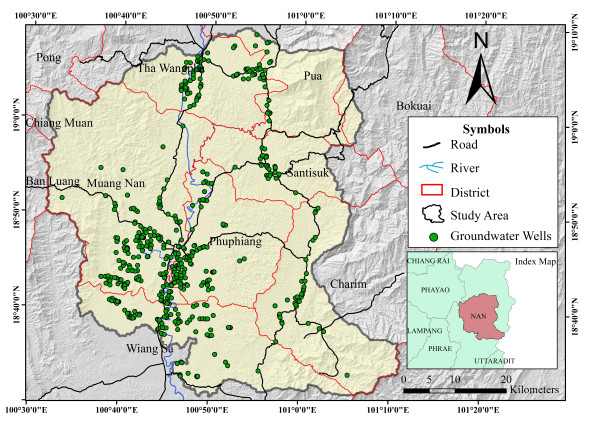
Assessing the capacity of groundwater is essential for efficient water management. Regrettably, evaluating the potential of groundwater in regions with limited data accessibility, particularly in mountainous regions, presents significant challenges. In the Nan basin of Thailand, where there is a scarcity of groundwater well data, we utilized remote sensing and geographic information system (GIS) techniques for evaluating and determining the potential of groundwater resources. The analysis included seven hydrological factors, including elevation, drainage density, lineament density, land use and land cover, slope, soil moisture, and geology. The quantification of groundwater potential was conducted by the utilization of linear combination overlays, employing weights derived from two distinct methodologies: the analytical hierarchy process (AHP) and the frequency ratio (FR). Interestingly, it is noteworthy that both the FR and AHP approaches demonstrated a very comparable range of accuracy levels (0.89–1.00) when subjected to cross-validation using field data pertaining to groundwater levels. Although the FR technique has shown efficacy in situations when data is well-distributed, it displayed constraints in regions with less data, which could potentially result in misinterpretations. On the other hand, the AHP provided a more accurate assessment of the potential of groundwater by taking into account the relative importance of the criteria throughout the full geographical scope of the study. Moreover, the AHP has demonstrated its significance in the prioritization of parameters within the context of water resource management. This research contributes to the development of sustainable strategies for managing groundwater resources.
Citation: Nudthawud Homtong, Wisaroot Pringproh, Kankanon Sakmongkoljit, Sattha Srikarom, Rungtiwa Yapun, Ben Wongsaijai. Remote sensing-based groundwater potential evaluation in a fractured-bedrock mountainous area[J]. AIMS Geosciences, 2024, 10(2): 242-262. doi: 10.3934/geosci.2024014
[1] | Watcharin Phoemphon, Bantita Terakulsatit . Assessment of groundwater potential zones and mapping using GIS/RS techniques and analytic hierarchy process: A case study on saline soil area, Nakhon Ratchasima, Thailand. AIMS Geosciences, 2023, 9(1): 49-67. doi: 10.3934/geosci.2023004 |
[2] | Palavai Venkateswara Rao, Mangalampalli Subrahmanyam, Bakuru Anandagajapathi Raju . Groundwater exploration in hard rock terrains of East Godavari district, Andhra Pradesh, India using AHP and WIO analyses together with geoelectrical surveys. AIMS Geosciences, 2021, 7(2): 244-267. doi: 10.3934/geosci.2021015 |
[3] | Maurizio Barbieri, Tiziano Boschetti, Giuseppe Sappa, Francesca Andrei . Hydrogeochemistry and groundwater quality assessment in a municipal solid waste landfill (central Italy). AIMS Geosciences, 2022, 8(3): 467-487. doi: 10.3934/geosci.2022026 |
[4] | Seyed Mohsen Mousavi, Ali Golkarian, Seyed Amir Naghibi, Bahareh Kalantar, Biswajeet Pradhan . GIS-based Groundwater Spring Potential Mapping Using Data Mining Boosted Regression Tree and Probabilistic Frequency Ratio Models in Iran. AIMS Geosciences, 2017, 3(1): 91-115. doi: 10.3934/geosci.2017.1.91 |
[5] | Evgeniy V. Torgashov, Aleksandra V. Varnavina . Site Characterization during Bridge Foundation Construction Using Electrical Resistivity Tomography. AIMS Geosciences, 2016, 2(3): 201-213. doi: 10.3934/geosci.2016.3.201 |
[6] | Diaka Sidibé, Mamadou Diallo, Ahmed Amara Konaté, Muhammad Zaheer . Geotechnical investigations of lead-silver ore processing residues at the Auzelles site, Auvergne (France). AIMS Geosciences, 2024, 10(4): 735-758. doi: 10.3934/geosci.2024036 |
[7] | Andre Vervoort . Impact of the closure of a coal district on the environmental issue of long-term surface movements. AIMS Geosciences, 2022, 8(3): 326-345. doi: 10.3934/geosci.2022019 |
[8] | Dujie Zhang . Rock strength degradation induced by salt precipitation: A new mechanical mechanism of sand production in ultra-deep fractured tight sandstone gas reservoirs. AIMS Geosciences, 2023, 9(3): 595-608. doi: 10.3934/geosci.2023032 |
[9] | Paul W. Mayne, Ethan Cargill, Bruce Miller . Geotechnical characteristics of sensitive Leda clay at Canada test site in Gloucester, Ontario. AIMS Geosciences, 2019, 5(3): 390-411. doi: 10.3934/geosci.2019.3.390 |
[10] | Eric Ariel L. Salas, Sakthi Subburayalu Kumaran, Robert Bennett, Leeoria P. Willis, Kayla Mitchell . Machine Learning-Based Classification of Small-Sized Wetlands Using Sentinel-2 Images. AIMS Geosciences, 2024, 10(1): 62-79. doi: 10.3934/geosci.2024005 |
Assessing the capacity of groundwater is essential for efficient water management. Regrettably, evaluating the potential of groundwater in regions with limited data accessibility, particularly in mountainous regions, presents significant challenges. In the Nan basin of Thailand, where there is a scarcity of groundwater well data, we utilized remote sensing and geographic information system (GIS) techniques for evaluating and determining the potential of groundwater resources. The analysis included seven hydrological factors, including elevation, drainage density, lineament density, land use and land cover, slope, soil moisture, and geology. The quantification of groundwater potential was conducted by the utilization of linear combination overlays, employing weights derived from two distinct methodologies: the analytical hierarchy process (AHP) and the frequency ratio (FR). Interestingly, it is noteworthy that both the FR and AHP approaches demonstrated a very comparable range of accuracy levels (0.89–1.00) when subjected to cross-validation using field data pertaining to groundwater levels. Although the FR technique has shown efficacy in situations when data is well-distributed, it displayed constraints in regions with less data, which could potentially result in misinterpretations. On the other hand, the AHP provided a more accurate assessment of the potential of groundwater by taking into account the relative importance of the criteria throughout the full geographical scope of the study. Moreover, the AHP has demonstrated its significance in the prioritization of parameters within the context of water resource management. This research contributes to the development of sustainable strategies for managing groundwater resources.
Long-term drought and population growth are threatening water supplies, leading to adverse consequences [1,2,3]. On a global scale, groundwater plays a vital role as a significant supply of fresh water with the capacity to address these challenges. Groundwater is typically less polluted than surface water, which enhances its significance for public water supply, agriculture, industrial, and residence purposes [4,5,6]. The aspects of population growth, urbanization, agricultural development, and climate change contribute to an increased demand for groundwater [7]. Therefore, it is necessary to estimate groundwater potential to lay the groundwork for its development and utilization [8]. An assessment of groundwater potential assists in determining an appropriate technique for artificial groundwater recharge, which can facilitate agricultural activities and increase agricultural income [9]. Groundwater is particularly vital in mountainous areas [10], where residents often face water supply challenges due to a scarcity of available surface water [11]. Additionally, the lack of wells in mountainous locations worldwide has created a data gap for groundwater, affecting these high-elevation, sparsely populated regions and presenting significant access challenges [12]. Forecasting groundwater supplies in mountainous areas necessitated a method that considered a broad collection of data and method availability, including various mathematical methodologies [13]. Previous studies have examined groundwater potential zones using integrated remote sensing (RS) and geographic information systems (GIS), which offer the advantage of covering broad areas, including inaccessible locations, and provide quick and cost-effective methods for obtaining crucial geological and morphological data [14,15,16,17,18,19,20]. Nowadays, advancements in information and technology are available, including GIS, remote sensing technologies, modeling, along with multi- criteria decision-making (MCDM) such as frequency ratio (FR), weights- of-evidence (WOE), certainty factor (CF), fuzzy logic index models (see [21,22,23]) allowing to move beyond direct investigation from fieldwork [24]. For instance, Aghlmand and Abbasi [25] utilized several sources of data, including geological, hydrogeological, hydrological, climatological, and geographical factors, to simulate groundwater resource systems. They deployed the MODFLOW model to analyze both qualitative and quantitative aspects of the system. Additionally, a number of researchers have studied groundwater evaluation in dry and semi-arid regions of developing nations using GIS and RS [26]. The GIS-based Multiple criteria decision making (MCDM) technique is a highly effective alternative that offers a range of methods for various applications. The most regularly used is the analytical hierarchy process (AHP); the relative impact of multiple criteria on each other when developing suitable areas produces valuable results in groundwater potential using the model designed specifically for this investigation [24]. Furthermore, the FR method is widely used for evaluating the potential of groundwater in multi-criteria decision making. It is a fundamental statistical method used in multiple domains to analyze two variables together [27]. The FR model is employed in geospatial evaluation to determine the probabilistic correlation between variables or classified thematic layers [28]. Groundwater evaluation is commonly accomplished through the use of RS and GIS data in the AHP [24]. The AHP technique was devised by Saaty [29]. The groundwater recharge zone in the Korba aquifer (northeastern Tunisia) was mapped by Zghibi et al. [23] using two MCDM approaches: the multi-influencing factors (MIF) and the AHP. The outcome demonstrated the practicality of using AHP methodologies for evaluating groundwater potential, providing a quick, accurate, and cost-effective assessment. Similarly, the study of Pande et al. [30] applied AHP and MIF for groundwater potential delineation in the Mula river bain, India. Moreover, the FR technique has been employed to evaluate landslides in the Kermanshah region of western Iran. This approach demonstrates high precision when compared to historical data on landslides and has the capability to forecast spatial landslides [31]. Also, the FR approach was utilized to project the mapping of groundwater potential in the Lower Valley of the Medjerda River sub-basin. Approximately 60 groundwater transmissivity data points were randomly divided into a training dataset, with 70% being used for model training and 30% for validation. The assessment of a map based on 15 parameters revealed a high degree of accuracy [32]. The FR method, it was believed, could also be used to estimate groundwater potential [33]. Integrating RS-GIS with the AHP and FR approaches decreases the expenses and duration required to address the intricate understanding of extensive areas, including inaccessible groundwater. These methods also help produce highly reliable results that can support further planning and sustainable groundwater resource utilization in water-stressed areas [34].
