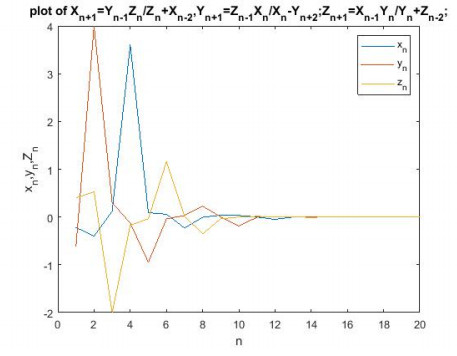
Fire incidents near power transmission lines pose significant safety hazards to the regular operation of the power system. Therefore, achieving fast and accurate smoke detection around power transmission lines is crucial. Due to the complexity and variability of smoke scenarios, existing smoke detection models suffer from low detection accuracy and slow detection speed. This paper proposes an improved model for smoke detection in high-voltage power transmission lines based on the improved YOLOv7-tiny. First, we construct a dataset for smoke detection in high-voltage power transmission lines. Due to the limited number of real samples, we employ a particle system to randomly generate smoke and composite it into randomly selected real scenes, effectively expanding the dataset with high quality. Next, we introduce multiple parameter-free attention modules into the YOLOv7-tiny model and replace regular convolutions in the Neck of the model with Spd-Conv (Space-to-depth Conv) to improve detection accuracy and speed. Finally, we utilize the synthesized smoke dataset as the source domain for model transfer learning. We pre-train the improved model and fine-tune it on a dataset consisting of real scenarios. Experimental results demonstrate that the proposed improved YOLOv7-tiny model achieves a 2.61% increase in mean Average Precision (mAP) for smoke detection on power transmission lines compared to the original model. The precision is improved by 2.26%, and the recall is improved by 7.25%. Compared to other object detection models, the smoke detection proposed in this paper achieves high detection accuracy and speed. Our model also improved detection accuracy on the already publicly available wildfire smoke dataset Figlib (Fire Ignition Library).
Citation: Chen Chen, Guowu Yuan, Hao Zhou, Yutang Ma, Yi Ma. Optimized YOLOv7-tiny model for smoke detection in power transmission lines[J]. Mathematical Biosciences and Engineering, 2023, 20(11): 19300-19319. doi: 10.3934/mbe.2023853
[1] | Ahmed Ghezal, Mohamed Balegh, Imane Zemmouri . Solutions and local stability of the Jacobsthal system of difference equations. AIMS Mathematics, 2024, 9(2): 3576-3591. doi: 10.3934/math.2024175 |
[2] | Hashem Althagafi, Ahmed Ghezal . Solving a system of nonlinear difference equations with bilinear dynamics. AIMS Mathematics, 2024, 9(12): 34067-34089. doi: 10.3934/math.20241624 |
[3] | M. T. Alharthi . Correction: On the solutions of some systems of rational difference equations. AIMS Mathematics, 2025, 10(2): 2277-2278. doi: 10.3934/math.2025105 |
[4] | M. T. Alharthi . On the solutions of some systems of rational difference equations. AIMS Mathematics, 2024, 9(11): 30320-30347. doi: 10.3934/math.20241463 |
[5] | Eunjung Lee, Dojin Kim . Stability analysis of the implicit finite difference schemes for nonlinear Schrödinger equation. AIMS Mathematics, 2022, 7(9): 16349-16365. doi: 10.3934/math.2022893 |
[6] | Shulan Kong, Chengbin Wang, Yawen Sun . A recursive filter for a class of two-dimensional nonlinear stochastic systems. AIMS Mathematics, 2025, 10(1): 1741-1756. doi: 10.3934/math.2025079 |
[7] | Yeyang Jiang, Zhihua Liao, Di Qiu . The existence of entire solutions of some systems of the Fermat type differential-difference equations. AIMS Mathematics, 2022, 7(10): 17685-17698. doi: 10.3934/math.2022974 |
[8] | Abdulghani R. Alharbi . Traveling-wave and numerical solutions to nonlinear evolution equations via modern computational techniques. AIMS Mathematics, 2024, 9(1): 1323-1345. doi: 10.3934/math.2024065 |
[9] | Ibraheem M. Alsulami, E. M. Elsayed . On a class of nonlinear rational systems of difference equations. AIMS Mathematics, 2023, 8(7): 15466-15485. doi: 10.3934/math.2023789 |
[10] | Nan Li, Jiachuan Geng, Lianzhong Yang . Some results on transcendental entire solutions to certain nonlinear differential-difference equations. AIMS Mathematics, 2021, 6(8): 8107-8126. doi: 10.3934/math.2021470 |
Fire incidents near power transmission lines pose significant safety hazards to the regular operation of the power system. Therefore, achieving fast and accurate smoke detection around power transmission lines is crucial. Due to the complexity and variability of smoke scenarios, existing smoke detection models suffer from low detection accuracy and slow detection speed. This paper proposes an improved model for smoke detection in high-voltage power transmission lines based on the improved YOLOv7-tiny. First, we construct a dataset for smoke detection in high-voltage power transmission lines. Due to the limited number of real samples, we employ a particle system to randomly generate smoke and composite it into randomly selected real scenes, effectively expanding the dataset with high quality. Next, we introduce multiple parameter-free attention modules into the YOLOv7-tiny model and replace regular convolutions in the Neck of the model with Spd-Conv (Space-to-depth Conv) to improve detection accuracy and speed. Finally, we utilize the synthesized smoke dataset as the source domain for model transfer learning. We pre-train the improved model and fine-tune it on a dataset consisting of real scenarios. Experimental results demonstrate that the proposed improved YOLOv7-tiny model achieves a 2.61% increase in mean Average Precision (mAP) for smoke detection on power transmission lines compared to the original model. The precision is improved by 2.26%, and the recall is improved by 7.25%. Compared to other object detection models, the smoke detection proposed in this paper achieves high detection accuracy and speed. Our model also improved detection accuracy on the already publicly available wildfire smoke dataset Figlib (Fire Ignition Library).
