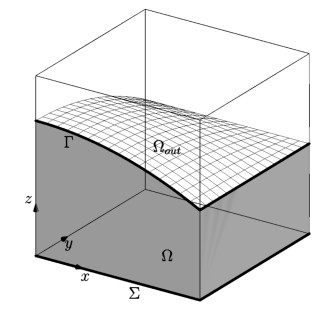
Our aim was to explore the aberrant intrinsic functional topology in methamphetamine-dependent individuals after six months of abstinence using resting-state functional magnetic imaging (rs-fMRI). Eleven methamphetamines (MA) abstainers who have abstained for six months and eleven healthy controls (HC) were recruited for rs-fMRI examination. The graph theory and functional connectivity (FC) analysis were employed to investigate the aberrant intrinsic functional brain topology between the two groups at multiple levels. Compared with the HC group, the characteristic shortest path length (Lp) showed a significant decrease at the global level, while the global efficiency (Eglob) and local efficiency (Eloc) showed an increase considerably. After FDR correction, we found significant group differences in nodal degree and nodal efficiency at the regional level in the ventral attentional network (VAN), dorsal attentional network (DAN), somatosensory network (SMN), visual network (VN) and default mode network (DMN). In addition, the NBS method presented the aberrations in edge-based FC, including frontoparietal network (FPN), subcortical network (SCN), VAN, DAN, SMN, VN and DMN. Moreover, the FC of large-scale functional brain networks revealed a decrease within the VN and SCN and between the networks. These findings suggest that some functions, e.g., visual processing skills, object recognition and memory, may not fully recover after six months of withdrawal. This leads to the possibility of relapse behavior when confronted with MA-related cues, which may contribute to explaining the relapse mechanism. We also provide an imaging basis for revealing the neural mechanism of MA-dependency after six months of abstinence.
Citation: Xiang Li, Jinyu Cong, Kunmeng Liu, Pingping Wang, Min Sun, Benzheng Wei. Aberrant intrinsic functional brain topology in methamphetamine-dependent individuals after six-months of abstinence[J]. Mathematical Biosciences and Engineering, 2023, 20(11): 19565-19583. doi: 10.3934/mbe.2023867
[1] | Hualong Du, Qiuyu Cui, Pengfei Liu, Xin Ma, He Wang . Reformative artificial bee colony algorithm based PID controller for radar servo system. Electronic Research Archive, 2022, 30(8): 2941-2963. doi: 10.3934/era.2022149 |
[2] | Wenbin Zheng, Jinjin Li, Shujiao Liao . Decision self-information based on parameterized fuzzy β neighborhood and its application in three-way multi-attribute group decision-making. Electronic Research Archive, 2022, 30(12): 4553-4573. doi: 10.3934/era.2022231 |
[3] | Meng-Xue Chang, Bang-Sheng Han, Xiao-Ming Fan . Global dynamics of the solution for a bistable reaction diffusion equation with nonlocal effect. Electronic Research Archive, 2021, 29(5): 3017-3030. doi: 10.3934/era.2021024 |
[4] | Wen Li, Jian Wang, Zhanpeng Du, Hongfeng Ma, Lijie Zhang, Libin Duan . Lightweight design method and application of MEWP bracket based on multi-level optimization. Electronic Research Archive, 2022, 30(12): 4416-4435. doi: 10.3934/era.2022224 |
[5] | Sida Lin, Lixia Meng, Jinlong Yuan, Changzhi Wu, An Li, Chongyang Liu, Jun Xie . Sequential adaptive switching time optimization technique for maximum hands-off control problems. Electronic Research Archive, 2024, 32(4): 2229-2250. doi: 10.3934/era.2024101 |
[6] | Peng Gao, Pengyu Chen . Blowup and MLUH stability of time-space fractional reaction-diffusion equations. Electronic Research Archive, 2022, 30(9): 3351-3361. doi: 10.3934/era.2022170 |
[7] | Mingtao Cui, Wennan Cui, Wang Li, Xiaobo Wang . A polygonal topology optimization method based on the alternating active-phase algorithm. Electronic Research Archive, 2024, 32(2): 1191-1226. doi: 10.3934/era.2024057 |
[8] | Ziqiang Wang, Chunyu Cen, Junying Cao . Topological optimization algorithm for mechanical-electrical coupling of periodic composite materials. Electronic Research Archive, 2023, 31(5): 2689-2707. doi: 10.3934/era.2023136 |
[9] | Yuhai Zhong, Huashan Feng, Hongbo Wang, Runxiao Wang, Weiwei Yu . A bionic topology optimization method with an additional displacement constraint. Electronic Research Archive, 2023, 31(2): 754-769. doi: 10.3934/era.2023037 |
[10] | Yineng Ouyang, Zhaotao Liang, Zhihui Ma, Lei Wang, Zhaohua Gong, Jun Xie, Kuikui Gao . A class of constrained optimal control problems arising in an immunotherapy cancer remission process. Electronic Research Archive, 2024, 32(10): 5868-5888. doi: 10.3934/era.2024271 |
Our aim was to explore the aberrant intrinsic functional topology in methamphetamine-dependent individuals after six months of abstinence using resting-state functional magnetic imaging (rs-fMRI). Eleven methamphetamines (MA) abstainers who have abstained for six months and eleven healthy controls (HC) were recruited for rs-fMRI examination. The graph theory and functional connectivity (FC) analysis were employed to investigate the aberrant intrinsic functional brain topology between the two groups at multiple levels. Compared with the HC group, the characteristic shortest path length (Lp) showed a significant decrease at the global level, while the global efficiency (Eglob) and local efficiency (Eloc) showed an increase considerably. After FDR correction, we found significant group differences in nodal degree and nodal efficiency at the regional level in the ventral attentional network (VAN), dorsal attentional network (DAN), somatosensory network (SMN), visual network (VN) and default mode network (DMN). In addition, the NBS method presented the aberrations in edge-based FC, including frontoparietal network (FPN), subcortical network (SCN), VAN, DAN, SMN, VN and DMN. Moreover, the FC of large-scale functional brain networks revealed a decrease within the VN and SCN and between the networks. These findings suggest that some functions, e.g., visual processing skills, object recognition and memory, may not fully recover after six months of withdrawal. This leads to the possibility of relapse behavior when confronted with MA-related cues, which may contribute to explaining the relapse mechanism. We also provide an imaging basis for revealing the neural mechanism of MA-dependency after six months of abstinence.
Reconstructing the stress and deformation history of a sedimentary basin is a challenging and important problem in the geosciences [32]. The mechanical response of a sedimentary basin is the consequence of complex multi-physics processes involving mechanical, geochemical, thermal and hydrological aspects. Among these, the inclusion of erosion or deposition of material plays a key role in the reconstruction of a sedimentary basin history.
A sedimentary basin is usually represented by a porous medium filled with water. To model surface erosion or deposition, we let the top surface of the basin evolve according to a given profile. The dynamics of erosion/deposition is affected by many complex phenomena, which are only partially accounted for in this study. Since the scope of this work is more focused on the methodology, precisely the development of a numerical solver, we address a simplified problem configuration which features a slab of porous, deformable material that is homogeneous, completely saturated with water and affected by a prescribed mixed erosion deposition profile on the top surface. In this way, we combine in a sigle model the mechanical, hydrological and erosion/deposition effects.
In this work we tackle the evolution of the top surface using the cut finite element method (CutFEM). This method is combined with a fixed-stress approach for solving the coupled flow and mechanics models. To deal with a moving boundary, the physical domain, is embedded in a larger computational grid. The evolution of top surface of the slab is described by the zero value of a time dependent level set function. The CutFEM approximation allows to handle the evolution of the top layer of the slab avoiding re-meshing techniques. However, depending on the intersection between the computational grid and the level set function, it can worsen the condition number of the problem. To overcome the ill-conditioning due to the CutFEM approximation, we propose the use of a stabilization terms and of a preconditioner applied to the fixed-stress splitting scheme.
On the basis of seminal works using the finite element method for the approximation of elliptic problems featuring unfitted boundaries and interfaces [9,15,20,21], the CutFEM method was developed and formalized as a general framework for simultaneously discretizing the geometry of the domain and the related partial differential equations [8]. Despite its many advantages, it is well known that this approach significantly worsens the conditioning of the discretized operators, because the finite element discretization is set on cut elements that may become arbitrarily small and skewed, according to the configuration of the unfitted boundary or interface with respect to the computational mesh. To override this issue, several techniques have been proposed. Stabilization techniques acting on the problem formulation have been successfully applied to this purpose [7,9,10,25,29]. It has been also shown that the ill-conditioning of the CutFEM discretization can be addressed by means of suitable preconditioners, [24,28,34]. Such techniques make CutFEM become a stable, efficient and robust method for a wide variety of problems, including second order elliptic equations [11,15,20], incompressible flow problems such as Stokes [19,22,25,28] and Navier-Stokes [29] and linear elasticity models [21]. However, the application of CutFEM to multi-physics problems involving the coupled CutFEM approximation of multiple variables is still rather unexplored. The scope of the present study is to shed light on some of the difficulties that appear in this context and to show how the available theory of CutFEM can be used to overcome these issues. In particular, we address a poromechanics problem modeled by the Biot equations. We believe that this is a particularly interesting case because it couples the equations for incompressible flow in porous media with a linear elasticity model for the deformations of the material. Indeed, it puts together the problems for which CutFEM has been thoroughly studied.
The work is organized as follows. In Section 2 we introduce the mathematical formulation and the discretization of the problem. In Section 3 we discuss the relation between the condition number of the discrete problem and the size of cut elements. Then, we develop the new numerical solver for Biot equations, based on the fixed stress approach combined with stabilization and preconditioners for CutFEM. Illustrative numerical examples are performed to test the proposed algorithm in Section 4, followed by concluding remarks in Section 5.
In this section we present the mathematical setting of the poromechanics problem. Then, we introduce the numerical discretization, obtained by casting the problem into the CutFEM framework.