Due to the high concentration of wells in the urbanized zone of the mountainous region of Nan province, Thailand, there are few unexplored areas in this region. The limited availability of direct information from a small number of groundwater wells within a localized area is a substantial obstacle to comprehending the local groundwater system. Most of the information regarding groundwater potential in this region is still to be acquired. The objective of this study is to evaluate the potential of groundwater in the Nan basin by utilizing GIS and RS techniques and applying the AHP and FR approaches. The use of GIS and RS tools enables a thorough comprehension of the hydrogeomorphological features that are pertinent to the groundwater potential in the area under investigation. In addition, the groundwater potential maps produced using the AHP and FR methodologies are verified by comparing them to groundwater level data collected from field investigations. These results provide a model for evaluating groundwater potential in areas lacking sufficient groundwater well data, especially mountainous regions. Moreover, the delineation of groundwater potential areas can facilitate strategic planning for the effective management and usage of this valuable resource.
Figure 1 depicts the location of the Nan Basin, which is positioned in the mountainous terrain of central Nan Province in northern Thailand. The basin has a total area of 3,327.13 square kilometers. The study area encompasses eight districts: Wiang Sa, Mae Charim, Phu Phiang, Mueang Nan, Santi Suk, Bo Kluea, Pua, and Tha Wang Pha. Elevations within the region vary from 143 to 1,885 meters above sea level, with north-south-oriented mountain ranges in the east and west. The Nan River flows through the research area. The southwest and northeast monsoons have an impact on the tropical climate that this region experiences, which is marked by hot summers and cold winters. Temperature decreases from October to February during the winter months, with an average temperature of 25.9 ∘C. The basin has an average annual precipitation of approximately 1,456 mm. Notably, the study area features numerous geological fractures, including cracks, faults, and joints, stemming from active faults [35]. As per data from the Department of Groundwater Resources of Thailand [36], groundwater potential assessments have primarily focused on areas with existing groundwater wells, mainly in urban zones, as depicted in Figure 2. The mountainous regions outside residential areas, where groundwater wells are lacking, have been categorized as low potential zones, with high levels of uncertainty.
This study examines seven hydrogeomorphological parameters that affect groundwater potential. These parameters include proven influential factors [37,38] such as lineament density, drainage density, geology, and slope. Additionally, other factors that influence groundwater potential are soil moisture, elevation, and land use and land cover. Secondary data were retrieved from various governmental databases and re-evaluated using GIS techniques. Remote sensing data from various databases were analyzed using GIS techniques. All data were delineated into thematic maps; data sources are indicated in Table 1. All data were recorded in the Universal Transverse Mercator Projection, northern zone 48, datum WGS84 during the data collection phase. Subsequently, ArcGIS 10.5, PCI Geomatics 2016, and eCognition Developer 64 were used to process the data. Figure 3 illustrates the complete procedure for determining groundwater potential zones in the designated study region.
Data | Sources | Scale/Resolution | Thematic maps |
Digital Elevation | Alaska Satellite | Spatial resolution | -Drainage density |
Model (DEM) | Facility (ASF) | 12.5 m. | -Elevation |
-Slope | |||
Geologic map of Nan Province | Department of Mineral | 1: 250,000 | -Geologic map |
Resources (DMR) | |||
Satellite image | European Space | Spatial resolution | -Lineament density |
(Sentinel-2) | Agency (ESA) | 10 m. | -Land use and land Cover |
-Soil moisture |
The AHP technique quantitatively converts measurable objects using a ratio scale. AHP is an application of mathematical principles, falling under the category of multi-criterion analyses, specifically MCDA. It facilitates the analysis and decision-making process for complex choices involving multiple criteria that may be challenging to evaluate and resolve. Consequently, all tangible and intangible factors must be considered. Ratings based on expert judgment are assigned using numbers ranging from 1 to 9 to prioritize factors and ensure consistency [39].
To minimize the need for extensive calculations during subsequent arithmetic operations on these scales, a standardized scale has been employed to assess the qualities of factors through pairwise comparisons [29]. By constructing a matrix for pairwise comparisons, each element at a higher level is compared to the items immediately below it. A numerical value between 1 and 9 is used to indicate the significance of a factor, with more significant factors being assigned higher numbers [39]. The pairwise comparison matrix is a necessary step in calculating the normalized principal eigenvector, which quantifies the relative values of the parameters [40].
The level of consistency attained in the ratings is quantified using a consistency ratio (C.R.), which represents the likelihood that the ratings in the matrix were generated randomly. The C.R. value of 0.1 or lower signifies a satisfactory reciprocal matrix, whereas a ratio above 0.1 suggests that the matrix needs to be modified [41]. Inconsistency can be interpreted as a required change to make the similarities more accurate [42]. Using the following formula, the C.R. is determined by
C.R.=C.I./R.I., |
C.I. = consistency index,
R.I. = random consistency index (see Table 2).
Order | 1 | 2 | 3 | 4 | 5 | 6 | 7 | 8 | 9 | 10 |
R.I. | 0 | 0 | 0.52 | 0.89 | 1.11 | 1.25 | 1.35 | 1.40 | 1.45 | 1.49 |
The consistency index of a matrix is determined follows,
C.I.=λmax−nn−1, |
where λmax is the principal eigen value and n is the number of comparisons. [41,42].
The AHP was utilized in this study to classify potential groundwater areas within the Nan watershed. The research focused on hydrogeological factors assessed by experts, which included land use and land cover, drainage density, elevation, geology, lineament density, slope, and soil moisture. The selection of key factors for decision-making is a critical aspect of the process. The size of the metrics is determined by the factors chosen for calculations in the weight matrix and the normalized principal eigenvector [29,39]. The quantitative evaluation of groundwater has been delineated by calculating the groundwater potential zone (GWPZ). The GWPZ for the research area was calculated using the subsequent equation:
GWPZ=EVwEViw+SLwSLiw+DDwDDiw+LDwLDiw+SMwSMiw+LULCwLULCiw+GGwGGiw, |
where w = normalized weight of a feature, iw = individual feature normalize weight, EV = elevation, SL = slope, DD = drainage density, LD = lineament density, SM = soil moisture, LULC = land use and land cover, and GG = geology.
The FR represents the probability of a specific attribute occurring, with a FR value of 1 indicating a high probability. When assessing the potential of groundwater, a frequency ratio below 1 implies a low likelihood of groundwater existence, whereas a ratio around 1 signifies a high likelihood. An evaluation was conducted to determine the sensitivity of each aspect and, afterwards, a probability frequency model was utilized to map the regional groundwater potential [44]. The groundwater potential frequency ratio was then calculated by superimposing the various components assigned to the frequency ratio, with a frequency ratio being assigned to each factor's class. To compute the FR value for each class of groundwater-related factor, we used the following equation:
FR=(W/G)/(M/T). |
For each conditioning factor, W is the number of pixels with a groundwater well; G represents the total number of groundwater wells in the study area; M is the number of pixels in the factor's class area; T is the total number of pixels.
The groundwater potential index (GPI) is measured by multiplying the ratio values of each factor and can be calculated by
GPI=∑FR, |
where Fr = Rating of each factors' type or range [28].