This paper is devoted to study the expressions forms of the solutions and periodic nature of the following third-order rational systems of difference equations
xn+1=yn−1znzn±xn−2,yn+1=zn−1xnxn±yn−2, zn+1=xn−1ynyn±zn−2, |
with initial conditions are non-zero real numbers.
In the recent years, there has been great concern in studying the systems of difference equations. One of the most important reasons for this is a exigency for some mechanization which can be used in discussing equations emerge in mathematical models characterizing real life situations in economic, genetics, probability theory, psychology, population biology and so on.
Difference equations display naturally as discrete peer and as numerical solutions of differential equations having more applications in ecology, biology, physics, economy, and so forth. For all that the difference equations are quite simple in expressions, it is frequently difficult to realize completely the dynamics of their solutions see [1,2,3,4,5,6,7,8,9,10,11,12,13,14,15,16,17,18,19] and the related references therein.
There are some papers dealed with the difference equations systems, for example, The periodic nature of the solutions of the nonlinear difference equations system
An+1=1Cn,Bn+1=BnAn−1Bn−1,Cn+1=1An−1, |
has been studied by Cinar in [7].
Almatrafi [3] determined the analytical solutions of the following systems of rational recursive equations
xn+1=xn−1yn−3yn−1(±1±xn−1yn−3),yn+1=yn−1xn−3xn−1(±1±yn−1xn−3). |
In [20], Khaliq and Shoaib studied the local and global asymptotic behavior of non-negative equilibrium points of a three-dimensional system of two order rational difference equations
xn+1=xn−1ε+xn−1yn−1zn−1,yn+1=yn−1ζ+xn−1yn−1zn−1, zn+1=zn−1η+xn−1yn−1zn−1. |
In [9], Elabbasy et al. obtained the form of the solutions of some cases of the following system of difference equations
xn+1=a1+a2yna3zn+a4xn−1zn, yn+1=b1zn−1+b2znb3xnyn+b4xnyn−1,zn+1=c1zn−1+c2znc3xn−1yn−1+c4xn−1yn+c5xnyn. |
In [12], Elsayed et al. have got the solutions of the systems of rational higher order difference equations
An+1=1An−pBn−p,Bn+1=An−pBn−pAn−qBn−q, |
and
An+1=1An−pBn−pCn−p,Bn+1=An−pBn−pCn−pAn−qBn−qCn−q,Cn+1=An−qBn−qCn−qAn−rBn−rCn−r. |
Kurbanli [25,26] investigated the behavior of the solutions of the following systems
An+1=An−1An−1Bn−1,Bn+1=Bn−1Bn−1An−1, Cn+1=1CnBn,An+1=An−1An−1Bn−1,Bn+1=Bn−1Bn−1An−1, Cn+1=Cn−1Cn−1Bn−1. |
In [32], Yalçınkaya has obtained the conditions for the global asymptotically stable of the system
An+1=BnAn−1+aBn+An−1,Bn+1=AnBn−1+aAn+Bn−1. |
Zhang et al. [39] investigated the persistence, boundedness and the global asymptotically stable of the solutions of the following system
Rn=A+1Qn−p, Qn=A+Qn−1Rn−rQn−s. |
Similar to difference equations and systems were studied see [21,22,23,24,27,28,29,30,31,32,33,34,35,36,37,38].
In this section, we obtain the expressions form of the solutions of the following three dimension system of difference equations
xn+1=yn−1znzn+xn−2,yn+1=zn−1xnxn+yn−2, zn+1=xn−1ynyn+zn−2, | (1) |
where n∈N0 and the initial conditions are non-zero real numbers.
Theorem 1. We assume that {xn,yn,zn} are solutions of system (1).Then
x6n−2=ak3nn−1∏i=0(a+(6i)k)(a+(6i+2)k)(a+(6i+4)k),x6n−1=bf3nn−1∏i=0(g+(6i+1)f)(g+(6i+3)f)(g+(6i+5)f),x6n=c3n+1n−1∏i=0(d+(6i+2)c)(d+(6i+4)c)(d+(6i+6)c),x6n+1=ek3n+1(a+k)n−1∏i=0(a+(6i+3)k)(a+(6i+5)k)(a+(6i+7)k), |
x6n+2=f3n+2(g+2f)n−1∏i=0(g+(6i+4)f)(g+(6i+6)f)(g+(6i+8)f),x6n+3=hc3n+2(d+c)(d+3c)n−1∏i=0(d+(6i+5)c)(d+(6i+7)c)(d+(6i+9)c), |
y6n−2=dc3nn−1∏i=0(d+(6i)c)(d+(6i+2)c)(d+(6i+4)c),y6n−1=ek3nn−1∏i=0(a+(6i+1)k)(a+(6i+3)k)(a+(6i+5)k),y6n=f3n+1n−1∏i=0(g+(6i+2)f)(g+(6i+4)f)(g+(6i+6)f),y6n+1=hc3n+1(d+c)n−1∏i=0(d+(6i+3)c)(d+(6i+5)c)(d+(6i+7)c),y6n+2=k3n+2(a+2k)n−1∏i=0(a+(6i+4)k)(a+(6i+6)k)(a+(6i+8)k),y6n+3=bf3n+2(g+f)(g+3f)n−1∏i=0(g+(6i+5)f)(g+(6i+7)f)(g+(6i+9)f), |
and
z6n−2=gf3nn−1∏i=0(g+(6i)f)(g+(6i+2)f)(g+(6i+4)f),z6n−1=hc3nn−1∏i=0(d+(6i+1)c)(d+(6i+3)c)(d+(6i+5)c),z6n=k3n+1n−1∏i=0(a+(6i+2)k)(a+(6i+4)k)(a+(6i+6)k),z6n+1=bf3n+1(g+f)n−1∏i=0(g+(6i+3)f)(g+(6i+5)f)(g+(6i+7)f), |
z6n+2=c3n+2(d+2c)n−1∏i=0(d+(6i+4)c)(d+(6i+6)c)(d+(6i+8)c),z6n+3=ek3n+2(a+k)(a+3k)n−1∏i=0(a+(6i+5)k)(a+(6i+7)k)(a+(6i+9)k), |
where x−2=a, x−1=b, x0=c, y−2=d, y−1=e, y0=f, z−2=g, z−1=h and z0=k.