We deal with the mechanical deformation of a porous medium. We consider for simplicity an isotropic material (named the skeleton) filled with an isothermal single-phase fluid. We also assume that the Hypothesis of Small Perturbations (HSP) holds true, so that the linearity of the problem is ensured. In particular HSP implies several consequences that are described in the following. The material particles of the skeleton are subject to small displacements. This hypothesis allows us to identify the initial spatial configuration and the current configuration of the system. Small variations of the porosity and the fluid density are also considered, namely
|ϕ−ϕ0ϕ0|≃0,|ρf−ρf0ρf0|≃0, | (2.1) |
where the index (0) refers to the reference/initial configuration. This assumption allows us to replace ϕ and ρf with ϕ0 and ρf0 whenever required. We remark that the poromechanics problem is solved only in the physical part of the domain, namely Ω(t). A sketch of a domain example is show in Figure 1.
In particular, it is shown that the upper boundary is denoted by Γ(t) and it is free to move. The lower boudary is denoted with Σ and it is fixed. The lateral sides of the domain are ∂Ω(t)∖(Γ(t)∪Σ). Under the small perturbations hypothesis, the poromechanics problem is modeled through the following system of equations:
−∇⋅σ(u)+α∇p=fin Ω(t), | (2.2) |
∂t(pM+α∇⋅u)−∇⋅K∇p=0in Ω(t), | (2.3) |
where u is the solid matrix displacement vector with respect to the unloaded reference configuration, represented in Figure 1. The function p is the pore pressure and the forcing term f is the volumetric force due to the mass of the porous medium, namely
f(t,x)=−((1−ϕ)ρs+ϕρf)R(t)gez | (2.4) |
being ρs,ρf the densities of the solid and fluid phases, respectively, g the standard gravity, ez the unit vector in the vertical direction and R(t) a ramp function R(t)=η2max[(t+δtgs+|t+δtgs|),1] where η is a suitable slope coefficient to be defined later on and δtgs is the temporal interval needed to reach the geostatic conditions.
We remind that ∂t denotes the standard partial derivative with respect to time in the Eulerian framework. The parameters α, M and K are the Biot number, the Biot modulus and the the hydraulic conductivity, respectively. We recall that the hydraulic conductivity is defined as the ratio between the permeability (ks) and the dynamic viscosity of the fluid (μf), namely K=ks/μf. To complete the definition of the problem we also assume the linear elasticity behavior for the skeleton. This implies that the stress tensor σ, appearing in (2.2), is defined by
σ(u):=2με(u)+λ∇⋅u, | (2.5) |
where μ and λ are the Lamé coefficients and ε(u) is the symmetric gradient of the skeleton displacement, defined as
ε(u):=12(∇u+∇ut). | (2.6) |
For further details on poromechanics, the interested reader is referred to e.g. [13,14].
For a well-posed problem we must complement the previous governing equations with appropriate boundary and initial conditions. We consider the following,
p=0,σ(u)⋅n=0,on Γ(t), | (2.7) |
K∇p⋅n=Kρfg,u=0,on Σ, | (2.8) |
K∇p⋅n=0,σ(u)⋅n=0,on∂Ω(t)∖(Γ(t)∪Σ), | (2.9) |
where n is the unit outward normal to the boundary. Concerning the initial condition we consider the basin to be in the reference unloaded state so that u=0, p=0 enforced at the beginning of simulations t=−δtgs. We point out that, as illustrated in Figure 2, by the time t=0 the basin has reached the geostatic state characterized by u=ugs, p=pgs.
By taking into account the conditions (2.7), (2.8), (2.9), the weak formulation of the problem described in (2.2)–(2.3) reads: for each t∈(0,T], find (u,p)∈V(t)×Q(t) such that
{a(u,v)−α(p,∇⋅v)=(f,v)∀v∈V(t),1M(∂tp,q)+α(∇⋅∂tu,q)+c(p,q)−∫Γ(t)K∇p⋅nq=∫ΣKρfgq∀q∈Q(t), | (2.10) |
where V(t):={u∈(H1(Ω(t)))n∣u∣Σ=0} and Q(t):={p∈H1(Ω(t))∣p∣Γ(t)=0}. Here, H1(Ω) denotes the Hilbert subspace of L2(Ω(t)) of functions with first weak derivatives in L2(Ω(t)), (⋅,⋅) is the standard inner product in the space L2(Ω(t)). Equation (2.10) is characterized by the following bilinear forms
a(u,v):=2∫Ω(t)με(u):ε(v)dΩ+∫Ωλ(∇⋅u)(∇⋅v)dΩ,c(p,q):=∫Ω(t)K∇p⋅∇qdΩ. |
The main difficulty of problem (2.10) consists of the time-dependence of the domain and of the functional spaces. We aim at removing this dependence at the level of numerical discretization by casting the discrete problem into the CutFEM framework.
In the cut finite element method, briefly CutFEM, the boundary of the physical domain is represented on a background grid using a level set function. The background grid is also used to approximate the solution of the governing problem. In this framework the boundary conditions are built into the discrete variational formulation, leading to a method that can handle a time varying domain avoiding mesh moving algorithms or re-meshing techniques. A comprehensive review which also cover the implementation aspects of the CutFEM method can be found in [8,19].
Let ΩT be a bounded computational domain, constant in time, with an interface Γ(t) dividing ΩT into two non-overlapping subdomains Ω(t) and Ωout(t), so that ¯ΩT=¯Ω(t)∪¯Ωout(t), as show in Figure 1. The active zone, Ω(t), represents the physical domain, while Ωout is a fictitious region.
Let Th:={T} denote a triangulation of ΩT, not necessarily conformal to the interface Γ(t). The collection of elements that are intersected by Γ is denoted with ΩΓ:=T∈Th:T∩Γ≠∅.
Let us introduce the discrete spaces
Vrh:={vh∈H1(ΩT):vh|T∈Pr(T),∀T∈Th}d,Qsh:={qh∈H1(ΩT):qh|T∈Ps(T),∀T∈Th}, |
where Ps denotes the space of scalar piecewise polynomials of order s.
We consider a decomposition of the finite element spaces Vrh,Qsh as proposed in [28] and also used in [34]. For simplicity, we address the decomposition for Vrh solely, the one for Qsh follows similarly. We separate the nodes related to each finite element space into the ones neighboring the interface IΓ(Vrh) from the remaining ones in the active part of the domain IΩ(Vrh) and those in the fictitious domain IΩout(Vrh). Precisely, let I(Vrh) be the set of all degrees of freedom of Vrh and let ϕk be the corresponding nodal basis functions. The degrees of freedom related to basis functions entirely supported in Ω and Ωout are IΩ(Vrh):={k: supp(ϕk)⊂Ω} and IΩout(Vrh):={k: supp(ϕk)⊂Ωout} respectively. Their complementary set is IΓ(Vrh)=I(Vrh)∖(IΩ(Vrh)∪IΩout(Vrh). Then, we use those sets to construct the following subspaces,
VΓh:=span{ϕk:k∈IΓ(Vrh)},VΩh:=span{ϕk:k∈IΩ(Vrh)},VΩouth:=span{ϕk:k∈IΩout(Vrh)}, |
and we decompose the finite element space as Vrh=VΓh⊕VΩh⊕VΩouth, so that any test function vh∈Vrh can be uniquely represented as as vh=vΓh+vΩh+vΩouth.
The semi-discrete scheme can be written as follows: find (uh,ph)∈Vrh×Qsh such that:
{a(uh,vh)−α(ph,∇⋅vh)=(f,vh)∀vh∈Vrh,1M(∂tph,qh)+α(∇⋅∂tuh,qh)+c(ph,qh)−∫Γ(t)K∇ph⋅nqh=∫ΣKρfgqh∀qh∈Qsh. | (2.11) |
Since all the integrals are defined either on Ω or on Γ, it would be equivalent to use test functions defined on VΓh⊕VΩh solely. In practice, we find more convenient to use formulation (2.11) and to set the degrees of freedom of IΩout(Vrh) and IΩout(Qsh) to zero.
For the imposition of the pressure constraint in the internal unfitted interface Γ(t) in the second equation of (2.11), we use Nitsche's method following the approaches proposed in the seminal work [20], see also [6,22] for applications of the method to Stokes problem and poromechanics. This technique allows to weakly enforce interface conditions at the discrete level by adding appropriate penalization terms to the variational formulation of the problem. This results in the modified problem formulation,
1M(∂tph,qh)+(α∇⋅∂tuh,qh)+c(ph,qh)−∫Γ(t)K∇ph⋅nqh−∫Γ(t)K∇qh⋅nph+γKh∫Γ(t)phqh=∫ΣKρfgqh∀qh∈Qsh, | (2.12) |
where h is the characteristic size of the quasi-uniform computational mesh and γ>0 denotes a penalization parameter independent on h and on the parameters of the problem. Finally, we consider a backward-Euler time discretization scheme. Denoting with τ the computational time step, the fully discretized problem at time tn, n=1,2,...,N reads as follows: find (unh,pnh)∈Vrh×Qsh such that
{a(unh,vh)−α(pnh,∇⋅vh)=(f,vh),1M(pnh,qnh)+α(∇⋅unh,qh)+τc(pnh,qh)−τ∫Γ(t)K∇pnh⋅nqh−τ∫Γ(t)K∇qh⋅npnh+γKhτ∫Γ(t)pnhqh=∫ΣKρfgqh+1M(pn−1h,qh)+α(∇⋅un−1h,qh) | (2.13) |
for all vh∈Vrh and qh∈Qsh. We notice that the finite element space of pn−1h may not coincide with the one of qh, relative to the current time tn. To compute the integrals on the right hand side, we project pn−1h onto the space QΓh⊕QΩh, such that the degrees of freedom of the projected pn−1h and qh are conformal. If IΩ,n−1(Qsh)∪IΓ,n−1(Qsh)⊂IΩ,n(Qsh)∪IΓ,n(Qsh) the projection is just a simple extension to zero of the new active degrees of freedom. Otherwise, we just discard at time n the degrees of freedom that were active at time n−1. We finally remark that bilinear forms of problem (2.13) are defined either on Ω or Γ. As a result the degrees of freedom IΩout(Vrh),IΩout(Qsh) are not properly constrained. In practice, all the matrix blocks related to these degrees of freedom are set to the identity, with a corresponding null right hand side, such that all the degrees of freedom on Ωout are constrained to vanish.
We focus the attention on the solution of the system (2.13) through the fixed stress iterative scheme as presented e.g. in [4,23,26,30] and used in several studies, see for example [1,3,17,33] as a solver and preconditioner for poromechanics problems.
In this algorithm the flow is solved first, followed by the solution of the mechanical problem. At each time step, the procedure is iterated until the solution converges within an acceptable tolerance.
