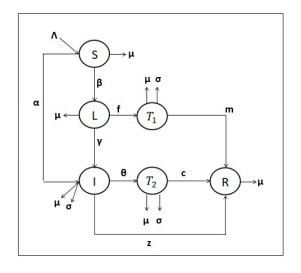
Compared to many infectious diseases, tuberculosis has a high mortality rate. Because of this, a great deal of illustrative research has been done on the modeling and study of tuberculosis using mathematics. In this work, a mathematical model is created by taking into account the underlying presumptions of this disease. One of the main novelties of the paper is to consider two different treatment strategies namely protective treatment for the latent populations from the disease and the main treatment applied to the infected populations. This situation can be regarded as the other novelty of the paper. The susceptible, latent, infected, and recovered populations, as well as the two mentioned treatment classes, are all included in the proposed six-dimensional model's compartmental framework. Additionally, a region that is biologically possible is presented, as well as the solution's positivity, existence, and uniqueness. The suggested model's solutions are carried out as numerical simulations using assumed and literature-based parameter values and analyzing its graphics. To get the results, a fourth-order Runge-Kutta numerical approach is used.
Citation: Mehmet Yavuz, Fatma Özköse, Müzeyyen Akman, Zehra Tuğba Taştan. A new mathematical model for tuberculosis epidemic under the consciousness effect[J]. Mathematical Modelling and Control, 2023, 3(2): 88-103. doi: 10.3934/mmc.2023009
[1] | J. O. Akanni, S. Ajao, S. F. Abimbade, Fatmawati . Dynamical analysis of COVID-19 and tuberculosis co-infection using mathematical modelling approach. Mathematical Modelling and Control, 2024, 4(2): 208-229. doi: 10.3934/mmc.2024018 |
[2] | Erick M. D. Moya, Diego Samuel Rodrigues . A mathematical model for the study of latent tuberculosis under 3HP and 1HP regimens. Mathematical Modelling and Control, 2024, 4(4): 400-416. doi: 10.3934/mmc.2024032 |
[3] | Oluwatayo Michael Ogunmiloro . A fractional order mathematical model of teenage pregnancy problems and rehabilitation in Nigeria. Mathematical Modelling and Control, 2022, 2(4): 139-152. doi: 10.3934/mmc.2022015 |
[4] | K. O. Achema, D. Okuonghae, C. J. Alhassan . A mathematical model for assessing the impact of dual-level toxicity on aquatic biospecies and its optimal control analysis. Mathematical Modelling and Control, 2022, 2(3): 100-121. doi: 10.3934/mmc.2022012 |
[5] | S. Y. Tchoumi, Y. Kouakep-Tchaptchie, D. J. Fotsa-Mbogne, J. C. Kamgang, J. M. Tchuenche . Optimal control of a malaria model with long-lasting insecticide-treated nets. Mathematical Modelling and Control, 2021, 1(4): 188-207. doi: 10.3934/mmc.2021018 |
[6] | Ankur Jyoti Kashyap, Arnab Jyoti Bordoloi, Fanitsha Mohan, Anuradha Devi . Dynamical analysis of an anthrax disease model in animals with nonlinear transmission rate. Mathematical Modelling and Control, 2023, 3(4): 370-386. doi: 10.3934/mmc.2023030 |
[7] | Paride O. Lolika, Mlyashimbi Helikumi . Global stability analysis of a COVID-19 epidemic model with incubation delay. Mathematical Modelling and Control, 2023, 3(1): 23-38. doi: 10.3934/mmc.2023003 |
[8] | Yaxin Ren, Yakui Xue . Modeling and optimal control of COVID-19 and malaria co-infection based on vaccination. Mathematical Modelling and Control, 2024, 4(3): 316-335. doi: 10.3934/mmc.2024026 |
[9] | Elijah B. Baloba, Baba Seidu . A mathematical model of anthrax epidemic with behavioural change. Mathematical Modelling and Control, 2022, 2(4): 243-256. doi: 10.3934/mmc.2022023 |
[10] | Anil Chavada, Nimisha Pathak, Sagar R. Khirsariya . A fractional mathematical model for assessing cancer risk due to smoking habits. Mathematical Modelling and Control, 2024, 4(3): 246-259. doi: 10.3934/mmc.2024020 |
Compared to many infectious diseases, tuberculosis has a high mortality rate. Because of this, a great deal of illustrative research has been done on the modeling and study of tuberculosis using mathematics. In this work, a mathematical model is created by taking into account the underlying presumptions of this disease. One of the main novelties of the paper is to consider two different treatment strategies namely protective treatment for the latent populations from the disease and the main treatment applied to the infected populations. This situation can be regarded as the other novelty of the paper. The susceptible, latent, infected, and recovered populations, as well as the two mentioned treatment classes, are all included in the proposed six-dimensional model's compartmental framework. Additionally, a region that is biologically possible is presented, as well as the solution's positivity, existence, and uniqueness. The suggested model's solutions are carried out as numerical simulations using assumed and literature-based parameter values and analyzing its graphics. To get the results, a fourth-order Runge-Kutta numerical approach is used.