Proof. For n=0 the result holds. Now assume that n>1 and that our assumption holds for n−1, that is,
x6n−8=ak3n−3n−2∏i=0(a+(6i)k)(a+(6i+2)k)(a+(6i+4)k),x6n−7=bf3n−3n−2∏i=0(g+(6i+1)f)(g+(6i+3)f)(g+(6i+5)f),x6n−6=c3n−2n−2∏i=0(d+(6i+2)c)(d+(6i+4)c)(d+(6i+6)c),x6n−5=ek3n−2(a+k)n−2∏i=0(a+(6i+3)k)(a+(6i+5)k)(a+(6i+7)k),x6n−4=f3n−1(g+2f)n−2∏i=0(g+(6i+4)f)(g+(6i+6)f)(g+(6i+8)f),x6n−3=hc3n−1(d+c)(d+3c)n−2∏i=0(d+(6i+5)c)(d+(6i+7)c)(d+(6i+9)c), |
y6n−8=dc3n−3n−2∏i=0(d+(6i)c)(d+(6i+2)c)(d+(6i+4)c),y6n−7=ek3n−3n−2∏i=0(a+(6i+1)k)(a+(6i+3)k)(a+(6i+5)k),y6n−6=f3n−2n−2∏i=0(g+(6i+2)f)(g+(6i+4)f)(g+(6i+6)f), |
y6n−5=hc3n−2(d+c)n−2∏i=0(d+(6i+3)c)(d+(6i+5)c)(d+(6i+7)c),y6n−4=k3n−1(a+2k)n−2∏i=0(a+(6i+4)k)(a+(6i+6)k)(a+(6i+8)k),y6n−3=bf3n−1(g+f)(g+3f)n−2∏i=0(g+(6i+5)f)(g+(6i+7)f)(g+(6i+9)f), |
and
z6n−8=gf3n−3n−2∏i=0(g+(6i)f)(g+(6i+2)f)(g+(6i+4)f),z6n−7=hc3n−3n−2∏i=0(d+(6i+1)c)(d+(6i+3)c)(d+(6i+5)c),z6n−6=k3n−2n−2∏i=0(a+(6i+2)k)(a+(6i+4)k)(a+(6i+6)k),z6n−5=bf3n−2(g+f)n−2∏i=0(g+(6i+3)f)(g+(6i+5)f)(g+(6i+7)f),z6n−4=c3n−1(d+2c)n−2∏i=0(d+(6i+4)c)(d+(6i+6)c)(d+(6i+8)c),z6n−3=ek3n−1(a+k)(a+3k)n−2∏i=0(a+(6i+5)k)(a+(6i+7)k)(a+(6i+9)k). |
It follows from Eq (1) that
x6n−2=y6n−4z6n−3z6n−3+x6n−5=(k3n−1(a+2k)n−2∏i=0(a+(6i+4)k)(a+(6i+6)k)(a+(6i+8)k) )(ek3n−1(a+k)(a+3k)n−2∏i=0(a+(6i+5)k)(a+(6i+7)k)(a+(6i+9)k) )(ek3n−1(a+k)(a+3k)n−2∏i=0(a+(6i+5)k)(a+(6i+7)k)(a+(6i+9)k) )+(ek3n−2(a+k)n−2∏i=0(a+(6i+3)k)(a+(6i+5)k)(a+(6i+7)k) )=(k3n(a+2k)n−2∏i=0(a+(6i+4)k)(a+(6i+6)k)(a+(6i+8)k))(a+3k)n−2∏i=0(a+(6i+9)k)[(k(a+3k)n−2∏i=0(a+(6i+9)k))+(1n−2∏i=0(a+(6i+3)k))]=(k3n(a+2k)n−2∏i=0(a+(6i+4)k)(a+(6i+6)k)(a+(6i+8)k))[k+((a+3k)n−2∏i=0(a+(6i+9)k)n−2∏i=0(a+(6i+3)k))]=(k3n(a+2k)n−2∏i=0(a+(6i+4)k)(a+(6i+6)k)(a+(6i+8)k))[k+(a+(6n−3)k)]=ak3na(a+2k)(a+(6n−2)k)n−2∏i=0(a+(6i+4)k)(a+(6i+6)k)(a+(6i+8)k). |
Then we see that
x6n−2=k3nn−1∏i=0(a+(6i)k)(a+(6i+2)k)(a+(6i+4)k). |
Also, we see from Eq (1) that
y6n−2=z6n−4x6n−3x6n−3+y6n−5=(c3n−1(d+2c)n−2∏i=0(d+(6i+4)c)(d+(6i+6)c)(d+(6i+8)c) )(hc3n−1(d+c)(d+3c)n−2∏i=0(d+(6i+5)c)(d+(6i+7)c)(d+(6i+9)c) )(hc3n−1(d+c)(d+3c)n−2∏i=0(d+(6i+5)c)(d+(6i+7)c)(d+(6i+9)c) )+(hc3n−2(d+c)n−2∏i=0(d+(6i+3)c)(d+(6i+5)c)(d+(6i+7)c) )=(c3n(d+2c)n−2∏i=0(d+(6i+4)c)(d+(6i+6)c)(d+(6i+8)c))(d+3c)n−2∏i=0(d+(6i+9)c)[(c(d+3c)n−2∏i=0(d+(6i+9)c))+(1n−2∏i=0(d+(6i+3)c))]=(c3n(d+2c)n−2∏i=0(d+(6i+4)c)(d+(6i+6)c)(d+(6i+8)c))[c+d+(6n−3)c]=c3n[d+(6n−2)c](d+2c)n−2∏i=0(d+(6i+4)c)(d+(6i+6)c)(d+(6i+8)c). |
Then
y6n−2=dc3nn−1∏i=0(d+(6i)c)(d+(6i+2)c)(d+(6i+4)c). |
Finally from Eq (1), we see that