The first step reads as follows: given (un,kh,pn,kh)∈Vrh×Qsh find pn,k+1h∈Qsh such that
(1M+β)(pn,k+1h,qh)+τc(pn,k+1h,qh)−τ∫ΓK∇pn,k+1h⋅nqhdΓ−τ∫ΓK∇qh⋅npn,k+1hdΓ+γKhτ∫Γpn,k+1hqhdΓ=∫ΣKρfgqh+1M(pn−1h,qh)+(α∇⋅un−1h,qh)+β(pn,kh,qh)−(α∇⋅un,kh,qh) ∀qh∈Qsh, | (2.14) |
where (⋅k) denotes the iteration index. The second step of the algorithm reads: given pn,k+1h∈Qsh find un,k+1h∈Vrh such that
a(un,k+1h,vh)=(f,vh)+α(pn,k+1h,∇⋅vh)∀vh∈Vrh. | (2.15) |
The iterative steps are performed until the following convergence criterion is fulfilled
pn,k+1h−pn,khpn,0h<ηp,un,k+1h−un,khun,0h<ηu, | (2.16) |
where ηp and ηu are the desired tolerances (here chosen equal to 10−7).
For notational convenience, we reformulate the fixed-stress steps in a more compact form as
step 1Bppn,k+1h=Lp(un−1h,un,kh,pn,kh,pn−1h),step 2Buun,k+1h=Lu(pn,k+1h). | (2.17) |
We will refer at Bp and Bu as the system matrices of the first (pressure) and the second (displacement) step of the fixed-stress algorithm.
The real number β≥0 is a stabilization parameter and has to be chosen such that the convergence of the scheme is ensured. We refer to e.g. [23,30] for the choice of β and to [1,4,5,26,31] for a mathematical interpretation and analysis of it. In particular, the optimization of the parameter β is performed in [31]. We remark that all the works mentioned above are referring to classical discretizations of the Biot equations, for the CutFEM method presented in this paper the convergence analysis of the iterative method is thoroughly addressed in Section 3.6.
In this section we analyze the performance of the original fixed stress algorithm, namely (2.17), in order to investigate the effects of the CutFEM discretization in the solution of the problem. To this purpose we use two computational test cases arising from different choices of the finite element space for the discretization of the displacement. In the first test case we take (uh,ph)∈V1h×Q1h while in the second case we choose (uh,ph)∈V2h×Q1h. We consider a cubic computational domain characterized by an edge of 4 Km. The physical domain is described by the negative part of the following level set function
f(x,y,z)=(z−(0.7+14((y/l)2−1)((x/l)2−1))l)(1−t+1.26×1011)+(z−0.844l)t+1.26×1011, | (2.18) |
where t+ is the physical time in the temporal window [0,4Ky] (Ky stands for kilo-years) and l is the characteristic length of the domain. A sketch of the initial geometry of the physical domain is shown in the top left part of Figure 2, while the material properties considered are reported in Table 1. According to (2.18), Γ(t) is such that in some points [x,y] the elevation of ground surface decreases as time evolves, while it increases in some other points. With this choice, we aim at demonstrating the capability of the approach to model erosion as well as deposition of material. On a sufficiently large spatial scale, the two phenomena must necessarily take place together, because of mass conservation.
Young Modulus | E | 1010 | Pa |
Rock density | ρs | 2.2×103 | Kg/m3 |
Rock permeability | ks | 10−13 | m2 |
Water density | ρl | 103 | Kg/m3 |
Water viscosity | μl | 10−3 | Pas |
Dimension | l | 4×103 | m |
To reach the geostatic condition we consider a temporal interval of 3.99Ky in wich the ramp function R(t), introduced in Eq. (2.4), reads R(t)=η2max[(t+δtgs+|t+δtgs|),1] with δtgs=3.99Ky and η=(tr+δtgs+|tr+δtgs|)−1 with tr≈−1Ky. A schematic of the simulation set up is reported in the timeline in the bottom part of Figure 2. We notice that the simulation is split in three parts: before t1=0Ky, between t1=0Ky and t3=3.99Ky and after t3=3.99Ky. The first part from t0=−δtgs to t1 is used to reach the geostatic condition that are reached at t1=0Ky. The second part of the simulation, namely from t1=0Ky to t3=3.99Ky is when the erosion/deposition take place, while between t3 and tend≈8Ky there isn't any change in the basin configuration. We use a time step size of 3.15⋅109s≈0.1Ky so that the simulation requires 40 time steps for the first, the second and the third part. The level set function is updated just for 40 time steps after t1=0 so that remains steady for t>t3=3.99Ky.
The performance of the numerical solver is measured by three indices:
a=npnmax,p,b=nunmax,u,c=nfs, | (2.19) |
where np and nu are the average number of GMRES iterations needed for the solution of the pressure and the displacement subproblem, namely step 1 and step 2 of (2.17), nmax,p and nmax,u are the maximum number of iterations for the pressure and the displacement problem respectively, while the number of fixed stress iterations per time step is nfs.
The behavior of a and b over time for the case (uh,ph)∈V1h×Q1h is reported in the left part of Figure 3. We notice that the average number of iterations needed for the solution of the pressure and the displacement sub-problem (a and b indices) is highly variable and a few peaks can be observed. We remark that the indices a and b are related to the convergence rate of GMRES and, as a consequence, to the conditioning of the two sub-problems. Depending on how the level set intersects the computational domain, the two sub-problems may become ill-conditioned and a larger number of iterations is needed for the fixed stress algorithm to converge. In the first test case, we notice that the indices a and b do not reach their maximum (unit) value, meaning that the sub-problems are properly solved at every time step. In such condition, as we see from the right panel of Figure 3 (c index), the number of fixed stress iterations at each time step remains almost constant.
The behavior of the three indices for the test case (uh,ph)∈V2h×Q1h is reported in Figure 4. We notice that the variability of the indices a and b is greater that in the previous case and furthermore, at certain time steps, the b index reaches its maximum. More precisely, the second step of the fixed stress algorithm does not reach convergence. The index c of Figure 4, shows that the ill-conditioning of the sub-problems affects also the global convergence of the fixed stress algorithm. In particular, we notice that the c index follows the same trend of b and severe peaks occur when the solver of the subproblem b does not converge.
From these preliminary test cases we infer that as long as the sub-problems are accurately solved, the global convergence of the fixed stress algorithm is not significantly affected, while is highly deteriorated when one of the two steps is not properly resolved. Then, the aim of this work is exploring different numerical techniques to overcome the dependence of the sub-problems form the size of cut-elements and, as a consequence, of the fixed stress algorithm from the position of the unfitted interface.
In this section we investigate the spectral properties of the systems associated to the two steps of the fixed stress algorithm. We show that the CutFEM approximation may cause the ill-conditioning of the sub-problems, depending on how the level set function intersects the computational grid. In the second part of the section, we introduce a stabilization term and a preconditioner that cure the ill-conditioning of the CutFEM approximation method.
In the CutFEM framework interface conditions are enforced weakly and numerical integration over sub-elements is required. The integrals over very small sub-elements lead to very small element matrix entries and therefore to ill-conditioned systems. Since the introduction of CutFEM various authors have proposed different techniques to avoid this type of ill-conditioning [7,9,10,24,25,28,29,34]. Among this non exhaustive list, we refer to [7], where the ghost penalty stabilization is introduced.
The idea of [7] is to add a penalty term in the interface zone that extends the coercivity of the (physical) operator also in the region of the computational domain where the solution has no physical significance. Let us introduce the following norms for any qh∈Qsh (for simplicity we use the scalar notation, but these definitions can be straightforwardly extended to vector valued functions)
||qh||0,Ω:=(qh,qh)1/2Ω, ||qh||0,Γ:=(qh,qh)1/2Γ, ||qh||1,h,Ω:=||∇qh||0,Ω+h−1/2||qh||0,Γ. |
Following [7] we introduce the following discrete inequalities that will be used in the analysis of the problem:
Poincaré-Friedrichs inequality||qh||0,Ω≤CP||qh||1,h,Ω, | (3.1) |
Inverse inequality||qh||1,h,Ω≤CIh−1||qh||0,ΩT, | (3.2) |
where the constants CP,CI are independent of the size of cut elements. Furthermore, we need estimates of the discrete functions in terms of their degrees of freedom. More precisely, we denote the degrees of freedom of qh∈Qsh with qh:=∑Ni=1qiϕh,i as q:={qi}Ni=1∈RN. Then, there exist constants cmin,0 and cmax,0 such that
c2min,0hd|q|2≤||qh||20,ΩT≤c2max,0hd|q|2. | (3.3) |
When properties of discrete functions are considered on meshes that are cut by the boundary, the smallest size of cut elements may affect the validity such properties. To define the smallest cut element, we assume there exist a node xn such that the basis function related to such node have small support in the physical domain, i.e. measd(∪T:xn∈TT∩Ω)<<hd. Let xh be the nodal basis function related to that node. Let zn be the node and zh be the basis function such that measd(∪T:zn∈TT∩Ω)/measd(T) is minimal among the whole mesh. Then we define the smallest (relative) support of a basis function defined on cut element as
ν:=measd(∪T:zn∈TT∩Ω)measd(∪T:zn∈TT). | (3.4) |
In the case of a mesh cut by the boundary, following [34] and in particular Lemma 3.3 and Lemma 3.4 respectively, for piecewise linear finite elements, namely r=1,s=1, and for the special function zh related to definition (3.4), we have that there exist constants cmin,1,cmin,2,cmax,1,cmax,2 such that
c2min,1hdν2/d+1|z|2≤||zh||20,Ω≤c2max,1hdν2/d+1|z|2, | (3.5) |
c2min,2hd−2ν|z|2≤||zh||21,h,Ω≤c2max,2hd−2ν|z|2. | (3.6) |
Finally, in Lemma 3.7 of [34] it is shown that there exists a constant CT, independent of the mesh cuts, such that for any qh∈Q1h it holds,
hν1/d||qh||20,Γ≤CT||qh||20,Ω, ∀qh∈Q1h. | (3.7) |
On the basis of these results, in the following we present the analysis of the two sub-problems of the fixed-stress approach, showing how the interface position affects their conditioning in both the standard and the stabilized formulation.
