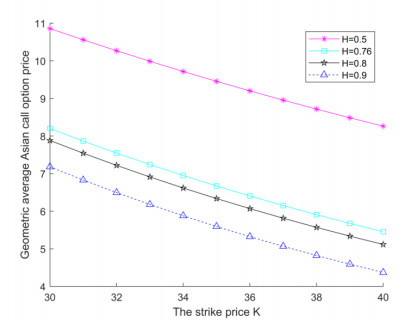
Since the beginning of settled civilizations, cities have represented places of innovation and meeting points for flows of goods, services, people, ideas, and cultural expressions. Cities as places of interaction help to develop new ideas, solutions, and applications. If the spreading of innovation in the past appeared as a spontaneous process, nowadays it is inserted into more structured business models for enterprises and companies and in development policies at central, regional, and local levels. This also involves cities, as investing in innovation can represent opportunities for their growth. Urban geography literature, for instance, examines the urban life cycle, which has evolved into what Florida terms the "New Urban Crisis". Based on the "demographic winter" facing Italy and other industrialized countries, the present work aims to observe the most recent urban dynamics in spatial and demographic changes, innovation, and digital transitions. This research combined an analysis of innovative cities in Italy, based on the innovation index (ICity Rank) with demographic data, considering metropolitan cities and their functional urban areas (FUAs), along with a set of mid-sized cities identified as "innovative" and dynamic. Cities were ranked within the urban life cycle model, employing LISA (Local Moran's I) as a method for analysis and clustering. Using spatial analytical techniques, the work focused on the Italian urban system, its capital cities and mid-size innovative cities, considering urban dynamics in terms of population change, income, and innovation, observing their characteristics and recent evolution (2019–2023). The findings highlight the formation of urban "champions" and their characteristics in terms of ability to attract people and expertise. The results show that innovative metropolitan cities are able to maintain population levels, particularly in suburban rings, while populations in the core areas tend to decline. However, certain innovative metropolitan cities in Southern Italy maintained or increased the core population. In general, medium-sized cities present more interesting dynamics, showing either population stability or a slower rate of decline.
Citation: Giuseppe Borruso, Ginevra Balletto. Cities as innovation poles in the digital transition. The Italian case[J]. AIMS Geosciences, 2024, 10(4): 918-938. doi: 10.3934/geosci.2024043
[1] | Yao Fu, Sisi Zhou, Xin Li, Feng Rao . Multi-assets Asian rainbow options pricing with stochastic interest rates obeying the Vasicek model. AIMS Mathematics, 2023, 8(5): 10685-10710. doi: 10.3934/math.2023542 |
[2] | Zhidong Guo, Xianhong Wang, Yunliang Zhang . Option pricing of geometric Asian options in a subdiffusive Brownian motion regime. AIMS Mathematics, 2020, 5(5): 5332-5343. doi: 10.3934/math.2020342 |
[3] | Kung-Chi Chen, Kuo-Shing Chen . Pricing green financial options under the mixed fractal Brownian motions with jump diffusion environment. AIMS Mathematics, 2024, 9(8): 21496-21523. doi: 10.3934/math.20241044 |
[4] | Boling Chen, Guohe Deng . Analytic approximations for European-style Asian spread options. AIMS Mathematics, 2024, 9(5): 11696-11717. doi: 10.3934/math.2024573 |
[5] | Xinyi Wang, Jingshen Wang, Zhidong Guo . Pricing equity warrants under the sub-mixed fractional Brownian motion regime with stochastic interest rate. AIMS Mathematics, 2022, 7(9): 16612-16631. doi: 10.3934/math.2022910 |
[6] | Chao Yue, Chuanhe Shen . Fractal barrier option pricing under sub-mixed fractional Brownian motion with jump processes. AIMS Mathematics, 2024, 9(11): 31010-31029. doi: 10.3934/math.20241496 |
[7] | Zhe Li, Xiao-Tian Wang . Valuation of bid and ask prices for European options under mixed fractional Brownian motion. AIMS Mathematics, 2021, 6(7): 7199-7214. doi: 10.3934/math.2021422 |
[8] | Jiajia Zhao, Zuoliang Xu . Calibration of time-dependent volatility for European options under the fractional Vasicek model. AIMS Mathematics, 2022, 7(6): 11053-11069. doi: 10.3934/math.2022617 |
[9] | Javed Hussain, Saba Shahid, Tareq Saeed . Pricing forward-start style exotic options under uncertain stock models with periodic dividends. AIMS Mathematics, 2024, 9(9): 24934-24954. doi: 10.3934/math.20241215 |
[10] | Min-Ku Lee, Jeong-Hoon Kim . Pricing vanilla, barrier, and lookback options under two-scale stochastic volatility driven by two approximate fractional Brownian motions. AIMS Mathematics, 2024, 9(9): 25545-25576. doi: 10.3934/math.20241248 |
Since the beginning of settled civilizations, cities have represented places of innovation and meeting points for flows of goods, services, people, ideas, and cultural expressions. Cities as places of interaction help to develop new ideas, solutions, and applications. If the spreading of innovation in the past appeared as a spontaneous process, nowadays it is inserted into more structured business models for enterprises and companies and in development policies at central, regional, and local levels. This also involves cities, as investing in innovation can represent opportunities for their growth. Urban geography literature, for instance, examines the urban life cycle, which has evolved into what Florida terms the "New Urban Crisis". Based on the "demographic winter" facing Italy and other industrialized countries, the present work aims to observe the most recent urban dynamics in spatial and demographic changes, innovation, and digital transitions. This research combined an analysis of innovative cities in Italy, based on the innovation index (ICity Rank) with demographic data, considering metropolitan cities and their functional urban areas (FUAs), along with a set of mid-sized cities identified as "innovative" and dynamic. Cities were ranked within the urban life cycle model, employing LISA (Local Moran's I) as a method for analysis and clustering. Using spatial analytical techniques, the work focused on the Italian urban system, its capital cities and mid-size innovative cities, considering urban dynamics in terms of population change, income, and innovation, observing their characteristics and recent evolution (2019–2023). The findings highlight the formation of urban "champions" and their characteristics in terms of ability to attract people and expertise. The results show that innovative metropolitan cities are able to maintain population levels, particularly in suburban rings, while populations in the core areas tend to decline. However, certain innovative metropolitan cities in Southern Italy maintained or increased the core population. In general, medium-sized cities present more interesting dynamics, showing either population stability or a slower rate of decline.
In 1973, the classic Black-Scholes (BS) model [1] provided an effective method for pricing financial derivatives and greatly simplifying the pricing process. Since then, many scholars have conducted research and promoted the model [2,3]. Asian options, a special type of financial derivative, are appealing due to their unique valuation method, which relies on the mean value of the underlying asset over a set contract period.
However, the BS model may not align with actual financial markets and could fail to provide accurate pricing for complex financial derivatives. In 1968, Mandelbrot and Van Ness [4] proposed a stochastic process: fractional Brownian motion (fBm). If the Hurst parameter H≠12, the fractional Brownian motion is not a semi-martingale, which implies that there must be arbitrage opportunities [5,6]. Bojdecki et al. [7] advanced that sub-fractional Brownian motion (sfBm), which not only possesses similar properties to fractional Brownian motion but also features non-stationary increments with weaker correlations on non-overlapping intervals and a faster decay of covariance, aligns better with financial market dynamics. Based on this, Tubor [8] investigated new properties of sfBm. Xu and Li [9] tackled the valuation conundrum linked to compound options. For further applications of sfBm in financial models, please refer to [10,11,12]. Despite these advancements, the use of sfBm as a stochastic driver may still result in arbitrage opportunities akin to those associated with fBm. Zhang and Xiao [13] showed that the Black-Scholes model driven by fractional Gaussian processes allows for arbitrage opportunities. Since sub-fractional Brownian motion is a more general Gaussian process and is also not a semi-martingale, the application of this model to financial markets necessitates the study of arbitrage possibilities. EI-Nouty and Zili [14] proposed the concept of mixed sub-fractional Brownian motion (msfBm), which lies between Brownian motion and sfBm. The mixed sub-fractional Brownian motion incorporates the semi-martingale condition when H∈(34,1), making this stochastic process more suitable for inclusion in option pricing models [15]. Furthermore, constructing an appropriate portfolio can enable models with the Hurst parameter H∈(0,1) to avoid arbitrage in financial markets [16]. For example, Guo et al. [17] combined the fractal option pricing model with a new intelligent algorithm to predict the implied volatility in financial assets. Cai et al. [18] found the LSE of the drift parameter of mixed sub-fractional O-U process.
The aforementioned studies collectively presupposed fixed short-term interest rates, which do not reflect the dynamic nature of real interest rates that exhibit mean reversion. The Vasicek model [19], a fundamental interest rate model in finance, describes the evolution of interest rates and has become a cornerstone in the analysis and management of interest rate risks. It provides a theoretical framework that aids in making informed financial decisions and developing sophisticated risk management strategies. Ewald et al. [20] priced options for Asian commodity futures contracts by incorporating stochastic convenience yields, stochastic interest rates, and commodity spot prices, and considered the scenario with jumps. More related studies can be found in [21,22,23,24,25] to further understand the importance of stochastic interest rate models in option pricing. Building on these studies, in this paper, a model for pricing geometric average Asian options is formulated under the msfBm regime, while the short rate follows the Vasicek process.
Then, in Section 2, we state the necessary foundational knowledge. In Section 3, we provide the formula for a zero-coupon bond under the msfBm, based on certain assumptions. In Section 4, we give the solution for valuing geometric average Asian options with fixed strike price. In Section 5, we present relevant numerical calculations and an empirical study to further explore the effects of varying parameters on the model.
The Vasicek model is recognized as a significant model for the short rate that can be combined with other pricing models. The incorporation of mixed sub-fractional Brownian motion can offer more comprehensive risk management solutions. Now, we introduce the relevant knowledge of msfBm, which is covered in [7,14,26].
Definition 2.1. The Gaussian process ξH={ξH(t),t⩾0} defined on a probability space (Ω,F,P) that satisfies the following conditions
(1) ξH(0)=0,
(2) E(ξH(t)⋅ξH(s))=s2H+t2H−12[(s+t)2H+|t−s|2H],
is called a sub-fractional Brownian motion, where H is the Hurst parameter with a value range of (0,1), and BH(t) is a standard Brownian motion when H=12.