z6n−2=x6n−4y6n−3y6n−3+z6n−5=(f3n−1(g+2f)n−2∏i=0(g+(6i+4)f)(g+(6i+6)f)(g+(6i+8)f) )(bf3n−1(g+f)(g+3f)n−2∏i=0(g+(6i+5)f)(g+(6i+7)f)(g+(6i+9)f) )(bf3n−1(g+f)(g+3f)n−2∏i=0(g+(6i+5)f)(g+(6i+7)f)(g+(6i+9)f) )+(bf3n−2(g+f)n−2∏i=0(g+(6i+3)f)(g+(6i+5)f)(g+(6i+7)f) )=(f3n(g+2f)n−2∏i=0(g+(6i+4)f)(g+(6i+6)f)(g+(6i+8)f))(g+3f)n−2∏i=0(g+(6i+9)f)[(f(g+3f)n−2∏i=0(g+(6i+9)f))+(1n−2∏i=0(g+(6i+3)f))]=(f3n(g+2f)n−2∏i=0(g+(6i+4)f)(g+(6i+6)f)(g+(6i+8)f))[f+((g+3f)n−2∏i=0(g+(6i+9)f)n−2∏i=0(g+(6i+3)f))]=(f3n(g+2f)n−2∏i=0(g+(6i+4)f)(g+(6i+6)f)(g+(6i+8)f))[f+(g+(6n−3)f)]=f3n(g+(6n−2)f)(g+2f)n−2∏i=0(g+(6i+4)f)(g+(6i+6)f)(g+(6i+8)f). |
Thus
z3n−2=gf3nn−1∏i=0(g+(6i)f)(g+(6i+2)f)(g+(6i+4)f). |
By similar way, one can show the other relations. This completes the proof.
Lemma 1. Let {xn,yn,zn} be a positive solution of system (1), then all solution of (1) is bounded and approaching to zero.
Proof. It follows from Eq (1) that
xn+1=yn−1znzn+xn−2≤yn−1, yn+1=zn−1xnxn+yn−2≤zn−1,zn+1=xn−1ynyn+zn−2≤xn−1, |
we see that
xn+4≤yn+2, yn+2≤zn, zn≤xn−2, ⇒ xn+4<xn−2,yn+4≤zn+2, zn+2≤xn, xn≤yn−2, ⇒ yn+4<yn−2,zn+4≤xn+2, xn+2≤yn, yn≤zn−2, ⇒ zn+4<zn−2, |
Then all subsequences of {xn,yn,zn} (i.e., for {xn} are {x6n−2}, {x6n−1}, {x6n}, {x6n+1}, {x6n+2}, {x6n+3} are decreasing and at that time are bounded from above by K,L and M since K=max{x−2,x−1,x0,x1,x2,x3}, L=max{y−2,y−1,y0,y1,y2,y3} and M=max{z−2,z−1,z0,z1,z2,z3}.
Example 1. We assume an interesting numerical example for the system (1) with x−2=−.22,x−1=−.4, x0=.12,y−2=−.62, y−1=4, y0=.3,z−2=.4,z−1=.53 andz0=−2. (See Figure 1).
In this section, we get the solution's form of the following system of difference equations
xn+1=yn−1znzn+xn−2,yn+1=zn−1xnxn+yn−2, zn+1=xn−1ynyn−zn−2, | (2) |
where n∈N0 and the initial values are non-zero real numbers with x−2≠±z0,≠−2z0, z−2≠y0,≠2y0,≠3y0 and y−2≠2x0,≠±x0.
Theorem 2. Assume that {xn,yn,zn} are solutions of (2). Then for n=0,1,2,...,
x6n−2=(−1)nk3na2n−1(a+2k)n, x6n−1=(−1)nbf3n(f−g)2n(3f−g)n, x6n=(−1)nc3n+1d2n(2c−d)n,x6n+1=ek3n+1(a−k)n(a+k)2n+1, x6n+2=(−1)nf3n+2gn(2f−g)2n+1, x6n+3=(−1)nhc3n+2(c−d)2n+1(c+d)n+1, |
y6n−2=(−1)nc3nd2n−1(2c−d)n, y6n−1=ek3n(a−k)n(a+k)2n, y6n=(−1)nf3n+1gn(2f−g)2n,y6n+1=(−1)nhc3n+1(c−d)2n(c+d)n+1, y6n+2=(−1)nk3n+2a2n(a+2k)n+1, y6n+3=(−1)nbf3n+2(f−g)2n+1(3f−g)n+1, |
and
z6n−2=(−1)nf3ngn−1(2f−g)2n, z6n−1=(−1)nhc3n(c−d)2n(c+d)n, z6n=(−1)nk3n+1a2n(a+2k)n,z6n+1=(−1)nbf3n+1(f−g)2n+1(3f−g)n, z6n+2=(−1)n+1c3n+2d2n+1(2c−d)n, z6n+3=−ek3n+2(a−k)n(a+k)2n+2, |
where x−2=a, x−1=b, x0=c, y−2=d, y−1=e, y0=f, z−2=g, z−1=h and z0=k.