Equation (2.14), with a generic right hand side, can be written in a more compact form as
mh(ph,qh)+τch(ph,qh)=(g,qh), | (3.8) |
where
mh(ph,qh):=(1M+β)(ph,qh)Ω,ch(ph,qh):=(∇ph,∇qh)−(∇ph⋅n,qh)Γ−(∇qh⋅n,ph)Γ+γKh(ph,qh)Γ. |
Before proceeding, we observe that using standard arguments based on the norms and the inequalities defined in the previous Section, it is straightforward to prove the following properties of the pressure problem, with constants mc and Mc uniformly independent to the mesh characteristic size and the cut elements,
Coercivitymc||ph||21,h,Ω≤ch(ph,ph), | (3.9) |
Continuity|ch(ph,qh)|≤Mc||ph||1,h,Ω||qh||1,h,Ω. | (3.10) |
To study the conditioning of the system matrix associated with (3.8), we address the spectral properties of the matrix Bp of (2.17). It is the sum of a mass ad a stiffness matrix, namely Bp=M+C. The eigenvalues/eigenfunctions of (3.8), namely ϑ,yh are the solution of the following problem
mh(yh,qh)+τch(yh,qh)=ϑ(yh,qh)Ω. | (3.11) |
Introducing a suitable parameter CB, equation (3.11) can be written as
τch(yh,qh)=(ϑ−CB)(yh,qh)Ω=ζ(yh,qh)Ω, | (3.12) |
and, as a consequence, the eigenvalues ϑ are related to the eigenvalues ζ associated to the operator ch. For this reason, we study the spectral properties of the matrix associated to (3.12).
Let k(C):=||C||||C−1|| be the condition number of a generic matrix C, where ||C||:=supx∈RN|Cx||x| and |⋅| denotes the euclidean norm on RN. Let us consider x∈RN, associated to xh∈Qsh such that supp(xh)⊂Ω, and therefore ||xh||0,Ω=||xh||0,ΩT. It can be shown, using the coercivity relation (3.9), the Poincaré inequality (3.1) and (3.3) that
||C||=supw∈RN|Cw||w|≥(Cx,x)|x|2≥ch(xh,xh)|x|2≥mc||xh||21,h,Ω|x|2≥mcC−2P||xh||20,Ω|x|2≥mcC−2Pc2min,0hd. |
We focus now on ||C−1||. By definition we have
||C−1||=supw∈RN|C−1w||w|=supw∈RN|w||Cw|≥|z||Cz|. |
Let us now take the special function zh of (3.4),
|Cz|=supw∈RN(Cz,w)|w|=supw∈RNch(zh,wh)|w|. |
Using the continuity of the bilinear form (3.10) combined with (3.6) applied to zh, (3.3) applied to wh, and reminding that |z|=1 we obtain that
ch(zh,wh)≤Mc||zh||1,h,Ω||wh||1,h,Ω≤Mccmax,2h(d−2)/2ν12|z|||wh||1,h,Ω≤Mccmax,2h(d−2)/2ν12CIh−1|z|||wh||0,ΩT≤Mccmax,2h(d−2)/2ν12CIcmax,0h−1hd/2|z||w|. | (3.13) |
In conclusion we have that
k(C)=||C||||C−1||≥mcC−2Pc2min,0McCIcmax,0cmax,2h2ν−1/2 |
meaning that the conditioning of the pressure sub-problem tends to infinity when ν→0.
Let EB be the set of element edges in the boundary zone, more precisely,
EB:={K∩K′, where either K∈ΩΓ or K′∈ΩΓ}. | (3.14) |
Following [11], for any ph and qh∈Q1h the ghost penalty stabilization operator j(⋅,⋅) is given by
jp(ph,qh)=∑E∈EB(γgh∇ph⋅nE,∇qh⋅nE)E, | (3.15) |
and Equation (3.8) is modified as follows,
mh(ph,qh)+τch(ph,qh)+τjp(ph,qh)=(g,qh). | (3.16) |
Then, the bilinear form of the stabilized pressure problem is
Ch(ph,qh):=ch(ph,qh)+jp(ph,qh), | (3.17) |
and we denote by G the related matrix.
In [7] it is shown that the stabilization operator does not affect the continuity and the coercivity of the problem, such that there exist constants mC,MC
mC|||ph|||21,h,ΩT≤Ch(ph,ph),|Ch(ph,qh)|≤MC|||ph|||1,h,ΩT|||qh|||1,h,ΩT. |
Using (3.3) and the standard inverse inequality we obtain,
||G||=supp∈RN|Gp||p|=supp∈RNsupw∈RNCh(ph,wh)|w||p|≤MCC2Ic2max,0hd−2. |
For ||G−1|| using the coercivity, the inverse inequality and the bound (3.3) we have,
mC|||ph|||21,h,ΩT≤Ch(ph,ph)≤|Gp||p|≤|Gp|c−1min,0h−d/2||ph||0,ΩT. |
Using the Poincaré type inequality (3.1) and the bound (3.3)
|||ph|||21,h,ΩT≥C−2P||ph||20,ΩT≥C−2Pcmin,0hd/2||ph||0,ΩT|p|. |
As a result we have
|Gp||p|≥mCC−2Pc2min,0hd,∀p∈RN. |
Using the previous inequality we estimate ||G−1|| as
||G−1||=supw∈RN|G−1w||w|=supp∈RN|p||Gp|≤1mCC−2Pc2min,0hd. |
It follows that k(G)=||G||||G−1||≃h−2 is not dependent by the smallest cut element. The condition number of G indeed scales with h as expected for the standard FEM method.
We focus on the operator a(u,v) defined as
a(uh,vh):=2∫Ωinμε(uh):ε(vh)dΩ+∫Ωinλ(∇⋅uh)(∇⋅vh)dΩ. | (3.18) |
For the upper bound of the bilinear form it can be shown that the following inequalities hold true,
|a(uh,vh)|≤2μ||ε(uh)||0,Ω||ε(vh)||0,Ω+λ||∇⋅uh||||∇⋅vh||≤(2μ+λd)||ε(uh)||0,Ω||ε(vh)||0,Ω≤Ma||uh||1,Ω||vh||1,Ω. | (3.19) |
For the lower bound of the bilinear form we have straightforwardly that a(uh,uh)≥ma||∇uh||20,Ω. Using Korn's inequality, in particular ||uh||0,Ω≤CK||∇uh||0,Ω,∀uh∈[H10(Ω)]d, (see for example [16]) we obtain that
a(uh,uh)≥maC−2K||uh||20,Ω,∀uh∈[H10(Ω)]d. |
We now estimate the condition number of the system matrix A as k(A)=||A||||A−1||. Let us consider xh∈V1h such that supp(xh)⊂Ω. Therefore we have ||xh||0,Ω=||xh||0,ΩT. Using this function in the coercivity of a(⋅,⋅) and exploiting the bound (3.3) we obtain
||A||=supw∈RdN|Aw||w|≥(Ax,x)|x|2≥a(xh,xh)|x|2≥mac2min,0hd. | (3.20) |
As in the previous case we evaluate ||A−1|| as
||A−1||=supw∈RdN|A−1w||w|=supw∈RdN|w||Aw|≥|z||Az|, | (3.21) |
where z is the vector of degrees of freedom of the function zh related to (3.5), (3.6). Then, there holds
|Az|=supw∈RdNa(zh,wh)|w|≤Ma||zh||1,Ω||wh||1,Ω|w|, | (3.22) |
and then following (3.13) we obtain
|Az|≤MaCIcmax,0cmax,2hd−2ν12|z|. |
The condition number is therefore bounded from below by the constant
k(A)=||A||||A−1||≥maC−2Kc2min,0MaCIcmax,0cmax,2h2ν−1/2. |
We notice that, also in the displacement subproblem, the conditioning of the system matrix depends on the smallest cut element.
We address the second step of the fixed-stress approach (2.17) associated to the linear elasticity problem that reads,
Buun,k+1h=Lu(pn,k+1h). | (3.23) |
On the basis of the decomposition Vrh=VΓh⊕VΩh⊕VΩouth, after filtering out the redundant degrees of freedom of VΩouth, the matrix Bu admits the following block representation,
Bu=[BΩuBΩΓu(BΩΓu)tBΓu], |
where
(uΩh)tBΩuvΩh:=a(uΩh,vΩh),(uΓh)tBΓuvΓh:=a(uΓh,vΓh),(uΩh)tBΩΓuvΓh:=a(uΩh,vΓh). |
The system (3.23) is naturally ill-conditioned because it consists of an almost incompressible elasticity problem. In addition, the corresponding matrix is assembled on possibly small cut-elements. We simultaneously reduce the stiffness of the system and cure the instability due to the small cut-elements by means of a preconditioner and a stabilization term. Following [34] we assume that the only genuinely stiff block of Bu is BΩu. As a result we propose the following preconditioner
P:=[BΩu00diag(BΓu)]. | (3.24) |
We solve the problem of Bu by means of preconditioned Krylov iteration that requires the inversion of P. The solution of the diagonal lower block sub-system diag(BΓu) is trivial, while the inversion of the upper block diag(BΩu) is computationally demanding. We observe that the latter operation is the solution of a standard linear elasticity problem. We achieve this task by means of an Algebraic Multigrid (AMG) algorithm, which has been successfully used for this class of operators, as discussed in [18].
We also adopt a stabilization operator for the elasticity problem, to ease the ill-conditioning related to the presence of small cut-elements. Following the approach described in section 3.3 we add to equation (2.15) the following ghost penalty operator
ju(uh,vh)=∑E∈EBr∑i=1(γgh2i−1∂iuh⋅nE,∂ivh⋅nE)E∀uh,vh∈Vrh, | (3.25) |
where ∂i denotes the i−th order derivative and EB is the set of element edges in the boundary zone defined in Eq. (3.14). This is a more general form of the ghost-penalty stabilization operator introduced for the pressure in section 3.3, which can be applied either to linear or quadratic finite elements, namely the case uh∈Vrh with r=1 and 2. For a comprehensive review of the ghost penalty stabilization approach we remand the interested reader to [12]. In conclusion, the stabilized form of the displacement sub-problem reads as,
a(uh,vh)+ju(uh,vh)=(f,vh)+α(ph,∇⋅vh)∀vh∈Vrh. | (3.26) |
In this section we investigate the convergence of the fixed stress algorithm in relation with ill-conditioning of the two sub-problems, due to the presence of small cut-elements. For this reason, we address the pressure and elasticity problems of (2.17) in absence of the ghost penalty stabilizations. Anyway, it is easy to see that the same conclusions of this section apply to the stabilized formulation including jp(⋅,⋅) and ju(⋅,⋅).
