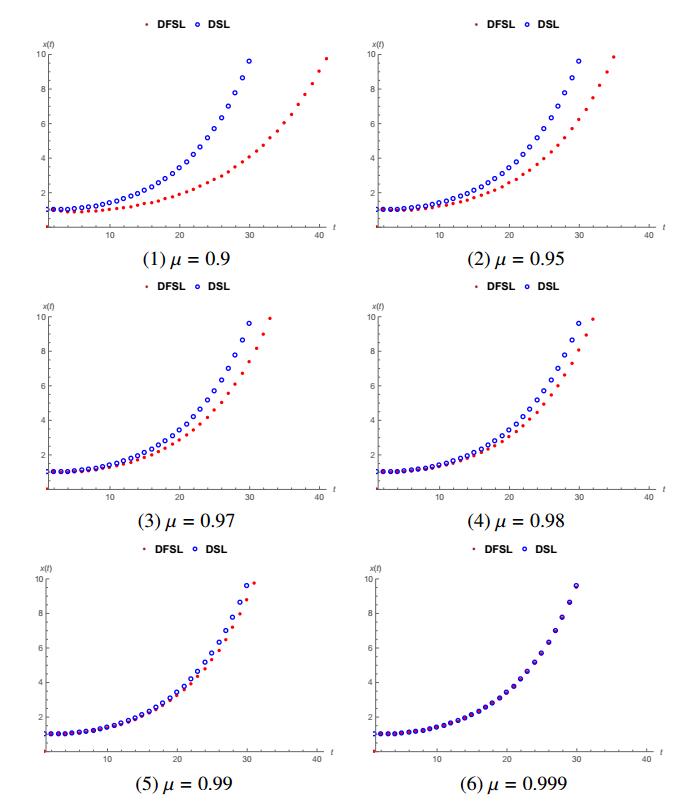
Entrepreneurial contexts may be marked by the presence of a 'cultural environment' that stimulates knowledge and innovation adoption, while other contexts may act as barriers toward change and innovation. Moreover, multiple paths of multifunctional agriculture bring about a call for "multifunctional farm advisory services" (MFAS), which consider both private and public goods provided by the farming sector. Set against the background of multiple roles of agriculture, how to identify sound and pertinent knowledge becomes of paramount, to specify the roles of agricultural extensionists and the mechanisms of governance of MFAS within the setting up of the Agricultural Knowledge and Innovation System (AKIS). Our aim of the study is either to analyze attitudes toward the privatization of extension services within a predominantly public system of regional governance and to identify advisors' profile and their suitability with the modern vision of multifunctional agriculture through the emergence of MFAS. Empirical analysis evidences the presence of a diversified set of advisory services with different degrees of coherence with the multifunctional agricultural model. Also, the more advisory services are oriented towards empowering multifunctional agriculture the less the propensity towards their privatization. The cluster analysis has demonstrated a relatively good advisor's capability to deal with the new demands of multifunctional agriculture. The idea of MFAS has important theoretical implications that the paper tries to excavate through the analysis of the mechanisms of governance (public/private) and the identification of the advisors' profile facing the growing complexity of the farming sector, grounded on multifunctional agriculture. The study tries to fill a gap in the literature, by providing an original contribution to modeling the profile of advisors in charge of supporting the transition towards multifunctionality.
Citation: Marcello De Rosa, Giuseppina Olivieri, Concetta Menna, Ferdinando Gandolfi, Teresa Del Giudice. Multifunctional farm advisory services in promoting change in agricultural systems: The case of Campania region of Italy[J]. AIMS Agriculture and Food, 2023, 8(4): 962-977. doi: 10.3934/agrfood.2023051
[1] | Yingying Xu, Chunhe Song, Chu Wang . Few-shot bearing fault detection based on multi-dimensional convolution and attention mechanism. Mathematical Biosciences and Engineering, 2024, 21(4): 4886-4907. doi: 10.3934/mbe.2024216 |
[2] | Fuhua Wang, Zongdong Zhang, Kai Wu, Dongxiang Jian, Qiang Chen, Chao Zhang, Yanling Dong, Xiaotong He, Lin Dong . Artificial intelligence techniques for ground fault line selection in power systems: State-of-the-art and research challenges. Mathematical Biosciences and Engineering, 2023, 20(8): 14518-14549. doi: 10.3934/mbe.2023650 |
[3] | Fang Luo, Yuan Cui, Xu Wang, Zhiliang Zhang, Yong Liao . Adaptive rotation attention network for accurate defect detection on magnetic tile surface. Mathematical Biosciences and Engineering, 2023, 20(9): 17554-17568. doi: 10.3934/mbe.2023779 |
[4] | Linchao Yang, Ying Liu, Guanglu Yang, Shi-Tong Peng . Dynamic monitoring and anomaly tracing of the quality in tobacco strip processing based on improved canonical variable analysis and transfer entropy. Mathematical Biosciences and Engineering, 2023, 20(8): 15309-15325. doi: 10.3934/mbe.2023684 |
[5] | Zhipeng Zhao, Zhenyu Hu, Zhiyu Zhao, Xiaoyu Du, Tianfei Chen, Lijun Sun . Fault-tolerant Hamiltonian cycle strategy for fast node fault diagnosis based on PMC in data center networks. Mathematical Biosciences and Engineering, 2024, 21(2): 2121-2136. doi: 10.3934/mbe.2024093 |
[6] | Giuseppe Ciaburro . Machine fault detection methods based on machine learning algorithms: A review. Mathematical Biosciences and Engineering, 2022, 19(11): 11453-11490. doi: 10.3934/mbe.2022534 |
[7] | Qiushi Wang, Zhicheng Sun, Yueming Zhu, Chunhe Song, Dong Li . Intelligent fault diagnosis algorithm of rolling bearing based on optimization algorithm fusion convolutional neural network. Mathematical Biosciences and Engineering, 2023, 20(11): 19963-19982. doi: 10.3934/mbe.2023884 |
[8] | Kwang Yoon Song, Youn Su Kim, In Hong Chang . Software reliability model for open-source software that considers the number of finite faults and dependent faults. Mathematical Biosciences and Engineering, 2023, 20(7): 11785-11804. doi: 10.3934/mbe.2023524 |
[9] | Xuyang Xie, Zichun Yang, Lei Zhang, Guoqing Zeng, Xuefeng Wang, Peng Zhang, Guobing Chen . An improved Autogram and MOMEDA method to detect weak compound fault in rolling bearings. Mathematical Biosciences and Engineering, 2022, 19(10): 10424-10444. doi: 10.3934/mbe.2022488 |
[10] | Jing Yang, Guo Xie, Yanxi Yang, Qijun Li, Cheng Yang . A multilevel recovery diagnosis model for rolling bearing faults from imbalanced and partially missing monitoring data. Mathematical Biosciences and Engineering, 2023, 20(3): 5223-5242. doi: 10.3934/mbe.2023242 |
Entrepreneurial contexts may be marked by the presence of a 'cultural environment' that stimulates knowledge and innovation adoption, while other contexts may act as barriers toward change and innovation. Moreover, multiple paths of multifunctional agriculture bring about a call for "multifunctional farm advisory services" (MFAS), which consider both private and public goods provided by the farming sector. Set against the background of multiple roles of agriculture, how to identify sound and pertinent knowledge becomes of paramount, to specify the roles of agricultural extensionists and the mechanisms of governance of MFAS within the setting up of the Agricultural Knowledge and Innovation System (AKIS). Our aim of the study is either to analyze attitudes toward the privatization of extension services within a predominantly public system of regional governance and to identify advisors' profile and their suitability with the modern vision of multifunctional agriculture through the emergence of MFAS. Empirical analysis evidences the presence of a diversified set of advisory services with different degrees of coherence with the multifunctional agricultural model. Also, the more advisory services are oriented towards empowering multifunctional agriculture the less the propensity towards their privatization. The cluster analysis has demonstrated a relatively good advisor's capability to deal with the new demands of multifunctional agriculture. The idea of MFAS has important theoretical implications that the paper tries to excavate through the analysis of the mechanisms of governance (public/private) and the identification of the advisors' profile facing the growing complexity of the farming sector, grounded on multifunctional agriculture. The study tries to fill a gap in the literature, by providing an original contribution to modeling the profile of advisors in charge of supporting the transition towards multifunctionality.
Fractional calculus is a notably attractive subject owing to having wide-ranging application areas of theoretical and applied sciences. Despite the fact that there are a large number of worthwhile mathematical works on the fractional differential calculus, there is no noteworthy parallel improvement of fractional difference calculus up to lately. This statement has shown that discrete fractional calculus has certain unforeseen hardship.
Fractional sums and differences were obtained firstly in Diaz-Osler [1], Miller-Ross [2] and Gray and Zhang [3] and they found discrete types of fractional integrals and derivatives. Later, several authors began to touch upon discrete fractional calculus; Goodrich-Peterson [4], Baleanu et al. [5], Ahrendt et al. [6]. Nevertheless, discrete fractional calculus is a rather novel area. The first studies have been done by Atıcı et al. [7,8,9,10,11], Abdeljawad et al. [12,13,14], Mozyrska et al. [15,16,17], Anastassiou [18,19], Hein et al. [20] and Cheng et al. [21] and so forth [22,23,24,25,26].
Self-adjoint operators have an important place in differential operators. Levitan and Sargsian [27] studied self-adjoint Sturm-Liouville differential operators and they obtained spectral properties based on self-adjointness. Also, they found representation of solutions and hence they obtained asymptotic formulas of eigenfunctions and eigenvalues. Similarly, Dehghan and Mingarelli [28,29] obtained for the first time representation of solution of fractional Sturm-Liouville problem and they obtained asymptotic formulas of eigenfunctions and eigenvalues of the problem. In this study, firstly we obtain self-adjointness of DFSL operator within nabla fractional Riemann-Liouville and delta fractional Grünwald-Letnikov operators. From this point of view, we obtain orthogonality of distinct eigenfunctions, reality of eigenvalues. In addition, we open a new gate by obtaining representation of solution of DFSL problem for researchers study in this area.
Self-adjointness of fractional Sturm-Liouville differential operators have been proven by Bas et al. [30,31], Klimek et al. [32,33]. Variational properties of fractional Sturm-Liouville problem has been studied in [34,35]. However, self-adjointness of conformable Sturm-Liouville and DFSL with Caputo-Fabrizio operator has been proven by [36,37]. Nowadays, several studies related to Atangana-Baleanu fractional derivative and its discrete version are done [38,39,40,41,42,43,44,45].