During field data collection, a low groundwater potential zone in the center of the study region provided a groundwater level map, which was used to validate the groundwater potential zone. A confusion matrix was employed to verify the prediction results. A true positive (TP) occurs when both the actual data and the projected value are positive. A false negative (FN) occurs when the anticipated value is negative, despite the actual output being positive. A true negative (TN) refers to a scenario when both the observed outcome and the prediction are negative. A false negative (FN) occurs when the anticipated value is negative, but the actual output is positive [45]. Binary classification metrics were used to evaluate default classification accuracy [46]. Accuracy, as shown in Equation (2.1), estimates the probability of true values (TP and TN) of the class. Precision, as shown in Equation (2.2), measures correctness. Sensitivity (or recall), as demonstrated in Equation (2.3), assesses the completeness or accuracy of positive examples. Specificity, represented in Equation (2.4), is the conditional probability of true negatives (TN) given [47].
Accuracy = (TP+TN) / (TP+TN+FP+FN), | (2.1) |
Precision = TP/ (TP+FP), | (2.2) |
Sensitivity = TP / (TP+FN), | (2.3) |
Specificity = TN / (TN+FP). | (2.4) |
Seven hydrogeomorphological factors–elevation, drainage density, lineament density, land use and land cover, slope, soil moisture, and geology–as indications of groundwater potential have been prepared for AHP and FR calculation to evaluate the groundwater potential of the Nan basin. The results are compared and discussed as follows.
In the pairwise comparison procedure, all seven factors were arranged in a matrix table to compare their relative influence on other factors according to the Saaty's 9-points scale [43], as shown in Table 3. In this mountainous study area, fracture-related factors, lineament density, drainage density, and geology were considered the most influential factors compared to others. The influential level of each factor is indicated in the pairwise matrix scale; 1 represents equal importance, and 9 represents a highly influential level.
Factors | EV | SL | DD | LD | SM | LULC | GG |
EV | 1.00 | 1/3 | 1/5 | 1/5 | 1/4 | 3.00 | 1/3 |
SL | 3.00 | 1.00 | 1/4 | 1/8 | 1/2 | 2.00 | 1/3 |
DD | 5.00 | 4.00 | 1.00 | 1.00 | 4.00 | 6.00 | 1.00 |
LD | 5.00 | 8.00 | 1.00 | 1.00 | 2.00 | 9.00 | 1.00 |
SM | 4.00 | 2.00 | 1/4 | 1/2 | 1.00 | 3.00 | 1/7 |
LULC | 1/3 | 1/2 | 1/6 | 1/9 | 1/3 | 1.00 | 1/4 |
GG | 3.00 | 3.00 | 1.00 | 1.00 | 7.00 | 4.00 | 1.00 |
Totals | 21.33 | 18.83 | 3.87 | 3.94 | 15.08 | 28.00 | 4.06 |
By dividing the amount of each factor column by the cell number given to each factor, the relative normalized weight of the criterion was determined. Furthermore, each factor was divided into five classes by the Natural Break Jenks technique and each class was assigned its own rank. Except for geology factors, classes and its assigned ranks were determined by permeability according to lithological properties of each rock formation. Weight and rank of hydrogeomorphological factors are shown in Table 4.
Criteria | Normalize weight | Class | Assigned rank | Feature normalized weight |
EV (m) | 0.0533 | 143–306 | 5 | 0.42 |
306–484 | 4 | 0.26 | ||
484–730 | 3 | 0.16 | ||
730–1078 | 2 | 0.10 | ||
1078–1884 | 1 | 0.06 | ||
SL (Degree) | 0.0681 | 0–7 | 5 | 0.42 |
7–13 | 4 | 0.26 | ||
13–20 | 3 | 0.16 | ||
20–29 | 2 | 0.10 | ||
29–72 | 1 | 0.06 | ||
DD (km/sq.km) | 0.2408 | 30–70 | 5 | 0.42 |
77–79 | 4 | 0.26 | ||
99–119 | 3 | 0.16 | ||
119–134 | 2 | 0.10 | ||
134–162 | 1 | 0.06 | ||
LD (km/sq.km) | 0.2675 | 0–0.42 | 1 | 0.06 |
0.42–0.81 | 2 | 0.10 | ||
0.81–1.22 | 3 | 0.16 | ||
1.22–1.74 | 4 | 0.26 | ||
1.74–2.80 | 5 | 0.42 | ||
SM | 0.0991 | Very–low | 1 | 0.06 |
Low | 2 | 0.10 | ||
Moderate | 3 | 0.16 | ||
High | 4 | 0.26 | ||
Very–high | 5 | 0.42 | ||
LULC | 0.0333 | Building | 3 | 0.10 |
Bare Soil | 2 | 0.16 | ||
Vegetation | 4 | 0.28 | ||
Water | 5 | 0.47 | ||
GG | 0.2380 | Qa | 5 | 0.19 |
Tr1 | 4 | 0.13 | ||
CP | 3 | 0.08 | ||
JKpw | 3 | 0.08 | ||
Png2 | 3 | 0.08 | ||
TRJ | 3 | 0.08 | ||
Trgr | 2 | 0.05 | ||
PTr | 2 | 0.05 | ||
JPK | 2 | 0.05 | ||
Tmm | 2 | 0.05 | ||
Jv | 1 | 0.03 | ||
Ptru | 1 | 0.03 | ||
Pr-2 | 1 | 0.03 | ||
Tr2 | 1 | 0.03 | ||
Trwc | 1 | 0.03 |
Wells distribution throughout the study area was a significant criterion for FR method calculation to determine a relationship between groundwater (potential) well locations and hydrogeomorphologic factors. According to the groundwater level database and groundwater measurement from the field study, high groundwater potential wells are limited, which is a challenge. The groundwater wells that were taken into consideration were low-potential groundwater wells mostly concentrated in the center of the study area. Thus, FR was analyzed based on a spatial distribution of low groundwater potential area (higher groundwater potential = low FR), listed in Table 5. A total of 46 wells were included in the field study, out of which 29 groundwater wells were selected based on their spatial distribution. Among these, 21 wells were utilized for training purposes, while the remaining 8 wells were used for validation.
Criteria | Sub-class | No. of pixels | Percentage of sub-class | No. of wells | Percentage of wells | FR | Weight |
EV(m) | 143–306 | 2343787 | 44.03 | 21 | 100 | 2.27 | 1.994 |
306–484 | 1637049 | 30.75 | 0 | 0 | 0.00 | ||
484–730 | 819445 | 15.39 | 0 | 0 | 0.00 | ||
730–1078 | 359929 | 6.76 | 0 | 0 | 0.00 | ||
1078–1884 | 163044 | 3.06 | 0 | 0 | 0.00 | ||
SL(Degree) | 0–7 | 1537601 | 28.88 | 21.00 | 100.00 | 3.46 | 1994 |
7–13 | 1236124 | 23.22 | 0.00 | 0.00 | 0.00 | ||
13–20 | 1252052 | 23.52 | 0.00 | 0.00 | 0.00 | ||
20–29 | 951705 | 17.88 | 0.00 | 0.00 | 0.00 | ||
29–72 | 345771 | 6.50 | 0.00 | 0.00 | 0.00 | ||
DD | 30–70 | 1155033 | 21.78 | 0.00 | 0.00 | 0.00 | 1.000 |
(km/sq.km) | 77–79 | 2203154 | 41.54 | 4.00 | 19.05 | 0.46 | |
99–119 | 1345062 | 25.36 | 3.00 | 14.29 | 0.56 | ||
119–134 | 499485 | 9.42 | 12.00 | 57.14 | 6.07 | ||
134–162 | 100851 | 1.90 | 2.00 | 9.52 | 5.01 | ||
LD (km/sq.km) | 0–0.42 | 1092610 | 20.60 | 0.00 | 0.00 | 0.00 | 1.579 |
0.42–0.81 | 1586183 | 29.91 | 3.00 | 14.29 | 0.48 | ||
0.81–1.22 | 1493621 | 28.16 | 17.00 | 80.95 | 2.87 | ||
1.22–1.74 | 906948 | 17.10 | 1.00 | 4.76 | 0.28 | ||
1.74–2.80 | 224344 | 4.23 | 0.00 | 0.00 | 0.00 | ||
SM | Very–low | 555729 | 10.48 | 2.00 | 9.52 | 0.91 | 1.219 |
Low | 663175 | 12.51 | 12.00 | 57.14 | 4.57 | ||
Moderate | 825390 | 15.56 | 6.00 | 28.57 | 1.84 | ||
High | 1590995 | 30.00 | 1.00 | 4.76 | 0.16 | ||
Very–high | 1667896 | 31.45 | 0.00 | 0.00 | 0.00 | ||
LULC | Building | 182961 | 3.45 | 16.00 | 76.19 | 22.08 | 1.951 |
Bare Soil | 1090802 | 20.57 | 1.00 | 4.76 | 0.23 | ||
Vegetation | 3999858 | 75.42 | 4.00 | 19.05 | 0.25 | ||
Water | 29504 | 0.56 | 0.00 | 0.00 | 0.00 |
Elevation reflects groundwater potential from various perspectives. The area's elevation provides insights into its surface geomorphology, reflecting the region's geological characteristics, such as basins or low areas capable of holding water and allowing it to seep underground [48]. Typically, higher elevations exhibit characteristics of groundwater recharge areas with higher water tables. The 12.5 meter-resolution digital elevation model (DEM) was employed to generate the elevation map (Figure 4 a). Elevation levels were categorized into five distinct ranges: very–low (143–306 m), low (306–484 m), moderate (484–730 m), high (730–1,078 m), and very–high altitude (1,078–1,884 m) above mean sea level. The research area is primarily a mountainous terrain, with lower heights in the central half, implying the presence of a ridge oriented in a north-south direction.