Proof. The result is true for n=0. Now suppose that n>0 and that our claim verified for n−1. That is,
x6n−8=(−1)n−1k3n−3a2n−3(a+2k)n−1, x6n−7=(−1)n−1bf3n−3(f−g)2n−2(3f−g)n−1, x6n−6=(−1)n−1c3n−2d2n−2(2c−d)n−1,x6n−5=ek3n−2(a−k)n−1(a+k)2n−1, x6n−4=(−1)n−1f3n−1gn−1(2f−g)2n−1, x6n−3=(−1)n−1hc3n−1(c−d)2n−1(c+d)n, |
y6n−8=(−1)n−1c3n−3d2n−3(2c−d)n−1, y6n−7=ek3n−3(a−k)n−1(a+k)2n−2, y6n−6=(−1)n−1f3n−2gn−1(2f−g)2n−2,y6n−5=(−1)n−1hc3n−2(c−d)2n−2(c+d)n, y6n−4=(−1)n−1k3n−1a2n−2(a+2k)n, y6n−3=(−1)n−1bf3n−1(f−g)2n−1(3f−g)n, |
and
z6n−8=(−1)n−1f3n−3gn−2(2f−g)2n−2, z6n−7=(−1)n−1hc3n−3(c−d)2n−2(c+d)n−1, z6n−6=(−1)n−1k3n−2a2n−2(a+2k)n−1,z6n−5=(−1)n−1bf3n−2(f−g)2n−1(3f−g)n−1, z6n−4=(−1)nc3n−1d2n−1(2c−d)n−1, z6n−3=−ek3n−1(a−k)n−1(a+k)2n. |
Now from Eq (2), it follows that
x6n−2=y6n−4z6n−3z6n−3+x6n−5=((−1)n−1k3n−1a2n−2(a+2k)n)(−ek3n−1(a−k)n−1(a+k)2n)(−ek3n−1(a−k)n−1(a+k)2n)+(ek3n−2(a−k)n−1(a+k)2n−1)=((−1)nk3na2n−2(a+2k)n)(−k+a+k)=(−1)nk3na2n−1(a+2k)n,y6n−2=z6n−4x6n−3x6n−3+y6n−5=((−1)nc3n−1d2n−1(2c−d)n−1)((−1)n−1hc3n−1(c−d)2n−1(c+d)n)((−1)n−1hc3n−1(c−d)2n−1(c+d)n)+((−1)n−1hc3n−2(c−d)2n−2(c+d)n)=((−1)nc3nd2n−1(2c−d)n−1)c+c−d=(−1)nc3nd2n−1(2c−d)n,z6n−2=x6n−4y6n−3y6n−3−z6n−5=((−1)n−1f3n−1gn−1(2f−g)2n−1)((−1)n−1bf3n−1(f−g)2n−1(3f−g)n)((−1)n−1bf3n−1(f−g)2n−1(3f−g)n)−((−1)n−1bf3n−2(f−g)2n−1(3f−g)n−1)=((−1)n−1f3ngn−1(2f−g)2n−1)(f−3f+g)=(−1)nf3ngn−1(2f−g)2n. |
Also, we see from Eq (2) that
x6n−1=y6n−3z6n−2z6n−2+x6n−4=((−1)n−1bf3n−1(f−g)2n−1(3f−g)n)((−1)nf3ngn−1(2f−g)2n)((−1)nf3ngn−1(2f−g)2n)+((−1)n−1f3n−1gn−1(2f−g)2n−1)=((−1)nbf3n(f−g)2n−1(3f−g)n)(−f+2f−g)=(−1)nbf3n(f−g)2n(3f−g)n,y6n−1=z6n−3x6n−2x6n−2+y6n−4=(−ek3n−1(a−k)n−1(a+k)2n)((−1)nk3na2n−1(a+2k)n)((−1)nk3na2n−1(a+2k)n)+((−1)n−1k3n−1a2n−2(a+2k)n)=(ek3n(a−k)n−1(a+k)2n)−k+a=ek3n(a−k)n(a+k)2n,z6n−1=x6n−3y6n−2y6n−2−z6n−4=((−1)n−1hc3n−1(c−d)2n−1(c+d)n)((−1)nc3nd2n−1(2c−d)n)((−1)nc3nd2n−1(2c−d)n)−((−1)nc3n−1d2n−1(2c−d)n−1)=((−1)n−1hc3n(c−d)2n−1(c+d)n)c−(2c−d)=(−1)nhc3n(c−d)2n(c+d)n. |
Also, we can prove the other relations.
Example 2. See below Figure 2 for system (2) with the initial conditions x−2=11,x−1=5, x0=13,y−2=6, y−1=7, y0=3,z−2=14, z−1=9 andz0=2.
Here, we obtain the form of solutions of the system
xn+1=yn−1znzn+xn−2,yn+1=zn−1xnxn−yn−2, zn+1=xn−1ynyn+zn−2, | (3) |
where n∈N0 and the initial values are non-zero real numbers with x−2≠±z0,≠2z0, z−2≠±y0,≠−2y0 and y−2≠x0,≠2x0,≠3x0.
