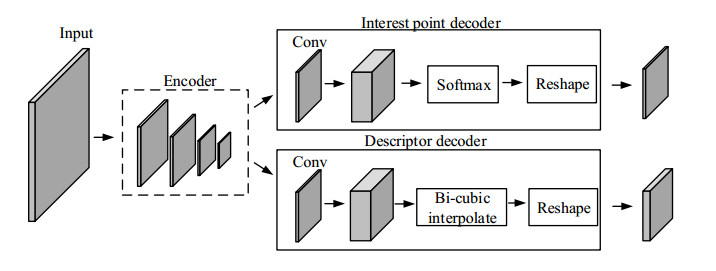
In this research, we aim to examine the moderating effect of environmental commitment on the relationships between organizational compliance culture and green culture on environmental behavior, under the theoretical stream of the Resource Based Theory (RBT) of the firm, and the Value-Belief-Norm theory (VBN). The research used a quantitative research approach, with a non-experimental transactional design. The sample consisted of 148 Mexican companies with a corporate value system that has implemented environmental sustainability practices, most of them incorporating the GRI guidelines, the SDGs and the Ten Principles of the UN Global Compact into their strategies, policies, procedures, and initiatives. This study contributes to the literature in the field of environmental sustainability, with a first theoretical PLS-SEM model that studies moderating and control variables, through organizational compliance on environmental behavior. Our proposed PLS model is a complex hierarchical component model that brings together and simultaneously maps a higher-order construct combined with three lower-order constructs, with moderation effects, multi-group analysis and predictive performance assessment. The major research findings are both the positive impacts between the proposed higher-order construct, organizational compliance culture, and green culture, on environmental behavior, and the moderating effect of environmental commitment on the relationship between green culture and environmental behavior. The insights obtained enhance the understanding of the factors that determine the environmental behavior through organizational compliance culture and green culture, with the moderating effect of environmental commitment, and help senior management in making strategic decisions to align their environmental objectives in compliance with the 2030 agenda in the area of environmental sustainability. This study highlights the need for companies to strengthen the role of environmental commitment to improve the environmental sustainability and it mentions practical implications both for managers of organizations that are responsible for meeting the objectives of sustainable development, specifically in the environmental field, and for policymakers and authorities that guide environmental policies.
Citation: Joseph F. Hair, Juan José García-Machado, Minerva Martínez-Avila. The impact of organizational compliance culture and green culture on environmental behavior: The moderating effect of environmental commitment[J]. Green Finance, 2023, 5(4): 624-657. doi: 10.3934/GF.2023024
[1] | Yongli Yan, Tiansheng Sun, Teng Ren, Li Ding . Enhanced grip force estimation in robotic surgery: A sparrow search algorithm-optimized backpropagation neural network approach. Mathematical Biosciences and Engineering, 2024, 21(3): 3519-3539. doi: 10.3934/mbe.2024155 |
[2] | Xiaoming Liu, Zhonghan Liu, Yaqin Pan, Yufeng Huang, Desheng Wu, Zhaoyu Ba . Full-endoscopic transforaminal procedure to treat the single-level adjacent segment disease after posterior lumbar spine fusion: 1–2 years follow-up. Mathematical Biosciences and Engineering, 2019, 16(6): 7829-7838. doi: 10.3934/mbe.2019393 |
[3] | Lufeng Yao, Haiqing Wang, Feng Zhang, Liping Wang, Jianghui Dong . Minimally invasive treatment of calcaneal fractures via the sinus tarsi approach based on a 3D printing technique. Mathematical Biosciences and Engineering, 2019, 16(3): 1597-1610. doi: 10.3934/mbe.2019076 |
[4] | Xuefei Deng, Yu Liu, Hao Chen . Three-dimensional image reconstruction based on improved U-net network for anatomy of pulmonary segmentectomy. Mathematical Biosciences and Engineering, 2021, 18(4): 3313-3322. doi: 10.3934/mbe.2021165 |
[5] | Akim Kapsalyamov, Shahid Hussain, Prashant K. Jamwal . A novel compliant surgical robot: Preliminary design analysis. Mathematical Biosciences and Engineering, 2020, 17(3): 1944-1958. doi: 10.3934/mbe.2020103 |
[6] | Hafiz Muhammad Muzzammil, Yong-De Zhang, Hassan Ejaz, Qihang Yuan, Muhammad Muddassir . A review on tissue-needle interaction and path planning models for bevel tip type flexible needle minimal intervention. Mathematical Biosciences and Engineering, 2024, 21(1): 523-561. doi: 10.3934/mbe.2024023 |
[7] | Wenlu Zhang, Ziyue Ma, Hong Wang, Juan Deng, Pengfei Li, Yu Jia, Yabin Dong, Hong Sha, Feng Yan, Wenjun Tu . Study on automatic ultrasound scanning of lumbar spine and visualization system for path planning in lumbar puncture surgery. Mathematical Biosciences and Engineering, 2023, 20(1): 613-623. doi: 10.3934/mbe.2023028 |
[8] | Maher Alwuthaynani, Raluca Eftimie, Dumitru Trucu . Inverse problem approaches for mutation laws in heterogeneous tumours with local and nonlocal dynamics. Mathematical Biosciences and Engineering, 2022, 19(4): 3720-3747. doi: 10.3934/mbe.2022171 |
[9] | Tianran Yuan, Hongsheng Zhang, Hao Liu, Juan Du, Huiming Yu, Yimin Wang, Yabin Xu . Watertight 2-manifold 3D bone surface model reconstruction from CT images based on visual hyper-spherical mapping. Mathematical Biosciences and Engineering, 2021, 18(2): 1280-1313. doi: 10.3934/mbe.2021068 |
[10] | Tian Ma, Boyang Meng, Jiayi Yang, Nana Gou, Weilu Shi . A half jaw panoramic stitching method of intraoral endoscopy images based on dental arch arrangement. Mathematical Biosciences and Engineering, 2024, 21(1): 494-522. doi: 10.3934/mbe.2024022 |
In this research, we aim to examine the moderating effect of environmental commitment on the relationships between organizational compliance culture and green culture on environmental behavior, under the theoretical stream of the Resource Based Theory (RBT) of the firm, and the Value-Belief-Norm theory (VBN). The research used a quantitative research approach, with a non-experimental transactional design. The sample consisted of 148 Mexican companies with a corporate value system that has implemented environmental sustainability practices, most of them incorporating the GRI guidelines, the SDGs and the Ten Principles of the UN Global Compact into their strategies, policies, procedures, and initiatives. This study contributes to the literature in the field of environmental sustainability, with a first theoretical PLS-SEM model that studies moderating and control variables, through organizational compliance on environmental behavior. Our proposed PLS model is a complex hierarchical component model that brings together and simultaneously maps a higher-order construct combined with three lower-order constructs, with moderation effects, multi-group analysis and predictive performance assessment. The major research findings are both the positive impacts between the proposed higher-order construct, organizational compliance culture, and green culture, on environmental behavior, and the moderating effect of environmental commitment on the relationship between green culture and environmental behavior. The insights obtained enhance the understanding of the factors that determine the environmental behavior through organizational compliance culture and green culture, with the moderating effect of environmental commitment, and help senior management in making strategic decisions to align their environmental objectives in compliance with the 2030 agenda in the area of environmental sustainability. This study highlights the need for companies to strengthen the role of environmental commitment to improve the environmental sustainability and it mentions practical implications both for managers of organizations that are responsible for meeting the objectives of sustainable development, specifically in the environmental field, and for policymakers and authorities that guide environmental policies.
Compared to traditional surgery, the advantages of minimally invasive surgery are minor trauma, minor infection, and minor pain [1], a trend of surgical treatment in modern medicine. In modern minimally invasive surgery technology, the endoscope and specialist instruments are inserted into the internal cavity through a small incision in the surgical site. The images captured by the endoscope are displayed on the screen in real-time [2]. The surgeon can monitor the patient's entire operation through the endoscopic transmission of images and can determine the patient's surgical condition [3]. For traditional minimally invasive surgery, there are still limitations in obtaining image information from the endoscope, such as the narrow field of view, uneven illumination and high reflection intensity, and the difficulty in obtaining depth information of the target area and locating the endoscope accurately. Under such complex environmental conditions, higher requirements are put forward for computer vision processing systems. Therefore, SLAM system is innovatively applied to the field of minimally invasive surgery to solve the bottleneck faced by modern minimally invasive surgery [4]. The endoscope acts as a robot in the field of minimally invasive surgery. It moves through the cavity of the human body, measures spatial information of the visible area of the cavity, completes the reconstruction of soft tissue, and displays it to the doctor. Minimally invasive surgery can help doctors make the right decisions by combining SLAM with better positioning of the endoscope and depth of the target area. It has crucial research significance and application value [5].