Slope is an important parameter that refers infiltration degree spatially. As the slope is the result of elevation changes, water permeability is an important hydrological factor for potential groundwater areas [24]. This is because the impact of gravity on the movement of water is a controlling factor. Inversely proportional to the slope value is the penetration rate of water into the underground. That is, areas with low slopes (flat areas) have elevated water penetration into the basement [49]. The slope plays a significant role in controlling the time cycle of overland flow, penetration, and through flow. Higher slope trends to influence water to flow over surface as runoff instead of infiltrating into the subsurface, and vice versa. Higher runoff, lower infiltration, and lower groundwater recharge are all effects of steeper slopes [46]. Thus, higher groundwater potential is expected in a lower slope area. The slope map was delineated in ArcMap software (version 10.5). The study area can be categorized into five different slope levels (Figure 4b): very–low slope with the value 0–7∘, low slope with 7–13∘, moderate slope with 13–20∘, high slope with 20–29∘, very–high slope with 29–72∘.
Drainage density is calculated by dividing the total length of all the rivers in a drainage basin by the total area of the drainage basin [50]. The drainage density illustrates the route of drainage within the studied area. The rate at which water enters the basement is inversely correlated with the density of the drainage. Areas with high drainage densities result in low infiltration rates into the basement [33]. The DD was calculated using 12.5 meters-resolution DEM. The study area can be categorized into five different DD (Figure 4c): areas with very–low drainage densities of 30–70 km./sq.km., low drainage densities of 77–79 km./sq.km., areas with moderate drainage density of 99–119 km./sq.km., areas with high drainage density of 119–134 km./sq.km., and very–high drainage densities of 134–162 km./sq.km. High to very–high drainage density areas were found in the middle part of the study area along the Nan River, resulting in low groundwater potential.
Lineament characteristics are the result of tectonic, which can be observed by using satellite images. These characteristics indicate geological structures such as faults, fractures, and joints, indicating water in secondary porosity. When the linear structure maps were analyzed together with the exploration drilling, they provided good drilling and exploration results [49]. Lineament density indicates the possible penetration of surface water into the groundwater. Areas with high lineament density will penetrate and fill the basement with water, which provides a guideline for potential groundwater research and exploration [51]. Groundwater feeding is more significant in areas where line density is elevated; groundwater feeding is lower in low-density areas [24]. Lineament density map is generated as a result of lineament map. Automatic lineament extraction from high-resolution sentinel 2 (band 8) satellite imagery was analyzed via PCI Geomatica (version 2016) software. Linear structures extracted using the Edge detection and thresholding command and the Curve extraction command determine the factors for assessing lineament structure. Finally, LD was analyzed with Arcmap 10.5 software using the Line density command in the Spatial analysis tool.
The density of lineaments reveals the presence of a zone of weakness in the research area. The density of lineaments is categorized into five distinct groups (Figure 4d): very–low LD 0.00–0.42 km./sq.km., low LD 0.42–0.81 km./sq.km., moderate LD 0.81–1.22 km./sq.km., high LD 1.22–1.74 km./sq.km., and very–high LD 1.74–2.80 km./sq.km., indicating a high groundwater potential area. A significant concentration of lineaments was identified in the southeastern portion of the research area.
The quantity of water in the soil is soil moisture. It is often located in an unsaturated zone, consistent with the ground cover, in the uncontaminated rock layer before the water table [48]. Areas of study play a key role in controlling runoff and permeability rates for skin and soil types, important factors in controlling potential groundwater areas. The permeability of soil is determined by the porosity of each type of soil, which is significantly affected by soil texture, structure, gap ratio, and saturation level [52].
Using the band ratio technique, the soil moisture map (Figure 4e) was created using satellite imagery with a spatial resolution of 10 m in order to construct the normalize different moisture index (NDMI). The soil moisture in the study area is categorized into five classes: very–low, low, moderate, high, and very–high. The study area revealed high soil moisture levels along its eastern and western borders. The distribution is also present across the mountainous region of the research area.
Water recharge, evapotranspiration, and runoff are all directly influenced by land use and land cover [53]. Water seepage into the groundwater has decreased as the built-up area has increased. The study area's land use and land cover type are used to determine runoff and permeability [48]. Human activities play an important role in the formation and distribution of groundwater [51]. Water surface covers, such as water bodies, contribute to higher groundwater areas than built-up areas. Cultivated areas prevent the seepage of water into the ground [24]. In the study area, LULC could be classified into four types (Figure 4f): 1) building-up, 2) bare soil 3) vegetation, and 4) water body. Most of the study areas were characterized as areas of vegetation, covering more than 75.42% spread along the mountain area.
The type of rock has an impact on the ability for groundwater recharge [34]. Geology is a consideration governing groundwater quality and quantities. The rock's characteristics provide information about the porosity and permeability of the aquifer material in the research area [49,54]. The different rocks have varying coefficients of permeability. Therefore, the permeability of surface water to groundwater has distinct characteristics [55]. High-porosity areas will lead to high permeability and groundwater retention; as a result, there is much water in that area [56]. The study area can be classified into 15 different geological types (Figure 4g) of unconsolidated sediments, igneous rocks, sedimentary rocks, and metamorphic rocks. The central area of the Quaternary sediment, which accumulates along the Nan River, is littered with unconsolidated sediment. This sediment has a high permeability, resulting in a high groundwater potential in the region. Igneous and metamorphic rocks were also discovered scattered about the study area. Because this rock has low primary porosity, that area has a low groundwater potential.
The AHP and FR approaches were utilized to evaluate the impact of all seven parameters on groundwater potential. The purpose of these analyses was to create groundwater potential maps for the Nan Basin in Nan Province, Thailand, which is an area with limited well data. The resulting groundwater potential maps, produced through AHP and FR methodologies, were categorized into four levels–very low, low, high, and very high–using the equal interval function in ArcGIS software (version 10.5), as summarized in Table 6. Significant differences exist between the groundwater potential zones identified by the AHP and FR techniques, especially in the category of very–high groundwater potential. The many variances will be thoroughly examined within the framework of each groundwater potential map.
AHP method | FR method | ||||
Class | Area (km2) | Area (%) | Area (km2) | Area (%) | |
Very–low | 131.60 | 3.99 | 367.97 | 11.15 | |
Low | 404.99 | 12.27 | 1835.53 | 55.61 | |
High | 677.94 | 20.55 | 955.27 | 28.94 | |
Very–high | 2084.81 | 63.19 | 142.10 | 4.30 |
The groundwater potential zone of the study region can be categorized into four levels using hydrogeological analysis through the AHP. These zones, which represent very high, high, low, and very low groundwater potential, cover 2085 sq.km. (63.19%), 678 sq.km. (20.55%), 405 sq.km. (12.27%), and 132 sq.km. (3.99%), respectively (Figure 5a). The southeastern half of the research region has a wide variety of groundwater potentials, varying from high to very–high. The study area included locations in the central part with low to extremely–low potential, as indicated by drainage densities. The FR approach involved the delineation of a groundwater potential map using data from 21 groundwater wells (plus 8 wells for validation). The map was then separated into four distinct levels: the area of very–high groundwater potential is 142 sq.km. (4.30%), high groundwater potential 955 sq.km. (28.94%), low groundwater potential 1,836 sq.km. (55.61%), and the area of very–low groundwater potential 368 sq.km. (11.15%) (Figure 5b). The Nan River, located in the central region, was assessed as having low to very–low potential for groundwater.
The accuracy of the groundwater potential maps was assessed through field data collection, specifically groundwater level measurements. To validate the methods, we employed a confusion matrix and binary classification. Out of the 46 groundwater levels measured within the study area, 30 wells located in moderate to very–low groundwater potential zones were selected for dual purposes: (1) FR analysis, encompassing both training and testing, and (2) serving as testing wells to validate the groundwater potential map generated using the AHP method. For the validation process, groundwater levels from nine wells were utilized, with five cross-validations conducted to assess the accuracy of the groundwater maps generated by both the AHP and FR methods. In each cross-validation, nine testing wells were randomly chosen. The results revealed that both the AHP groundwater potential map and the FR method demonstrated comparable accuracy levels within the range of 0.89–1.00.