Theorem 3. If {xn,yn,zn} are solutions of system (3) where x−2=a, x−1=b, x0=c, y−2=d, y−1=e, y0=f, z−2=g, z−1=h and z0=k. Then for n=0,1,2,...,
x6n−2=k3na2n−1(a−2k)n, x6n−1=(−1)nbf3n(f−g)n(f+g)2n, x6n=(−1)nc3n+1dn(d−2c)2n,x6n+1=(−1)nek3n+1(a−k)2n(a+k)n+1, x6n+2=(−1)nf3n+2g2n(2f+g)n+1, x6n+3=(−1)nhc3n+2(c−d)2n+1(3c−d)n+1, |
y6n−2=(−1)nc3ndn−1(d−2c)2n, y6n−1=(−1)nek3n(a−k)2n(a+k)n, y6n=(−1)nf3n+1g2n(2f+g)n,y6n+1=(−1)nhc3n+1(c−d)2n+1(3c−d)n, y6n+2=−k3n+2a2n+1(a−2k)n, y6n+3=(−1)nbf3n+2(f−g)n(f+g)2n+2, |
and
z6n−2=(−1)nf3ng2n−1(2f+g)n, z6n−1=(−1)nhc3n(c−d)2n(3c−d)n, z6n=k3n+1a2n(a−2k)n,z6n+1=(−1)nbf3n+1(f−g)n(f+g)2n+1, z6n+2=(−1)nc3n+2dn(2c−d)2n+1, z6n+3=(−1)n+1ek3n+2(a−k)2n+1(a+k)n+1. |
Proof. As the proof of Theorem 2 and so will be left to the reader.
Example 3. We put the initials x−2=8,x−1=15, x0=13,y−2=6,y−1=7, y0=3,z−2=14,z−1=19 andz0=2, for the system (3), see Figure 3.
The following systems can be treated similarly.
In this section, we deal with the solutions of the following system
xn+1=yn−1znzn−xn−2,yn+1=zn−1xnxn+yn−2, zn+1=xn−1ynyn+zn−2, | (4) |
where n∈N0 and the initial values are non-zero real with x−2≠z0,≠2z0,≠3z0, z−2≠±y0,≠2y0 and y−2≠±x0,≠−2x0.
Theorem 4. The solutions of system (4) are given by
x6n−2=(−1)nk3nan−1(a−2k)2n, x6n−1=(−1)nbf3n(f−g)2n(f+g)n, x6n=(−1)nc3n+1d2n(d+2c)n,x6n+1=−ek3n+1(a−k)2n+1(a−3k)n, x6n+2=(−1)n+1f3n+2g2n+1(2f−g)n, x6n+3=(−1)n+1hc3n+2(c−d)n(c+d)2n+2, |
y6n−2=(−1)nc3nd2n−1(d+2c)n, y6n−1=ek3n(a−k)2n(a−3k)n, y6n=(−1)nf3n+1g2n(2f−g)n,y6n+1=(−1)nhc3n+1(c+d)2n+1(c−d)n, y6n+2=−k3n+2an(a−2k)2n+1, y6n+3=(−1)nbf3n+2(f−g)2n+1(f+g)n+1, |
and
z6n−2=(−1)nf3ng2n−1(2f−g)n, z6n−1=(−1)nhc3n(c+d)2n(c−d)n, z6n=(−1)nk3n+1an(a−2k)2n,z6n+1=(−1)nbf3n+1(f−g)2n(f+g)n+1, z6n+2=(−1)nc3n+2d2n(2c+d)n+1, z6n+3=ek3n+2(a−k)2n+1(a−3k)n+1, |
where x−2=a, x−1=b, x0=c, y−2=d, y−1=e, y0=f, z−2=g, z−1=h and z0=k.
Example 4. Figure 4 shows the behavior of the solution of system (4) with x−2=18,x−1=−15, x0=3,y−2=6, y−1=.7, y0=−3, z−2=4,z−1=−9 andz0=5.
In this section, we obtain the solutions of the difference system
xn+1=yn−1znzn−xn−2,yn+1=zn−1xnxn−yn−2, zn+1=xn−1ynyn−zn−2, | (5) |
where the initials are arbitrary non-zero real numbers with x−2≠z0, z−2≠y0 and y−2≠x0.
Theorem 5. If {xn,yn,zn} are solutions of system (5) where x−2=a, x−1=b, x0=c, y−2=d, y−1=e, y0=f, z−2=g, z−1=h and z0=k. Then
x6n−2=k3na3n−1, x6n−1=bf3n(f−g)3n, x6n=c3n+1d3n,x6n+1=ek3n+1(k−a)3n+1, x6n+2=f3n+2g3n+1, x6n+3=hc3n+2(c−d)3n+2, |
y6n−2=c3nd3n−1, y6n−1=ek3n(k−a)3n, y6n=f3n+1g3n,y6n+1=hc3n+1(c−d)3n+1, y6n+2=k3n+2a3n+1, y6n+3=bf3n+2(f−g)3n+2, |
and
z6n−2=f3ng3n−1, z6n−1=hc3n(c−d)3n, z6n=k3n+1a3n,z6n+1=bf3n+1(f−g)3n+1, z6n+2=c3n+2d3n+1, z6n+3=ek3n+2(k−a)3n+2. |
Example 5. Figure 5 shows the dynamics of the solution of system (5) with x−2=18,x−1=−15,x0=3,y−2=6,y−1=.7, y0=−3,z−2=4,z−1=−9 andz0=5.
