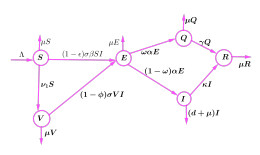
In this study, a fractional-order model for COVID-19 disease transmission is proposed and studied. First, the disease-free equilibrium and the basic reproduction number, R0 of the model has been communicated. The local and global stability of the disease-free equilibrium have been proved using well-constructed Lyapunov functions. Moreover, a normalized sensitivity analysis for the model parameters has been performed to identify their influence on R0. Real data on COVID-19 disease from Wuhan in China has been used to validate the proposed model. Finally, a simulation of the model has been performed to determine the effects of memory and control strategies. Overall, one can note that vaccination and quarantine have the potential to minimize the spread of COVID-19 in the population.
Citation: Mlyashimbi Helikumi, Paride O. Lolika. Dynamics and analysis of COVID-19 disease transmission: The effect of vaccination and quarantine[J]. Mathematical Modelling and Control, 2023, 3(3): 192-209. doi: 10.3934/mmc.2023017
[1] | J. O. Akanni, S. Ajao, S. F. Abimbade, Fatmawati . Dynamical analysis of COVID-19 and tuberculosis co-infection using mathematical modelling approach. Mathematical Modelling and Control, 2024, 4(2): 208-229. doi: 10.3934/mmc.2024018 |
[2] | Yaxin Ren, Yakui Xue . Modeling and optimal control of COVID-19 and malaria co-infection based on vaccination. Mathematical Modelling and Control, 2024, 4(3): 316-335. doi: 10.3934/mmc.2024026 |
[3] | Ihtisham Ul Haq, Nigar Ali, Shabir Ahmad . A fractional mathematical model for COVID-19 outbreak transmission dynamics with the impact of isolation and social distancing. Mathematical Modelling and Control, 2022, 2(4): 228-242. doi: 10.3934/mmc.2022022 |
[4] | Paride O. Lolika, Mlyashimbi Helikumi . Global stability analysis of a COVID-19 epidemic model with incubation delay. Mathematical Modelling and Control, 2023, 3(1): 23-38. doi: 10.3934/mmc.2023003 |
[5] | Ankur Jyoti Kashyap, Arnab Jyoti Bordoloi, Fanitsha Mohan, Anuradha Devi . Dynamical analysis of an anthrax disease model in animals with nonlinear transmission rate. Mathematical Modelling and Control, 2023, 3(4): 370-386. doi: 10.3934/mmc.2023030 |
[6] | Anita T. Kurniawati, Fatmawati, Chidozie W. Chukwu, Windarto, Faishal F. Herdicho . Optimal control of dengue fever model with a logistically growing human population. Mathematical Modelling and Control, 2025, 5(1): 48-60. doi: 10.3934/mmc.2025004 |
[7] | Salma Al-Tuwairqi, Asma Badrah . A qualitative analysis of a model on alpha-synuclein transport and aggregation in neurons. Mathematical Modelling and Control, 2023, 3(2): 104-115. doi: 10.3934/mmc.2023010 |
[8] | Monica Veronica Crankson, Olusegun Olotu, Ayodeji Sunday Afolabi, Afeez Abidemi . Modeling the vaccination control of bacterial meningitis transmission dynamics: a case study. Mathematical Modelling and Control, 2023, 3(4): 416-434. doi: 10.3934/mmc.2023033 |
[9] | Abdul-Fatawu O. Ayembillah, Baba Seidu, C. S. Bornaa . Mathematical modeling of the dynamics of maize streak virus disease (MSVD). Mathematical Modelling and Control, 2022, 2(4): 153-164. doi: 10.3934/mmc.2022016 |
[10] | Elijah B. Baloba, Baba Seidu . A mathematical model of anthrax epidemic with behavioural change. Mathematical Modelling and Control, 2022, 2(4): 243-256. doi: 10.3934/mmc.2022023 |
In this study, a fractional-order model for COVID-19 disease transmission is proposed and studied. First, the disease-free equilibrium and the basic reproduction number, R0 of the model has been communicated. The local and global stability of the disease-free equilibrium have been proved using well-constructed Lyapunov functions. Moreover, a normalized sensitivity analysis for the model parameters has been performed to identify their influence on R0. Real data on COVID-19 disease from Wuhan in China has been used to validate the proposed model. Finally, a simulation of the model has been performed to determine the effects of memory and control strategies. Overall, one can note that vaccination and quarantine have the potential to minimize the spread of COVID-19 in the population.
Coronavirus Disease (COVID-19) is a highly contagious and viral disease that spreads easily from person to person through contact [1]. It can also be contracted through respiratory droplets released when an infected person coughs, sneezes, breathes, sings or talks [2]. The common symptoms of COVID-19 include fever, cough, chills, headache, muscles aches, vomiting and diarrhea [3]. Other symptoms include breathing difficulties, loss of speech and in severe cases, pneumonia, stroke and blood clots have been the most common problems in COVID-19 patients [4]. The disease affects most people with high blood pressure, cancer, heart failure, overweight, obesity, liver disease and weakened immune systems [5]. Evidence from literature shows that old age has high risk of serious illness from COVID-19 and the risk increases with age [6].
The World Health Organization (WHO) declared COVID-19 a public health problem on 30th January 2020 [7] and a pandemic on 11th March 2020 [7,8]. The first case report of COVID-19 in Tanzania was declared in Arusha before spreading to other parts of the country. Then, on 29th April 2020, the Ministry of Health, Community Development, Gender, Elderly and Children reported a total of 480 confirmed cases and 16 deaths from the disease [9].
Public health prevention measures, including banning all large gatherings, limiting the number of people attending burials, physical distancing and wearing masks, were implemented all over the world [10]. Unlike other East African countries, Tanzania did not enforce lock-down, and people were allowed to continue with income-generating activities normally [9]. However, health education campaigns on prevention measures, such as wearing masks, hygiene practices, avoiding public gatherings and keeping physical distance, were intensively encouraged through mass media and social media [11]. Despite these prevention measures, vaccination remains the most powerful tool in preventing the spread of COVID-19 in the population [12]. Globally, WHO reported that more than 116,135,492 and 2,581,976 confirmed cases and deaths, respectively, and 249,160,837 vaccine doses have been administrated worldwide, including Tanzania [9,13]. However, the disease still persists in the population and there are several cases of resurgences globally. Understanding the dynamics of COVID-19 and its control strategies is a substantially important in order to minimize the spread of the disease in the community [14,15].
Mathematical models of disease transmission have been widely studied since the work of Kermack and McKendrick [16], Greenwood and Yule [17], Ross [18], Bernoulli [19], Brownlee [20], Soper [21], Greenwood [22] and also for the details of history in disease modeling (see, [23,24]) and the references therein. Mathematical models with fractional-order differential equations have particulary received greater attention (see, [25,26,27,28,29,30,31]) and are widely used in disease modeling compared to the integer-order derivatives [32,33]. Fractional-order operators are more accurate in modeling dynamical systems than classical operators as fractional-order models allow more degree of freedom [34,35]. It is worth mentioning here that fractional-order operators have more advantages than classical-order operators as fractional-order models adequately capture hereditary properties, long-range interactions and memory effects that exist in many biological systems [32,33,34,35]. In contrast, it has been documented in the literature that models that utilize integer-order derivatives do not adequately capture hereditary properties, and memory effects that exist in many biological systems [33]. Besides, when compared to the classical integer-order models, fractional-order models provide a higher level of precision and give a better fit to the actual data [36].
