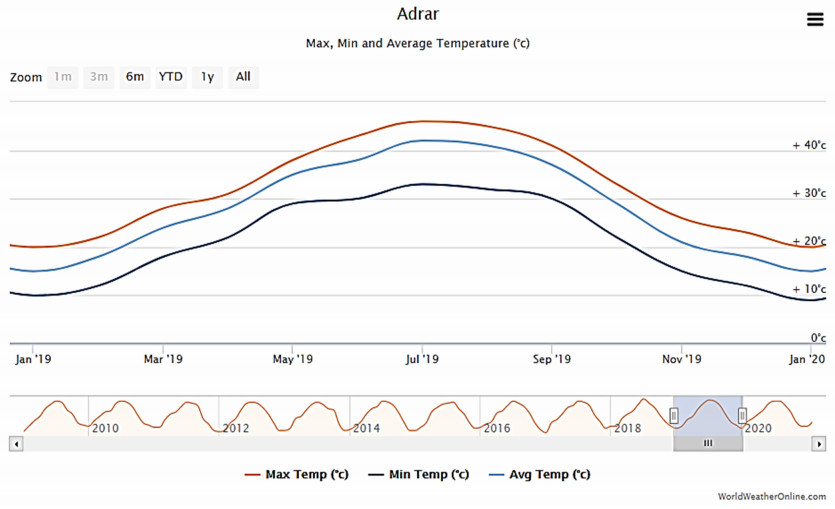
In modern power systems, efficient ground fault line selection is crucial for maintaining stability and reliability within distribution networks, especially given the increasing demand for energy and integration of renewable energy sources. This systematic review aims to examine various artificial intelligence (AI) techniques employed in ground fault line selection, encompassing artificial neural networks, support vector machines, decision trees, fuzzy logic, genetic algorithms, and other emerging methods. This review separately discusses the application, strengths, limitations, and successful case studies of each technique, providing valuable insights for researchers and professionals in the field. Furthermore, this review investigates challenges faced by current AI approaches, such as data collection, algorithm performance, and real-time requirements. Lastly, the review highlights future trends and potential avenues for further research in the field, focusing on the promising potential of deep learning, big data analytics, and edge computing to further improve ground fault line selection in distribution networks, ultimately enhancing their overall efficiency, resilience, and adaptability to evolving demands.
Citation: Fuhua Wang, Zongdong Zhang, Kai Wu, Dongxiang Jian, Qiang Chen, Chao Zhang, Yanling Dong, Xiaotong He, Lin Dong. Artificial intelligence techniques for ground fault line selection in power systems: State-of-the-art and research challenges[J]. Mathematical Biosciences and Engineering, 2023, 20(8): 14518-14549. doi: 10.3934/mbe.2023650
[1] | Kong Fah Tee, Sayedali Mostofizadeh . Numerical and experimental investigation of concrete with various dosages of fly ash. AIMS Materials Science, 2021, 8(4): 587-607. doi: 10.3934/matersci.2021036 |
[2] | Ketut Aswatama Wiswamitra, Sri Murni Dewi, Moch. Agus Choiron, Ari Wibowo . Heat resistance of lightweight concrete with plastic aggregate from PET (polyethylene terephthalate)-mineral filler. AIMS Materials Science, 2021, 8(1): 99-118. doi: 10.3934/matersci.2021007 |
[3] | Abdul Saboor Karzad, Moussa Leblouba, Zaid A. Al-Sadoon, Mohamed Maalej, Salah Altoubat . Modeling the flexural strength of steel fibre reinforced concrete. AIMS Materials Science, 2023, 10(1): 86-111. doi: 10.3934/matersci.2023006 |
[4] | G. Sri Harsha, P. Poluraju, Veerendrakumar C. Khed . Computation of shear strength equation for shear deformation of reinforced concrete deep beams using finite element method. AIMS Materials Science, 2021, 8(1): 42-61. doi: 10.3934/matersci.2021004 |
[5] | Rein Terje Thorstensen . Preventing early age chloride migration into low-carbon concrete. AIMS Materials Science, 2019, 6(6): 1020-1032. doi: 10.3934/matersci.2019.6.1020 |
[6] | Ashhad Imam, Shashank Mishra, Yeetendra Kumar Bind . Review study towards corrosion mechanism and its impact on the durability of concrete structures. AIMS Materials Science, 2018, 5(2): 276-300. doi: 10.3934/matersci.2018.2.276 |
[7] | Marcílio M. A. Filho, Paulo A. G. Piloto, Carlos Balsa . The load-bearing of composite slabs with steel deck under natural fires. AIMS Materials Science, 2022, 9(1): 150-171. doi: 10.3934/matersci.2022010 |
[8] | Ayumu Yasue, Keita Hayashi, Shogo Yamamoto, Toshitsugu Inukai, Shigeru Fujimori . Influence of concrete bleeding due to mix proportion on the drilling speed of hardened surface layer. AIMS Materials Science, 2021, 8(3): 486-500. doi: 10.3934/matersci.2021030 |
[9] | Masanori Kikuchi . Study on multiple surface crack growth and coalescence behaviors. AIMS Materials Science, 2016, 3(4): 1623-1631. doi: 10.3934/matersci.2016.4.1623 |
[10] | Elisa Padovano, Francesco Trevisan, Sara Biamino, Claudio Badini . Processing of hybrid laminates integrating ZrB2/SiC and SiC layers. AIMS Materials Science, 2020, 7(5): 552-564. doi: 10.3934/matersci.2020.5.552 |
In modern power systems, efficient ground fault line selection is crucial for maintaining stability and reliability within distribution networks, especially given the increasing demand for energy and integration of renewable energy sources. This systematic review aims to examine various artificial intelligence (AI) techniques employed in ground fault line selection, encompassing artificial neural networks, support vector machines, decision trees, fuzzy logic, genetic algorithms, and other emerging methods. This review separately discusses the application, strengths, limitations, and successful case studies of each technique, providing valuable insights for researchers and professionals in the field. Furthermore, this review investigates challenges faced by current AI approaches, such as data collection, algorithm performance, and real-time requirements. Lastly, the review highlights future trends and potential avenues for further research in the field, focusing on the promising potential of deep learning, big data analytics, and edge computing to further improve ground fault line selection in distribution networks, ultimately enhancing their overall efficiency, resilience, and adaptability to evolving demands.
In an arid climate, and hot weather, and very low relative humidity, significant additional stresses and strains can occur in the elements of a concrete structure due to shrinkage and temperature variation. Concrete cracking is expected to occur when these stresses' combined effects exceed the concrete's tensile strength. Moreover, the presence of cracks is not only undesirable from the aesthetic point of view; but more significantly. Moreover, it could adversely affect the durability of concrete members and their structural integrity in some situations.
The region of Adrar, located in southern Algeria, is an arid area where the concrete construction system has already replaced the old clay-based adobe construction system to improve structures' mechanical strength. However, worrying problems, such as cracking in most constructions, even in recent ones, were observed.
Researchers have provided different perspectives on classifying the early-age crack types in hardened concrete [1]. Cracking in concrete structures, if not avoided, may present one of the most severe problems in construction because it can reduce their durability and lifetime [2]. It is worth noting that the durability of concrete structures depends on their behavior in the face of severe climatic and environmental conditions [3] Shrinkage and thermal stresses experienced during concrete curation and hydration often lead to severe early-age cracks [4]. These problems may become even more severe when cracking occurs before the concrete begins to harden [5].
It is well known that the excessive evaporation of the mixing water dramatically influences the durability of concrete. It should be remembered that the temperature in these southern regions can reach significant values in the summer period; it can even exceed 50 ℃ [6]. Compressive concrete strength at the initial days was deficient and increased with an increase in the age up to 28 days [7].
Furthermore, very low relative humidity can also be responsible for cracking in concrete [8]. Reinforced concrete structures are often exposed to numerous undesirable physicochemical attacks, increasing the risk of cracking [9]. Moreover, the concrete formulation may also affect plastic cracking from chemical and physical points of view [10,11]. It is worth citing, by way of example, the constituents' chemical composition, the amount of cement, the ratio (W/C), the quantity, shape, and size of fines, and the distribution of agammaegates [12,13]. Cracks can be also developed due to various factors like internal/external pressure or loading criteria, curing, material, mixing, environmental effects, improper compaction, etc. [14]. Therefore, to improve the durability of concrete structures in engineering, cracks, wildly penetrating cracks, caused by early-age shrinkage must be mitigated [15]. However, the concrete mixed workability is increased and maintained using more superplastic is added to increase the concrete's durability [16].
