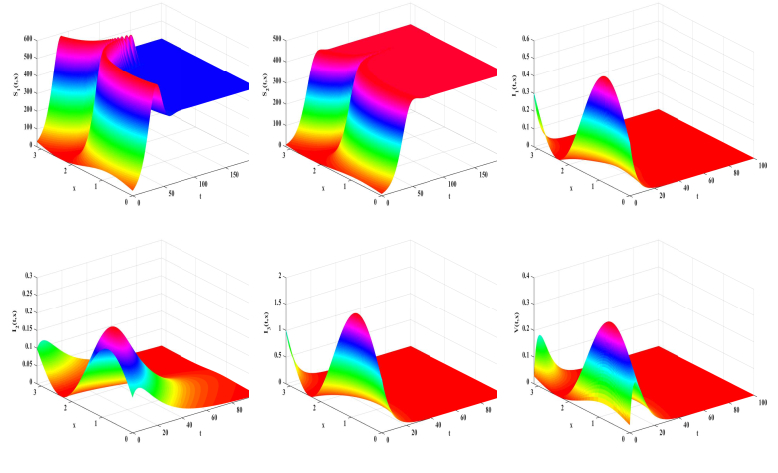
In this study, we formulate a reaction-diffusion Zika model which incorporates vector-bias, environmental transmission and spatial heterogeneity. The main question of this paper is the analysis of the threshold dynamics. For this purpose, we establish the mosquito reproduction number R1 and basic reproduction number R0. Then, we analyze the dynamical behaviors in terms of R1 and R0. Numerically, we find that the ignorance of the vector-bias effect will underestimate the infection risk of the Zika disease, ignorance of the spatial heterogeneity effect will overestimate the infection risk, and the environmental transmission is indispensable.
Citation: Liping Wang, Xinyu Wang, Dajun Liu, Xuekang Zhang, Peng Wu. Dynamical analysis of a heterogeneous spatial diffusion Zika model with vector-bias and environmental transmission[J]. Electronic Research Archive, 2024, 32(2): 1308-1332. doi: 10.3934/era.2024061
[1] | Xiang Zhang, Tingting Zheng, Yantao Luo, Pengfei Liu . Analysis of a reaction-diffusion AIDS model with media coverage and population heterogeneity. Electronic Research Archive, 2025, 33(1): 513-536. doi: 10.3934/era.2025024 |
[2] | Xiangyun Shi, Dan Zhou, Xueyong Zhou, Fan Yu . Predicting the trend of leptospirosis in China via a stochastic model with vector and environmental transmission. Electronic Research Archive, 2024, 32(6): 3937-3951. doi: 10.3934/era.2024176 |
[3] | Ziang Chen, Chunguang Dai, Lei Shi, Gaofang Chen, Peng Wu, Liping Wang . Reaction-diffusion model of HIV infection of two target cells under optimal control strategy. Electronic Research Archive, 2024, 32(6): 4129-4163. doi: 10.3934/era.2024186 |
[4] | Jiani Jin, Haokun Qi, Bing Liu . Hopf bifurcation induced by fear: A Leslie-Gower reaction-diffusion predator-prey model. Electronic Research Archive, 2024, 32(12): 6503-6534. doi: 10.3934/era.2024304 |
[5] | Nan Xiang, Aying Wan, Hongyan Lin . Diffusion-driven instability of both the equilibrium solution and the periodic solutions for the diffusive Sporns-Seelig model. Electronic Research Archive, 2022, 30(3): 813-829. doi: 10.3934/era.2022043 |
[6] | Wenxuan Li, Suli Liu . Dynamic analysis of a stochastic epidemic model incorporating the double epidemic hypothesis and Crowley-Martin incidence term. Electronic Research Archive, 2023, 31(10): 6134-6159. doi: 10.3934/era.2023312 |
[7] | Zhili Zhang, Aying Wan, Hongyan Lin . Spatiotemporal patterns and multiple bifurcations of a reaction- diffusion model for hair follicle spacing. Electronic Research Archive, 2023, 31(4): 1922-1947. doi: 10.3934/era.2023099 |
[8] | Ting-Ying Chang, Yihong Du . Long-time dynamics of an epidemic model with nonlocal diffusion and free boundaries. Electronic Research Archive, 2022, 30(1): 289-313. doi: 10.3934/era.2022016 |
[9] | Gaohui Fan, Ning Li . Application and analysis of a model with environmental transmission in a periodic environment. Electronic Research Archive, 2023, 31(9): 5815-5844. doi: 10.3934/era.2023296 |
[10] | Yike Lv, Xinzhu Meng . Evolution of infectious diseases induced by epidemic prevention publicity and interaction between heterogeneous strains. Electronic Research Archive, 2024, 32(8): 4858-4886. doi: 10.3934/era.2024223 |
In this study, we formulate a reaction-diffusion Zika model which incorporates vector-bias, environmental transmission and spatial heterogeneity. The main question of this paper is the analysis of the threshold dynamics. For this purpose, we establish the mosquito reproduction number R1 and basic reproduction number R0. Then, we analyze the dynamical behaviors in terms of R1 and R0. Numerically, we find that the ignorance of the vector-bias effect will underestimate the infection risk of the Zika disease, ignorance of the spatial heterogeneity effect will overestimate the infection risk, and the environmental transmission is indispensable.
Zika, caused by the Zika virus, is a mosquito borne disease. It is mainly transmitted to humans through mosquito bites. In March 2015, a large outbreak in Brazil attracted worldwide attention. The World Health Organization stated Zika as a Public Health Emergency of International Concern in February 2016 [1]. Zika is associated with Guillain-Barrˊe syndrome and microcephaly [2]. It poses a major threat to global health in developing countries [3].
Differential equation models are an excellent tool in studying the spread of infectious diseases [4,5,6,7,8,9,10,11]. Recently, reaction-diffusion models play an important role in exploring the effects of spatial heterogeneity on the spread of Zika [12,13,14,15,16,17,18,19]. Recently, researches showed that the Zika virus in a water environment could be transmitted to aquatic mosquitoes via breeding [20]. This suggested that determination of the route of Zika transmission needs to take environmental factors into account. In our previous article [21], the environmental transmission route was introduced into Zika model. We researched dynamical analysis of the system for three incidence functions related to the environmental transmission rate. Then, we extended [21] to consider sexual transmissions and spatial heterogeneity [22,23]. But, the above models did not consider the vector-bias. In fact, the vector-bias is important for Zika transmission [24,25]. However, few Zika models consider the vector-bias, environmental transmission, and spatial heterogeneity simultaneously.
