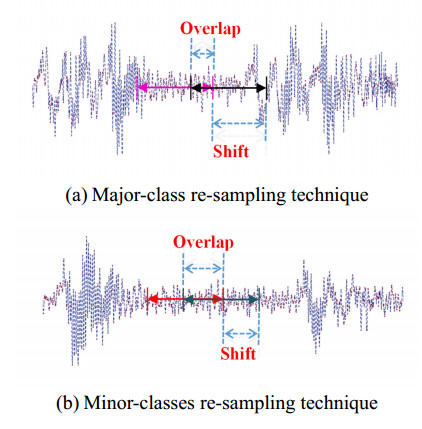
As an indispensable part of large Computer Numerical Control machine tool, rolling bearing faults diagnosis is particularly important. However, due to the imbalanced distribution and partially missing of collected monitoring data, such diagnostic issue generally emerging in manufacturing industry is still hardly to be solved. Thus, a multilevel recovery diagnosis model for rolling bearing faults from imbalanced and partially missing monitoring data is formulated in this paper. Firstly, a regulable resampling plan is designed to handle the imbalanced distribution of data. Secondly, a multilevel recovery scheme is formed to deal with partially missing. Thirdly, an improved sparse autoencoder based multilevel recovery diagnosis model is built to identify the health status of rolling bearings. Finally, the diagnostic performance of the designed model is verified by artificial faults and practical faults tests, respectively.
Citation: Jing Yang, Guo Xie, Yanxi Yang, Qijun Li, Cheng Yang. A multilevel recovery diagnosis model for rolling bearing faults from imbalanced and partially missing monitoring data[J]. Mathematical Biosciences and Engineering, 2023, 20(3): 5223-5242. doi: 10.3934/mbe.2023242
[1] | Yajing Zhou, Xinyu Long, Mingwei Sun, Zengqiang Chen . Bearing fault diagnosis based on Gramian angular field and DenseNet. Mathematical Biosciences and Engineering, 2022, 19(12): 14086-14101. doi: 10.3934/mbe.2022656 |
[2] | Xuyang Xie, Zichun Yang, Lei Zhang, Guoqing Zeng, Xuefeng Wang, Peng Zhang, Guobing Chen . An improved Autogram and MOMEDA method to detect weak compound fault in rolling bearings. Mathematical Biosciences and Engineering, 2022, 19(10): 10424-10444. doi: 10.3934/mbe.2022488 |
[3] | Qiushi Wang, Zhicheng Sun, Yueming Zhu, Chunhe Song, Dong Li . Intelligent fault diagnosis algorithm of rolling bearing based on optimization algorithm fusion convolutional neural network. Mathematical Biosciences and Engineering, 2023, 20(11): 19963-19982. doi: 10.3934/mbe.2023884 |
[4] | Jinyi Tai, Chang Liu, Xing Wu, Jianwei Yang . Bearing fault diagnosis based on wavelet sparse convolutional network and acoustic emission compression signals. Mathematical Biosciences and Engineering, 2022, 19(8): 8057-8080. doi: 10.3934/mbe.2022377 |
[5] | Guanghua Fu, Qingjuan Wei, Yongsheng Yang . Bearing fault diagnosis with parallel CNN and LSTM. Mathematical Biosciences and Engineering, 2024, 21(2): 2385-2406. doi: 10.3934/mbe.2024105 |
[6] | Cong Wang, Chang Liu, Mengliang Liao, Qi Yang . An enhanced diagnosis method for weak fault features of bearing acoustic emission signal based on compressed sensing. Mathematical Biosciences and Engineering, 2021, 18(2): 1670-1688. doi: 10.3934/mbe.2021086 |
[7] | Hao Chen, Shengjie Li, Xi Lu, Qiong Zhang, Jixining Zhu, Jiaxin Lu . Research on bearing fault diagnosis based on a multimodal method. Mathematical Biosciences and Engineering, 2024, 21(12): 7688-7706. doi: 10.3934/mbe.2024338 |
[8] | Zhibo Liu, Yu Yuan, Ling Yu, Yingjie Li, Jiyou Fei . A novel threshold segmentation instantaneous frequency calculation approach for fault diagnosis. Mathematical Biosciences and Engineering, 2020, 17(5): 5395-5413. doi: 10.3934/mbe.2020291 |
[9] | Long Wen, Liang Gao, Yan Dong, Zheng Zhu . A negative correlation ensemble transfer learning method for fault diagnosis based on convolutional neural network. Mathematical Biosciences and Engineering, 2019, 16(5): 3311-3330. doi: 10.3934/mbe.2019165 |
[10] | Yan Yan, Yong Qian, Hongzhong Ma, Changwu Hu . Research on imbalanced data fault diagnosis of on-load tap changers based on IGWO-WELM. Mathematical Biosciences and Engineering, 2023, 20(3): 4877-4895. doi: 10.3934/mbe.2023226 |
As an indispensable part of large Computer Numerical Control machine tool, rolling bearing faults diagnosis is particularly important. However, due to the imbalanced distribution and partially missing of collected monitoring data, such diagnostic issue generally emerging in manufacturing industry is still hardly to be solved. Thus, a multilevel recovery diagnosis model for rolling bearing faults from imbalanced and partially missing monitoring data is formulated in this paper. Firstly, a regulable resampling plan is designed to handle the imbalanced distribution of data. Secondly, a multilevel recovery scheme is formed to deal with partially missing. Thirdly, an improved sparse autoencoder based multilevel recovery diagnosis model is built to identify the health status of rolling bearings. Finally, the diagnostic performance of the designed model is verified by artificial faults and practical faults tests, respectively.
As a typical mechatronic product, large CNC (Computer Numerical Control) machine tools are widely used and the market demand is huge. Consequently, the condition monitoring and performance evaluation of machine tools play a crucial role in enterprise development. Since rolling bearings are the core components of machine tools, identification of their health status is essential. However, device health status displays non-mutability, that is, any failure of rolling bearing is not formed instantaneously. Therefore, it is urgent to achieve bearings health status recognition and fault level estimation accurately and timely [1,2,3,4].
Actually, vibration signals are generated by running rolling bearings, which is useful for fault diagnosis. However, in real industrial application, for the complexity of equipment and disturbance of the environment, these vibration signals often disturbed by noise, showing nonstationarity and nonlinear characteristics [5,6]. In addition, inevitable correlation between components of device raises the diagnostic difficulty [7,8]. Therefore, rolling bearing health status recognition and fault level estimation is always a focus and knotty issue.