This paper discussed the expression's form and boundedness of some systems of rational third order difference equations. In Section 2, we studied the qualitative behavior of system xn+1=yn−1znzn+xn−2,yn+1=zn−1xnxn+yn−2, zn+1=xn−1ynyn+zn−2, first we have got the form of the solutions of this system, studied the boundedness and gave numerical example and drew it by using Matlab. In Section 3, we have got the solution's of the system xn+1=yn−1znzn+xn−2,yn+1=zn−1xnxn+yn−2, zn+1=xn−1ynyn−zn−2, and take a numerical example. In Sections 4–6, we obtained the solution of the following systems respectively, xn+1=yn−1znzn+xn−2,yn+1=zn−1xnxn−yn−2, zn+1=xn−1ynyn+zn−2, xn+1=yn−1znzn−xn−2,yn+1=zn−1xnxn+yn−2, zn+1=xn−1ynyn+zn−2, and xn+1=yn−1znzn−xn−2,yn+1=zn−1xnxn−yn−2, zn+1=xn−1ynyn−zn−2. Also, in each case we take a numerical example to illustrates the results.
This project was funded by the Deanship of Scientific Research (DSR) at King Abdulaziz University, Jeddah, under grant no. (G: 233–130–1441). The authors, therefore, acknowledge with thanks DSR for technical and financial support.
All authors declare no conflicts of interest in this paper.
[1] |
Z. B. Zhao, Z. G. Jiang, Y. X. Li, Y. C. Qi, Y. J. Zhai, W. Q. Zhao, et al., Overview of visual defect detection of transmission line components, J. Image Graphics, 26 (2021), 2545–2560. https://doi.org/10.11834/jig.200689 doi: 10.11834/jig.200689
![]() |
[2] |
Y. Sui, P. F. Ning, P. J. Niu, C. Y. Wang, D. Zhao, W. L. Zhang, et al, Review on mounted UAV for transmission line inspection, Power Syst. Technol., 45 (2021), 3636–3648. http://doi.org/10.13335/j.1000-3673.pst.2020.1178 doi: 10.13335/j.1000-3673.pst.2020.1178
![]() |
[3] |
Z. Y. Liu, X. R. Miu, J. Chen, H. Jiang, Review of visible image intelligent processing for transmission line inspection, Power Syst. Technol., 44 (2020), 1057–1069. http://doi.org/10.13335/j.1000-3673.pst.2019.0349 doi: 10.13335/j.1000-3673.pst.2019.0349
![]() |
[4] |
S. Khan, K. Muhammad, S. Mumtaz, S. W. Baik, V. H. C. Albuquerque, Energy-efficient deep CNN for smoke detection in foggy IoT environment, IEEE Internet Things J., 6 (2019), 9237–9245. http://doi.org/10.1109/JIOT.2019.2896120 doi: 10.1109/JIOT.2019.2896120
![]() |
[5] | H. Yin, Y. R. Wei, An improved algorithm based on convolutional neural network for smoke detection, in 2019 IEEE International Conferences on Ubiquitous Computing & Communications (IUCC) and Data Science and Computational Intelligence (DSCI) and Smart Computing, Networking and Services (SmartCNS), IEEE, (2019), 207–211. http://doi.org/10.1109/IUCC/DSCI/SmartCNS.2019.00063 |
[6] |
C. H. Li, B. Yang, H. Ding, H. L. Shi, X. P. Jiang, J. Sun, Real-time video-based smoke detection with high accuracy and efficiency, Fire Saf. J., 117 (2020), 103184. http://doi.org/10.1016/j.firesaf.2020.103184 doi: 10.1016/j.firesaf.2020.103184
![]() |
[7] |
M. H. Jiang, Y. X. Zhao, F. Yu, C. L. Zhou, T. Peng, A self-attention network for smoke detection, Fire Saf. J., 129 (2022), 103547. http://doi.org/10.1016/j.firesaf.2022.103547 doi: 10.1016/j.firesaf.2022.103547
![]() |
[8] |
Z. Q. Li, A. Khananian, R. H. Fraser, J. Cihlar, Automatic detection of fire smoke using artificial neural networks and threshold approaches applied to AVHRR imagery, IEEE Trans. Geosci. Remote Sens., 39 (2001), 1859–1870. http://doi.org/10.1109/36.951076 doi: 10.1109/36.951076
![]() |
[9] |
K. Muhammad, J. Ahmad, I. Mehmood, S. Rho, S. W. Baik, Convolutional neural networks based fire detection in surveillance videos, IEEE Access, 6 (2018), 18174–18183. http://10.1109/ACCESS.2018.2812835 doi: 10.1109/ACCESS.2018.2812835
![]() |
[10] | W. B. Cai, C. Y. Wang, H. Huang, T. Z. Wang, A real-time smoke detection model based on YOLO-smoke algorithm, in 2020 Cross Strait Radio Science & Wireless Technology Conference (CSRSWTC), IEEE, (2020), 1–3. http://10.1109/CSRSWTC50769.2020.9372453 |
[11] |
F. R. Zhou, G. Wen, Y. Ma, Y. F. Wang, Y. T. Ma, G. F. Wang, et al., Multilevel feature cooperative alignment and fusion for unsupervised domain adaptation smoke detection, Front. Phys., 11 (2023), 81. https://doi.org/10.3389/fphy.2023.1136021 doi: 10.3389/fphy.2023.1136021
![]() |
[12] |
S. G. Zhang, F. Zhang, Y. Ding, Y. Li, Swin-YOLOv5: Research and application of fire and smoke detection algorithm based on YOLOv5, Comput. Intell. Neurosci., 2022 (2022). https://doi.org/10.1155/2022/6081680 doi: 10.1155/2022/6081680