In this study, we consider DFSL operators within Riemann-Liouville and Grünwald-Letnikov sense, and we prove the self-adjointness, orthogonality of distinct eigenfunctions, reality of eigenvalues of DFSL operator. However, we get sum representation of solutions for DFSL equation by means Laplace transform for nabla fractional difference equations. Finally, we compare the results for the solution of DFSL problem, discrete Sturm-Liouville (DSL) problem with the second order, fractional Sturm-Liouville (FSL) problem and classical Sturm-Liouville (CSL) problem with the second order. The aim of this paper is to contribute to the theory of DFSL operator.
We discuss DFSL equations in three different ways with;
i) Self-adjoint (nabla left and right) Riemann-Liouville (R-L) fractional operator,
L1x(t)=∇μa(p(t)b∇μx(t))+q(t)x(t)=λr(t)x(t), 0<μ<1, |
ii) Self-adjoint (delta left and right) Grünwald-Letnikov (G-L) fractional operator,
L2x(t)=Δμ−(p(t)Δμ+x(t))+q(t)x(t)=λr(t)x(t), 0<μ<1, |
iii)(nabla left) DFSL operator is defined by R-L fractional operator,
L3x(t)=∇μa(∇μax(t))+q(t)x(t)=λx(t), 0<μ<1. |
Definition 2.1. [4] Delta and nabla difference operators are defined by respectively
Δx(t)=x(t+1)−x(t),∇x(t)=x(t)−x(t−1). | (1) |
Definition 2.2. [46] Falling function is defined by, α∈R
tα_=Γ(α+1)Γ(α+1−n), | (2) |
where Γ is Euler gamma function.
Definition 2.3. [46] Rising function is defined by, α∈R,
t¯α=Γ(t+α)Γ(t). | (3) |
Remark 1. Delta and nabla operators have the following properties
Δtα_=αtα−1_, | (4) |
∇t¯α=αt¯α−1. |
Definition 2.4. [2,7] Fractional sum operators are defined by,
(i) The left defined nabla fractional sum with order μ>0 is defined by
∇−μax(t)=1Γ(μ)t∑s=a+1(t−ρ(s))¯μ−1x(s), t∈Na+1, | (5) |
(ii) The right defined nabla fractional sum with order μ>0 is defined by
b∇−μx(t)=1Γ(μ)b−1∑s=t(s−ρ(t))¯μ−1x(s), t∈ b−1N, | (6) |
where ρ(t)=t−1 is called backward jump operators, Na={a,a+1,...}, bN={b,b−1,...}.
Definition 2.5. [47] Fractional difference operators are defined by,
(i) The nabla left fractional difference of order μ>0 is defined
∇μax(t)=∇n∇−(n−μ)ax(t)=∇nΓ(n−μ)t∑s=a+1(t−ρ(s))¯n−μ−1x(s), t∈Na+1, | (7) |
(ii) The nabla right fractional difference of order μ>0 is defined
b∇μx(t)=(−1)nΔn b∇−(n−μ)x(t)=(−1)nΔnΓ(n−μ)b−1∑s=t(s−ρ(t))¯n−μ−1x(s), t∈ b−1N. | (8) |
Fractional differences in (7−8) are called the Riemann-Liouville (R-L) definition of the μ-th order nabla fractional difference.
Definition 2.6. [1,21,48] Fractional difference operators are defined by,
(i) The left defined delta fractional difference of order μ, 0<μ≤1, is defined by
Δμ−x(t)=1hμt∑s=0(−1)sμ(μ−1)...(μ−s+1)s!x(t−s), t=1,...,N. | (9) |
(ii) The right defined delta fractional difference of order μ, 0<μ≤1, is defined by
Δμ+x(t)=1hμN−t∑s=0(−1)sμ(μ−1)...(μ−s+1)s!x(t+s), t=0,..,N−1. | (10) |
Fractional differences in (9−10) are called the Grünwald-Letnikov (G-L) definition of the μ-th order delta fractional difference.
Theorem 2.7. [47] We define the summation by parts formula for R-L fractional nabla difference operator, u is defined on bN and v is defined on Na, then
b−1∑s=a+1u(s)∇μav(s)=b−1∑s=a+1v(s)b∇μu(s). | (11) |
Theorem 2.8. [26,48] We define the summation by parts formula for G-L delta fractional difference operator, u, v is defined on {0,1,...,n}, then
n∑s=0u(s)Δμ−v(s)=n∑s=0v(s)Δμ+u(s). | (12) |
Definition 2.9. [20] f:Na→R, s∈ℜ, Laplace transform is defined as follows,
La{f}(s)=∞∑k=1(1−s)k−1f(a+k), |
where ℜ=C∖{1} and ℜ is called the set of regressive (complex) functions.
Definition 2.10. [20] Let f,g:Na→R, all t∈Na+1, convolution property of f and g is given by
(f∗g)(t)=t∑s=a+1f(t−ρ(s)+a)g(s), |
where ρ(s) is the backward jump function defined in [46] as
ρ(s)=s−1. |
Theorem 2.11. [20] f,g:Na→R, convolution theorem is expressed as follows,
La{f∗g}(s)=La{f}La{g}(s). |
Lemma 2.12. [20] f:Na→R, the following property is valid,
La+1{f}(s)=11−sLa{f}(s)−11−sf(a+1). |
Theorem 2.13. [20] f:Na→R, 0<μ<1, Laplace transform of nabla fractional difference
La+1{∇μaf}(s)=sμLa+1{f}(s)−1−sμ1−sf(a+1),t∈Na+1. |
Definition 2.14. [20] For |p|<1, α>0, β∈R and t∈Na, discrete Mittag-Leffler function is defined by
Ep,α,β(t,a)=∞∑k=0pk(t−a)¯αk+βΓ(αk+β+1), |
where t¯n={t(t+1)⋯(t+n−1),n∈ZΓ(t+n)Γ(t),n∈R is rising factorial function.
Theorem 2.15. [20] For |p|<1, α>0, β∈R, |1−s|<1, and |s|α>p, Laplace transform of discrete Mittag-Leffler function is as follows,
La{Ep,α,β(.,a)}(s)=sα−β−1sα−p. |
Definition 2.16. Laplace transform of f(t)∈R+, t≥0 is defined as follows,
L{f}(s)=∞∫0e−stf(t)dt. |
Theorem 2.17. For z, θ∈C,Re(δ)>0, Mittag-Leffler function with two parameters is defined as follows
Eδ,θ(z)=∞∑k=0zkΓ(δk+θ). |
Theorem 2.18. Laplace transform of Mittag-Leffler function is as follows
L{tθ−1Eδ,θ(λtδ)}(s)=sδ−θsδ−λ. |
Property 2.19. [28] f:Na→R, 0<μ<1, Laplace transform of fractional derivative in Caputo sense is as follows, 0<α<1,
L{CDα0+f}(s)=sαL{f}(s)−sα−1f(0). |
Property 2.20. [28] f:Na→R, 0<μ<1, Laplace transform of left fractional derivative in Riemann-Liouville sense is as follows, 0<α<1,
L{Dα0+f}(s)=sαL{f}(s)−I1−α0+f(t)|t=0, |
here Iα0+ is left fractional integral in Riemann-Liouville sense.
We consider discrete fractional Sturm-Liouville equations in three different ways as follows:
First Case: Self-adjoint L1 DFSL operator is defined by (nabla right and left) R-L fractional operator,
L1x(t)=∇μa(p(t)b∇μx(t))+q(t)x(t)=λr(t)x(t), 0<μ<1, | (13) |
where p(t)>0, r(t)>0, q(t) is a real valued function on [a+1,b−1] and real valued, λ is the spectral parameter, t∈[a+1,b−1], x(t)∈l2[a+1,b−1]. In ℓ2(a+1,b−1), the Hilbert space of sequences of complex numbers u(a+1),...,u(b−1) with the inner product is given by,
⟨u(n),v(n)⟩=b−1∑n=a+1u(n)v(n), |
for every u∈DL1, let's define as follows
DL1={u(n), v(n)∈ℓ2(a+1,b−1):L1u(n), L1v(n)∈ℓ2(a+1,b−1)}. |
Second Case: Self-adjoint L2 DFSL operator is defined by(delta left and right) G-L fractional operator,
L2x(t)=Δμ−(p(t)Δμ+x(t))+q(t)x(t)=λr(t)x(t), 0<μ<1, | (14) |
where p,r,λ is as defined above, q(t) is a real valued function on [0,n], t∈[0,n], x(t)∈l2[0,n]. In ℓ2(0,n), the Hilbert space of sequences of complex numbers u(0),...,u(n) with the inner product is given by, n is a finite integer,
⟨u(i),r(i)⟩=n∑i=0u(i)r(i), |
for every u∈DL2, let's define as follows
DL2={u(i), v(i)∈ℓ2(0,n):L2u(n), L2r(n)∈ℓ2(0,n)}. |
Third Case:L3 DFSL operator is defined by (nabla left) R-L fractional operator,
L3x(t)=∇μa(∇μax(t))+q(t)x(t)=λx(t), 0<μ<1, | (15) |
p,r,λ is as defined above, q(t) is a real valued function on [a+1,b−1], t∈[a+1,b−1].
Firstly, we consider the first case and give the following theorems and proofs;
Theorem 3.1. DFSL operator L1 is self-adjoint.
Proof.
u(t)L1v(t)=u(t)∇μa(p(t)b∇μv(t))+u(t)q(t)v(t), | (16) |
v(t)L1u(t)=v(t)∇μa(p(t)b∇μu(t))+v(t)q(t)u(t). | (17) |
If (16−17) is subtracted from each other
u(t)L1v(t)−v(t)L1u(t)=u(t)∇μa(p(t)b∇μv(t))−v(t)∇μa(p(t)b∇μu(t)) |
and sum operator from a+1 to b−1 to both side of the last equality is applied, we get
b−1∑s=a+1(u(s)L1v(s)−v(s)L1u(s))=b−1∑s=a+1u(s)∇μa(p(s)b∇μv(s)) | (18) |
−b−1∑s=a+1v(s)∇μa(p(s)b∇μu(s)). |
If we apply the summation by parts formula in (11) to right hand side of (18), we have
b−1∑s=a+1(u(s)L1v(s)−v(s)L1u(s))=b−1∑s=a+1p(s)b∇μv(s)b∇μu(s)−b−1∑s=a+1p(s)b∇μu(s)b∇μv(s)=0, |
⟨L1u,v⟩=⟨u,L1v⟩. |
Hence, the proof completes.