For realizing fault diagnosis as well as enhance equipment performance, scholars persevere in seeking solutions and proposing various signal features extraction strategies [9,10,11,12]. Compared with analytical model based processing technology, the diagnosis scheme based on data-driven strategy is more suitable for condition monitoring and performance evaluation of modern large and complex equipment. For example, a diagnostic method based on Local mean decomposition (LMD) and adaptive neuro-fuzzy inference system was designed by Chen et al. [13] for planet wheel failures. By means of K-Nearest Neighbor (KNN), a gearbox fault diagnosis method was formed by Praveen and Saimurugan [14]. In order to achieve the friction impact fault diagnosis, Prosvirin [15] given a solution adopting hybrid feature model and Intrinsic Mode Function (IMF) selection strategy integrated Ensemble Empirical Mode Decomposition (EEMD). A Wavelet Packet Transform (WPT) and manifold learning based fault detection method of rolling bearings was designed by Wang et al. [16]. The well diagnostic results of these studies are inseparable from the subjective experience of designers, which hinders the adaptability as well as generalization capability of the scheme. More seriously, diagnostic performance of these methods drops sharply with the substantial increase of monitoring data.
Fortunately, with the advent of deep learning technology [17,18,19], especially the autoencoder with excellent performance has been widely adopted in many areas [20,21,22]. Actually, autoencoder framework based diagnostic scheme for mechanic faults has formed abundant achievements [23]. Lu et al. [24] proposed a stacked denoising autoencoder-based health state identification method. By constructing a diagnostic network with three hidden layers and adopting a fixed noise level strategy, the fault mode classification of rolling bearing was realized. Sohaib et al. [25] designed a SAE-based fault diagnosis scheme of bearing that needed a hybrid feature pool constructed by handcrafted features as the input of the diagnostic network. The fault mode classification and the severity degree determination were achieved hierarchically. In addition, the diagnostic performance for rolling fault was degraded severely. Sun et al. [26] combined compressed sensing and Sparse Auto-encoder (SAE) to realize rolling bearing fault diagnosis without load fluctuation, and the diagnostic accuracy needs to be further improved. Liu et al. [27] achieved the gearbox fault diagnosis by constructing a stacked autoencoder-based diagnostic network with three hidden layers. However, these known methods expose limitations when handling the large quantity, imbalanced distribution and partially missing data.
At present, the studies based on resampling strategy to solve the problem of data imbalanced distribution have achieved remarkable results. Such as Qian et al. [28] designed a resampling ensemble algorithm for classification of imbalance problems and realized to classify UCI datasets. Cateni et al. [29] proposed a method for resampling imbalanced datasets, which solves the problem of binary classification. For imbalanced credit datasets, Han et al. [30] designed a resampling strategy that solved the binary classification problem of credit scoring effectively.
Therefore, in order to complete such diagnostic challenges and advance the diagnostic accuracy and efficiency, a multilevel recovery diagnosis model for rolling bearing fault from imbalanced and partially missing monitoring data is formulated in this paper. Firstly, to handle the issue caused by data imbalanced distribution, a regulable resampling plan is designed; then, a multilevel recovery scheme is formed to deal with the issue of partially missing; finally, an improved sparse autoencoder based multilevel recovery diagnosis model is built to identify the health status of rolling bearings.
The contribution of this paper is as follows.1) By designing and employing a regulable resampling plan, the adverse effects of data imbalanced distribution on the minor-classes (i.e., faults) diagnosis are tackled. 2) By designing and employing a multilevel recovery scheme and an adaptive loss function, the robustness and diversity of SAE feature learning is improved. 3) The accurate and fast diagnosis of weak and scarce rolling bearing faults is achieved by the proposed fault diagnosis model. 4) The effectiveness and practicability of the proposed method on the rolling bearing real fault diagnosis is verified.
The rest of the study is organized as follows. In Section 2, the sparse auto-encoder is briefly introduced. In Section 3, the designed multilevel recovery diagnosis model for rolling bearing faults from imbalanced and partially missing monitoring data is fully introduced. In Section 4, the diagnostic tests are developed and analyzed. Finally, conclusions are given in Section 5.
Traditional autoencoder is one of the classic networks in deep learning techniques. In practical applications, sparse autoencoder is formed when sparse condition is introduced into autoencoder. Like the general neural network, the sparse autoencoder is composed of input layer, hidden layer and output layer. Particularly, the input and hidden layer modules perform coding operations to effectively extract the feature information contained in the input signals, and then the original input signals are reconstructed from encoded information based on decoding function implemented by the hidden and output layer's modules. Actually, the outputs of hidden layers are the low-dimensional features of the input signals after dimensionality reduction, and based on the optimal weights and biases search, the inputs can be reconstructed as accurately as possible by the outputs.
Firstly, specify {din}Ain=1 as an unlabeled input signal set, where din∈RN×1 is the inth input signal, and A represents the input signal size, while N is the dimension of the input signal. Then, the coding mapping function is specified as fih, and the feature feih of the hidden layer can be calculated by formula (1).
feih=fih(din)=Si(Wihdin+bih) | (1) |
where Si(∙) indicates the sigmoid function, Wih and bih respectively represent the weight matrices and bias vectors of the coding module.
Secondly, the mapping function of decoding module is specified as fho, so reconstitution ˆdin of signal of the input can be achieved by formula (2).
ˆdin=fho(feih)=Whofeih+bho | (2) |
where Who and bho respectively indicate the weight matrices and bias vectors of the decoding module.
The restructure error of autoencoder is commonly achieved based on formula (3).
Jre=12A∑Ain=1‖din−ˆdin‖2 | (3) |
In order to gain valuable features and prevent the output information from mechanically copying the input signals, Kullback-Leibler (KL) divergence function is generally introduced into autoencoder as a sparse condition, resulting in a more practical sparse autoencoder. So the sparse representation of feih is achieved, and the average activation of feih is expressed as formula (4).
ˆρde=1A∑Aih=1fedeih,de=1,2,3,…,D | (4) |
where D is the feature dimension of the hidden layer. Therefore, the sparse condition item is calculated as formula (5) below.
KL(ρ∥ˆρde)=ρlogρˆρde+(1−ρ)1−ρ1−ˆρde | (5) |
where ρ denotes a near-zero parameter for sparsification.
Subsequently, the sparse autoencoder's training process is updated to the solution for the optimization problem in formula (6).
minW,bJre+δ∑Dde=1KL(ρ∥ˆρde) | (6) |
where W=[Wih,Who],b=[bih,bho], δ is a parameter employed to adjust the restructure error and the sparse condition item.
Comprehensively analyzing, those limitations of previous studies by monitoring data mining for rolling bearing's faults are as follows. 1) Compound faults recognition prevalent in bearings are not studied. 2) Adaptability and generalization ability of the designed diagnostic methods need improvement. 3) It is difficult to apply to the diagnostic task with weak fault symptoms. 4) The non-variety of feature extraction hinders the reliability of diagnostic results. 5) Diagnostic challenges caused by imbalanced distribution and partially missing of the collected monitoring data generally are not involved.