Definition 2.2. Let (Ω,F,P) be a probability space. The mixed sub-fractional Brownian motion Mβ,γ,H={Mβ,γ,Ht,t⩾0}is a stochastic process with H∈(0,1), defined by
Mβ,γ,H=βB(t)+γξH(t),β⩾0,γ⩾0, |
where B(t) is a Brownian motion and ξH(t) is a sub-fractional Brownian motion. We have
E(Mβ,γ,Ht⋅Mβ,γ,Hs)=β2min |
where M_t^{\beta, \gamma, H} is a sfBm when \beta = 0 and \gamma = 1 , M_t^{\beta, \gamma, H} is a standard Brownian motion when \beta = 1 and \gamma = 0 or when \beta = 0 , \gamma = 1 and H = \frac{1}{2} .
Definition 2.3. Asian options are divided into geometric average Asian options and arithmetic average Asian options. Taking fixed strike Asian call options as an example, the payoff is {\left({{J_T} - K} \right)^ + } , where K is the strike price, T is the expiration date, and {J_t} is the average price of the underlying asset over the predetermined interval. In continuous time, the arithmetic average is represented by
{J_t} = \frac{1}{t}\int_0^t {{S_\tau }} {\text{d}}\tau , |
the geometric average is represented by
{J_t} = \exp \left( {\frac{1}{t}\int_0^t {\ln {S_\tau }{\text{d}}\tau } } \right). |
Then, we present some basic assumptions of this paper.
(ⅰ) Short selling is allowed without penalty; there are no taxes and friction; stocks do not pay dividends; investors borrow and lend at the risk-free rate.
(ⅱ) The stock price S\left(t \right) satisfies the mixed sub-fractional Brownian motion of risk neutrality, given by
{\text{d}}S\left( t \right) = r\left( t \right)S\left( t \right){\text{d}}t + {\sigma _{{S_1}}}S\left( t \right){\text{d}}{B^S}\left( t \right) + {\sigma _{{S_2}}}S\left( t \right){\text{d}}\xi _H^S\left( t \right), | (2.1) |
where r\left(t \right) is a short rate and satisfies the following msfBm-Vasicek model
{\text{d}}r\left( t \right) = a\left( {b - r\left( t \right)} \right){\text{d}}t + {\sigma _{{r_1}}}{\text{d}}{B^r}\left( t \right) + {\sigma _{{r_2}}}{\text{d}}\xi _H^r\left( t \right), | (2.2) |
where {\sigma _{{S_1}}} , {\sigma _{{S_2}}} , {\sigma _{{r_1}}} , and {\sigma _{{r_2}}} are constants, {B^S}\left(t \right) , \xi _H^S\left(t \right) , {B^r}\left(t \right) , and \xi _H^r\left(t \right) are independent of each other.
A zero-coupon bond is issued at a price below its face value and does not pay any interest during the period. Upon maturity at time T , investors are entitled to receive a cash return equivalent to \$ 1. The price of such a bond is influenced by the passage of time and the variability in interest rates. We denote the price of a zero-coupon bond at time t , maturing at time T , as P\left({r, t; T} \right) .
Theorem 3.1. In the mixed sub-fractional Vasicek process, the price of a zero-coupon bond with maturity T at time t \in \left[{0, T} \right] is given by
P\left( {r, t;T} \right) = {e^{ - A\left( {t, T} \right) - rB\left( {t, T} \right)}}, | (3.1) |
where
\left\{ \begin{array}{l} A\left( {t, T} \right) = b\left( {T - t} \right) - bB\left( {t, T} \right) - \frac{1}{2}\sigma _{{r_1}}^2\int_t^T {{B^2}\left( {s, T} \right){\text{d}}s} - H\left( {2 - {2^{2H - 1}}} \right)\sigma _{{r_2}}^2\int_t^T {{s^{2H - 1}}{B^2}\left( {s, T} \right){\text{d}}s} , \hfill \\ B\left( {t, T} \right) = \frac{{1 - {e^{ - a(T - t)}}}}{a}, \hfill \end{array} \right. |
Proof. By using the risk hedging formula and Itô formula [19], select two zero-coupon bonds with different maturities, denoted as {P_1} = {P_1}\left({r, t; {T_1}} \right) and {P_2} = {P_2}\left({r, t; {T_2}} \right) , to hedge the risks. Now consider a portfolio \Pi consisting of one unit of {P_1} and \Delta units of {P_2} , we can obtain
\Pi = {P_1} - \Delta {P_2}. |
The change in the portfolio over the time interval \left({t, t + {\text{d}}t} \right) is given by
{\text{d}}\Pi = \frac{{\partial {P_1}}}{{\partial t}}{\text{d}}t + \frac{{\partial {P_1}}}{{\partial r}}{\text{d}}r + \frac{1}{2}\frac{{{\partial ^2}{P_1}}}{{\partial {r^2}}}{\left( {{\text{d}}r} \right)^2} - \Delta \left( {\frac{{\partial {P_2}}}{{\partial t}}{\text{d}}t + \frac{{\partial {P_2}}}{{\partial r}}{\text{d}}r + \frac{1}{2}\frac{{{\partial ^2}{P_2}}}{{\partial {r^2}}}{{\left( {{\text{d}}r} \right)}^2}} \right), | (3.2) |
where {\left({{\text{d}}r} \right)^2} = \left({\sigma _{{r_1}}^2 + 2H{t^{2H - 1}}\left({2 - {2^{2H - 1}}} \right)\sigma _{{r_2}}^2} \right){\text{d}}t + o\left({{\text{d}}t} \right).
Let \Delta = \frac{{\partial {P_1}/\partial r}}{{\partial {P_2}/\partial r}} , Eq (3.2) becomes
\begin{array}{l} {\text{d}}\Pi = \frac{{\partial {P_1}}}{{\partial t}}{\text{d}}t + \frac{1}{2}\left( {\sigma _{{r_1}}^2 + 2H{t^{2H - 1}}\left( {2 - {2^{2H - 1}}} \right)\sigma _{{r_2}}^2} \right)\frac{{{\partial ^2}{P_1}}}{{\partial {r^2}}}{\text{d}}t \\ - \frac{{\partial {P_1}/\partial r}}{{\partial {P_2}/\partial r}}\left( {\frac{{\partial {P_2}}}{{\partial t}}{\text{d}}t + \frac{1}{2}\left( {\sigma _{{r_1}}^2 + 2H{t^{2H - 1}}\left( {2 - {2^{2H - 1}}} \right)\sigma _{{r_2}}^2} \right)\frac{{{\partial ^2}{P_2}}}{{\partial {r^2}}}{\text{d}}t} \right). \end{array} | (3.3) |
Furthermore, since the investment portfolio is risk-free, that is, E\left({{\text{d}}\Pi } \right) = r\left(t \right)\Pi {\text{d}}t , we have
\begin{array}{l} \left( {\frac{{\partial {P_1}}}{{\partial t}} + \frac{1}{2}\left( {\sigma _{{r_1}}^2 + 2H{t^{2H - 1}}\left( {2 - {2^{2H - 1}}} \right)\sigma _{{r_2}}^2} \right)\frac{{{\partial ^2}{P_1}}}{{\partial {r^2}}} - r{P_1}} \right)/\frac{{\partial {P_1}}}{{\partial r}} \hfill \\ = \left( {\frac{{\partial {P_2}}}{{\partial t}} + \frac{1}{2}\left( {\sigma _{{r_1}}^2 + 2H{t^{2H - 1}}\left( {2 - {2^{2H - 1}}} \right)\sigma _{{r_2}}^2} \right)\frac{{{\partial ^2}{P_2}}}{{\partial {r^2}}} - r{P_2}} \right)/\frac{{\partial {P_2}}}{{\partial r}}. \hfill \end{array} | (3.4) |
Then, we can obtain
\left( {\frac{{\partial P}}{{\partial t}} + \frac{1}{2}\left( {\sigma _{{r_1}}^2 + 2H{t^{2H - 1}}\left( {2 - {2^{2H - 1}}} \right)\sigma _{{r_2}}^2} \right)\frac{{{\partial ^2}P}}{{\partial {r^2}}} - rP} \right)/\frac{{\partial P}}{{\partial r}} = - a\left( {b - r\left( t \right)} \right). |
Thus, the zero-coupon bond P\left({r, t; T} \right) satisfies the following partial differential equation given by
\left\{ \begin{array}{l} \frac{{\partial P}}{{\partial t}} + a\left( {b - r\left( t \right)} \right)\frac{{\partial P}}{{\partial r}} + \frac{1}{2}\sigma _{{r_1}}^2\frac{{{\partial ^2}P}}{{\partial {r^2}}} + H{t^{2H - 1}}\left( {2 - {2^{2H - 1}}} \right)\sigma _{{r_2}}^2\frac{{{\partial ^2}P}}{{\partial {r^2}}} - rP = 0, \hfill \\ P\left( {r, T;T} \right) = 1. \hfill \end{array} \right. | (3.5) |
Given that A\left({T, T} \right) = 0 and B\left({T, T} \right) = 0 , it is not difficult to find a solution for the price of a zero-coupon bond at time t of the following form
\left\{ \begin{array}{l} \frac{{\partial P}}{{\partial t}} = P\left[ { - A'\left( {t, T} \right) - rB'\left( {t, T} \right)} \right], \hfill \\ \frac{{\partial P}}{{\partial r}} = - PB\left( {t, T} \right), \hfill \\ \frac{{{\partial ^2}P}}{{\partial {r^2}}} = P{B^2}\left( {t, T} \right). \hfill \end{array} \right. | (3.6) |
Substituting Eq (3.6) into Eq (3.5), we can derive
- r\left( {B'\left( {t, T} \right) - aB\left( {t, T} \right) + 1} \right) - A'\left( {t, T} \right) - abB\left( {t, T} \right) + \frac{1}{2}\sigma _{{r_1}}^2{B^2}\left( {t, T} \right) + H{t^{2H - 1}}\left( {2 - {2^{2H - 1}}} \right)\sigma _{{r_2}}^2{B^2}\left( {t, T} \right) = 0. |
After simplification, we have
\left\{ \begin{array}{l} B'\left( {t, T} \right) - aB\left( {t, T} \right) + 1 = 0, \hfill \\ A'\left( {t, T} \right) + abB\left( {t, T} \right) - \frac{1}{2}\sigma _{{r_1}}^2{B^2}\left( {t, T} \right) - H{t^{2H - 1}}\left( {2 - {2^{2H - 1}}} \right)\sigma _{{r_2}}^2{B^2}\left( {t, T} \right) = 0. \hfill \end{array} \right. |
Then, we can obtain
\left\{ \begin{array}{l} A\left( {t, T} \right) = b\left( {T - t} \right) - bB\left( {t, T} \right) - \frac{1}{2}\sigma _{{r_1}}^2\int_t^T {{B^2}\left( {s, T} \right){\text{d}}s} - H\left( {2 - {2^{2H - 1}}} \right)\sigma _{{r_2}}^2\int_t^T {{s^{2H - 1}}{B^2}\left( {s, T} \right){\text{d}}s} , \hfill \\ B\left( {t, T} \right) = \frac{{1 - {e^{ - a(T - t)}}}}{a}. \hfill \end{array} \right. |
Proof is completed.