Humanity has fought epidemics for centuries, and millions of people have lost their lives. From time to time, these outbreaks manifest themselves in the form of plague, smallpox, HIV/AIDS, SARS, avian flu, and influenza. One of these epidemics is tuberculosis (TB) which is popularly known. Tuberculosis is a long-term bacterial and infectious disease caused by the microbe "mycobacterium tuberculosis (MTB)". The way of transmission is often caused by sputum that a tuberculosis patient spits into the environment, or by Bacillus-laden droplets that are scattered when coughing. Although tuberculosis is an infection that can occur in more than one organ, it is mainly observed in the lungs and mediastinal lymph nodes of the lungs. Bacillus is emitted from an active tuberculosis patient by coughing, sneezing, or other means through droplets into the air in saliva, and the infection is spread by removing particles suspended in the air. It is one of the oldest known diseases, that can be maintained, although still continues to be one of the world's most common and deadly infectious diseases, and more than three million people per year are died due to TB [1]. People who experience tuberculosis can continue their healthy lives for months without any symptoms. During this period, the person's immune system tries to prevent the development of the disease by fighting against the MTB bacterium. But in cases where the immune system cannot show sufficient resistance, tuberculosis microbes become active and tuberculosis disease occurs [2].
24th of March is commemorated every year as "World Tuberculosis Day", dedicated to the day of March 24, 1882, when MTB Bacillus was discovered by Robert Koch in order to end the global TB epidemic and paved the way for the diagnosis and treatment of the disease. About a quarter of the world's population has been infected with tuberculosis Bacillus. The increase in HIV/AIDS infection in the world also increases the global threat of TB. 30.000 people are being infected with tuberculosis and 4.500 of them die due to tuberculosis every day. In the world, 54 million lives have been saved and tuberculosis has been reduced by 42% by global efforts since 2000. The World Health Organization (WHO) has implemented its global tuberculosis eradication strategy and the WHO European Region Tuberculosis Action Plan 2016-2020, identifying the steps to be taken after 2015. In this context, it is aimed to reduce TB incidence by 90% all over the world by 2030 [3].
The first mathematical model given by Waaler et al. [4] was established by taking into account relationships with other social sciences, and the potentials of this model are shown in three examples that show how the model can help predict the tendency of tuberculosis, either in a given state, which develops spontaneously suddenly or under the influence of certain control programs. In particular, these examples emphasize that the model can be profitable in evaluating specific control programs, reflecting the effects of tuberculosis on its natural tendency. Schulzer et al. (1994) [5] presented a mathematical model to study the accelerating effect of HIV infection on TB disease, while Castillo-Chavez and Feng (1997) [6] revealed differences between two TB individuals with and without drug-resistant TB. In another study, it was stated that the effect of exogen on the qualitative dynamics of TB was too great [7]. Monte-Carlo simulations were conducted to determine the likelihood that 10,000 clinical patients receiving different doses of moxifloxacin could reach or exceed the point of exposure to the drug required to suppress their resistance to moxifloxacin in TB [8]. In addition, it was concluded that advanced TB diagnostic techniques have a significant impact on t-related disease and death rates in HIV endemic areas, and it was emphasized that as TB rates continue to increase, advanced diagnostic techniques should be considered as TB control strategies [9].
