
F33 | 3.95E−01±7.47E−02≈ | 4.80E−01±4.01E−01+ | 9.06E−01±3.06E−01+ | 6.22E−01±8.09E−01≈ | 3.95E−01±7.47E−02+ | 3.66E−01±1.33E−01 |
F34 | 8.52E+00±4.08E+00+ | 9.20E+00±2.93E+00+ | 4.27E+00±1.21E+00≈ | 1.05E+01±4.19E+00+ | 4.41E+00±1.47E+00≈ | 4.55E+00±1.77E+00 |
F35 | 1.05E−01±6.19E−02+ | 3.86E−02±1.90E−02≈ | 6.52E−02±2.62E−02+ | 1.00E−02±8.04E−03− | 5.13E−02±2.18E−02≈ | 3.33E−02±2.24E−02 |
F36 | 3.15E−01±5.71E−01+ | 4.36E−01±5.00E−01+ | 1.59E−01±7.46E−02+ | 4.76E−01±5.95E−01+ | 1.99E−03±2.78E−03+ | 3.89E−04±1.69E−03 |
F37 | 4.41E+02±2.38E+02+ | 4.66E+02±1.71E+02+ | 1.47E+02±7.73E+01≈ | 7.55E+02±2.49E+02+ | 2.49E+02±1.11E+02+ | 1.61E+02±1.30E+02 |
F38 | 1.99E+00±4.61E−01+ | 2.21E+00±4.28E−01+ | 2.03E+00±2.62E−01+ | 3.04E+00±4.87E−01+ | 1.65E+00±3.53E−01+ | 1.31E+00±6.48E−01 |
F39 | 1.32E−01±6.08E−02≈ | 6.99E−02±3.05E−02− | 1.75E−01±3.92E−02+ | 1.23E−01±4.73E−02≈ | 1.01E−01±3.46E−02− | 1.30E−01±3.08E−02 |
F40 | 1.42E+01±9.09E+00≈ | 1.46E+01±8.32E+00≈ | 1.88E+01±3.91E+00+ | 1.93E+01±3.65E+00≈ | 1.54E+01±8.57E+00≈ | 1.21E+01±9.91E+00 |
+/≈/− | 5/5/0 | 5/3/2 | 8/2/0 | 4/5/1 | 4/5/1 | / |
IEEE CEC2019 | EPSO | SDPSO | MRFO | I-GWO | BeSD | MPSORL |
mean±std | mean±std | mean±std | mean±std | mean±std | mean±std | |
F31 | 3.20E+04±2.87E+04≈ | 4.42E+04±5.14E+04≈ | 0.00E+00±0.00E+00− | 7.24E+04±1.35E+05≈ | 0.00E+00±0.00E+00− | 5.93E+04±6.88E+04 |
F32 | 2.30E+02±8.66E+01≈ | 2.58E+02±1.05E+02≈ | 3.70E+00±3.69E−01− | 1.06E+03±3.38E+02+ | 4.84E+00±1.97E+00− | 2.44E+02±1.03E+02 |
F33 | 3.41E−01±1.55E−01≈ | 3.55E−01±1.40E−01≈ | 3.95E−01±7.47E−02≈ | 1.95E+00±1.18E+00+ | 9.35E−01±2.89E−01+ | 3.66E−01±1.33E−01 |
F34 | 5.82E+00±1.98E+00+ | 4.48E+00±1.50E+00≈ | 2.50E+01±1.55E+01+ | 1.98E+01±4.20E+00+ | 8.50E+00±1.96E+00+ | 4.55E+00±1.77E+00 |
F35 | 8.57E−02±4.08E−02+ | 4.81E−02±3.37E−02≈ | 1.11E−01±1.07E−01+ | 5.26E−01±9.12E−02+ | 5.04E−02±1.27E−02≈ | 3.33E−02±2.24E−02 |
F36 | 2.46E−01±2.42E−01+ | 2.72E−02±1.21E−01≈ | 2.13E+00±1.70E+00+ | 1.93E−01±3.60E−01+ | 1.34E−01±1.17E−01+ | 3.89E−04±1.69E−03 |
F37 | 2.40E+02±1.47E+02≈ | 1.80E+02±1.04E+02≈ | 7.29E+02±3.16E+02+ | 7.33E+02±2.42E+02+ | 4.46E+02±1.29E+02+ | 1.61E+02±1.30E+02 |
F38 | 1.65E+00±4.69E−01+ | 1.19E+00±5.05E−01≈ | 2.45E+00±3.70E−01+ | 2.06E+00±2.53E−01+ | 2.50E+00±1.64E−01+ | 1.31E+00±6.48E−01 |
F39 | 1.27E−01±3.64E−02≈ | 1.32E−01±2.78E−02≈ | 2.05E−01±7.63E−02+ | 1.84E−01±4.15E−02+ | 1.11E−01±1.76E−02− | 1.30E−01±3.08E−02 |
F40 | 1.24E+01±9.65E+00+ | 1.22E+01±9.85E+00≈ | 1.50E+01±9.02E+00+ | 1.50E+01±9.03E+00+ | 1.76E+01±4.33E+00+ | 1.21E+01±9.91E+00 |
+/≈/− | 5/5/0 | 0/10/0 | 7/1/2 | 9/1/0 | 6/1/3 | / |
MPSORL | Non-uniform vs. Uniform status division | |||||
Wins (+) | Losses (−) | Ties | R+ | R− | p-value | |
30D | 14 | 15 | 0 | 220 | 215 | 0.957 |
50D | 19 | 10 | 0 | 278 | 157 | 0.191 |
100D | 20 | 9 | 0 | 297 | 138 | 0.086 |
Function type | No. | Function | F(x∗) | D | search range |
Bowl-Shaped | F41 | Sphere Function | 0 | 2 | [-100 100] |
Many Local Minima | F42 | Rastrigin Function | 0 | 2 | [-5.12 5.12] |
Many Local Minima | F43 | Ackley Function | 0 | 2 | [-32.768, 32.768] |
Plate-Shaped | F44 | Matyas Function | 0 | 2 | [-10 10] |