The most significant negative impact of hot weather on fresh concrete is cracking due to plastic shrinkage [17]. Almusallam et al. studied the effect of mixing proportions on plastic shrinkage cracking of concrete in hot environments, the cumulative effect of these parameters on the plastic shrinkage of concrete was evaluated by measuring the bleeding rate, water evaporation, time and cracking intensity. The results indicate that cement content and water-cement ratio significantly affect the parameters controlling the plastic shrinkage of concrete. The mix design studied can be advantageously used in hot environments to minimize plastic shrinkage cracking [17].
Also, the effects of a hot climate on the cooling and curing properties of self-hardening concrete and normal conventional concrete in hot weather were investigated by Rizzuto et al., They used a water-soluble self-hardening polymer, polyethylene glycol, was added to the self-curing mixes. They tested the properties, as workability, compressive strength, tensile strength and flexural strength, but the results could not be simply attributed to the mix's water retention by the self-hardening admixture [18]. A large span of floor slabs, exposed to dry environmental conditions, promotes high evaporation rates [19].
With this in mind, and to study the impact of arid climatic conditions on concrete cracking, it decided to conduct the following experimental study. For this, concrete mixtures, similar to those prepared on local sites, were prepared using the same local materials and to respect the same method of formulation of the concrete, while maintaining the same severe climatic conditions.
The first step consisted of identifying and characterizing the materials brought from the most recognized quarries in the region. The preparation of concrete mixes in the laboratory was carried out following the Faury method [20], which is generally applied in the region of Adrar. Then, the concrete was placed in standard molds. After demolding, the specimens were exposed to the summer climate during which the temperature could reach 48 ℃, with a humidity level lower than 10%. Two conservation methods were adopted; some samples were stored inside the laboratory, and the others were kept outside, in the open air, according to the American Standard ASTM C 1579 [21].
The region of Adrar is located in the southwestern part of Algeria, where the climate is desert and hot, according to the Köppen BWh classification [22]. Adrar is known for its high temperatures during the summer season (spread over more than 6 months) and shallow temperatures in winter, in addition to its year-round drought (few mm of annual precipitation), and a deficient humidity level in summer [23].
The average maximum temperatures vary between 46 and 50 ℃, which confirms that Adrar is one of the world's hottest cities.
The World Weather Online site, dedicated to providing global weather forecasts and weather content [24], gives the variations in temperature and relative humidity recorded during the year 2019. These variations are shown in Figures 1 and 2. It is easy to see that July and August are the hottest months of the year. It is for this reason that the present experimental study was carried out during that period.
It is worth noting that all the constituents of our concretes come from locally sourced materials, which are virtually used in the majority of private or public construction sites in the region of Adrar.
The cement used is type CEMII, CPJ 42.5, and comes from the Aoulef Cement Plant located 150 km south of the capital; its commercial name is STG. Its composition is given in Table 1.
Element | Content (%) |
SiO2 | 21.30 |
Al2O3 | 4.83 |
Fe2O3 | 3.31 |
CaO | 64.53 |
SO3 | 2.28 |
MgO | 1.43 |
Free CaO | 0.61 |
The sand is generally brought from dunes in the locality of Bouda, 20 km west of Adrar.
Particle-size analysis of dune sand:
The analysis of the agammaegates used, including sand, is displayed below (Figure 3), following Standard NF P 18-560 [25].
The water content is 1.6% for dune sand.
The absolute volumetric mass density of dune sand is of the order of 1.63 g/cm3.
The sand equivalent is an indicator that characterizes the cleanliness of sand. It also indicates the content of fines, which are elements with a diameter smaller than 0.5 mm.
The tests were carried out according to Standard NF EN 933-8/IN1[26]. The values obtained, illustrated in Figure 4, allow concluding that the dune sand from Bouda is spotless.
Fineness modulus of dune sand: according to Standard NF P 18 304 [27], is equal to one-hundredth of the sum of rejects, expressed as a percentage by weight, on the various sieves in the series, except the 0.08 sieve. The sand of the dune of Bouda is a little too fine, with a modulus of fineness Mf = 1.90.
The first step consisted of physically and mechanically characterizing the materials used. Note that the gravel comes from the Koussane quarry, located about 8 km from Adrar.
The absolute volumetric mass of the agammaegates:
According to Standard NF EN 12620+A1[28], the absolute volumetric mass densities are around 2.5 g/cm3 for agammaegates 3/8 and 8/15. However, the apparent volumetric mass densities are 1.39 g/cm3 for the agammaegate 3/8 and 1.48 g/cm3 for the agammaegate 8/15.
The water content of agammaegates is between 1% to 2% for the agammaegates 3/8 and 8/15.
Coefficient of absorption of agammaegates is defined as the ratio of an increase in the mass of a water-soaked sample to that sample's dry mass. Table 2 shows the coefficient values of the different agammaegates.
Granular class | Ab (%) |
3/8 | 2.68 |
8/15 | 2.88 |
The hardness and abrasion of agammaegates were evaluated using the Los-Angeles (LA) and Micro-Deval (MDE) tests, and the results obtained are reported in Figure 5.
Note that the sample is more resistant to wear when the Micro-Deval coefficient is low.
The absorption coefficient of agammaegates is defined as the ratio of an increase in the mass of a water-soaked sample to that sample's dry mass.
As for the mixing water used, it comes from the drinking water supply network from the Oued-Ezzine (Adrar) water table.
The adjuvant, namely MEDAFLOW 3041 R, a high-water reducer and setting retarder, was used by standard NF EN 934-2 [29]. It is prepared based on synthetic and combined polymers. The adjuvant MEDAFLOW 3041 R has a setting retarding effect as a secondary function. This allows us to achieve also a sharp increase in compressive strength [30].
Following Standard NF P 18-370 [31], and for the sake of protecting the prepared concrete against desiccation, a curing product named MEDACURE was used. This product comes in the form of a whitish liquid, intended to cover fresh concretes and mortars.
The Faury method for concrete mix design was selected for this study as it is the most widely used in the region of Adrar. It is essential to mention that some project developers use adjuvants and even curing agents to protect the concrete. Indeed, several studies have focused on the incorporation of adjuvants in concrete [25,26,27,28,31].
The mixing curves: based on the granular curves of Figure 6, it was deduced that the percentages obtained for each constituent, namely (dune sand + cement), gravel 3/8, and gravel 8/15, were respectively 44%, 24%, and 32%. The dosages of the various constituents for 1 m3 of concrete are summarized in Table 3.
Materials | Masses for control concrete and with curing (kg/m3) | Masses for concretes with adjuvant (kg/m3) |
Cement | 350 | 350 |
Dune sand | 581 | 602 |
Gravel 3/8 | 471 | 497 |
Gravel 8/15 | 628 | 661 |
Water | 255 | 198 |
Adjuvant | 0 | 5.8 |
Several tests were carried out on slabs to study the potential of plastic shrinkage cracking in concrete. Each set of three slabs was subjected to two types of preservation, i.e., one inside the laboratory and the other outside.
According to ASTM C 1579, cracking usually; occurs in the middle of the specimens [32].
Among the crack mitigation methods previously investigated by some researchers, the one relating to the introduction of adjuvants into concrete proved to be interesting [33].
It is worth specifying that these adjuvants can reduce the surface tension of water in concrete, which in turn; reducing water evaporation, settlement of fresh concrete, and capillary pressures. These are the main effects of reducing plastic shrinkage [11,34]. Also, the curing compounds used on construction sites can effectively prevent rapid water loss from concrete and minimizing cracking risk. To this end, mixtures of concrete with adjuvants similar to those used in the region's construction sites were examined.
After determining the various percentages and quantities of the components making up our concretes, it was decided to proceed with the preparation of the mixtures and the casting of small slabs, according to Standard ASTM 1579 [21].
Since many other parameters, such as granulometry, concrete formulation procedures, slump, concrete temperature, can also influence potential cracking, care should be taken to ensure that they are very similar.
Molds were then made according to Standard ASTM C1579, as shown in Figure 7, and the concrete mixtures were prepared according to the method of Faury [20].
The indicator showed a temperature around 37 ℃ inside the laboratory. The unfavorable climatic conditions, generally encountered in summer (high temperature, low relative humidity, dry air, wind, and sun) during the placement of exposed concrete can contribute to the rapid loss of water and may therefore give rise to crazing and cracking problems, particularly during the setting period and at the onset of concrete hardening.
The adjuvants were incorporated during the mixing of concrete at a rate of 0.5% of the cement mass in the concrete mix, according to Standard NF EN 934-2/A2 [35].
Curing was also used to protect concrete against rapid drying and minimize the risk of cracking. Following the disruption of hydration due to lack of water in early concrete, a curing product was applied by spraying to the surface of fresh concrete according to Standard NF P 18-370 [31]. In certain cases, this curing product is compulsory (Standard NF EN 13670) [36].
Three sets of specimens were adopted, with each set containing six slabs; three were kept outside the laboratory, in the open air, and the other three were stored inside the laboratory. The various concrete mixtures produced are:
-Concrete without adjuvant (control mixture);
-Concrete with adjuvant;
-Concrete treated with a curing product.