The concept of minimally invasive surgery and the issue of visual SLAM were first proposed in 1983 and 1986, respectively. The use of vision technology based on endoscope images in minimally invasive surgery has obvious advantages without introducing additional equipment to the already very complex surgical device [6]. SLAM is most frequently used in minimally invasive surgery in the abdomen, where deformation and tissue movement are minimal. At the initial stage of application, SLAM was added to the algorithm as an auxiliary means for positioning [7]. In 2009, Grasa et al. used monocular laparoscopy to generate a sparse map of the environment from SLAM and then estimated the three-dimensional information of the scene through sequential images. In 2009 and 2010, the Mountney P team respectively proposed the application of an SLAM system based on the monocular endoscope and binocular endoscope in minimally invasive surgery for endoscope positioning and 3D modeling, and the monocular system based on the framework of SLAM to establish a motion model to estimate the motion of the camera and soft tissue effectively [8]. The binocular system constructs a 3D texture model of a minimally invasive surgery environment, a method model of dynamic view expansion [9]. In recent years, there have been more and more studies on the application of visual SLAM in minimally invasive surgery [10,11,12], most of which are devoted to solving the bottleneck of minimally invasive surgery. Vision-based techniques are good at restoring 3D structures and estimating endoscope motion. However, this research has a long way to go to solve the problems of accuracy [13].
This paper mainly studies visual SLAM in minimally invasive surgery. For the deficiencies of traditional minimally invasive surgery, visual SLAM technology is used to complete the positioning and 3D mapping of the endoscope [14,15]. In combination with the K-means algorithm and the Super point algorithm, the feature information of the image in the inner cavity is extracted. It solves the problem that it is difficult to obtain the depth information of the target area and accurately locate the endoscope position in the environment with narrow vision, uneven illumination and high reflection intensity of minimally invasive surgical endoscope. Then, the iterative nearest point method was used to estimate the position and attitude of the endoscope. Finally, the stereo matching method was used to reconstruct the lumen image, and the point cloud image of the surgical area was recovered. Improve surgical accuracy and shorten the surgical time, thus optimizing the operation of the entire system [16].
The structure of this paper is as follows. Section 2 discusses the visual SLAM and the method of extracting image features through Superpoint algorithm, as well as the method of extracting image feature points based on Superpoint algorithm and k-means algorithm. In Section 3, experiments are carried out to verify the effectiveness of our proposed method. Section 4 summarizes the whole article.
The final output of the Super point (self supervised interest point detection and description) algorithm is the feature point algorithm with descriptors [17]. The network diagram is shown in Figure 1.
In this network, the initial feature detector is obtained by training the basic graph with undisputed feature points in a self-supervised way, and the image feature information is further extracted by the neural network. The stability of feature extraction is improved while the sensitivity of the feature extraction algorithm to illumination is reduced. The network is divided into two parts [18], the network for detecting basic graph corners and the network for extracting image features and outputting descriptors. The specific steps are as follows:
(1) Feature point detector pre-training. For an image, it is difficult to define which points are the real feature points, but if it is a simple graph with defined corner positions, it is easy to determine the truth. Therefore, the pre-training is carried out by extracting undisputed feature points from an unlabelled virtual simple image, obtaining pseudo-truths, and using the resulting pseudo-truths to determine the true feature points. Then, the pseudo-true values are combined with the true values to retrain the feature detector to obtain the extracted feature. The resulting pseudo-truths are combined with the true values to retrain the feature detector to obtain the extracted feature point model.
(2) The feature point detection network is divided into two parts: encoding and decoding. Image coding is to input the image to be processed into the shared coding network and reduce the dimension of the image to reduce the computation of the network. After dimensionality reduction, the image size is 1/8 of the original image. The main function of the encoder in the network is to map the input image to the spatial tensor, which has a smaller spatial dimension and larger channel depth. A probability is calculated for each pixel, which represents the probability that this pixel is a feature point. When decoding feature points, subpixel convolution is adopted to avoid the possibility of network overload caused by excessive computation in the process of extracting feature points. The input dimension of the decoder is
(3) Description sub detection network. Extracting the descriptor is a decoding operation. The descriptors are obtained from feature points. First, the image size and feature point position are normalized.
K-Means clustering algorithm will use all the data as a whole, the initial clustering center is
After the Super point algorithm extracts the feature points and descriptors of the image, the heatmap of the feature map can be obtained. Each feature point in the heatmap has an index value, and the K-means method is used to cluster these index values. When feature points are matched, a confidence probability of a correct match is set for each index value. According to this probability, whether this point is the correct match of the points to be matched is determined. Set the maximum number of matching point pairs and match according to the index of feature points. When all feature points are matched, or the maximum number of matching points is reached, the matching graph is output. The K-means clustering algorithm is applied to avoid the successful matching between the reflective spot and the strong light point in the inner cavity and reduce the probability of mismatching. Because the k-means algorithm is very simple, very fast, time complexity is nearly linear, and it is suitable for mining large-scale datasets, combining it with the super point algorithm not only improves the matching degree, but also shortens the time it takes to compute the k-means algorithm.
The pose estimation process is actually to find the corresponding point between the 2D image and the 3D spatial position. When representing an object's 3D spatial position and orientation, a coordinate system rotating with the object is usually established with the object as the coordinate origin. The transformation relationship between the rotating coordinate system and the reference coordinate system can determine the spatial position of the object. That is, the solution of pose estimation discusses the transformation relationship between coordinate systems.
In visual SLAM, there are usually two methods to estimate the pose of the sensor. One is to use a linear method to estimate the pose information of the visual sensor. After obtaining the initial pose, the pose information is further optimized by constructing and solving the least square problem. The other is to directly integrate the position of the space point and the position of the sensor to solve the pose information. In the estimation of the pose information of the endoscope, since the position of the endoscope is not continuous and uniform, the first method is selected to solve the pose information of the endoscope. The initial pose is estimated first, and then the results are optimized to increase the accuracy and robustness of the SLAM system.
The iterative closest point (ICP) is a standard algorithm that estimates pose between completed 3D Point pairs. Suppose there are a pair of 3D point pairs matched and their centroids:
P={p1,…,pn},P′={p′1,…,p′n}p=1nn∑i=1(pi),p′=1nn∑i=1(p′i) | (1) |
where:
To perform pose estimation is actually to find a Euclidean transform that satisfies:
∀i,pi=Rp′i+t | (2) |
The result of the above formula is solved through the algebraic method of singular value decomposition, and the error term of the point
ei=pi−(Rpi′+t) | (3) |
Constructing the least-squares problem:
minR,tJ=12n∑i=1‖pi−(Rp′i+t)‖22=12n∑i=1‖pi−p−R(p′i−p′)‖22+‖p−Rp′−t‖22 | (4) |
Solve the above equation and get
qi=pi−p, q′i=p′i−p′ | (5) |
The rotation matrix and translation vector are calculated according to the following formula:
{R∗=argminR12n∑i=1‖qi−Rq′i‖22t∗=p−Rp′ | (6) |
Define matrix:
W=n∑i=1qiqi′T | (7) |
R=UVT | (8) |
Substitute the solved rotation matrix into Eq (8) to solve the translation vector.
Firstly, the disparity value of the target region is obtained and optimized by the SGBM stereo matching method; Secondly, the depth value is calculated by using the parallax value to form the depth image; Finally, the 3D point cloud data are obtained by further calculation.
Parallax refers to that when binocular stereo vision obtains two frames of images at the same time through the left and right cameras, the corresponding relationship between features is established at the imaging points on the left and right imaging planes of the camera through the corresponding three-dimensional space points so that the human eye can feel the depth. Observe the imaging difference of the image on the camera plane. This difference is called parallax. In short, parallax describes the horizontal pixel difference between the corresponding imaging points of the left and right eyes [21].