The vector-bias describes how mosquitoes prefer to bite infected people over susceptible ones. To account for the vector-bias in the model, we introduce the parameters p and l. p and l represent the probabilities that an adult mosquito arrives at a human at random and bites the human if he/she is infectious and susceptible, respectively. Let q=pl (q≥1). Then q is called a vector-bias parameter. In this study, we modify and add the vector-bias to our previous model in [23]. We assume that the mosquitoes and humans are living in a bounded domain Γ with smooth boundary ∂Γ. S1(t,x) and I1(t,x) indicate densities of susceptible and infectious aquatic mosquitoes at time t and position x, respectively. S2(t,x) and I2(t,x) represent densities of susceptible and infectious adult mosquitoes at time t and position x, respectively. The densities of infectious humans at time t and position x are denoted by I3(t,x). The densities of the Zika virus in the water environment are denoted by V(t,x) at time t and position x. We assume that a susceptible human is unchanging [19,24]. The density of susceptible humans is denoted by H∗(x). Inspired by article [24], we set the number of newly infectious adult mosquitoes and humans per unit time to α2(x)pI3pI3+lH∗(x)S2 and α3(x)lH∗(x)pI3+lH∗(x)I2, respectively. α2(x) denotes the transmission rate from I3 to S2. α3(x) denotes the transmission rate from I2 to S3. Then, we propose a reaction-diffusion Zika model as follows
{∂S1∂t=τ(x)(S2+I2)(1−S1+I1K1(x))−α1(x)VK2(x)+VS1−ω(x)S1−δ1(x)S1,x∈Γ,∂I1∂t=α1(x)VK2(x)+VS1−ω(x)I1−δ1(x)I1,x∈Γ,∂S2∂t=ω(x)S1−α2(x)pI3pI3+lH∗(x)S2−δ2(x)S2+∇⋅(d1(x)∇S2),x∈Γ,∂I2∂t=ω(x)I1+α2(x)pI3pI3+lH∗(x)S2−δ2(x)I2+∇⋅(d1(x)∇I2),x∈Γ,∂I3∂t=α3(x)lH∗(x)pI3+lH∗(x)I2−γ(x)I3+∇⋅(d2(x)∇I3),x∈Γ,∂V∂t=η(x)I3−ϱ(x)V,x∈Γ.∂S2∂n=∂I2∂n=∂I3∂n=0,x∈∂Γ,S1(0,x)=S10(x),I1(0,x)=I10(x),S2(0,x)=S20(x),x∈Γ,I2(0,x)=I20(x),I3(0,x)=I30(x),V(0,x)=V0(x),x∈Γ, | (1.1) |
for t>0. τ(x) represents the birth rate of susceptible aquatic mosquitoes. K1(x) represents the maximal capacity of aquatic mosquitoes in the water environment. α1(x) denotes the transmission rate from V to S1. K2(x) denotes the half-saturation constant, which can cause a 50% chance of catching the Zika virus. ω(x) denotes the maturity rate of aquatic mosquitoes. δ1(x) and δ2(x) denote the death rate of aquatic and adult mosquitoes, respectively. γ(x) represents the recovery and death rate of infected humans. The rate of excreting the Zika virus for each infected human is denoted by η(x). The clearance rate of V(x,t) is denoted by ϱ(x). d1(x) and d2(x) represent the diffusion coefficients of adult mosquitoes and humans. Here we employ the Neumann boundary condition ∂U∂n=0, (U=S2,I2,I3), where n represents the outward unit normal vector on ∂Γ. Assume that τ(x), K1(x), K2(x), α1(x), α2(x), α3(x), ω(x), δ1(x), δ2(x), γ(x), η(x), ϱ(x), d1(x), d2(x), and H∗(x) are continuous and positive functions of x.
The remainder of this paper is organized as follows: In the next section, we give the well-posedness of system (1.1). In Section 3, the mosquito reproduction number R1 and basic reproduction number R0 will be established. In Section 4, threshold dynamical behaviors are analyzed. In Section 5, we conduct some numerical simulations. This study ends with a brief conclusion.
Let G:=C(ˉΓ,R6) be a Banach space with the supremum norm ∥⋅∥G. Let G+:=C(ˉΓ,R6+). We then have that (G,G+) is a strongly ordered Banach space. Denote
GK:={θ=(θ1,θ2,θ3,θ4,θ5,θ6,θ6)T∈G+:0≤θ1(x)+θ2(x)≤K1(x),∀x∈ˉΓ}. |
Throughout, we denote ¯g:=maxx∈ˉΩg(x), g_:=minx∈ˉΩg(x).
Set F:=C(ˉΓ,R) and F+:=C(ˉΓ,R+). Assume that Υ2(t) and Υ3(t) : F→F+ are the evolution operators associated with
∂v2∂t=−δ2(x)v2+∇⋅(d1(x)∇v2),x∈Γ,∂v3∂t=−γ(x)v3+∇⋅(d2(x)∇v3),x∈Γ,∂v2∂n=∂v3∂n=0,x∈∂Γ. |
We have that Υ2(t) and Υ3(t) are strongly positive and compact (see Chapter Ⅱ in [26] and Theorems 7.3.1 and 7.4.1 in [27]). According to Subsection 2.1 in [28], one has
(Υi(t)ψ)(x)=∫ΓFi(t,x,y)ψ(y)dy,i=2,3, |
for t≥0, ψ∈F. Here, F2(t,x,y) and F3(t,x,y) are the Green functions associated with −δ2(x)v2+∇⋅(d1(x)∇v2) and −γ(x)v3+∇⋅(d2(x)∇v3) subject to the Neumann boundary condition, respectively.
Let a1 and a2 be the principle eigenvalue of −δ2(x)v2+∇⋅(d1(x)∇v2) and −γ(x)v3+∇⋅(d2(x)∇v3) subject to the Neumann boundary condition, respectively. From [28], we obtain that there is M>0 such that ∥Υi(t)∥≤Meait ∀t≥0, i=2,3. According to Theorem 2.27 in [29], one has that there exists some b2>0 and b3>0 such that F2(t,x,y)≤b2e−δ2_t and F3(t,x,y)≤b3e−γ_t.
Define Υ1(t) and Υ4(t) : F→F+ by
Υ1(t)ψ(x)=e−(ω(x)+δ1(x))tψ(x),Υ4(t)ψ(x)=e−ϱ(x)tψ(x). |
Denote Υ= diag(Υ1(t),Υ1(t),Υ2(t),Υ2(t),Υ3(t),Υ4(t)). Define H=(H1,H2,H3,H4,H5,H6):GK→G by
H1(θ)=τ(⋅)(θ3+θ4)(1−θ1+θ2K1(⋅))−α1(⋅)θ6K2(⋅)+θ6θ1,H2(θ)=α1(⋅)θ6K2(⋅)+θ6θ1,H3(θ)=ω(⋅)θ1−α2(⋅)pθ5θ3pθ5+lH∗(⋅),H4(θ)=ω(⋅)θ2+α2(⋅)pθ5θ3pθ5+lH∗(⋅),H5(θ)=α3(⋅)lH∗(⋅)θ4pθ5+lH∗(⋅),H6(θ)=η(⋅)θ5, |
for t≥0,x∈ˉΓ, and θ=(θ1,θ2,θ3,θ4,θ5,θ6)∈GK. Then, rewrite system (1.1) as
{dwdt=Bw+H(w),t>0,w(0)=θ∈GK, | (2.1) |
where B= diag (B1,B1,B2,B2,B3,B4) and Bj(j=1,2,3,4) are given by
D(Bi)={θ∈C2(ˉΓ)},i=1,4,D(Bj)={θ∈C2(ˉΓ):∂θ∂n=0on∂Γ},j=2,3,B1θ=−(ω(x)+δ1(x))θ,θ∈D(B1),B2θ=−δ2(x)θ+∇⋅(d1(x)∇θ),θ∈D(B2),B3θ=−γ(x)θ+∇⋅(d2(x)∇θ),θ∈D(B3),B4θ=−ϱ(x)θ,θ∈D(B4). |
System (1.1) is also equivalent to an integral equation as follows:
{w(t,θ)=Υ(t)θ+∫t0Υ(t−s)H(w(s,⋅))ds,t>0,w(0)=θ∈GK, |
where w:=(S1,I1,S2,I2,I3,V).
Lemma 2.1. For every θ∈GK, system (1.1) admits a unique nonnegative solution w(t,⋅,θ)∈GK on [0,∞) with w(0,⋅,θ)=θ. Moreover, the solution is uniformly bounded and ultimately bounded.