Theorem 3.2. Two eigenfunctions, u(t,λα) and v(t,λβ), of the equation (13) are orthogonal as λα≠λβ.
Proof. Let λα and λβ are two different eigenvalues corresponds to eigenfunctions u(t) and v(t) respectively for the the equation (13),
∇μa(p(t)b∇μu(t))+q(t)u(t)−λαr(t)u(t)=0,∇μa(p(t)b∇μv(t))+q(t)v(t)−λβr(t)v(t)=0. |
If we multiply last two equations by v(t) and u(t) respectively, subtract from each other and apply definite sum operator, owing to the self-adjointness of the operator L1, we have
(λα−λβ)b−1∑s=a+1r(s)u(s)v(s)=0, |
since λα≠λβ,
b−1∑s=a+1r(s)u(s)v(s)=0,⟨u(t),v(t)⟩=0. |
Hence, the proof completes.
Theorem 3.3. All eigenvalues of the equation (13) are real.
Proof. Let λ=α+iβ, owing to the self-adjointness of the operator L1, we can write
⟨L1u(t),u(t)⟩=⟨u(t),L1u(t)⟩,⟨λru(t),u(t)⟩=⟨u(t),λr(t)u(t)⟩, |
(λ−¯λ)⟨u(t),u(t)⟩r=0. |
Since ⟨u(t),u(t)⟩r≠0,
λ=¯λ |
and hence β=0. The proof completes.
Secondly, we consider the second case and give the following theorems and proofs;
Theorem 3.4. DFSL operator L2 is self-adjoint.
Proof.
u(t)L2v(t)=u(t)Δμ−(p(t)Δμ+v(t))+u(t)q(t)v(t), | (19) |
v(t)L2u(t)=v(t)Δμ−(p(t)Δμ+u(t))+v(t)q(t)u(t). | (20) |
If (19−20) is subtracted from each other
u(t)L2v(t)−v(t)L2u(t)=u(t)Δμ−(p(t)Δμ+v(t))−v(t)Δμ−(p(t)Δμ+u(t)) |
and definite sum operator from 0 to t to both side of the last equality is applied, we have
t∑s=0(u(s)L1v(s)−v(s)L2u(s))=t∑s=0u(s)Δμ−(p(s)Δμ+v(s))−t∑s=0v(s)Δμ−(p(s)Δμ+u(s)). | (21) |
If we apply the summation by parts formula in (12) to r.h.s. of (21), we get
t∑s=0(u(s)L2v(s)−v(s)L2u(s))=t∑s=0p(s)Δμ+v(s)Δμ+u(s)−t∑s=0p(s)Δμ+u(s)Δμ+v(s)=0, |
⟨L2u,v⟩=⟨u,L2v⟩. |
Hence, the proof completes.
Theorem 3.5. Two eigenfunctions, u(t,λα) and v(t,λβ), of the equation (14) are orthogonal as λα≠λβ. orthogonal.
Proof. Let λα and λβ are two different eigenvalues corresponds to eigenfunctions u(t) and v(t) respectively for the the equation (14),
Δμ−(p(t)Δμ+u(t))+q(t)u(t)−λαr(t)u(t)=0,Δμ−(p(t)Δμ+v(t))+q(t)v(t)−λβr(t)v(t)=0. |
If we multiply last two equations to v(t) and u(t) respectively, subtract from each other and apply definite sum operator, owing to the self-adjointness of the operator L2, we get
(λα−λβ)t∑s=0r(s)u(s)v(s)=0, |
since λα≠λβ,
t∑s=0r(s)u(s)v(s)=0⟨u(t),v(t)⟩=0. |
So, the eigenfunctions are orthogonal. The proof completes.
Theorem 3.6. All eigenvalues of the equation (14) are real.
Proof. Let λ=α+iβ, owing to the self-adjointness of the operator L2
⟨L2u(t),u(t)⟩=⟨u(t),L2u(t)⟩,⟨λr(t)u(t),u(t)⟩=⟨u(t),λr(t)u(t)⟩, |
(λ−¯λ)⟨u,u⟩r=0. |
Since ⟨u,u⟩r≠0,
λ=¯λ, |
and hence β=0. The proof completes.
Now, we consider the third case and give the following theorem and proof;
Theorem 3.7.
L3x(t)=∇μa(∇μax(t))+q(t)x(t)=λx(t),0<μ<1, | (22) |
x(a+1)=c1,∇μax(a+1)=c2, | (23) |
where p(t)>0, r(t)>0, q(t) is defined and real valued, λ is the spectral parameter. The sum representation of solution of the problem (22)−(23) is found as follows,
x(t)=c1[(1+q(a+1))Eλ,2μ,μ−1(t,a)−λEλ,2μ,2μ−1(t,a)] | (24) |
+c2[Eλ,2μ,2μ−1(t,a)−Eλ,2μ,μ−1(t,a)]−t∑s=a+1Eλ,2μ,2μ−1(t−ρ(s)+a)q(s)x(s), |
where |λ|<1, |1−s|<1, and |s|α>λ from Theorem 2.15.
Proof. Let's use the Laplace transform of both side of the equation (22) by Theorem 2.13, and let q(t)x(t)=g(t),
La+1{∇μa(∇μax)}(s)+La+1{g}(s)=λLa+1{x}(s),=sμLa+1{∇μax}(s)−1−sμ1−sc2=λLa+1{x}(s)−La+1{g}(s),=sμ(sμLa+1{x}(s)−1−sμ1−sc1)−1−sμ1−sc2=λLa+1{x}(s)−La+1{g}(s), |
=La+1{x}(s)=1−sμ1−s1s2μ−λ(sμc1+c2)−1s2μ−λLa+1{g}(s), |
from Lemma 2.12, we get
La{x}(s)=c1(sμ−λs2μ−λ)−1−ss2μ−λ(11−sLa{g}(s)−11−sg(a+1))+c2(1−sμs2μ−λ). | (25) |
Applying inverse Laplace transform to the equation (25), then we get representation of solution of the problem (22)−(23),
x(t)=c1((1+q(a+1))Eλ,2μ,μ−1(t,a)−λEλ,2μ,2μ−1(t,a))+c2(Eλ,2μ,2μ−1(t,a)−Eλ,2μ,μ−1(t,a))−t∑s=a+1Eλ,2μ,2μ−1(t−ρ(s)+a)q(s)x(s). |
Now, let us consider comparatively discrete fractional Sturm-Liouville (DFSL) problem, discrete Sturm-Liouville (DSL) problem, fractional Sturm-Liouville (FSL) problem and classical Sturm-Liouville (CSL) problem respectively as follows by taking q(t)=0,
DFSL problem:
∇μ0(∇μ0x(t))=λx(t), | (26) |
x(1)=1, ∇μax(1)=0, | (27) |
and its analytic solution is as follows by the help of Laplace transform in Lemma 2.12
x(t)=Eλ,2μ,μ−1(t,0)−λEλ,2μ,2μ−1(t,0), | (28) |
DSL problem:
∇2x(t)=λx(t), | (29) |
x(1)=1, ∇x(1)=0, | (30) |
and its analytic solution is as follows
x(t)=12(1−λ)−t[(1−√λ)t(1+√λ)−(−1+√λ)(1+√λ)t], | (31) |
FSL problem:
CDμ0+(Dμ0+x(t))=λx(t), | (32) |
I1−μ0+x(t)|t=0=1, Dμ0+x(t)|t=0=0, | (33) |
and its analytic solution is as follows by the help of Laplace transform in Property 2.19 and 2.20
x(t)=tμ−1E2μ,μ(λt2μ), | (34) |
CSL problem:
x′′(t)=λx(t), | (35) |
x(0)=1, x′(0)=0, | (36) |
and its analytic solution is as follows
x(t)=cosht√λ, | (37) |
where the domain and range of function x(t) and Mittag-Leffler functions must be well defined. Note that we may show the solution of CSL problem can be obtained by taking μ→1 in the solution of FSL problem and similarly, the solution of DSL problem can be obtained by taking μ→1 in the solution of DFSL problem.