Analysis of the mathematical model created in research on multidrug-resistant (CID) and common drug-resistant (YID) strains in South Africa, the region with the highest TB rate worldwide, has yielded important results for the next 10 years. It showed that the spread of TB culture and drug sensitivity among adults in South Africa could save more than 47.000 lives and prevent more than 7.000 cases of CID-TB in the period from 2008 to 2017. This corresponds to a 17% decrease in total TB deaths and a 47% decrease in CID-TB deaths [10]. Bowong and Tewa (2009) [11] proved that the TB system they studied was asymptotically globally stable and has a single stable equilibrium, and showed that depending on the basic reproductive rate, this stable structure occurs either in a regional disease state or in disease-free situations. Aparicio and Castillo-Chavez (2009) analyzed a TB model they established on three separate classes: a homogeneous mixture with the probability of standard virus hit, a non-homogeneous mixture containing "household-household" contacts, and a mixture grouped by age, and discussed the factors affecting TB. From these factors, they examined in detail the effects of population growth, stochasticism, clustering of contacts, and age structure on disease dynamics. In a study where a new deterministic mathematical model was created, Liu and Zhang (2011) [12] described the effects of vaccination and treatment on the spread of tuberculosis. Tewa et al. (2012) [13] took into account that it is possible for TB-sensitive individuals to switch from one part to another, and proved the existence-uniqueness of related endemic balances in quadratic forms using the Lyapunov function. Trauer et al. [14] The model presented by (2014) simulated program-based responses to tuberculosis in extreme endemic countries in the Asia-Pacific region and stated that the model could not be adjusted according to the predicted rate of a hit without allowing reinfection during the delay. In another study, by adding vaccine parameters to the mathematical model, it was aimed to infect the least people among susceptible individuals and to minimize the number of infected individuals [15].
January 2005 December 2012 in a mathematical model created with a focus on tuberculosis data in China [16] fit the relevant data and thus determined the optimal parameter values of the model using the chi-square test; with these parameters, they calculated the effective number of disease reproduction each year. Dodd et al. (2016) [17], in their study of children in the 22 countries where TB cases are most common, they set a benchmark for the rates of tuberculosis cases at the country level and developed recommendations to prevent TB cases at these rates. In another study [18], a model describing the impact of a public health education campaign for tuberculosis and comparing it with data from Senegal was developed. Taking into account the results found, they suggested that some data could be added to the model, such as chronological age and/or spatial structure, which takes into account sensitivity by age, to observe the difference between the approaches of individuals in rural and urban areas to TB. Vinh et al. (2018) [19] the passage of the virus in the world is one of the cities most likely HIV/AIDS and TB happens very fast synchronization between the dynamics of a city, Ho Chi Minh City (HCMC-Vietnam). In their study, a mathematical model to integrate many data sources HCMC the dynamics of TB was explored. This study was the first detailed study on TB at HCMC and provides information on TB dynamics at HCMC from 1996 to 2005. In [20], the authors considered two different treatment strategies: one of them is to point out the impact of treating latent TB infection (LTBI) in the elderly in addition to current TB control strategies. The other one is the treatment of infected individuals with anti-TB drugs. A lot of modeling studies have also been conducted in relation to significant area of science and infectious illnesses including COVID-19 [21,22,23,24,25,26,27,28,29,30,31,32], cancer cells-cancer stem cells [33], optimal control and bifurcation [34], alcoholism [35], cholera [36,37], Parkinson's disease [38], disturbance effect in intracellular calcium dynamic on fibroblast cells [39], HIV [40,41], babesiosis [42], predator-prey [43,44], viral system with the non-cytolytic immune assumption [45], Nipah virus [46], an epidemic model with general interference function and high-order perturbation [47], potential scenarios for wastewater treatment [48], Gompertz growth model [49], a general epidemic model with logistic growth [50], synchronization [51], a sewage treatment model [52].