Proof. For any θ∈GK and c>0, then one has
θ(x)+cH(θ)(x)=(θ1(x)+cτ(x)(θ3(x)+θ4(x))(1−θ1(x)+θ2(x)K1(x))−cα1(x)θ6(x)K2(x)+θ6(x)θ1(x)θ2(x)+cα1(x)θ6(x)K2(x)+θ6(x)θ1(x)θ3(x)+cω(x)θ1(x)−cα2(x)pθ5(x)θ3(x)pθ5(x)+lH∗(x)θ4(x)+ω(x)θ2(x)+cα2(x)pθ5(x)θ3(x)pθ5(x)+lH∗(x)θ5(x)+cα3(x)lH∗(x)θ4(x)pθ5(x)+lH∗(x)θ6(x)+cη(x)θ5(x))≥(θ1(x)(1−c¯α1K2_θ6(x))θ2(x)θ3(x)(1−c¯α2plH∗_θ5(x))θ4(x)θ5(x)θ6(x)), |
and
K1(x)−(θ1(x)+cH1(θ)(x)+θ2(x)+cH2(θ)(x)=K1(x)−(θ1(x)+θ2(x)+cτ(x)(θ3(x)+θ4(x))(1−θ1(x)+θ2(x)K1(x)))=(K1(x)−(θ1(x)+θ2(x))(1−cτ(x)(θ3(x)+θ4(x))K1(x)). | (2.2) |
So, for small enough c>0, one has θ+cH(θ)∈GK, and
limc→0+1cdist(θ+cH(θ),GK)=0,∀θ∈GK. |
By Corollary 4 in [30], we can obtain that for any t in its maximal existence interval [0,tθ) with tθ≤∞, system (1.1) has a unique mild solution w(t,⋅,θ) with w(0,⋅,θ)=θ, and w(0,⋅,θ)∈GK. Moreover, w(t,⋅,θ)∈GK ∀ t∈[0,tθ). w(t,⋅,θ) is a classical solution.
Let M1(t,x)=S1(t,x)+I1(t,x), M2(t,x)=S2(t,x)+I2(t,x). From (1.1), one has that (M1(t,x),M2(t,x)) satisfies
{∂M1∂t=τ(x)M2(1−M1K1(x))−(ω(x)+δ1(x))M1,x∈Γ,∂M2∂t=ω(x)M1−δ2(x)M2+∇⋅(d1(x)∇M2),x∈Γ,∂M2∂n=0,x∈∂Γ, | (2.3) |
for t>0. Since w(t,⋅,θ)∈GK ∀ t∈[0,tθ), we have M1(t,x)=S1(t,x)+I1(t,x)≤K1(x) for t∈[0,tθ), x∈ˉΓ. That is, S1(t,⋅) and I1(t,⋅) are bounded on [0,tθ). From the second equation of (2.3), we have
{∂M2∂t≤ω(x)K1(x)−δ2(x)M2+∇⋅(d1(x)∇M2),≤¯ω¯K1−δ2_M2+∇⋅(d1(x)∇M2),x∈Γ,∂M2∂n=0,x∈∂Γ. | (2.4) |
Consider a comparison system as follows
{∂v∂t=¯ω¯K1−δ2_v+∇⋅(d1(x)∇v),x∈Γ,∂v∂n=0,x∈∂Γ. | (2.5) |
Let ι=¯ω¯K1δ2_. We then have that ∂ι∂t−∇⋅(d1(x)∇ι)−(¯ω¯K1−δ2_ι)≥0. Therefore, ι is an upper solution of (2.5). By the comparison principle, we have M2(t,x)≤ι for t∈[0,tθ), x∈ˉΓ. That is, S2(t,⋅) and I2(t,⋅) are bounded on [0,tθ).
From the fifth equation of (1.1), one has
{∂I3∂t≤¯α3ι−γ_I3+∇⋅(d2(x)∇I3),x∈Γ,∂I2∂n=0,x∈∂Γ. |
It follows from the comparison principle that I3(t,x) is bounded on [0,tθ). So, there exists a positive constant D and along with the sixth equation of (1.1), we have ∂V∂t≤¯ηD−ϱ_V. By the comparison principle, we can obtain that V(t,x) is bounded on [0,tθ). Therefore, for any initial value θ∈GK, solutions of system (1.1) exist globally on [0,+∞).
Since S1(t,x)+I1(t,x)≤K1(x) for x∈ˉΓ,t≥0, we have that S1(t,x) and I1(t,x) are ultimately bounded. From the third equation of (1.1), we have
{∂S2∂t≤¯ω¯K1−δ2_S2+∇⋅(d1(x)∇S2),x∈Γ,∂S2∂n=0,x∈∂Γ. |
According to the comparison principle, S2(t,x) is ultimately bounded. So, there exists t0 such that S2(t,x)≤2¯ω¯K1δ2_=2ι for t>t0, x∈ˉΓ.
From the fourth equation of (1.1), one has
{∂I2∂t≤¯ω¯K1+2ι¯α2−δ2_I2+∇⋅(d1(x)∇I2),x∈Γ,∂I2∂n=0,x∈∂Γ. |
So, I2(t,x) is ultimately bounded. There exists t01 such that I2(t,x)≤2¯ω¯K1+2ι¯α2δ2_ for t>t01, x∈ˉΓ.
From the fifth equation of (1.1), one has
{∂I3∂t≤¯α3ι1−γ_I3+∇⋅(d2(x)∇I3),x∈Γ,∂I2∂n=0,x∈∂Γ, |
where ι1=2¯ω¯K1+2ι¯α2δ2_. According to the comparison principle, I3(t,x) is ultimately bounded. So, there exists t02 such that I3(t,x)≤2¯α3ι1γ_ for t>t02, x∈ˉΓ. Similarly, we can get that V(t,x) is ultimately bounded. Thus, the solution w(t,x) is ultimately bounded.
Next, we give the proof of the uniform boundedness of solutions for system (1.1).
For any θ∈GK, one has θ1(x)+θ2(x)≤K1(x), ∀x∈ˉΓ, and the solution of system (1.1) w(t,⋅,θ)∈GK. Thus, w1(t,⋅,θ)+w2(t,⋅,θ)≤K1(⋅). That is, S1(t,x) and I1(t,x) are uniformly bounded.
From the third equation of (1.1), we have
{∂S2∂t≤ω(x)K1(x)−δ2(x)S2+∇⋅(d1(x)∇S2),x∈Γ,∂S2∂n=0,x∈∂Γ. |
Then, for any initial value θ∈GK, we can obtain
S2(t,x)≤Υ2(t)θ3(x)+∫t0Υ2(t−s)ω(x)K1(x)ds≤Mea2t∥θ3∥F+∫t0∫ΓF2(t−s,x,y)ω(y)K1(y)dyds≤Mea2t∥θ3∥F+∫t0b2e−δ2_(t−s)¯ω¯K1∣Γ∣ds≤M∥θ3∥F+b2¯ω¯K1∣Γ∣δ2_. |
For any c1>0, choose N(c1)=Mc1+b2¯ω¯K1∣Γ∣δ2_>0. Then for any initial value ∥θ∥G≤c1 and ∀t≥0, we have S2(t,x)≤N(c1). So, S2(t,x) is uniformly bounded. Similarly, for any c2>0, choose N(c2)=Mc2+b2(¯ω¯K1+¯α2N(c1))∣Γ∣δ2_>0, then, for any initial value ∥θ∥G≤c2 and ∀t≥0, we have I2(t,x)≤N(c2). So, I2(t,x) is uniformly bounded. From the fifth equation of (1.1), for any c3>0, choose N(c3)=Mc3+b3¯α3N(c2)∣Γ∣γ_>0, then, for any initial value ∥θ∥G≤c3 and ∀t≥0, we have I3(t,x)≤N(c3). So, we can obtain that I3(t,x) is uniformly bounded. From the last equation of (1.1), we can obtain V(t,x)≤e−ρ_t∥θ6∥F+¯ηN(c3)∣Γ∣ρ_(1−e−ρ_t)≤∥θ6∥F+¯ηN(c3)∣Γ∣ρ_. for any c4>0, we choose N(c4)=c4+¯ηN(c3)∣Γ∣ρ_ such that V(t,x)≤N(c4) for any initial value ∥θ∥G≤c4 and ∀t≥0. Thus, V(t,x) is uniformly bounded. In short, the solution w(t,x) of system (1.1) is uniformly bounded. This completes the proof of Lemma 2.1.
In this section, the mosquito reproduction number R1 and basic reproduction number R0 will be established though applying the theorem in article [31].