Firstly, we compare the solutions of DFSL and DSL problems and from here we show that the solutions of DFSL problem converge to the solutions of DSL problem as μ→1 in Figure 1 for discrete Mittag-Leffler function Ep,α,β(t,a)=1000∑k=0pk(t−a)¯αk+βΓ(αk+β+1); let λ=0.01,
Secondly, we compare the solutions of DFSL, DSL, FSL and CSL problems for discrete Mittag-Leffler function Ep,α,β(t,a)=1000∑k=0pk(t−a)¯αk+βΓ(αk+β+1). At first view, we observe the solution of DSL and CSL problems almost coincide in any order μ, and we observe the solutions of DFSL and FSL problem almost coincide in any order μ. However, we observe that all of the solutions of DFSL, DSL, FSL and CSL problems almost coincide to each other as μ→1 in Figure 2. Let λ=0.01,
Thirdly, we compare the solutions of DFSL problem (22−23) with different orders, different potential functions and different eigenvalues for discrete Mittag-Leffler function Ep,α,β(t,a)=1000∑k=0pk(t−a)¯αk+βΓ(αk+β+1) in the Figure 3;
Eigenvalues of DFSL problem (22−23), correspond to some specific eigenfunctions for numerical values of discrete Mittag-Leffler function Ep,α,β(t,a)=i∑k=0pk(t−a)¯αk+βΓ(αk+β+1), is given with different orders while q(t)=0 in Table 1;
i | λ1,i | λ2,i | λ3,i | λ1,i | λ2,i | λ3,i | λ1,i | λ2,i | λ3,i |
750 | −0.992 | −0.982 | −0.057 | −0.986 | −0.941 | −0.027 | −0.483 | −0.483 | 0 |
1000 | −0.989 | −0.977 | −0.057 | −0.990 | −0.954 | −0.027 | −0.559 | −0.435 | 0 |
2000 | −0.996 | −0.990 | −0.057 | −0.995 | −0.978 | −0.027 | −0.654 | −0.435 | 0 |
x(5),μ=0.5 | x(10),μ=0.9 | x(2000),μ=0.1 | |||||||
i | λ1,i | λ2,i | λ3,i | λ1,i | λ2,i | λ3,i | λ1,i | λ2,i | λ3,i |
750 | −0.951 | −0.004 | 0 | −0.868 | −0.793 | −0.0003 | −0.190 | −3.290×10−6 | 0 |
1000 | −0.963 | −0.004 | 0 | −0.898 | −0.828 | −0.0003 | −0.394 | −3.290×10−6 | 0 |
2000 | −0.981 | −0.004 | 0 | −0.947 | −0.828 | −0.0003 | −0.548 | −3.290×10−6 | 0 |
x(20),μ=0.5 | x(100),μ=0.9 | x(1000),μ=0.7 | |||||||
i | λ1,i | λ2,i | λ3,i | λ1,i | λ2,i | λ3,i | λ1,i | λ2,i | λ3,i |
750 | −0.414 | −9.59×10−7 | 0 | −0.853 | −0.0003 | 0 | −0.330 | −4.140×10−6 | 0 |
1000 | −0.478 | −9.59×10−7 | 0 | −0.887 | −0.0003 | 0 | −0.375 | −4.140×10−6 | 0 |
2000 | −0.544 | −9.59×10−7 | 0 | −0.940 | −0.0003 | 0 | −0.361 | −4.140×10−6 | 0 |
x(1000),μ=0.3 | x(100),μ=0.8 | x(1000),μ=0.9 | |||||||
i | λ1,i | λ2,i | λ3,i | λ1,i | λ2,i | λ3,i | λ1,i | λ2,i | λ3,i |
750 | −0.303 | −3.894×10−6 | 0 | −0.192 | −0.066 | 0 | −0.985 | −0.955 | −0.026 |
1000 | −0.335 | −3.894×10−6 | 0 | −0.197 | −0.066 | 0 | −0.989 | −0.941 | −0.026 |
2000 | −0.399 | −3.894×10−6 | 0 | −0.289 | −0.066 | 0 | −0.994 | −0.918 | −0.026 |
x(1000),μ=0.8 | x(2000),μ=0.6 | x(10),μ=0.83 |
Finally, we give the solutions of DFSL problem (22−23) with different orders, different potential functions and different eigenvalues for discrete Mittag-Leffler function Ep,α,β(t,a)=100∑k=0pk(t−a)¯αk+βΓ(αk+β+1) in Tables 2–4;
x(t) | μ=0.1 | μ=0.2 | μ=0.5 | μ=0.7 | μ=0.9 |
x(1) | 1 | 1 | 1 | 1 | 1 |
x(2) | 0.125 | 0.25 | 0.625 | 0.875 | 1.125 |
x(3) | 0.075 | 0.174 | 0.624 | 1.050 | 1.575 |
x(5) | 0.045 | 0.128 | 0.830 | 1.968 | 4.000 |
x(7) | 0.0336 | 0.111 | 1.228 | 4.079 | 11.203 |
x(9) | 0.0274 | 0.103 | 1.878 | 8.657 | 31.941 |
x(12) | 0.022 | 0.098 | 3.622 | 27.05 | 154.56 |
x(15) | 0.0187 | 0.0962 | 7.045 | 84.75 | 748.56 |
x(16) | 0.0178 | 0.0961 | 8.800 | 124.04 | 1266.5 |
x(18) | 0.0164 | 0.0964 | 13.737 | 265.70 | 3625.6 |
x(20) | 0.0152 | 0.0972 | 21.455 | 569.16 | 10378.8 |
x(t) | q(t)=1 | q(t)=t | q(t)=√t |
x(1) | 1 | 1 | 1 |
x(2) | 0.2261 | 0.1505 | 0.1871 |
x(3) | 0.1138 | 0.0481 | 0.0767 |
x(5) | 0.0518 | 0.0110 | 0.0252 |
x(7) | 0.0318 | 0.0043 | 0.0123 |
x(9) | 0.0223 | 0.0021 | 0.0072 |
x(12) | 0.0150 | 0.0010 | 0.0039 |
x(15) | 0.0110 | 0.0005 | 0.0025 |
x(16) | 0.0101 | 0.0004 | 0.0022 |
x(18) | 0.0086 | 0.0003 | 0.0017 |
x(20) | 0.0075 | 0.0002 | 0.0014 |
x(t) | q(t)=1 | q(t)=t | q(t)=√t |
x(1) | 1 | 1 | 1 |
x(2) | 0.2261 | 0.1505 | 0.1871 |
x(3) | 0.1138 | 0.0481 | 0.0767 |
x(5) | 0.0518 | 0.0110 | 0.0252 |
x(7) | 0.0318 | 0.0043 | 0.0123 |
x(9) | 0.0223 | 0.0021 | 0.0072 |
x(12) | 0.0150 | 0.0010 | 0.0039 |
x(15) | 0.0110 | 0.0005 | 0.0025 |
x(16) | 0.0101 | 0.0004 | 0.0022 |
x(18) | 0.0086 | 0.0003 | 0.0017 |
x(20) | 0.0075 | 0.0002 | 0.0014 |
Now, let's consider the problems together DFSL (26)−(27), DSL (29)−(30), FSL (32)−(33) and CSL (35)−(36). Eigenvalues of these problems are the roots of the following equation
x(35)=0. |
Thus, if we apply the solutions (28), (31), (34) and (37) of these four problems to the equation above respectively, we can find the eigenvalues of these problems for the orders μ=0.9 and μ=0.99 respectively in Table 5, and Table 6,
λ1 | λ2 | λ3 | λ4 | λ5 | λ6 | λ7 | λ8 | λ9 | λ10 | |
DFSL | −0.904 | −0.859 | −0.811 | −0.262 | −0.157 | −0.079 | −0.029 | −0.003 | 0.982 | |
FSL | −0.497 | −0.383 | −0.283 | −0.196 | −0.124 | −0.066 | −0.026 | −0.003 | 0 | ... |
DSL | −1.450 | −0.689 | −0.469 | −0.310 | −0.194 | −0.112 | −0.055 | −0.019 | −0.002 | |
CSL | −0.163 | −0.128 | −0.098 | −0.072 | −0.050 | −0.032 | −0.008 | −0.002 | 0 |
λ1 | λ2 | λ3 | λ4 | λ5 | λ6 | λ7 | λ8 | λ9 | λ10 | |
DFSL | −0.866 | −0.813 | −0.200 | −0.115 | −0.057 | −0.020 | −0.002 | 0 | 0.982 | |
FSL | −0.456 | −0.343 | −0.246 | −0.165 | −0.100 | −0.051 | −0.018 | −0.002 | 0 | ... |
DSL | −1.450 | −0.689 | −0.469 | −0.310 | −0.194 | −0.112 | −0.055 | −0.019 | −0.002 | ... |
CSL | −0.163 | −0.128 | −0.098 | −0.072 | −0.050 | −0.032 | −0.008 | −0.002 | 0 |
In here, we observe that these four problems have real eigenvalues under different orders μ=0.9 and μ=0.99, hence we can find eigenfunctions putting these eigenvalues into the four solutions. Furthermore, as the order changes, we can see that eigenvalues change for DFSL problems.
We consider firstly discrete fractional Sturm-Liouville (DFSL) operators with nabla Riemann-Liouville and delta Grünwald-Letnikov fractional operators and we prove self-adjointness of the DFSL operator and fundamental spectral properties. However, we analyze DFSL problem, discrete Sturm-Liouville (DSL) problem, fractional Sturm-Liouville (FSL) problem and classical Sturm-Liouville (CSL) problem by taking q(t)=0 in applications. Firstly, we compare the solutions of DFSL and DSL problems and we observe that the solutions of DFSL problem converge to the solutions of DSL problem when μ→1 in Fig. 1. Secondly, we compare the solutions of DFSL, DSL, FSL and CSL problems in Fig. 2. At first view, we observe the solutions of DSL and CSL problems almost coincide with any order μ, and we observe the solutions of DFSL and FSL problem almost coincide with any order μ. However, we observe that all of solutions of DFSL, DSL, FSL and CSL problems almost coincide with each other as μ→1. Thirdly, we compare the solutions of DFSL problem (22−23) with different orders, different potential functions and different eigenvalues in Fig. 3.
Eigenvalues of DFSL problem (22−23) corresponded to some specific eigenfunctions is given with different orders in Table 1. We give the eigenfunctions of DFSL problem (22−23) with different orders, different potential functions and different eigenvalues in Table 2, Table 3 and Table 4.
In Section 4.1, we consider DFSL, DSL, FSL and CSL problems together and thus, we can compare the eigenvalues of these four problems in Table 5 and Table 6 for different values of μ. We observe that these four problems have real eigenvalues under different values of μ, from here we can find eigenfunctions corresponding eigenvalues. Moreover, when the order change, eigenvalues change for DFSL problems.
Consequently, important results in spectral theory are given for discrete Sturm-Liouville problems. These results will lead to open gates for the researchers studied in this area. Especially, representation of solution will be practicable for future studies. It worths noting that visual results both will enable to be understood clearly by readers and verify the results to the integer order discrete case while the order approaches to one.
This paper includes a part of Ph.D. thesis data of Ramazan OZARSLAN.
The authors declare no conflict of interest.