In this section, examine a mixed sub-fractional version of the BS model, i.e., a simple financial market consisting of zero-coupon bonds, underlying assets, and options on underlying assets.
Theorem 4.1. The value of geometric average Asian call options with fixed strike price is denoted as V = V\left({S, J, r, t} \right) . Based on assumptions (2.1) and (2.2), the partial differential equation and boundary conditions are given by
\left\{ \begin{array}{l} \frac{{\partial V}}{{\partial t}} + \frac{J}{t}\ln \left( {\frac{S}{J}} \right)\frac{{\partial V}}{{\partial J}} + \frac{1}{2}\sigma _{{S_1}}^2{S^2}\frac{{{\partial ^2}V}}{{\partial {S^2}}} + H{t^{2H - 1}}\left( {2 - {2^{2H - 1}}} \right)\sigma _{{S_2}}^2{S^2}\frac{{{\partial ^2}V}}{{\partial {S^2}}}, \hfill \\ + \frac{1}{2}\sigma _{{r_1}}^2\frac{{{\partial ^2}V}}{{\partial {r^2}}} + H{t^{2H - 1}}\left( {2 - {2^{2H - 1}}} \right)\sigma _{{r_2}}^2\frac{{{\partial ^2}V}}{{\partial {r^2}}} + a\left( {b - r\left( t \right)} \right)\frac{{\partial V}}{{\partial r}} + rS\frac{{\partial V}}{{\partial S}} - rV = 0, \hfill \\ V\left( {S, J, r, T} \right) = {\left( {J - K} \right)^ + }. \hfill \end{array} \right. | (4.1) |
Proof. Considering that the portfolio \Pi consists of one unit option V\left({S, J, r, t} \right) , {\Delta _{1t}} units of underlying assets and {\Delta _{2t}} units of zero-coupon bonds P\left({r, t; T} \right) , the value of the portfolio at time t is given by
{\Pi _t} = {V_t} - {\Delta _{1t}}{S_t} - {\Delta _{2t}}{P_t}, | (4.2) |
Choosing the appropriate {\Delta _{1t}} and {\Delta _{2t}} makes the portfolio risk-free in \left({t, t + {\text{d}}t} \right) , we can obtain
\begin{array}{l} {\text{d}}{\Pi _t} = {\text{d}}{V_t} - {\Delta _{1t}}{\text{d}}{S_t} - {\Delta _{2t}}{\text{d}}{P_t} \hfill \\ = \left( {\frac{{\partial V}}{{\partial t}} + \frac{J}{t}\ln \left( {\frac{S}{J}} \right)\frac{{\partial V}}{{\partial J}} + \frac{1}{2}\sigma _{{S_1}}^2{S^2}\frac{{{\partial ^2}V}}{{\partial {S^2}}} + H{t^{2H - 1}}\left( {2 - {2^{2H - 1}}} \right)\sigma _{{S_2}}^2{S^2}\frac{{{\partial ^2}V}}{{\partial {S^2}}}} \right){\text{d}}t \hfill \\ + \left( {\frac{1}{2}\sigma _{{r_1}}^2\frac{{{\partial ^2}V}}{{\partial {r^2}}} + H{t^{2H - 1}}\left( {2 - {2^{2H - 1}}} \right)\sigma _{{r_2}}^2\frac{{{\partial ^2}V}}{{\partial {r^2}}}} \right){\text{d}}t + \left( {\frac{{\partial V}}{{\partial S}} - {\Delta _{1t}}} \right){\text{d}}S + \left( {\frac{{\partial V}}{{\partial r}} - {\Delta _{2t}}\frac{{\partial P}}{{\partial r}}} \right){\text{d}}r \hfill \\ - {\Delta _{2t}}\left( {\frac{{\partial P}}{{\partial t}} + \frac{1}{2}\sigma _{{r_1}}^2\frac{{{\partial ^2}P}}{{\partial {r^2}}} + H{t^{2H - 1}}\left( {2 - {2^{2H - 1}}} \right)\sigma _{{r_2}}^2\frac{{{\partial ^2}P}}{{\partial {r^2}}}} \right){\text{d}}t. \hfill \end{array} |
Let {\Delta _{1t}} = \frac{{\partial V}}{{\partial S}} and {\Delta _{2t}} = \frac{{\partial V/\partial r}}{{\partial P/\partial r}} , using the principle of no arbitrage, we have
E\left( {{\text{d}}{\Pi _t}} \right) = r\left( t \right)\Pi {\text{d}}t = r\left( {{V_t} - {\Delta _{1t}}{S_t} - {\Delta _{2t}}{P_t}} \right){\text{d}}t, | (4.3) |
we can calculate that
\left\{ \begin{array}{l} \frac{{\partial V}}{{\partial t}} + \frac{J}{t}\ln \left( {\frac{S}{J}} \right)\frac{{\partial V}}{{\partial J}} + \frac{1}{2}\sigma _{{S_1}}^2{S^2}\frac{{{\partial ^2}V}}{{\partial {S^2}}} + H{t^{2H - 1}}\left( {2 - {2^{2H - 1}}} \right)\sigma _{{S_2}}^2{S^2}\frac{{{\partial ^2}V}}{{\partial {S^2}}} \hfill \\ + \frac{1}{2}\sigma _{{r_1}}^2\frac{{{\partial ^2}V}}{{\partial {r^2}}} + H{t^{2H - 1}}\left( {2 - {2^{2H - 1}}} \right)\sigma _{{r_2}}^2\frac{{{\partial ^2}V}}{{\partial {r^2}}} + a\left( {b - r\left( t \right)} \right)\frac{{\partial V}}{{\partial r}} + rS\frac{{\partial V}}{{\partial S}} - rV = 0, \hfill \\ V\left( {S, J, r, T} \right) = {\left( {J - K} \right)^ + }. \hfill \end{array} \right. |
Proof is completed.
Theorem 4.2. Assuming that the stock price satisfies Eq (2.1), and the interest rate satisfies Eq (2.2), the price of the geometric average Asian call option at time t \in \left[{0, T} \right] with strike price K and maturity date T is given by
V\left( {S, J, r, t} \right) = P{\left( {r, t;T} \right)^{\frac{t}{T}}}{J^{\frac{t}{T}}}{S^{\frac{{T - t}}{T}}}{e^L}N\left( {{d_1}} \right) - P\left( {r, t;T} \right)KN\left( {{d_2}} \right), |
where
\left\{ \begin{array}{l} {d_1} = \frac{{\frac{{t\ln J + \left( {T - t} \right)\ln \frac{S}{{P\left( {r, t;T} \right)}}}}{T} + \int_t^T {{\beta _4}\left( s \right){\text{d}}s} + 2\int_t^T {{\beta _3}\left( s \right){\text{d}}s - \ln K} }}{{\sqrt {2\int_t^T {{\beta _3}\left( s \right){\text{d}}s} } }}, \hfill \\ {d_2} = {d_1} - \sqrt {2\int_t^T {{\beta _3}\left( s \right){\text{d}}s} } , \hfill \\ \beta _1^2\left( t \right) = \tilde \sigma _S^2 + \tilde \sigma _r^2{B^2}\left( {t, T} \right), \hfill \\ {\beta _2}\left( t \right) = - A\left( {t, T} \right) - rB\left( {t, T} \right), \hfill \\ {\beta _3}\left( t \right) = {\left( {\frac{{T - t}}{T}} \right)^2}\beta _1^2\left( t \right), \hfill \\ {\beta _4}\left( t \right) = \frac{1}{T}{\beta _2}\left( t \right) - \frac{{T - t}}{T}\beta _1^2\left( t \right), \hfill \\ L = \int_t^T {{\beta _4}\left( s \right){\text{d}}s} + \int_t^T {{\beta _3}\left( s \right){\text{d}}s} , \hfill \\ \tilde \sigma _S^2 = \frac{1}{2}\sigma _{{S_1}}^2 + H{t^{2H - 1}}\left( {2 - {2^{2H - 1}}} \right)\sigma _{{S_2}}^2, \hfill \\ \tilde \sigma _r^2 = \frac{1}{2}\sigma _{{r_1}}^2 + H{t^{2H - 1}}\left( {2 - {2^{2H - 1}}} \right)\sigma _{{r_2}}^2, \hfill \\ N(x) = \frac{1}{{\sqrt {2\pi } }}\int_{ - \infty }^x {{e^{ - \frac{{{t^2}}}{2}}}} {\text{d}}t. \hfill \end{array} \right. |
Proof. To simplify the variable-coefficient equation with three variables down to one with two variables, we perform a variable substitution. Thus, let
y = \frac{S}{{P\left( {r, t;T} \right)}}, {V_1}\left( {y, J, t} \right) = \frac{{V\left( {S, J, r, t} \right)}}{{P\left( {r, t;T} \right)}}. | (4.4) |
Sometimes, we denote P\left({r, t; T} \right) as P to simplify notation. Then, through calculation, we have
\left\{ \begin{array}{l} \frac{{\partial V}}{{\partial J}} = P\frac{{\partial {V_1}}}{{\partial J}}, \hfill \\ \frac{{\partial V}}{{\partial t}} = {V_1}\frac{{\partial P}}{{\partial t}} + P\frac{{\partial {V_1}}}{{\partial t}} - y\frac{{\partial {V_1}}}{{\partial y}}\frac{{\partial P}}{{\partial t}}, \hfill \\ \frac{{\partial V}}{{\partial r}} = {V_1}\frac{{\partial P}}{{\partial r}} - y\frac{{\partial {V_1}}}{{\partial y}}\frac{{\partial P}}{{\partial r}}, \hfill \\ \frac{{\partial V}}{{\partial S}} = \frac{{\partial {V_1}}}{{\partial y}}, \hfill \\ \frac{{{\partial ^2}V}}{{\partial {r^2}}} = {V_1}\frac{{{\partial ^2}P}}{{\partial {r^2}}} - y\frac{{\partial {V_1}}}{{\partial y}}\frac{{{\partial ^2}P}}{{\partial {r^2}}} + {y^2}\frac{{{\partial ^2}{V_1}}}{{\partial {y^2}}}\frac{1}{P}{\left( {\frac{{\partial P}}{{\partial r}}} \right)^2}, \hfill \\ \frac{{{\partial ^2}V}}{{\partial r\partial S}} = - y\frac{{{\partial ^2}{V_1}}}{{\partial {y^2}}}\frac{1}{P}\frac{{\partial P}}{{\partial r}}, \hfill \\ \frac{{{\partial ^2}V}}{{\partial {S^2}}} = \frac{1}{P}\frac{{{\partial ^2}{V_1}}}{{\partial {y^2}}}. \hfill \end{array} \right. | (4.5) |