Linearizing system (2.3) at (0,0), we can get
{∂M1∂t=τ(x)M2+(ω(x)+δ1(x))M1,x∈Γ,t>0,∂M2∂t=ω(x)M1−δ2(x)M2+∇⋅(d1(x)∇M2),x∈Γ,t>0,∂M2∂n=0,x∈∂Γ. | (3.1) |
Define P1 as
P1(x)=(−(ω(x)+δ1(x))τ(x)ω(x)δ2(x)+∇⋅(d1(x)∇)). | (3.2) |
In addition, the eigenvalue problem of system (3.1) is given by
{τ(x)χ2+(ω(x)+δ1(x))χ1=λ1χ1,x∈Γ,t>0,ω(x)χ1−δ2(x)χ2+∇⋅(d1(x)∇χ2)=λ1χ2,x∈Γ,t>0,∂χ2∂n=0,x∈∂Γ. | (3.3) |
According to Lemma 2.2 in [32] and Theorem 7.6.1 in [27], the following Lemma can be obtained:
Lemma 3.1. Let ν∗p1:=s(P1), where s represents the spectral bound. If ν∗p1≥0, then ν∗p1 is a principal eigenvalue of eigenvalue problem (3.3) with a strongly positive eigenfunction.
Define
F1(⋅)=(0τ(⋅)00),−V1(⋅)=(−(ω(⋅)+δ1(⋅))0ω(⋅)−δ2(⋅)). |
Assume that A1(t): C(ˉΓ,R2)→C(ˉΓ,R2) is the C0-semigroup associated with the following linear system
{(∂ˉw1∂t∂ˉw2∂t)=(0∇⋅(d1(x)∇ˉw2))+V1(x)(ˉw1ˉw2),x∈Γ,t>0,∂ˉw2∂n=0,x∈∂Γ. |
Let ϕm(x)∈C(ˉΓ,R2) be the density of initial fertile mosquitoes, and L1:C(ˉΓ,R2)→C(ˉΓ,R2) be defined by
L1(ϕm)(⋅):=∫∞0F1(⋅)A1(t)ϕm(⋅)dt. | (3.4) |
Here, L1(ϕm)(⋅) denotes the distribution of the total new aquatic/adult mosquitoes generated by initial density ϕm. So, the spectral radius of L1 is R1, that is,
R1:=r(L1). | (3.5) |
When system (1.1) is spatially homogeneous, we can give an explicit representation of R1 as follows:
R1=τωδ2(ω+δ1). |
We can then get the following Lemma according to [31]:
Lemma 3.2. R1−1 has the same sign as ν∗p1.
We can then get the following Lemma to hold applying to Lemma 2.5 in [32]:
Lemma 3.3. Let B:={(M10,M20)T∈C(ˉΓ,R2+):0≤M10(x)≤K1(x)),∀x∈ˉΓ}. Assume that R1>1. Then, we can obtain
limt→∞(M1(t,x),M2(t,x))=(M∗1(x),M∗2(x)),uniformlyforx∈ˉΓ, |
with (M10,M20)∈B∖{(0,0)}. Moreover, 0<M∗1(x)<K1(x), and M∗2(x)>0 ∀x∈ˉΓ.
System (1.1) has two infection-free steady states E0(x)=(0,0,0,0,0,0) and E1(x)=(M∗1(x),0,M∗2(x),0,0,0). When R1>1, E1(x) exists. Linearizing system (1.1) at E1(x) and considering infection compartments, we can then get
{∂I1∂t=α1(x)M∗1(x)K2(x)V−(ω(x)+δ1(x))I1,x∈Γ,∂I2∂t=ω(x)I1+pα2(x)M∗2(x)lH∗(x)I3−δ2(x)I2+∇⋅(d1(x)∇I2),x∈Γ,∂I3∂t=α3(x)I2−γ(x)I3+∇⋅(d2(x)∇I3),x∈Γ,∂V∂t=η(x)I3−ϱ(x)V,x∈Γ.∂I2∂n=∂I3∂n=0,x∈∂Γ, | (3.6) |
for t>0. Denote P2 as
P2(x)=(−(ω(x)+δ1(x))00α1(x)M∗1(x)K2(x)ω(x)−δ2(x)+∇⋅(d1(x)∇)pα2(x)M∗2(x)lH∗(x)00α3(x)−γ(x)+∇⋅(d2(x)∇)000η(x)−ϱ(x)). | (3.7) |
In addition, the eigenvalue problem of system (3.6) is given by
{α1(x)M∗1(x)K2(x)ˉχ4−(ω(x)+δ1(x))ˉχ1=λ2ˉχ1,x∈Γ,ω(x)ˉχ1+pα2(x)M∗2(x)lH∗(x)ˉχ3−δ2(x)ˉχ2+∇⋅(d1(x)∇ˉχ2)=λ2ˉχ2,x∈Γ,α3(x)ˉχ2−γ(x)ˉχ3+∇⋅(d2(x)∇ˉχ3)=λ2ˉχ3,x∈Γ,η(x)ˉχ3−ϱ(x)ˉχ4=λ2ˉχ4,x∈Γ,∂ˉχ2∂n=∂ˉχ3∂n=0,x∈∂Γ, | (3.8) |
for t>0. According to Theorem 7.6.1 in [27], the following Lemma can be obtained:
Lemma 3.4. Let ν∗p2:=s(P2), where s represents the spectral bound. If ν∗p2≥0, then ν∗p2 is a principal eigenvalue of eigenvalue problem (3.8) with a strongly positive eigenfunction.
Define
F2(⋅)=(000α1(⋅)M∗1(⋅)K2(⋅)00pα2(⋅)M∗2(⋅)lH∗(⋅)00α3(⋅)000000),−V2(⋅)=(−(ω(⋅)+δ1(⋅))000ω(⋅)−δ2(⋅)0000−γ(⋅)000η(⋅)−ϱ(⋅)). |
Let u=(I1,I2,I3,V)T, ∇⋅(d(x)∇u)=(0,∇⋅(d1(x)∇I2),∇⋅(d2(x)∇I3),0)T. Assume that A2(t): C(ˉΓ,R4)→C(ˉΓ,R4) is the C0-semigroup associated with the linear system
{∂u∂t=−V2(x)u+∇⋅(d(x)∇u),x∈Γ,∂I2∂n=∂I3∂n=0,x∈∂Γ, |
for t>0. Let ϕm2(x)∈C(ˉΓ,R4) be the density of initial infectious individuals, and L2:C(ˉΓ,R4)→C(ˉΓ,R4) be defined by
L2(ϕm2)(⋅):=∫∞0F2(⋅)A2(t)ϕm2(⋅)dt. | (3.9) |
Here, L2(ϕm2)(⋅) denotes the distribution of new productive infected individuals generated by initial density ϕm2. So, the spectral radius of L2 is R0, that is,
R0:=r(L2). | (3.10) |
When all parameters of system (1.1) are constants, we can give the actual formula of R0 by
R0=√pα2α3M∗2lγδ2H∗+ηωα1α3M∗1γK2ρδ2(ω+δ1). |
We can then get the following Lemma according to [31]:
Lemma 3.5. R0−1 has the same sign as ν∗p2.
According to Theorem 4.1 in [23], we know that E0(x) is globally attractive when R1<1. Biologically, the mosquito population will vanish. Under this assumption, it is pointless to study the spread of the Zika disease. Thus, in this study, we just consider the case R1>1.
Theorem 4.1. Assume R1>1 and R0<1. Then, the disease free state E1(x)=(M∗1(x),0,M∗2(x),0,0,0) is globally attractive.
Proof. Since R0<1, it follows from Lemma 3.5 that ν∗p2<0. Then, there exists a sufficiently small ς>0 such that ν∗p2ς<0, where ν∗p2ς is the principal eigenvalue of the following eigenvalue problem
{α1(x)(M∗1(x)+ς)K2(x)˘χ4−(ω(x)+δ1(x))˘χ1=λ˘χ1,x∈Γ,ω(x)˘χ1+pα2(x)(M∗2(x)+ς)lH∗(x)˘χ3−δ2(x)˘χ2+∇⋅(d1(x)∇˘χ2)=λ˘χ2,x∈Γ,α3(x)˘χ2−γ(x)˘χ3+∇⋅(d2(x)∇˘χ3)=λ˘χ3,x∈Γ,η(x)˘χ3−ϱ(x)˘χ4=λ˘χ4,x∈Γ,∂˘χ2∂n=∂˘χ3∂n=0,x∈∂Γ, | (4.1) |
with a strongly positive eigenfunction (˘χ1,˘χ2,˘χ3,˘χ4).