[1] |
Van Huylenbroeck G, Vandermeulen V, Mettepenningen E, et al. (2007) Multifunctionality of agriculture: A review of definitions, evidence and instruments. Living Rev Landsc Res 1: 1–43. https://doi.org/10.12942/lrlr-2007-3 doi: 10.12942/lrlr-2007-3
![]() |
[2] | Rogers E M (1983) Diffusion of innovations. 3rd Ed., New York: Free Press, 15. |
[3] |
Hermans F, Klerkx L, Roep D (2015) Structural conditions for collaboration and learning in innovation networks: Using an innovation system performance lens to analyze agricultural knowledge systems. J Agric Educ Ext 21: 35–54. https://doi.org/10.1080/1389224X.2014.991113 doi: 10.1080/1389224X.2014.991113
![]() |
[4] |
Lioutas ED, Charatsari C, Černič Istenič M, et al. (2019) The challenges of setting up the evaluation of extension systems by using a systems approach: the case of Greece, Italy and Slovenia. J Agric Educ Ext 25: 139–160. https://doi.org/10.1080/1389224X.2019.1583818 doi: 10.1080/1389224X.2019.1583818
![]() |
[5] | Klerkx L, Van Mierlo B, Leeuwis C (2012) Evolution of systems approaches to agricultural innovation: Concepts, analysis, and interventions, In: Darnhofer I, Gibbon D, Dedieu B (Eds.), Farming Systems Research into the 21st Century: The New Dynamic, Springer Dordrecht, 457–483. https://doi.org/10.1007/978-94-007-4503-2_20 |
[6] |
Klerkx L, Leeuwis C (2008) Balancing multiple interests: Embedding innovation intermediation in the agricultural knowledge infrastructure. Technovation 28: 364–378. https://doi.org/10.1016/j.technovation.2007.05.005 doi: 10.1016/j.technovation.2007.05.005
![]() |
[7] | EU SCAR (2012) Agricultural knowledge, and innovation systems in transition—a reflection paper, Brussels, p.13. Available from: https://ec.europa.eu/eip/agriculture/en/publications/agricultural-knowledge-and-innovation-systems.html. |
[8] | EU SCAR AKIS (2019) Preparing for Future AKIS in Europe. |
[9] |
Klerkx L (2020) Advisory services and transformation, plurality and disruption of agriculture and food systems: Towards a new research agenda for agricultural education and extension studies. J Agric Educ Ext 26: 131–140. https://doi.org/10.1080/1389224X.2020.1738046 doi: 10.1080/1389224X.2020.1738046
![]() |
[10] |
Welter F (2011) Contextualizing entrepreneurship—conceptual challenges and ways forward. Entrep Theory Pract 35: 165–184. https://doi.org/10.1111/j.1540-6520.2010.00427.x doi: 10.1111/j.1540-6520.2010.00427.x
![]() |
[11] | McElwee G, Smith R (2014) Chapter 14 Researching rural enterprise. In: Fayolle A (Ed.), Handbook of Research On Entrepreneurship: What We Know and What We Need to Know, Edward Elgar Publishing, 307. https://doi.org/10.4337/9780857936929.00022 |
[12] |
Labarthe P, Laurent C (2013) Privatization of agricultural extension services in the EU: Towards a lack of adequate knowledge for small-scale farms? Food Policy 38: 240–252. https://doi.org/10.1016/j.foodpol.2012.10.005 doi: 10.1016/j.foodpol.2012.10.005
![]() |
[13] | Davis K (2019) Agricultural Extension and Education for the Future, Closing keynote in 24th European Seminar on Extension and Education. Available from: https://www.google.com/url?sa = t & rct = j & q = & esrc = s & source = web & cd = & ved = 2ahUKEwiRjPnbrJuBAxW3xwIHHVGeA1gQFnoECBAQAQ & url = https%3A%2F%2Fwww.reterurale.it%2Fflex%2Fcm%2Fpages%2FServeAttachment.php%2FL%2FIT%2FD%2F2%25252Fb%25252F2%25252FD.b2f20be86583c28041cc%2FP%2FBLOB%253AID%253D19744%2FE%2Fpdf & usg = AOvVaw0tuNdggviVs-Zu1iIVxlLW & opi = 89978449. |
[14] | EU Commission (2012) The future of food and farming. |
[15] | ASHBY J (2009) Fostering farmer first methodological innovation: Organizational learning and change in international agricultural research. Farmer First Revisited: Innovation Agric Res Dev 2009: 39–45. |
[16] |
Sutherland LA, Madureira L, Dirimanova V, et al. (2017) New knowledge networks of small-scale farmers in Europe's periphery. Land Use Policy 63: 428–439. https://doi.org/10.1016/j.landusepol.2017.01.028 doi: 10.1016/j.landusepol.2017.01.028
![]() |
[17] | EU Commission (2009) Report from the Commission to the European Parliament and the Council on the Application of the Farm Advisory System as Defined in Article 12 and 13 of Council Regulation (EC) No 73/2009. Available from: ttps: //eurlex.europa.eu/LexUriServ/LexUriServ.do?uri = OJ: L: 2009: 030: 0016: 0099: en: PDF. |
[18] |
Knierim A, Labarthe P, Laurent C, et al. (2017) Pluralism of agricultural advisory service providers—Facts and insights from Europe. J Rural Stud 55: 45–58. https://doi.org/10.1016/j.jrurstud.2017.07.018 doi: 10.1016/j.jrurstud.2017.07.018
![]() |
[19] |
Vecchio Y, De Castro P, Masi M, et al. (2021) Do rural development policies really help small farms? A reflection from Italy. EuroChoices 20: 75–80. https://doi.org/10.1111/1746-692X.12338 doi: 10.1111/1746-692X.12338
![]() |
[20] |
van der Ploeg JD, Barjolle D, Bruil J, et al. (2019) The economic potential of agroecology: Empirical evidence from Europe. J Rural Stud 71: 46–61. https://doi.org/10.1016/j.jrurstud.2019.09.003 doi: 10.1016/j.jrurstud.2019.09.003
![]() |
[21] |
Birner R, Davis K, Pender J, et al. (2009) From best practice to best fit: A framework for designing and analyzing pluralistic agricultural advisory services worldwide. J Agric Educ Ext 15: 341–355. https://doi.org/10.1080/13892240903309595 doi: 10.1080/13892240903309595
![]() |
[22] |
Klerkx L, Petter Stræ te E, Kvam G T, et al. (2017) Achieving best-fit configurations through advisory subsystems in AKIS: case studies of advisory service provisioning for diverse types of farmers in Norway. J Agric Educ Ext 23: 213–229. https://doi.org/10.1080/1389224X.2017.1320640 doi: 10.1080/1389224X.2017.1320640
![]() |
[23] |
Marsden T, Sonnino R (2012) Human health and wellbeing and the sustainability of urban-regional food systems. Curr Opin Environ Sustain 4: 427–430. https://doi.org/10.1016/j.cosust.2012.09.004 doi: 10.1016/j.cosust.2012.09.004
![]() |
[24] |
Landini F, Brites W, Mathot y Rebolé M I (2017) Towards a new paradigm for rural extensionists' in-service training. J Rural Stud 51: 158–167. https://doi.org/10.1016/j.jrurstud.2017.02.010 doi: 10.1016/j.jrurstud.2017.02.010
![]() |
[25] | OECD (2000) Multifunctionality. Towards an Analytical Framework. |
[26] |
Wilson G (2008) From 'weak' to 'strong' multifunctionality: Conceptualizing farm-level multifunctional transitional pathways. J Rural Stud 24: 367–383. https://doi.org/10.1016/j.jrurstud.2007.12.010 doi: 10.1016/j.jrurstud.2007.12.010
![]() |
[27] | Poppe K (2014) The role of the European innovation partnership in linking innovation and research in agricultural knowledge and innovation systems. Agriregionieuropa 10: 37. |
[28] |
Foti R, Nyakudya I, Moyo M, et al. (2007) Determinants of farmer demand for 'fee-for-service' extension in Zimbabwe: The case of Mashonaland Central province. J Agric Educ Ext 14: 95–104. https://doi.org/10.5191/jiaee.2007.14108 doi: 10.5191/jiaee.2007.14108
![]() |
[29] | Vanni F (2014) Agriculture and Public Goods: The Role of Collective Action. https://doi.org/10.1007/978-94-007-7457-5 |
[30] |
Acheson JM (2006) Institutional failure in resource management. Annu Rev Anthropol 35: 117–134. https://doi.org/10.1146/annurev.anthro.35.081705.123238 doi: 10.1146/annurev.anthro.35.081705.123238
![]() |
[31] |
Andrew B (2008) Market failure, government failure and externalities in climate change mitigation: The case for a carbon tax. Public Adm Dev 28: 393–401. https://doi.org/10.1002/pad.517 doi: 10.1002/pad.517
![]() |
[32] | Stiglitz J (2009) Government failure vs. market failure: Principles of regulation. In: Balleisen E, Moss D (Eds.), Government and Markets: Toward a New Theory of Regulation, Cambridge: Cambridge University Press, 13–51. https://doi.org/10.1017/CBO9780511657504.002 |