The first step was to control the variation in fresh concrete temperatures in the three concretes, as clearly shown in Figure 8.
The measurement of the temperature of fresh concrete with the measuring instrument, i.e., the concrete thermometer. The recorded values are shown in the histogram shown in Figure 9.
The temperature of fresh control concrete without adjuvant was equal to 37 ℃; it is identical to that of the concrete treated with the curing agent. On the other hand, for the concrete with adjuvant, the indicator registered on average the temperature of 36 ℃. This was due to the introduction of the adjuvant into the concrete.
The slump is determined by using the Abrams cone (Standard NF P18-451) which measures concrete slump [37]. We proceeded to mix the concrete so that the workability is maintained constant at plastic consistency [38].
The slump estimates the concrete's consistency, as shown in Figure 10. The value of the Abrams cone slump for all concretes is given in Figure 11. These values obtained for the three types of concretes are divided into two classes; the first is that of the control and cured concretes, and the second is that of the concrete containing the admixture.
The control slabs and those treated with the curing agent showed an average sag of 8 cm, which means that it is plastic concrete. However, the adjuvant's slabs exhibited a sag of about 10 cm, making it very plastic concrete.
In studies on cracking, especially cracking of fresh concrete, it is essential to have appropriate methods to quantify free shrinkage: the mechanism and cracking: the consequence. Several disadvantages and limitations of the available methods are as follows.
The breaking mechanism of plastic shrinkage (cracking) in the dry state can be summarized as follows. The rapid evaporation of water from the surface pores, while the entire body is still saturated, leads to large deformations. The evaporation from the surface pores causes the formation of menisci in these pores and increases capillary pressure. The capillary pressure causes a dramatic increase in rigidity, which leads to a corresponding increase in stress. When these stresses exceed the breaking limit, cracking begins [39].
Three types of concrete were made and divided into two sets; the first was stored inside the laboratory, and the second was outside, in the open air. To simplify the reading, the following names were given to the various slabs:
CSO: concretes without adjuvant (control), slabs stored outside the laboratory;
CSI: concretes without adjuvant (control), slabs stored inside the laboratory;
SAO: concretes with adjuvant, slabs stored outside the laboratory;
SAI: concretes with adjuvant, slabs stored inside the laboratory;
SCO: concretes treated with a curing product, slabs stored outside the laboratory;
SCI: concretes treated with a curing product, slabs stored inside the laboratory.
After each casting of the slabs, the cracks' evolution and their development in lengths and widths were monitored from their first appearance as shown in Figure 12. To better interpret the results, this progression the standard deviation (S.D) is given in the form of graphs.
The performance of passive plastic shrinkage crack mitigation methods was compared according to the ASTM standard, comparing final crack sizes.
Figure 13a shows that, after the second hour, cracks suddenly appeared remarkably, in the middle of the three slabs, with significant lengths (from 10 to over 20 cm). After about two hours, this crack length increased by about 10 cm for all slabs. After 5 hours of casting, these values kept increasing and stabilized around 33 cm. It should be noted that the crack's width could reach even 0.6 mm (Figure 13a).
On the other hand, for the same formulation, the control slabs stored inside the laboratory, sheltered from the sun and wind (Figure 13b), presented the same aspect of progression over time. However, after the sixteenth hour, the cracks' initiation was not observed; by the nineteenth hour, these cracks' progression stopped altogether, for an average length of 17 cm. In this case, the progression of the cracks lasted only three hours. It should be noted that the length of these cracks was significantly smaller than that of cracks on the slabs kept outside the laboratory. Their widths did not exceed 0.2 mm (Figure 14a), which is three times less than those observed on slabs kept outside the laboratory. This difference in crack width proves the strong impact of the climate on concrete structures in this region. This phenomenon is mainly due to the rapid evaporation of water.
For the concretes incorporating the adjuvant and the three slabs cast and kept in the open air outside the laboratory (Figure 14c), the curve shows cracks in the third hour. In contrast, the length of cracks remained constant in all the slabs throughout the observation period. Indeed, the recorded crack lengths varied between 12 and 14 cm. Furthermore, it was found that the crack widths were about 0.2 mm. They are smaller than those of concretes without adjuvant. This is undoubtedly due to the effect of the adjuvant.
Cracks appeared on the slabs with the adjuvant and were stored in the laboratory (Figure 13d). The cracks' progression rate was nearly the same; the average length value was about 32 cm. After three hours, the progression of cracks in all the slabs stopped completely. It should also be noted that the width of cracks was smaller than that observed on the slabs kept outside the laboratory (Figure 14b); indeed, it did not exceed 0.1 mm, which supports the climate impact on concrete.
The concrete treatment results with a curing product are shown in (Figure 13e), where small cracks appeared from the first hour. Under the curing agent treatment effect, which prevented the rapid evaporation of water present in the concrete, the evolution of cracking became slower until the sixteenth hour of hardening. Note that the crack length increased from 5 to about 20 cm, with a crack width varying between 0.2 and 0.3 mm. This represents a value less than half of that observed in the control slabs (without curing treatment).
On the other hand, concerning the slabs treated with a curing product and stored outside the laboratory, cracks appeared within the first two hours (Figure 13f); their length was constant and about 26 cm. These cracks did not exceed 0.1 mm in width (Figure 14c). It is worth noting that the curing effect aided in the retention of the mixed water inside concrete.
High ambient temperature, low humidity, and high-wind speed increase water evaporation from concrete surfaces with the possibility of plastic shrinkage cracking.
This evaporation is all the more critical when the ambient air is dry (low RH), when the temperature is high and when the wind speed is essential.
Particularly in arid areas, chemical reactions accelerate and modify the crystallization of hydrates. Also, the hydration of cement is an exothermic reaction. The resulting plastic deformations are a complex combination of mechanisms appearing at different scales whose effect is cumulative to two types of drying; drying at high ambient temperatures with the effect of the and drying at high ambient temperatures without effect the wind.
Air moisture is of paramount importance to the water balance of concrete. When the concrete's moisture content is higher than that of the air (as in arid areas), water tends to evaporate into the air.
These phenomena lead, in particular, to an increase in shrinkage and risks of early cracking.
According to the results obtained and shown in the Figures 13 and 14, the appearance of cracks and their development is also mentioned in Table 4.
Slabs | Average onset of cracking (h) | Average initial crack length (cm) | Average final crack length (cm) | Average crack width (mm) |
CSO | 2.68 | 163.3 | 337.0 | 0.57 |
CSI | 16.53 | 102.7 | 173.0 | 0.20 |
SAO | 2.30 | 116.0 | 120.0 | 0.23 |
SAI | 2.68 | 130.0 | 234.7 | 0.10 |
SCO | 1.21 | 45.0 | 199.0 | 0.24 |
SCI | 2.33 | 244.3 | 266.7 | 0.10 |
Based on the analysis of the values presented in Table 4, and by comparing the experimental results of slabs kept inside the laboratory with those kept outside, we found that the first crack always appears in slabs kept outside the laboratory (CSO, SAO, and SCO). Also, the cracks' lengths and widths' critical values are observed in the slabs kept outside the laboratory (SCI, SAI, and SCI), which is explained by the fact that outside the laboratory, the climate is hot and dry. The development of cracks in slabs stored outside the laboratory is faster and more critical than that of slabs stored indoors.
Consequently, the appearance of cracks in this hot and arid climate can start to appear, in general, from the second hour of pouring and then accelerate. However, the addition of the admixture reduces the water's surface tension in the concrete, and treatment with the curing agent prevents the water loss from the concrete. These treatments reduce plastic shrinkage, delay the appearance of cracks and slow their development. They considerably reduce their widths.
The standard deviation for concrete is the method for determining the reliability of a batch of concrete. The standard deviation is the basis for controlling the variability of concrete test results for the same concrete batch.
The maximum standard deviations obtained were 8 and 10 mm, in crack length for all slabs and various formulations outside the laboratory. The same goes for those inside the laboratory (Figure 13a, c, e). Figure 13b, d, f show the lowest value for that inside the laboratory.
The standard deviation is between 0 and 0.1 mm width cracks. These values gave a good indication of the presence of crack and the values correlated.
The crack was visible at an exact position indicated by a characteristic response of the crack and its location. Further, the confidence interval obtained from the standard deviation was very low, which proved the repeatability and feasibility of these results.
This study aimed to investigate the effect of severe climatic conditions, which characterize Adrar's region (arid zone), on the cracking of concrete constructions in that region, using local materials. It was decided to introduce an adjuvant on the one hand, and on the other hand, a curing product into the concretes under study.
The first step consisted of carrying out experimental tests to characterize of materials, calculate subsidence, and measure the temperature of fresh concrete. The second step focused on the evolution of cracks and their dimensions (length and width of the crack).