According to Lemma 3.3, when RM0>1, for the above ς, there exists t1>0 such that 0<S1(t,x)≤M∗1(x)+ς,0<S2(t,x)≤M∗2(x)+ς, ∀x∈ˉΓ, t≥t1. So, for t≥t1, we can get
{∂I1∂t≤α1(x)(M∗1(x)+ς)K2(x)V−(ω(x)+δ1(x))I1,x∈Γ,∂I2∂t≤ω(x)I1+pα2(x)(M∗2(x)+ς)lH∗(x)I3−δ2(x)I2+∇⋅(d1(x)∇I2),x∈Γ,∂I3∂t≤α3(x)I2−γ(x)I3+∇⋅(d2(x)∇I3),x∈Γ,∂V∂t≤η(x)I3−ϱ(x)V,x∈Γ,∂I2∂n=∂I3∂n=0,x∈∂Γ. |
For any initial value θ∈GK, there exists some h1>0 such that (I1(t1,x,θ),I2(t1,x,θ),I3(t1,x,θ),V(t1,x,θ))≤h1(˘χ1,˘χ2,˘χ3,˘χ4), ∀x∈ˉΓ.
Next, consider a comparison system as follows:
{∂ˉu1∂t=α1(x)(M∗1(x)+ς)K2(x)ˉu4−(ω(x)+δ1(x))ˉu1,x∈Γ,∂ˉu2∂t=ω(x)ˉu1+pα2(x)(M∗2(x)+ς)lH∗(x)ˉu3−δ2(x)ˉu2+∇⋅(d1(x)∇ˉu2),x∈Γ,∂ˉu3∂t=α3(x)ˉu2−γ(x)ˉu3+∇⋅(d2(x)∇ˉu3),x∈Γ,∂ˉu4∂t=η(x)ˉu3−ϱ(x)ˉu4,x∈Γ,∂ˉu2∂n=∂ˉu3∂n=0,x∈∂Γ, | (4.2) |
for t≥t1. Then, system (4.2) admits a solution h1eν∗p2ς(t−t1)(˘χ1,˘χ2,˘χ3,˘χ4), ∀t≥t1. According to the comparison principle, we can obtain
(I1(t,x),I2(t,x),I3(t,x),V(t,x))≤h1eν∗p2ς(t−t1)(˘χ1,˘χ2,˘χ3,˘χ4),∀t≥t1,x∈ˉΓ. |
Hence, limt→∞(I1(t,x),I2(t,x),I3(t,x),V(t,x))=(0,0,0,0), uniformly for x∈ˉΓ. According to Lemma 3.3, we have limt→∞S1(t,x)=M∗1(x) and limt→∞S2(t,x)=M∗2(x), uniformly for x∈ˉΓ.
Remark 1 Biologically, when R1>1 and R0<1, Theorem 4.1 shows that the Zika disease will eventually disappear.
Let w(t,x):=(S1(t,x),I1(t,x),S2(t,x),I2(t,x),I3(t,x),V(t,x)) and θ(x):=w(0,x). Define
P={θ∈GK:θ2(⋅)≢0,θ4(⋅)≢0,θ5(⋅)≢0,θ6(⋅)≢0},∂P:=GK∖P={θ∈GK:θ2(⋅)≡0,orθ4(⋅)≡0,orθ5(⋅)≡0,orθ6(⋅)≡0}. |
Define the solution semiflow of system (1.1) as Π(t):GK→GK and Π(t)θ=w(t,⋅,θ) for any t≥0. Applying the method described in [33,34,35], we can obtain the following Lemma:
Lemma 4.1. Assume that R1>1, and θ2≢0, and θ4≢0. If there is a positive constant κ1 such that lim inft→+∞w5(t,x,θ)≥κ1, uniformly for all x∈ˉΓ, then there is a positive constant κ2 such that
lim inft→+∞(w1(t,x),w2(t,x),w3(t,x),w4(t,x),w5(t,x),w6(t,x),)≥(κ2,κ2,κ2,κ2,κ2,κ2), | (4.3) |
uniformly for all x∈ˉΓ.
Lemma 4.2. LetP∂:={θ∈∂P:Π(t)θ∈∂P,∀t≥0}. Define ϖ(θ) as the omega limit set of {Π(t)θ:t≥0} and Q={E0}∪{E1}. Then, ⋃θ∈P∂ϖ(θ)=Q.
Proof. Since θ∈P∂, we know Π(t)θ∈∂P for any t≥0. That is, w2(t,⋅,θ)≡0, w4(t,⋅,θ)≡0, w5(t,⋅,θ)≡0, or w6(t,⋅,θ)≡0 for any t≥0. In the case where w2(t,⋅,θ)≡0 for any t≥0, it follows from the second equation of system (1.1) that w1(t,⋅,θ)≡0 or w6(t,⋅,θ)≡0 for any t≥0.
Assume w1(t,⋅,θ)≡0. From the first equation of system (1.1), one has w3(t,⋅,θ)≡0 and w4(t,⋅,θ)≡0. w5(t,⋅,θ) satisfies
{∂w5∂t=−γ(x)w5+∇⋅(d2(x)∇w5),x∈Γ,∂w5∂n=0,x∈∂Γ. |
Thus, limt→∞w5(t,x,θ)=0 uniformly for x∈ˉΓ. From the sixth equation of (1.1), one has limt→∞w6(t,x,θ)=0 uniformly for x∈ˉΓ. In other words,
limt→∞(w1(t,x),w2(t,x),w3(t,x),w4(t,x),w5(t,x),w6(t,x))=E0(x),uniformlyforx∈ˉΓ. |
If w1(t,⋅,θ)≡0 does not hold, then w6(t,⋅,θ)≡0 holds. From the sixth equation of (1.1), one has w5(t,⋅,θ)≡0 for any t≥0. So, w4(t,⋅,θ)≡0 for t≥0. In this case, assume w3(t,⋅,θ)≡0 for any t≥0, and then w1(t,⋅,θ)≡0 for any t≥0. This contradicts our assumption. Thus, w3(t3,⋅,θ)≢0 for some t3. From Lemma 3.3, we can obtain limt→∞(w1(t,x,θ),w1(t,x,θ))=(M∗1(x),M∗2(x)) uniformly for x∈ˉΓ. Thus,
limt→∞(w1(t,x),w2(t,x),w3(t,x),w4(t,x),w5(t,x),w6(t,x))=E1(x),uniformlyforx∈ˉΓ. |
Assume that there exists t4>0 such that w2(t4,⋅,θ)≢0. From Lemma 3.3, one has w2(t,⋅,θ)>0 for any t>t4. So, w4(t,⋅,θ)≡0, w5(t,⋅,θ)≡0, w6(t,⋅,θ)≡0 for t>t4. Assume w4(t,⋅,θ)≡0 for t>t4. Thus, w5(t,⋅,θ)≡0 and w6(t,⋅,θ)≡0 for t>t4. So, w2(t,⋅,θ)≡0 for t>t4. This contradicts our assumption. Similarly, if w5(t,⋅,θ)≡0 or w6(t,⋅,θ)≡0 for t>t4, then w2(t,⋅,θ)≡0 for t>t4, which contradicts our assumption. Thus, ⋃θ∈P∂ϖ(θ)=Q.
Lemma 4.3. Assume that R1>1. Then, E0(x) is a uniform weak repeller for P in the sense that there exists μ1>0 such that
lim supt→+∞‖Π(t)θ−E0(⋅)‖G≥μ1, | (4.4) |
with initial value θ∈P.