Let us introduce the splitting error of the fixed stress approach as
ekp=ph−pkhandeku=uh−ukh. | (3.27) |
We subtract the fixed-stress equations form the monolithic problem formulation. The pressure equation becomes
(1M+β)(ekp,qh)+τc(∇ekp,∇qh)+τγKh(ekp,qh)Γ+τ(K∇ekp⋅n,qh)Γ−τ(K∇qh⋅n,ekp)Γ=β(ek−1p,qh)−α(∇⋅ek−1u,qh),∀qh∈Qsh. | (3.28) |
The displacement error equation reads
2μ(ε(eku),ε(vh))+λ(∇⋅eku,∇⋅vh)=α(ekp,∇vh),∀vh∈Vrh. | (3.29) |
First, we choose the test function as qh=ekp and vh=ek−1u. Equation (3.28) becomes now
(1M+β)||ekp||20,Ω+τK||∇ekp||20,Ω+τγKh||ekp||20,Γ−2Kτ(∇ekp⋅n,ekp)Γ=β(ek−1p,ekp)−α(∇⋅ek−1u,ekp), | (3.30) |
while (3.29) gives
2μ(ε(eku),ε(ek−1u))+λ(∇⋅eku,∇⋅ek−1u)=α(ekp,∇ek−1u). | (3.31) |
Using the polarization identity a⋅b=1/4(a+b)2−1/4(a−b)2 we get
(ε(eku),ε(ek−1u))=14||ε(eku)+ε(ek−1u)||20,Ω−14||ε(eku)−ε(ek−1u)||20,Ω, | (3.32) |
(∇⋅eku,∇⋅ek−1u)=14‖∇⋅eku‖20,Ω−14‖∇⋅ek−1u‖20,Ω, | (3.33) |
and by the the binomial identity (a−b,a)=12a2+12(a−b)2−12b2 we obtain
β||ekp||0,Ω−β(ek−1p,ekp)=β(ekp−ek−1p,ekp)=12||ekp||20,Ω+12||ekp−ek−1p||20,Ω−12||ek−1p||20,Ω. | (3.34) |
Regarding the terms arising from the CutFEM approximation, using (3.7) we obtain,
τK(∇ekp⋅n,ekp)Γ≤τK2δh−1||ekp||2Γ+δ2τKh||∇ekp⋅n||2Γ≤τK2δh−1||ekp||20,Γ+12δτKCTν−1/d||∇ekp||20,Ω. |
By choosing δ=ν1/dCT, 1−δ2CTν1/d=12 and γ=CTν1/d we obtain
τK(1−δ2CTν1/d)||∇ekp||20,Ω+τKh(γ−12δ)||ekp||20,Γ=τK2(||∇ekp||20,Ω+CThν1/d||ekp||20,Γ). | (3.35) |
Using (3.30), (3.31) (3.32), (3.33), (3.34) and (3.35) we get
2M||ekp||20,Ω+τK||∇ekp||20,Ω+τKCThν1/d||ekp||2Γ+β||ekp||20,Ω+β||ekp−ek−1p||20,Ω+μ||ε(eku)+ε(ek−1u)||20,Ω+λ2||∇⋅eku+∇⋅ek−1u||20,Ω−μ||ε(eku)−ε(ek−1u)||20,Ω−λ2||∇⋅eku−∇⋅ek−1u||20,Ω≤β||ek−1p||20,Ω. | (3.36) |
Second, we address the terms arising from the splitting error of the displacement problem. From equation (3.31) taken at iteration k and k−1 and using the test function vh=eku−ek−1u, we have
2μ‖ε(eku−ek−1u)‖20,Ω+λ‖∇⋅(eku−ek−1u)‖20,Ω=α(ekp−ek−1p,∇⋅(eku−ek−1u)). | (3.37) |
We recall that
||∇⋅(eku−ek−1u)||20,Ω≤d||ε(eku−ek−1u)||20,Ω. |
Using Cauchy-Schwarz and Young's inequality we obtain,
α(ekp−ek−1p,∇⋅(eku−ek−1u))≤α2212μd+λ‖ekp−ek−1p‖20,Ω+12(2μd+λ)‖∇⋅(eku−ek−1u)‖20,Ω≤α2212μd+λ‖ekp−ek−1p‖20,Ω+μ||ε(eku−ek−1u)||20,Ω+λ2‖∇⋅(eku−ek−1u)‖20,Ω. |
As a result we have,
μ||ε(eku−ek−1u)||20,Ω+λ2||∇⋅(eku−ek−1u)||20,Ω≤α22(2μd+λ)||ekp−ek−1p||20,Ω. | (3.38) |
Equation (3.38) shows the convergence of the displacement is dominated by the convergence of the pressure. Then, combining inequalities (3.36) and (3.38) we obtain,
2M||ekp||20,Ω+τK||∇ekp||20,Ω+τKCThν1/d||ekp||2Γ+β||ekp||20,Ω+(β−α22(2μd+λ))||ekp−ek−1p||20,Ω+μ||ε(eku)+ε(ek−1u)||20,Ω+λ2||∇⋅eku+∇⋅ek−1u||20,Ω≤β||ek−1p||20,Ω, | (3.39) |
which, on the basis of the Banach contraction theorem, proves that ||ek−1p||20,Ω→0, provided that
β≥α22(2μd+λ). |
Finally, owning to the Poincaré inequality, for the splitting error of the pressure we obtain,
||∇ekp||20,Ω+CThν1/d||ekp||20,Γ≥C−1Pmin(CTν1/d,1)||ekp||20,Ω, | (3.40) |
showing that the global convergence of the fixed stress algorithm is not affected by the position of the unfitted interface Γ, because when ν→0 the constant of (3.40) is uniformly bounded. We conclude that as long as the two sub-problems are well posed, in the case of piecewise linear finite elements for the pressure approximation, the global convergence of the fixed stress algorithm depends only on the material properties of the problem and we expect a constant number of fixed stress iteration. This theoretical result is in accordance on what is obtained in the preliminary test case where the c index remains almost constant as shown in the right part of Figure 3.
In this section we investigate the performance of the stabilization term introduced in Section 3.2 and of the preconditioner described in Section 3.5, by means of several numerical experiments. In Test 1 we consider a simple two dimensional problem, because in such simplified configuration the smallest (relative) size of the cut element (ν) is easily controlled. In the second test, named Test 2, we consider a more realistic three dimensional case.
We consider a squared domain as shown in the left part of Figure 5 with a characteristics length l=4 Km divided into the physical (Ω) and fictitious region (Ωout) by the curve Γ. The material properties are the ones reported in the right panel of Figure 1. Regarding the boundary conditions, we consider vanishing displacement and null fluid flow on the bottom surface y=0, while free stress condition and vanishing pressure are imposed on the remaining faces. The surface Γ is defined as the zero value of the level set function Γ(y)=y−H. Using this type of level set function and a uniform triangulation, we control the minimum dimension of the cut element as shown in the right panel of Figure 5.
Both the first and the second step of the fixed stress algorithm (2.17) (pressure and displacement step, respectively) are solved by means of GMRES. The number of iterations performed by each step is used to investigate the performance of the pressure stabilization and the preconditioner for the displacement. In Figure 6 (panel A) we show the conditioning of the matrices associated to the fixed-stress sub-problems with respect to the minimum cut-element size. From that figure we notice that the numerical experiments are consistent with the lower bound (dashed line) derived in Section 3. However, we notice that the theoretical bound is not sharp, because when ν tends to zero the conditioning of both matrices explode with a rate higher than ν−12. When the pressure stabilization and the preconditioning of the displacement are applied, the condition number of the pressure and the displacement systems become insensitive to the parameter ν, as shown in Figure 6 (panel B). The effect of the pressure stabilization term can be seen in Figure 6 (panel C), where we show the number of GMRES iterations needed by the pressure step of the fixed stress algorithm. We notice that the introduction of the stabilization term makes the number of iterations become insensitive to the parameter ν. Concerning the solver for the displacement, the application of the preconditioner (3.24) requires the inversion of the matrix
P:=[BΩu00diag(BΓu)]. |
In alternative to P, we also consider the diagonal matrix P1 defined as
P1:=[diag(BΩu)00diag(BΓu)]. |
The number of GMRES iterations for the displacement system using these preconditioners, is shown in Figure 6 (Panel D). We notice that both preconditioners optimally cure the stiffness of the matrix due to presence of small cut-elements, because in both cases the convergence rate of GMRES seems to be independent of ν. However, the full preconditioner P is more effective, because it highly reduces the number of GMRES iterations.
In this section we show and comment the results for a more realistic test case. We consider a tridimensional slab subject to mechanical compaction. The physical domain is described by the intersection of a cube, of edge l=4 Km, and the negative part of the level set function defined in (2.18).
A sketch of the physical domain is shown in the top part of Figure 2 at different time steps. We model the slab as a water saturated porous material, characterized by the properties reported in the Table 1. Such parameters are in the range used for modeling sedimentary basins. We remand the interested reader to [2,13,14,27] for further details.
Concerning the boundary conditions we assume that the base of the slab is impermeable and fixed. The lateral surfaces are impermeable, while a free stress condition is imposed for the displacement problem. On the unfitted boundary at the top, we prescribe an homogeneous Dirichlet and an homogeneous Neuman conditions for the pressure and the displacement, respectively. We study the evolution of the slab in a temporal interval as described in Section 2.4. In particular for the second part of the simulation, after the geostatic condition is reached, we investigate the evolution of the slab for 8Ky using a time step of approximately 0.1Ky. In the top part of Figure 7 we show the displacement field at t1=0, t2=1.99 and t3=3.99Ky. The deformed domains (in blue) are obtained warping the current configuration (in gray) by the displacement field magnified by a factor of 60 so that they can be appreciated. We notice that the displacement field is highly driven by the shape of the physical domain. In particular when the top surface is not planar, the largest deformation occurs in correspondence of the thickest zones of the slab while, in the final part of the simulation when the level set is planar, the displacement field becomes almost constant in the cross section of the slab. Similar considerations also apply to the pressure field, as confirmed by the lower part of Figure 7 where we show the geostatic pressure on the left and the difference between the current and the geostatic pressure at t2 and t3.