Substituting Eq (4.5) into Eq (4.1) and organizing it, we can obtain
\begin{array}{l} \frac{{\partial {V_1}}}{{\partial t}} + \left( {\frac{1}{2}\frac{1}{{{P^2}}}\sigma _{{S_1}}^2{S^2} + \frac{1}{{{P^2}}}H{t^{2H - 1}}\left( {2 - {2^{2H - 1}}} \right)\sigma _{{S_2}}^2{S^2} + \frac{1}{2}{y^2}\frac{1}{{{P^2}}}{{\left( {\frac{{\partial P}}{{\partial r}}} \right)}^2}\sigma _{{r_1}}^2} \right)\frac{{{\partial ^2}{V_1}}}{{\partial {y^2}}} \hfill \\ + {y^2}\frac{1}{{{P^2}}}{\left( {\frac{{\partial P}}{{\partial r}}} \right)^2}\left( {2 - {2^{2H - 1}}} \right)H\sigma _{{r_2}}^2{t^{2H - 1}}\frac{{{\partial ^2}{V_1}}}{{\partial {y^2}}} + \frac{J}{t}\ln \left( {\frac{S}{J}} \right)\frac{{\partial {V_1}}}{{\partial J}} \hfill \\ - \frac{1}{P}y\left( {\frac{{\partial P}}{{\partial t}} + \frac{1}{2}\frac{{{\partial ^2}P}}{{\partial {r^2}}}\sigma _{{r_1}}^2 + H{t^{2H - 1}}\left( {2 - {2^{2H - 1}}} \right)\sigma _{{r_2}}^2\frac{{{\partial ^2}P}}{{\partial {r^2}}} + a\left( {b - r\left( t \right)} \right)\frac{{\partial P}}{{\partial r}} - \frac{{rS}}{y}} \right)\frac{{\partial {V_1}}}{{\partial y}} \hfill \\ + \frac{{{V_1}}}{P}\left( {\frac{{\partial P}}{{\partial t}} + \frac{1}{2}\frac{{{\partial ^2}P}}{{\partial {r^2}}}\sigma _{{r_1}}^2 + \frac{{{\partial ^2}P}}{{\partial {r^2}}}H{t^{2H - 1}}\left( {2 - {2^{2H - 1}}} \right)\sigma _{{r_2}}^2 + a\left( {b - r\left( t \right)} \right)\frac{{\partial P}}{{\partial r}} - rP} \right) = 0. \hfill \end{array} |
By integrating Eqs (4.4) and (3.5), we can derive
\frac{{\partial {V_1}}}{{\partial t}} + \frac{J}{t}\ln \left( {\frac{S}{J}} \right)\frac{{\partial {V_1}}}{{\partial J}} + {y^2}\beta _1^2\left( t \right)\frac{{{\partial ^2}{V_1}}}{{\partial {y^2}}} = 0, | (4.6) |
where
\left\{ \begin{array}{l} \beta _1^2\left( t \right) = \tilde \sigma _S^2 + \tilde \sigma _r^2{B^2}\left( {t, T} \right), \hfill \\ \tilde \sigma _S^2 = \frac{1}{2}\sigma _{{S_1}}^2 + H{t^{2H - 1}}\left( {2 - {2^{2H - 1}}} \right)\sigma _{{S_2}}^2, \hfill \\ \tilde \sigma _r^2 = \frac{1}{2}\sigma _{{r_1}}^2 + H{t^{2H - 1}}\left( {2 - {2^{2H - 1}}} \right)\sigma _{{r_2}}^2. \hfill \end{array} \right. |
By substituting the deformation of Eq (4.4) into Eq (4.6), the equation can be transformed into
\frac{{\partial {V_1}}}{{\partial t}} + \frac{J}{t}\left( {{\beta _2}\left( t \right) + \ln \frac{y}{J}} \right)\frac{{\partial {V_1}}}{{\partial J}} + {y^2}\beta _1^2\left( t \right)\frac{{{\partial ^2}{V_1}}}{{\partial {y^2}}} = 0, | (4.7) |
where {\beta _2}\left(t \right) = - A\left({t, T} \right) - rB\left({t, T} \right).
To simplify Eq (4.7), we can make the following variable substitution
x = \frac{{t\ln J + \left( {T - t} \right)\ln y}}{T}, {V_2}\left( {x, t} \right) = {V_1}\left( {y, J, t} \right). | (4.8) |
It can be determined that
\left\{ \begin{array}{l} \frac{{\partial {V_1}}}{{\partial J}} = \frac{t}{{TJ}}\frac{{\partial {V_2}}}{{\partial x}}, \hfill \\ \frac{{\partial {V_1}}}{{\partial t}} = \frac{{\partial {V_2}}}{{\partial t}} + \frac{{\ln J - \ln y}}{T}\frac{{\partial {V_2}}}{{\partial x}}, \hfill \\ \frac{{\partial {V_1}}}{{\partial y}} = \frac{{T - t}}{{Ty}}\frac{{\partial {V_2}}}{{\partial x}}, \hfill \\ \frac{{{\partial ^2}{V_1}}}{{\partial {y^2}}} = {\left( {\frac{{T - t}}{{Ty}}} \right)^2}\frac{{{\partial ^2}{V_2}}}{{\partial {x^2}}} - \frac{{T - t}}{{T{y^2}}}\frac{{\partial {V_2}}}{{\partial x}}. \hfill \end{array} \right. | (4.9) |
Substituting the above results into Eq (4.7), we find that
\left\{ \begin{array}{l} \frac{{\partial {V_2}}}{{\partial t}} + {\beta _3}\left( t \right)\frac{{{\partial ^2}{V_2}}}{{\partial {x^2}}} + {\beta _4}\left( t \right)\frac{{\partial {V_2}}}{{\partial x}} = 0, \hfill \\ {V_2}\left( {x, T} \right) = {\left( {{e^x} - K} \right)^ + }, \hfill \end{array} \right. | (4.10) |
where {\beta _3}\left(t \right) = {\left({\frac{{T - t}}{T}} \right)^2}\beta _1^2\left(t \right) and {\beta _4}\left(t \right) = \frac{1}{T}{\beta _2}\left(t \right) - \frac{{T - t}}{T}\beta _1^2\left(t \right) .
The last time using variable substitution to simplify Eq (4.10) into a heat conduction equation, let
{V_2}\left( {x, t} \right) = \mu \left( {\eta , \theta } \right), \eta = x + \int_t^T {{\beta _4}\left( s \right){\text{d}}s} , \theta = \int_t^T {{\beta _3}\left( s \right){\text{d}}s} . | (4.11) |
It can be deduced that
\left\{ \begin{array}{l} \frac{{\partial {V_2}}}{{\partial t}} = - {\beta _4}\left( t \right)\frac{{\partial \mu }}{{\partial \eta }} - {\beta _3}\left( t \right)\frac{{\partial \mu }}{{\partial \theta }}, \hfill \\ \frac{{\partial {V_2}}}{{\partial x}} = \frac{{\partial \mu }}{{\partial \eta }}, \hfill \\ \frac{{{\partial ^2}{V_2}}}{{\partial {x^2}}} = \frac{{{\partial ^2}\mu }}{{\partial {\eta ^2}}}. \hfill \end{array} \right. | (4.12) |
Then, Eq (4.10) can be converted to
\left\{ \begin{array}{l} \frac{{{\partial ^2}\mu }}{{\partial {\eta ^2}}} = \frac{{\partial \mu }}{{\partial \theta }}, \hfill \\ \mu \left( {\eta , T} \right) = {\left( {{e^\eta } - K} \right)^ + }. \hfill \end{array} \right. | (4.13) |
According to the heat conduction theory, the solution of this equation can be expressed as
{V_2}\left( {x, t} \right) = \mu \left( {\eta , \theta } \right) = {e^{\eta + \theta }}N\left( {{d_5}} \right) - KN\left( {{d_6}} \right) = {e^{x + L}}N\left( {{d_5}} \right) - KN\left( {{d_6}} \right), | (4.14) |
where
{d_5} = \frac{{\eta - \ln K + 2\theta }}{{\sqrt {2\theta } }} = \frac{{x + \int_t^T {{\beta _4}\left( s \right){\text{d}}s} + 2\int_t^T {{\beta _3}\left( s \right){\text{d}}s - \ln K} }}{{\sqrt {2\int_t^T {{\beta _3}\left( s \right){\text{d}}s} } }}, |
{d_6} = {d_5} - \sqrt {2\theta } = {d_5} - \sqrt {2\int_t^T {{\beta _3}\left( s \right){\text{d}}s} } , |
L = \int_t^T {{\beta _4}\left( s \right){\text{d}}s} + \int_t^T {{\beta _3}\left( s \right){\text{d}}s} . |
Substituting Eq (4.8) into Eq (4.14), we can obtain
{V_1}\left( {y, J, t} \right) = {J^{\frac{t}{T}}}{y^{\frac{{T - t}}{t}}}{e^L}N\left( {{d_3}} \right) - KN\left( {{d_4}} \right), | (4.15) |
where
{d_3} = \frac{{\frac{{t\ln J + \left( {T - t} \right)\ln y}}{T} + \int_t^T {{\beta _4}\left( s \right){\text{d}}s} + 2\int_t^T {{\beta _3}\left( s \right){\text{d}}s - \ln K} }}{{\sqrt {2\int_t^T {{\beta _3}\left( s \right){\text{d}}s} } }}, |
{d_4} = {d_3} - \sqrt {2\int_t^T {{\beta _3}\left( s \right){\text{d}}s} } . |
Combining Eqs (4.4) and (4.15), it follows that
V\left( {S, J, r, t} \right) = {V_1}\left( {\frac{S}{{P\left( {r, t;T} \right)}}, J, t} \right)P\left( {r, t;T} \right) = P{\left( {r, t;T} \right)^{\frac{t}{T}}}{J^{\frac{t}{T}}}{S^{\frac{{T - t}}{T}}}{e^L}N\left( {{d_1}} \right) - P\left( {r, t;T} \right)KN\left( {{d_2}} \right), |
where
{d_1} = \frac{{\frac{{t\ln J + \left( {T - t} \right)\ln \frac{S}{{P\left( {r, t;T} \right)}}}}{T} + \int_t^T {{\beta _4}\left( s \right){\text{d}}s} + 2\int_t^T {{\beta _3}\left( s \right){\text{d}}s - \ln K} }}{{\sqrt {2\int_t^T {{\beta _3}\left( s \right){\text{d}}s} } }}, |
{d_2} = {d_1} - \sqrt {2\int_t^T {{\beta _3}\left( s \right){\text{d}}s} } . |
Proof is completed.