Recently, Bushnaq et al. [37], Owusu et al. [38], Ahmed et al. [39], Baba et al. [40], Omame et al. [41] and Aslam et al. [42] utilized the fractional-order derivatives to investigate the effect of memory on COVID-19 disease transmission. Mathematical studies of fractional order differential equations in disease modeling are also found in [43,44,45,46,47,48] and the references therein. For instance, Singh et al. [44] proposed and studied a fractional order model using Atangana-Baleanu Caputo sense to investigate the effect of quarantine on the spread of COVID-19 disease in the population. In conclusion the authors mentioned that quarantine of the infected individuals is effective to minimize the spread of disease in the population. Rehman et al. [43] formulated a fractional order model using the Caputo derivative to investigate the dynamics of COVID-19 and dengue co-infections in the population. The authors simulated the graphs of both COVID-19 and dengue co-infection to compare the results in the sense of Caputo, Caputo-Fabrizio and Atangana-Baleanu. From numerical simulations, their results demonstrated that Caputo sense had better results in the form of stability compared to other operators. Anggriani and Beay [47], formulated a mathematical model of COVID-19 to study the impact of self-isolation and hospitalization. The authors performed a global sensitivity analysis of the model using Latin Hypercube sampling and partial correlation coefficient methods. Their results revealed that parameters representing self-isolation and hospitalization have negative relations. Lolika and Mlyashimbi [48] proposed and studied a COVID-19 epidemic model with incubation delay. The authors computed the basic reproduction number and used to establish the conditions for global stability of equilibrium points. Furthermore, the authors concluded that quarantine of asymptomatic and symptomatic individuals have an impact on minimizing the spread of COVID-19 in the population.
Fractional derivatives have many definitions. In this study, we have chosen to utilize the renowned Caputo fractional operator due to the fact that, the ability to use classical initial conditions in the model formulation is the key benefits of the Caputo fractional derivatives compared to other fractional operators [49,50,51,52,53]. It is worthwhile to mention that the Caputo fractional derivative of any constant is zero. Furthermore, the Caputo fractional operator has a singular non-kernel, which is missing in other operators [54,55,56]. Therefore, we proposed and studied a Caputo fractional-order model of COVID-19 disease transmission to assess the effects of vaccination and quarantine in order to minimize the spread of disease in the population.
The rest of the paper is organized as follows: In Section 2, the proposed model and its analytical results are presented. Results discussion are provided in Section 3. Finally, the concluding remarks are presented in Section 4.
In this section, the Caputo fractional-order derivative has been used to define the model differential equations for COVID-19 transmission. The compartments proposed in this study are used to represent the epidemiological status of each human population. The proposed model consists of six sub-divided compartments: susceptible S(t), exposed E(t), vaccinated V(t), infectious I(t), quarantine Q(t) and recovered R(t) human populations. Thus, the total human population is denoted by N(t) which is, N(t)=S(t)+E(t)+V(t)+Iv(t)+Q(t)+R(t). Throughout the article, variables and parameters are assumed to be none-negative and are defined as follows: Λ and ν1 represent the rate of new recruitment and transition rate from susceptible to vaccinated classes respectively. Thus; ϕ represents the efficacy of vaccination for vaccinated susceptible individuals; 1α denotes the average time humans spend in incubation period; ω represents the rate of quarantine in the population; following successful treatment, I(t) infected humans recover from disease after 1κ days; μ and γ represent the human natural mortality and the transition rate of quarantined people to recovered classes, respectively; d represents the death rate of infected humans. Additionally, it is assumed that once the people become aware of COVID-19 transmission, they change their behavior and take precautions, such as hand-washing, wearing masks, keeping social distances and even quarantining themselves. Thus, the parameter ϵ represents the reduction rate of COVID-19 transmission of susceptible individuals due to health education campaigns. Furthermore, it was assumed that β represents the probability of disease transmission following the successful contact rate σ between infected and susceptible individuals.
Our assumptions on the dynamics of COVID-19 in this study are illustrated in figure 1 and the corresponding model differential equations are presented in model (2.1):
{cbDθtS(t)=Λθ−(1−ϵθ)σθβθS(t)I(t)−(νθ1+μθ)S(t),cbDθtV(t)=νθ1S(t)−(1−ϕq)σθβθI(t)V(t)−μθV(t),cbDθtE(t)=(1−ϵθ)σθβθI(t)S(t)+(1−ϕθ)σθβθI(t)V(t)−(αθ+μθ)E(t),cbDθtQ(t)=ωθαθE(t)−(γθ+μθ)Q(t),cbDθtI(t)=(1−ωθ)αθE(t)−(κθ+μθ+dθ)I(t),cbDθtR(t)=γθQ(t)+κθI(t)−μθR(t). | (2.1) |
We begin by introducing the definition of Caputo fractional derivative and state the related theorems (see, [56,57,58,59]) that we will utilize to derive important results in this work.
Definition 2.1. Suppose that θ>0,t>b,θ,b,t∈R, the Caputo fractional derivative is given by:
cbDθtf(t)1Γ(n−θ)∫tbfn(ξ)(t−ξ)θ+1−ndξ,n−1<θ,n∈N. | (2.2) |
Definition 2.2. (Linearity property [56]). Let f(t),g(t):[b,d]→R be such that cbDθtf(t) and cbDθtg(t) exist almost everywhere and let c1,c2∈R. Then, cbDθt(c1f(t))+cbDθt(c2g(t)) exists everywhere, and
cbDθt(c1f(t)+c2g(t))=c1 cbDqtf(t)+c2 cbDθtg(t). | (2.3) |
Definition 2.3. (Caputo derivative of a constant [59]). The fractional derivative for a constant function f(t)=c is zero, that is:
cbDθtc=0. | (2.4) |
Let us consider the following general type of fractional differential equations involving the Caputo derivative:
cbDθtx(t)=f(t,x(t)),θ∈(0,1), | (2.5) |
with initial condition x0=x(t0).
Definition 2.4. (see [56]). The constant x∗ is an equilibrium point of the Caputo fractional dynamic system (2.5) if and only if f(t,x∗)=0.
In what follows, we present an extension of the Lyapunov direct method for Caputo type fractional order for nonlinear systems [56,60].
Theorem 2.1. (Uniform Asymptotic Stability [56,60]). Let x∗ be an equilibrium point for the non-autonomous fractional order system (2.5) and Ω⊂Rn be a domain containing x∗. Let L:[0,∞)×Ω→R be a continuously differentiable function such that:
M1(x)≤N(t,x(t))≤M2(x), |
and:
cbDθtN(t,x(t))≤M3(x), |
for all q∈(0,1) and all x∈Ω, where M1(x), M2(x) and M3(x) are continuous positive definite functions on Ω. Then, the equilibrium point of system (2.5) is uniformly asymptotically stable.
The following theorem summarizes a lemma proved in [56], where a Volterra-type Lyapunov function is obtained for fractional-order epidemic systems.
Lemma 2.1. (see [56]. Let x(⋅) be a continuous and differentiable function with x(t)∈R+. Then, for any time instant t≥b, one has:
cbDθt(x(t)−x∗−x∗lnx(t)x∗)≤(1−x∗x(t)) cbDθtx(t),x∗∈R+,∀θ∈(0,1). |
Theorem 2.2. For the model (2.1), there exists a unique solution in (0,∞); however, the solution is always positive for all values of t≥0 and remains in R6+.
Proof. From the model (2.1), we first show that
R6+= {N(t) ∈R6+:N(t)≥0} is a positive invariant set. Then, we have to demonstrate that each hyper-plane bounding the positive orthant and the vector field points to R6+. Now consider the following: let us assume that there exists a t∗>t0 such that N(t∗)=0, and N(t)<0 for t∈(t∗,t1), where t1 is sufficiently close to t∗, if N(t∗)=0, then we have that,
cbDθtN(t∗)−Λθ>0. This implies that cbDθtN(t)>0 for all t∈[t∗,t1]. The above discussion shows that the three hyper-plane bounding the orthants that is the vector field points to R6+. This shows that all the solutions of the model (2.1) remains positive for all t≥0.