Therefore, in order to solve these issues and improve the diagnostic accuracy and efficiency, a multilevel recovery diagnosis model for rolling bearing faults from imbalanced and partially missing monitoring data is formulated and tested in this paper.
To handle the diagnostic challenges of rolling bearing faults from imbalanced and partially missing monitoring data, the innovation points developed in this study is as follows.
Plan Ⅰ: Raw vibration signals pre-processing. For rolling bearing in practical industrial system, the normal vibration signals are easy to access, while abnormal ones are scarce resources. Therefore, considering the balance of different signal characteristics in fault diagnosis, a regulable resampling plan is constructed, and is given in Figure 1. To be specific, a large shift and less/no overlap are adopted for the major-class while a small shift and more overlap for the minor-class. Consequently, Figure 1(a) is the re-sampling plan designed for the normal vibration signals, while Figure 1(b) is the one for the abnormal vibration signals. By adopting this resampling plan, the issue caused by imbalanced distribution of data is solved, and a balanced diagnostic sample set of rolling bearings is simultaneously acquired.
The original data sequence is defined as X=[1,2,i,⋯,n], where i represents the data point constituting the data sequence X, and n indicates the total number of data points. Then, the sample set S achieved by the proposed resampling scheme is given in formula (7),
S=[s1,s2,sj,⋯,sm] | (7) |
where sj represents the sample in the sample set S, j=1,2,⋯,m, and m is the sample size. Specifically, sj is calculated by formula (8),
{sj=[1,2,⋯,l],j=1sj=[(1+(y−1)∗(j−1)),⋯,(y−1)∗(j−1)+l],j>1 | (8) |
where l is the length of a sample, that is, the number of data points constituting each sample, and y represents the shift size.
Plan Ⅱ: Multilevel recovery diagnosis model building. To handle the issue of partially missing, a multilevel recovery scheme is formed, and subsequently a modified sparse auto-encoder based multilevel recovery diagnosis model is built to identify the health status of rolling bearings. Specifically, multiple levels of noise are mixed into each diagnostic sample of rolling bearings, and the noised samples are got. Subsequently, the diagnosis model performs feature extraction and signal classification on the noised samples.
Define the multiple levels of noise order is {n0,n1,…,ni,…,nE}, where n0 denote the first noise grade, ni is the ith noise grade, and nE is the last noise grade, i=0,1,2,…,E, and n0>n1>⋯>nE≥0. Then, every noise level is successively employed to train the diagnostic model. In fact, with such training strategy, the general characteristics of the diagnostic signals would be mined first, while the local characteristics of the samples are mined one by one. To be specific, given the pre-processed input sample as dini, and then based on the uniform distribution Qu with different probability Pi, the random masking noise is injected into dini, so as to get the noised sample ˆdini Qu(ˆdini|dini,Pi). Thus, this signal processing plan is actually correspond to injecting a "blank" data points with probability Pi into dini, and let the amount of information in dini is reduced. Finally, for each noise level, the reconstructed sample −dini is acquired based on encoding and decoding formulas (9) and (10), respectively.
feihi=fih(ˆdini)=fR(Wihˆdini+bih) | (9) |
−dini=fR(Whofeihi+bho) | (10) |
where fR(∙) is ReLU, while {Wih,Who},{bih,bho} are the weights and biases of coding and decoding modules respectively.
This designed multilevel recovery diagnosis model attempts to populate the information at different noise level ni, and then based on mining ˆdini, the data structure of dini is finally learned. Such a processing strategy not only mines the characteristics of the original signal dini, but also improves the robustness of feature learning.
Plan Ⅲ: Weights conditions forming. For enriching feature information mining and highlighting the most discriminative features, weights conditions as shown in formulas (11)–(13) are formed.
Who=(Wih)' | (11) |
Wih=2∗F(a,b)∗Rn(a,b)−F(a,b) | (12) |
‖Wih‖=∑Dimx=1√∑My=1W2xy | (13) |
where a and b are the sizes of intput layer and hidden layer, respectively. F(⋅)=√6/a+b represents a defined function, and Rn(∙)=rand(∙) is a random function. Further, Dim is the input sample dimension, while M denotes the feature dimension.
Plan Ⅳ: Sparse condition item improving. Considering the efficiency of network training, the sparse condition item in formula (5) is improved as follows.
∑Aih‖feihi‖1=∑Aih∑Jj|feihj| | (14) |
Finally, the designed improved cost-function of the multilevel recovery diagnosis model developed in the study is given as formula (15).
{minWih,Who,bih,bho12A∑Ain=1‖din−ˆdin‖2+δ∑Aih‖feihi‖1+γ∑Ll−1l=1∑nlx=1∑nl+1y=1(W(l)yx)2+μ‖Wih‖Who=(Wih)'Wih=2∗F(a,b)∗Rn(a,a)−F(a,b) | (15) |
Next, after employing the well-trained improved sparse autoencoder based multilevel recovery network to accomplish feature mining, softmax module is determined as the output module of the designed model to complete the diagnostic missions.
To sum up, the realization of health status identification and fault level classification for rolling bearing by adopting the improved sparse autoencoder based multilevel recovery model developed in this study is shown in Figure 2.
Actually, the fine diagnostic outcomes hardly to be obtained without reasonable model hyper-parameters. Therefore, the optimal hyper-parameters of the designed multilevel recovery diagnosis model are found by the way of tests in this study. It is worth noting that, these optimal hyper-parameters can be directly adopted for other diagnostic tasks. To be specific, taking the deep groove ball bearing with ten health conditions (i.e., normal and nine faults) as an example, the vibration monitoring signals of it are adopted and then developed fifteen times tests. In addition, the normal and fault data are skewed, thus, the regulable resampling plan is used to get the balanced diagnostic sample set.
Generally, in deep networks, the hidden nodes follows the principle that the size of the later layer is less than or equal to the size of the previous one. In this study, the optimal hidden nodes size of the proposed multilevel recovery diagnosis model is displayed in Figure 3. Specifically, the red lines mark the accuracy range of the fifteen times tests. Comprehensive accuracy, stability and time consumption, the optimal hidden nodes sizes of the previous layer and the latter one are identified as 200 and 100 respectively, as circled by the black dotted box in the figure.
Actually, the training/testing sample sizes are really affect the diagnosis accuracy in deep network, therefore, the tests are carried out to search the optimal training/testing sample size of the proposed multilevel recovery diagnosis model, the outcomes are given in Figure 4. The green lines mark the accuracy range of the fifteen times tests. Comprehensive accuracy, stability and over-fitting issue, the optimal training/testing sample sizes of the designed model are identified as 6/4, as circled by the blue dotted box in the figure.
In formula (13), the cost-function weight terms optimization of the developed multilevel recovery diagnosis model is introduced as follows.