Theorem 4.3. The call-put parity relationship for geometric average Asian options with fixed strike price is given by
V\left( {S, J, r, t} \right) - p\left( {S, J, r, t} \right) = P\left( {r, t;T} \right){J^{\frac{t}{T}}}{\left( {\frac{S}{{P\left( {r, t;T} \right)}}} \right)^{\frac{{T - t}}{T}}}{e^L} - P\left( {r, t;T} \right)K, |
where p\left({S, J, r, t} \right) is the price of geometric average Asian put options and
\left\{ \begin{array}{l} \beta _1^2\left( t \right) = \tilde \sigma _S^2 + \tilde \sigma _r^2{B^2}\left( {t, T} \right), \hfill \\ {\beta _2}\left( t \right) = - A\left( {t, T} \right) - rB\left( {t, T} \right), \hfill \\ {\beta _3}\left( t \right) = {\left( {\frac{{T - t}}{T}} \right)^2}\beta _1^2\left( t \right), \hfill \\ {\beta _4}\left( t \right) = \frac{1}{T}{\beta _2}\left( t \right) - \frac{{T - t}}{T}\beta _1^2\left( t \right), \hfill \\ L = \int_t^T {{\beta _4}\left( s \right){\text{d}}s} + \int_t^T {{\beta _3}\left( s \right){\text{d}}s} , \hfill \\ \tilde \sigma _S^2 = \frac{1}{2}\sigma _{{S_1}}^2 + H{t^{2H - 1}}\left( {2 - {2^{2H - 1}}} \right)\sigma _{{S_2}}^2, \hfill \\ \tilde \sigma _r^2 = \frac{1}{2}\sigma _{{r_1}}^2 + H{t^{2H - 1}}\left( {2 - {2^{2H - 1}}} \right)\sigma _{{r_2}}^2. \hfill \end{array} \right. |
Proof. Let
W\left( {S, J, r, t} \right) = V\left( {S, J, r, t} \right) - p\left( {S, J, r, t} \right). |
According to Theorem 4.1, W\left({S, J, r, t} \right) satisfies the following definite solution problem
\left\{ \begin{array}{l} \frac{{\partial W}}{{\partial t}} + \frac{J}{t}\ln \left( {\frac{S}{J}} \right)\frac{{\partial W}}{{\partial J}} + \frac{1}{2}\sigma _{{S_1}}^2{S^2}\frac{{{\partial ^2}W}}{{\partial {S^2}}} + H{t^{2H - 1}}\left( {2 - {2^{2H - 1}}} \right)\sigma _{{S_2}}^2{S^2}\frac{{{\partial ^2}W}}{{\partial {S^2}}} \hfill \\ + \frac{1}{2}\sigma _{{r_1}}^2\frac{{{\partial ^2}W}}{{\partial {r^2}}} + H{t^{2H - 1}}\left( {2 - {2^{2H - 1}}} \right)\sigma _{{r_2}}^2\frac{{{\partial ^2}W}}{{\partial {r^2}}} + a\left( {b - r\left( t \right)} \right)\frac{{\partial W}}{{\partial r}} + rS\frac{{\partial W}}{{\partial S}} - rW = 0, \hfill \\ W\left( {S, J, r, T} \right) = J - K. \hfill \end{array} \right. | (4.16) |
Making the following variable substitutions
y = \frac{S}{{P\left( {r, t;T} \right)}}, {W_1}\left( {y, J, t} \right) = \frac{{W\left( {S, J, r, t} \right)}}{{P\left( {r, t;T} \right)}}. | (4.17) |
Substituting Eq (4.17) into Eq (4.16), we have
\frac{{\partial {W_1}}}{{\partial t}} + \frac{J}{t}\ln \left( {\frac{S}{J}} \right)\frac{{\partial {W_1}}}{{\partial J}} + {y^2}\beta _1^2\left( t \right)\frac{{{\partial ^2}{W_1}}}{{\partial {y^2}}} = 0, | (4.18) |
where
\left\{ \begin{array}{l} \beta _1^2\left( t \right) = \tilde \sigma _S^2 + \tilde \sigma _r^2{B^2}\left( {t, T} \right), \hfill \\ \tilde \sigma _S^2 = \frac{1}{2}\sigma _{{S_1}}^2 + H{t^{2H - 1}}\left( {2 - {2^{2H - 1}}} \right)\sigma _{{S_2}}^2, \hfill \\ \tilde \sigma _r^2 = \frac{1}{2}\sigma _{{r_1}}^2 + H{t^{2H - 1}}\left( {2 - {2^{2H - 1}}} \right)\sigma _{{r_2}}^2. \hfill \end{array} \right. |
Let
\xi = \frac{{t\ln J + \left( {T - t} \right)\ln y}}{T}, {W_2}\left( {\xi , t} \right) = {W_1}\left( {y, J, t} \right). | (4.19) |
By inserting Eq (4.19) into Eq (4.18), this yields
\left\{ \begin{array}{l} \frac{{\partial {W_2}}}{{\partial t}} + {\beta _3}\left( t \right)\frac{{{\partial ^2}{W_2}}}{{\partial {\xi ^2}}} + {\beta _4}\left( t \right)\frac{{\partial {W_2}}}{{\partial \xi }} = 0, \hfill \\ {W_2}\left( {\xi , T} \right) = {e^\xi } - K, \hfill \end{array} \right. | (4.20) |
where
{\beta _2}\left( t \right) = - A\left( {t, T} \right) - rB\left( {t, T} \right), |
{\beta _3}\left( t \right) = {\left( {\frac{{T - t}}{T}} \right)^2}\beta _1^2\left( t \right), |
{\beta _4}\left( t \right) = \frac{1}{T}{\beta _2}\left( t \right) - \frac{{T - t}}{T}\beta _1^2\left( t \right). |
By letting
{W_2}\left( {\xi , t} \right) = a\left( t \right){e^\xi } - b\left( t \right)K, | (4.21) |
then, we can obtain
\left\{ \begin{array}{l} \frac{{\partial {W_2}}}{{\partial t}} = a'\left( t \right){e^\xi } - b'\left( t \right)K, \hfill \\ \frac{{\partial {W_2}}}{{\partial \xi }} = a\left( t \right){e^\xi }, \hfill \\ \frac{{{\partial ^2}{W_2}}}{{\partial {\xi ^2}}} = a\left( t \right){e^\xi }, \hfill \end{array} \right. |
and
\left( {a'\left( t \right) + {\beta _3}\left( t \right)a\left( t \right) + {\beta _4}\left( t \right)a\left( t \right)} \right){e^\xi } - b'\left( t \right)K = 0. | (4.22) |
To solve Eq (4.22), we select appropriate a\left(t \right) and b\left(t \right) so that
\left\{ \begin{array}{l} a'\left( t \right) + {\beta _3}\left( t \right)a\left( t \right) + {\beta _4}\left( t \right)a\left( t \right) = 0, \hfill \\ b'\left( t \right) = 0, \hfill \\ a\left( T \right) = 1, \hfill \\ b\left( T \right) = 1. \hfill \end{array} \right. |
Thus, we can derive
a\left( t \right) = {e^L}, b\left( t \right) = 1, | (4.23) |
where
L = \int_t^T {{\beta _4}\left( s \right){\text{d}}s} + \int_t^T {{\beta _3}\left( s \right){\text{d}}s} . |
Substituting Eqs (4.21) and (4.23) into Eq (4.17), we have
V\left( {S, J, r, t} \right) - p\left( {S, J, r, t} \right) = P\left( {r, t;T} \right){W_1}\left( {y, J, t} \right) = P\left( {r, t;T} \right){J^{\frac{t}{T}}}{\left( {\frac{S}{{P\left( {r, t;T} \right)}}} \right)^{\frac{{T - t}}{T}}}{e^L} - P\left( {r, t;T} \right)K. |
Proof is completed.