The main conclusions drawn from this study are as follows:
● Crack initiation always occurs first on slabs stored outside the laboratory, with more rapid evolution. On the other hand, the cracks are slightly delayed on slabs kept inside the laboratory;
● Comparison of crack widths on slabs kept outside the laboratory and on those kept inside the laboratory confirmed the significant impact of the arid climate of this region on concrete;
● The findings indicated that the crack widths on slabs kept inside the laboratory were about 66% smaller than those kept outside the laboratory;
● The introduction of the adjuvant into the mixture significantly reduced the progress of cracking. A cracking reduction rate of approximately 50% in length and 66% in width was recorded;
● The treatment with a curing product contributed to slowing down the evolution of cracks by protecting the concrete against rapid drying, by approximately 40% and 50% in length and width, respectively, compared with the control slabs kept outside the laboratory. This drop was approximately 50% for the crack width than that observed on slabs kept outside the laboratory;
● Cracking due to plastic shrinkage is significant in this arid region. It would be interesting to develop low-shrinkage concretes that minimize cracking. It is therefore essential to cure the surface of the concrete during the first two or three days. It can also be achieved by using a shrinkage-reducing admixture;
● Based on the results obtained from our experimental program and in comparison, with control slabs (without addition and treatment), it is recommended to improve the concrete formulation based on local materials with better thermo-mechanical properties.
All authors declare no conflicts of interest in this paper.
[1] |
R. Usman, P. Mirzania, S. W. Alnaser, P. Hart, C. Long, Systematic review of demand-side management strategies in power systems of developed and developing countries, Energies, 15 (2022), 7858. https://doi.org/10.3390/en15217858 doi: 10.3390/en15217858
![]() |
[2] |
Y. Shi, H. Li, X. Fu, R. Luan, Y. Wang, N. Wang, et al., Self-powered difunctional sensors based on sliding contact-electrification and tribovoltaic effects for pneumatic monitoring and controlling, Nano Energy, 110 (2023), 108339. https://doi.org/10.1016/j.nanoen.2023.108339 doi: 10.1016/j.nanoen.2023.108339
![]() |
[3] |
Y. Shi, L. Li, J. Yang, Y. Wang, S. Hao, Center-based transfer feature learning with classifier adaptation for surface defect recognition, Mech. Syst. Signal Process., 188 (2023), 110001. https://doi.org/10.1016/j.ymssp.2022.110001 doi: 10.1016/j.ymssp.2022.110001
![]() |
[4] |
J. P. Lopes, N. Hatziargyriou, J. Mutale, P. Djapic, N. Jenkins, Integrating distributed generation into electric power systems: A review of drivers, challenges and opportunities, Electr. Power Syst. Res., 77 (2007), 1189–1203. https://doi.org/10.1016/j.epsr.2006.08.016 doi: 10.1016/j.epsr.2006.08.016
![]() |
[5] |
P. Dondi, D. Bayoumi, C. Haederli, D. Julian, M. Suter, Network integration of distributed power generation, J. Power Sources, 106 (2002), 1–9. https://doi.org/10.1016/S0378-7753(01)01031-X doi: 10.1016/S0378-7753(01)01031-X
![]() |
[6] |
B. G. De Soto, B. T. Adey, Preliminary resource-based estimates combining artificial intelligence approaches and traditional techniques, Procedia Eng., 164 (2016), 261–268. https://doi.org/10.1016/j.proeng.2016.11.618 doi: 10.1016/j.proeng.2016.11.618
![]() |
[7] |
A. E. L. Rivas, T. Abrao, Faults in smart grid systems: Monitoring, detection and classification, Electr. Power Syst. Res., 189 (2020), 106602. https://doi.org/10.1016/j.epsr.2020.106602 doi: 10.1016/j.epsr.2020.106602
![]() |
[8] |
D. Wang, X. Wang, Y. Zhang, L. Jin, Detection of power grid disturbances and cyber-attacks based on machine learning, J. Inf. Secur. Appl., 46 (2019), 42–52. https://doi.org/10.1016/j.jisa.2019.02.008 doi: 10.1016/j.jisa.2019.02.008
![]() |
[9] |
A. K. Jain, J. Mao, K. M. Mohiuddin, Artificial neural networks: A tutorial, Comput., 29 (1996), 31–44. https://doi.org/10.1109/2.485891 doi: 10.1109/2.485891
![]() |
[10] | J. Xie, L. Zhang, L. Duan, J. Wang, On cross-domain feature fusion in gearbox fault diagnosis under various operating conditions based on transfer component analysis, in Proc. IEEE Int. Conf. Prognost. Health Manage., IEEE, 2016, 1–6. https://doi.org/10.1109/ICPHM.2016.7542845 |
[11] |
R. Janarthanan, R. U. Maheshwari, P. K. Shukla, P. K. Shukla, S. Mirjalili, M. Kumar, Intelligent detection of the pv faults based on artificial neural network and type 2 fuzzy systems, Energies, 14 (2021), 6584. https://doi.org/10.3390/en14206584 doi: 10.3390/en14206584
![]() |
[12] |
A. Mellit, S. Kalogirou, Artificial intelligence and internet of things to improve efficacy of diagnosis and remote sensing of solar photovoltaic systems: Challenges, recommendations and future directions, Renew. Sust. Energy Rev., 143 (2021), 110889. https://doi.org/10.1016/j.rser.2021.110889 doi: 10.1016/j.rser.2021.110889
![]() |
[13] |
P. Dehghanian, S. Aslan, P. Dehghanian, Maintaining electric system safety through an enhanced network resilience, IEEE Trans. Ind. Appl., 54 (2018), 4927–4937. https://doi.org/10.1109/TIA.2018.2828389 doi: 10.1109/TIA.2018.2828389
![]() |
[14] |
M. Mitolo, R. Musca, G. Zizzo, A cost-effective solution for clearing high-impedance ground faults in overhead low-voltage lines, IEEE Trans. Ind. Appl., 55 (2018), 1208–1213. https://doi.org/10.1109/TIA.2018.2884927 doi: 10.1109/TIA.2018.2884927
![]() |
[15] |
P. Velmurugan, A. B. Chattopadhayay, Sensitivity analysis of a single phase to ground fault system in connection with high impedance faults: A case study, Cogent Eng., 7 (2020), 1770916. https://doi.org/10.1080/23311916.2020.1770916 doi: 10.1080/23311916.2020.1770916
![]() |
[16] |
J. M. Guerrero, G. Navarro, K. Mahtani, C. A. Platero, Double line-to-ground faults detection method in dc-ac converters, IEEE Trans. Ind. Appl.. https://doi.org/10.1109/TIA.2022.3212348 doi: 10.1109/TIA.2022.3212348
![]() |
[17] |
M.-S. Choi, S.-J. Lee, S.-I. Lim, D.-S. Lee, X. Yang, A direct three-phase circuit analysis-based fault location for line-to-line fault, IEEE Trans. Power Deliv., 22 (2007), 2541–2547. https://doi.org/10.1109/TPWRD.2007.905535 doi: 10.1109/TPWRD.2007.905535
![]() |
[18] | S. Bakanagari, A. M. Kumar, M. Cheenya, Three phase fault analysis with auto reset for temporary fault and trip for permanent fault, Int. J. Eng. Res. Appl., 3 (2013), 1082–1086. |
[19] | J. P. Nelson, System grounding and ground fault protection in the petrochemical industry: A need for a better understanding, in Record of Conference Papers. IEEE Trans. Ind. Appl. Soc. Forty-Eighth Annual Conference. 2001 Petroleum and Chemical Industry Technical Conference (Cat. No. 01CH37265), IEEE, 2001, 1–9. https://doi.org/10.1109/PCICON.2001.960500 |
[20] |
J. Yang, J. E. Fletcher, J. O'Reilly, Short-circuit and ground fault analyses and location in vsc-based dc network cables, IEEE Trans. Ind. Electron., 59 (2011), 3827–3837. https://doi.org/10.1109/TIE.2011.2162712 doi: 10.1109/TIE.2011.2162712
![]() |
[21] | G. D. Gregory, G. W. Scott, The arc-fault circuit interrupter, an emerging product, in 1998 IEEE Ind. Commer. Power Syst. Tech. Conf. Conference Record. Papers Presented at the 1998 Annual Meeting (Cat. No. 98CH36202), IEEE, 1998, 48–55. https://doi.org/10.1109/ICPS.1998.692533 |