Theorem 2.3. Let Φ(t)=N(t) be the unique solution of the model (2.1) for all t≥0. Then, the solution Φ(t) is bounded above, that is, Φ(t)∈Ω, where Ω which is the feasible region is defined as,
Ω={N(t)∈R6+0≤N(t)≤CN.} |
and its interior denoted by int(Ω) is given by,
int(Ω)={N(t)∈R6+0≤N(t)≤CN}. |
Proof. Here, we prove that the solutions of model (2.1) are bounded for all t≥0. Biologically, the lowest possible value of each state of model (2.1) is zero. Next, we determine the upper-bound of states. Based on this discussion, it is easy to show that the following condition holds for biological relevance of species. 0≤N(t)≤CN. From this condition one gets:
cbDθtN(t)≤Λθ−μθN(t). |
From the Laplace transformation condition one gets:
SθL[N(t)]−Sθ−1N(0)≤ΛθS−μθL[N(t)]. |
Collecting the likely terms we have:
L[N(t)]≤ΛθS−1Sθ+μθ+N(0)Sq−1Sθ+μθ=ΛθSθ−(1+θ)Sθ+μθ+N(0)Sθ−1Sq+μθ. |
Using the inverse Laplace transform we have:
N(t)≤L−1{pθΛθSθ−(1+θ)Sθ+μθ}−N(0)L−1{Sθ−1Sθ+μθ}≤ΛθtθEq,θ+1(−μθ)tθ+N(0)Eθ,1(−μθ)tθ≤ΛθμθtθEθ,θ+1(−μθ)tθ+N(0)Eθ,1(−μθ)tθ≤Max{Λθμθ,N(0)}(tθEθ,θ+1(−μθ)tθ+Eθ,1(−μθ)tθ)=CΓ(1)=CN. |
Where, CN=Max{Λθμθ,N(0)}. Therefore, N(t) is bounded above and this completes the proof.
Since R(t) does not appear in all the equations in model (2.1), it is sufficient to analyze the solutions of model (2.6) for the behavior of model differential equations (2.1).
{cbDθtS(t)=Λθ−(1−ϵθ)σθβθS(t)I(t)−(νθ1+μθ)S(t),cbDθtV(t)=νθ1S(t)−(1−ϕθ)σθβθI(t)V(t)−μθV(t),cbDθtE(t)=(1−ϵθ)σθβθI(t)S(t)+(1−ϕq)σθβθI(t)V(t)−(αθ+μθ)E(t),cbDθtQ(t)=ωθαθE(t)−(γθ+μθ)Q(t),cbDθtI(t)=(1−ωθ)αθE(t)−(κθ+μθ+dθ)I(t). | (2.6) |
In what follows, we compute the threshold quantity, R0 which determines the power of the disease to spread in the population. The model (2.6) always has a disease-free equilibrium, E0 given by:
E0:(S0,V0,E0,Q0,I0,R0)=(Λθνθ1+μθ,νθ1Λθμθ(νθ1+μθ),0,0,0). |
Following the next generation matrix approach as used in [31,61], the non-negative matrix F that denotes the generation of new infections and the non-singular matrix V that denotes the disease transfer among compartments evaluated at E0 are defined as follows:
F=(00(1−ϵθ)σθβθS0+(1−ϕ)σθβθV0000000), | (2.7) |
V=(αθ+μθ00−ωθαθγθ+μθ0−(1−ωθ)αθ0κθ+μθ+dθ). | (2.8) |
Therefore, from (2.7) and (2.8) it can easily be verified that the basic reproduction number R0 of model (2.1) is:
R0=Λθνθ1+μθ(1−ωθ)αθαθ+μθ((1−ϵθ)σθβθ(κθ+μθ+dθ)+1−ϕθκθ+μθ+dθν1μθ). |
The basic reproduction number R0 is defined as the expected number of secondary cases of humans produced in a completely susceptible population by one infected individual during its lifetime as infectious. The terms Λθνθ1+μθ, νθ1μθ1 and (1−ωθ)αθαθ+μθ represent the total life span of humans and the average life span of vaccinated and quarantined individuals respectively.
Our goal in this section is to investigate the global stability of the disease-free equilibrium and the endemic equilibrium of the model (2.6).
Theorem 2.4. If R0<1, the disease free-equilibrium point of the model (2.1) is locally asymptotically stable and unstable if R0>1.
Proof. To prove theorem (2.4), we evaluate the Jacobian matrix of the model (2.6) at the disease-free equilibrium and investigate the behavior of eigenvalues. In what follows, the Jacobian matrix of the model (2.6) evaluated at the disease free-equilibrium is given by:
JDFE=(−(νθ1+μθ)000−(1−ϵθ)σθβθS0νθ1−μq00−(1−ϵθ)σθβθV000−(αθ+μθ)0(1−ϵθ)σθβθS0+(1−ϕθ)σθβθV000ωθαθ−(γθ+μθ)000(1−ωθ)αθ0−(κθ+μθ+dθ)). | (2.9) |
The first three eigenvalues of matrix (2.9) are λ1=−(νθ1+μθ),λθ2=−μθ, and λ3=−(γθ+μθ) which are non-positive. The remaining two eigenvalues are obtained in the following matrix;
M=(−(αθ+μθ)(1−ϵθ)σθβθS0+(1−ϕθ)σθβθV0(1−ωθ)αθκθ+μθ+dθ). | (2.10) |
It follows that we find the characteristic polynomial of the matrix (2.10) which is given as follows:
λ2+(αθ+2μθ+κθ+dθ)λ+(1−R0)=0. | (2.11) |
Since the coefficients of characteristic polynomial (2.11) are all non-negative for R0<1, we conclude that the disease-free equilibrium E0 of the model (2.2) is locally asymptotically stable and this completes the proof.
Theorem 2.5. The disease-free equilibrium E0 is globally asymptotically stable if R0≤1, otherwise is unstable.
Proof. To prove the theorem (2.5), we first evaluate the model (2.6) at the point E0 and this leads to the following model;
{cbDθtS(t)=S(t)(Λθ(1S(t)−1S0)−(1−ϵθ)βθI(t)),cbDθtV(t)=V(t)(σθνθ1(S(t)V(t)−S0V0)−(1−ν1)σθI(t)),cbDθtE(t)=(1−ϵθ)(S0+(S(t)−S0))+(1−ν1)βθI(t)(V0+(V(t)−V0)−(αθ+μθ)E(t)),cbDθtQ(t)=ωθαθE(t)−(γθ+μθ)Q(t),cbDθtI(t)=(1−ωθ)αθE(t)−(κθ+μθ+dθ)I(t). | (2.12) |
In the followings, we consider the following Lyapunov function:
L0(t)={S(t)−S0−S0lnS(t)S0}+{V(t)−V0−V0lnV(t)V0}+Q(t)+E(t)+(1−ωθ)αθ+μθ(1−ωθ)αθI(t). |
Taking the derivative of L0(t) along the model (2.12) and making simplifications, one gets:
cbDθtL0(t)≤Λθ{2−S(t)S0−S0S}−νθ1S0{1+S(t)S0−S(t)S0V0V(t)−V(t)V0}−{γq+μθ}Q+{{1−ωθ}αθ{κθ+μθ+dθ}}{1−ωθ}αq{R0−1}I(t). |
Since all the parameters and variables in system (2.13) are non-negative, it follows that cbDθtL0(t)<0 holds if R0<1. Moreover, cbDθtL0(t)=0 if and only if S(t)=0, V(t)=0, E(t)=0, Q(t)=0, I(t)=0, for all t≥0. Thus, L0(t) is Lyapunov function on Ω. Using Lasalle Invariance principle [62] it implies that every solution of the system (2.6) approaches the disease-free equilibrium E0 as t→∞. Therefore, we conclude that the disease-free equilibrium of system (2.6) is globally asymptotically stable whenever R0≤1. This completes the proof.
Theorem 2.6. The Model (2.6) has endemic equilibrium E∗ point which is globally asymptotically stable for R0>1.