As a result, both the FR and AHP methods for potential groundwater mapping exhibited identical accuracy levels in areas characterized by a concentrated distribution of wells within relatively low groundwater potential zones. The FR method's effectiveness in groundwater potential assessment was determined based on the spatial distribution of wells across different classes [57]. Notably, the FR technique offers the advantage of not requiring user-defined weights and ranks [58]. It is a particularly convenient approach when dealing with large datasets [59]. The FR method is likely to yield reliable results when groundwater wells are distributed evenly across all factor classes. In contrast, AHP requires deep knowledge of the physical meaning of the influencing factors [58]. Moreover, AHP is not suitable for complex determination, and uncertainty can be associated with experts' opinions [33]. AHP is commonly used in multi-criteria decision analysis because it is flexible, and the influencing factors can be rated in numerical values [33]. Although both the AHP and the FR approaches yielded comparable accuracy in generating groundwater potential maps, using the groundwater map generated by the AHP method is recommended. The AHP results can indicate factors influencing groundwater potential and their weight. Additionally, the application of the AHP in this study resulted in the generation of a spatial representation of groundwater potential that aligns with established theoretical criteria. Notably, areas with high groundwater potential corresponded with regions of high elevation, high lineament density, and substantial soil moisture. Hence, AHP can offer a conceptual framework for groundwater management in the basin, specifically in terms of devising management plans for each hydrogeomorphological factor. More information on groundwater levels in a well-lacking mountainous area will improve the accuracy of the groundwater potential map. Therefore, it is recommended to do additional local groundwater potential research if the groundwater potential map generated from this study is to be used for groundwater drilling and utilization purposes.
Assessing groundwater potential in mountainous areas presents unique challenges due to the scarcity of groundwater wells. The Nan Basin in Thailand is characterized by a concentration of wells in urban areas with high groundwater utilization. Evaluating groundwater potential in such mountainous regions holds promise as an alternative groundwater resource. Utilizing GIS and RS techniques offers a broader perspective for spatial groundwater potential assessment.
This study utilized two distinct multi-criteria decision analysis techniques: AHP and FR. We considered seven hydrogeomorphological factors that influence groundwater potential, with their weights determined using the AHP method: lineament density, drainage density, geology, soil moisture, slope, elevation, and land use and land cover. Notably, the most influential factors in the AHP method were associated with fractured rock properties. As a result, we generated groundwater potential maps using both the AHP and FR methods and evaluated their accuracy by comparing them with groundwater level data collected from 46 wells in the study area. The results indicated that both methods achieved a comparable accuracy range, ranging from 0.89 to 1.00. However, despite their similar accuracy levels, the AHP method is recommended for this study due to its ability to provide valuable factor weights that can inform groundwater exploration and basin management.
By leveraging GIS and RS techniques, our groundwater maps offer a broader perspective for evaluating groundwater potential in areas lacking sufficient well data, thereby enhancing groundwater utilization beyond well-concentrated areas. As a result, the groundwater potential maps produced in this study can contribute to a more comprehensive groundwater resource management compared to existing maps from the Department of Groundwater Resources (DGR) of Thailand. Our findings have the potential for application in other well-lacking areas, particularly in additional mountainous regions where groundwater potential assessment is currently lacking. Furthermore, the choice between AHP and FR methods should take into account the availability of well data in each basin. The FR technique for assessing groundwater potential is appropriate for regions with a substantial number of accessible groundwater wells. On the other hand, the AHP technique is recommended for assessing groundwater in a limited number of wells where there is a lack of well distribution. The AHP technique enables experts to examine the significant hydrogeomorphological factors affecting groundwater potential while including statistical support to ensure accuracy. Therefore, it is essential for the researcher to take into account the quantity and spatial distribution of groundwater wells before adopting an appropriate method for groundwater potential evaluation.
The authors declare they have not used Artificial Intelligence (AI) tools in the creation of this article.
The research was supported by CMU Junior Research Fellowship Program.
Authors declare no conflict of interest.
[1] |
Chenini I, Mammou AB (2010) Groundwater recharge study in arid region: An approach using GIS techniques and numerical modeling. Comput Geosci 36: 801–817. https://doi.org/10.1016/j.cageo.2009.06.014 doi: 10.1016/j.cageo.2009.06.014
![]() |
[2] |
Parisi A, Monno V, Fidelibus MD (2018) Cascading vulnerability scenarios in the management of groundwater depletion and salinization in semi-arid areas. Int J Disaster Risk Reduct 30: 292–305. https://doi.org/10.1016/j.ijdrr.2018.03.004 doi: 10.1016/j.ijdrr.2018.03.004
![]() |
[3] | Pavelic P, Karthikeyan B, Giriraj A, et al. (2015) Controlling floods and droughts through underground storage: from concept to pilot implementation in the Ganges River Basin, International Water Management Institute (IWMI). |
[4] |
Choubin B, Malekian A (2017) Combined gamma and M-test-based ANN and ARIMA models for groundwater fluctuation forecasting in semiarid regions. Environ Earth Sci 76: 538. https://doi.org/10.1007/s12665-017-6870-8 doi: 10.1007/s12665-017-6870-8
![]() |
[5] |
Gopinath G, Seralathan P (2004) Identification of groundwater prospective zones using irs-id liss iii and pump test methods. J Indian Soc Remote Sens 32: 329–342. https://doi.org/10.1007/BF03030858 doi: 10.1007/BF03030858
![]() |
[6] |
Velis M, Conti KI, Biermann F (2017) Groundwater and human development: synergies and trade-offs within the context of the sustainable development goals. Sustain Sci 12: 1007–1017. https://doi.org/10.1007/s11625-017-0490-9 doi: 10.1007/s11625-017-0490-9
![]() |
[7] |
Okello C, Tomasello B, Greggio N, et al. (2015) Impact of Population Growth and Climate Change on the Freshwater Resources of Lamu Island, Kenya. Water 7: 1264–1290. https://doi.org/10.3390/w7031264 doi: 10.3390/w7031264
![]() |
[8] |