There is a point
The parallax values in the parallax map can be calculated to obtain the corresponding depth values. The depth map shows the form of the image by integrating these depth values. The depth map contains the depth information of each pixel value, and the pixel value in the depth map is the depth value. According to the geometric relation of parallel binocular vision, the relation between parallax value and object depth information is as follows:
bdep=(b+xr)−xldep−f | (9) |
where:
dep=b×fxl−xr=f×bd | (10) |
where,
According to the coordinate transformation relation, the transformation relation between the world coordinate system and the pixel coordinate system can be obtained as follows:
zp[uv1]=[fx0cx0fycy001][R,t][XYZ1] | (11) |
where: The first matrix on the right of the equation is the endoscope internal parameter; matrix
{z=zpx=(u−cx)×zp/fxy=(v−cy)×zp/fy | (12) |
The calculated data can be saved in the created point cloud data, and the point cloud image can be displayed by using some libraries. The depth image of point cloud data can be calculated through the reverse calculation of the above operations.
In order to verify whether the improved algorithm has better feature extraction and matching effect in the strong reflection environment in minimally invasive surgery, the feature extraction results of the improved algorithm and the improved algorithm in the lumen environment are compared, and the simulation results are compared and analyzed. The original image pairs for feature extraction are shown in a) and b) Figure 5. By comparing the feature extraction results of various methods on the image pairs, the effectiveness of the improved extraction network is shown [22].
The results of image feature extraction using the original Super point algorithm are shown in c) in Figure 5, and the results of feature extraction using the improved Super point algorithm are shown in d) in Figure 5. As can be seen from the figure, the number of feature points extracted by the Super point algorithm in the inner cavity environment under a strong reflection environment is more and more uniform than that extracted by the traditional algorithm, but there are too many failed matching points and many invalid points. These matching failed points and invalid points are caused by the narrow field of vision, uneven lighting and high reflection intensity of minimally invasive surgical endoscope, The numerical value of the depth map physically means the distance from the camera. A lot of black and a lot of white are similar distances from you. The feature points are difficult to extract, and the resolution of the depth map is low, which reduces the number of feature points that can be extracted. False matching situations are reasonable. However, the distribution of feature points extracted by the improved Super point algorithm is more uniform, and effective features can be extracted from the edge part of the insufficient light. e) in Figure 5 is the matching result of feature points extracted by the original Super point algorithm, and f) in Figure 5 is the matching result of feature points extracted by the improved Super point algorithm. It can be easily seen from the figure that the improved algorithm has a better matching effect on feature points, and the number of invalid feature points is 0, which is better than the original algorithm and the traditional algorithm. As can be seen from Figure 5 and Table 1, after the Super point algorithm and clustering algorithm are combined and improved, the number of feature extraction effects of the inner cavity image increases and is evenly distributed, without any redundant points that fail to be matched successfully, and the percentage of successfully matched points in the total number of extractions is the highest and the false matching rate is the lowest. Although the proportion of effective points extracted by SIFT, SURF and ORB algorithms can reach 100%, their speed is very slow when there are too many matching points, and there are many wrong matching point pairs. The original Superpoint algorithm can not only ensure the number of feature points, but also ensure the speed, but it has a very low proportion of effective points. Compared with the traditional feature extraction algorithm and the original Super point algorithm, it shows the best effect.
SIFT | SURF | ORB | Superpoint | Improving Superpoint | |
Extracting time /s | 1.282 | 0.182 | 0.125 | 0.103 | 0.101 |
Match time /s | 0.187 | 0.078 | 0.031 | 0.018 | 0.021 |
Extract point pairs/pairs | 334 | 121 | 268 | 352 | 349 |
Match point pairs/pairs | 334 | 121 | 268 | 263 | 349 |
False match point pair/pair | 79 | 25 | 31 | 13 | 15 |
Effective point proportion /% | 100.00 | 100.00 | 100.00 | 74.72 | 100.00 |
False match rate /% | 23.65 | 20.66 | 11.57 | 4.94 | 4.30 |
Table 1 shows the numerical results of each algorithm. From the two indicators of Extracting time and Match time in the table, the time of the improved algorithm is significantly shorter than that of other algorithms, which also proves that the improved algorithm has the minimum time consumption, the maximum number of matching success points and the minimum mismatch rate, and the effective point ratio is significantly improved compared with that before the improvement.
Different from large-scale scenarios, when the endoscope is in the special environment of minimally invasive surgery, the estimation of the position information of the endoscope only needs numerical values. Since the movement process of the endoscope is irregular, it is not meant to form the map of its position information [23]. In this topic, will the SLAM system of camera pose estimation algorithm used in endoscopic minimally invasive surgery, using the image information of the real environment of minimally invasive surgery for endoscopic frame only when the estimate judgment in endoscopic movement between rotation and translation in the process of calculation is accurate, lumen mapping results of image feature points as shown in Figure 6.
The blue point in Figure 6 is the feature point of the current frame, and the green point is the feature point of the next frame. The current frame is directly processed without any operation. The position difference between blue and green is why the camera moves when shooting different frames, and the red point is the position of the green point after the rotation matrix and the motion vector move left. The distance between blue dots and red dots is smaller than that between green dots, with an average error of 5.43 pixels.
The disparity image and depth image before and after optimization are obtained by using the algorithm in this chapter as follows:
Figure 7 shows the point cloud before and after optimization of the lumen image in the actual scene, which is displayed in the gray form, and the cavity area is more obvious. The image information with too dark illumination in the original image has the condition that the visual difference has not been calculated, so the depth value cannot be calculated, which is reflected as the whole area in the point cloud image. The point cloud image before optimization has obvious segmentation lines. After optimization, this phenomenon is weakened, and most of the information is effectively restored. The details of the main areas are more delicate, and some smaller blood vessels that are not easy to extract can also be restored [24].
Figure 8 is a pure point cloud image without adding mesh and texture. The color depth in the figure indicates the distance between the point and the endoscope. Although the edge results of the disparity map and depth map are not satisfactory, it can be seen from b) of Figures 7 and 8 that the reconstruction method adopted can have a good effect on the restoration of images in the lumen environment.
As can be seen from a) and b) in Figure 9, except for the empty part with extremely weak light, the image has a filling effect, and the edge is partially optimized. Although the "boundary" between the core area and the fat part of the image does not have an excellent restoration result, the edge is smoothed. c) is a pure point cloud image without mesh and texture, and the depth of color in the figure represents the distance between the point and the endoscope. d) is the point cloud diagram of the inner cavity image in the real scene. In the case of the image information with a too-dark light in the original image, the parallax value is not calculated, so the depth value cannot be calculated, which is reflected in the point cloud image as the empty area in the figure. Most of the information in the image can be effectively restored. The details of the main areas are delicate, and some small vessels that are not easy to be extracted can also be recovered. The reconstruction method used in this paper can restore the image in the inner cavity with good effect.
In view of the problem that it is difficult to obtain depth information on the target area and accurately locate the position of the endoscope in the environment of the narrow field of vision, uneven illumination, and high reflection intensity of minimally invasive surgery endoscope, the method based on the combination of K-means and Super point algorithm is first used to extract image feature points and match. Compared with the traditional algorithm, the simulation results are more accurate and stable. Compared with the Super point, the logarithm of successful matching points is increased by 32.69%, the proportion of effective points is increased by 25.28%, the false matching rate is reduced by 0.64%, and the extraction time is reduced by 1.98%. In the real minimally invasive surgery scene, the feature points in the endovascular image were mapped to verify the accuracy of the calculated rotation and translation, with an average error of 5 pixels. Finally, the stereo matching algorithm is used to calculate the disparity map of the original image, and then the disparity map is used to calculate the depth information so as to calculate the point cloud image with depth information, which can well reconstruct the small features such as blood vessels in the image, and also have a good reconstruction effect on the edge part of the insufficient light [25].
Funding: This research was supported by the Natural Science Foundation of Heilongjiang Province (LH2019F024), China, 2019–2022, and Key R & D Program Guidance Projects of Heilongjiang Province (Grant No. GZ20210065), 2021–2024
The authors declare there is no conflict of interest.