[33] |
Randall A (2002) Valuing the outputs of multifunctional agriculture. Eur Rev Agric Econ 29: 289–307. https://doi.org/10.1093/eurrag/29.3.289 doi: 10.1093/eurrag/29.3.289
![]() |
[34] |
Eastwood C, Klerkx L, Nettle R (2017) Dynamics and distribution of public and private research and extension roles for technological innovation and diffusion: Case studies of the implementation and adaptation of precision farming technologies. J Rural Stud 49: 1–12. https://doi.org/10.1016/j.jrurstud.2016.11.008 doi: 10.1016/j.jrurstud.2016.11.008
![]() |
[35] |
Faure G, Desjeux Y, Gasselin P (2012) New challenges in agricultural advisory services from a research perspective: A literature review, synthesis and research agenda. J Agric Educ Ext 18: 461–492. https://doi.org/10.1080/1389224X.2012.707063 doi: 10.1080/1389224X.2012.707063
![]() |
[36] |
Vargo SL, Lusch RF (2017) Service-dominant logic 2025. Int J Res Mark 34: 46–67. https://doi.org/10.1016/j.ijresmar.2016.11.001 doi: 10.1016/j.ijresmar.2016.11.001
![]() |
[37] |
Prager K, Labarthe P, Caggiano M, et al. (2016) How does commercialization impact on the provision of farm advisory services? Evidence from Belgium, Italy, Ireland and the UK. Land Use Policy 52: 329–344. https://doi.org/10.1016/j.landusepol.2015.12.024 doi: 10.1016/j.landusepol.2015.12.024
![]() |
[38] |
Ward JH (1963) Hierarchical grouping to optimize an objective function. J Am Stat Assoc 58: 236–244. https://doi.org/10.1080/01621459.1963.10500845 doi: 10.1080/01621459.1963.10500845
![]() |
[39] |
Pigford AAE, Hickey GM, Klerkx L (2018) Beyond agricultural innovation systems? Exploring an agricultural innovation ecosystems approach for niche design and development in sustainability transitions. Agric Syst 164: 116–121. https://doi.org/10.1016/j.agsy.2018.04.007 doi: 10.1016/j.agsy.2018.04.007
![]() |
[40] | Van der Ploeg JD, Marsden T (2008) Unfolding webs: The dynamics of regional rural development van Gorcum, Assen. |
[41] |
Renting H, Rossing WAH, Groot JCJ, et al. (2009) Exploring multifunctional agriculture. A review of conceptual approaches and prospects for an integrative transitional framework. J Environ Manage 90: S112–S123. https://doi.org/10.1016/j.jenvman.2008.11.014 doi: 10.1016/j.jenvman.2008.11.014
![]() |
1. | Yanbo Li, Qing He, Damin Zhang, Dual graph convolutional networks integrating affective knowledge and position information for aspect sentiment triplet extraction, 2023, 17, 1662-5218, 10.3389/fnbot.2023.1193011 | |
2. | Fang Huang, Hongqiang Sang, Fen Liu, Rui Han, Dimensional optimisation and an inverse kinematic solution method of a safety‐enhanced remote centre of motion manipulator, 2024, 20, 1478-5951, 10.1002/rcs.2579 | |
3. | Yaqin Zhao, Yuqing Song, Longwen Wu, Puqiu Liu, Ruchen Lv, Hikmat Ullah, Lightweight micro-motion gesture recognition based on MIMO millimeter wave radar using Bidirectional-GRU network, 2023, 35, 0941-0643, 23537, 10.1007/s00521-023-08978-z | |
4. | Ameerah Abdulwahhab Flaifel, Abbas Fadel Mohammed, Fatima kadhem Abd, Mahmood H. Enad, Ahmad H. Sabry, Early detection of arc faults in DC microgrids using wavelet-based feature extraction and deep learning, 2024, 18, 1863-2386, 195, 10.1007/s11761-024-00420-z | |
5. | Yu Cao, Yongyi Chen, Dan Zhang, Mohammed Abdulaal, ALTASN: A few-shot learning fault diagnosis method for rotating machinery of unmanned surface vehicles based on attention mechanism, 2024, 0142-3312, 10.1177/01423312241239380 | |
6. | Raz Halaly, Elishai Ezra Tsur, Autonomous driving controllers with neuromorphic spiking neural networks, 2023, 17, 1662-5218, 10.3389/fnbot.2023.1234962 | |
7. | Shakra Mehak, John D. Kelleher, Michael Guilfoyle, Maria Chiara Leva, Action Recognition for Human–Robot Teaming: Exploring Mutual Performance Monitoring Possibilities, 2024, 12, 2075-1702, 45, 10.3390/machines12010045 | |
8. | Meichao Xing, Qiaoling Du, Zhenlong Bi, Research on DEM geomorphic factor terrain recognition algorithm using probabilistic neural networks based on tactile systems, 2024, 46, 0142-3312, 2174, 10.1177/01423312241231489 | |
9. | He Xu, Bin Lv, Jie Chen, Lei Kou, Hailin Liu, Min Liu, Research on a Prediction Model of Water Quality Parameters in a Marine Ranch Based on LSTM-BP, 2023, 15, 2073-4441, 2760, 10.3390/w15152760 | |
10. | Won‐Kyu Choi, Se‐Han Kim, Ji‐Hoon Bae, Series‐arc‐fault diagnosis using feature fusion‐based deep learning model, 2024, 1225-6463, 10.4218/etrij.2023-0457 | |
11. | Bo Guo, Huaming Liu, Lei Niu, Safe physical interaction with cobots: a multi-modal fusion approach for health monitoring, 2023, 17, 1662-5218, 10.3389/fnbot.2023.1265936 | |
12. | Yekang Zhao, Wangchen Dai, Shiren Wang, Liang Xi, Shenqing Wang, Feng Zhang, A Review of Cuckoo Filters for Privacy Protection and Their Applications, 2023, 12, 2079-9292, 2809, 10.3390/electronics12132809 | |
13. | Fang Zhang, Hongjuan Wang, Lukun Wang, Yue Wang, Dual-task attention-guided character image generation method, 2023, 45, 10641246, 4725, 10.3233/JIFS-231289 | |
14. | Chih-Chien Hu, Exploring the Impact of CPS-Based Robot-Assisted Teaching in STEM Education: Enhancing Knowledge, Skills, and Attitudes, 2024, 40, 1044-7318, 7193, 10.1080/10447318.2023.2262278 | |
15. | Xiaoxing Su, Engineering fault intelligent monitoring system based on Internet of Things and GIS, 2023, 12, 2192-8029, 10.1515/nleng-2022-0322 | |
16. | Suqi Xue, Farong Gao, Xudong Wu, Qun Xu, Xuecheng Weng, Qizhong Zhang, MUNIX repeatability evaluation method based on FastICA demixing, 2023, 20, 1551-0018, 16362, 10.3934/mbe.2023730 | |
17. | Ling Chen, Tianxin Zhang, Jing Chen, 2023, Embroidery Robotics: An Innovative Approach to AI-based Online Learning and Image Generation, 979-8-3503-0732-0, 496, 10.1109/WRCSARA60131.2023.10261779 | |
18. | Chunpeng Tian, Mengyao Song, Beibei Niu, Jingqing Li, Pu Sun, 2023, Enhancing Arc Fault Detection in DC Series Circuits Using a Sample Entropy and High-Order Cumulants-Based System, 979-8-3503-2936-0, 365, 10.1109/ICCSN57992.2023.10297396 | |
19. | Fengpei Yuan, Robert Bray, Michael Oliver, Joshua Duzan, Monica Crane, Xiaopeng Zhao, A Social Robot-facilitated Performance Assessment of Self-care Skills for People with Alzheimer’s: A Preliminary Study, 2024, 1875-4791, 10.1007/s12369-024-01174-6 | |
20. | Sergey Nesteruk, Svetlana Illarionova, Ilya Zherebzov, Claire Traweek, Nadezhda Mikhailova, Andrey Somov, Ivan Oseledets, PseudoAugment: Enabling Smart Checkout Adoption for New Classes Without Human Annotation, 2023, 11, 2169-3536, 76869, 10.1109/ACCESS.2023.3296854 | |
21. | Kotcharat Kitchat, Meng-Hong Lin, Hao-Sheng Chen, Min-Te Sun, Kazuya Sakai, Wei-Shinn Ku, Thattapon Surasak, A deep reinforcement learning system for the allocation of epidemic prevention materials based on DDPG, 2024, 242, 09574174, 122763, 10.1016/j.eswa.2023.122763 | |
22. | Mengyuan Chen, Hangrong Guo, Runbang Qian, Guangqiang Gong, Hao Cheng, Visual simultaneous localization and mapping (vSLAM) algorithm based on improved Vision Transformer semantic segmentation in dynamic scenes, 2024, 15, 2191-916X, 1, 10.5194/ms-15-1-2024 | |
23. | Meizhen Deng, Yimeng Liu, Ling Chen, AI-driven innovation in ethnic clothing design: an intersection of machine learning and cultural heritage, 2023, 31, 2688-1594, 5793, 10.3934/era.2023295 | |
24. | Xuecheng Weng, Chang Mei, Farong Gao, Xudong Wu, Qizhong Zhang, Guangyu Liu, A gait stability evaluation method based on wearable acceleration sensors, 2023, 20, 1551-0018, 20002, 10.3934/mbe.2023886 | |
25. | S. Rubin Bose, V. Sathiesh Kumar, C. Sreekar, In-situ enhanced anchor-free deep CNN framework for a high-speed human-machine interaction, 2023, 126, 09521976, 106980, 10.1016/j.engappai.2023.106980 | |