[22] | D. K. Neitzel, T. L. Gauthier, Ground fault protection-gfci or gfpe-there is a difference, in 2013 IEEE IAS Electr. Saf. Workshop, IEEE, 2013,207–210. https://doi.org/10.1109/ESW.2013.6509025 |
[23] | F. Amiel, C. Clavier, M. Tunstall, Fault analysis of dpa-resistant algorithms, in Fault Diagn. Toler. Cryptogr.: Third International Workshop, FDTC 2006, Yokohama, Japan, October 10, 2006. Proceedings, Springer, 2006,223–236. https://doi.org/10.1007/11889700_20 |
[24] |
S. Visacro, R. Alipio, M. H. M. Vale, C. Pereira, The response of grounding electrodes to lightning currents: The effect of frequency-dependent soil resistivity and permittivity, IEEE Trans. Electromagn. Compat., 53 (2011), 401–406. https://doi.org/10.1109/TEMC.2011.2106790 doi: 10.1109/TEMC.2011.2106790
![]() |
[25] |
V. P. Androvitsaneas, K. D. Damianaki, C. A. Christodoulou, I. F. Gonos, Effect of soil resistivity measurement on the safe design of grounding systems, Energies, 13 (2020), 3170. https://doi.org/10.3390/en13123170 doi: 10.3390/en13123170
![]() |
[26] |
N. Hayakawa, Y. Maeno, H. Kojima, Fault current limitation coordination in electric power grid with superconducting fault current limiters, IEEE Trans. Appl. Supercond., 28 (2018), 1–4. https://doi.org/10.1109/TASC.2018.2818279 doi: 10.1109/TASC.2018.2818279
![]() |
[27] | L. Hewitson, M. Brown, R. Balakrishnan, Practical power system protection, Elsevier, 2004. |
[28] |
N. Permal, M. Osman, M. Z. A. A. Kadir, A. M. Ariffin, Review of substation grounding system behavior under high frequency and transient faults in uniform soil, IEEE Access, 8 (2020), 142468–142482. https://doi.org/10.1109/ACCESS.2020.3013657 doi: 10.1109/ACCESS.2020.3013657
![]() |
[29] |
S. Beheshtaein, R. Cuzner, M. Savaghebi, J. M. Guerrero, Review on microgrids protection, IET Gener. Transm. Distrib., 13 (2019), 743–759. https://doi.org/10.1049/iet-gtd.2018.5212 doi: 10.1049/iet-gtd.2018.5212
![]() |
[30] | D. Greene, A. L. Hoffmann, L. Stark, Better, nicer, clearer, fairer: A critical assessment of the movement for ethical artificial intelligence and machine learning. |
[31] | P. P. Barker, R. W. De Mello, Determining the impact of distributed generation on power systems. i. radial distribution systems, in 2000 Power Eng. Soc. Summer Meet. (Cat. No. 00CH37134), vol. 3, IEEE, 2000, 1645–1656. https://doi.org/10.1109/PESS.2000.868775 |
[32] |
H. Su, A. Mariani, S. E. Ovur, A. Menciassi, G. Ferrigno, E. De Momi, Toward teaching by demonstration for robot-assisted minimally invasive surgery, IEEE Trans. Autom. Sci. Eng., 18 (2021), 484–494. https://doi.org/10.1109/TASE.2020.3045655 doi: 10.1109/TASE.2020.3045655
![]() |
[33] |
Z. Liu, D. Yang, Y. Wang, M. Lu, R. Li, Egnn: Graph structure learning based on evolutionary computation helps more in graph neural networks, Appl. Soft Comput., 110040. https://doi.org/10.1016/j.asoc.2023.110040 doi: 10.1016/j.asoc.2023.110040
![]() |
[34] |
Y. Wang, Z. Liu, J. Xu, W. Yan, Heterogeneous network representation learning approach for ethereum identity identification, IEEE Trans. Comput. Soc. Syst.. https://doi.org/10.1109/TCSS.2022.3164719 doi: 10.1109/TCSS.2022.3164719
![]() |
[35] |
W. Qi, H. Fan, H. R. Karimi, H. Su, An adaptive reinforcement learning-based multimodal data fusion framework for human–robot confrontation gaming, Neural Netw., 164 (2023), 489–496. https://doi.org/10.1016/j.neunet.2023.04.043 doi: 10.1016/j.neunet.2023.04.043
![]() |
[36] |
C. Tian, Z. Xu, L. Wang, Y. Liu, Arc fault detection using artificial intelligence: Challenges and benefits, Math. Biosci. Eng., 20 (2023), 12404–12432. https://doi.org/10.3934/mbe.2023552 doi: 10.3934/mbe.2023552
![]() |
[37] |
H. Salehi, R. Burgueño, Emerging artificial intelligence methods in structural engineering, Eng. Struct., 171 (2018), 170–189. https://doi.org/10.1016/j.engstruct.2018.05.084 doi: 10.1016/j.engstruct.2018.05.084
![]() |
[38] |
P. Boza, T. Evgeniou, Artificial intelligence to support the integration of variable renewable energy sources to the power system, Appl. Energy, 290 (2021), 116754. https://doi.org/10.1016/j.apenergy.2021.116754 doi: 10.1016/j.apenergy.2021.116754
![]() |
[39] |
M. S. Saeed, M. W. Mustafa, N. N. Hamadneh, N. A. Alshammari, U. U. Sheikh, T. A. Jumani, et al., Detection of non-technical losses in power utilities—a comprehensive systematic review, Energies, 13 (2020), 4727. https://doi.org/10.3390/en13184727 doi: 10.3390/en13184727
![]() |
[40] |
W. Qi, S. E. Ovur, Z. Li, A. Marzullo, R. Song, Multi-sensor guided hand gesture recognition for a teleoperated robot using a recurrent neural network, IEEE Robot. Autom. Lett., 6 (2021), 6039–6045. https://doi.org/10.1109/LRA.2021.3089999 doi: 10.1109/LRA.2021.3089999
![]() |
[41] |
S.-Y. Park, J. Ahn, Deep neural network approach for fault detection and diagnosis during startup transient of liquid-propellant rocket engine, Acta Astronaut., 177 (2020), 714–730. https://doi.org/10.1016/j.actaastro.2020.08.019 doi: 10.1016/j.actaastro.2020.08.019
![]() |
[42] |
W. Qi, H. Su, A cybertwin based multimodal network for ecg patterns monitoring using deep learning, IEEE Trans. Ind. Inform., 18 (2022), 6663–6670. https://doi.org/10.1109/TII.2022.3159583 doi: 10.1109/TII.2022.3159583
![]() |
[43] |
A. Eskandari, M. Aghaei, J. Milimonfared, A. Nedaei, A weighted ensemble learning-based autonomous fault diagnosis method for photovoltaic systems using genetic algorithm, Int. J. Electr. Power Energy Syst., 144 (2023), 108591. https://doi.org/10.1016/j.ijepes.2022.108591 doi: 10.1016/j.ijepes.2022.108591
![]() |
[44] |
H. Su, W. Qi, J. Chen, D. Zhang, Fuzzy approximation-based task-space control of robot manipulators with remote center of motion constraint, IEEE Trans. Fuzzy Syst., 30 (2022), 1564–1573. https://doi.org/10.1109/TFUZZ.2022.3157075 doi: 10.1109/TFUZZ.2022.3157075
![]() |
[45] |
A. A. Selçuk, A guide for systematic reviews: Prisma, Turk. Arch. Otorhinolaryngol., 57 (2019), 57. https://doi.org/10.5152/tao.2019.4058 doi: 10.5152/tao.2019.4058
![]() |
[46] | N. Diaz, A. Luna, O. Duarte, Improved mppt short-circuit current method by a fuzzy short-circuit current estimator, in 2011 IEEE Energy Convers. Congr. Expo., IEEE, 2011,211–218. https://doi.org/10.1109/ECCE.2011.6063771 |
[47] |
H. A. Sher, A. F. Murtaza, A. Noman, K. E. Addoweesh, K. Al-Haddad, M. Chiaberge, A new sensorless hybrid mppt algorithm based on fractional short-circuit current measurement and p & o mppt, IEEE Trans. Sustain. Energy, 6 (2015), 1426–1434. https://doi.org/10.1109/TSTE.2015.2438781 doi: 10.1109/TSTE.2015.2438781
![]() |
[48] |
R. K. Varma, S. A. Rahman, V. Atodaria, S. Mohan, T. Vanderheide, Technique for fast detection of short circuit current in pv distributed generator, IEEE Power Energy Technol. Syst. J., 3 (2016), 155–165. https://doi.org/10.1109/JPETS.2016.2592465 doi: 10.1109/JPETS.2016.2592465
![]() |
[49] |
C. Li, C. Zhao, J. Xu, Y. Ji, F. Zhang, T. An, A pole-to-pole short-circuit fault current calculation method for dc grids, IEEE Trans. Power Syst., 32 (2017), 4943–4953. https://doi.org/10.1109/TPWRS.2017.2682110 doi: 10.1109/TPWRS.2017.2682110
![]() |
[50] |