We now investigate the performance of the numerical solver based on the fixed stress algorithm applied to Test 2. In particular we show that the pressure stabilization together with the preconditioning of the elasticity system are effective to reduce the computational cost of the problem. We run numerical experiments for two discretization choices, (uh,ph)∈V1h×Q1h and (uh,ph)∈V2h×Q1h.
In Figure 8 we show the three indices introduced in Section 2.4, obtained for the case (r,s)=(1,1). We compare the performance of the fixed stress algorithm in the original set up (black curves) and using the stabilization term for the pressure problem, namely jp(⋅,⋅) and the preconditioner P for the elasticity problem, as described in (3.24) (red curves).
From the index a we notice that, using the stabilization term the number of iterations for the pressure problem is reduced. Precisely, the peak that occurs near the time step 30 for the black curve, is no longer present for the red one. Focusing on the index b, we notice that the preconditioner P effectively reduces the number of iterations needed for the solution of the displacement problem. Finally, from the index c we see that the number of fixed stress iterations is almost constant both in the original and the stabilized formulation of the problem. This observation supports the conclusions of Section 3.6, confirming that the global convergence of the fixed stress does not depend on the position of the level set, resulting in an almost constant number of iterations.
The results obtained in the case of ((r,s)=(1,2)) are shown in Figure 9. In particular we compare the following cases:
(nsnp) non stabilized, non preconditioned (black line); it refers to the original formulation of the problem.
(sp) pressure-stabilized and preconditioned (red line); it refers to the stabilized formulation of the pressure problem and the preconditioner P for the elasticity problem.
(ssp) pressure and displacement stabilized and preconditioned (blue line); these data are are obtained using the ghost penalty stabilization ju(⋅,⋅) in the elasticity problem.
Again, we see that the number of iterations for each subproblem of the fixed stress algorithm decreases for both the sp and ssp cases. Concerning the index b, the overall number of iterations for the displacement significantly lowers in the sp and ssp cases, despite some peaks that are still present. However, the major advantage of using the preconditioner and the stabilization term of the displacement is appreciated from the index c. More precisely, we notice that the peaks of the fixed stress iterations drastically decrease in the sp case and almost vanish in the ssp cases, resulting in a great reduction in the computational cost of the whole coupled system.
In this work we have considered a free-boundary poromechanic problem, relevant for studying the erosion/deposition of a sedimentary basin. The mathematical model for this problem consists of Biot's equations with a moving boundary. We have proposed a discretization method based on CutFEM and the fixed-stress splitting scheme. The use of CutFEM avoids re-meshing the computational domain along with the evolution of the boundary. However, it worsens the conditioning of the matrices in the presence of cut-elements of small size. To overcome this issue, we have introduced a stabilisation term into the problem formulation and used a preconditioner for the numerical solver. Illustrative numerical examples have shown that these numerical techniques are effective and enable the application of the combined CutFEM/fixed stress approach to realistic applications in geomechanics.
The authors D.C. and P.Z. acknowledge financial support from ENI S.p.A. The authors F.A.R. and P.Z. thank Equinor for the travel grant through the Akademia program.
The authors declare no conflict of interest in this paper.
[1] |
B. Han, W. M. Compton, C. M. Jones, E. B. Einstein, N. D. Volkow, Methamphetamine use, methamphetamine use disorder, and associated overdose deaths among US adults, JAMA psychiatry, 78 (2021), 1329–1342. https://doi.org/10.1001/jamapsychiatry.2021.2588 doi: 10.1001/jamapsychiatry.2021.2588
![]() |
[2] |
M. Shukla, B. Vincent, Methamphetamine abuse disturbs the dopaminergic system to impair hippocampal-based learning and memory: An overview of animal and human investigations, Neurosci. Biobehav. Rev., 131 (2021), 541–559. https://doi.org/10.1016/j.neubiorev.2021.09.016 doi: 10.1016/j.neubiorev.2021.09.016
![]() |
[3] |
V. Manja, A. Nrusimha, Y. Gao, A. Sheikh, M. McGovern, P. A. Heidenreich, et al., Methamphetamine-associated heart failure: A systematic review of observational studies, Heart, 109 (2023), 168–177. https://doi.org/10.1136/heartjnl-2022-321610 doi: 10.1136/heartjnl-2022-321610
![]() |
[4] |
C. W. Li, S. W. W. Ku, P. Y. Huang, L. Y. Chen, H. T. Wei, C. Strong, et al., Factors associated with methamphetamine dependency among men who have sex with men engaging in chemsex: Findings from the COMeT study in Taiwan, Int. J. Drug Policy, 93 (2021), 103119. https://doi.org/10.1016/j.drugpo.2021.103119 doi: 10.1016/j.drugpo.2021.103119
![]() |
[5] |
S. P. Xu, K. Zhang, T. Y. Luo, Development of the risk of relapse assessment scale for methamphetamine abusers in China, Drug Alcohol Depend., 227 (2021), 108992. https://doi.org/10.1016/j.drugalcdep.2021.108992 doi: 10.1016/j.drugalcdep.2021.108992
![]() |
[6] |
H. Yuan, X. Yu, X. Li, S. Qin, G. Liang, T. Bai, et al., Research on resting spontaneous brain activity and functional connectivity of acupuncture at uterine acupoints, Digital Chin. Med., 5 (2022), 59–67. https://doi.org/10.1016/j.dcmed.2022.03.006 doi: 10.1016/j.dcmed.2022.03.006
![]() |
[7] | X. Li, B. Wei, T. Li, N. Zhang, MwoA auxiliary diagnosis via RSN-based 3D deep multiple instance learning with spatial attention mechanism, in 2020 11th International Conference on Awareness Science and Technology (iCAST), IEEE, (2020), 1–6. https://doi.org/10.1109/iCAST51195.2020.9319486 |
[8] |
A. P. Daiwile, S. Jayanthi, J. L. Cadet, Sex differences in methamphetamine use disorder perused from pre-clinical and clinical studies: Potential therapeutic impacts, Neurosci. Biobehav. Rev., 137 (2022), 104674. https://doi.org/10.1016/j.neubiorev.2022.104674 doi: 10.1016/j.neubiorev.2022.104674
![]() |
[9] |
H. Mizoguchi, K. Yamada, Methamphetamine use causes cognitive impairment and altered decision-making, Neurochem. Int., 124 (2019), 106–113. https://doi.org/10.1016/j.neuint.2018.12.019 doi: 10.1016/j.neuint.2018.12.019
![]() |
[10] |
S. Sabrini, G. Y. Wang, J. C. Lin, J. K. Ian, L. E. Curley, Methamphetamine use and cognitive function: A systematic review of neuroimaging research, Drug Alcohol Depend., 194 (2019), 75–87. https://doi.org/10.1016/j.drugalcdep.2018.08.041 doi: 10.1016/j.drugalcdep.2018.08.041
![]() |
[11] |
S. J. Nieto, L. A. Ray, Applying the addictions neuroclinical assessment to derive neurofunctional domains in individuals who use methamphetamine, Behav. Brain Res., 427 (2022), 113876. https://doi.org/10.1016/j.bbr.2022.113876 doi: 10.1016/j.bbr.2022.113876
![]() |
[12] |
G. X. Liang, X. Li, H. Yuan, M. Sun, S. J. Qin, B. Z. Wei, Abnormal static and dynamic amplitude of low-frequency fluctuations in multiple brain regions of methamphetamine abstainers, Math. Biosci. Eng., 20 (2023), 13318–13333. https://doi.org/10.3934/mbe.2023593 doi: 10.3934/mbe.2023593
![]() |
[13] |
Z. X. Zhang, L. He, S. C. Huang, L. D. Fan, Y. N. Li, P. Li, et al., Alteration of brain structure with long-term abstinence of methamphetamine by voxel-based morphometry, Front. Psychiatry, 9 (2018), 722. https://doi.org/10.3389/fpsyt.2018.00722 doi: 10.3389/fpsyt.2018.00722
![]() |
[14] |
X. T. Li, H. Su, N. Zhong, T. Z. Chen, J. Du, K. Xiao, et al., Aberrant resting-state cerebellar-cerebral functional connectivity in methamphetamine-dependent individuals after six months abstinence, Front. Psychiatry, 11 (2020), 191. https://doi.org/10.3389/fpsyt.2020.00191 doi: 10.3389/fpsyt.2020.00191
![]() |
[15] |
L. Fan, Q. Zhang, S. Liang, H. Li, Z. He, J. Sun, et al., Imaging changes in brain microstructural in long-term abstinent from methamphetamine-dependence (in Chinese), J. Cent. South Univ. (Med. Sci.), 44 (2019), 491–500. https://doi.org/10.11817/j.issn.1672-7347.2019.05.004 doi: 10.11817/j.issn.1672-7347.2019.05.004
![]() |
[16] |
X. Y. Qi, Y. Y. Wang, Y. Z. Lu, Q. Zhao, Y. F. Chen, C. L. Zhou, et al., Enhanced brain network flexibility by physical exercise in female methamphetamine users, Cognit. Neurodyn., 2022 (2022), 1–17. https://doi.org/10.1007/s11571-022-09848-5 doi: 10.1007/s11571-022-09848-5
![]() |
[17] |