Corollary 4.1. Let the price of the arithmetic average Asian call options with fixed strike price be denoted as \hat V\left({S, J, r, t} \right) , and let the price of the put options be \hat p\left({S, J, r, t} \right) . The put-call parity formula for arithmetic average Asian options is
\hat V\left( {S, J, r, t} \right) - \hat p\left( {S, J, r, t} \right) = P\left( {r, t;T} \right)\left( {\frac{{tJ}}{T} - K} \right) + \frac{S}{T}\int_t^T {{e^{ - A\left( {s, T} \right) - rB\left( {s, T} \right)}}} {\text{d}}s, |
where
\left\{ \begin{array}{l} A\left( {t, T} \right) = b\left( {T - t} \right) - bB\left( {t, T} \right) - \frac{1}{2}\sigma _{{r_1}}^2\int_t^T {{B^2}\left( {s, T} \right){\text{d}}s} - H\left( {2 - {2^{2H - 1}}} \right)\sigma _{{r_2}}^2\int_t^T {{s^{2H - 1}}{B^2}\left( {s, T} \right){\text{d}}s} , \hfill \\ B\left( {t, T} \right) = \frac{{1 - {e^{ - a(T - t)}}}}{a}. \hfill \end{array} \right. |
Proof. Denoting
W\left( {S, J, r, t} \right) = \hat V\left( {S, J, r, t} \right) - \hat p\left( {S, J, r, t} \right). |
According to Theorem 4.1 and Theorem 4.3, we can similarly derive
\left\{ \begin{array}{l} \frac{{\partial W}}{{\partial t}} + \frac{{S - J}}{t}\frac{{\partial W}}{{\partial J}} + \frac{1}{2}\sigma _{{S_1}}^2{S^2}\frac{{{\partial ^2}W}}{{\partial {S^2}}} + H{t^{2H - 1}}\left( {2 - {2^{2H - 1}}} \right)\sigma _{{S_2}}^2{S^2}\frac{{{\partial ^2}W}}{{\partial {S^2}}} \hfill \\ + \frac{1}{2}\sigma _{{r_1}}^2\frac{{{\partial ^2}W}}{{\partial {r^2}}} + H{t^{2H - 1}}\left( {2 - {2^{2H - 1}}} \right)\sigma _{{r_2}}^2\frac{{{\partial ^2}W}}{{\partial {r^2}}} + a\left( {b - r\left( t \right)} \right)\frac{{\partial W}}{{\partial r}} + rS\frac{{\partial W}}{{\partial S}} - rW = 0, \hfill \\ W\left( {S, J, r, T} \right) = J - K. \hfill \end{array} \right. | (4.24) |
By employing a change of variables, we define
y = \frac{S}{{P\left( {r, t;T} \right)}}, {W_1}\left( {y, J, t} \right) = \frac{{W\left( {S, J, r, t} \right)}}{{P\left( {r, t;T} \right)}}. | (4.25) |
Substituting Eq (4.25) into Eq (4.24), we can obtain
\frac{{\partial {W_1}}}{{\partial t}} + \frac{{yP - J}}{t}\frac{{\partial {W_1}}}{{\partial J}} + {y^2}\beta _1^2\left( t \right)\frac{{{\partial ^2}{W_1}}}{{\partial {y^2}}} = 0, | (4.26) |
where
\left\{ \begin{array}{l} \beta _1^2\left( t \right) = \tilde \sigma _S^2 + \tilde \sigma _r^2{B^2}\left( {t, T} \right), \hfill \\ \tilde \sigma _S^2 = \frac{1}{2}\sigma _{{S_1}}^2 + H{t^{2H - 1}}\left( {2 - {2^{2H - 1}}} \right)\sigma _{{S_2}}^2, \hfill \\ \tilde \sigma _r^2 = \frac{1}{2}\sigma _{{r_1}}^2 + H{t^{2H - 1}}\left( {2 - {2^{2H - 1}}} \right)\sigma _{{r_2}}^2. \hfill \end{array} \right. |
Let
\xi = \frac{{TK - tJ}}{y}, {W_2}\left( {\xi , t} \right) = \frac{T}{y}{W_1}\left( {y, J, t} \right). | (4.27) |
By substituting Eq (4.27) into Eq (4.26), we can deduce
\left\{ \begin{array}{l} \frac{{\partial {W_2}}}{{\partial t}} + \beta _1^2\left( t \right)\frac{{{\partial ^2}{W_2}}}{{\partial {\xi ^2}}}{\xi ^2} - P\frac{{\partial {W_2}}}{{\partial \xi }} = 0, \hfill \\ {W_2}\left( {\xi , T} \right) = - \xi . \hfill \end{array} \right. | (4.28) |
Let
{W_2}\left( {\xi , t} \right) = a\left( t \right)\xi + b\left( t \right), | (4.29) |
then, we have
\left\{ \begin{array}{l} \frac{{\partial {W_2}}}{{\partial t}} = a'\left( t \right)\xi + b'\left( t \right), \hfill \\ \frac{{\partial {W_2}}}{{\partial \xi }} = a\left( t \right), \hfill \\ \frac{{{\partial ^2}{W_2}}}{{\partial {\xi ^2}}} = 0, \hfill \end{array} \right. |
and
a'\left( t \right)\xi + b'\left( t \right) - a\left( t \right)P = 0. | (4.30) |
By combining Eqs (4.28) and (4.30), we can compare the coefficients to obtain
\left\{ \begin{array}{l} a'\left( t \right) = 0, \hfill \\ b'\left( t \right) - a\left( t \right)P = 0, \hfill \\ a\left( T \right) = - 1, \hfill \\ b\left( T \right) = 0. \hfill \end{array} \right. |
Solving the aforementioned system of equations yields
a(t) = - 1, b(t) = \int_t^T {{e^{ - A\left( {s, T} \right) - rB\left( {s, T} \right)}}} {\text{d}}s. |
Finally, we can derive
W\left( {S, J, r, t} \right) = \hat V\left( {S, J, r, t} \right) - \hat p\left( {S, J, r, t} \right) = P\left( {r, t;T} \right)\left( {\frac{{tJ}}{T} - K} \right) + \frac{S}{T}\int_t^T {{e^{ - A\left( {s, T} \right) - rB\left( {s, T} \right)}}} {\text{d}}s. |
In this section, we provide specific values for each parameter to conduct the following numerical calculations.
t = 0, S = 30, {\sigma _{{S_1}}} = 0.5, {\sigma _{{S_2}}} = 0.4, {\sigma _{{r_1}}} = 0.3, {\sigma _{{r_2}}} = 0.2, a = 2, b = 0.05, r = 0.06, T = 1. |
From Figure 1, it can be observed that when H = 0.5 , the msfBm simplifies to a geometric Brownian motion. At this point, the price of geometric average Asian call options peaks, whereas it reaches its minimum at H = 0.9 . An increment in the Hurst index is indicative of the manifestation of long-range correlations in the asset price, suggesting a persistent price trend. In specific situations, the expected small fluctuations in the asset price in the market will lead to a decrease in the option value. Figure 2 indicates that as the short rate rises, the price of the options gradually increases. This is because an increase in the interest rate could potentially raise the payoff of the options at maturity, thereby enhancing the holding value of the options. Figure 3 shows that the valuation of the options is positively influenced by a rise in the initial stock price. From Figure 4, the price of the options increases with the extension of the expiration date. As the remaining time for the option contract increases, it gives the holders more time to wait for a potential rise in the stock price. Therefore, the possibility of the investors making a profit is greater, and the price of the options will also increase accordingly. Conversely, it diminishes with an upsurge in the strike price. As the strike price increases, buyers have to pay a higher price to exercise the options, which compresses the profit margin and thus reduces the value of the options. Figure 5 illustrates that the pricing of options under the current model closely mirrors that of the sub-fractional Vasicek model. Notably, the pricing discrepancy for options between our model and Vasicek model widens initially and then narrows, and the pricing gap between our model and BS model exhibits a consistent upward trend.
According to Tables 1 and 2, it can be observed that the lowest pricing for geometric average Asian call options is associated with the BS model. In the case of a brief expiration period, the price of the options under the sfBm-Vasicek model marginally surpasses that of our model, especially with an increasing stock price, and there is minimal variation between the two models. For longer expiration dates, the option price under our model exceeds that under the sfBm-Vasicek model, with the price difference becoming more pronounced. Moreover, the price of options under our model and the Vasicek model are getting closer. Overall, our model demonstrates a degree of rationality and is deemed suitable for developing option pricing models in financial markets.
S | Our price | {V_{{\text{BS}}}} | {V_{{\text{Vasicek}}}} | {V_{{\text{sfBm - Vasicek}}}} |
20 | 0.2435 | 0.0005 | 1.8389 | 0.2208 |
23 | 0.5498 | 0.0066 | 2.8183 | 0.5202 |
26 | 1.0480 | 0.0426 | 3.9897 | 1.0211 |
29 | 1.7685 | 0.1748 | 5.3360 | 1.7589 |
32 | 2.7255 | 0.5146 | 6.8397 | 2.7506 |
35 | 3.9194 | 1.1863 | 8.4843 | 3.9968 |
38 | 5.3398 | 2.2779 | 10.2543 | 5.4858 |
41 | 6.9698 | 3.8119 | 12.1360 | 7.1977 |
44 | 8.7884 | 5.7485 | 14.1169 | 9.1087 |
47 | 10.7735 | 8.0118 | 16.1861 | 11.1933 |
50 | 12.9033 | 10.5160 | 18.3337 | 13.4270 |
S | Our price | {V_{{\text{BS}}}} | {V_{{\text{Vasicek}}}} | {V_{{\text{sfBm - Vasicek}}}} |
20 | 5.1411 | 0.2085 | 2.3002 | 0.9705 |
23 | 7.4411 | 0.4863 | 4.8981 | 2.7039 |
26 | 9.8921 | 0.9501 | 7.6053 | 4.5926 |
29 | 12.4656 | 1.6332 | 10.3988 | 6.6067 |
32 | 15.1403 | 2.5519 | 13.2620 | 8.7238 |
35 | 17.8998 | 3.7072 | 16.1825 | 10.9266 |
38 | 20.7312 | 5.0881 | 19.1509 | 13.2019 |
41 | 23.6243 | 6.6762 | 22.1600 | 15.5389 |
44 | 26.5707 | 8.4490 | 25.2039 | 17.9291 |
47 | 29.5636 | 10.3825 | 28.2781 | 20.3655 |
50 | 32.5974 | 12.4535 | 31.3787 | 22.8423 |
We select copper options for three-month futures as the sample, with a cutoff time of October 2006, and the data are sourced from the London Metal Exchange. Since all trading products on the LME are priced in US dollars, we adopt the interest rate of one-year US Treasury bonds.