1) Weight term γ
As is known to all, the weight regularization item in classic deep network is an adequate response to over-fitting issue, however, unsuitable weight item γ for this regularization item would hinder the feature extraction ability of the model. Thus, the tests are developed to seek the optimal weight item γ of the proposed multilevel recovery diagnosis model, the results are given in Figure 5. Apparently, the optimal γ is 3e−2, as marked by the red dotted box in the figure.
2) Weight term δ
For an autoencoder network, the sparse condition is introduced to prevent the outcome from mechanically reproducing the input, so as to enhance the performance of feature extraction. However, the reasonable weight item δ for this condition in cost-function of the model is essential to mine data. So, the tests are produced to find the optimal weight item δ of the proposed multilevel recovery diagnosis model, the results are shown in Figure 6. Evidently, the optimal δ is 3, as circled by the green dotted box in the figure.
3) Weight term μ
In the designed cost-function of the proposed model, for the most distinctive features acquisition, the weight condition of the weight matrices in coding module is introduced. In fact, it is necessary to search the appropriate weight item μ for this condition. Therefore, the tests are carried out to get the optimal weight item μ of the proposed multilevel recovery diagnosis model, as is shown in Figure 7. Comprehensive accuracy and stability, the optimal μ is identified as 3e-2, as tagged by the yellow dotted box in the figure.
In Test1, the datasets provided by Case Western Reserve University (CWRU) data center [31] are employed to develop the validation experiments. Specifically, 1) the sampling frequency of original signals at motor driving end is 12 kHz. 2) The health states of bearing are normal state (N), rolling element failure (REF), inner ring failure (IRF) and outer ring failure (ORF), respectively. 3) The fault levels arranged by the Electro-discharge Machining (EDM) technology of bearing are respectively 0.18, 0.36 and 0.53 mm. 4) Data in normal state are regarded as major-class, and the ones in failure states are regarded as minor-classes. A resampling plan with 2048 and 128 is adopted for diagnostic data in 4) to get the balanced training sample set. Detailed description for this test is shown in Table 1.
Load (hp) | Sample Size | Health Status | Fault Level |
3 | 200 | N | 0 |
200 | IRF1 | 0.18 mm | |
200 | IRF2 | 0.36 mm | |
200 | IRF3 | 0.53 mm | |
200 | ORF1 | 0.18 mm | |
200 | ORF2 | 0.36 mm | |
200 | ORF3 | 0.53 mm | |
200 | REF1 | 0.18 mm | |
200 | REF2 | 0.36 mm | |
200 | REF3 | 0.53 mm |
The hyper-parameters of the proposed diagnostic model are as follows. 1) The number of neurons in input layer and output layer is 1200 and 10, respectively. 2) The test sample set is formed based on data interception technology. 3) The first noise grade and the last noise grade are set to be 0.5 and 0.05, respectively. The noise grade decrease step size is set to be 0.05. 4) Random masking noise is added to 20% of the elements for the selected samples. 5) The weight matrices of two hidden layers are respectively initialized by formulas (9) and (10). 6) The bias vectors are initialized to be zeros. It is worth noting that the training set and the test set in this test are not identically distributed.
Considering the stability and generality of the test results, the diagnostic sample set in Table 1 is tested for ten times continuously. In addition, for robustness verification, sixty percent of data in the test sample set are randomly used to diagnose in every trial, and the trials accuracy is shown in Figure 8. It displays that the designed diagnostic scheme developed in this study can achieve the artificial fault diagnosis of loaded rolling bearing, with an average accuracy over 99.2%.
The statistical correctly identified sample sizes of each health state for ten trials are given in Figure 9. It can be seen that the correctly identified sample sizes of IRF3 and ORF2 are slightly smaller. Furthermore, all samples of bearing in normal state are correctly identified, and none of the faulty samples are misdiagnosed as normal ones. In fact, with the load interference, the improved sparse autoencoder based multilevel recovery model developed in this study has no misdiagnosis except a small amount of missed diagnosis in ten trials.
Further, the average recognition accuracy of each health state in Figure 9 is computed and listed in Table 2. It shows that the test results of each health state are different. Actually, since the physical structure of bearing determines the complexity of different faults, the test results of each health state are hardly to be equal. However, the trial accuracy of the designed multilevel recovery model for loaded rolling bearing artificial fault diagnosis is not less than 98.045%.
Health state | Average test accuracy (%) |
N | 100.000 |
IRF1 | 98.879 |
IRF2 | 100.000 |
IRF3 | 98.241 |
ORF1 | 100.000 |
ORF2 | 98.045 |
ORF3 | 100.000 |
REF1 | 100.000 |
REF2 | 98.866 |
REF3 | 98.440 |
Next, for revealing the specifics of incorrectly identified samples, the classification confusion matrix of ten trials is produced and displayed in Figure 10. Specifically, the numbers on the diagonal show the correctly identified sample size, while the numbers outside the diagonal give the incorrectly identified ones. It can be seen that the error identification mainly emerges between IRF1 and REF2, IRF3 and ORF2. Further, REF3 is wrongly diagnosed as REF2. Actually, the matrix again verifies that the designed method does not have the issue of missed diagnosis when diagnosing artificial faults of rolling bearing.
Finally, the test performance criteria of the designed model are shown in Table 3. Specifically, the average test accuracy exceeds to 99.2%, the time for model training is lower than 22s, while the time for final recognition is not greater than 17 ms. Therefore, although with the unbalanced sample data structure, the improved sparse autoencoder based multilevel recovery model developed in this study can accurately and quickly identify the artificial faults of rolling bearing and determine severity level at the same time.
Average accuracy | Training time | Recognition time |
99.250% | 21.897s | 16.300ms |
In order to understand the diagnostic performance of the proposed multilevel recovery model, the comparative tests are developed by means of unchanged diagnostic set in Table 1. Considering the stability of diagnosis, ten consecutive trials are performed, and the average results are shown in Table 4. It can be seen that the deep framework -based schemes possess the better diagnostic performance than the shallow Artificial Neural Network (ANN)-based structure in handling mass data samples. Further, the empirical-based feature parameters selection and then feature extraction conducted restricts the performance of the diagnostic schemes formed in [32] and [33], and three hidden layers based plan designed in [34] increases the model training time. Moreover, none of these schemes considered the issue of the data imbalanced and partially missing. Distinctively, the model developed in this study solves the above problems well, and then accurately and quickly realizes artificial fault identification and severity level determination.