Proof. First, we consider a linear system as follows:
{∂ˆv∂t=P1(μ1)ˆv,x∈Γ,∂ˆv2∂n=0,x∈∂Γ. | (4.5) |
Here, ˆv=(ˆv1,ˆv2)T, and
P1(μ1)=(−(α1(x)K2(x)μ1+ω(x)+δ1(x))τ(x)(1−2μ1K1(x))ω(x)α2(x)plH∗(x)μ1+δ2(x)+∇⋅(d1(x)∇)). |
Clearly, P1(0)=P1. By Lemma 3.1, it follows from R1>1 that ν∗p1=s(P1)>0. Since P1(μ1) is a continuous for small enough μ1, one has s(P1(μ1))>0 for small enough μ1. Let ν∗p1μ1:=s(P1(μ1)). Then, ν∗p1μ1>0. Denote (ˆϕ1,ˆϕ2) as the positive eigenfunction corresponding to ν∗p1μ1. Then, system (4.5) has a solution (ˆv(t,x),ˆv(t,x))=eν∗p1μ1t(ˆϕ1,ˆϕ2).
Next, assume (4.7) does not hold. That is,
lim supt→+∞‖Π(t)ˆθ−E0(⋅)‖G<μ1, | (4.6) |
for some ˆθ∈P. Then, there is a constant t5>0 such that 0<w1(t,x,ˆθ), w2(t,x,ˆθ), w3(t,x,ˆθ), w4(t,x,ˆθ), w5(t,x,ˆθ), w6(t,x,ˆθ)<μ1, x∈ˉΓ. Then, w1(t,x,ˆθ) and w3(t,x,ˆθ) satisfy
{∂w1∂t=τ(x)(1−2μ1K1(x))w2+(ω(x)+δ1(x))w1,x∈Γ,t>t4,∂w2∂t=ω(x)w1−(α2(x)plH∗(x)μ1+δ2(x))w2+∇⋅(d1(x)∇w2),x∈Γ,t>t5,∂w2∂n=0,x∈∂Γ. |
Since w1(t,x,ˆθ)>0 and w3(t,x,ˆθ)>0 for t≥t5, there exists ˆυ1>0 such that (w1(t5,x,ˆθ),w1(t5,x,ˆθ))≥ˆυ1(ˆϕ1,ˆϕ2). Applying the comparison principle, one has
(w1(t,x,ˆθ),w1(t,x,ˆθ))≥ˆυ1eν∗p1μ1(t−t5)(ˆϕ1,ˆϕ2),for∀t≥t5,x∈ˉΓ. |
Due to ν∗p1μ1>0, one has w1(⋅,x,ˆθ)→+∞ and w3(⋅,x,ˆθ)→+∞ as t→∞. This contradicts our assumption. It implies that {E0(x)} is an isolated invariant set in P, and Ws({E0(x)})∩P=∅.
Similar to the proof method of Lemma 4.3, we can draw the following conclusion:
Lemma 4.4. Assume that R0>1. Then, E1(x) is a uniform weak repeller for P in the sense that there exists μ2>0 such that
lim supt→+∞‖Π(t)θ−E1(⋅)‖G≥μ2, | (4.7) |
with initial value θ∈P.
Next, based on Lemmas 4.2, 4.3, and 4.4, we can obtain the following result:
Theorem 4.2. If R1>1 and R0>1, then there exists ε>0 such that, for every initial state θ∈P, the solution of system (1.1) w(t,⋅,θ) satisfies
lim inft→+∞(w1(t,⋅,θ),w2(t,⋅,θ),w3(t,⋅,θ),w4(t,⋅,θ),w5(t,⋅,θ),w6(t,⋅,θ))≥(ε,ε,ε,ε,ε,ε). | (4.8) |
Proof. By Lemmas 4.3 and 4.4, we can get that Q is an isolated invariant set for Π in P, and Ws(Q)∩P=∅, where Ws(Q) is the stable set of Q for Π. From Lemma 4.2, we know that any orbit of Π(t) in P∂ converges to Q as t→∞. So, no subset of Q forms a cycle in ∂P. By the acyclicity theorem on uniform persistence for maps (see Theorem 1.3.1 and Remark 1.3.1 in [36]), we conclude that Π is uniformly persistent with respect to (P,∂P). In addition, the uniform boundedness of the solution of (1.1) implies that Π(t) is point dissipative. According to Theorem 3.7 and Remark 3.10 in [35], we have that Π(t) admits a global attractor A in P. It follows from A=Π(t)A that θ2(⋅)>0,θ4(⋅)>0,θ5(⋅)>0, and θ6(⋅)>0 for all θ∈A. Let E=⋃t≥0Π(t)A. Then, E⊂P and limt→+∞d(Π(t)θ,E)=0 for all θ∈P.
Define a continuous function Y: GK→[0,+∞) by
Y(θ)=min{minx∈ˉΓθ2(x),minx∈ˉΓθ4(x),minx∈ˉΓθ5(x),minx∈ˉΓθ6(x)},∀θ∈GK. |
Clearly, Y(θ)>0 for all θ∈E. Since E is a compact subset of P, we can get infθ∈EY(θ)=minθ∈EY(θ)>0. It follows from the attractiveness of E that there is a positive constant ˉκ1 such that
lim inft→+∞Y(Π(t)θ)=lim inft→+∞minx∈ˉΓ(w2(⋅,x,θ),w4(⋅,x,θ),w5(⋅,x,θ),w6(⋅,x,θ))≥(ˉκ1,ˉκ1,ˉκ1,ˉκ1). |
From Lemma 4.1, there exists some ε>0 such that
lim inft→+∞(w1(t,⋅,θ),w2(t,⋅,θ),w3(t,⋅,θ),w4(t,⋅,θ),w5(t,⋅,θ),w6(t,⋅,θ))≥(ε,ε,ε,ε,ε,ε). |
Remark 2 Biologically, when R1>1 and R0>1, Theorem 4.2 shows that the disease will persists.
In this section, we give numerical simulations for the dynamical behaviors of the solutions and the effect of some key factors on the transmission of the Zika disease. For this purpose, some parameters are selected with the following values: Γ=(0,π), τ(x)=1, K1(x)=500, K2(x)=16, ω(x)=0.05, δ1(x)=0.15, δ2(x)=0.05, η(x)=0.1, ρ(x)=0.3, H∗(x)=100, γ(x)=0.14, d1(x)=0.001, d2(x)=0.008, p=0.6, l=0.3. Under this set of parameters, we calculate the mosquito reproduction number R1=5>1.
Initial values are selected as S1(0,x)=30(1+sin(2x)), I1(0,x)=0.3(1+sin(2x)), S2(0,x)=10(1+sin(2x)), I2(0,x)=0.1(1+sin(2x)), I3(0,x)=1+sin(2x), V(0,x)=0.03(1+sin(2x)). To simulate the result of Theorem 4.1, we choose α1(x)=0.001(1−cos(2x)), α2(x)=0.004(1−cos(2x)), α3(x)=0.005(1−cos(2x)). We calculate the basic reproduction number to be R0=0.0343<1. Figure 1 shows that the Zika disease will be eliminated.
To simulate to result of Theorem 4.2, we set the transmission rate functions as follows:
α1(x)=0.05(1−cos(2x)),α2(x)=0.14(1−cos(2x)),α3(x)=0.158(1−cos(2x)). | (5.1) |
Then, we calculate R0=1.0832>1. From Theorem 4.2, we know that system (1.1) is uniformly persistent, which is shown in Figure 2.
Here, we choose α2(x)=0.14 and d2(x)=0.011. Other parameter values remain unchanged, and other transmission rate functions are selected as in (5.1). Figure 3 shows that the basic reproduction number R0 is an increasing function with q. When q=1, i.e., p=l, this case indicates no vector-bias, and one has R0=0.9908<1. The Zika disease will disappear from Theorem 4.1. Then, R0 increases as q increases. When q>q∗ (hereq∗=2.9089), we know R0>1. The Zika disease persists from Theorem 4.2. It implies that the vector-bias can cause disease outbreaks. Figure 3 indicates that neglecting the vector-bias will underestimate the risk of disease.