Proof. To prove the theorem (2.6), we consider the following Lyapunov functional:
L1(t)=A1{S(t)−S∗−S∗lnS(t)S∗}+A2{V(t)−V∗−V∗lnV(t)V0}+A3{E(t)−E∗−E∗lnE(t)E∗}+A4{Q(t)−Q∗−Q∗lnQ(t)Q∗}+A5{I(t)−I∗−I∗lnI(t)I∗}. |
Differentiating L1(t) one gets the following:
cbDθtL1(t)≤A1(1−S∗S)cbDθtS(t)+A2(1−V∗V(t))cbDθtV(t)+A3(1−E∗E(t))cbDθtE(t)+A4(1−Q∗Q(t))cbDθtQ(t)+A5(1−I∗I(t))cbDθtI(t). | (2.13) |
In what follows, we substitute (2.1) in (2.13) and get the following:
cbDθtL1(t)≤A1(1−S∗S(t))(Λθ−(1−ϵθ)σθβθS(t)I(t)−(ηθρθ+ϵθ+μθ)S(t))+A2(1−V∗V(t))(νθ1S(t)−(1−ϕθ)σθβθI(t)V(t)−μθV(t))+A3(1−E∗E(t))((1−ϕθ)σθβθI(t)S(t)+(1−ϕθ)σθβθI(t)V(t)−(αθ+μθ)E(t))+A4(1−Q∗Q(t))(ωθαθE(t)−(γθ+μθ)Q(t))+A5(1−I∗I(t))((1−ωθ)αθE(t)−(κθ+μθ+dθ)I(t)). | (2.14) |
Setting the model (2.6) at the endemic equilibrium point,
{νθ1+μq=ΛθS∗−(1−ϵθ)σθβθI∗μθ=νθ1S∗V∗−(1−ν1)θθβθI∗(αθ+μθ)=(1−ϵθ)σθβθS∗I∗E∗+(1−ϕθ)σθI∗V∗E∗,(γθ+μθ)=ωθαθE∗Q∗,(κθ+μθ+dθ)=(1−ωθ)αθE∗I∗. | (2.15) |
By substituting (2.15) in (2.14) and solving the constants Ai for i=1,2,...5, one gets the following after simplifications:
cbDθtL1(t)≤νθ1S∗(2−S(t)S∗−S∗S(t))+(1−ωθ)αθE∗(3−V(t)V∗−S(t)S∗−S(t)S∗V∗V)+(1−ϵθ)σθβθ(3−S(t)S∗−E(t)E∗I∗I(t)−S(t)S∗I(t)I∗E∗E(t))+(1−ϕθ)σθI∗V∗(4−S∗S(t)−S∗S(t)V∗V(t)−E(t)E∗I(t)I∗−I(t)I∗V(t)V∗E∗E(t)). | (2.16) |
Since the arithmetic mean is greater than or equal to the geometrical mean, it follows that, from (2.16) we have the following:
(2−S(t)S∗−S∗S(t))≤0. | (2.17) |
Furthermore, let Φ(z)=1−z−ln(z) for z>0. One can note that Φ(z)≤0 if and only if z=1. Using the aforementioned properties of Φ(z), from (2.16) one can note that:
(3−V(t)V∗−S(t)S∗−S(t)S∗V∗V)=Φ(S(t)S∗V(t)V∗)−V(t)V∗−S(t)S∗≤ln(V(t)V∗)−V(t)V∗+ln(S(t)S∗)−S(t)S∗≤0. | (2.18) |
(3−S(t)S∗−E(t)E∗I∗I−S(t)S∗II∗E∗E(t))=Φ(S(t)S∗E(t)E∗I∗I)−S(t)S∗−E(t)E∗I∗I(t)≤ln(S(t)S∗)−S(t)S∗+ln(E(t)E∗I∗I(t))−E(t)E∗I∗I(t)≤0. | (2.19) |
(4−S∗S(t)−S∗S(t)V∗V(t)−E(t)E∗II∗−I(t)I∗V(t)V∗E∗E(t))=Φ(I(t)I∗V(t)V∗E∗E(t))+Φ(E(t)E∗II∗)−S∗S(t)−S∗S(t)V∗V(t)≤ln(S(t)S∗)−S(t)S∗+ln(S∗S(t)V∗V(t))−S∗S(t)V∗V(t)≤0. | (2.20) |
From (2.17), (2.19), (2.18), and (2.20), one can note that cbDθtL1(t)≤0 whenever R0>1. Therefore, using Lasalle Invariance principle [62], the model (2.1) has a global asymptotically stable equilibrium point for all R0≥1 and this completes the proof.
In this section, we perform the numerical simulations of the model (2.1) to justify the analytical results. Most of the parameter values that are not available in the literature have been estimated. Additionally, for the simulation initial conditions of the model (2.1), was assumed to be S(0)=900,V(0)=0,E(0)=200,Q(0)=0 and I(0)=2. Using the similar concept in [63], the fractional Adam-Bashforth-Moulton scheme for the model (2.1) has the following form:
{S(tn+1)=S0+hθΓ(θ+2)fS(tn+1,Sp(tn+1),Vp(tn+1),Ep(tn+1),Ip(tn+1),Qp(tn+1),Rp(tn+1))+hθΓ(θ+2)n∑m=0am,n+1fS(tm,S(tm),V(tm),E(tm),I(tm),Q(tm),R(tm)),V(tn+1)=V0+hθΓ(θ+2)fV(tn+1,Sp(tn+1),Vp(tn+1),Ep(tn+1),Ip(tn+1),Qp(tn+1),Rp(tn+1))+hqΓ(θ+2)n∑m=0am,n+1fV(tm,S(tm),V(tm),E(tm),I(tm),Q(tm),R(tm)),E(tn+1)=E0+hθΓ(θ+2)fE(tn+1,Sp(tn+1),Vp(tn+1),Eθ(tn+1),Ip(tn+1),Qp(tn+1),Rp(tn+1))+hθΓ(q+2)n∑m=0am,n+1fE(tm,S(tm),V(tm),E(tm),I(tm),Q(tm)),R(tm)I(tn+1)=I0+hθΓ(θ+2)fI(tn+1,Sp(tn+1),Vp(tn+1),Ep(tn+1),Ip(tn+1),Qp(tn+1),Rp(tn+1))+hθΓ(θ+2)n∑m=0am,n+1fI(tm,S(tm),V(tm),E(tm),I(tm),Q(tm),R(tm)),Q(tn+1)=Q0+hθΓ(θ+2)fQ(tn+1,Sp(tn+1),Vp(tn+1),Ep(tn+1),Ip(tn+1),Qp(tn+1),Rp(tn+1))+hθΓ(θ+2)n∑m=0am,n+1fQ(tm,S(tm),V(tm),E(tm),I(tm),Q(tm),R(tm)),R(tn+1)=R0+hθΓ(θ+2)fR(tn+1,Sp(tn+1),Vp(tn+1),Ep(tn+1),Ip(tn+1),Qp(tn+1),Rp(tn+1))+hθΓ(θ+2)n∑m=0am,n+1fR(tm,S(tm),V(tm),E(tm),I(tm),Q(tm),R(tm)). | (3.1) |
Where:
{Sp(tn+1)=S0+1Γθn∑m=0bm,n+1fS(tm,S(tm),V(tm),E(tm),I(tm),Q(tm)),R(tm)Vp(tn+1)=V0+1Γθn∑m=0bm,n+1fV(tm,S(tm),V(tm),E(tm),I(tm),Q(tm)),R(tm)Ep(tn+1)=E0+1Γθn∑m=0bm,n+1fE(tm,S(tm),V(tm),E(tm),I(tm),Q(tm)),R(tm)Ip(tn+1)=I0+1Γθn∑m=0bm,n+1fI(tm,S(tm),V(tm),E(tm),I(tm),Q(tm)),R(tm)Qp(tn+1)=Q0+1Γθn∑m=0bm,n+1fQ(tm,S(tm),V(tm),E(tm),I(tm),Q(tm)),R(tm)Rp(tn+1)=R0+1Γθn∑m=0bm,n+1fR(tm,S(tm),V(tm),E(tm),I(tm),Q(tm)),R(tm). | (3.2) |
In what follows we have:
{fS(tm,S(tm),V(tm),E(tm),I(tm),Q(tm),R(tm))=cbDαtS(t),fV(tm,S(tm),V(tm),E(tm),I(tm),Q(tm),R(tm))=cbDαtV(t),fE(tm,S(tm),V(tm),E(tm),I(tm),Q(tm),R(tm))=cbDαtE(t),fI(tm,S(tm),V(tm),E(tm),I(tm),Q(tm),R(tm))=cbDαtI(t),fQ(tm,S(tm),V(tm),E(tm),I(tm),Q(tm),R(tm))=cbDαtQ(t),fR(tm,S(tm),V(tm),E(tm),I(tm),Q(tm),R(tm))=cbDαtR(t). | (3.3) |
Additionally, the quantities:
{fS(tn+1,Sp(tn+1),Vp(tn+1),Ep(tn+1),Ip(tn+1),Qp(tn+1),Rp(tn+1)),fV(tn+1,Sp(tn+1),Vp(tn+1),Ep(tn+1),Ip(tn+1),Qp(tn+1),Rp(tn+1)),fE(tn+1,Sp(tn+1),Vp(tn+1),Ep(tn+1),Ip(tn+1),Qp(tn+1),Rp(tn+1)),fI(tn+1,Sp(tn+1),Vp(tn+1),Ep(tn+1),Ip(tn+1),Qp(tn+1),Rp(tn+1)),fQ(tn+1,Sp(tn+1),Vp(tn+1),Ep(tn+1),Ip(tn+1),Qp(tn+1),Rp(tn+1)),fR(tn+1,Sp(tn+1),Vp(tn+1),Ep(tn+1),Ip(tn+1),Qp(tn+1),Rp(tn+1)). | (3.4) |
are the derivatives from (3.3) at the point tn+1,n=1,2,3,...m..