Method | ANN-based | EEMD+AR+SAE [32] | LMD+RWSVM [33] | SAE-based [34] | The proposed method |
Average accuracy (%) | 59.963 | 94.513 | 91.615 | 95.813 | 99.250 |
Average training time (s) | 20.116 | 147.078 | 54.510 | 37.913 | 21.897 |
Average recognition time (ms) | 16.547 | 39.667 | 28.343 | 31.025 | 16.300 |
AR: Autoregressive; SAE: Sparse autoencoder; RWSVM: Reproducing wavelet support vector machines. |
In this test, the dataset shared by Padborn University [35] is employed to promote practical fault diagnosis of rolling bearings. The faulty data of bearings in this dataset are collected based on accelerated lifetime tests. Specifically, 1) the sampling frequency of vibration data is 64 kHz. 2) Health statuses of these bearings respectively are normal state, IRF and ORF. 3) Failure modes are single point (SP), repetitive (R) and multiple (M), respectively. 4) Failure levels are divided into 1, 2 and 3. 5) The normal bearing's signal is regarded as the major-class, and the ones of failure bearings are regarded as minor-classes. A resampling plan with 5120 and 256 is adopted for diagnostic data in 5) to get the balanced training sample set. In addition, each health condition contains 100 samples, and each sample is composed of 2560 data points. Consequently, the sample set contains 1300 samples. Detailed description of this dataset is shown in Table 5.
No. | Damage | Health status | Fault mode | Fault form | Level |
01 | - | - | - | - | 0 |
02 | Pi | ORF | SP | SP | 1 |
03 | In | ORF | SP | SP | 1 |
04 | Pi | ORF | R | SP | 2 |
05 | In | ORF | R | Distributed | 1 |
06 | Pi | IRF | M | SP | 1 |
07 | Pi | IRF | SP | SP | 3 |
08 | Pi | IRF | R | SP | 1 |
09 | Pi | IRF | SP | SP | 2 |
10 | Pi | IRF | SP | SP | 1 |
11 | Pi | IRF+(ORF) | M | SP | 2 |
12 | Pi | IRF+(ORF) | M | Distributed | 3 |
13 | In | ORF+IRF | M | Distributed | 1 |
Note: Pi: Pitting; In: Indentations. |
Apparently, the failure modes are diverse, and this dataset even contains a variety of composite faults. Thus, compared with Test1, this test for practical fault diagnosis is more complex and difficult.
The hyper-parameters of the proposed diagnostic model are as follows. 1) The number of neurons in input layer and output layer is 2560 and 13, respectively. 2) The test sample set is formed based on data interception technology. 3) The configuration of the remaining hyper-parameters is still the same as that in Test1, so as to highlight the excellent generalization performance of the designed model. Then, considering the stability and generality of the test results, the diagnostic sample set in Table 5 is tested for ten times continuously. In addition, for robustness verification, sixty percent of data in the test sample set are randomly used to diagnose in every trial, and the results are given as Figure 11. It displays that designed diagnostic scheme developed in this study can achieve the practical fault diagnosis of loaded rolling bearings, with average-accuracy over 99.4%.
The statistical correctly identified sample sizes of each rolling bearing for ten trials are given in Figure 12. It can be seen that the correctly identified sample sizes of 03 are a bit less than the original ones. Furthermore, the correctly identified size of other ones is pretty close to or equal to the real size. Remarkably, all samples of bearing in normal state are correctly identified, and none of bearings in failure state is misdiagnosed as normal ones. In fact, with the load interference, the improved sparse autoencoder based multilevel recovery model developed in this study has no misdiagnosis except a small amount of missed diagnosis for practical fault diagnosis.
Further, the average recognition accuracy of each bearing in Figure 12 is computed and listed in Table 6. It shows that the test results of each bearing are different. Actually, since the differences in health status, the test results of each bearing are hardly to be equal. However, the trial accuracy of the designed multilevel recovery model for loaded rolling bearing practical fault diagnosis is still above 98.330%.
No. | Average diagnostic accuracy (%) |
01 | 100.000 |
02 | 100.000 |
03 | 98.330 |
04 | 99.500 |
05 | 99.063 |
06 | 100.000 |
07 | 99.316 |
08 | 100.000 |
09 | 98.972 |
10 | 99.737 |
11 | 100.000 |
12 | 98.783 |
13 | 99.778 |
Next, for revealing the specifics of incorrectly identified samples, the classification confusion matrix of ten trials is produced and displayed in Figure 13. Specifically, the numbers on the diagonal show the correctly identified sample size of each bearing, while the numbers outside the diagonal give the incorrectly identified ones. With the existence of load as well as the diversity and coupling of failure modes, the error identification mainly emerges between 03 and 05, as well as between the single-failure and its correlative multiple ones. Actually, the matrix again verifies that the designed method does not have the issue of missed diagnosis when diagnosing practical faults of rolling bearings, highlighting the well recognition performance and practicability of this designed model in actual industry sector.
Finally, the test performance criteria of the designed model are shown in Table 7. Specifically, the average test result is exceeds 99.4%, the time for model training is lower than 38s, and the time for final recognition is not greater than 19.9ms. Therefore, even with the unbalanced sample data structure, the improved sparse autoencoder based multilevel recovery model developed in this study can accurately and quickly identify the practical faults of rolling bearings and determine severity level at the same time.
Average accuracy | Training time | Recognition time |
99.481% | 37.646s | 19.900ms |
For surveying the performance of the proposed multilevel recovery model to practical faults diagnosis, the comparative tests are formulated based on the same diagnostic sample set in Table 5. Taking reliability into account, ten consecutive trials are performed, and the average results are shown in Table 8. Apparently, compared with Table 4, only the diagnostic accuracy of the method designed in this study does not reduce for practical faults, while the accuracy of other methods decreases significantly. To be specific, for the reason of ignoring the issue of unbalanced monitoring data structure, the shallow ANN-based structure hardly discriminated the practical faults of rolling bearings. In addition, determination of feature parameters in [32] and [33] was based on artificial fault properties, which hindered the diagnostic performance of these methods to practical faults. Since the diagnostic scheme established in [34] based on the deep framework, the diagnostic effect of this method is relatively better. In contrast, with the influence of load and unbalanced monitoring data, the designed model can handle practical fault diagnosis issues and show excellent diagnostic performance.
Method | ANN-based | EEMD+AR+SAE [32] | LMD+RWSVM [33] | SAE-based [34] | The proposed method |
Average accuracy (%) | 57.961 | 93.846 | 90.173 | 94.423 | 99.481 |
Objectively, differences are existed between the practical faults and artificial faults. Considering the effectiveness and practicability of diagnostic method, it is more meaningful to be able to realize the practical fault modes identification and the failure level determination with excellent performance.
In this study, a multilevel recovery diagnosis model for rolling bearing faults from imbalanced and partially missing monitoring data is formulated, which completed the scarce multiple complex faults of rolling bearings with excellent performance. The test outputs were exhibited that this designed model significantly outperforms the other competing techniques on artificial and practical fault data sets. The findings and innovative points of this study are as follows. 1) The designed regulable resampling plan effectively handled the adverse effects of data imbalanced distribution on the minor-classes. 2) The multilevel recovery scheme made a outstanding contribution to partially missing data. 3) The developed loss function improved the robustness and diversity of SAE feature learning. 4) The proposed diagnosis model displayed excellent performance and provided reference for the solution of other fault diagnosis issues.