Ni B, Wang D, Deng Z, et al. (2018) Review on the Groundwater Potential Evaluation Based on Remote Sensing Technology. IOP Conf Ser Mater Sci Eng 394: 052038. https://doi.org/10.1088/1757-899X/394/5/052038 doi: 10.1088/1757-899X/394/5/052038
![]() |
[9] | Rao NS, Gugulothu S, Das R (2022) Deciphering artificial groundwater recharge suitability zones in the agricultural area of a river basin in Andhra Pradesh, India using geospatial techniques and analytical hierarchical process method. |
[10] |
Pathak D, Maharjan R, Maharjan N, et al. (2021) Evaluation of parameter sensitivity for groundwater potential mapping in the mountainous region of Nepal Himalaya. Groundwater Sustainable Dev 13: 100562. https://doi.org/10.1016/j.gsd.2021.100562 doi: 10.1016/j.gsd.2021.100562
![]() |
[11] |
Amfo-Otu R, Agyenim J, Nimba-Bumah G (2014) Correlation Analysis of Groundwater Colouration from Mountainous Areas, Ghana. Environ Res Eng Manage 1: 16–24. https://doi.org/10.5755/j01.erem.67.1.4545 doi: 10.5755/j01.erem.67.1.4545
![]() |
[12] |
Voeckler H, Allen DM (2012) Estimating regional-scale fractured bedrock hydraulic conductivity using discrete fracture network (DFN) modeling, Hydrogeol J 20: 1081–1100. https://doi.org/10.1007/s10040-012-0858-y doi: 10.1007/s10040-012-0858-y
![]() |
[13] |
Smerdon BD, Allen DM, Grasby SE, et al. (2009) An approach for predicting groundwater recharge in mountainous watersheds. J Hydrol 365: 156–172. https://doi.org/10.1016/j.jhydrol.2008.11.023 doi: 10.1016/j.jhydrol.2008.11.023
![]() |
[14] |
Elewa H, Qaddah A (2011) Groundwater potentiality mapping in the Sinai Peninsula, Egypt, using remote sensing and GIS-watershed-based modeling. Hydrogeol J 19: 613–628. https://doi.org/10.1007/s10040-011-0703-8 doi: 10.1007/s10040-011-0703-8
![]() |
[15] |
Elmahdy S (2012) Hydromorphological Mapping and Analysis for Characterizing Darfur Paleolake, NW Sudan Using Remote Sensing and GIS. Int J Geosci 3: 25–36. https://doi.org/10.4236/ijg.2012.31004 doi: 10.4236/ijg.2012.31004
![]() |
[16] |
Jagannathan K, Kumar NV, Jayaraman V, et al. (1996) An approach to demarcate Ground water potential zones through Remote Sensing and Geographic Information System. Int J Remote Sens 17: 1867–1884. https://doi.org/10.1080/01431169608948744 doi: 10.1080/01431169608948744
![]() |
[17] | Pande CB (2020) Sustainable Watershed Development Planning. In: Sustainable Watershed Development. SpringerBriefs in Water Science and Technology. Springer, Cham. https://doi.org/10.1007/978-3-030-47244-3 |
[18] |
Pande CB, Moharir KN, Singh SK, et al. (2022) Groundwater flow modeling in the basaltic hard rock area of Maharashtra, India. Appl Water Sci 12: 12. https://doi.org/10.1007/s13201-021-01525-y doi: 10.1007/s13201-021-01525-y
![]() |
[19] |
Saraf A, Choudhury P, Roy B, et al. (2004) GIS based surface hydrological modelling in identification of groundwater recharge zones, Int J Remote Sens 25: 5759–5770. https://doi.org/10.1080/0143116042000274096 doi: 10.1080/0143116042000274096
![]() |
[20] |
Swetha TV, Gopinath G, Thrivikramji KP (2017) Geospatial and MCDM tool mix for identification of potential groundwater prospects in a tropical river basin, Kerala. Environ Earth Sci 76: 428. https://doi.org/10.1007/s12665-017-6749-8 doi: 10.1007/s12665-017-6749-8
![]() |
[21] |
Bhadran A, Girishbai D, Jesiya NP, et al. (2022) A GIS based Fuzzy-AHP for delineating groundwater potential zones in tropical river basin, southern part of India. Geosyst Geoenviron 1: 100093. https://doi.org/10.1016/j.geogeo.2022.100093 doi: 10.1016/j.geogeo.2022.100093
![]() |
[22] |
Magesh NS, Chandrasekar N (2012) Soundranayagam, J.P. Delineation of groundwater potential zones in Theni district, Tamil Nadu, using remote sensing, GIS and MIF techniques. Geosci Front 3: 189–196, https://doi.org/10.1016/j.gsf.2011.10.007 doi: 10.1016/j.gsf.2011.10.007
![]() |
[23] |
Zghibi A, Mirchi A, Msaddek MH, et al. (2020) Using Analytical Hierarchy Process and Multi-Influencing Factors to Map Groundwater Recharge Zones in a Semi-Arid Mediterranean Coastal Aquifer. Water 12: 2525. https://doi.org/10.3390/w12092525 doi: 10.3390/w12092525
![]() |
[24] |
Çelik R (2019) Evaluation of Groundwater Potential by GIS-Based Multicriteria Decision Making as a Spatial Prediction Tool: Case Study in the Tigris River Batman-Hasankeyf Sub-Basin, Turkey. Water 11: 2630. https://doi.org/10.3390/w11122630 doi: 10.3390/w11122630
![]() |
[25] |
Aghlmand R, Abbasi A (2019) Application of MODFLOW with Boundary Conditions Analyses Based on Limited Available Observations: A Case Study of Birjand Plain in East Iran. Water 11: 1904. https://doi.org/10.3390/w11091904 doi: 10.3390/w11091904
![]() |
[26] |
Saiz-Rodríguez JA, Lomeli Banda MA, Salazar-Briones C, et al. (2019) Allocation of Groundwater Recharge Zones in a Rural and Semi-Arid Region for Sustainable Water Management: Case Study in Guadalupe Valley, Mexico. Water 11: 1586. https://doi.org/10.3390/w11081586 doi: 10.3390/w11081586
![]() |
[27] |
Amare S, Langendoen E, Keesstra S, et al. (2021) Susceptibility to Gully Erosion: Applying Random Forest (RF) and Frequency Ratio (FR) Approaches to a Small Catchment in Ethiopia. Water 13: 216. https://doi.org/10.3390/w13020216 doi: 10.3390/w13020216
![]() |
[28] |
Oh HJ, Kim YS, Choi JK, et al. (2011) GIS mapping of regional probabilistic groundwater potential in the area of Pohang City, Korea. J Hydrol 399: 158–172. https://doi.org/10.1016/j.jhydrol.2010.12.027 doi: 10.1016/j.jhydrol.2010.12.027
![]() |
[29] |
Saaty TL (1990) How to make a decision: The analytic hierarchy process. Eur J Oper Res 48: 9–26. https://doi.org/10.1016/0377-2217(90)90057-I doi: 10.1016/0377-2217(90)90057-I
![]() |
[30] |
Pande CB, Moharir KN, Panneerselvam B, et al. (2021) Delineation of groundwater potential zones for sustainable development and planning using analytical hierarchy process (AHP), and MIF techniques. Appl Water Sci 11: 186. https://doi.org/10.1007/s13201-021-01522-1 doi: 10.1007/s13201-021-01522-1
![]() |
[31] | Mirnazari J, Ahmad B, Mojaradi B., et al. (2014) Using Frequency Ratio Method for Spatial Landslide Prediction. Res J Appl Sci Eng Technol 7: 3174–3180. |
[32] | Trabelsi F, Lee S, Slaheddine K, et al. (2019) Frequency Ratio Model for Mapping Groundwater Potential Zones Using GIS and Remote Sensing; Medjerda Watershed Tunisia. In: Chaminé H, Barbieri M, Kisi O, et al. (eds), Advances in Sustainable and Environmental Hydrology, Hydrogeology, Hydrochemistry and Water Resources. CAJG 2018. Advances in Science, Technology & Innovation. Springer, Cham, 341–345. https://doi.org/10.1007/978-3-030-01572-5_80 |
[33] |
Tiwari A, Shoab M, Dixit A (2021) GIS-based forest fire susceptibility modeling in Pauri Garhwal, India: a comparative assessment of frequency ratio, analytic hierarchy process and fuzzy modeling techniques. Nat Hazards 105: 1189–1230. https://doi.org/10.1007/s11069-020-04351-8 doi: 10.1007/s11069-020-04351-8
![]() |
[34] |
Allafta H, Opp C, Patra S (2020) Identification of Groundwater Potential Zones Using Remote Sensing and GIS Techniques: A Case Study of the Shatt Al-Arab Basin. Remote Sens 13: 112. https://doi.org/10.3390/rs13010112 doi: 10.3390/rs13010112