Symbol | Description | Value | Units | Source |
Λ | Per capita human recruitment rate | 11826 | day −1 | [64] |
d | Disease induced death rate | day −1 | 0.0413 | [9] |
μ | Natural death rate | day −1 | 0.2 | [9] |
β | Force of infections | day −1 | Fitted | |
α | Incubation period | day −1 | 0.5171 | [65] |
σ | Average per capita contact rate | day −1 | Fitted | |
ϕ | Efficacy rate of vaccination | day −1 | 0:854 | [64] |
ν1 | Vaccination rate | day −1 | 0.0313 | [65] |
γ | Recovery rate | day−1 | 0.362 | [64] |
ϵ | Human awareness rate | day−1 | Vary | Assumed |
κ | Treatment rate of infected individuals | unit-less | Vary | Assumed |
ω | Quarantine rate of suspected humans | unit-less | Vary | Assumed |
In this section, the sensitivity analysis of the model (2.1) has been performed to demonstrate the influence of each parameter on the magnitude of the threshold quantity R0. Most of the parameters used in this study have been drawn from literature and some are estimated using reasonable ranges for the purpose of simulation.
Definition 3.1. (See, [66]) The normalized sensitivity index of R0, which depends on differentiability of parameter, ω is defined as follows:
ΨR0ω=∂R0∂ω×ωR0 | (3.5) |
Figure 2 demonstrates the relationship between the basic reproduction number R0 and the model parameters of the model (2.1). Overall, one can note that the model parameters β, γ, σ and Λ have a positive influence on the R0, that is whenever they are increased, the size of R0 increases. On the other-hand, parameters with negative index values have a negative influence on R0, that is, whenever they increased, the value of R0 decreases.
In this section, we use the daily cases of COVID-19 from Wuhan in China as reported in [67] and estimate the parameters (β,σ) that minimize the deviation of real data from prediction of model system (2.1). The main advantage of fractional-order differential equation is that the order of fractional can be any real positive number, so one can choose the one that has best fit of real data to the model and predict the future evolution of the disease in the population. Therefore, in this study, we use both the least squares and Nelder mead algorithm methods as presented in [71] to fit and estimate the parameters (d,β,θ) of the model (2.1). The real data used in this study are daily reported cases as shown in table (2), and the commutative new infections predicted by the model (2.1) is obtained using the equation (3.6)
cbDθtC(t)=(1−ϵ)σθβθI(t)S(t)+(1−ϕ)σθβθI(t)V(t) | (3.6) |
Day | 1 | 2 | 3 | 4 | 5 | 6 | 7 | 8 |
Cases | 6 | 12 | 19 | 25 | 31 | 38 | 44 | 60 |
Day | 9 | 10 | 11 | 12 | 13 | 14 | 15 | 16 |
Cases | 80 | 131 | 259 | 3839 | 469 | 688 | 776 | 1776 |
Day | 17 | 18 | 19 | 20 | 21 | 22 | 23 | |
Cases | 1460 | 1739 | 1984 | 2101 | 2590 | 2827 | 3233 |
We use the following function to compute the best fitting:
F:R2(β,σ)→R(β,σ) | (3.7) |
where β,σ are parameters such that:
(1) For a given (β,σ), we numerically solve the system (2.1) to get a solution ˆYi(t)=(ˆS,ˆV,ˆE,ˆQ,ˆI,ˆR which is an estimation of daily reported cases Y(t) of COVID-19 from Wuhan in China.
(2) Set t0=1 (the fitting process starts in day 1) and for t=2,3,...,23, corresponding to daily in where data are available, evaluate the computed numerical solution for I(t); that is., ˆI(1), ˆI(2), ˆI(3),.....,ˆI(23).
(3) Compute the root mean square (RMSE) of the difference between ˆI(1),ˆIh(2),....,ˆI(23) and real data. This function F returns the root-mean-square error (RMSE) where:
RMSE=√1n23∑k=1(I(k)−ˆI(k))2, | (3.8) |
(4) Determine a global minimum for the RMSE using Nelder-Mead algorithm. The function F takes values in R2 and returns a positive real number.
Using the formula (3.8), we computed the RMSE that measures the closeness of the model prediction to the real data and was found to be 0.1186. This shows that the system (2.1) has a good fit to the daily reported cases of COVID-19. On performing the fitting process we set the following initial conditions S(0)=9999, V(0)=7990, E(0)=10, I(0)=5, Q(0)=5 R(0)=3 and the model parameters are in Table (1). Note that since the fractional-order is any positive real number θ∈(0,1], one can choose the one that better fits the model to the real data. Based on this assertion, the fractional-order θ were assumed to be 0.59,0.6,0.61,0.62 and 0.63 that had a better fit of model to the real data reported in [67].
The outbreak of the COVID-19 depends on the close contact between infected and susceptible individuals in the community. Vaccination and quarantine are proposed as the most effective control strategies that minimize the spread of the COVID-19 disease in the population. In this study, a fractional-order model for COVID-19 has been proposed and studied to assess the effects of the aforementioned control strategies. To analyze the model, the important threshold parameter, R0, has been computed to investigate the stability analysis of the steady states of the model. The results from the analysis demonstrated that both the disease-free equilibrium and endemic equilibrium points are globally stable whenever the basic reproduction number is less and greater than unity, respectively. A Normalized sensitivity index of the basic reproduction number was been performed to establish the relationship between the threshold and model parameters. Overall, one can note that model parameters with a positive index increased the disease persistence in the population. Furthermore, to support the analytical results, matlab software was used to simulate the proposed model and the results demonstrated that both vaccination and quarantine have the potential to minimize the spread of disease in the population. In particular, the disease can die out from the population by vaccinating 70 susceptible people and quarantine 60 of suspected individuals. Besides, real data of COVID-19 disease from Wuhan city in China has been used to fit and validate the proposed model. From the numerical findings, it can be deduced70 that the model fits well with reported cases of COVID-19 in China. Additional simulation of the model to assess the effect of memory on the spread of COVID-19 disease has been performed and the results demonstrated that memory effect has an influence on the spread of COVID-19 in the population. In future, the proposed model presented in this study will be improved by incorporating time delay and assessing its effect on the spread of COVID-19 disease in the population.
All authors are grateful to their respective institutions for the support during preparation of the manuscript. Paride O. Lolika acknowledges the support from the University of Juba, South Sudan.
The authors declare that they have no conflicts of interest in this paper.
All authors have equal contributions and they read and approved the final version of the paper.