Furthermore, for rolling bearing faults diagnosis, the effectiveness of the diagnosis of the severe data imbalance and the overall missing data remains to be confirmed, and will be a focus of our future work. In addition, a diagnosis platform is considered to be developed for the practical engineering applications.
This work is supported by the National Natural Science Foundation of China (No.62063032) and the Natural Science Foundation of Gansu Province (No. 21JR1RE296).
The authors declare that they have no known competing financial interests or personal relationships that could have appeared to influence the work reported in this paper.
[1] |
P. Yang, Z. Li, Y. Yu, J. Shi, M. Sun, Studies on fault diagnosis of dissolved oxygen sensor based on GA-SVM, Math. Biosci. Eng., 18 (2021), 386–399. https://doi.org/10.3934/mbe.2021021 doi: 10.3934/mbe.2021021
![]() |
[2] |
J. Yang, G. Xie, Y. Yang, X. Li, L. Mu, S. Takahashi, H. Mochizuki, An improved deep network for intelligent diagnosis of machinery faults with similar features, IEEJ, 14 (2019), 1851–1864. https://doi.org/10.1002/tee.23012 doi: 10.1002/tee.23012
![]() |
[3] |
G. Xie, J. Yang, Y. Yang, An improved sparse autoencoder and multi-level denoising strategy for diagnosing early multiple intermittent faults, IEEE Trans. Syst. Man Cybern.: Syst., 52 (2022), 869–880. https://doi.org/10.1109/TSMC.2020.3005433 doi: 10.1109/TSMC.2020.3005433
![]() |
[4] |
Y. Zhou, A. Kumar, C. Parkash, G. Vashishtha, H. Tang, J. Xiang, A novel entropy-based sparsity measure for prognosis of bearing defects and development of a sparsogram to select sensitive filtering band of an axial piston pump, Measurement, 203 (2022), 111997. https://doi.org/10.1016/j.measurement.2022.111997 doi: 10.1016/j.measurement.2022.111997
![]() |
[5] |
N. Xu, G. Zhang, L. Yang, Z. Shen, M. Xu, L. Chang, Research on thermoeconomic fault diagnosis for marine low speed two stroke diesel engine, Math. Biosci. Eng., 19 (2022), 5393–5408. https://doi.org/10.3934/mbe.2022253 doi: 10.3934/mbe.2022253
![]() |
[6] |
J. Yang, G. Xie, Y. Yang, A key-factor denoising strategy for quasi periodic non-stationary incipient faults diagnosis, Measurement, 197 (2022), 111304. https://doi.org/10.1016/j.measurement.2022.111304 doi: 10.1016/j.measurement.2022.111304
![]() |
[7] |
J. Yang, G. Xie, Y. Yang, Y. Zhang, W. Liu, Deep model integrated with data correlation analysis for multiple intermittent faults diagnosis, ISA Trans., 95 (2019), 306–319. https://doi.org/10.1016/j.isatra.2019.05.021 doi: 10.1016/j.isatra.2019.05.021
![]() |
[8] |
J. Yang, Y. Yang, G. Xie, Diagnosis of incipient fault based on sliding-scale resampling strategy and improved deep autoencoder, IEEE Sens. J., 20 (2020), 8336–8348. https://doi.org/10.1109/JSEN.2020.2976523 doi: 10.1109/JSEN.2020.2976523
![]() |
[9] |
Y. Wang, D. Zhao, Y. Li, S. X. Ding, Unbiased minimum variance fault and state estimation for linear discrete time-varying two-dimensional systems, IEEE Trans. Autom. Control, 62 (2017), 5463–5469. https://doi.org/10.1109/TAC.2017.2697210 doi: 10.1109/TAC.2017.2697210
![]() |
[10] |
R. Sun, Y. Han, Y. Wang, Design of generalized fault diagnosis observer and active adaptive fault tolerant controller for aircraft control system, Math. Biosci. Eng., 19 (2022), 5591–5609. https://doi.org/10.3934/mbe.2022262 doi: 10.3934/mbe.2022262
![]() |
[11] |
R. Zhao, R. Yan, Z. Chen, K. Mao, P. Wang, R. X. Gao, Deep learning and its applications to machine health monitoring, Mech. Syst. Signal Process., 115 (2019), 213–237. https://doi.org/10.1016/j.ymssp.2018.05.050 doi: 10.1016/j.ymssp.2018.05.050
![]() |
[12] |
W. Li, X. Zhong, H. Shao, B. Cai, X. Yang, Multi-mode data augmentation and fault diagnosis of rotating machinery using modified ACGAN designed with new framework, Adv. Eng. Inf., 52 (2022), 101552. https://doi.org/10.1016/j.aei.2022.101552 doi: 10.1016/j.aei.2022.101552
![]() |
[13] |
X. Chen, G. Cheng, H. Li, M. Zhang, Diagnosing planetary gear faults using the fuzzy entropy of LMD and ANFIS, J. Mech. Sci. Technol., 30 (2016), 2453–2462. https://doi.org/10.1007/s12206-016-0505-y doi: 10.1007/s12206-016-0505-y
![]() |
[14] |
M. R. Praveen, M. Saimurugan, Health monitoring of a gear box using vibration signal analysis, Appl. Mech. Mater., 813–814 (2015), 1012–1017. https://doi.org/10.4028/www.scientific.net/AMM.813-814.1012 doi: 10.4028/www.scientific.net/AMM.813-814.1012
![]() |
[15] |
A. E. Prosvirin, M. Islam, J. Kim, J. Kim, Rub-impact fault diagnosis using an effective IMF selection technique in ensemble empirical mode decomposition and hybrid feature models, Sensors, 18 (2018), 2040. https://doi.org/10.3390/s18072040 doi: 10.3390/s18072040
![]() |
[16] |
Y. Wang, G. Xu, L. Liang, K. Jiang, Detection of weak transient signals based on wavelet packet transform and manifold learning for rolling element bearing fault diagnosis, Mech. Syst. Signal Process., 54–55 (2015), 259–276. https://doi.org/10.1016/j.ymssp.2014.09.002 doi: 10.1016/j.ymssp.2014.09.002
![]() |
[17] |
Y. LeCun, Y. Bengio, G. Hinton, Deep learning, Nature, 521 (2015), 436–444. https://doi.org/10.1038/nature14539 doi: 10.1038/nature14539
![]() |
[18] |