C. Y. Jia, Q. F. Long, T. Ernst, Y. Q. Shang, L. D. Chang, T. Adali, Independent component and graph theory analyses reveal normalized brain networks on resting-state functional MRI after working memory training in people with HIV, J. Magn. Reson. Imaging, 57 (2023), 1552–1564. https://doi.org/10.1002/jmri.28439 doi: 10.1002/jmri.28439
![]() |
[18] |
O. Sporns, Graph theory methods: applications in brain networks, Dialogues Clin. Neurosci., 20 (2018), 111–121. https://doi.org/10.31887/DCNS.2018.20.2/osporns doi: 10.31887/DCNS.2018.20.2/osporns
![]() |
[19] |
W. Li, L. Wang, Z. Lyu, J. J. Chen, Y. B. Li, Y. C. Sun, et al., Difference in topological organization of white matter structural connectome between methamphetamine and heroin use disorder, Behav. Brain Res., 422 (2022), 113752. https://doi.org/10.1016/j.bbr.2022.113752 doi: 10.1016/j.bbr.2022.113752
![]() |
[20] |
M. Siyah Mansoory, M. A. Oghabian, A. H. Jafari, A. Shahbabaie, Analysis of resting-state fMRI topological graph theory properties in methamphetamine drug users applying box-counting fractal dimension, Basic Clin. Neurosci., 8 (2017), 371–385. https://doi.org/10.18869/nirp.bcn.8.5.371 doi: 10.18869/nirp.bcn.8.5.371
![]() |
[21] |
Y. A. Zhou, Y. Hu, Q. J. Wang, Z. Yang, J. G. Li, Y. J. Ma, et al., Association between white matter microstructure and cognitive function in patients with methamphetamine use disorder, Hum. Brain Mapp., 44 (2023), 304–314. https://doi.org/10.1002/hbm.26020 doi: 10.1002/hbm.26020
![]() |
[22] |
M. S. Mansoory, A. Allahverdy, M. Behboudi, M. Khodamoradi, Local efficiency analysis of restingstate functional brain network in methamphetamine users, Behav. Brain Res., 434 (2022), 114022. https://doi.org/10.1016/j.bbr.2022.114022 doi: 10.1016/j.bbr.2022.114022
![]() |
[23] |
F. Miraglia, F. Vecchio, C. Pappalettera, L. Nucci, M. Cotelli, E. Judica, et al., Brain connectivity and graph theory analysis in Alzheimer's and Parkinson's Disease: The contribution of electrophysiological techniques, Brain Sci., 12 (2022), 402. https://doi.org/10.3390/brainsci12030402 doi: 10.3390/brainsci12030402
![]() |
[24] |
C. G. Yan, X. D. Wang, X. N. Zuo, Y. F. Zang, DPABI: Data processing & analysis for (resting-state) brain imaging, Neuroinformatics, 14 (2016), 339–351. https://doi.org/10.1007/s12021-016-9299-4 doi: 10.1007/s12021-016-9299-4
![]() |
[25] |
C. G. Yan, R. C. Craddock, Y. He, M. P. Milham, Addressing head motion dependencies for small-world topologies in functional connectomics, Front. Hum. Neurosci., 7 (2013), 910. https://doi.org/10.3389/fnhum.2013.00910 doi: 10.3389/fnhum.2013.00910
![]() |
[26] |
C. Yan, Y. Zang, DPARSF: A MATLAB toolbox for "pipeline" data analysis of resting-state fMRI, Front. Syst. Neurosci., 4 (2010), 13. https://doi.org/10.3389/fnsys.2010.00013 doi: 10.3389/fnsys.2010.00013
![]() |
[27] |
N. U. F. Dosenbach, B. Nardos, A. L. Cohen, D. A. Fair, J. D. Power, J. A. Church, et al., Prediction of individual brain maturity using fMRI, Science, 329 (2010), 1358–1361. https://doi.org/10.1126/science.1194144 doi: 10.1126/science.1194144
![]() |
[28] |
M. Koutrouli, E. Karatzas, D. Paez-Espino, G. A. Pavlopoulos, A Guide to conquer the biological network Era using graph theory, Front. Bioeng. Biotechnol., 8 (2020), 34. https://doi.org/10.3389/fbioe.2020.00034 doi: 10.3389/fbioe.2020.00034
![]() |
[29] |
B. T. T. Yeo, F. M. Krienen, J. Sepulcre, M. R. Sabuncu, D. Lashkari, M. Hollinshead, et al., The organization of the human cerebral cortex estimated by intrinsic functional connectivity, J. Neurophysiol., 106 (2011), 1125–1165. https://doi.org/10.1152/jn.00338.2011 doi: 10.1152/jn.00338.2011
![]() |
[30] |
G. Li, Y. D. Luo, Z. R. Zhang, Y. T. Xu, W. D. Jiao, Y. H. Jiang, et al., Effects of mental fatigue on < i > Small-World < /i > brain functional network organization, Neural Plast., 2019 (2019), 1716074. https://doi.org/10.1155/2019/1716074 doi: 10.1155/2019/1716074
![]() |
[31] |
L. J. Nestor, D. G. Ghahremani, E. D. London, Reduced neural functional connectivity during working memory performance in methamphetamine use disorder, Drug Alcohol Depend., 243 (2023), 109764. https://doi.org/10.1016/j.drugalcdep.2023.109764 doi: 10.1016/j.drugalcdep.2023.109764
![]() |
[32] |
M. Ahmadlou, K. Ahmadi, M. Rezazade, E. Azad-Marzabadi, Global organization of functional brain connectivity in methamphetamine abusers, Clin. Neurophysiol., 124 (2013), 1122–1131. https://doi.org/10.1016/j.clinph.2012.12.003 doi: 10.1016/j.clinph.2012.12.003
![]() |
[33] |
S. Arvin, A. N. Glud, K. Yonehara, Short- and long-range connections differentially modulate the dynamics and state of small-world networks, Front. Comput. Neurosci., 15 (2022), 783474. https://doi.org/10.3389/fncom.2021.783474 doi: 10.3389/fncom.2021.783474
![]() |
[34] |
Y. Liu, Q. Li, T. Y. Zhang, L. Wang, Y. R. Wang, J. J. Chen, et al., Differences in small-world networks between methamphetamine and heroin use disorder patients and their relationship with psychiatric symptoms, Brain Imaging Behav., 16 (2022), 1973–1982. https://doi.org/10.1007/s11682-022-00667-0 doi: 10.1007/s11682-022-00667-0
![]() |
[35] |
H. Khajehpour, B. Makkiabadi, H. Ekhtiari, S. Bakht, A. Noroozi, F. Mohagheghian, Disrupted resting-state brain functional network in methamphetamine abusers: A brain source space study by EEG, PLoS One, 14 (2019), e0226249. https://doi.org/10.1371/journal.pone.0226249 doi: 10.1371/journal.pone.0226249
![]() |
[36] |
F. X. Vollenweider, K. H. Preller, Psychedelic drugs: neurobiology and potential for treatment of psychiatric disorders, Nat. Rev. Neurosci., 21 (2020), 611–624. https://doi.org/10.1038/s41583-020-0367-2 doi: 10.1038/s41583-020-0367-2
![]() |
[37] |
B. Kim, J. Yun, B. Park, Methamphetamine-induced neuronal damage: Neurotoxicity and neuroinflammation, Biomol. Ther., 28 (2020), 381–388. https://doi.org/10.4062/biomolther.2020.044 doi: 10.4062/biomolther.2020.044
![]() |
[38] |
J. Zhang, H. Su, J. Y. Tao, Y. Xie, Y. M. Sun, L. R. Li, et al., Relationship of impulsivity and depression during early methamphetamine withdrawal in Han Chinese population, Addict. Behav., 43 (2015), 7–10. https://doi.org/10.1016/j.addbeh.2014.10.032 doi: 10.1016/j.addbeh.2014.10.032
![]() |
[39] |
A. Scalabrini, B. Vai, S. Poletti, S. Damiani, C. Mucci, C. Colombo, et al., All roads lead to the default-mode network-global source of DMN abnormalities in major depressive disorder, Neuropsychopharmacology, 45 (2020), 2058–2069. https://doi.org/10.1038/s41386-020-0785-x doi: 10.1038/s41386-020-0785-x
![]() |
[40] |
B. L. Foster, S. R. Koslov, L. Aponik-Gremillion, M. E. Monko, B. Y. Hayden, S. R. Heilbronner, A tripartite view of the posterior cingulate cortex, Nat. Rev. Neurosci., 24 (2023), 173–189. https://doi.org/10.1038/s41583-022-00661-x doi: 10.1038/s41583-022-00661-x
![]() |
[41] |
C. Caldinelli, R. Cusack, The fronto-parietal network is not a flexible hub during naturalistic cognition, Hum. Brain Mapp., 43 (2022), 750–759. https://doi.org/10.1002/hbm.25684 doi: 10.1002/hbm.25684
![]() |
[42] |
H. Zheng, Q. Zhou, J. J. Yang, Q. Lu, H. D. Qiu, C. He, et al., Altered functional connectivity of the default mode and frontal control networks in patients with insomnia, CNS Neurosci. Ther., 29 (2023), 2318–2326. https://doi.org/10.1111/cns.14183 doi: 10.1111/cns.14183
![]() |
[43] |
R. De Micco, N. Piramide, F. Di Nardo, M. Siciliano, M. Cirillo, A. Russo, et al., Resting-state network connectivity changes in drug-naive Parkinson's disease patients with probable REM sleep behavior disorder, J. Neural Transm., 130 (2023), 43–51. https://doi.org/10.1007/s00702-022-02565-7 doi: 10.1007/s00702-022-02565-7
![]() |
[44] |
S. Tikoo, F. Cardona, S. Tommasin, C. Giannì, G. Conte, N. Upadhyay, et al., Resting-state functional connectivity in drug-naive pediatric patients with Tourette syndrome and obsessive-compulsive disorder, J. Psychiatr. Res., 129 (2020), 129–140. https://doi.org/10.1016/j.jpsychires.2020.06.021 doi: 10.1016/j.jpsychires.2020.06.021
![]() |
[45] |
G. Dong, E. DeVito, J. Huang, X. Du, Diffusion tensor imaging reveals thalamus and posterior cingulate cortex abnormalities in internet gaming addicts. J. Psychiatr. Res., 46 (2012), 1212–1216. https://doi.org/10.1016/j.jpsychires.2012.05.015 doi: 10.1016/j.jpsychires.2012.05.015
![]() |
[46] |
Y. Katsumi, D. Putcha, R. Eckbo, B. Wong, M. Quimby, S. McGinnis, et al., Anterior dorsal attention network tau drives visual attention deficits in posterior cortical atrophy, Brain, 146 (2023), 295–306. https://doi.org/10.1093/brain/awac245 doi: 10.1093/brain/awac245
![]() |
[47] |
H. Y. Tan, T. Z. Chen, J. Du, R. J. Li, H. F. Jiang, C. L. Deng, et al., Drug-related virtual reality cue reactivity is associated with gamma activity in reward and executive control circuit in methamphetamine use disorders, Arch. Med. Res., 50 (2019), 509–517. https://doi.org/10.1016/j.arcmed.2019.09.003 doi: 10.1016/j.arcmed.2019.09.003