[1] |
M. Higazy, Novel fractional order SIDARTHE mathematical model of COVID-19 pandemic, Chaos, Solitons & Fractals, 139 (2020), 110007. http://doi.org/10.1016/j.chaos.2020.110007 doi: 10.1016/j.chaos.2020.110007
![]() |
[2] |
C. H. Jeong, K. J. Wan, R. S. Ki, K. Y. Kyung, S. J. Soo, M. J. Ho, et al., COVID-19 transmission and blood transfusion: A case report, J. INFECT. PUBLIC HEAL., 13 (2020), 1678–1679. http://doi.org/10.1016/j.jiph.2020.05.001 doi: 10.1016/j.jiph.2020.05.001
![]() |
[3] |
R. J. Larsen, R. M. Margaret, D. J. Martin, K. Peter, B. J. Hicks, Modeling the onset of symptoms of COVID-19, Front. Public Health, 8 (2020), 473. http://doi.org/10.3389/fpubh.2020.00473 doi: 10.3389/fpubh.2020.00473
![]() |
[4] |
H. Abdul, A. Shmmon, S. S. Ali, A. Mumtaz, M. Shruti, A review of COVID-19 (Coronavirus Disease-2019) diagnosis, treatments and prevention, Ejmo, 4 (2020), 116–125. http://doi.org/10.14744/ejmo.2020.90853 doi: 10.14744/ejmo.2020.90853
![]() |
[5] |
R. Yelena, B. Jennifer, M. Haley, H. Whitney, B. Karen, C. J. Rhonda, A model of disparities: risk factors associated with COVID-19 infection, International journal for equity in health, 19 (2020), 1–10. http://doi.org/10.1186/s12939-020-01242-z doi: 10.1186/s12939-020-01242-z
![]() |
[6] |
B. A. William, G. O. Ouslander, B. A. William, G. O. Joseph, COVID-19 Presents High Risk to Older Persons, J. AM. GERIATR. SOC., 68 (2020), 681. https://doi.org/10.1111/jgs.16426 doi: 10.1111/jgs.16426
![]() |
[7] |
C. Shao-Chung, C. Yuan-Chia, C. Y-L. Fan, C. Yu-Chan, C. Mingte, Y. Chin-Hua, et al., First case of Coronavirus Disease 2019 (COVID-19) pneumonia in Taiwan, J. FORMOS. MED. ASSOC., 119 (2020), 747–751. http://doi.org/10.1016/j.jfma.2020.02.007 doi: 10.1016/j.jfma.2020.02.007
![]() |
[8] |
A. Khadijah, B. Y. Abdul, Y. Maryam, T. Javaid, S. I. Abira. Progress of COVID-19 Epidemic in Pakistan, Asia Pacific Journal of Public Health, 32 (2020), 154–156 http://doi.org/10.1177/1010539520927259 doi: 10.1177/1010539520927259
![]() |
[9] |
J. M. Abdul-Rahman, K. H. Alfred, Mathematical modelling on COVID-19 transmission impacts with preventive measures: a case study of Tanzania, J. Biol. Dyn., 14 (2020), 748–766. http://doi.org/10.1080/17513758.2020.1823494 doi: 10.1080/17513758.2020.1823494
![]() |
[10] |
A. Imran, ML. A. Omar, COVID-19: Disease, management, treatment, and social impact, Sci. Total Environ., 728 (2020), 138861 http://doi.org/10.1016/j.scitotenv.2020.138861 doi: 10.1016/j.scitotenv.2020.138861
![]() |
[11] |
M. I. Baltazar, N. J. Samwel, M. Melina, S. L. Philip, P. J. Jackson, A. Caroline, et al., Community engagement in COVID-19 prevention: experiences from Kilimanjaro region, Northern Tanzania, The Pan African Medical Journal, 35 (2020), 146. http://doi.org/10.11604/pamj.supp.2020.35.2.24473 doi: 10.11604/pamj.supp.2020.35.2.24473
![]() |
[12] |
L. Marc, E. D. Natalie, Understanding COVID-19 vaccine efficacy, Science, 370 (2020), 763–765. http://doi.org/10.1126/science.abe5938 doi: 10.1126/science.abe5938
![]() |
[13] | S. Adekunle, O. Chuku, H. Zaheeda, P. Risha, D. Priyank, P. Stephanie, et al., Global pandemicity of COVID-19: situation report, Infectious Diseases: Research and Treatment, 14 (2020). |
[14] |
R. R. Kumar, K. Subhas, T. P. Kumar, V. Ezio, M. A. Kumar, Impact of social media advertisements on the transmission dynamics of COVID-19 pandemic in India, J. Appl. Math. Comput., 68 (2020), 19–44. http://doi.org/10.1007/s12190-021-01507-y doi: 10.1007/s12190-021-01507-y
![]() |
[15] |
G. Said, B. Yassir, A. Abdelghafour, B. Mostafa, K. Fahd, M. Driss, An adaptive social distancing SIR model for COVID-19 disease spreading and forecasting, Epidemiologic Methods, 10 (2021), 20200044. http://doi.org/10.1515/em-2020-0044 doi: 10.1515/em-2020-0044
![]() |
[16] |
K. W. Ogilvy, G. M. Anderson, Contributions to the mathematical theory of epidemics. Ⅱ.—The problem of endemicity, The Royal Society London, 138 (1932), 55–83. http://doi.org/10.1007/BF02464424 doi: 10.1007/BF02464424
![]() |
[17] |
G. Major, G. Y. Udny, An inquiry into the nature of frequency distributions representative of multiple happenings with particular reference to the occurrence of multiple attacks of disease or of repeated accidents, Journal of the Royal statistical society, 83 (1920), 255–279. http://doi.org/10.2307/2341080 doi: 10.2307/2341080
![]() |
[18] |
R. Ronald, An application of the theory of probabilities to the study of a priori pathometry.—Part I, The Royal Society London, 92 (1916), 204–230. http://doi.org/10.1098/rspa.1917.0014 doi: 10.1098/rspa.1917.0014
![]() |
[19] |
B. Daniel, Essai d'une nouvelle analyse de la mortalité causée par la petite vérole, et des avantages de l'inoculation pour la prévenir, Des Math. And Phis., Mem., (1960), 1–45. doi: 10.1177/003591571801101305
![]() |
[20] |
B. John, Certain Aspects of the Theory of Epidemiology in Special Relation to Plague, Proceedings of the Royal Society of medicine, 11 (1918), 85–132. http://doi.org/10.1177/003591571801101305 doi: 10.1177/003591571801101305
![]() |
[21] |
E. S. Herbert, The interpretation of periodicity in disease prevalence, JSTOR, 92 (1929), 34–73. http://doi.org/10.2307/2341437 doi: 10.2307/2341437
![]() |
[22] |
G. Major, The statistical study of infectious diseases, JSTOR, 109 (1946), 85–110. http://doi.org/10.2307/2981176 doi: 10.2307/2981176
![]() |
[23] | TJ. B. Norman, The mathematical theory of infectious diseases and its applications, Charles Griffin & Company Ltd, 5a Crendon Street, High Wycombe, Bucks HP13 6LE., 1975. http://doi.org/10.2307/3009004 |
[24] | M. A. Roy, The population dynamics of infectious diseases: theory and applications, Springer, 2013. http://doi.org/10.2307/4361 |
[25] |
H. R. Thieme, Convergence results and a Poincare-Bendison trichotomy for asymptotical autonomous differential equations, J. Math. Biol., 30 (1992), 755–763. http://doi.org/10.1007/bf00173267 doi: 10.1007/bf00173267
![]() |
[26] | S. Lenhart, JT. Workman, Optimal Control Applied to Biological Models, Chapman and Hall/CRC, London, 2007. http://doi.org/10.1201/9781420011418 |
[27] |
W. Wang, X-Q. Zha, Threshold dynamics for compartment epidemic models in periodic environments, J. Dyn. Differ. Equ., 20 (2008), 699–717. http://doi.org/10.1007/s10884-008-9111-8 doi: 10.1007/s10884-008-9111-8
![]() |
[28] |
SF. Dowel, Seasonal Variation in Host Susceptibility and Cycles of Certain Infectious Diseases, EMERG. INFECT. DIS., 7 (2001), 369–374. http://doi.org/10.3201/eid0703.010301 doi: 10.3201/eid0703.010301
![]() |
[29] |