L. M. Castro, D. Guillen, F. Trillaud, On short-circuit current calculations including superconducting fault current limiters (scfcls), IEEE Trans. Power Deliv., 33 (2018), 2513–2523. https://doi.org/10.1109/TPWRD.2018.2800732 doi: 10.1109/TPWRD.2018.2800732
![]() |
[51] |
S. Mohsenzade, J. Naghibi, K. Mehran, Reliability enhancement of power igbts under short-circuit fault condition using short-circuit current limiting-based technique, Energies, 14 (2021), 7397. https://doi.org/10.3390/en14217397 doi: 10.3390/en14217397
![]() |
[52] |
B. Gustavsen, Study of transformer resonant overvoltages caused by cable-transformer high-frequency interaction, IEEE Trans. Power Deliv., 25 (2010), 770–779. https://doi.org/10.1109/TPWRD.2010.2040292 doi: 10.1109/TPWRD.2010.2040292
![]() |
[53] |
Y. Choi, T. Alkhalifah, Source-independent time-domain waveform inversion using convolved wavefields: Application to the encoded multisource waveform inversion, Geophysics, 76 (2011), R125–R134. https://doi.org/10.1190/geo2010-0210.1 doi: 10.1190/geo2010-0210.1
![]() |
[54] |
G. Vignoli, G. Fiandaca, A. V. Christiansen, C. Kirkegaard, E. Auken, Sharp spatially constrained inversion with applications to transient electromagnetic data, Geophys. Prospect., 63 (2015), 243–255. https://doi.org/10.1111/1365-2478.12185 doi: 10.1111/1365-2478.12185
![]() |
[55] | D. Stoller, S. Ewert, S. Dixon, Wave-u-net: A multi-scale neural network for end-to-end audio source separation, arXiv preprint arXiv: 1806.03185. https://doi.org/10.48550/arXiv.1806.03185 |
[56] | N. Zhi, H. Zhang, N. Li, J. Yang, System-level design and stability analysis of dc microgrid, in 2014 IEEE Int. Power Electron. Appl. Conf. Expo., IEEE, 2014, 1134–1137. https://doi.org/10.1109/PEAC.2014.7038020 |
[57] |
P.-J. Kim, H.-G. Kim, B.-H. Cho, Evaluation of electrical impedance ratio measurements in accuracy of electronic apex locators, Restor. Dent. Endod., 40 (2015), 113–122. https://doi.org/10.5395/rde.2015.40.2.113 doi: 10.5395/rde.2015.40.2.113
![]() |
[58] |
J. Liu, N. Tai, C. Fan, Y. Yang, Transient measured impedance-based protection scheme for dc line faults in ultra high-voltage direct-current system, IET Gener. Transm. Distrib., 10 (2016), 3597–3609. https://doi.org/10.1049/iet-gtd.2016.0408 doi: 10.1049/iet-gtd.2016.0408
![]() |
[59] |
T. P. S. Bains, T. S. Sidhu, Z. Xu, I. Voloh, M. R. D. Zadeh, Impedance-based fault location algorithm for ground faults in series-capacitor-compensated transmission lines, IEEE Trans. Power Deliv., 33 (2017), 189–199. https://doi.org/10.1109/TPWRD.2017.2711358 doi: 10.1109/TPWRD.2017.2711358
![]() |
[60] |
X. Wang, H. Zhang, F. Shi, Q. Wu, V. Terzija, W. Xie, et al., Location of single phase to ground faults in distribution networks based on synchronous transients energy analysis, IEEE Trans. Smart Grid, 11 (2019), 774–785. https://doi.org/10.1109/TSG.2019.2938667 doi: 10.1109/TSG.2019.2938667
![]() |
[61] |
R. A. F. Pereira, L. G. W. da Silva, M. Kezunovic, J. R. S. Mantovani, Improved fault location on distribution feeders based on matching during-fault voltage sags, IEEE Trans. Power Deliv., 24 (2009), 852–862. https://doi.org/10.1109/TPWRD.2009.2014480 doi: 10.1109/TPWRD.2009.2014480
![]() |
[62] |
A. H. A. Bakar, M. Ali, C. Tan, H. Mokhlis, H. Arof, H. Illias, High impedance fault location in 11 kv underground distribution systems using wavelet transforms, Int. J. Electr. Power Energy Syst., 55 (2014), 723–730. https://doi.org/10.1016/j.ijepes.2013.10.003 doi: 10.1016/j.ijepes.2013.10.003
![]() |
[63] |
L. J. Awalin, H. Mokhlis, M. Rahmat, S. Shilpa, F. Albatsh, et al., Fault distance identification using impedance and matching approaches on distribution network, Indones. J. Electr. Eng. Comput. Sci., 8 (2017), 770–778. https://doi.org/10.11591/ijeecs.v8.i3.pp770-778 doi: 10.11591/ijeecs.v8.i3.pp770-778
![]() |
[64] |
C. Zhang, X. Yuan, M. Shi, J. Yang, H. Miao, Fault location method based on svm and similarity model matching, Math. Probl. Eng., 2020 (2020), 1–9. https://doi.org/10.1155/2020/2898479 doi: 10.1155/2020/2898479
![]() |
[65] | N. Song, C. Zhang, Z. Wang, Fault line selection of distribution networks based on feature matching of power disturbance data, in 2020 Chinese Automation Congress (CAC), IEEE, 2020, 2058–2062. https://doi.org/10.1109/CAC51589.2020.9327430 |
[66] | K. Li, L. Lai, A. David, Application of artificial neural network in fault location technique, in DRPT2000. International Conference on Electric Utility Deregulation and Restructuring and Power Technologies. Proceedings (Cat. No. 00EX382), IEEE, 2000,226–231. https://doi.org/10.1109/DRPT.2000.855668 |
[67] | S. Singh, K. Mamatha, S. Thejaswini, Intelligent fault identification system for transmission lines using artificial neural network, IOSR J. Comput. Eng., 16 (2014), 23–31. |
[68] |
M. Jamil, S. K. Sharma, R. Singh, Fault detection and classification in electrical power transmission system using artificial neural network, SpringerPlus, 4 (2015), 1–13. https://doi.org/10.1186/s40064-015-1080-x doi: 10.1186/s40064-015-1080-x
![]() |
[69] |
J. James, Y. Hou, A. Y. Lam, V. O. Li, Intelligent fault detection scheme for microgrids with wavelet-based deep neural networks, IEEE Trans. Smart Grid, 10 (2017), 1694–1703. https://doi.org/10.1109/TSG.2017.2776310 doi: 10.1109/TSG.2017.2776310
![]() |
[70] | M. Dashtdar et al., Fault location in distribution network based on fault current analysis using artificial neural network, Mapta Journal of Electrical and Computer Engineering (MJECE), 1 (2018), 18–32. |
[71] | A. Yadav, S. Goad, Fault detection on transmission lines using artificial neural network, (2021). |
[72] | V. N. OGAR, S. Hussain, K. A. Gamage, The use of artificial neural network for low latency of fault detection and localisation in transmission line, Available at SSRN 4178882. https://doi.org/10.1016/j.heliyon.2023.e13376 |
[73] |
B. K. Sangeeth, V. Vinod, High impedance fault detection using multi-domain feature with artificial neural network, Electr. Power Compon. Syst., 51 (2023), 366–379. https://doi.org/10.1080/15325008.2023.2172091 doi: 10.1080/15325008.2023.2172091
![]() |
[74] |
A. Quawi, Y. M. Shuaib, M. Manikandan, Power quality improvement using ann controller for hybrid power distribution systems., Intell. Autom. Soft Comput., 36. https://doi.org/10.32604/iasc.2023.035001 doi: 10.32604/iasc.2023.035001
![]() |
[75] | Z. Wang, P. Zhao, Fault location recognition in transmission lines based on support vector machines, in 2009 2nd IEEE international conference on computer science and information technology, IEEE, 2009,401–404. https://doi.org/10.1109/ICCSIT.2009.5234528 |
[76] |
U. B. Parikh, B. Das, R. Maheshwari, Fault classification technique for series compensated transmission line using support vector machine, Int. J. Electr. Power Energy Syst., 32 (2010), 629–636. https://doi.org/10.1016/j.ijepes.2009.11.020 doi: 10.1016/j.ijepes.2009.11.020
![]() |
[77] |
S. Ekici, Support vector machines for classification and locating faults on transmission lines, Appl. Soft Comput., 12 (2012), 1650–1658. https://doi.org/10.1016/j.asoc.2012.02.011 doi: 10.1016/j.asoc.2012.02.011
![]() |
[78] |
P. Ray, D. P. Mishra, Support vector machine based fault classification and location of a long transmission line, Eng. Sci. Technol., Int. J., 19 (2016), 1368–1380. https://doi.org/10.1016/j.jestch.2016.04.001 doi: 10.1016/j.jestch.2016.04.001