![]() |
[48] |
H. C. Zhao, M. J. Ge, O. Turel, A. Bechara, Q. H. He, Brain modular connectivity interactions can predict proactive inhibition in smokers when facing smoking cues, Addict. Biol., 28 (2023), e13284. https://doi.org/10.1111/adb.13284 doi: 10.1111/adb.13284
![]() |
[49] |
S. Yilmaz, Impaired biological rhythm in men with methamphetamine use disorder: The relationship with sleep quality and depression, J. Subst. Use, 28 (2023), 280–286. https://doi.org/10.1080/14659891.2022.2098847 doi: 10.1080/14659891.2022.2098847
![]() |
[50] |
L. Cerliani, M. Mennes, R. M. Thomas, A. Di Martino, M. Thioux, C. Keysers, Increased functional connectivity between subcortical and cortical resting-state networks in autism spectrum disorder, JAMA Psychiatry, 72 (2015), 767–777. https://doi.org/10.1001/jamapsychiatry.2015.0101 doi: 10.1001/jamapsychiatry.2015.0101
![]() |
[51] |
A. Moaveni, Y. F. Feyzi, S. T. Rahideh, R. Arezoomandan, The relationship between serum brain-derived neurotrophic level and neurocognitive functions in chronic methamphetamine users, Neurosci. Lett., 772 (2022), 136478. https://doi.org/10.1016/j.neulet.2022.136478 doi: 10.1016/j.neulet.2022.136478
![]() |
[52] |
R. Nusslock, G. H. Brody, C. C. Armstrong, A. L. Carroll, L. H. Sweet, T. Y. Yu, et al., Higher peripheral inflammatory signaling associated with lower resting-state functional brain connectivity in emotion regulation and central executive networks, Biol. Psychiatry, 86 (2019), 153–162. https://doi.org/10.1016/j.biopsych.2019.03.968 doi: 10.1016/j.biopsych.2019.03.968
![]() |
[53] |
M. Esposito, M. Tamietto, G. C. Geminiani, A. Celeghin, A subcortical network for implicit visuo-spatial attention: Implications for Parkinson's disease, Cortex, 141 (2021), 421–435. https://doi.org/10.1016/j.cortex.2021.05.003 doi: 10.1016/j.cortex.2021.05.003
![]() |
[54] |
Y. Z. Sun, Y. Ding, T. Y. Yu, C. Y. Chen, P. Li, X. G. Yang, et al., Effect of Tiaoshen acupuncture on anxiety after methamphetamine withdrawal (in Chinese), Chin. Acupunct. Moxibust., 42 (2022), 277–280. https://doi.org/10.13703/j.0255-2930.20210213-k0001 doi: 10.13703/j.0255-2930.20210213-k0001
![]() |
[55] |
R. K. Meleppat, C. R. Fortenbach, Y. F. Jian, E. S. Martinez, K. Wagner, B. S. Modjtahedi, et al., In vivo imaging of retinal and choroidal morphology and vascular plexuses of vertebrates using swept-source optical coherence tomography, Transl. Vision Sci. Technol., 11 (2022), 11. https://doi.org/10.1167/tvst.11.8.11 doi: 10.1167/tvst.11.8.11
![]() |
[56] |
R. K. Meleppat, K. E. Ronning, S. J. Karlen, M. E. Burns, E. N. Pugh, R. J. Zawadzki, In vivo multimodal retinal imaging of disease-related pigmentary changes in retinal pigment epithelium, Sci. Rep., 11 (2021), 16252. https://doi.org/10.1038/s41598-021-95320-z doi: 10.1038/s41598-021-95320-z
![]() |
[57] |
R. K. Meleppat, P. F. Zhang, M. J. Ju, S. K. Manna, Y. Jian, E. N. Pugh, et al., Directional optical coherence tomography reveals melanin concentration-dependent scattering properties of retinal pigment epithelium, J. Biomed. Opt., 24 (2019), 066011. https://doi.org/10.1117/1.Jbo.24.6.066011 doi: 10.1117/1.Jbo.24.6.066011
![]() |
[58] |
K. M. Ratheesh, L. K. Seah, V. M. Murukeshan, Spectral phase-based automatic calibration scheme for swept source-based optical coherence tomography systems, Phys. Med. Biol., 61 (2016), 7652–7663. https://doi.org/10.1088/0031-9155/61/21/7652 doi: 10.1088/0031-9155/61/21/7652
![]() |
1. | Yuhai Zhong, Huashan Feng, Hongbo Wang, Runxiao Wang, Weiwei Yu, A bionic topology optimization method with an additional displacement constraint, 2023, 31, 2688-1594, 754, 10.3934/era.2023037 | |
2. | Giovanna C. Andrade, Sandra A. Santos, A level-set-based topology optimization strategy using radial basis functions and a Hilbertian velocity extension, 2022, 111, 0307904X, 108, 10.1016/j.apm.2022.06.026 | |
3. | Fengling Jin, Ye Yang, Zhibin Xiao, Boqing Gao, Haopeng Lou, Shear wall layout optimization of multi-tower buildings based on conceptual design and extended evolutionary structural optimization method, 2023, 0305-215X, 1, 10.1080/0305215X.2022.2161535 | |
4. | Davide Cortellessa, Nicola Ferro, Simona Perotto, Stefano Micheletti, Enhancing level set-based topology optimization with anisotropic graded meshes, 2023, 447, 00963003, 127903, 10.1016/j.amc.2023.127903 | |
5. | An Xu, Shumin Li, Jiyang Fu, Anil Misra, Ruohong Zhao, A hybrid method for optimization of frame structures with good constructability, 2023, 276, 01410296, 115338, 10.1016/j.engstruct.2022.115338 | |
6. | Anatoliy Martynyuk, Gani Stamov, Ivanka Stamova, Yulya Martynyuk–Chernienko, Regularization scheme for uncertain fuzzy differential equations: Analysis of solutions, 2023, 31, 2688-1594, 3832, 10.3934/era.2023195 | |
7. | Feiteng Cheng, Qinghai Zhao, Zibin Mao, Fajie Wang, Mechanical Response of Gradient Lattice Structures Based on Topology Optimization, 2024, 26, 1438-1656, 10.1002/adem.202301887 | |
8. | Qianfeng Cao, Ning Dai, Jiuyang Bai, Hongqing Dai, A dimensionality reduction optimization method for thin-walled parts to realize mass center control and lightweight design, 2024, 0305-215X, 1, 10.1080/0305215X.2024.2350648 | |
9. | Martin Sotola, Pavel Marsalek, David Rybansky, Jan Kopacka, Dusan Gabriel, Ondrej Jezek, Ludek Kovar, Josef Tejc, Miloslav Pasek, Radim Halama, Michal Barnovsky, Application of Surface-Based Smoothing Methods for Topology Optimization Results, 2024, 16, 1758-8251, 10.1142/S1758825124500868 | |
10. | Mingtao Cui, Wang Li, Guang Li, Xiaobo Wang, The asymptotic concentration approach combined with isogeometric analysis for topology optimization of two-dimensional linear elasticity structures, 2023, 31, 2688-1594, 3848, 10.3934/era.2023196 | |
11. | Qianqian Sui, Jun Yan, Zhirui Fan, Zhihui Liu, Stress-constrained topology optimization for multi-material thermo-hyperelastic compliant mechanisms based on inverse motion, 2024, 0305-215X, 1, 10.1080/0305215X.2023.2301552 | |
12. | Mingtao Cui, Wennan Cui, Wang Li, Xiaobo Wang, A polygonal topology optimization method based on the alternating active-phase algorithm, 2024, 32, 2688-1594, 1191, 10.3934/era.2024057 | |
13. | Mohammed Hameed Hafeeth, Ying Liu, An efficient volume-preserving binary filter for additive manufacturing in topology optimization, 2024, 0305-215X, 1, 10.1080/0305215X.2024.2382799 | |
14. | Xingjun Gao, Longhua Li, Yingxiong Li, Meiling Dai, Robust topology optimization of multi-material structures based on the material-field series-expansion method, 2024, 0305-215X, 1, 10.1080/0305215X.2024.2340062 | |
15. | Xingjun Gao, Longhua Li, Jingtong Chen, Yingxiong Li, Robust topology optimization of multi-material structures with overhang angle constraints using the material field series-expansion method, 2024, 69, 23520124, 107359, 10.1016/j.istruc.2024.107359 | |
16. | J. Li, B. Kang, Y. Sun, Y. Huang, J. Guo, Z. Gao, Dynamic Characteristic Analysis of the Shield Machine Three-Stage Planetary Reducer Considering Tooth Wear, 2024, 12, 2523-3920, 7291, 10.1007/s42417-023-01188-w | |
17. | Yun Ni, Jinqing Zhan, Min Liu, Topological design of continuum structures with global stress constraints considering self-weight loads, 2023, 31, 2688-1594, 4708, 10.3934/era.2023241 | |
18. | Wangyu Liu, Guanghui Huang, Weigui Xie, An efficient cross-platform multi-material topology optimization approach occupying enhanced BESO method, 2024, 0025-6455, 10.1007/s11012-024-01916-w | |
19. | Hongshuo Fan, Jianli Liu, Haobo Zhang, Tao Nie, Jingui Yu, Jianzhong Yang, Zhaohui Xia, Evolutionary topology optimization for elastoplastic structures via isogeometric analysis, 2025, 0305-215X, 1, 10.1080/0305215X.2024.2443738 | |
20. | Qiwei Hu, Shengbo Hu, Mengxia Liu, A Multi-Objective Nutcracker Optimization Algorithm Based on Cubic Chaotic Map for Numerical Association Rule Mining, 2025, 15, 2076-3417, 1611, 10.3390/app15031611 | |
21. | Rongbo Zhou, Shuli Sun, A degree-based topological optimization method for triangular meshes, 2025, 0020-7160, 1, 10.1080/00207160.2025.2466765 | |
22. | Fangyi Li, Yuming Yin, Rong Zeng, Dachang Zhu, Topology optimization and mechanical performance analysis of P-type triply periodic minimal surface structures, 2025, 47, 1678-5878, 10.1007/s40430-025-05439-7 | |
23. | Tianbo Wang, Zhaoyi Luo, Minxiang Wei, Jiang Wu, Wenshuai Xue, Ranran Liu, An improved scheme based on the variable density method for structural topology optimization, 2025, 0954-4062, 10.1177/09544062251324422 |
Young Modulus | E | 1010 | Pa |
Rock density | ρs | 2.2×103 | Kg/m3 |
Rock permeability | ks | 10−13 | m2 |
Water density | ρl | 103 | Kg/m3 |
Water viscosity | μl | 10−3 | Pas |
Dimension | l | 4×103 | m |