Next, we demonstrate the effect of the vector-bias q=pl on the infected adult mosquitoes I2(t,x) and infected humans I3(t,x). In Figure 4, the red solid curve represents q=1. It indicates no vector-bias. The blue solid curve represents q=5. It indicates that there is a vector-bias. We find that the presence of the vector-bias leads to an increased peak value of I2(t,x) and I3(t,x). In addition, as time goes on, the vector-bias has an increasingly strong effect on the peak of the distribution of infected adult mosquitoes and infected humans.
Here, we choose d2(x)=0.01. Other parameter values remain unchanged and the transmission rate functions are selected as in (5.1). Figure 5 gives that the basic reproduction number R0 is an increasing function with α1. When α1<α∗1, we have R0<1. From Theorem 4.1, the Zika disease will disappear. R0 increases as α1 increases. When α1>α∗1 (hereα∗1=0.2818), one has R0>1. The Zika disease persists according to Theorem 4.2. Figure 5 indicates that the environmental transmission is important and indispensable.
Next, we will present the effect of the environmental transmission rate α1 on the distribution of I1(t,x) and V(t,x). We change the value of α1, and other parameter values remain unchanged. We consider three different values: α1=0.1,0.2,0.3. From Figure 6, when the time is fixed, the peak value of infected aquatic mosquitoes increases significantly with the increase of the environmental transmission rate α1. As time goes on, the environmental transmission rate has an increasingly strong effect on the peak of the distribution of infected aquatic mosquitoes and the densities of the Zika virus in the water environment.
Here, we just consider the effect of the human diffusion rate d2 on R0. The transmission rate functions are selected as in (5.1). Figure 7 gives that the basic reproduction number R0 is a decreasing function with d2, which is consistent with the results obtained in [37,38]. When d2=0, this case indicates no diffusion in human population, and one has R0=2.2571>1. The Zika disease persists according to Theorem 4.2. As d2 increases, R0 decreases. When d2>d∗2 (hered∗2=0.0098), R0<1. From Theorem 4.1, the Zika disease will disappear. Figure 7 shows that neglecting the human diffusion will overestimate the risk of the Zika disease.
From the Brazil Ministry of Health [39], we collect the weekly reported accumulated Zika cases in Brazil from March 25, 2016 to April 14, 2018. We use model (1.1) to fit the real-world data and take a week as the time unit. Some other parameters can be selected from previous literature [21]. τ(x)=1, ω(x)=0.05×7, δ1(x)=0.15×7, γ(x)=0.1×7, δ2(x)=0.05×7, α2(x)=0.025×7, α3(x)=0.028×7. H∗(x)=2.05×108 [40]. Other parameters will be estimated by applying the least-squares estimation method. α1(x)=0.0001×7, η(x)=0.1×7, ρ(x)=0.3×7, d1(x)=0.001, d2(x)=0.008, p=0.4, l=0.3. K1(x)=1.02×109, K2(x)=3.28×107. We can get R0<1, and from Theorem 4.1, the Zika disease will disappear in Brazil. The fitting result for the accumulated cases is given in Figure 8.
This study investigated a reaction-diffusion Zika model based on our previous model in [23]. We introduced the vector-bias in the model in article [23]. In this study, we considered the combined effects of vector-bias, environmental transmission, and spatial heterogeneity on spread of Zika disease. We defined two threshold indexes: the mosquito reproduction number R1 and basic reproduction number R0. Dynamical behaviors in terms of R1 and R0 were analyzed. Finally, we simulated the effects of the vector-bias q=pl, the environmental transmission rate α1, and the human diffusion rate d2 on R0. We found that the ignorance of the vector-bias effect will underestimate the infection risk of the Zika disease and the ignorance of the human diffusion rate effect will overestimate the infection risk.
The authors declare they have not used Artificial Intelligence (AI) tools in the creation of this article.
The work is partially supported by the National Natural Science Foundation of China (No.12201007, 12201557, 12101003), the Natural Science Foundation of Anhui province (No. 2108085QA07), Foundation of Zhejiang provincial education department (No. Y202249921), Natural Science Research Project of Anhui Educational Committee (No. 2023AH030021) and Pre-Research National Natural Science Foundation of China of Anhui Polytechnic University (No. Xjky2020134).
The authors declare there are no conflicts of interest.
[1] | World Health Organization (WHO), Emergency Committee on Zika virus and observed increase in neurological disorders and neonatal malformations, 2016. Available from: https://www.chinadaily.com.cn/world/2016-02/02/content_23348858.htm. |
[2] |
G. Lucchese, D. Kanduc, Zika virus and autoimmunity: from microcephaly to Guillain-Barré syndrome, and beyond, Autoimmun Rev., 15 (2016), 801–808. https://doi.org/10.1016/j.autrev.2016.03.020 doi: 10.1016/j.autrev.2016.03.020
![]() |
[3] |
I. Bogoch, O.Brady, M. Kraemer, M. German, M. Creatore, S. Brent, et al., Potential for Zika virus introduction and transmission in resource-limited countries in Africa and the Asia-Pacific region: a modelling study, Lancet Infect Dis., 16 (2016), 1237–1245. https://doi.org/10.1016/S1473-3099(16)30270-5 doi: 10.1016/S1473-3099(16)30270-5
![]() |
[4] |
B. Zheng, L. Chang, J. Yu, A mosquito population replacement model consisting of two differential equations, Electron. Res. Arch., 30 (2016), 978–994. https://doi.org/10.3934/era.2022051 doi: 10.3934/era.2022051
![]() |
[5] |
Z. Lv, J. Zeng, Y. Ding, X. Liu, Stability analysis of time-delayed SAIR model for duration of vaccine in the context of temporary immunity for COVID-19 situation, Electron. Res. Arch., 31 (2023), 1004–1030. https://doi.org/10.3934/era.2023050 doi: 10.3934/era.2023050
![]() |
[6] |
H. Cao, M. Han, Y. Bai, S. Zhang, Hopf bifurcation of the age-structured SIRS model with the varying population sizes, Electron. Res. Arch., 30 (2022), 3811–3824. https://doi.org/10.3934/era.2022194 doi: 10.3934/era.2022194
![]() |
[7] |
X. Zhou, X. Shi, Stability analysis and backward bifurcation on an SEIQR epidemic model with nonlinear innate immunity, Electron. Res. Arch., 30 (2022), 3481–3508. https://doi.org/10.3934/era.2022178 doi: 10.3934/era.2022178
![]() |
[8] |
G. Fan, N. Li, Application and analysis of a model with environmental transmission in a periodic environmen, Electron. Res. Arch., 31 (2023), 5815–5844. https://doi.org/10.3934/era.2023296 doi: 10.3934/era.2023296
![]() |
[9] |
S. Guo, X. Yang, Z. Zheng, Global dynamics of a time-delayed malaria model with asymptomatic infections and standard incidence rate, Electron. Res. Arch., 31 (2023), 3534–3551. https://doi.org/10.3934/era.2023179 doi: 10.3934/era.2023179
![]() |
[10] |