![]() |
[35] |
Wood S, Charusiri P, Fenton C (2003) Recent paleoseismic investigations in Northern and Western Thailand. Ann Geophys 46. https://doi.org/10.4401/ag-3464 doi: 10.4401/ag-3464
![]() |
[36] | DGR (2001) Groundwater Map of Nan Province. |
[37] |
Rao NS (2009) A numerical scheme for groundwater development in a watershed basin of basement terrain: a case study from India. Hydrogeol J 17: 379–396. https://doi.org/10.1007/s10040-008-0402-2 doi: 10.1007/s10040-008-0402-2
![]() |
[38] | Rao NS (2012) Indicators for occurrence of groundwater in the rocks of Eastern Ghats. Curr Sci 103: 352–353. https://www.jstor.org/stable/24085075 |
[39] |
Saaty T (2008) Decision making with the Analytic Hierarchy Process. Int J Serv Sci 1: 83–98. https://doi.org/10.1504/IJSSCI.2008.017590 doi: 10.1504/IJSSCI.2008.017590
![]() |
[40] | Yahaya S, Ahmad N, Abdalla R (2010) Multicriteria analysis for flood vulnerable areas in Hadejia-Jama'are River basin, Nigeria. Eur J Sci Res 42: 1450–1216. |
[41] |
Maheswaran G, Selvarani AG, Elangovan K (2016) Groundwater resource exploration in salem district, Tamil nadu using GIS and remote sensing. J Earth Syst Sci 125: 311–328. https://doi.org/10.1007/s12040-016-0659-0 doi: 10.1007/s12040-016-0659-0
![]() |
[42] | Saaty T, Vargas L (2006) The Analytic Network Process, Decision Making with the Analytic Network Process, 195: 1–26. |
[43] | Saaty TL (1980) The Analytic Hierarchy Process; McGraw-Hill: New York. |
[44] |
Manap MA, Nampak H, Pradhan B, et al. (2014) Application of probabilistic-based frequency ratio model in groundwater potential mapping using remote sensing data and GIS. Arab J Geosci 7: 711–724. https://doi.org/10.1007/s12517-012-0795-z doi: 10.1007/s12517-012-0795-z
![]() |
[45] |
Razavi-Termeh SV, Sadeghi-Niaraki A, Choi SM (2019) Groundwater Potential Mapping Using an Integrated Ensemble of Three Bivariate Statistical Models with Random Forest and Logistic Model Tree Models. Water 11: 1596. https://doi.org/10.3390/w11081596 doi: 10.3390/w11081596
![]() |
[46] | Koyejo O, Natarajan N, Ravikumar P (2014) Consistent binary classification with generalized performance metrics. Adv Neural Inf Process Syst 3: 2744–2752. |
[47] | Bekkar M, Djema H, Alitouche TA (2013) Evaluation measures for models assessment over imbalanced data sets. J Inf Eng Appl 3: 27–38. |
[48] |
Saranya T, Saravanan S (2020) Groundwater potential zone mapping using analytical hierarchy process (AHP) and GIS for Kancheepuram District, Tamilnadu, India. Model Earth Syst Environ 6: 1105–1122. https://doi.org/10.1007/s40808-020-00744-7 doi: 10.1007/s40808-020-00744-7
![]() |
[49] |
Mohammadi-Behzad HR, Charchi A, Kalantari N, et al. (2019) Delineation of groundwater potential zones using remote sensing (RS), geographical information system (GIS) and analytic hierarchy process (AHP) techniques: a case study in the Leylia–Keynow watershed, southwest of Iran. Carbonates Evaporites 34: 1307–1319. https://doi.org/10.1007/s13146-018-0420-7 doi: 10.1007/s13146-018-0420-7
![]() |
[50] |
Yeh HF, Cheng YS, Lin HI, et al. (2016) Mapping groundwater recharge potential zone using a GIS approach in Hualian River, Taiwan. Sustainable Environ Res 26: 33–43. https://doi.org/10.1016/j.serj.2015.09.005 doi: 10.1016/j.serj.2015.09.005
![]() |
[51] |
Jahan CS, Rahaman MF, Arefin R, et al. (2019) Delineation of Groundwater Potential Zones of Atrai-Sib River Basin in North-West Bangladesh using Remote Sensing and GIS Techniques. Sustain Water Resour Manag 5: 689–702. https://doi.org/10.1007/s40899-018-0240-x doi: 10.1007/s40899-018-0240-x
![]() |
[52] |
Biswas S, Mukhopadhyay BP, Bera A (2020) Delineating groundwater potential zones of agriculture dominated landscapes using GIS based AHP techniques: a case study from Uttar Dinajpur district, West Bengal. Environ Earth Sci 79: 302. https://doi.org/10.1007/s12665-020-09053-9 doi: 10.1007/s12665-020-09053-9
![]() |
[53] |
Yıldırım Ü (2021) Identification of Groundwater Potential Zones Using GIS and Multi-Criteria Decision-Making Techniques: A Case Study Upper Coruh River Basin (NE Turkey). ISPRS Int J Geo-Inf 10: 396. https://doi.org/10.3390/ijgi10060396 doi: 10.3390/ijgi10060396
![]() |
[54] |
Benjmel K, Amraoui F, Boutaleb S, et al. (2020) Mapping of Groundwater Potential Zones in Crystalline Terrain Using Remote Sensing, GIS Techniques, and Multicriteria Data Analysis (Case of the Ighrem Region, Western Anti-Atlas, Morocco). Water 12: 471. https://doi.org/10.3390/w12020471 doi: 10.3390/w12020471
![]() |
[55] |
Maity DK, Mandal S (2019) Identification of groundwater potential zones of the Kumari river basin, India: an RS & GIS based semi-quantitative approach. Environ Dev Sustain 21: 1013–1034. https://doi.org/10.1007/s10668-017-0072-0 doi: 10.1007/s10668-017-0072-0
![]() |
[56] | Aouragh MH, Essahlaoui ALI, Abdelhadi O, et al. (2015) Using Remote Sensing and GIS-Multicriteria decision Analysis for Groundwater Potential Mapping in the Middle Atlas Plateaus, Morocco. Res J Recent Sci 4: 1–9. |
[57] |
Boughariou E, Allouche N, Brahim FB (2021) Delineation of groundwater potentials of Sfax region, Tunisia, using fuzzy analytical hierarchy process, frequency ratio, and weights of evidence models. Environ Dev Sustain 23: 14749–14774. https://doi.org/10.1007/s10668-021-01270-x doi: 10.1007/s10668-021-01270-x
![]() |
[58] |
Ahmadi H, Kaya OA, Babadagi E., et al. (2021) GIS-Based Groundwater Potentiality Mapping Using AHP and FR Models in Central Antalya, Turkey. Environ Sci Proc 5: 11. https://doi.org/10.3390/IECG2020-08741 doi: 10.3390/IECG2020-08741
![]() |
[59] |
Gautam P, Kubota T, Sapkota LM, et al. (2021) Landslide susceptibility mapping with GIS in high mountain area of Nepal: a comparison of four methods. Environ Earth Sci 80: 359. https://doi.org/10.1007/s12665-021-09650-2 doi: 10.1007/s12665-021-09650-2
![]() |
Data | Sources | Scale/Resolution | Thematic maps |
Digital Elevation | Alaska Satellite | Spatial resolution | -Drainage density |
Model (DEM) | Facility (ASF) | 12.5 m. | -Elevation |
-Slope | |||
Geologic map of Nan Province | Department of Mineral | 1: 250,000 | -Geologic map |
Resources (DMR) | |||
Satellite image | European Space | Spatial resolution | -Lineament density |
(Sentinel-2) | Agency (ESA) | 10 m. | -Land use and land Cover |
-Soil moisture |
Order | 1 | 2 | 3 | 4 | 5 | 6 | 7 | 8 | 9 | 10 |
R.I. | 0 | 0 | 0.52 | 0.89 | 1.11 | 1.25 | 1.35 | 1.40 | 1.45 | 1.49 |
Factors | EV | SL | DD | LD | SM | LULC | GG |
EV | 1.00 | 1/3 | 1/5 | 1/5 | 1/4 | 3.00 | 1/3 |
SL | 3.00 | 1.00 | 1/4 | 1/8 | 1/2 | 2.00 | 1/3 |
DD | 5.00 | 4.00 | 1.00 | 1.00 | 4.00 | 6.00 | 1.00 |
LD | 5.00 | 8.00 | 1.00 | 1.00 | 2.00 | 9.00 | 1.00 |
SM | 4.00 | 2.00 | 1/4 | 1/2 | 1.00 | 3.00 | 1/7 |
LULC | 1/3 | 1/2 | 1/6 | 1/9 | 1/3 | 1.00 | 1/4 |
GG | 3.00 | 3.00 | 1.00 | 1.00 | 7.00 | 4.00 | 1.00 |
Totals | 21.33 | 18.83 | 3.87 | 3.94 | 15.08 | 28.00 | 4.06 |
Criteria | Normalize weight | Class | Assigned rank | Feature normalized weight |
EV (m) | 0.0533 | 143–306 | 5 | 0.42 |
306–484 | 4 | 0.26 | ||
484–730 | 3 | 0.16 | ||
730–1078 | 2 | 0.10 | ||