26. | Haoming Zhao, Xinling Zhang, Design of nonlinear control system for motion trajectory of industrial handling robot, 2024, 6, 2578-0727, 10.1002/adc2.165 | |
27. | Fuhua Wang, Zongdong Zhang, Kai Wu, Dongxiang Jian, Qiang Chen, Chao Zhang, Yanling Dong, Xiaotong He, Lin Dong, Artificial intelligence techniques for ground fault line selection in power systems: State-of-the-art and research challenges, 2023, 20, 1551-0018, 14518, 10.3934/mbe.2023650 | |
28. | Linlin Yu, Xiaojun Tang, Ruihua Si, Yan Xie, Peng Jia, Lixin Li, Qidi Huo, Shaoxuan Zhu, Multi‐objective optimization method for control parameters of flexible direct current transmission converters based on intelligent algorithms, 2024, 18, 1751-8687, 921, 10.1049/gtd2.13038 | |
29. | Yang Yang, Xing Liu, Zhengxiong Liu, Panfeng Huang, Learner engagement regulation of dual-user training based on deep reinforcement learning, 2024, 42, 0263-5747, 179, 10.1017/S0263574723001418 | |
30. | Yueliang Wu, Aolong Yi, Chengcheng Ma, Ling Chen, Artificial intelligence for video game visualization, advancements, benefits and challenges, 2023, 20, 1551-0018, 15345, 10.3934/mbe.2023686 | |
31. | Ying Shi, Keqi Mei, Adaptive nonsingular terminal sliding mode controller for PMSM drive system using modified extended state observer, 2023, 20, 1551-0018, 18774, 10.3934/mbe.2023832 | |
32. | Song Liu, Hui Chai, Rui Song, Yibin Li, Yueyang Li, Qin Zhang, Peng Fu, Jianli Liu, Zhiyuan Yang, Contact Force/Motion Hybrid Control for a Hydraulic Legged Mobile Manipulator via a Force-Controlled Floating Base, 2024, 29, 1083-4435, 2316, 10.1109/TMECH.2023.3323541 | |
33. | Feilong Wang, Furong Chen, Xiaolong Yang, Qi Yong, Yanling Dong, Xinming Zhang, Long Zheng, Hang Su, 2023, Integrating Sensor Fusion for Teleoperation Control of Anthropomorphic Dual-Arm Robots*, 979-8-3503-0732-0, 145, 10.1109/WRCSARA60131.2023.10261850 | |
34. | Yi Deng, Zhiguo Wang, Lin Dong, Yu Lei, Yanling Dong, Immersive innovations: an examination of the efficacy and evolution of virtual reality in human movement training, 2023, 43, 2754-6969, 551, 10.1108/RIA-05-2023-0072 | |
35. | Zhong Wang, Lanfang Lei, Peibei Shi, Smoking behavior detection algorithm based on YOLOv8-MNC, 2023, 17, 1662-5188, 10.3389/fncom.2023.1243779 | |
36. | Sandra Hellmers, Elias Krey, Arber Gashi, Jessica Koschate, Laura Schmidt, Tim Stuckenschneider, Andreas Hein, Tania Zieschang, Comparison of machine learning approaches for near-fall-detection with motion sensors, 2023, 5, 2673-253X, 10.3389/fdgth.2023.1223845 | |
37. | Furong Chen, Feilong Wang, Xiaolong Yang, Qi Yong, Yanling Dong, Yi Gao, Long Zheng, Hang Su, 2023, Sensor Fusion Based Humanoid Robotic arm Control*, 979-8-3503-0732-0, 151, 10.1109/WRCSARA60131.2023.10261786 | |
38. | Kun Zhu, Longfei Zhao, Application of conjugated materials in sports training, 2023, 11, 2296-2646, 10.3389/fchem.2023.1275448 | |
39. | Yuanhong Li, Jiapeng Liao, Jing Wang, Yangfan Luo, Yubin Lan, Prototype Network for Predicting Occluded Picking Position Based on Lychee Phenotypic Features, 2023, 13, 2073-4395, 2435, 10.3390/agronomy13092435 | |
40. | Joe Louca, John Vrublevskis, Kerstin Eder, Antonia Tzemanaki, Elicitation of trustworthiness requirements for highly dexterous teleoperation systems with signal latency, 2023, 17, 1662-5218, 10.3389/fnbot.2023.1187264 | |
41. | Ashwin Jayakumar, Javier Bermejo-García, Daniel Rodríguez Jorge, Rafael Agujetas, Francisco Romero-Sánchez, Francisco J. Alonso-Sánchez, Design, Control, and Assessment of a Synergy-Based Exosuit for Patients with Gait-Associated Pathologies, 2023, 12, 2076-0825, 309, 10.3390/act12080309 | |
42. | Yan-Jiang Zhao, Ji-Long Lu, Yong-De Zhang, He Zhang, Yan-Yi Pei, Structural Design and Puncture Control of Insertion Robot for FBG Embedded Cannula Flexible Needle, 2023, 11, 2169-3536, 79295, 10.1109/ACCESS.2023.3298949 | |
43. | Haopeng Zhou, Zhenlin Li, Tong Wu, Changxi Wang, Kang Li, Prognostic and Health Management of CT Equipment via a Distance Self-Attention Network Using Internet of Things, 2024, 11, 2327-4662, 31338, 10.1109/JIOT.2024.3421365 | |
44. | Xiaohui Li, Dongfang Fan, Yi Deng, Yu Lei, Owen Omalley, Sensor fusion-based virtual reality for enhanced physical training, 2024, 44, 2754-6969, 48, 10.1108/RIA-08-2023-0103 | |
45. | Kaiqiang Tang, Huiqiao Fu, Guizhou Deng, Xinpeng Wang, Chunlin Chen, Hierarchical Multicontact Motion Planning of Hexapod Robots With Incremental Reinforcement Learning, 2024, 16, 2379-8920, 1327, 10.1109/TCDS.2023.3345539 | |
46. | Yongyong Zhao, Jinghua Wang, Guohua Cao, Yi Yuan, Xu Yao, Luqiang Qi, Intelligent Control of Multilegged Robot Smooth Motion: A Review, 2023, 11, 2169-3536, 86645, 10.1109/ACCESS.2023.3304992 | |
47. | Jia Li, Yu-qian Zhou, Qiu-yan Zhang, Metric networks for enhanced perception of non-local semantic information, 2023, 17, 1662-5218, 10.3389/fnbot.2023.1234129 | |
48. | Di Zhang, Chen Chen, Fa Tan, Beibei Qian, Wei Li, Xuan He, Susan Lei, Multi-view and multi-scale behavior recognition algorithm based on attention mechanism, 2023, 17, 1662-5218, 10.3389/fnbot.2023.1276208 | |
49. | Zhongming Xiao, Xinzhu Lu, Jun Ning, Dapei Liu, COLREGs-compliant unmanned surface vehicles collision avoidance based on improved differential evolution algorithm, 2024, 237, 09574174, 121499, 10.1016/j.eswa.2023.121499 | |
50. | Wei Chen, Yi Han, Jie Zhao, Chong Chen, Bin Zhang, Ziran Wu, Zhenquan Lin, Lightweight Arc Fault Detection Method Based on Adam-Optimized Neural Network and Hardware Feature Algorithm, 2024, 17, 1996-1073, 1412, 10.3390/en17061412 | |
51. | Yu Lei, Yi Deng, Lin Dong, Xiaohui Li, Xiangnan Li, Zhi Su, A Novel Sensor Fusion Approach for Precise Hand Tracking in Virtual Reality-Based Human—Computer Interaction, 2023, 8, 2313-7673, 326, 10.3390/biomimetics8030326 | |
52. | Yan Wang, Wei Wang, Yueri Cai, Qiming Zhao, Yuyang Wang, Preoperative Planning Framework for Robot-Assisted Dental Implant Surgery: Finite-Parameter Surrogate Model and Optimization of Instrument Placement, 2023, 10, 2306-5354, 952, 10.3390/bioengineering10080952 | |
53. | Jie Zhang, Shuxia Wang, Weiping He, Jianghong Li, Shixin Wu, Zhiwei Cao, Manxian Wang, Perception and Decision-Making for Multi‑Modal Interaction Based on Fuzzy Theory in the Dynamic Environment, 2023, 1044-7318, 1, 10.1080/10447318.2023.2291607 | |
54. | Leena Jenefa, Dr.Karuppasamy Ramanathan, Namita Mishra, Vishnu Vishnu, M. Jayakumar, S. Ashok, 2024, chapter 6, 9798369322840, 127, 10.4018/979-8-3693-2284-0.ch006 | |
55. | Yanyan Lv, Jialin Shao, Gailing Bai, Preparation and application of graphene oxide hybrid polyaniline based flexible electrode materials, 2024, 618, 0015-0193, 1996, 10.1080/00150193.2024.2322371 | |
56. | 鲁普东 Lu Pudong, 吴超 Wu Chao, 王顺利 Wang Shunli, 基于超宽禁带半导体镓氧氮的深紫外光电探测器及其电弧检测应用, 2025, 52, 0258-7025, 0103002, 10.3788/CJL241061 | |
57. | Chan-Woong Park, Yun-Sung Kim, Woo-Cheol Lee, Study on the Detection of Serial Arcs in AC System with 120 Hz Components of Received Signal Strength Indicator (RSSI), 2025, 1975-0102, 10.1007/s42835-025-02153-1 | |
58. | Shangze Chen, Kamal Chandra Paul, Tiefu Zhao, 2024, Enhancing Arc Fault Detection Performance through Data Augmentation with Artificial Intelligence Technology: An Approach to Time Series Dataset Enlargement, 979-8-3503-7606-7, 1866, 10.1109/ECCE55643.2024.10861637 | |
59. | Reji G., Sapavat Jagan, Selvajyothi K., 2024, Series Arc Fault Detection in DC Networks and Electric Vehicles, 979-8-3503-7392-9, 1, 10.1109/ISGTAsia61245.2024.10876231 | |