In the present TB model, population compartments consist of six populations: Susceptible (S), Latent (L), Treatment for latently infected individuals (T1), Infected (I), Treatment for the actively infected individuals (T2) and Recovered (R) which mean that Susceptible: Individuals susceptible to disease, Latent: those who are latently infected by tuberculosis, that is, individuals who do not experience the disease actively, Treatment 1: Preventive treatment applied optionally to patients with latent tuberculosis, that is, conscious individuals who accept this treatment, Infected: Individuals who are actively living the disease with reduced body resistance, Treatment 2: Curative treatment applied to infected individuals, Recovered: Individuals who have survived tuberculosis disease. In Tuberculosis (TB) model that we have constructed, the Λ parameter includes all people, and a certain part of them are thought to be susceptible to the disease. In other words, the state of being susceptible to this disease is not considered for all people. Because some people completely isolate themselves from society. For this reason, these individuals do not get the disease. There is also a protective treatment (consciousness) compartment that covers conscious individuals, considering that it will play a significant role in ending the disease. Transitions between compartments depend on the proportions which fall into the range [0.1]. In this model, from the susceptible compartment at the rate of β to the hidden TB compartment, and also from the susceptible compartment at the rate of α, individuals switch to the infected compartment. Although the hidden TB compartment has high body resistance and contains the virus in its body, it has not yet actively experienced the disease and cannot transmit it to other individuals in the society where it is located. For this reason, protective treatment is applied to the individuals in this compartment to ensure that the recovered individuals are transferred to the compartment in which they are stated. Individuals who accepted this treatment have been also considered as conscious individuals. Protective treatment has been applied to individuals in the ratio of f individuals in the hidden TB compartment, and individuals in the ratio of m have given positive responses to the treatment and moved to the recovered compartment.
Infected individuals live actively and can infect other people. At the same time, the disease becomes active due to the decrease in body resistance of individuals at the rate of γ from the hidden TB compartment and passes to the compartment where the infected individuals are located. Individuals at the rate of z in the infected compartment can join those who recover without any treatment method. Meanwhile, by applying the main treatment method to the infected individuals at the ratio of θ, individuals at the rate of c in this compartment join the individuals who have overcome the disease and recovered. Normal death occurs in the rate of μ in all of these compartments, but death from disease occurs in the fraction of σ in the individuals I, T1 and T2 compartments as shown in Figure 1.
The model is therefore given by the following set of ordinary differential equations:
dSdt=Λ−(βL+αI+μ)S,dLdt=βSL−(γI+f+μ)L,dT1dt=fL−(m+μ+σ)T1,dIdt=γLI+αSI−(θ+z+μ+σ)I,dT2dt=θI−(c+μ+σ)T2,dRdt=mT1+cT2+zI−μR, | (2.1) |
subject to the initial conditions (IC),
S(0)=S0,L(0)=L0,T1(0)=T10,I(0)=I0,T2(0)=T20,R(0)=R0, | (2.2) |
where (S(t),L(t),T1(t),I(t),T2(t),R(t))∈R6+. The functions S(t), L(t), T1(t), I(t), T2(t), R(t) and their derivatives are considered to be continuous at t≥0 in this situation.
The positivity and boundedness of the solution for the recommended model (2.1) are given in this section. The existence conditions and stability findings for the equilibria are then given.
We begin with the following theorem to this subsection:
Theorem 1. The proposed model (2.1)'s solution set {S(t),L(t),T1(t),I(t),T2(t),R(t)} combined with the IC (2.2), is non-negative for all t>0.