![]() |
[79] | Z. Yi, A. H. Etemadi, A novel detection algorithm for line-to-line faults in photovoltaic (pv) arrays based on support vector machine (svm), in 2016 IEEE Power and Energy Society General Meeting (PESGM), IEEE, 2016, 1–4. https://doi.org/10.1109/PESGM.2016.7742026 |
[80] |
Z. Yi, A. H. Etemadi, Line-to-line fault detection for photovoltaic arrays based on multiresolution signal decomposition and two-stage support vector machine, IEEE Trans. Ind. Electron., 64 (2017), 8546–8556. https://doi.org/10.1109/TIE.2017.2703681 doi: 10.1109/TIE.2017.2703681
![]() |
[81] | M. Shafiullah, M. Ijaz, M. Abido, Z. Al-Hamouz, Optimized support vector machine & wavelet transform for distribution grid fault location, in 2017 11th IEEE International Conference on Compatibility, Power Electronics and Power Engineering (CPE-POWERENG), IEEE, 2017, 77–82. https://doi.org/10.1109/CPE.2017.7915148 |
[82] | L. J. Awalin, K. Naidu, H. Suyono, Fault types classification using support vector machine (svm), in AIP Conf. Proc., vol. 2129, AIP Publishing LLC, 2019, 020132. https://doi.org/10.1063/1.5118140 |
[83] |
M. Sarwar, F. Mehmood, M. Abid, A. Q. Khan, S. T. Gul, A. S. Khan, High impedance fault detection and isolation in power distribution networks using support vector machines, J. King Saud Univ.-Eng. Sci., 32 (2020), 524–535. https://doi.org/10.1016/j.jksues.2019.07.001 doi: 10.1016/j.jksues.2019.07.001
![]() |
[84] |
A. Said, M. H. Saad, S. M. Eladl, Z. S. Elbarbary, A. I. Omar, M. A. Saad, Support vector machine parameters optimization for 500 kv long ohtl fault diagnosis, IEEE Access. https://doi.org/10.1109/ACCESS.2023.3235592 doi: 10.1109/ACCESS.2023.3235592
![]() |
[85] | G. Sharma, O. P. Mahela, M. Kumar, N. Kumar, Detection and classification of transmission line faults using stockwell transform and rule based decision tree, in 2018 International Conference on Smart Electric Drives and Power System (ICSEDPS), IEEE, 2018, 1–5. https://doi.org/10.1109/ICSEDPS.2018.8536006 |
[86] | A. Malhotra, O. P. Mahela, H. Doraya, Detection and classification of power system faults using discrete wavelet transform and rule based decision tree, in 2018 International Conference on Computing, Power and Communication Technologies (GUCON), IEEE, 2018,142–147. https://doi.org/10.1109/GUCON.2018.8674922 |
[87] | S. K. Mohanty, A. Karn, S. Banerjee, Decision tree supported distance relay for fault detection and classification in a series compensated line, in 2020 IEEE international conference on power electronics, smart grid and renewable energy (PESGRE2020), IEEE, 2020, 1–6. https://doi.org/10.1109/PESGRE45664.2020.9070724 |
[88] |
S. H. Asman, N. F. Ab Aziz, U. A. Ungku Amirulddin, M. Z. A. Ab Kadir, Decision tree method for fault causes classification based on rms-dwt analysis in 275 kv transmission lines network, Appl. Sci., 11 (2021), 4031. https://doi.org/10.3390/app11094031 doi: 10.3390/app11094031
![]() |
[89] |
S. K. Mohanty, A. Swetapadma, P. K. Nayak, O. P. Malik, Decision tree approach for fault detection in a tcsc compensated line during power swing, Int. J. Electr. Power Energy Syst., 146 (2023), 108758. https://doi.org/10.1016/j.ijepes.2022.108758 doi: 10.1016/j.ijepes.2022.108758
![]() |
[90] |
S. Adhikari, N. Sinha, T. Dorendrajit, Fuzzy logic based on-line fault detection and classification in transmission line, SpringerPlus, 5 (2016), 1–14. https://doi.org/10.1186/s40064-016-2669-4 doi: 10.1186/s40064-016-2669-4
![]() |
[91] | P. Ray, D. P. Mishra, S. Mohaptra, Fault classification of a transmission line using wavelet transform & fuzzy logic, in 2016 IEEE 1st International Conference on Power Electronics, Intelligent Control and Energy Systems (ICPEICES), IEEE, 2016, 1–6. https://doi.org/10.1109/ICPEICES.2016.7853293 |
[92] |
M. Dehghani, M. H. Khooban, T. Niknam, Fast fault detection and classification based on a combination of wavelet singular entropy theory and fuzzy logic in distribution lines in the presence of distributed generations, Int. J. Electr. Power Energy Syst., 78 (2016), 455–462. https://doi.org/10.1016/j.ijepes.2015.11.048 doi: 10.1016/j.ijepes.2015.11.048
![]() |
[93] | A. J. Lekie, D. Idoniboyeobu, S. Braide, Fault detection on distribution line using fuzzy logic, Int. J. Sci. Eng. Res., 9 (2018), 490–503. |
[94] | A. U. Q. Bhat, A. Prakash, V. K. Tayal, P. Choudekar, Three-phase fault analysis of distributed power system using fuzzy logic system (fls), in Advances in Smart Communication and Imaging Systems: Select Proceedings of MedCom 2020, Springer, 2021,615–624. https://doi.org/10.1007/978-981-15-9938-5_57 |
[95] |
H. M. Hasanien, S. Muyeen, Design optimization of controller parameters used in variable speed wind energy conversion system by genetic algorithms, IEEE Trans. Sustain. Energy, 3 (2012), 200–208. https://doi.org/10.1109/TSTE.2012.2182784 doi: 10.1109/TSTE.2012.2182784
![]() |
[96] | A. S. Ahmed, M. A. Attia, N. M. Hamed, A. Y. Abdelaziz, Comparison between genetic algorithm and whale optimization algorithm in fault location estimation in power systems, in 2017 Nineteenth International Middle East Power Systems Conference (MEPCON), IEEE, 2017,631–637. https://doi.org/10.1109/MEPCON.2017.8301247 |
[97] | Y. Song, H. Huang, Y. Chen, The method of bp algorithm for genetic simulated annealing algorithm in fault line selection, in J. Phys.: Conf. Ser., vol. 1650, IOP Publishing, 2020, 032187. https://doi.org/10.1088/1742-6596/1650/3/032187 |
[98] | K. Moloi, A. Yusuff, Power distribution system fault diagnostic using genetic algorithm and neural network, in 2021 Southern African Universities Power Engineering Conference/Robotics and Mechatronics/Pattern Recognition Association of South Africa (SAUPEC/RobMech/PRASA), IEEE, 2021, 1–5. https://doi.org/10.1109/SAUPEC/RobMech/PRASA52254.2021.9377241 |
[99] |
A. Hichri, M. Hajji, M. Mansouri, K. Abodayeh, K. Bouzrara, H. Nounou, et al., Genetic-algorithm-based neural network for fault detection and diagnosis: Application to grid-connected photovoltaic systems, Sustainability, 14 (2022), 10518. https://doi.org/10.3390/su141710518 doi: 10.3390/su141710518
![]() |
[100] |
Q. Wan, S. Zheng, C. Shi, A rapid diagnosis technology of short circuit fault in dc microgrid, Int. J. Electr. Power Energy Syst., 147 (2023), 108878. https://doi.org/10.1016/j.ijepes.2022.108878 doi: 10.1016/j.ijepes.2022.108878
![]() |
[101] | H.-H. Chang, R. Zhang, Machine learning techniques for single-line-to-ground fault classification in nonintrusive fault detection of extra high-voltage transmission network systems, in Proceedings of the Fifth Euro-China Conference on Intelligent Data Analysis and Applications 5, Springer, 2019,109–116. https://doi.org/10.1007/978-3-030-03766-6_12 |
[102] | A. Aljohani, T. Sheikhoon, A. Fataa, M. Shafiullah, M. Abido, Design and implementation of an intelligent single line to ground fault locator for distribution feeders, in 2019 International Conference on Control, Automation and Diagnosis (ICCAD), IEEE, 2019, 1–6. https://doi.org/10.1109/ICCAD46983.2019.9037950 |
[103] |