Y. Zhou, G. Zhi, W. Chen, Q. Qian, D. He, B. Sun, et. al., A new tool wear condition monitoring method based on deep learning under small samples, Measurement, 189 (2022), 110622. https://doi.org/10.1016/j.measurement.2021.110622 doi: 10.1016/j.measurement.2021.110622
![]() |
[19] |
S. Jia, Z. Yu, A. Onken, Y. Tian, T. Huang, J. K. Liu, Neural system identification with spike-triggered non-negative matrix factorization, IEEE Trans. Cybern., 52 (2022), 4772–4783. https://doi.org/10.1109/TCYB.2020.3042513 doi: 10.1109/TCYB.2020.3042513
![]() |
[20] |
J. Yang, Y. Bai, G. Li, M. Liu, X. Liu, A novel method of diagnosing premature ventricular contraction based on sparse auto-encoder and softmax regression, Bio.-Med. Mater. Eng., 26 (2015), 1549–1558. https://doi.org/10.3233/BME-151454 doi: 10.3233/BME-151454
![]() |
[21] | J. Deng, Z. Zhang, E. Marchi, B. Schuller, Sparse autoencoder-based feature transfer learning for speech emotion recognition, in 2013 Humaine Association Conference on Affective Computing and Intelligent Interaction, (2013), 511–516. https://doi.org/10.1109/ACⅡ.2013.90 |
[22] |
J. Yang, G. Xie, Yanxi Yang, An improved ensemble fusion autoencoder model for fault diagnosis from imbalanced and incomplete data, Control Eng. Pract., 98 (2020), 104358. https://doi.org/10.1016/j.conengprac.2020.104358 doi: 10.1016/j.conengprac.2020.104358
![]() |
[23] |
R. Liu, B. Yang, E. Zio, X. Chen, Artificial intelligence for fault diagnosis of rotating machinery: A review, Mech. Syst. Signal Process., 108 (2018), 33–47. https://doi.org/10.1016/j.ymssp.2018.02.016 doi: 10.1016/j.ymssp.2018.02.016
![]() |
[24] |
C. Lu, Z. Wang, W. Qin, J. Ma, Fault diagnosis of rotary machinery components using a stacked denoising autoencoder-based health state identification, Signal Process., 130 (2017), 377–388. https://doi.org/10.1016/j.sigpro.2016.07.028 doi: 10.1016/j.sigpro.2016.07.028
![]() |
[25] |
M. Sohaib, C. Kim, J. Kim, A hybrid feature model and deep-learning-based bearing fault diagnosis, Sensors, 17 (2017), 2876–2891. https://doi.org/10.3390/s17122876 doi: 10.3390/s17122876
![]() |
[26] |
J. Sun, C. Yan, J. Wen, Intelligent bearing fault diagnosis method combining compressed data acquisition and deep learning, IEEE Trans. Instrum. Meas., 67 (2018), 185–195. https://doi.org/10.1109/TIM.2017.2759418 doi: 10.1109/TIM.2017.2759418
![]() |
[27] |
G. Liu, H. Bao, B. Han, A stacked autoencoder-based deep neural network for achieving gearbox fault diagnosis, Math. Probl. Eng., 2018 (2018), 1–10. https://doi.org/10.1155/2018/5105709 doi: 10.1155/2018/5105709
![]() |
[28] |
Y. Qian, Y. Liang, M. Li, G. Feng, X. Shi, A resampling ensemble algorithm for classification of imbalance problems, Neurocomputing, 143 (2014), 57–67. https://doi.org/10.1016/j.neucom.2014.06.021 doi: 10.1016/j.neucom.2014.06.021
![]() |
[29] |
S. Cateni, V. Colla, M. Vannucci, A method for resampling imbalanced datasets in binary classification tasks for real-world problems, Neurocomputing, 135 (2014), 32–41. https://doi.org/10.1016/j.neucom.2013.05.059 doi: 10.1016/j.neucom.2013.05.059
![]() |
[30] |
X. Han, R. Cui, Y. Lan, Y. Kang, J. Deng, N. Jia, A Gaussian mixture model based combined resampling algorithm for classification of imbalanced credit data sets, Int. J. Mach. Learn. Cybern., 10 (2019), 3687–3699. https://doi.org/10.1007/s13042-019-00953-2 doi: 10.1007/s13042-019-00953-2
![]() |
[31] | K. Loparo, Case western reserve university bearing data center, 2013. Available from: http://csegroups.case.edu/bearingdatacenter/pages. |
[32] |
Y. Qi, C. Shen, D. Wang, J. Shi, X. Jiang, Z. Zhu, Stacked sparse autoencoder-based deep network for fault diagnosis of rotating machinery, IEEE Access, 5 (2017), 15066–15079. https://doi.org/10.1109/ACCESS.2017.2728010 doi: 10.1109/ACCESS.2017.2728010
![]() |
[33] |
Z. Liu, X. Chen, Z. He, Z. Shen, LMD method and multi-class RWSVM of fault diagnosis for rotating machinery using condition monitoring information, Sensors, 13 (2013), 8679–8694. https://doi.org/10.3390/s130708679 doi: 10.3390/s130708679
![]() |
[34] |
F. Zhou, Y. Gao, C. Wen, A novel multimode fault classification method based on deep learning, J. Control Sci. Eng., 2017 (2017), 1–14. https://doi.org/10.1155/2017/3583610 doi: 10.1155/2017/3583610
![]() |
[35] | C. Lessmeier, J. K. Kimotho, D. Zimmer, W. Sextro, Condition monitoring of bearing damage in electromechanical drive systems by using motor current signals of electric motors: A benchmark data set for data-driven classification, in 2016 European Conference of the Prognostics and Health Management Society, (2016), 1–18. |
1. | Lintao Li, Hongkai Jiang, Ruixin Wang, Qiao Yang, A reinforcement neural architecture search convolutional neural network for rolling bearing fault diagnosis, 2023, 34, 0957-0233, 115122, 10.1088/1361-6501/acec06 | |
2. | Bin Chen, Yang Zhao, Yuteng Zhang, Yuyan Jiang, Hongliang Zhang, Haiyang Pan, Analysis of hot spots and trends in rolling bearing fault diagnosis research based on scientific knowledge mapping, 2024, 6, 2631-8695, 025536, 10.1088/2631-8695/ad4847 | |
3. | Heng Wang, Peng Wang, Siyuan Wang, Danqing Li, Rolling bearing fault diagnosis method based on dynamic simulated model from source domain to target domain with improved alternating transfer learning, 2024, 0924-090X, 10.1007/s11071-024-10310-w | |