Z. Shuai, J. A. P. Heesterbeek, P. van den Driessche, Extending the type reproduction number to infectious disease control targeting contacts between types, J. Math. Biol., 67 (2013), 1067–1082. http://doi.org/10.1007/s00285-012-0579-9 doi: 10.1007/s00285-012-0579-9
![]() |
[30] | LS. Pontryagin, VT. Boltyanskii, RV. Gamkrelidze, EF. Mishcheuko, The mathematical theory of optimal processes, Wiley, New Jersey, 1962. http://doi.org/10.1057/jors.1965.92 |
[31] |
P. Van den Driessche, J. Watmough, Reproduction number and subthreshold endemic equilibria for compartment models of disease transmission, Math. Biosci., 180, (2002), 29–48. https://doi.org/10.1016/S0025-5564(02)00108-6 doi: 10.1016/S0025-5564(02)00108-6
![]() |
[32] |
M. Saeedian, M. Khalighi, N. A.-Tafreshi, GR. Jafari, A. Marcel, Memory effects on epidemic evolution: The susceptible-infected-recovered epidemic model, Phys. Rev. E, 95 (2017), 022409. http://doi.org/10.1103/physreve.95.022409 doi: 10.1103/physreve.95.022409
![]() |
[33] |
FA. Rihan, QM. A-Mdallal, HJ. AlSakaji, A. H. Adel, A fractional-order epidemic model with time-delay and nonlinear incidence rate, Chaos, Solitons & Fractals, 126 (2019), 97–105. http://doi.org/10.1016/j.chaos.2019.05.039 doi: 10.1016/j.chaos.2019.05.039
![]() |
[34] |
H. Nur'Izzati, K. Adem, Analysis of the fractional order dengue transmission model: a case study in Malaysia, Adv. Differ. Equations, 1 (2019), 1–13. http://doi.org/10.1186/s13662-019-1981-z doi: 10.1186/s13662-019-1981-z
![]() |
[35] | M. Abderrahim, B. Adnane, H. Khalid, Y. Noura, A fractional order SIR epidemic model with nonlinear incidence rate, Springer, 2018. http://doi.org/10.1186/s13662-018-1613-z |
[36] |
M. Awais, F. S. Alshammari, S. Ullah, M. A. Khan, S. Islam, Modeling and simulation of the novel coronavirus in Caputo derivative, Results Phys., 19 (2020), 103588. http://doi.org/10.1016/j.rinp.2020.103588 doi: 10.1016/j.rinp.2020.103588
![]() |
[37] |
B. Samia, S. Tareq, FM. T. Delfim, Z. Anwar, Control of COVID-19 dynamics through a fractional-order model, Alex. Eng. J., 60 (2020), 3587–3592. http://doi.org/10.1016/j.aej.2021.02.022 doi: 10.1016/j.aej.2021.02.022
![]() |
[38] |
O.-M. Isaac, A. Lanre, O.Bismark, S. I. Olaniyi, A fractional order approach to modeling and simulations of the novel COVID-19, Adv. Differ. Equations, 1 (2020), 1–21. http://doi.org/10.1186/s13662-020-03141-7 doi: 10.1186/s13662-020-03141-7
![]() |
[39] |
A. Idris, B. I. Abdullahi, Y. Abdullahi, K. Poom, K. Wiyada, Analysis of Caputo fractional-order model for COVID-19 with lockdown, Adv. Differ. Equations, 2020 (2020), 394. http://doi.org/10.1186/s13662-020-02853-0 doi: 10.1186/s13662-020-02853-0
![]() |
[40] |
B. B. Abdullahi, B. Bulent, Optimal control of a fractional order model for the COVID–19 pandemic, Chaos, Solitons & Fractals, 144 (2021), 110678. http://doi.org/10.1016/j.chaos.2021.110678 doi: 10.1016/j.chaos.2021.110678
![]() |
[41] |
A. Omame, M. Abbas, CP. Onyenegecha, A fractional-order model for COVID-19 and tuberculosis co-infection using Atangana–Baleanu derivative, Chaos, Solitons & Fractals, 153 (2021), 111486 http://doi.org/10.1016/j.chaos.2021.111486 doi: 10.1016/j.chaos.2021.111486
![]() |
[42] |
A. Muhammad, F. Muhammad, A. Ali, S. Meng, Modeling and simulation of fractional order COVID-19 model with quarantined-isolated people, Math. Methods Appl. Sci., 44 (2021), 6389–6405. http://doi.org/10.1002/mma.7191 doi: 10.1002/mma.7191
![]() |
[43] |
A. Ul Rehman, R. Singh, Ram, P. Agarwal, Modeling, analysis and prediction of new variants of covid-19 and dengue co-infection on complex network, Chaos, Solitons & Fractals, 150 (2021), 111008. http://doi.org/10.1016/j.chaos.2021.111008 doi: 10.1016/j.chaos.2021.111008
![]() |
[44] |
R. Singh, P. Tiwari, S. S. Band, A. Ul Rehman, S. Mahajan, Y. Ding, et al., Impact of quarantine on fractional order dynamical model of Covid-19, Comput. Biol. Med., 151 (2022), 106266. http://doi.org/10.1016/j.compbiomed.2022.106266 doi: 10.1016/j.compbiomed.2022.106266
![]() |
[45] |
P. Agarwal, R. Singh, A. Ul Rehman, Numerical solution of hybrid mathematical model of dengue transmission with relapse and memory via Adam–Bashforth–Moulton predictor-corrector scheme, Chaos, Solitons & Fractals, 143 (2021), 110564. http://doi.org/10.1016/j.chaos.2020.110564 doi: 10.1016/j.chaos.2020.110564
![]() |
[46] |
N. Sharma, R. Singh, J. Singh, O. Castillo, Modeling assumptions, optimal control strategies and mitigation through vaccination to zika virus, Chaos, Solitons & Fractals, 150 (2021), 111137. http://doi.org/10.1016/j.chaos.2021.111137 doi: 10.1016/j.chaos.2021.111137
![]() |
[47] |
A. Nursanti, B. L. Kalvein, Modeling of COVID-19 spread with self-isolation at home and hospitalized classes, Results Phys., 36 (2022), 105378. http://doi.org/10.1016/j.rinp.2022.105378 doi: 10.1016/j.rinp.2022.105378
![]() |
[48] |
P. O. Lolika, M. Helikumi, Global stability analysis of a COVID-19 epidemic model with incubation delay, Math. Model. Control, 3 (2023), 23–38. http://doi.org/10.3934/mmc.2023003 doi: 10.3934/mmc.2023003
![]() |
[49] |
J. Huo, H. Zhao, L. Zhu, The effect of vaccines on backward bifurcation in a fractional order HIV model, NONLINEAR ANAL-REAL., 26 (2015), 289–305. http://doi.org/10.1016/j.nonrwa.2015.05.014 doi: 10.1016/j.nonrwa.2015.05.014
![]() |
[50] |
P. A. Naik, J. Zu, K. M. Owolabi, Global dynamics of a fractional order model for the transmission of HIV epidemic with optimal control, Chaos, Solitons & Fractals, 138 (2020), 109826. http://doi.org/10.1016/j.chaos.2020.109826 doi: 10.1016/j.chaos.2020.109826
![]() |
[51] |
K. M. Owolabi, Behavioural study of symbiosis dynamics via the Caputo and Atangana–Baleanu fractional derivatives, Chaos, Solitons & Fractals, 122 (2019), 89–101. http://doi.org/10.1016/j.chaos.2019.03.014 doi: 10.1016/j.chaos.2019.03.014
![]() |
[52] |
K. M. Owolabi, A. Atangana, Mathematical analysis and computational experiments for an epidemic system with nonlocal and nonsingular derivative, Chaos, Solitons & Fractals, 126 (2019), 41–49. http://doi.org/10.1016/j.chaos.2019.06.001 doi: 10.1016/j.chaos.2019.06.001
![]() |
[53] |
Z. U. A. Zafar, K. Rehan, M. Mushtaq, HIV/AIDS epidemic fractional-order model, J. Differ. Equations Appl., 23 (2017), 1298–1315. http://doi.org/10.1080/10236198.2017.1321640 doi: 10.1080/10236198.2017.1321640
![]() |
[54] |
H. L. Li, L. Zhang, C. Hu, Y. L. Jiang, Z. Teng, Dynamical analysis of a fractional-order predator-prey model incorporating a prey refuge, J. Appl. Math. Comput., 54 (2017), 435–449. http://doi.org/10.1007/s12190-016-1017-8 doi: 10.1007/s12190-016-1017-8