1078–1884 | 1 | 0.06 | ||
SL (Degree) | 0.0681 | 0–7 | 5 | 0.42 |
7–13 | 4 | 0.26 | ||
13–20 | 3 | 0.16 | ||
20–29 | 2 | 0.10 | ||
29–72 | 1 | 0.06 | ||
DD (km/sq.km) | 0.2408 | 30–70 | 5 | 0.42 |
77–79 | 4 | 0.26 | ||
99–119 | 3 | 0.16 | ||
119–134 | 2 | 0.10 | ||
134–162 | 1 | 0.06 | ||
LD (km/sq.km) | 0.2675 | 0–0.42 | 1 | 0.06 |
0.42–0.81 | 2 | 0.10 | ||
0.81–1.22 | 3 | 0.16 | ||
1.22–1.74 | 4 | 0.26 | ||
1.74–2.80 | 5 | 0.42 | ||
SM | 0.0991 | Very–low | 1 | 0.06 |
Low | 2 | 0.10 | ||
Moderate | 3 | 0.16 | ||
High | 4 | 0.26 | ||
Very–high | 5 | 0.42 | ||
LULC | 0.0333 | Building | 3 | 0.10 |
Bare Soil | 2 | 0.16 | ||
Vegetation | 4 | 0.28 | ||
Water | 5 | 0.47 | ||
GG | 0.2380 | Qa | 5 | 0.19 |
Tr1 | 4 | 0.13 | ||
CP | 3 | 0.08 | ||
JKpw | 3 | 0.08 | ||
Png2 | 3 | 0.08 | ||
TRJ | 3 | 0.08 | ||
Trgr | 2 | 0.05 | ||
PTr | 2 | 0.05 | ||
JPK | 2 | 0.05 | ||
Tmm | 2 | 0.05 | ||
Jv | 1 | 0.03 | ||
Ptru | 1 | 0.03 | ||
Pr-2 | 1 | 0.03 | ||
Tr2 | 1 | 0.03 | ||
Trwc | 1 | 0.03 |
Criteria | Sub-class | No. of pixels | Percentage of sub-class | No. of wells | Percentage of wells | FR | Weight |
EV(m) | 143–306 | 2343787 | 44.03 | 21 | 100 | 2.27 | 1.994 |
306–484 | 1637049 | 30.75 | 0 | 0 | 0.00 | ||
484–730 | 819445 | 15.39 | 0 | 0 | 0.00 | ||
730–1078 | 359929 | 6.76 | 0 | 0 | 0.00 | ||
1078–1884 | 163044 | 3.06 | 0 | 0 | 0.00 | ||
SL(Degree) | 0–7 | 1537601 | 28.88 | 21.00 | 100.00 | 3.46 | 1994 |
7–13 | 1236124 | 23.22 | 0.00 | 0.00 | 0.00 | ||
13–20 | 1252052 | 23.52 | 0.00 | 0.00 | 0.00 | ||
20–29 | 951705 | 17.88 | 0.00 | 0.00 | 0.00 | ||
29–72 | 345771 | 6.50 | 0.00 | 0.00 | 0.00 | ||
DD | 30–70 | 1155033 | 21.78 | 0.00 | 0.00 | 0.00 | 1.000 |
(km/sq.km) | 77–79 | 2203154 | 41.54 | 4.00 | 19.05 | 0.46 | |
99–119 | 1345062 | 25.36 | 3.00 | 14.29 | 0.56 | ||
119–134 | 499485 | 9.42 | 12.00 | 57.14 | 6.07 | ||
134–162 | 100851 | 1.90 | 2.00 | 9.52 | 5.01 | ||
LD (km/sq.km) | 0–0.42 | 1092610 | 20.60 | 0.00 | 0.00 | 0.00 | 1.579 |
0.42–0.81 | 1586183 | 29.91 | 3.00 | 14.29 | 0.48 | ||
0.81–1.22 | 1493621 | 28.16 | 17.00 | 80.95 | 2.87 | ||
1.22–1.74 | 906948 | 17.10 | 1.00 | 4.76 | 0.28 | ||
1.74–2.80 | 224344 | 4.23 | 0.00 | 0.00 | 0.00 | ||
SM | Very–low | 555729 | 10.48 | 2.00 | 9.52 | 0.91 | 1.219 |
Low | 663175 | 12.51 | 12.00 | 57.14 | 4.57 | ||
Moderate | 825390 | 15.56 | 6.00 | 28.57 | 1.84 | ||
High | 1590995 | 30.00 | 1.00 | 4.76 | 0.16 | ||
Very–high | 1667896 | 31.45 | 0.00 | 0.00 | 0.00 | ||
LULC | Building | 182961 | 3.45 | 16.00 | 76.19 | 22.08 | 1.951 |
Bare Soil | 1090802 | 20.57 | 1.00 | 4.76 | 0.23 | ||
Vegetation | 3999858 | 75.42 | 4.00 | 19.05 | 0.25 | ||
Water | 29504 | 0.56 | 0.00 | 0.00 | 0.00 |
AHP method | FR method | ||||
Class | Area (km2) | Area (%) | Area (km2) | Area (%) | |
Very–low | 131.60 | 3.99 | 367.97 | 11.15 | |
Low | 404.99 | 12.27 | 1835.53 | 55.61 | |
High | 677.94 | 20.55 | 955.27 | 28.94 | |
Very–high | 2084.81 | 63.19 | 142.10 | 4.30 |
Data | Sources | Scale/Resolution | Thematic maps |
Digital Elevation | Alaska Satellite | Spatial resolution | -Drainage density |
Model (DEM) | Facility (ASF) | 12.5 m. | -Elevation |
-Slope | |||
Geologic map of Nan Province | Department of Mineral | 1: 250,000 | -Geologic map |
Resources (DMR) | |||
Satellite image | European Space | Spatial resolution | -Lineament density |
(Sentinel-2) | Agency (ESA) | 10 m. | -Land use and land Cover |
-Soil moisture |
Order | 1 | 2 | 3 | 4 | 5 | 6 | 7 | 8 | 9 | 10 |
R.I. | 0 | 0 | 0.52 | 0.89 | 1.11 | 1.25 | 1.35 | 1.40 | 1.45 | 1.49 |
Factors | EV | SL | DD | LD | SM | LULC | GG |
EV | 1.00 | 1/3 | 1/5 | 1/5 | 1/4 | 3.00 | 1/3 |
SL | 3.00 | 1.00 | 1/4 | 1/8 | 1/2 | 2.00 | 1/3 |
DD | 5.00 | 4.00 | 1.00 | 1.00 | 4.00 | 6.00 | 1.00 |
LD | 5.00 | 8.00 | 1.00 | 1.00 | 2.00 | 9.00 | 1.00 |
SM | 4.00 | 2.00 | 1/4 | 1/2 | 1.00 | 3.00 | 1/7 |
LULC | 1/3 | 1/2 | 1/6 | 1/9 | 1/3 | 1.00 | 1/4 |
GG | 3.00 | 3.00 | 1.00 | 1.00 | 7.00 | 4.00 | 1.00 |
Totals | 21.33 | 18.83 | 3.87 | 3.94 | 15.08 | 28.00 | 4.06 |
Criteria | Normalize weight | Class | Assigned rank | Feature normalized weight |
EV (m) | 0.0533 | 143–306 | 5 | 0.42 |
306–484 | 4 | 0.26 | ||
484–730 | 3 | 0.16 | ||
730–1078 | 2 | 0.10 | ||
1078–1884 | 1 | 0.06 | ||
SL (Degree) | 0.0681 | 0–7 | 5 | 0.42 |
7–13 | 4 | 0.26 | ||
13–20 | 3 | 0.16 | ||
20–29 | 2 | 0.10 | ||
29–72 | 1 | 0.06 | ||
DD (km/sq.km) | 0.2408 | 30–70 | 5 | 0.42 |
77–79 | 4 | 0.26 | ||
99–119 | 3 | 0.16 | ||
119–134 | 2 | 0.10 | ||
134–162 | 1 | 0.06 | ||
LD (km/sq.km) | 0.2675 | 0–0.42 | 1 | 0.06 |
0.42–0.81 | 2 | 0.10 | ||
0.81–1.22 | 3 | 0.16 | ||
1.22–1.74 | 4 | 0.26 | ||
1.74–2.80 | 5 | 0.42 | ||
SM | 0.0991 | Very–low | 1 | 0.06 |
Low | 2 | 0.10 | ||
Moderate | 3 | 0.16 | ||
High | 4 | 0.26 | ||
Very–high | 5 | 0.42 | ||
LULC | 0.0333 | Building | 3 | 0.10 |
Bare Soil | 2 | 0.16 | ||
Vegetation | 4 | 0.28 | ||
Water | 5 | 0.47 | ||
GG | 0.2380 | Qa | 5 | 0.19 |
Tr1 | 4 | 0.13 | ||
CP | 3 | 0.08 | ||
JKpw | 3 | 0.08 | ||
Png2 | 3 | 0.08 | ||
TRJ | 3 | 0.08 | ||
Trgr | 2 | 0.05 | ||
PTr | 2 | 0.05 | ||
JPK | 2 | 0.05 | ||
Tmm | 2 | 0.05 | ||
Jv | 1 | 0.03 | ||
Ptru | 1 | 0.03 | ||
Pr-2 | 1 | 0.03 | ||
Tr2 | 1 | 0.03 | ||
Trwc | 1 | 0.03 |
Criteria | Sub-class | No. of pixels | Percentage of sub-class | No. of wells | Percentage of wells | FR | Weight |
EV(m) | 143–306 | 2343787 | 44.03 | 21 | 100 | 2.27 | 1.994 |
306–484 | 1637049 | 30.75 | 0 | 0 | 0.00 | ||
484–730 | 819445 | 15.39 | 0 | 0 | 0.00 | ||
730–1078 | 359929 | 6.76 | 0 | 0 | 0.00 | ||
1078–1884 | 163044 | 3.06 | 0 | 0 | 0.00 | ||
SL(Degree) | 0–7 | 1537601 | 28.88 | 21.00 | 100.00 | 3.46 | 1994 |
7–13 | 1236124 | 23.22 | 0.00 | 0.00 | 0.00 | ||
13–20 | 1252052 | 23.52 | 0.00 | 0.00 | 0.00 | ||
20–29 | 951705 | 17.88 | 0.00 | 0.00 | 0.00 | ||
29–72 | 345771 | 6.50 | 0.00 | 0.00 | 0.00 | ||
DD | 30–70 | 1155033 | 21.78 | 0.00 | 0.00 | 0.00 | 1.000 |
(km/sq.km) | 77–79 | 2203154 | 41.54 | 4.00 | 19.05 | 0.46 | |
99–119 | 1345062 | 25.36 | 3.00 | 14.29 | 0.56 | ||
119–134 | 499485 | 9.42 | 12.00 | 57.14 | 6.07 | ||
134–162 | 100851 | 1.90 | 2.00 | 9.52 | 5.01 | ||
LD (km/sq.km) | 0–0.42 | 1092610 | 20.60 | 0.00 | 0.00 | 0.00 | 1.579 |
0.42–0.81 | 1586183 | 29.91 | 3.00 | 14.29 | 0.48 | ||
0.81–1.22 | 1493621 | 28.16 | 17.00 | 80.95 | 2.87 | ||
1.22–1.74 | 906948 | 17.10 | 1.00 | 4.76 | 0.28 | ||
1.74–2.80 | 224344 | 4.23 | 0.00 | 0.00 | 0.00 | ||
SM | Very–low | 555729 | 10.48 | 2.00 | 9.52 | 0.91 | 1.219 |
Low | 663175 | 12.51 | 12.00 | 57.14 | 4.57 | ||
Moderate | 825390 | 15.56 | 6.00 | 28.57 | 1.84 | ||
High | 1590995 | 30.00 | 1.00 | 4.76 | 0.16 | ||
Very–high | 1667896 | 31.45 | 0.00 | 0.00 | 0.00 | ||
LULC | Building | 182961 | 3.45 | 16.00 | 76.19 | 22.08 | 1.951 |
Bare Soil | 1090802 | 20.57 | 1.00 | 4.76 | 0.23 | ||
Vegetation | 3999858 | 75.42 | 4.00 | 19.05 | 0.25 | ||
Water | 29504 | 0.56 | 0.00 | 0.00 | 0.00 |
AHP method | FR method | ||||
Class | Area (km2) | Area (%) | Area (km2) | Area (%) | |
Very–low | 131.60 | 3.99 | 367.97 | 11.15 | |
Low | 404.99 | 12.27 | 1835.53 | 55.61 | |
High | 677.94 | 20.55 | 955.27 | 28.94 | |
Very–high | 2084.81 | 63.19 | 142.10 | 4.30 |