60. | Yufei Mao, Sadaf Safa, Gianni Smith, Leon Wurth, Roland Weiss, Jens Hagemeyer, Why AI: A Comparative Study for Detection Methods in DC Series Arc Fault, 2025, 13, 2169-3536, 42703, 10.1109/ACCESS.2025.3548309 | |
61. | Jimin Li, Xihao Zhang, Lu Pei, Zhaoming Wu, Yaping Du, 2025, Chapter 59, 978-981-96-4674-6, 584, 10.1007/978-981-96-4675-3_59 | |
62. | Zhaoming Wu, Chuanzhen Jia, Fengshu Ye, Junjie Tan, Zhaohe Huang, Qingsha S. Cheng, 2025, Chapter 66, 978-981-96-4674-6, 643, 10.1007/978-981-96-4675-3_66 |
i | λ1,i | λ2,i | λ3,i | λ1,i | λ2,i | λ3,i | λ1,i | λ2,i | λ3,i |
750 | −0.992 | −0.982 | −0.057 | −0.986 | −0.941 | −0.027 | −0.483 | −0.483 | 0 |
1000 | −0.989 | −0.977 | −0.057 | −0.990 | −0.954 | −0.027 | −0.559 | −0.435 | 0 |
2000 | −0.996 | −0.990 | −0.057 | −0.995 | −0.978 | −0.027 | −0.654 | −0.435 | 0 |
x(5),μ=0.5 | x(10),μ=0.9 | x(2000),μ=0.1 | |||||||
i | λ1,i | λ2,i | λ3,i | λ1,i | λ2,i | λ3,i | λ1,i | λ2,i | λ3,i |
750 | −0.951 | −0.004 | 0 | −0.868 | −0.793 | −0.0003 | −0.190 | −3.290×10−6 | 0 |
1000 | −0.963 | −0.004 | 0 | −0.898 | −0.828 | −0.0003 | −0.394 | −3.290×10−6 | 0 |
2000 | −0.981 | −0.004 | 0 | −0.947 | −0.828 | −0.0003 | −0.548 | −3.290×10−6 | 0 |
x(20),μ=0.5 | x(100),μ=0.9 | x(1000),μ=0.7 | |||||||
i | λ1,i | λ2,i | λ3,i | λ1,i | λ2,i | λ3,i | λ1,i | λ2,i | λ3,i |
750 | −0.414 | −9.59×10−7 | 0 | −0.853 | −0.0003 | 0 | −0.330 | −4.140×10−6 | 0 |
1000 | −0.478 | −9.59×10−7 | 0 | −0.887 | −0.0003 | 0 | −0.375 | −4.140×10−6 | 0 |
2000 | −0.544 | −9.59×10−7 | 0 | −0.940 | −0.0003 | 0 | −0.361 | −4.140×10−6 | 0 |
x(1000),μ=0.3 | x(100),μ=0.8 | x(1000),μ=0.9 | |||||||
i | λ1,i | λ2,i | λ3,i | λ1,i | λ2,i | λ3,i | λ1,i | λ2,i | λ3,i |
750 | −0.303 | −3.894×10−6 | 0 | −0.192 | −0.066 | 0 | −0.985 | −0.955 | −0.026 |
1000 | −0.335 | −3.894×10−6 | 0 | −0.197 | −0.066 | 0 | −0.989 | −0.941 | −0.026 |
2000 | −0.399 | −3.894×10−6 | 0 | −0.289 | −0.066 | 0 | −0.994 | −0.918 | −0.026 |
x(1000),μ=0.8 | x(2000),μ=0.6 | x(10),μ=0.83 |
x(t) | μ=0.1 | μ=0.2 | μ=0.5 | μ=0.7 | μ=0.9 |
x(1) | 1 | 1 | 1 | 1 | 1 |
x(2) | 0.125 | 0.25 | 0.625 | 0.875 | 1.125 |
x(3) | 0.075 | 0.174 | 0.624 | 1.050 | 1.575 |
x(5) | 0.045 | 0.128 | 0.830 | 1.968 | 4.000 |
x(7) | 0.0336 | 0.111 | 1.228 | 4.079 | 11.203 |
x(9) | 0.0274 | 0.103 | 1.878 | 8.657 | 31.941 |
x(12) | 0.022 | 0.098 | 3.622 | 27.05 | 154.56 |
x(15) | 0.0187 | 0.0962 | 7.045 | 84.75 | 748.56 |
x(16) | 0.0178 | 0.0961 | 8.800 | 124.04 | 1266.5 |
x(18) | 0.0164 | 0.0964 | 13.737 | 265.70 | 3625.6 |
x(20) | 0.0152 | 0.0972 | 21.455 | 569.16 | 10378.8 |
x(t) | q(t)=1 | q(t)=t | q(t)=√t |
x(1) | 1 | 1 | 1 |
x(2) | 0.2261 | 0.1505 | 0.1871 |
x(3) | 0.1138 | 0.0481 | 0.0767 |
x(5) | 0.0518 | 0.0110 | 0.0252 |
x(7) | 0.0318 | 0.0043 | 0.0123 |
x(9) | 0.0223 | 0.0021 | 0.0072 |
x(12) | 0.0150 | 0.0010 | 0.0039 |
x(15) | 0.0110 | 0.0005 | 0.0025 |
x(16) | 0.0101 | 0.0004 | 0.0022 |
x(18) | 0.0086 | 0.0003 | 0.0017 |
x(20) | 0.0075 | 0.0002 | 0.0014 |
x(t) | q(t)=1 | q(t)=t | q(t)=√t |
x(1) | 1 | 1 | 1 |
x(2) | 0.2261 | 0.1505 | 0.1871 |
x(3) | 0.1138 | 0.0481 | 0.0767 |
x(5) | 0.0518 | 0.0110 | 0.0252 |
x(7) | 0.0318 | 0.0043 | 0.0123 |
x(9) | 0.0223 | 0.0021 | 0.0072 |
x(12) | 0.0150 | 0.0010 | 0.0039 |
x(15) | 0.0110 | 0.0005 | 0.0025 |
x(16) | 0.0101 | 0.0004 | 0.0022 |
x(18) | 0.0086 | 0.0003 | 0.0017 |
x(20) | 0.0075 | 0.0002 | 0.0014 |
λ1 | λ2 | λ3 | λ4 | λ5 | λ6 | λ7 | λ8 | λ9 | λ10 | |
DFSL | −0.904 | −0.859 | −0.811 | −0.262 | −0.157 | −0.079 | −0.029 | −0.003 | 0.982 | |
FSL | −0.497 | −0.383 | −0.283 | −0.196 | −0.124 | −0.066 | −0.026 | −0.003 | 0 | ... |
DSL | −1.450 | −0.689 | −0.469 | −0.310 | −0.194 | −0.112 | −0.055 | −0.019 | −0.002 | |
CSL | −0.163 | −0.128 | −0.098 | −0.072 | −0.050 | −0.032 | −0.008 | −0.002 | 0 |
λ1 | λ2 | λ3 | λ4 | λ5 | λ6 | λ7 | λ8 | λ9 | λ10 | |
DFSL | −0.866 | −0.813 | −0.200 | −0.115 | −0.057 | −0.020 | −0.002 | 0 | 0.982 | |
FSL | −0.456 | −0.343 | −0.246 | −0.165 | −0.100 | −0.051 | −0.018 | −0.002 | 0 | ... |
DSL | −1.450 | −0.689 | −0.469 | −0.310 | −0.194 | −0.112 | −0.055 | −0.019 | −0.002 | ... |
CSL | −0.163 | −0.128 | −0.098 | −0.072 | −0.050 | −0.032 | −0.008 | −0.002 | 0 |
i | λ1,i | λ2,i | λ3,i | λ1,i | λ2,i | λ3,i | λ1,i | λ2,i | λ3,i |
750 | −0.992 | −0.982 | −0.057 | −0.986 | −0.941 | −0.027 | −0.483 | −0.483 | 0 |
1000 | −0.989 | −0.977 | −0.057 | −0.990 | −0.954 | −0.027 | −0.559 | −0.435 | 0 |
2000 | −0.996 | −0.990 | −0.057 | −0.995 | −0.978 | −0.027 | −0.654 | −0.435 | 0 |
x(5),μ=0.5 | x(10),μ=0.9 | x(2000),μ=0.1 | |||||||
i | λ1,i | λ2,i | λ3,i | λ1,i | λ2,i | λ3,i | λ1,i | λ2,i | λ3,i |
750 | −0.951 | −0.004 | 0 | −0.868 | −0.793 | −0.0003 | −0.190 | −3.290×10−6 | 0 |
1000 | −0.963 | −0.004 | 0 | −0.898 | −0.828 | −0.0003 | −0.394 | −3.290×10−6 | 0 |
2000 | −0.981 | −0.004 | 0 | −0.947 | −0.828 | −0.0003 | −0.548 | −3.290×10−6 | 0 |
x(20),μ=0.5 | x(100),μ=0.9 | x(1000),μ=0.7 | |||||||
i | λ1,i | λ2,i | λ3,i | λ1,i | λ2,i | λ3,i | λ1,i | λ2,i | λ3,i |
750 | −0.414 | −9.59×10−7 | 0 | −0.853 | −0.0003 | 0 | −0.330 | −4.140×10−6 | 0 |
1000 | −0.478 | −9.59×10−7 | 0 | −0.887 | −0.0003 | 0 | −0.375 | −4.140×10−6 | 0 |
2000 | −0.544 | −9.59×10−7 | 0 | −0.940 | −0.0003 | 0 | −0.361 | −4.140×10−6 | 0 |
x(1000),μ=0.3 | x(100),μ=0.8 | x(1000),μ=0.9 | |||||||
i | λ1,i | λ2,i | λ3,i | λ1,i | λ2,i | λ3,i | λ1,i | λ2,i | λ3,i |
750 | −0.303 | −3.894×10−6 | 0 | −0.192 | −0.066 | 0 | −0.985 | −0.955 | −0.026 |
1000 | −0.335 | −3.894×10−6 | 0 | −0.197 | −0.066 | 0 | −0.989 | −0.941 | −0.026 |
2000 | −0.399 | −3.894×10−6 | 0 | −0.289 | −0.066 | 0 | −0.994 | −0.918 | −0.026 |
x(1000),μ=0.8 | x(2000),μ=0.6 | x(10),μ=0.83 |
x(t) | μ=0.1 | μ=0.2 | μ=0.5 | μ=0.7 | μ=0.9 |
x(1) | 1 | 1 | 1 | 1 | 1 |
x(2) | 0.125 | 0.25 | 0.625 | 0.875 | 1.125 |
x(3) | 0.075 | 0.174 | 0.624 | 1.050 | 1.575 |
x(5) | 0.045 | 0.128 | 0.830 | 1.968 | 4.000 |
x(7) | 0.0336 | 0.111 | 1.228 | 4.079 | 11.203 |
x(9) | 0.0274 | 0.103 | 1.878 | 8.657 | 31.941 |
x(12) | 0.022 | 0.098 | 3.622 | 27.05 | 154.56 |
x(15) | 0.0187 | 0.0962 | 7.045 | 84.75 | 748.56 |
x(16) | 0.0178 | 0.0961 | 8.800 | 124.04 | 1266.5 |
x(18) | 0.0164 | 0.0964 | 13.737 | 265.70 | 3625.6 |
x(20) | 0.0152 | 0.0972 | 21.455 | 569.16 | 10378.8 |
x(t) | q(t)=1 | q(t)=t | q(t)=√t |
x(1) | 1 | 1 | 1 |
x(2) | 0.2261 | 0.1505 | 0.1871 |
x(3) | 0.1138 | 0.0481 | 0.0767 |
x(5) | 0.0518 | 0.0110 | 0.0252 |
x(7) | 0.0318 | 0.0043 | 0.0123 |
x(9) | 0.0223 | 0.0021 | 0.0072 |
x(12) | 0.0150 | 0.0010 | 0.0039 |
x(15) | 0.0110 | 0.0005 | 0.0025 |
x(16) | 0.0101 | 0.0004 | 0.0022 |
x(18) | 0.0086 | 0.0003 | 0.0017 |
x(20) | 0.0075 | 0.0002 | 0.0014 |
x(t) | q(t)=1 | q(t)=t | q(t)=√t |
x(1) | 1 | 1 | 1 |
x(2) | 0.2261 | 0.1505 | 0.1871 |
x(3) | 0.1138 | 0.0481 | 0.0767 |
x(5) | 0.0518 | 0.0110 | 0.0252 |
x(7) | 0.0318 | 0.0043 | 0.0123 |
x(9) | 0.0223 | 0.0021 | 0.0072 |
x(12) | 0.0150 | 0.0010 | 0.0039 |
x(15) | 0.0110 | 0.0005 | 0.0025 |
x(16) | 0.0101 | 0.0004 | 0.0022 |
x(18) | 0.0086 | 0.0003 | 0.0017 |
x(20) | 0.0075 | 0.0002 | 0.0014 |
λ1 | λ2 | λ3 | λ4 | λ5 | λ6 | λ7 | λ8 | λ9 | λ10 | |
DFSL | −0.904 | −0.859 | −0.811 | −0.262 | −0.157 | −0.079 | −0.029 | −0.003 | 0.982 | |
FSL | −0.497 | −0.383 | −0.283 | −0.196 | −0.124 | −0.066 | −0.026 | −0.003 | 0 | ... |
DSL | −1.450 | −0.689 | −0.469 | −0.310 | −0.194 | −0.112 | −0.055 | −0.019 | −0.002 | |
CSL | −0.163 | −0.128 | −0.098 | −0.072 | −0.050 | −0.032 | −0.008 | −0.002 | 0 |
λ1 | λ2 | λ3 | λ4 | λ5 | λ6 | λ7 | λ8 | λ9 | λ10 | |
DFSL | −0.866 | −0.813 | −0.200 | −0.115 | −0.057 | −0.020 | −0.002 | 0 | 0.982 | |
FSL | −0.456 | −0.343 | −0.246 | −0.165 | −0.100 | −0.051 | −0.018 | −0.002 | 0 | ... |
DSL | −1.450 | −0.689 | −0.469 | −0.310 | −0.194 | −0.112 | −0.055 | −0.019 | −0.002 | ... |
CSL | −0.163 | −0.128 | −0.098 | −0.072 | −0.050 | −0.032 | −0.008 | −0.002 | 0 |