Proof 1. According to the study's suggestion [55], we evaluate the first equation while accounting for the nonlinear system of equations (2.1):
dSdt=Λ−(βL+αI+μ)S, | (3.1) |
which means that
dSdt≥−(βL+αI+μ)S. | (3.2) |
By integrating Equation (3.2) and using the exponential growth condition, we get
S(t)≥S(0)e−(βL+αI+μ)t, | (3.3) |
this gives
S(t)≥0. | (3.4) |
Theorem 2. In area A⊂R6+, given by following, the solutions of system (2.1) with IC (2.2) are specified:
A={(S(t),L(t),T1(t),I(t),T2(t),R(t))∈R6+| N(t)≤Λμ}. | (3.5) |
Proof 2. By taking the total population, we have
dN(t)dt=dS(t)dt+dL(t)dt+dT1(t)dt+dI(t)dt+dT2(t)dt+dR(t)dt. | (3.6) |
Then we have the following for the whole population
dNdt=Λ−μN−σ(T1+T2)≤Λ−μN. | (3.7) |
Equation (3.7)'s solution is presented as
N(t)≤Λμ−(Λμ−N0)e−μt, | (3.8) |
where N0=N(0) is the definition of the beginning population. With the aid of the Birkhoff-Rota theorem, we can state that if N0<Λμ, then as t→∞, asymptotically N(t)→Λμ in Eq (3.5), and the overall population size becomes N(t)→Λμ, then 0≤N≤Λμ. As a result, region A is where all of the model's viable solutions converge [57].
Firstly, we start by the following Volterra-type integral equations:
S(t)−S(0)=∫t0[Λ−(βL(τ)+αI(τ)+μ)S(τ)]dτ,L(t)−L(0)=∫t0[βS(τ)L(τ)−(γI(τ)+f+μ)L(τ)]dτ,T1(t)−T1(0)=∫t0[fL(τ)−(m+μ+σ)T1(τ)]dτ,I(t)−I(0)=∫t0[γL(τ)I(τ)+αS(τ)I(τ)−(θ+z+μ+σ)I(τ)]dτ,T2(t)−T2(0)=∫t0[θI(τ)−(c+μ+σ)T2(τ)]dτ,R(t)−R(0)=∫t0[mT1(τ)+cT2(τ)+zI(τ)−μR(τ)]dτ. | (3.9) |
Let us define the following kernels as
φ1(t,S)=[Λ−(βL(t)+αI(t)+μ)S(t)],φ2(t,L)=[βS(t)L(t)−(γI(t)+f+μ)L(t)],φ3(t,T1)=[fL(t)−(m+μ+σ)T1(t)],φ4(t,I)=[γL(t)I(t)+αS(t)I(t)−(θ+z+μ+σ)I(t)],φ5(t,T2)=[θI(t)−(c+μ+σ)T2(t)],φ6(t,R)=[mT1(t)+cT2(t)+zI(t)−μR(t)]. | (3.10) |
The following theorem then emerges:
Theorem 3. If the following inequality is confirmed, then φ1,φ2,φ3,φ4,φ5 and φ6 are in accordance with the Lipschitz assumptions and contractions:
0≤q1,q2,q3,q4,q5,q6<1, | (3.11) |
where, ‖S‖≤m1, ‖L‖≤m2, ‖T1‖≤m3, ‖I‖≤m4, ‖T2‖≤m5, ‖R‖≤m6, q1=βm2+αm4+μ, q2=βm1+γm4+f+μ, q3=m+μ+σ, q4=γm2+αm1+θ+z+μ+σ, q5=c+μ+σ and q6=μ.