We calculate the value of H using the R/S method. Define a return sequence \left\{ {{R_t}, {R_t} = \frac{{\ln {P_{t + 1}}}}{{\ln {P_t}}}} \right\} of length N and divide it into A consecutive sub-intervals of length n . Label each sub-interval as {I_a}, a = 1, \cdots, A. Thus, each point in {I_a} can be represented as
{R_{k, a}}, k = 1, \cdots , n;a = 1, \cdots , A. |
For each sub-interval {I_a} of length n , calculate its mean as
{e_a} = \frac{1}{n}\sum\limits_{k = 1}^n {{R_{k, a}}} . |
The cumulative mean deviation {X_{k, a}} for a single sub-interval is calculated as
{X_{k, a}} = \sum\limits_{i = 1}^k {\left( {{R_{i, a}} - {e_a}} \right)} , k = 1, 2, \cdots , n. |
The sum of the cumulative mean deviation sequence \left\{ {{X_{1, a}}, {X_{2, a}}, \cdots {X_{n, a}}} \right\} for a single sub-interval is zero. The range of an individual sub-interval is defined as
{R_{{I_a}}} = \mathop {\max }\limits_k \left( {{X_{k, a}}} \right) - \mathop {\min }\limits_k \left( {{X_{k, a}}} \right), k = 1, 2, \cdots , n. |
Subsequently, the standard deviation {S_{{I_a}}} for each sub-interval is given by
{S_{{I_a}}} = \sqrt {\frac{1}{n}\sum\limits_{k = 1}^n {{{\left( {{E_{k, a}} - {e_a}} \right)}^2}} } . |
Therefore, for the partition length n , we can compute the average rescaled range for A sub-intervals as
{\left( {R/S} \right)_n} = \frac{1}{A}\sum\limits_{a = 1}^A {\left( {\frac{{{R_{{I_a}}}}}{{{S_{{I_a}}}}}} \right)} . |
Repeat the above calculation process for different partition lengths (i.e., different time scales) n to obtain multiple average rescaled range values. There is a linear relationship between \log \left({\frac{R}{S}} \right) and \log \left(n \right) [27]
\log {\left( {\frac{R}{S}} \right)_n} = a + H\log \left( n \right). |
Finally, a double logarithmic regression is performed on n and R/S, and the slope is the parameter of long-range correlations, that is, the Hurst index. Therefore, we can obtain H = 0.6 .
From Table 3, it is evident that the RMSE of our model is the smallest, indicating that the option price derived from our model is the closest to the market price. Furthermore, the price from our model is also quite close to that of the sfBm-Vasicek model. This suggests that it is essential to include factors such as the long-range correlations of the underlying asset and stochastic interest rate in the option pricing model, as they significantly influence the option price.
K | Market price | Our price | {V_{{\text{BS}}}} | {V_{{\text{Vasicek}}}} | {V_{{\text{sfBm - Vasicek}}}} |
7800 | 750 | 750.8065 | 747.9587 | 752.3958 | 749.0275 |
7500 | 694 | 693.9719 | 691.9232 | 696.2100 | 692.5075 |
7850 | 737 | 737.8847 | 734.8516 | 739.3860 | 736.1443 |
7900 | 742 | 741.1881 | 739.7236 | 744.2764 | 739.8953 |
7550 | 670 | 669.3538 | 667.6885 | 672.1255 | 668.1860 |
7650 | 658 | 658.1121 | 655.5799 | 660.1722 | 656.8193 |
7700 | 630 | 629.6739 | 627.5145 | 632.1150 | 628.5006 |
7300 | 584 | 584.4501 | 581.6303 | 586.0389 | 583.1820 |
7600 | 572 | 572.5130 | 569.3085 | 574.0554 | 571.3721 |
7450 | 541 | 540.6392 | 538.3216 | 542.9253 | 539.7519 |
7400 | 508 | 509.9819 | 505.4722 | 510.0512 | 508.4733 |
RMSE | 0.8064 | 2.3756 | 2.1638 | 1.2832 |
Geometric average Asian options are important exotic options. The mixed sub-fractional Brownian motion, chosen as a stochastic process, is capable of more accurately depicting the characteristics of long-range correlations. Since the Vasicek model is a very classical model, it is combined with the msfBm to simultaneously address the pricing issue. By using partial differential equations and multiple variable substitution methods, the valuation equations for the model are deduced. Finally, numerical computations are utilized to assess the effects of diverse parameters. The efficacy of our model is demonstrated through a comparative analysis of the pricing discrepancies for options between different models, which provides a reference for option pricing theory and practice.
Xinyi Wang: Conceptualization, Methodology, Writing—original draft, Writing—review & editing, Funding acquisition; Chunyu Wang: Writing—review & editing. All authors have read and approved the final version of the manuscript for publication.
This work was supported by the General Project of Philosophy and Social Science Research in Colleges and Universities of Jiangsu Province (2023SJYB1714).
The authors declare no conflicts of interest.
[1] |
Saleh R, Brem A (2023) Creativity for sustainability: An integrative literature review. J Cleaner Prod 388: 135848. https://doi.org/10.1016/j.jclepro.2023.135848 doi: 10.1016/j.jclepro.2023.135848
![]() |
[2] |
Cohen B (2006) Urbanization in developing countries: Current trends, future projections, and key challenges for sustainability. Technol Soc 28: 63–80. https://doi.org/10.1016/j.techsoc.2005.10.005 doi: 10.1016/j.techsoc.2005.10.005
![]() |
[3] |
Sato Y, Yamamoto K (2005) Population concentration, urbanization, and demographic transition. J Urban Econ 58: 45–61. https://doi.org/10.1016/j.jue.2005.01.004 doi: 10.1016/j.jue.2005.01.004
![]() |
[4] |
Cividino S, Halbac-Cotoara-Zamfir R, Salvati L (2020) Revisiting the "City Life Cycle": Global Urbanization and Implications for Regional Development. Sustainability 12: 1151. https://doi.org/10.3390/su12031151 doi: 10.3390/su12031151
![]() |
[5] | McCann P (2017) Urban futures, population ageing and demographic decline. Camb J Reg Econ Soc 10: 543–557. |
[6] |
Alonso W (1960) A Theory of The Urban Land Market. Pap Reg Sci 6: 149–157. https://doi.org/10.1111/j.1435-5597.1960.tb01710.x doi: 10.1111/j.1435-5597.1960.tb01710.x
![]() |
[7] | Van den Berg L, Roy Drewett R, Klaassen LH (1982) A Study of Growth and Decline. Urban Europe. Vol. 1, Pergamon Press. |
[8] |
Koomen E, Van Bemmel MS, Van Huijstee J, et al. (2023) An integrated global model of local urban development and population change. Comput Environ Urban Syst 100: 101935. https://doi.org/10.1016/j.compenvurbsys.2022.101935 doi: 10.1016/j.compenvurbsys.2022.101935
![]() |
[9] |
Seyedabadi MR, Eicker U (2023) A critical review of urban scale life cycle assessment of the built environment. Sustainability Anal Model 3: 100026. https://doi.org/10.1016/j.samod.2023.100026 doi: 10.1016/j.samod.2023.100026
![]() |
[10] |
Dyson T (2011) The role of the demographic transition in the process of urbanization. Popul Dev Rev 37: 34–54. https://doi.org/10.1111/j.1728-4457.2011.00377.x doi: 10.1111/j.1728-4457.2011.00377.x
![]() |
[11] | Florida R (2017) The new urban crisis: How our cities are increasing inequality, deepening segregation, and failing the middle class-and what we can do about it. London: Hachette UK |
[12] |
Egidi G, Cividino S, Vinci S, et al. (2020) Towards Local Forms of Sprawl: A Brief Reflection on Mediterranean Urbanization. Sustainability 12: 582. https://doi.org/10.3390/su12020582 doi: 10.3390/su12020582
![]() |
[13] |
Henderson JV, Venables AJ (2009) The dynamics of city formation. Rev Econ Dyn 12: 233–254. https://doi.org/10.1016/j.red.2008.06.003 doi: 10.1016/j.red.2008.06.003
![]() |
[14] | Cuthbert AR (2008) The form of cities: Political economy and urban design. John Wiley & Sons. https://doi.org/10.1002/9780470774915 |
[15] |
Borruso G, Balletto G (2022) The image of the smart city: New challenges. Urban Sci 6: 5. https://doi.org/10.3390/urbansci6010005 doi: 10.3390/urbansci6010005
![]() |
[16] | Bezrukikh VA, Kuznetsova OA, Ligaeva NA, et al. (2018). Current systems of protected areas integrated with urban agglomerations. In IOP Conference Series: Materials Science and Engineering 463: 042055. https://doi.org/10.1088/1757-899X/463/4/042055 |
[17] | OECD European Commission (2020) A new perspective on urbanization, OECD European Commission. Available from: https://www.oecd.org/publications/cities-in-the-world-d0efcbda-en.htm |
[18] |
Schiavina M, Melchiorri M, Freire S, et al. (2022) Land use efficiency of functional urban areas: Global pattern and evolution of development trajectories Habitat Int 123: 102543. https://doi.org/10.1016/j.habitatint.2022.102543 doi: 10.1016/j.habitatint.2022.102543
![]() |
[19] | Sestini A (1949) Le regioni italiane come base geografica della struttura dello stato, In: AA.VV., Atti del XIV Congresso Geografico Italiano (Bologna 8–12 aprile 1947), Bologna, Zanichelli: 128–143. |