![]() |
[55] |
D. Barros, L. C. Lopes, M. M. S. Pedro, F. Esmi, E. Santos, D. E. Sánchez, The memory effect on fractional calculus: an application in the spread of COVID-19, Comput. Appl. Math., 40 (2021), 1–21. http://doi.org/10.1007/s40314-021-01456-z doi: 10.1007/s40314-021-01456-z
![]() |
[56] |
V.-De-Leó n, Volterra-type Lyapunov functions for fractional-order epidemic systems, Commun. Nonlinear Sci. Numer. Simul., 24 (2015), 75–85. http://doi.org/10.1016/j.cnsns.2014.12.013 doi: 10.1016/j.cnsns.2014.12.013
![]() |
[57] |
M. Caputo, Linear models of dissipation whose Q is almost frequency independent-Ⅱ, Geophys. J. Int., 13 (1967), 529–539. http://doi.org/10.1111/j.1365-246x.1967.tb02303.x doi: 10.1111/j.1365-246x.1967.tb02303.x
![]() |
[58] |
K. Diethelm, The Analysis of Fractional Differential Equations: An Application-Oriented Exposition using Differential Operators of Caputon type, Lecture Notes in Mathematics, 247 (2010). https://doi.org/10.1007/978-3-642-14574-2 doi: 10.1007/978-3-642-14574-2
![]() |
[59] | I. Podlubny, Fractional Differential Equations, San Diego: Academic Pres, 1999. |
[60] |
H. Delavari, D. Baleanu, J. Sadati, Stability analysis of Caputo fractional-order nonlinear systems revisited, Nonlinear Dyn., 67 (2012), 2433–2439. http://doi.org/10.1007/s11071-011-0157-5 doi: 10.1007/s11071-011-0157-5
![]() |
[61] |
O. Diekmann, J. A. P. Heesterbeek, J. A. J. Metz, On the definition and the computation of the basic reproduction ratioR0 in models for infectious diseases in heterogeneous populations, J. Math. Biol., 28 (1990), 365–382. https://doi.org/10.1007/BF00178324 doi: 10.1007/BF00178324
![]() |
[62] | J. P. LaSalle, The Stability of Dynamical Systems, Society for Industrial and Applied Mathematics, 1976. |
[63] |
H. Mlyashimbi, E. Gideon, M. Steady, Dynamics of a Fractional-Order Chikungunya Model with Asymptomatic Infectious Class, Computational and Mathematical Methods in Medicine, (2022). http://doi.org/10.1155/2022/5118382 doi: 10.1155/2022/5118382
![]() |
[64] |
A. Dénes, B. A. Gumel, Modeling the impact of quarantine during an outbreak of Ebola virus disease, Infectious Disease Modelling, 4 (2019), 12–27. http://doi.org/10.1016/j.idm.2019.01.003 doi: 10.1016/j.idm.2019.01.003
![]() |
[65] |
A. Aatif, U. Saif, M. A. Khan, The impact of vaccination on the modeling of COVID-19 dynamics: a fractional order model, Nonlinear Dynam., (2022), 1–20. http://doi.org/10.1007/s11071-022-07798-5 doi: 10.1007/s11071-022-07798-5
![]() |
[66] | A. Leon, J. Hyman, Forward and adjoint sensitivity analysis with applications in dynamical systems, Lecture Notes in Linear Algebra and Optimization, (2005). |
[67] |
N. Faïçal, A. Iván, J. N. Juan, FM. T. Delfim, Mathematical modeling of COVID-19 transmission dynamics with a case study of Wuhan, Chaos, Solitons & Fractals, 135 (2020), 109846. http://doi.org/10.1016/j.chaos.2020.109846 doi: 10.1016/j.chaos.2020.109846
![]() |
[68] |
F. Wang, L. Cao, X. Song, Mathematical modeling of mutated COVID-19 transmission with quarantine, isolation and vaccination, Math. Biosci. Eng., 19 (2022), 8035–8056. https://doi.org/10.3934/mbe.2022376 doi: 10.3934/mbe.2022376
![]() |
[69] |
Y. B. Ruhomally, M. Mungur, A. A. H. Khoodaruth, V. Oree, M. A. Dauhoo, Assessing the Impact of Contact Tracing, Quarantine and Red Zone on the Dynamical Evolution of the Covid-19 Pandemic using the Cellular Automata Approach and the Resulting Mean Field System: A Case study in Mauritius, Appl. Math. Model., 111 (2022), 567–589. http://doi.org/10.1016/j.apm.2022.07.008 doi: 10.1016/j.apm.2022.07.008
![]() |
[70] |
C. Hou, J. Chen, Y. Zhou, L. Hua, J. Yuan, S. He, et al., The effectiveness of quarantine of Wuhan city against the Corona Virus Disease 2019 (COVID-19): A well-mixed SEIR model analysis, J. Med. Virol., 92 (2020), 841–848. http://doi.org/10.1002/jmv.25827 doi: 10.1002/jmv.25827
![]() |
[71] |
J. Nelder, R. Mead, A simplex method for function minimization, The computer Journal, 7 (1964), 308–313. http://doi.org/10.1093/comjnl/7.4.308 doi: 10.1093/comjnl/7.4.308
![]() |
1. | Ahmed Refaie Ali, Daniyal Ur Rehman, Najeeb Alam Khan, Muhammad Ayaz, Asmat Ara, M. Ijaz Khan, Integrating fractional-order SEI1I2I3QCR model with awareness and non-pharmaceutical interventions for optimal COVID-19 pandemic, 2025, 25, 1471-2288, 10.1186/s12874-024-02452-7 | |
2. | Mohamad Chakroun, Samir Haddad, Wafaa M.R. Shakir, Jinane Sayah, Chadi Kallab, Salah Falou, 2024, Recent Monte Carlo-Based Simulations for Modeling Disease Spread, 979-8-3503-6756-0, 1, 10.1109/ICCA62237.2024.10927993 |
Symbol | Description | Value | Units | Source |
Λ | Per capita human recruitment rate | 11826 | day −1 | [64] |
d | Disease induced death rate | day −1 | 0.0413 | [9] |
μ | Natural death rate | day −1 | 0.2 | [9] |
β | Force of infections | day −1 | Fitted | |
α | Incubation period | day −1 | 0.5171 | [65] |
σ | Average per capita contact rate | day −1 | Fitted | |
ϕ | Efficacy rate of vaccination | day −1 | 0:854 | [64] |
ν1 | Vaccination rate | day −1 | 0.0313 | [65] |
γ | Recovery rate | day−1 | 0.362 | [64] |
ϵ | Human awareness rate | day−1 | Vary | Assumed |
κ | Treatment rate of infected individuals | unit-less | Vary | Assumed |
ω | Quarantine rate of suspected humans | unit-less | Vary | Assumed |
Day | 1 | 2 | 3 | 4 | 5 | 6 | 7 | 8 |
Cases | 6 | 12 | 19 | 25 | 31 | 38 | 44 | 60 |
Day | 9 | 10 | 11 | 12 | 13 | 14 | 15 | 16 |
Cases | 80 | 131 | 259 | 3839 | 469 | 688 | 776 | 1776 |
Day | 17 | 18 | 19 | 20 | 21 | 22 | 23 | |
Cases | 1460 | 1739 | 1984 | 2101 | 2590 | 2827 | 3233 |
Symbol | Description | Value | Units | Source |
Λ | Per capita human recruitment rate | 11826 | day −1 | [64] |
d | Disease induced death rate | day −1 | 0.0413 | [9] |
μ | Natural death rate | day −1 | 0.2 | [9] |
β | Force of infections | day −1 | Fitted | |
α | Incubation period | day −1 | 0.5171 | [65] |
σ | Average per capita contact rate | day −1 | Fitted | |
ϕ | Efficacy rate of vaccination | day −1 | 0:854 | [64] |
ν1 | Vaccination rate | day −1 | 0.0313 | [65] |
γ | Recovery rate | day−1 | 0.362 | [64] |
ϵ | Human awareness rate | day−1 | Vary | Assumed |
κ | Treatment rate of infected individuals | unit-less | Vary | Assumed |
ω | Quarantine rate of suspected humans | unit-less | Vary | Assumed |
Day | 1 | 2 | 3 | 4 | 5 | 6 | 7 | 8 |
Cases | 6 | 12 | 19 | 25 | 31 | 38 | 44 | 60 |
Day | 9 | 10 | 11 | 12 | 13 | 14 | 15 | 16 |
Cases | 80 | 131 | 259 | 3839 | 469 | 688 | 776 | 1776 |
Day | 17 | 18 | 19 | 20 | 21 | 22 | 23 | |
Cases | 1460 | 1739 | 1984 | 2101 | 2590 | 2827 | 3233 |