[1] |
Abeysekera I (2022) A framework for sustainability reporting. Sust Account Manage Policy 13: 1386–1409. https://doi.org/10.1108/SAMPJ-08-2021-0316 doi: 10.1108/SAMPJ-08-2021-0316
![]() |
[2] |
Abbas J, Dogan E (2022) The impacts of organizational green culture and corporate social responsibility on employees' responsible behaviour towards the society. Environ Sci Pollut Res 29: 60024–60034. https://doi.org/10.1007/s11356-022-20072-w doi: 10.1007/s11356-022-20072-w
![]() |
[3] | Adam DH, Siregar ZME, Supriadi YN (2021) Environmental concern and environmental knowledge, attitude toward pro-environmental behavior as predictors of pro-environmental behavior: Evidence from textile industry in Indonesia. J Inf 22: 138–144. |
[4] |
Aftab J, Veneziani M (2023) How does green human resource management contribute to saving the environment? Evidence of emerging market manufacturing firms. Bus Strategy Environ, 1–17. https://doi.org/10.1002/bse.3508 doi: 10.1002/bse.3508
![]() |
[5] |
Ahmad J, Mamun A, Masukujjama M, et al. (2023) Modeling the workplace pro-environmental behavior through green human resource management and organizational culture: Evidence from an emerging economy. Heliyon 9: 1–17. https://doi.org/10.1016/j.heliyon.2023.e19134 doi: 10.1016/j.heliyon.2023.e19134
![]() |
[6] |
Al-Mamun A, Hayat N, Mohiuddin M, et al. (2022) Modelling the significance of value-belief-norm theory in predicting workplace energy conservation behaviour. Front Energy Res 10: 1–15. https://doi.org/10.3389/fenrg.2022.940595 doi: 10.3389/fenrg.2022.940595
![]() |
[7] |
Al-Swidi AK, Gelaidan HM, Saleh RM (2021) The joint impact of green human resource management, leadership and organizational culture on employees' green behaviour and organisational environmental performance. J Clean Prod 316: 128112. https://doi.org/10.1016/j.jclepro.2021.128112 doi: 10.1016/j.jclepro.2021.128112
![]() |
[8] | Alvesson M (2013) Understanding Organizational Culture, 2nd edition. London: SAGE. |
[9] |
An H, Razzaq A, Nawaz A, et al. (2021) Nexus between green logistic operations and triple bottom line: Evidence from infrastructure-led Chinese outward foreign direct investment in Belt and Road host countries. Environ Sci Pollut Res 28: 51022–51045. https://doi.org/10.1007/s11356-021-12470-3 doi: 10.1007/s11356-021-12470-3
![]() |
[10] |
Ansari NY, Farrukh M, Raza A (2021) Green human resource management and employees pro‐environmental behaviours: Examining the underlying mechanism. Corp Soc Responsib Environ Manag 28: 229–238. https://doi.org/10.1002/csr.2044 doi: 10.1002/csr.2044
![]() |
[11] |
Appiah MK, Sam A, Osei-Agyemang V, et al. (2023) Green culture, environmental innovativeness, green intellectual capital, and circular economy implementation behaviour: A sequential mediation model. Cogent Eng 10: 1–17. https://doi.org/10.1080/23311916.2023.2220496 doi: 10.1080/23311916.2023.2220496
![]() |
[12] |
Aragón-Correa JA, Matı́as-Reche F, Senise-Barrio ME (2004) Managerial discretion and corporate commitment to the natural environment. J Bus Res 57: 964–975. https://doi.org/10.1016/S0148-2963(02)00500-3 doi: 10.1016/S0148-2963(02)00500-3
![]() |
[13] | Asmui M, Mokhtar NM, Musa ND, et al. (2016) The implementation of organizational green culture in higher educational institution, In: Abdullah, M., Yahya, W., Ramli, N., Mohamed, S., Ahmad, B. (eds) Regional Conference on Science, Technology and Social Sciences (RCSTSS 2014), Springer, Singapore: 321–330. https://doi.org/10.1007/978-981-10-1458-1_31 |
[14] |
Aziz NAA, Manab NA, Othman SN (2015) Exploring the Perspectives of Corporate Governance and Theories on Sustainability Risk Management (SRM). Asian Econ Financ Rev 5: 1148–1158. https://doi.org/10.18488/journal.aefr/2015.5.10/102.10.1148.1158 doi: 10.18488/journal.aefr/2015.5.10/102.10.1148.1158
![]() |
[15] |
Bakhsh H, Ong TS, Ho JA, et al. (2018) Organizational Culture and Environmental Performance. Sustainability 10: 1–17. https://doi.org/10.3390/su10082690 doi: 10.3390/su10082690
![]() |
[16] |
Barney JB (1986) Organizational Culture: Can It Be a Source of Sustained Competitive Advantage? Acade Manage Rev 11: 656–665. https://doi.org/10.2307/258317 doi: 10.2307/258317
![]() |
[17] |
Barney JB (1991) Firm Resources and Sustained Competitive Advantage. J Manage 17: 99–120. https://doi.org/10.1177/014920639101700108 doi: 10.1177/014920639101700108
![]() |
[18] |
Barney JB, Ketchen DJ, Wright M (2011) The Future of Resource-Based Theory: Revitalization or Decline? J Manage 37: 1299–1315. https://doi.org/10.1177/0149206310391805 doi: 10.1177/0149206310391805
![]() |
[19] |
Bayard B, Jolly C (2007) Environmental behavior structure and socio-economic conditions of hillside farmers: A multiple-group structural equation modeling approach. Ecol Econ 62: 433–440. https://doi.org/10.1016/j.ecolecon.2006.07.004 doi: 10.1016/j.ecolecon.2006.07.004
![]() |
[20] |
Benjamin A, David I (2012) Human resource development climate and employee commitment in recapitalized Nigerian banks. Int J Bus Manage 7: 90–99. https://doi.org/10.5539/ijbm.v7n5p91 doi: 10.5539/ijbm.v7n5p91
![]() |
[21] |
Bissing-Olson MJ, Fieldin KS, Iyer A (2016) Experiences of pride, not guilt, predict pro-environmental behavior when pro-environmental descriptive norms are more positive. J Environ Psychol 45: 145–153. https://doi.org/10.1016/j.jenvp.2016.01.00 doi: 10.1016/j.jenvp.2016.01.00
![]() |
[22] |
Branco MC, Rodrigues LL (2008) Factors influencing social responsibility disclosure by Portuguese companies. J Bus Ethics 83: 685–701. https://doi.org/10.1007/s10551-007-9658-z doi: 10.1007/s10551-007-9658-z
![]() |
[23] | Brechin S, Kempton W (1994) Global environmentalism: a challenge to the post-materialism thesis? Soc Sci Q 75: 245–269. |
[24] |
Brown ME, Treviño LK, Harrison DA (2005) Ethical leadership: A social learning perspective for construct development and testing. Organ. Behav Hum Decis Process 97: 117–134. https://doi.org/10.1016/j.obhdp.2005.03.002 doi: 10.1016/j.obhdp.2005.03.002
![]() |
[25] | Cameron KS, Quinn RE (2006) Diagnosing and Changing Organizational Culture: Based on the Competing Values Framework San Francisco, CA: Jossey-Bass. |
[26] |
Castro-Casal C, Vila-Vazquez G, Pardo-Gayoso Á (2019) Sustaining affective commitment and Extra-Role service among hospitality employees: interactive effect of empowerment and service training. Sustainability 11: 4092. https://doi.org/10.3390/su11154092 doi: 10.3390/su11154092
![]() |
[27] |
Chen YS, Chang CH (2013) Enhance environmental commitments and green intangible assets toward green competitive advantages: an analysis of structural equation modeling (SEM). Qual Quant 47: 529–543. https://doi.org/10.1007/s11135-011-9535-9 doi: 10.1007/s11135-011-9535-9
![]() |
[28] | Chin WW (1998) The partial least squares approach to structural equation modeling, In: Marcoulides GA (Ed.), Modern Methods for Business Research, Lawrence Erlbaum Associates Publisher, Mahwah, NJ 295–336. |