[20] | Gambi L (1963) L'equivoco fra compartimenti statistici e regioni costituzionali, Faenza: Lega. |
[21] | Cori B (2006) Geografia Urbana, Utet: Torino. |
[22] | Memoli M, Governa F (2011) Geografia dell'Urbano. Spazi, politiche, pratiche della città, Roma: Carocci. |
[23] | Donolo C (2012) Sul governo possibile delle città, In: Dematteis G. (ed), Le grandi città italiane. Società e territori da ricomporre, Venezia: Marsilio, 175–206. |
[24] | Castelnovi M (2013) Il riordino territoriale dello Stato. Riflessioni e proposte della geografia italiana, Roma: Società Geografica Italiana. |
[25] | Dini F, Zilli S (2015), Il riordino territoriale dello Stato. Roma: Società Geografica Italiana. |
[26] | Benetazzo C (2019) Le Province a cinque anni dalla legge "Delrio": profili partecipativi e funzionali organizzativi. Federalismi.it 5: 2–49. |
[27] | Dini F, Zilli S (2019) Neo centralismo e territorio fra Città metropolitane, aree vaste e intercomunalità. Introduzione, In: Salvatori F. (ed.), L'apporto della Geografia fra rivoluzioni e riforme, Roma: AGeI, 2213–2218. |
[28] | Scaramellini G (1990) Funzioni centrali, funzioni metropolitane, reti urbane, Milano: Franco Angeli. |
[29] |
Salone C, Governa F (2005) Italy and European spatial policies: polycentrism, urban networks and local innovation practices. Eur Plann Stud 13: 265–283. https://doi.org/10.1080/0965431042000321820 doi: 10.1080/0965431042000321820
![]() |
[30] | Crisci M, Gemmiti R, Proietti E, et al. (2014) Urban sprawl e shrinking cities in Italia. Trasformazione urbana e redistribuzione della popolazione nelle aree metropolitane, IRES, CNR. |
[31] | Turri E (2000) La megalopoli padana, Marsilio, Padova. |
[32] | OECD (2012) Redefining "Urban": A New Way to Measure Metropolitan Areas, Paris: OECD Publishing. https://doi.org/10.1787/9789264174108-en. |
[33] | Dijkstra L, Poelman H, Veneri P (2019) The EU-OECD definition of a functional urban area OECD Regional Development Working Papers, 11, Paris: OECD Publishing. https://doi.org/10.1787/d58cb34d-en. |
[34] | ISTAT Demografia in cifre, Popolazione residente per sesso, età e stato civile al 1° gennaio 2023, 2024. Available from: https://demo.istat.it/app/?i = POS & l = it. |
[35] | ISTAT, Demografia in cifre, Popolazione residente per sesso, età e stato civile al 1° gennaio 2019, 2020. Available from: https://demo.istat.it/app/?l = it & a = 2019 & i = POS |
[36] | ISTAT, BASI TERRITORIALI E VARIABILI CENSUARIE, 2024. Available from: https://www.istat.it/it/archivio/104317 |
[37] | ForumPA (2019) ICity Rank – Rapporto Annuale 2019, ForumPA. Available from: https://profilo.forumpa.it/doc/?file = 2019/i_city_rank_2019.pdf |
[38] |
Moreno-Monroy AI, Schiavina M, Veneri P (2021) Metropolitan areas in the world. Delineation and population trends J Urban Econ 125: 103242. https://doi.org/10.1016/j.jue.2020.103242 doi: 10.1016/j.jue.2020.103242
![]() |
[39] | Tobler WR (1970) A Computer Movie Simulating Urban Growth in the Detroit Region. Econ Geogr 46: 234–240. |
[40] | Murgante B, Scorza F (2023) Autocorrelazione Spaziale e Pianificazione del Territorio: Principli ed Applicazioni, Libria: Melfi. |
[41] |
Ceci M, Corizzo R, Malerba D, et al. (2019) Spatial autocorrelation and entropy for renewable energy forecasting. Data Min Knowl Disc 33: 698–729. https://doi.org/10.1007/s10618-018-0605-7 doi: 10.1007/s10618-018-0605-7
![]() |
[42] |
Luo Q, Griffith DA, Wu H (2019) Spatial autocorrelation for massive spatial data: verification of efficiency and statistical power asymptotics J Geogr Syst 21: 237–269. https://doi.org/10.1007/s10109-019-00293-3 doi: 10.1007/s10109-019-00293-3
![]() |
[43] | O' Sullivan D, Unwin D (2002) Geographic Information Analysis, Chichester: Wiley. |
[44] |
Guerra Y (2022) Il ruolo delle città metropolitane alla luce della sentenza n. 240 del 2021: governance metropolitana e funzioni. Le Regioni 50: 499–517. https://doi.org/10.1443/106522 doi: 10.1443/106522
![]() |
[45] | Borruso G, Balletto G (2024) The Urban Life Cycle in the Italian Demographic Winter. An Analysis on Metropolitan Cities. In: Gervasi O, Murgante B, Garau C, et al. (eds), Computational Science and Its Applications – ICCSA 2024 Workshops. ICCSA 2024. Lecture Notes in Computer Science, vol 14817. Springer, Cham. https://doi.org/10.1007/978-3-031-65238-7_11 |
![]() |
![]() |
S | Our price | {V_{{\text{BS}}}} | {V_{{\text{Vasicek}}}} | {V_{{\text{sfBm - Vasicek}}}} |
20 | 0.2435 | 0.0005 | 1.8389 | 0.2208 |
23 | 0.5498 | 0.0066 | 2.8183 | 0.5202 |
26 | 1.0480 | 0.0426 | 3.9897 | 1.0211 |
29 | 1.7685 | 0.1748 | 5.3360 | 1.7589 |
32 | 2.7255 | 0.5146 | 6.8397 | 2.7506 |
35 | 3.9194 | 1.1863 | 8.4843 | 3.9968 |
38 | 5.3398 | 2.2779 | 10.2543 | 5.4858 |
41 | 6.9698 | 3.8119 | 12.1360 | 7.1977 |
44 | 8.7884 | 5.7485 | 14.1169 | 9.1087 |
47 | 10.7735 | 8.0118 | 16.1861 | 11.1933 |
50 | 12.9033 | 10.5160 | 18.3337 | 13.4270 |
S | Our price | {V_{{\text{BS}}}} | {V_{{\text{Vasicek}}}} | {V_{{\text{sfBm - Vasicek}}}} |
20 | 5.1411 | 0.2085 | 2.3002 | 0.9705 |
23 | 7.4411 | 0.4863 | 4.8981 | 2.7039 |
26 | 9.8921 | 0.9501 | 7.6053 | 4.5926 |
29 | 12.4656 | 1.6332 | 10.3988 | 6.6067 |
32 | 15.1403 | 2.5519 | 13.2620 | 8.7238 |
35 | 17.8998 | 3.7072 | 16.1825 | 10.9266 |
38 | 20.7312 | 5.0881 | 19.1509 | 13.2019 |
41 | 23.6243 | 6.6762 | 22.1600 | 15.5389 |
44 | 26.5707 | 8.4490 | 25.2039 | 17.9291 |
47 | 29.5636 | 10.3825 | 28.2781 | 20.3655 |
50 | 32.5974 | 12.4535 | 31.3787 | 22.8423 |
K | Market price | Our price | {V_{{\text{BS}}}} | {V_{{\text{Vasicek}}}} | {V_{{\text{sfBm - Vasicek}}}} |
7800 | 750 | 750.8065 | 747.9587 | 752.3958 | 749.0275 |
7500 | 694 | 693.9719 | 691.9232 | 696.2100 | 692.5075 |
7850 | 737 | 737.8847 | 734.8516 | 739.3860 | 736.1443 |
7900 | 742 | 741.1881 | 739.7236 | 744.2764 | 739.8953 |
7550 | 670 | 669.3538 | 667.6885 | 672.1255 | 668.1860 |
7650 | 658 | 658.1121 | 655.5799 | 660.1722 | 656.8193 |
7700 | 630 | 629.6739 | 627.5145 | 632.1150 | 628.5006 |
7300 | 584 | 584.4501 | 581.6303 | 586.0389 | 583.1820 |
7600 | 572 | 572.5130 | 569.3085 | 574.0554 | 571.3721 |
7450 | 541 | 540.6392 | 538.3216 | 542.9253 | 539.7519 |
7400 | 508 | 509.9819 | 505.4722 | 510.0512 | 508.4733 |
RMSE | 0.8064 | 2.3756 | 2.1638 | 1.2832 |
S | Our price | {V_{{\text{BS}}}} | {V_{{\text{Vasicek}}}} | {V_{{\text{sfBm - Vasicek}}}} |
20 | 0.2435 | 0.0005 | 1.8389 | 0.2208 |
23 | 0.5498 | 0.0066 | 2.8183 | 0.5202 |
26 | 1.0480 | 0.0426 | 3.9897 | 1.0211 |
29 | 1.7685 | 0.1748 | 5.3360 | 1.7589 |
32 | 2.7255 | 0.5146 | 6.8397 | 2.7506 |
35 | 3.9194 | 1.1863 | 8.4843 | 3.9968 |
38 | 5.3398 | 2.2779 | 10.2543 | 5.4858 |
41 | 6.9698 | 3.8119 | 12.1360 | 7.1977 |
44 | 8.7884 | 5.7485 | 14.1169 | 9.1087 |
47 | 10.7735 | 8.0118 | 16.1861 | 11.1933 |
50 | 12.9033 | 10.5160 | 18.3337 | 13.4270 |
S | Our price | {V_{{\text{BS}}}} | {V_{{\text{Vasicek}}}} | {V_{{\text{sfBm - Vasicek}}}} |
20 | 5.1411 | 0.2085 | 2.3002 | 0.9705 |
23 | 7.4411 | 0.4863 | 4.8981 | 2.7039 |
26 | 9.8921 | 0.9501 | 7.6053 | 4.5926 |
29 | 12.4656 | 1.6332 | 10.3988 | 6.6067 |
32 | 15.1403 | 2.5519 | 13.2620 | 8.7238 |
35 | 17.8998 | 3.7072 | 16.1825 | 10.9266 |
38 | 20.7312 | 5.0881 | 19.1509 | 13.2019 |
41 | 23.6243 | 6.6762 | 22.1600 | 15.5389 |
44 | 26.5707 | 8.4490 | 25.2039 | 17.9291 |
47 | 29.5636 | 10.3825 | 28.2781 | 20.3655 |
50 | 32.5974 | 12.4535 | 31.3787 | 22.8423 |
K | Market price | Our price | {V_{{\text{BS}}}} | {V_{{\text{Vasicek}}}} | {V_{{\text{sfBm - Vasicek}}}} |
7800 | 750 | 750.8065 | 747.9587 | 752.3958 | 749.0275 |
7500 | 694 | 693.9719 | 691.9232 | 696.2100 | 692.5075 |
7850 | 737 | 737.8847 | 734.8516 | 739.3860 | 736.1443 |
7900 | 742 | 741.1881 | 739.7236 | 744.2764 | 739.8953 |
7550 | 670 | 669.3538 | 667.6885 | 672.1255 | 668.1860 |
7650 | 658 | 658.1121 | 655.5799 | 660.1722 | 656.8193 |
7700 | 630 | 629.6739 | 627.5145 | 632.1150 | 628.5006 |
7300 | 584 | 584.4501 | 581.6303 | 586.0389 | 583.1820 |
7600 | 572 | 572.5130 | 569.3085 | 574.0554 | 571.3721 |
7450 | 541 | 540.6392 | 538.3216 | 542.9253 | 539.7519 |
7400 | 508 | 509.9819 | 505.4722 | 510.0512 | 508.4733 |
RMSE | 0.8064 | 2.3756 | 2.1638 | 1.2832 |