F. I. Jabbar, D. M. Soomro, A. H. Tawafan, M. N. B. Abdullah, N. H. B. M. Radzi, M. H. Baloch, Optimization of detection of single line to ground fault by controlling peterson coil through anfis, IAES Int. J. Artif. Intell., 9 (2020), 409. https://doi.org/10.11591/ijai.v9.i3.pp409-416 doi: 10.11591/ijai.v9.i3.pp409-416
![]() |
[104] |
M.-F. Guo, J.-H. Gao, X. Shao, D.-Y. Chen, Location of single-line-to-ground fault using 1-d convolutional neural network and waveform concatenation in resonant grounding distribution systems, IEEE Trans. Instrum. Meas., 70 (2020), 1–9. https://doi.org/10.1109/TIM.2020.3014006 doi: 10.1109/TIM.2020.3014006
![]() |
[105] |
P. Liu, S. Du, K. Sun, J. Zhu, D. Xie, Y. Liu, Single-line-to-ground fault feeder selection considering device polarity reverse installation in resonant grounding system, IEEE Trans. Power Del., 36 (2020), 2204–2212. https://doi.org/10.1109/TPWRD.2020.3022422 doi: 10.1109/TPWRD.2020.3022422
![]() |
[106] | L. Zhengyi, D. Changhong, Y. Huamei, F. Hu, A lstm line selection method of single-phase ground fault based on big-data platform, in 2021 3rd Asia Energy and Electrical Engineering Symposium (AEEES), IEEE, 2021,776–780. https://doi.org/10.1109/AEEES51875.2021.9402971 |
[107] |
J. B. Awotunde, C. Chakraborty, A. E. Adeniyi, Intrusion detection in industrial internet of things network-based on deep learning model with rule-based feature selection, Wirel. Commun. Mob. Comput., 2021 (2021), 1–17. https://doi.org/10.1155/2021/7154587 doi: 10.1155/2021/7154587
![]() |
[108] |
B. T. Jijo, A. M. Abdulazeez, Classification based on decision tree algorithm for machine learning, evaluation, 6. https://doi.org/10.38094/jastt20165 doi: 10.38094/jastt20165
![]() |
[109] |
T. Han, L. Zhang, Z. Yin, A. C. Tan, Rolling bearing fault diagnosis with combined convolutional neural networks and support vector machine, Meas., 177 (2021), 109022. https://doi.org/10.1016/j.measurement.2021.109022 doi: 10.1016/j.measurement.2021.109022
![]() |
[110] |
X. Guo, L. Chen, C. Shen, Hierarchical adaptive deep convolution neural network and its application to bearing fault diagnosis, Meas., 93 (2016), 490–502. https://doi.org/10.1016/j.measurement.2016.07.054 doi: 10.1016/j.measurement.2016.07.054
![]() |
[111] |
G. Baldini, R. Giuliani, F. Geib, On the application of time frequency convolutional neural networks to road anomalies' identification with accelerometers and gyroscopes, Sensors, 20 (2020), 6425. https://doi.org/10.3390/s20226425 doi: 10.3390/s20226425
![]() |
[112] |
S. Lan, M.-J. Chen, D.-Y. Chen, A novel hvdc double-terminal non-synchronous fault location method based on convolutional neural network, IEEE Trans. Power Del., 34 (2019), 848–857. https://doi.org/10.1109/TPWRD.2019.2901594 doi: 10.1109/TPWRD.2019.2901594
![]() |
[113] |
S. Phoemphon, C. So-In, D. T. Niyato, A hybrid model using fuzzy logic and an extreme learning machine with vector particle swarm optimization for wireless sensor network localization, Appl. Soft Comput., 65 (2018), 101–120. https://doi.org/10.1016/j.asoc.2018.01.004 doi: 10.1016/j.asoc.2018.01.004
![]() |
[114] |
S. R. K. Joga, P. Sinha, M. K. Maharana, A novel graph search and machine learning method to detect and locate high impedance fault zone in distribution system, Eng. Rep., 5 (2023), e12556. https://doi.org/10.1002/eng2.12556 doi: 10.1002/eng2.12556
![]() |
[115] | L. Shilong, T. Yufei, L. Xiaopeng, Z. Huajie, F. Shilin, Fault line selection of single phase grounding fault in small-current ground system based on reactive current, in 2019 IEEE Innov. Smart Grid Technol. - Asia (ISGT Asia), IEEE, 2019,138–143. https://doi.org/10.1109/ISGT-Asia.2019.8881442 |
[116] |
C. Huang, B. Zhang, Y. Ma, F. Zhou, J. He, Analysis of short-circuit current characteristics and its distribution of artificial grounding faults on dc transmission lines, IEEE Trans. Power Del., 33 (2017), 520–528. https://doi.org/10.1109/TPWRD.2017.2732483 doi: 10.1109/TPWRD.2017.2732483
![]() |
[117] | F. Liao, H. Li, J. Chen, High-impedance ground fault detection based on phase current increment ratio, in 2019 IEEE 8th International Conference on Advanced Power System Automation and Protection (APAP), IEEE, 2019,841–845. https://doi.org/10.1109/APAP47170.2019.9224929 |
[118] |
H. Su, W. Qi, Y. Hu, H. R. Karimi, G. Ferrigno, E. De Momi, An incremental learning framework for human-like redundancy optimization of anthropomorphic manipulators, IEEE Trans. Ind. Inform., 18 (2020), 1864–1872. https://doi.org/10.1109/TII.2020.3036693 doi: 10.1109/TII.2020.3036693
![]() |
[119] |
Y. Zhao, T. Li, X. Zhang, C. Zhang, Artificial intelligence-based fault detection and diagnosis methods for building energy systems: Advantages, challenges and the future, Renew. Sustain. Energy Rev., 109 (2019), 85–101. https://doi.org/10.1016/j.rser.2019.04.021 doi: 10.1016/j.rser.2019.04.021
![]() |
[120] | A. A. Ayokunle, O. M. Peter, A. S. Isaac, Artificial neural networks for intelligent fault location on the 33-kv nigeria transmission line, Artificial Neural Networks for Intelligent, 54 (2017), 147–155. |
[121] | D. Jayamaha, N. Lidula, A. Rajapakse, Wavelet based artificial neural networks for detection and classification of dc microgrid faults, in 2019 IEEE Power & Energy Society General Meeting (PESGM), IEEE, 2019, 1–5. https://doi.org/10.1109/PESGM40551.2019.8974108 |
[122] | S. Cheng, Z. Fu, Single-phase ground fault line selection for distribution network based on frequency domain parameter identification method, in IOP Conf. Ser. Earth Environ. Sci., vol. 267, 2019, 042130. https://doi.org/10.1088/1755-1315/267/4/042130 |
1. | Talal O. Alshammari, Maurizio Guadagnini, Kypros Pilakoutas, The Effect of Harsh Environmental Conditions on Concrete Plastic Shrinkage Cracks: Case Study Saudi Arabia, 2022, 15, 1996-1944, 8622, 10.3390/ma15238622 |
Element | Content (%) |
SiO2 | 21.30 |
Al2O3 | 4.83 |
Fe2O3 | 3.31 |
CaO | 64.53 |
SO3 | 2.28 |
MgO | 1.43 |
Free CaO | 0.61 |
Granular class | Ab (%) |
3/8 | 2.68 |
8/15 | 2.88 |
Materials | Masses for control concrete and with curing (kg/m3) | Masses for concretes with adjuvant (kg/m3) |
Cement | 350 | 350 |
Dune sand | 581 | 602 |
Gravel 3/8 | 471 | 497 |
Gravel 8/15 | 628 | 661 |
Water | 255 | 198 |
Adjuvant | 0 | 5.8 |
Slabs | Average onset of cracking (h) | Average initial crack length (cm) | Average final crack length (cm) | Average crack width (mm) |
CSO | 2.68 | 163.3 | 337.0 | 0.57 |
CSI | 16.53 | 102.7 | 173.0 | 0.20 |
SAO | 2.30 | 116.0 | 120.0 | 0.23 |
SAI | 2.68 | 130.0 | 234.7 | 0.10 |
SCO | 1.21 | 45.0 | 199.0 | 0.24 |
SCI | 2.33 | 244.3 | 266.7 | 0.10 |
Element | Content (%) |
SiO2 | 21.30 |
Al2O3 | 4.83 |
Fe2O3 | 3.31 |
CaO | 64.53 |
SO3 | 2.28 |
MgO | 1.43 |
Free CaO | 0.61 |
Granular class | Ab (%) |
3/8 | 2.68 |
8/15 | 2.88 |
Materials | Masses for control concrete and with curing (kg/m3) | Masses for concretes with adjuvant (kg/m3) |
Cement | 350 | 350 |
Dune sand | 581 | 602 |
Gravel 3/8 | 471 | 497 |
Gravel 8/15 | 628 | 661 |
Water | 255 | 198 |
Adjuvant | 0 | 5.8 |
Slabs | Average onset of cracking (h) | Average initial crack length (cm) | Average final crack length (cm) | Average crack width (mm) |
CSO | 2.68 | 163.3 | 337.0 | 0.57 |
CSI | 16.53 | 102.7 | 173.0 | 0.20 |
SAO | 2.30 | 116.0 | 120.0 | 0.23 |
SAI | 2.68 | 130.0 | 234.7 | 0.10 |
SCO | 1.21 | 45.0 | 199.0 | 0.24 |
SCI | 2.33 | 244.3 | 266.7 | 0.10 |