[29] | Chin WW, Dibbern J (2010) An Introduction to a Permutation Based Procedure for Multi-Group PLS Analysis: Results of Tests of Differences on Simulated Data and a Cross Cultural Analysis of the Sourcing of Information System Services Between Germany and the USA, In: Esposito Vinzi V., Chin W, Henseler J, Wang H (eds), Handbook of Partial Least Squares, Concepts, Methods, and Applications. Springer Handbooks of Computational Statistics: 171–193. |
[30] |
Cho YN, Thyroff A, Rapert MI, et al. (2013) To be or not to be green: Exploring individualism and collectivism as antecedents of environmental behavior. J Bus Res 66: 1052–1059. https://doi.org/10.1016/j.jbusres.2012.08.020 doi: 10.1016/j.jbusres.2012.08.020
![]() |
[31] |
Claessens S, Kelly D, Sibley CG, et al. (2022) Cooperative phenotype predicts climate change belief and pro-environmental behaviour. Sci Rep 12: 1–10. https://doi.org/10.1038/s41598-022-16937-2 doi: 10.1038/s41598-022-16937-2
![]() |
[32] |
Cop S, Alola UV, Alola AA (2020) Perceived behavioral control as a mediator of hotels' green training, environmental commitment, and organizational citizenship behavior: A sustainable environmental practice. Bus Strateg Environ 29: 3495–3508. https://doi.org/10.1002/bse.2592 doi: 10.1002/bse.2592
![]() |
[33] |
Crane A (2000) Corporate greening as amortization. Organ Stud 21: 673–696. https://doi.org/10.1177/0170840600214001 doi: 10.1177/0170840600214001
![]() |
[34] |
Dahling JJ, Chau SL, Mayer DM, et al. (2012) Breaking rules for the right reasons? An investigation of pro-social rule breaking. J Organ Behav 33: 21–42. https://doi.org/10.1002/job.730 doi: 10.1002/job.730
![]() |
[35] |
Delmas MA, Toffel MW (2008) Organizational responses to environmental demands: opening the black box. Strateg Manag J 29: 1027–1055. https://doi.org/10.1002/smj.701 doi: 10.1002/smj.701
![]() |
[36] |
Dierking LD, Adelman LM, Ogden J, et al. (2004) Using a Behavior Change Model to Document the Impact of Visits to Disney's Animal Kingdom: A Study Investigating Intended Conservation Action. Curator Museum J 47: 322–343. https://doi.org/10.1111/j.2151-6952.2004.tb00128.x doi: 10.1111/j.2151-6952.2004.tb00128.x
![]() |
[37] |
Dobson A (2007) Environmental Citizenship: Towards Sustainable Development. Sustainable Development. Sustain Dev 15: 276–285. https://doi.org/10.1002/sd.344 doi: 10.1002/sd.344
![]() |
[38] | European Commission (2005) Winning the Battle Against Global Climate Change. Brussels. |
[39] |
Faul F, Erdfelder E, Lang AG, et al. (2007) G*Power 3: a flexible statistical power analysis program for the social, behavioral, and biomedical sciences. Behav Res Methods 39: 175–191. https://doi.org/10.3758/BF03193146 doi: 10.3758/BF03193146
![]() |
[40] |
Faul F, Erdfelder E, Buchner A, et al. (2009). Statistical power analyses using G-Power 3.1: Tests for correlation and regression analyses. Behav Res Methods 41: 1149–1160. https://doi.org/10.3758/BRM.41.4.1149 doi: 10.3758/BRM.41.4.1149
![]() |
[41] |
Felipe CM, Roldán J, Leal-Rodríguez AL (2017) Impact of Organizational Culture Values on Organizational Agility. Sustainability 9: 1–23. https://doi.org/10.3390/su9122354 doi: 10.3390/su9122354
![]() |
[42] | Ferrel OC, Fraedrich J, Ferrell LC (2019) Business Ethics Ethical Decision Making and Cases. OH, USA: Cengage Publishing. |
[43] |
Fietz B, Günther E (2021). Changing Organizational Culture to Establish Sustainability. Control Manag Rev 65: 32–40. https://doi.org/10.1007/s12176-021-0379-4 doi: 10.1007/s12176-021-0379-4
![]() |
[44] |
Fornell C, Larcker DF (1981) Structural Equation Models with Unobservable Variables and Measurement Error: Algebra and Statistics. J Mark Res 18: 382–388. https://doi.org/10.1177/002224378101800313 doi: 10.1177/002224378101800313
![]() |
[45] |
García-Machado JJ, Barbadilla E, Gutiérrez C (2020) A PLS Multigroup Analysis of the Role Businesswomen in the Tourism in Andalusia. Forum Scientiae Oeconomia 8: 37–57. https://doi.org/10.23762/FSO_VOL8_NO2_3 doi: 10.23762/FSO_VOL8_NO2_3
![]() |
[46] |
Gifford R (2014) Environmental psychology matters. Annu Rev Psychol 65: 1–39. https://doi.org/10.1146/annurev-psych-010213-115048 doi: 10.1146/annurev-psych-010213-115048
![]() |
[47] |
Grant RM (1991) The resource-based theory of competitive advantage: Implications for strategy formulation. Calif Manag Rev 33: 114–135. https://doi.org/10.2307/41166664 doi: 10.2307/41166664
![]() |
[48] |
Gregor SD (2006) The Nature of Theory in Information Systems. MIS Q 30: 611–642. https://doi.org/10.2307/25148742 doi: 10.2307/25148742
![]() |
[49] | Hair JF, Black WC, Babin BJ, et al. (2019a) Multivariate Data Analysis (8th ed.). Cengage Learning, U.K. |
[50] | Hair JF, Hult TG, Ringle CM, et al. (2019b) Manual de Partial Least Squares Structural Equation Modeling (PLS-SEM). SAGE: Spain. |
[51] |
Hair JF, Risher JJ, Sarstedt M, et al. (2019c) When to use and how to report the result of PLS-SEM. Eur Bus Rev 33: 2–24. https://doi.org/10.1108/EBR-11-2018-0203 doi: 10.1108/EBR-11-2018-0203
![]() |
[52] |
Hair JF, Howard M, Nitzl C (2020) Assessing measurement model quality in PLS-SEM using confirmatory composite analysis. J Bus Res 109: 101–110. https://doi.org/10.1016/j.jbusres.2019.11.069 doi: 10.1016/j.jbusres.2019.11.069
![]() |
[53] |
Hair JF, Sarstedt M (2021) Explanation plus Prediction – The Logical Focus of Project Management Research. Proj Manag J 52: 319–322. https://doi.org/10.1177/87569728219999 doi: 10.1177/87569728219999
![]() |
[54] | Hair JF, Hult GTM, Ringle CM, et al. (2022) A Primer on Partial Least Squares Structural Equation Modeling (PLS-SEM), 3rd ed., Sage, Thousand Oaks, CA. |
[55] |
Hart ST (1995) A Natural-Resource-Based View of the Firm. Acad Manage Rev 20: 986–1014. https://doi.org/10.2307/258963 doi: 10.2307/258963
![]() |
[56] |
Hiratsuk J, Perlaviciute G, Steg L (2018) Testing VBN theory in Japan: Relationships between values, beliefs, norms, and acceptability and expected effects of a car pricing policy. Transp Res Part F Traffic Psychol Behav 53: 74–83. https://doi.org/10.1016/j.trf.2017.12.015 doi: 10.1016/j.trf.2017.12.015
![]() |
[57] |
Hu Q, Dinev T, Hart P, et al. (2012) Managing Employee Compliance with Information Security Policies: The Critical Role of Top Management and Organizational Culture. Decis Sci 43: 615–660. https://doi.org/10.1111/j.1540-5915.2012.00361.x doi: 10.1111/j.1540-5915.2012.00361.x
![]() |
[58] |
Interligi L (2010) Compliance culture: A conceptual framework. J Manag Organ 16: 235–249. https://doi.org/10.5172/jmo.16.2.235 doi: 10.5172/jmo.16.2.235
![]() |
[59] |
Isensee C, Teuteberg F, Griese K, et al. (2020) The relationship between organizational culture, sustainability, and digitalization in SMEs: A systematic review. J Clean Prod 275: 1–19. https://doi.org/10.1016/j.jclepro.2020.122944 doi: 10.1016/j.jclepro.2020.122944
![]() |
[60] |
Jody SL, Davis JL, Green JD, et al. (2009) Interdependence with the environment: Commitment, interconnectedness, and environmental behavior. J Environ Psychol 29: 173–180. https://doi.org/10.1016/j.jenvp.2008.11.001 doi: 10.1016/j.jenvp.2008.11.001