![]() |
[13] | C. Y. Wang, A. Bochkovskiy, H. Y. M. Liao, YOLOv7: Trainable bag-of-freebies sets new state-of-the-art for real-time object detectors, in Proceedings of the IEEE/CVF Conference on Computer Vision and Pattern Recognition, (2023), 7464–7475. https://doi.org/10.48550/arXiv.2207.02696 |
[14] |
Y. C. Zhou, L. H. Fang, X. Y. Zheng, X. L. Chen, Virtual battlefield smoke effect simulation based on particle system, Comput. Simul., 32 (2015), 417–420. https://doi.org/10.3969/j.issn.1006-9348.2015.07.093 doi: 10.3969/j.issn.1006-9348.2015.07.093
![]() |
[15] | A. Bochkovskiy, C. Y. Wang, H. Y. M. Liao, YOLOv4: Optimal speed and accuracy of object detection, preprint, arXiv: 2004.10934. https://doi.org/10.48550/arXiv.2004.10934 |
[16] | G. Jocher, A. Stoken, J. Borovec, L. Changyu, A. Hogan, L. Diaconu, et al., ultralytics/yolov5: v3. 0, Zenodo, 2020. Available from: https://ui.adsabs.harvard.edu/abs/2020zndo...3983579J/abstract. |
[17] | Y. Liu, X. Wang, Sar ship detection based on improved YOLOv7-tiny, in 2022 IEEE 8th International Conference on Computer and Communications (ICCC), IEEE, (2022), 2166–2170. https://doi.org/10.1109/ICCC56324.2022.10065775 |
[18] | T. Y. Lin, P. Dollár, R. Girshick, K. He, B. Hariharan, S. Belongie, Feature pyramid networks for object detection, in Proceedings of the IEEE Conference on Computer Vision and Pattern Recognition, (2017), 2117–2125. https://doi.org/10.1109/CVPR.2017.106 |
[19] | L. Yang, R. Y. Zhang, L. Li, X. Xie, Simam: A simple, parameter-free attention module for convolutional neural networks, in International Conference on Machine Learning, (2021), 11863–11874. |
[20] | R. Sunkara, T. Luo, No more strided convolutions or pooling: A new CNN building block for low-resolution images and small objects, in Machine Learning and Knowledge Discovery in Databases, Springer Nature, Cham, Switzerland, (2023), 443–459. https://doi.org/10.1007/978-3-031-26409-2_27 |
[21] |
Q. Tian, R. Hu, Z. Li, Y. Cai, Z. Yu, Insulator detection based on se-YOLOv5s, Chin. J. Intell. Sci. Technol., 3 (2021), 312–321. https://doi.org/10.11959/j.issn.2096-6652.202132 doi: 10.11959/j.issn.2096-6652.202132
![]() |
[22] |
B. S. Webb, N. T. Dhruv, S. G. Solomon, C. Tailby, P. Lennie, Early and late mechanisms of surround suppression in striate cortex of macaque, J. Neurosci., 25 (2005), 11666–11675. https://doi.org/10.1523/JNEUROSCI.3414-05.2005 doi: 10.1523/JNEUROSCI.3414-05.2005
![]() |
[23] | J. Hu, L. Shen, G. Sun, Squeeze-and-excitation networks, in Proceedings of the IEEE Conference on Computer Vision and Pattern Recognition, (2018), 7132–7141. https://doi.org/10.1109/TPAMI.2019.2913372 |
[24] | Q. L. Wang, B. G. Wu, P. F. Zhu, P. H. Li, W. M. Zuo, Q. H. Hu, ECA-Net: Efficient channel attention for deep convolutional neural networks, in Proceedings of the IEEE/CVF Conference on Computer Vision and Pattern Recognition, (2020), 11531–11539. https://doi.org/10.1109/CVPR42600.2020.01155 |
[25] | S. Woo, J. Park, J. Y. Lee, I. S. Kweon, CBAM: convolutional block attention module, in Proceedings of the European Conference on Computer Vision (ECCV), (2018), 3–19. https://doi.org/10.48550/arXiv.1807.06521 |
[26] |
A. Dewangan, Y. Pande, H. W. Braun, F. Vernon, I. Perez, I. Altintas, et al., Figlib & smokeynet: Dataset and deep learning model for real-time wildland fire smoke detection, Remote Sens., 14 (2022), 1007. https://doi.org/10.3390/rs14041007 doi: 10.3390/rs14041007
![]() |
[27] |
K. Govil, M. L. Welch, J. T. Ball, C. R. Pennypacker, Preliminary results from a wildfire detection system using deep learning on remote camera images, Remote Sens., 12 (2020), 166. https://doi.org/10.3390/rs12010166 doi: 10.3390/rs12010166
![]() |
1. | Khalil S. Al-Basyouni, Elsayed M. Elsayed, On Some Solvable Systems of Some Rational Difference Equations of Third Order, 2023, 11, 2227-7390, 1047, 10.3390/math11041047 | |
2. | Ibraheem M. Alsulami, E. M. Elsayed, On a class of nonlinear rational systems of difference equations, 2023, 8, 2473-6988, 15466, 10.3934/math.2023789 | |
3. | E.M. Elsayed, B.S. Alofi, The periodic nature and expression on solutions of some rational systems of difference equations, 2023, 74, 11100168, 269, 10.1016/j.aej.2023.05.026 | |
4. | Hashem Althagafi, Dynamics of difference systems: a mathematical study with applications to neural systems, 2025, 10, 2473-6988, 2869, 10.3934/math.2025134 |