4. | Jing Yang, Jianwen Chen, Xuan Zhan, Chuanshui Liu, Cheng Yang, A feature reconstruction and SAE model based diagnosis method for multiple mixed faults, 2024, 35, 0957-0233, 086130, 10.1088/1361-6501/ad4c8b | |
5. | Jing Yang, Jianwen Chen, Chuanshui Liu, Xuan Zhan, Cheng Yang, Guo Xie, 2024, Bispectrum analysis and Convolutional Neural Network Based Feature Extraction and Classification, 979-8-3503-8778-0, 3931, 10.1109/CCDC62350.2024.10587814 | |
6. | Jing Yang, Yanping Bai, Yunyun Cheng, Rong Cheng, Wendong Zhang, Guojun Zhang, A new model for bearing fault diagnosis based on optimized variational mode decomposition correlation coefficient weight threshold denoising and entropy feature fusion, 2023, 111, 0924-090X, 17337, 10.1007/s11071-023-08728-9 | |
7. | Jing Yang, Yanping Bai, Xiuhui Tan, Rong Cheng, Hongping Hu, Peng Wang, Wendong Zhang, A new model for bearing fault diagnosis based on mutual mapping of signals and images and sparse representation, 2024, 35, 0957-0233, 046122, 10.1088/1361-6501/ad1d4a | |
8. | Chuanshui Liu, Jing Yang, Xuan Zhan, Jianwen Chen, Xihui Liang, Application of Bispectral Technology and Deep Learning for Feature Extraction and Classification, 2025, 2025, 1070-9622, 10.1155/vib/8119232 |
Load (hp) | Sample Size | Health Status | Fault Level |
3 | 200 | N | 0 |
200 | IRF1 | 0.18 mm | |
200 | IRF2 | 0.36 mm | |
200 | IRF3 | 0.53 mm | |
200 | ORF1 | 0.18 mm | |
200 | ORF2 | 0.36 mm | |
200 | ORF3 | 0.53 mm | |
200 | REF1 | 0.18 mm | |
200 | REF2 | 0.36 mm | |
200 | REF3 | 0.53 mm |
Health state | Average test accuracy (%) |
N | 100.000 |
IRF1 | 98.879 |
IRF2 | 100.000 |
IRF3 | 98.241 |
ORF1 | 100.000 |
ORF2 | 98.045 |
ORF3 | 100.000 |
REF1 | 100.000 |
REF2 | 98.866 |
REF3 | 98.440 |
Average accuracy | Training time | Recognition time |
99.250% | 21.897s | 16.300ms |
Method | ANN-based | EEMD+AR+SAE [32] | LMD+RWSVM [33] | SAE-based [34] | The proposed method |
Average accuracy (%) | 59.963 | 94.513 | 91.615 | 95.813 | 99.250 |
Average training time (s) | 20.116 | 147.078 | 54.510 | 37.913 | 21.897 |
Average recognition time (ms) | 16.547 | 39.667 | 28.343 | 31.025 | 16.300 |
AR: Autoregressive; SAE: Sparse autoencoder; RWSVM: Reproducing wavelet support vector machines. |
No. | Damage | Health status | Fault mode | Fault form | Level |
01 | - | - | - | - | 0 |
02 | Pi | ORF | SP | SP | 1 |
03 | In | ORF | SP | SP | 1 |
04 | Pi | ORF | R | SP | 2 |
05 | In | ORF | R | Distributed | 1 |
06 | Pi | IRF | M | SP | 1 |
07 | Pi | IRF | SP | SP | 3 |
08 | Pi | IRF | R | SP | 1 |
09 | Pi | IRF | SP | SP | 2 |
10 | Pi | IRF | SP | SP | 1 |
11 | Pi | IRF+(ORF) | M | SP | 2 |
12 | Pi | IRF+(ORF) | M | Distributed | 3 |
13 | In | ORF+IRF | M | Distributed | 1 |
Note: Pi: Pitting; In: Indentations. |
No. | Average diagnostic accuracy (%) |
01 | 100.000 |
02 | 100.000 |
03 | 98.330 |
04 | 99.500 |
05 | 99.063 |
06 | 100.000 |
07 | 99.316 |
08 | 100.000 |
09 | 98.972 |
10 | 99.737 |
11 | 100.000 |
12 | 98.783 |
13 | 99.778 |
Average accuracy | Training time | Recognition time |
99.481% | 37.646s | 19.900ms |
Load (hp) | Sample Size | Health Status | Fault Level |
3 | 200 | N | 0 |
200 | IRF1 | 0.18 mm | |
200 | IRF2 | 0.36 mm | |
200 | IRF3 | 0.53 mm | |
200 | ORF1 | 0.18 mm | |
200 | ORF2 | 0.36 mm | |
200 | ORF3 | 0.53 mm | |
200 | REF1 | 0.18 mm | |
200 | REF2 | 0.36 mm | |
200 | REF3 | 0.53 mm |
Health state | Average test accuracy (%) |
N | 100.000 |
IRF1 | 98.879 |
IRF2 | 100.000 |
IRF3 | 98.241 |
ORF1 | 100.000 |
ORF2 | 98.045 |
ORF3 | 100.000 |
REF1 | 100.000 |
REF2 | 98.866 |
REF3 | 98.440 |
Average accuracy | Training time | Recognition time |
99.250% | 21.897s | 16.300ms |
Method | ANN-based | EEMD+AR+SAE [32] | LMD+RWSVM [33] | SAE-based [34] | The proposed method |
Average accuracy (%) | 59.963 | 94.513 | 91.615 | 95.813 | 99.250 |
Average training time (s) | 20.116 | 147.078 | 54.510 | 37.913 | 21.897 |
Average recognition time (ms) | 16.547 | 39.667 | 28.343 | 31.025 | 16.300 |
AR: Autoregressive; SAE: Sparse autoencoder; RWSVM: Reproducing wavelet support vector machines. |
No. | Damage | Health status | Fault mode | Fault form | Level |
01 | - | - | - | - | 0 |
02 | Pi | ORF | SP | SP | 1 |
03 | In | ORF | SP | SP | 1 |
04 | Pi | ORF | R | SP | 2 |
05 | In | ORF | R | Distributed | 1 |
06 | Pi | IRF | M | SP | 1 |
07 | Pi | IRF | SP | SP | 3 |
08 | Pi | IRF | R | SP | 1 |
09 | Pi | IRF | SP | SP | 2 |
10 | Pi | IRF | SP | SP | 1 |
11 | Pi | IRF+(ORF) | M | SP | 2 |
12 | Pi | IRF+(ORF) | M | Distributed | 3 |
13 | In | ORF+IRF | M | Distributed | 1 |
Note: Pi: Pitting; In: Indentations. |
No. | Average diagnostic accuracy (%) |
01 | 100.000 |
02 | 100.000 |
03 | 98.330 |
04 | 99.500 |
05 | 99.063 |
06 | 100.000 |
07 | 99.316 |
08 | 100.000 |
09 | 98.972 |
10 | 99.737 |
11 | 100.000 |
12 | 98.783 |
13 | 99.778 |
Average accuracy | Training time | Recognition time |
99.481% | 37.646s | 19.900ms |
Method | ANN-based | EEMD+AR+SAE [32] | LMD+RWSVM [33] | SAE-based [34] | The proposed method |
Average accuracy (%) | 57.961 | 93.846 | 90.173 | 94.423 | 99.481 |