K. Wang, H. Zhao, H. Wang, R. Zhang, Traveling wave of a reaction-diffusion vector-borne disease model with nonlocal effects and distributed delay, J. Dyn. Differ. Equations, 35 (2023), 3149–3185. https://doi.org/10.1007/s10884-021-10062-w doi: 10.1007/s10884-021-10062-w
![]() |
[11] |
K. Wang, H. Wang, H. Zhao, Aggregation and classification of spatial dynamics of vector-borne disease in advective heterogeneous environment, J. Differ. Equations, 343 (2023), 285–331. https://doi.org/10.1016/j.jde.2022.10.013 doi: 10.1016/j.jde.2022.10.013
![]() |
[12] |
W. E. Fitzgibbon, J. J. Morgan, G. F. Webb, An outbreak vector-host epidemic model with spatial structure: the 2015–2016 Zika outbreak in Rio De Janeiro, Theor. Biol. Med. Model., 14 (2017), 1–17. https://doi.org/10.1186/s12976-017-0051-z doi: 10.1186/s12976-017-0051-z
![]() |
[13] |
T. Y. Miyaoka, S. Lenhart, J. F. Meyer, Optimal control of vaccination in a vector-borne reaction-diffusion model applied to Zika virus, J. Math. Biol., 79 (2019), 1077–1104. https://doi.org/10.1007/s00285-019-01390-z doi: 10.1007/s00285-019-01390-z
![]() |
[14] |
K. Yamazaki, Zika virus dynamics partial differential equations model with sexual transmission route, Nonlinear Anal.-Real, 50 (2019), 290–315. https://doi.org/10.1016/j.nonrwa.2019.05.003 doi: 10.1016/j.nonrwa.2019.05.003
![]() |
[15] |
Y. Cai, K. Wang, W. Wang, Global transmission dynamics of a zika virus model, Appl. Math. Lett., 92 (2019), 190–195. https://doi.org/10.1016/j.aml.2019.01.015 doi: 10.1016/j.aml.2019.01.015
![]() |
[16] |
L. Duan, L. Huang, Threshold dynamics of a vector-host epidemic model with spatial structure and nonlinear incidence rate, Proc. Amer. Math. Soc., 149 (2021), 4789–4797. https://doi.org/10.1090/proc/15561 doi: 10.1090/proc/15561
![]() |
[17] |
P. Magal, G. Webb, Y. Wu, On a vector-host epidemic model with spatial structure, Nonlinearity, 31 (2018), 5589–5614. https://doi.org/10.1088/1361-6544/aae1e0 doi: 10.1088/1361-6544/aae1e0
![]() |
[18] |
P. Magal, G. Webb, Y. Wu, On the basic reproduction number of reaction-diffusion epidemic models, SIAM J. Appl. Math., 79 (2019), 284–304. https://doi.org/10.1137/18M1182243 doi: 10.1137/18M1182243
![]() |
[19] |
F. Li, X. Q. Zhao, Global dynamics of a reaction–diffusion model of Zika virus transmission with seasonality, B. Math. Biol., 83 (2021), 43. https://doi.org/10.1007/s11538-021-00879-3 doi: 10.1007/s11538-021-00879-3
![]() |
[20] |
S. Du, Y. Liu, J. Liu, J. Zhao, C. Champagne, L. Tong, et al., Aedes mosquitoes acquire and transmit Zika virus by breeding in contaminated aquatic environments, Nat. Commun., 10 (2019), 1324. https://doi.org/10.1038/s41467-019-09256-0 doi: 10.1038/s41467-019-09256-0
![]() |
[21] |
L. Wang, H. Zhao, Modeling and dynamics analysis of Zika transmission with contaminated aquatic environments, Nonlinear Dyn., 104 (2021), 845–862. https://doi.org/10.1007/s11071-021-06289-3 doi: 10.1007/s11071-021-06289-3
![]() |
[22] |
L. Wang, P. Wu, M. Li, L. Shi, Global dynamics analysis of a Zika transmission model with environment transmission route and spatial heterogeneity, AIMS Math., 7 (2022), 4803–4832. https://doi.org/10.3934/math.2022268 doi: 10.3934/math.2022268
![]() |
[23] |
L. Wang, P. Wu, Threshold dynamics of a Zika model with environmental and sexual transmissions and spatial heterogeneity, Z. Angew. Math. Phys., 73 (2022), 1–22. https://doi.org/10.1007/s00033-022-01812-x doi: 10.1007/s00033-022-01812-x
![]() |
[24] |
J. Wang, Y. Chen, Threshold dynamics of a vector-borne disease model with spatial structure and vector-bias, Appl. Math. Lett., 100 (2020), 106052. https://doi.org/10.1016/j.aml.2019.106052 doi: 10.1016/j.aml.2019.106052
![]() |
[25] |
Y. Pan, S. Zhu, J. Wang, A note on a ZIKV epidemic model with spatial structure and vector-bias, AIMS Math., 7 (2022), 2255–2265. https://doi.org/10.3934/math.2022128 doi: 10.3934/math.2022128
![]() |
[26] | P. Hess, Periodic-parabolic boundary value problems and positivity, Longman Scientific and Technical, Harlow, 1991. https://doi.org/10.1112/blms/24.6.619 |
[27] | H. L. Smith, Monotone Dynamical Systems: An Introduction to the Theory of Competitive and Cooperative Systems, American Mathematical Society, Rhode Island, 1995. https://doi.org/10.1090/surv/041 |
[28] |
X. Ren, Y. Tian, L. Liu, X. Liu, A reaction–diffusion within-host HIV model with cell-to-cell transmission, J. Math. Biol., 76 (2018), 1831–1872. https://doi.org/10.1007/s00285-017-1202-x doi: 10.1007/s00285-017-1202-x
![]() |
[29] | M. Wang, Nonlinear Elliptic Equations, Science Publication, Beijing, 2010. |
[30] |
R. H. Martin, H. L. Smith, Abstract functional-differential equations and reaction-diffusion systems, Trans. Amer. Math. Soc., 321 (1990), 1–44. https://doi.org/10.1090/S0002-9947-1990-0967316-X doi: 10.1090/S0002-9947-1990-0967316-X
![]() |
[31] |
W. Wang, X. Q. Zhao, Basic reproduction numbers for reaction-diffusion epidemic models, SIAM J. Appl. Dyn. Syst., 11 (2012), 1652–1673. https://doi.org/10.1137/120872942 doi: 10.1137/120872942
![]() |
[32] |
Y. C. Shyu, R. N. Chien, F. B. Wang, Global dynamics of a West Nile virus model in a spatially variable habitat, Nonlinear Anal.-Real, 41 (2018), 313–333. https://doi.org/10.1016/j.nonrwa.2017.10.017 doi: 10.1016/j.nonrwa.2017.10.017
![]() |
[33] |
S. B. Hsu, F. B. Wang, X. Q. Zhao, Global dynamics of zooplankton and harmful algae in flowing habitats, J. Differ. Equations, 255 (2013), 265–297. https://doi.org/10.1016/j.jde.2013.04.006 doi: 10.1016/j.jde.2013.04.006
![]() |
[34] |
S. B. Hsu, F. B. Wang, X. Q. Zhao, Dynamics of a periodically pulsed bio-reactor model with a hydraulic storage zone, J. Dyn. Differ. Equations, 23 (2011), 817–842. https://doi.org/10.1007/s10884-011-9224-3 doi: 10.1007/s10884-011-9224-3
![]() |
[35] |
P. Magal, X. Q. Zhao, Global attractors and steady states for uniformly persistent dynamical systems, SIAM J. Math. Anal., 37 (2005), 251–275. https://doi.org/10.1137/S0036141003439173 doi: 10.1137/S0036141003439173
![]() |
[36] | X. Q. Zhao, Dynamical Systems in Population Biology, 2nd edition, Springer, New York, 2017. https://doi.org/10.1007/978-3-319-56433-3 |
[37] |
R. Wu, X. Q. Zhao, A reaction-diffusion model of vector-borne disease with periodic delays, J Nonlinear Sci., 29 (2019), 29–64. https://doi.org/10.1007/s00332-018-9475-9 doi: 10.1007/s00332-018-9475-9
![]() |
[38] |
F. Li, X. Q. Zhao, Global dynamics of a nonlocal periodic reaction-diffusion model of bluetongue disease, J. Differ. Equations, 272 (2021), 127–163. https://doi.org/10.1016/j.jde.2020.09.019 doi: 10.1016/j.jde.2020.09.019
![]() |
[39] | Brazil Ministry of Health, Zika cases from the Brazil Ministry of Health, 2018. Available from: http://portalms.saude.gov.br/boletins-epidemiologicos. |
[40] | City Populations Worldwide, Brazil population, 2018. Available from: http://population.city/brazil/. |