![]() |
[61] |
Karatepe T, Ozturen A, Karatepe OM, et al. (2022) Management commitment to the ecological environment, green work engagement and their effects on hotel employees' green work outcomes. Int J Contemp Hosp Manag 34: 3084–3112. https://doi.org/10.1108/IJCHM-10-2021-1242 doi: 10.1108/IJCHM-10-2021-1242
![]() |
[62] |
Karp DD (1996) Values and their effect on pro-environmental behavior. Environ. Behav 28: 111–133. https://doi.org/10.1177/0013916596281006 doi: 10.1177/0013916596281006
![]() |
[63] |
Kock N (2015) Common method bias in PLS-SEM: A full collinearity assessment approach. Int J e-Collab 11: 1–10. https://doi.org/10.4018/ijec.2015100101 doi: 10.4018/ijec.2015100101
![]() |
[64] |
Kollmuss A, Agyeman J (2002) Mind the Gap: Why do people act environmentally and what are the barriers to pro-environmental behavior? Environ Educ Res 8: 239–260. https://doi.org/10.1080/13504620220145401 doi: 10.1080/13504620220145401
![]() |
[65] | Kondalkar VG (2007) Organizational Behavior. New Delhi, India: New Age International Publisher. |
[66] |
Kot S, Haque A, Kozlovski E (2019) Strategic SCM's mediating effect on the sustainable operations: Multinational perspective. Organizacija 52: 219–235. https://doi.org/10.2478/orga-2019-0014 doi: 10.2478/orga-2019-0014
![]() |
[67] |
Kotler P (2011) Reinventing marketing to manage the environmental imperative. J Mark 75: 132–135. https://doi.org/10.1509/jmkg.75.4.132 doi: 10.1509/jmkg.75.4.132
![]() |
[68] |
Lee SC, Huang SYB, Hu L, et al. (2023) Why Do Employees Show Pro-Environmental Behaviors? A Perspective of Environment Social Responsibility. Behav Sci 13: 463. https://doi.org/10.3390/bs13060463 doi: 10.3390/bs13060463
![]() |
[69] |
Lee TH (2011) How recreation involvement, place attachment and conservation commitment affect environmentally responsible behavior. J Sustain Tour 19: 895–915. https://doi.org/10.1080/09669582.2011.570345 doi: 10.1080/09669582.2011.570345
![]() |
[70] |
Leonidou LC, Leonidou CN, Fotiadis TA, et al. (2013) Resources and capabilities as drivers of hotel environmental marketing strategy: implications for competitive advantage and performance. Tourism Manage 35: 94–110. https://doi.org/10.1016/j.tourman.2012.06.003 doi: 10.1016/j.tourman.2012.06.003
![]() |
[71] |
Li Y (2014) Environmental innovation practices and performance: moderating effect of resource commitment. J Clean Prod 66: 450–458. https://doi.org/10.1016/j.jclepro.2013.11.044 doi: 10.1016/j.jclepro.2013.11.044
![]() |
[72] |
Linnenluecke MK, Griffiths A (2010) Corporate sustainability and organizational culture. J World Bus 45: 357–366. https://doi.org/10.1016/j.jwb.2009.08.006 doi: 10.1016/j.jwb.2009.08.006
![]() |
[73] |
Liu N, Tang SY, Zhan X, et al. (2018) Political commitment, policy ambiguity, and corporate environmental practices. Policy Stud J 46: 190–214. https://doi.org/10.1111/psj.12130LiuP, doi: 10.1111/psj.12130LiuP,
![]() |
[74] |
Teng M, Han C (2020) How does environmental knowledge translate into pro-environmental behaviors? The mediating role of environmental attitudes and behavioral intentions. Sci Total Environ 28: 1–14. https://doi.org/10.1016/j.scitotenv.2020.138126 doi: 10.1016/j.scitotenv.2020.138126
![]() |
[75] |
Liu X, Lin KL (2020) Green Organizational Culture, Corporate Social Responsibility Implementation, and Food Safet. Front Psychol 11: 1–7. https://doi.org/10.3389/fpsyg.2020.585435 doi: 10.3389/fpsyg.2020.585435
![]() |
[76] |
Lozano R, Nummert B, Ceulemans K (2016) Elucidating the relationship between Sustainability Reporting and Organisational Change Management for Sustainability. J Clean Prod 125: 168–188. https://doi.org/10.1016/j.jclepro.2016.03.021 doi: 10.1016/j.jclepro.2016.03.021
![]() |
[77] |
Luque-Vílchez M, Mesa-Pérez E, Husillos J, et al. (2019) The influence of pro-environmental managers' personal values on environmental disclosure: The mediating role of the environmental organizational structure. Sustain Account Manag Policy J 10: 41–61. https://doi.org/10.1108/SAMPJ-01-2018-0016 doi: 10.1108/SAMPJ-01-2018-0016
![]() |
[78] |
McCarty JA, Shrum LJ (2001) The influence of individualism, collectivism and locus of control on environmental beliefs and behavior. J Public Policy Mark 20: 93–104. https://doi.org/10.1509/jppm.20.1.93.17291 doi: 10.1509/jppm.20.1.93.17291
![]() |
[79] | McLennan M (2021) The Global Risks Report 2021, 16th Edition. USA: World Economic Forum. |
[80] | Mendis MVS, Welmilla I (2021) Green consciousness of employees, In: Human Resource Management in Challenging Environments: University of Kelaniya, Sri Lanka: 88–106. |
[81] |
Mallett RK, Melchiori K, Strickroth T (2013) Self-confrontation via a carbon footprint calculator increases guilt and support for a proenvironmental group. Ecopsychology 5: 9–16. https://doi.org/10.1089/eco.2012.0067 doi: 10.1089/eco.2012.0067
![]() |
[82] |
Marsina S, Hamranova A, Hrivikova T, et al. (2019) How can project orientation contribute to pro-environmental behavior in private organizations in Slovakia. J Clean Prod 231: 772–782. https://doi.org/10.1016/j.jclepro.2019.05.186 doi: 10.1016/j.jclepro.2019.05.186
![]() |
[83] |
Minelgaitė A, Liobikienė G (2021) Changes in pro-environmental behaviour and its determinants during long-term period in a transition country as Lithuania. Environ Dev Sustain 23: 6083–16099. https://doi.org/10.1007/s10668-021-01329-9 doi: 10.1007/s10668-021-01329-9
![]() |
[84] |
Mirahsani N, Azizan O, Shahriari M, et al. (2023) Green culture toward employee green behavior; the mediation roles of perceived support and green identity. Environ Dev Sustain, 1–14. https://doi.org/10.1007/s10668-023-03291-0 doi: 10.1007/s10668-023-03291-0
![]() |
[85] |
Mirhadian N, Azizan O, Shahriari M (2023) The impact of green culture on employee organizational commitment: The mediating role of green identity. J Hum Behav Soc Environ, 1–21. https://doi.org/10.1080/10911359.2023.2222292 doi: 10.1080/10911359.2023.2222292
![]() |
[86] |
Mohamed YH, Adah-Kole E, Onjewu Witold N (2021) Environmental commitment and innovation as catalysts for export performance in family firms. Technol Forecast Soc Chang 173: 1–2. https://doi.org/10.1016/j.techfore.2021.121085 doi: 10.1016/j.techfore.2021.121085
![]() |
[87] |
Morrison EW (2006) Doing the Job Well: An Investigation of Pro-Social Rule Breaking. J Manage 32: 5–28. https://doi.org/10.1177/0149206305277790 doi: 10.1177/0149206305277790
![]() |
[88] |
Nejati M, Rabiei S, Jabbour CJC (2017). Envisioning the invisible: understanding the synergy between Green Human Resource Management and Green Supply Chain Management in Manufacturing Firms in Iran in light of the moderating effect of employees' resistance to change. J Clean Prod 168: 163–172. https://doi.org/10.1016/j.jclepro.2017.08.213 doi: 10.1016/j.jclepro.2017.08.213
![]() |
[89] |
Nemcsicsné ZA (2007) The role of organisational culture in the environmental awareness of companies. J East Eur Manag Stud 12: 109–131. http://dx.doi.org/10.5771/0949-6181-2007-2-109 doi: 10.5771/0949-6181-2007-2-109
![]() |
[90] | Norton TA, Zacher H, Ashkanasy NM (2015) Pro-environmental organizational culture and climate, In: the psychology of green organizations, Oxford: Oxford Univerisity Press, 322–348. |
[91] |
Park H, Russell C, Lee J (2007) National culture and environmental sustainability: a cross-national analysis. J Econ Finan 31: 104–121. https://doi.org/10.1007/BF02751516 doi: 10.1007/BF02751516
![]() |
[92] |
Pettigrew AM (1979) On Studying Organizational Cultures. Adm Sci Q 24: 570–581. https://doi.org/10.2307/2392363 doi: 10.2307/2392363
![]() |
[93] |
Piwowar-Sule K (2020) Pro-Environmental Organizational Culture: Its Essence and a Concept for Its Operationalization. Sustainability 12: 1–16. https://doi.org/10.3390/su12104197 doi: 10.3390/su12104197
![]() |
[94] |
Podsakoff PM, MacKenzie SB, Lee JY, et al. (2003) Common method biases in behavioral research: A critical review of the literature and recommended remedies. J Appl Psychol 88: 879–903. https://doi.org/10.1037/0021-9010.88.5.879 doi: 10.1037/0021-9010.88.5.879
![]() |
[95] |
Podsakoff PM, MacKenzie SB, Podsakoff NP (2012) Sources of methods bias in social science and recommendations on how to control it. Annu Rev Psychol 63: 539–569. https://doi.org/10.1146/annurev-psych-120710-100452 doi: 10.1146/annurev-psych-120710-100452
![]() |
[96] |
Porter LW, Steers RM, Mowday RT, et al. (1974) Organizational commitment, job satisfaction, and turnover among psychiatric technicians. J Appl Psychol 59: 603–609. https://doi.org/10.1037/h0037335 doi: 10.1037/h0037335
![]() |
[97] |
Post JE, Altma BW (1994) Managing the Environmental Change Process: Barriers and Opportunities. J Organ Chang Manage 7: 64–81. https://doi.org/10.1108/09534819410061388 doi: 10.1108/09534819410061388
![]() |
[98] |
Rahman I, Reynolds D (2016) Predicting green hotel behavioral intentions using a theory of environmental commitment and sacrifice for the environment. Int J Hosp Manag 52: 107–116. https://doi.org/10.1016/j.ijhm.2015.09.007 doi: 10.1016/j.ijhm.2015.09.007
![]() |
[99] |
Reser JP, Bentrupperbaumer JM (2005) What and where are environmental values assessing the impact of current diversity of use of environmental and world heritage values. J Environ Psychol 25: 125–146. https://doi.org/10.1016/j.jenvp.2005.03.002 doi: 10.1016/j.jenvp.2005.03.002
![]() |
[100] |
Riepe C, Liebe U, Fujitani M, et al. (2021) Values, Beliefs, Norms, and Conservation-Oriented Behaviors Native Fish Biodiversity in Rivers: Evidence from Four European Countries. Soc Nat Resour 34: 703–724. https://doi.org/10.1080/08941920.2021.1890865 doi: 10.1080/08941920.2021.1890865
![]() |
[101] | Ringle C, Wende S, Becker J (2015) SmartPLS 3. Boenningstedt: SmartPLS. Available from: https://www.smartpls.com. |
[102] |
Ringov D, Zollo M (2007) The impact of national culture on corporate social performance. Corp Gov 7: 476–485. https://doi.org/10.1108/14720700710820551 doi: 10.1108/14720700710820551
![]() |
[103] | Roldán JL, Cepeda G (2020) Curso de Modelos de Ecuaciones Estructurales Basados en la Varianza: Partial Least Squares (PLS) para Investigadores en Ciencias Sociales (IX Edición), in Módulo 1: Fundamentos básicos (mimeo). Sevilla: Centro de Formación Permanente (Universidad de Sevilla), 164–180. |
[104] |
Saleem M, Qadeer F, Mahmood F, et al. (2021) Inculcation of Green Behavior in Employees: A Multilevel Moderated Mediation Approach. Int J Environ Res Public Health 18: 1–21. https://doi.org/10.3390/ijerph18010331 doi: 10.3390/ijerph18010331
![]() |
[105] | Sanyal U, Pal D (2017) Effect of organizational culture in environmental awareness on pro-environmental behaviour at workplace: A new perspective on organizational sustainability. Int J Comm Manage Res, 60–65. |
[106] |
Sarstedt M, Hair JF, Cheah JH, et al. (2019) How to specify, estimate, and validate higher-order constructs in PLS-SEM. Australas Mark J 27: 197–211. https://doi.org/10.1016/j.ausmj.2019.05.003 doi: 10.1016/j.ausmj.2019.05.003
![]() |
[107] | Sarstedt M, Henseler J, Ringle CM (2011) Multigroup Analysis in Partial Least Squares (PLS) Path Modeling: Alternative Methods and Empirical Results, In: Sarstedt M, Schwaiger M, Taylor CR (Ed.), Measurement and Research Methods in International Marketing (Advances in International Marketing 22), Emerald Group Publishing Limited, Leeds, 195–218. https://doi.org/10.1108/S1474-7979(2011)0000022012 |
[108] | Schein E (1985) Organizational Culture and Leadership: A Dynamic View, Jossey-Bass, San Francisco, CA. |
[109] |
Sendawula K, Bagire V, Mbidde CI, et al. (2021) Environmental commitment and environmental sustainability practices of manufacturing small and medium enterprises in Uganda. J Enter Communities People Places Global Economy 15: 588–607. https://doi.org/10.1108/JEC-07-2020-0132 doi: 10.1108/JEC-07-2020-0132
![]() |
[110] |
Shahriari M, Riahi MT, Azizan O, et al. (2023) The effect of green organizational culture on organizational commitment: The mediating role of job satisfaction. J Hum Behav Soc Environ 33: 180–197. https://doi.org/10.1080/10911359.2022.2029789 doi: 10.1080/10911359.2022.2029789
![]() |
[111] |
Sharma S, Prakash G, Anil K, et al. (2021) Analysing the relationship of adaption of green culture, innovation, green performance for achieving sustainability: Mediating role of employee commitment. J Clean Prod 303: 1–14. https://doi.org/10.1016/j.jclepro.2021.127039 doi: 10.1016/j.jclepro.2021.127039
![]() |
[112] |
Shmueli G, Ray S, Velasquez Estrada JM, et al. (2016) The elephant in the room: Predictive performance of PLS models. J Bus Res 69: 4552–4564. https://doi.org/10.1016/j.jbusres.2016.03.049 doi: 10.1016/j.jbusres.2016.03.049
![]() |
[113] |
Shmueli G, Sarstedt M, Hair J, et al. (2019) Predictive model assessment in PLS-SEM: Guidelines for using PLSpredict. Eur J Market 53: 2322–2347. https://doi.org/10.1108/EJM-02-2019-0189 doi: 10.1108/EJM-02-2019-0189
![]() |
[114] |
Singh H. (2008) Watching (out for) each other: the role of clan controls in managing project teams. Acad Manage Proc 8: 1–6. https://doi.org/10.5465/ambpp.2008.33725181 doi: 10.5465/ambpp.2008.33725181
![]() |
[115] |
Solomon G, Brown I (2020) The influence of organisational culture and information security culture on employee compliance behaviour. J Enterp Inf Manag 34: 1203–1228. https://doi.org/10.1108/JEIM-08-2019-0217 doi: 10.1108/JEIM-08-2019-0217
![]() |
[116] | Steg L, Nordlund A (2019) Theories to explain environmental behaviour, In: Steg L, de Groot JIM (eds.) Environmental psychology: an introduction, 2nd ed., Hoboken, NJ: Wiley & Sons, 217–227. |
[117] |
Stern PC (2000) Toward a coherent theory of environmentally significant behavior. J Soc Issues 56: 407–424. https://doi.org/10.1111/0022-4537.00175 doi: 10.1111/0022-4537.00175
![]() |
[118] |
Sugita M, Takahashi T (2013) Influence of Corporate Culture on Environmental Management Performance: An Empirical Study of Japanese Firms. Corp Soc Responsib Environ Manag 22: 182–192. https://doi.org/10.1002/csr.1346 doi: 10.1002/csr.1346
![]() |
[119] |
Sun X, El Askary A, Meo MS, et al. (2022). Green transformational leadership and environmental performance in small and medium enterprises. Ekon Istraz 35: 5273–5291. https://doi.org/10.1080/1331677X.2021.2025127 doi: 10.1080/1331677X.2021.2025127
![]() |
[120] |
Tapia-Fonllem C, Corral-Verdugo V, Fraijo-Sing B, et al. (2013) Assessing Sustainable Behavior and its Correlates: A Measure of Pro-Ecological, Frugal, Altruistic and Equitable Actions. Sustainability 5: 711–723. https://doi.org/10.3390/su5020711 doi: 10.3390/su5020711
![]() |
[121] |
Tarique AA, Logan J, Thomas E, et al. (2015) Phenotypic, functional, and plasticity features of classical and alternatively activated human macrophages. Am J Respir Cell Mol Biol 53: 676–688. https://doi.org/10.1165/rcmb.2015-0012OC doi: 10.1165/rcmb.2015-0012OC
![]() |
[122] |
Tilleman S (2012) Is employee organizational commitment related to firm environmental sustainability? J Small Bus Entrep 25: 417–431. https://doi.org/10.1080/08276331.2012.10593582 doi: 10.1080/08276331.2012.10593582
![]() |
[123] |
Varela-Candamio L, Novo-Corti I, García-Álvarez MT (2018) The importance of environmental education in the determinants of green behavior: A meta-analysis approach. J Clean Prod 170: 1565–1578. https://doi.org/10.1016/j.jclepro.2017.09.214 doi: 10.1016/j.jclepro.2017.09.214
![]() |
[124] |
Wang CH (2019) How organizational green culture influences green performance and competitive advantage. The mediating role of green innovation. J Manuf Technol Manag. 30: 666–683. https://doi.org/10.1108/JMTM-09-2018-0314 doi: 10.1108/JMTM-09-2018-0314
![]() |
[125] |
Wang S, Li J, Zhao D (2017) Institutional Pressures and Environmental Management Practices: The Moderating Effects of Environmental Commitment and Resource Availability. Bus Strateg Environ 27: 52–69. https://doi.org/10.1002/bse.1983 doi: 10.1002/bse.1983
![]() |
[126] |
Weaver GR, Trevino LK, Cochran PL (1999) Integrated and decoupled corporate social performance: management commitments, external pressures, and corporate ethics practices. Acad Manage J 42: 539–552. https://doi.org/10.2307/256975 doi: 10.2307/256975
![]() |
[127] |
Wernerfelt B (1995) The Resource-Based View of the Firm: Ten Years After. Strateg Manag J 16: 171–174. https://doi.org/10.1002/smj.4250160303 doi: 10.1002/smj.4250160303
![]() |
[128] |
Wijethilake C, Lama T (2019) Sustainability core values and sustainability risk management: Moderating effects of top management commitment and stakeholder pressure. Bus Strateg Environ 28: 143–154. https://doi.org/10.1002/bse.2245 doi: 10.1002/bse.2245
![]() |
[129] |
Williams KJ, Cary J (2002) Landscape preferences, Ecological Quality, and Biodiversity Protection Environ Behav 34: 257–274. https://doi.org/10.1177/0013916502034002006 doi: 10.1177/0013916502034002006
![]() |
[130] | Wong CA, Mohammad SH, Ramachandran S, et al. (2018) Conceptualizing environmental literacy and factors affecting pro-environmental. Int J Bus Soc 19: 28–139. |
[131] |
Wynveen CJ, Wynveen BJ, Sutton SG (2015) Applying the value-belief-norm theory to marine contexts: Implications for encouraging pro-environmental behavior. Coast Manag 43: 84–103. https://doi.org/10.1080/08920753.2014.989149 doi: 10.1080/08920753.2014.989149
![]() |
[132] |
Yang Y, Sun L, Han B, et al. (2023) The Trajectory of Anthropomorphism and Pro-Environmental Behavior: A Serial Mediation Model. Int J Environ Res Public Health 20: 32393. https://doi.org/10.3390/ijerph20032393 doi: 10.3390/ijerph20032393
![]() |
[133] |
Yang-Spencer S, Adams C, Yapa PWS (2013) The mediating effects of the adoption of an environmental information system on top management's commitment and environmental performance. Sustain Account Manag Policy J 4: 75–102. https://doi.org/10.1108/SAMPJ-10-2011-0030 doi: 10.1108/SAMPJ-10-2011-0030
![]() |
[134] |
Ying M, Faraz NA, Ahmed F, et al. (2020) How Does Servant Leadership Foster Employees' Voluntary Green Behavior? A Sequential Mediation Model. Int J Environ Res Public Health 17: 1–21. https://doi.org/10.3390/ijerph17051792 doi: 10.3390/ijerph17051792
![]() |
[135] |
Yong JY, Yusliza MY, Fawehinmi OO (2020) Green human resource management: A systematic literature review from 2007 to 2019. Benchmarking Int J 27: 2005–2027. https://doi.org/10.1108/BIJ-12-2018-0438 doi: 10.1108/BIJ-12-2018-0438
![]() |
[136] |
Yu J, Win-Hung C, Hon P (2017) Organizational visibility, stakeholder environmental pressure and corporate environmental responsiveness in China. Bus Strateg Environ 26: 371–384. https://doi.org/10.1002/bse.1923 doi: 10.1002/bse.1923
![]() |
[137] |
Yu TK, Lin F, Kao KY, et al. (2019). Encouraging Environmental Commitment to Sustainability: An Empirical Study of Environmental Connectedness Theory to Undergraduate Students. Sustainability 11: 342. https://doi.org/10.3390/su11020342 doi: 10.3390/su11020342
![]() |
[138] |
Yusliza MY, Amirudin A, Rahadi RA, et al. (2020) An Investigation of Pro-Environmental Behaviour and Sustainable Development in Malaysia. Sustainability 12: 7083. https://doi.org/10.3390/su12177083 doi: 10.3390/su12177083
![]() |
[139] |
Zaid, AA, Jaaron AAM, Talib Bon A (2018) The impact of green human resource management and green supply chain management practices on sustainable performance: An empirical study. J Clean Prod 204: 965–979. https://doi.org/10.1016/j.jclepro.2018.09.062 doi: 10.1016/j.jclepro.2018.09.062
![]() |
![]() |
![]() |
1. | Le Ren, Peng Yang, Rongchuan Sun, Shumei Yu, Gang Wang, Lining Sun, 2024, Semi-dense Map Reconstruction of Bronchus Based on Prior Feature Correlation, 979-8-3503-6479-8, 263, 10.1109/ISoIRS63136.2024.00058 | |
2. | Bowen Deng, 2024, 3144, 0094-243X, 030008, 10.1063/5.0215489 | |
3. | Hao Qu, 2024, 3194, 0094-243X, 020017, 10.1063/5.0222938 | |
4. | Hao Qu, 2024, 3194, 0094-243X, 020006, 10.1063/5.0222939 |
SIFT | SURF | ORB | Superpoint | Improving Superpoint | |
Extracting time /s | 1.282 | 0.182 | 0.125 | 0.103 | 0.101 |
Match time /s | 0.187 | 0.078 | 0.031 | 0.018 | 0.021 |
Extract point pairs/pairs | 334 | 121 | 268 | 352 | 349 |
Match point pairs/pairs | 334 | 121 | 268 | 263 | 349 |
False match point pair/pair | 79 | 25 | 31 | 13 | 15 |
Effective point proportion /% | 100.00 | 100.00 | 100.00 | 74.72 | 100.00 |
False match rate /% | 23.65 | 20.66 | 11.57 | 4.94 | 4.30 |
SIFT | SURF | ORB | Superpoint | Improving Superpoint | |
Extracting time /s | 1.282 | 0.182 | 0.125 | 0.103 | 0.101 |
Match time /s | 0.187 | 0.078 | 0.031 | 0.018 | 0.021 |
Extract point pairs/pairs | 334 | 121 | 268 | 352 | 349 |
Match point pairs/pairs | 334 | 121 | 268 | 263 | 349 |
False match point pair/pair | 79 | 25 | 31 | 13 | 15 |
Effective point proportion /% | 100.00 | 100.00 | 100.00 | 74.72 | 100.00 |
False match rate /% | 23.65 | 20.66 | 11.57 | 4.94 | 4.30 |