Proof 3. Let S1 and S2 be two functions for the kernel φ1; L1 and L2 be two functions for the kernel φ2; T11 and T12 be two functions for the kernel φ3; I1 and I2 be two functions for the kernel φ4; T21 and T22 be two functions for the kernel φ5; and R1 and R2 be two functions for the kernel φ6. Then we have
‖φ1(t,S1)−φ1(t,S2)‖=‖[Λ−(βL+αI+μ)S1]−[Λ−(βL+αI+μ)S2]‖≤(βm2+αm4+μ))‖S1−S2‖=q1‖T1−T2‖,‖φ2(t,L1)−φ2(t,L2)‖=‖[βSL1−(γI+f+μ)L1]−[βSL2−(γI+f+μ)L2]‖≤(βm1+γm4+f+μ)‖L1−L2‖=q2‖L1−L2‖,‖φ3(t,T11)−φ3(t,T12)‖=‖[fL−(m+μ+σ)T11]−[fL−(m+μ+σ)T12]‖≤(m+μ+σ)‖T11−T12‖=q3‖T11−T12‖,‖φ4(t,I1)−φ4(t,I2)‖=‖[γLI1+αSI1−(θ+z+μ+σ)I1]−[γLI2+αSI2−(θ+z+μ+σ)I2]‖≤(γm2+αm1+θ+z+μ+σ)‖I1−I2‖=q4‖I1−I2‖,‖φ5(t,T21)−φ5(t,T22)‖=‖[θI−(c+μ+σ)T21]−[θI−(c+μ+σ)T22]‖≤(c+μ+σ)‖T21−T22‖=q5‖T21−T22‖, | (3.12) |
and
‖φ6(t,R1)−φ6(t,R2)‖=‖[mT1+cT2+zI−μR1]−[mT1+cT2+zI−μR2]‖≤μ‖R1−R2‖=q6‖R1−R2‖. |
As a result, kernels φ1, φ2, φ3, φ4, φ5 and φ6 satisfy the Lipschitz requirements, and if 0≤q1,q2,q3,q4,q5,q6<1, then q1,q2,q3,q4,q5 and q6 is likewise a contraction of kernels φ1, φ2, φ3, φ4, φ5 and φ6, respectively. It establishes the theorem.
In light of kernels φ1, φ2, φ3, φ4, φ5 and φ6, the system described in Eq (3.9) can be rewritten as follows:
S(t)=S(0)+∫t0φ1(τ,S)dτ,L(t)=L(0)+∫t0φ2(τ,L)dτ,T1(t)=T1(0)+∫t0φ3(τ,T1)dτ,I(t)=I(0)+∫t0φ4(τ,I)dτ,T2(t)=T2(0)+∫t0φ5(τ,T2)dτ,R(t)=R(0)+∫t0φ6(τ,R)dτ. | (3.13) |
We can proceed with the following recursive formula
Sn(t)=S(0)+∫t0φ1(τ,Sn−1)dτ,Ln(t)=L(0)+∫t0φ2(τ,Ln−1)dτ,T1n(t)=T1(0)+∫t0φ3(τ,T1n−1)dτ,In(t)=I(0)+∫t0φ4(τ,In−1)dτ,T2n(t)=T2(0)+∫t0φ5(τ,T2n−1)dτ,Rn(t)=R(0)+∫t0φ6(τ,Rn−1)dτ, | (3.14) |
where S0(t)=S(0), L0(t)=L(0), T10(t)=T1(0), I0(t)=I(0), T20(t)=T2(0) and R0(t)=R(0). Then we can write
Ψn(t)=Sn(t)−Sn−1(t)=∫t0[φ1(τ,Sn−1)−φ1(τ,Sn−2)]dτ,Δn(t)=Ln(t)−Ln−1(t)=∫t0[φ2(τ,Ln−1)−φ2(τ,Ln−2)]dτ,Φn(t)=T1n(t)−T1n−1(t)=∫t0[φ3(τ,T1n−1)−φ3(τ,T1n−2)]dτ,Πn(t)=In(t)−In−1(t)=∫t0[φ4(τ,In−1)−φ4(τ,In−2)]dτ,Θn(t)=T2n(t)−T2n−1(t)=∫t0[φ5(τ,T2n−1)−φ5(τ,T2n−2)]dτ,Ξn(t)=Rn(t)−Rn−1(t)=∫t0[φ6(τ,Rn−1)−φ6(τ,Rn−2)]dτ, | (3.15) |
where Sn(t)=∑nj=1Ψn(t), Ln(t)=∑nj=1Δn(t), T1n(t)=∑nj=1Φn(t), In(t)=∑nj=1Πn(t), T2n(t)=∑nj=1Θn(t) and Rn(t)=∑nj=1Ξn(t). By taking the norm of both sides of Eq (3.15), we have
‖Ψn(t)‖=‖Sn(t)−Sn−1(t)‖≤‖∫t0[φ1(τ,Sn−1)−φ1(τ,Sn−2)]dτ‖,‖Δn(t)‖=‖Ln(t)−Ln−1(t)‖≤‖∫t0[φ2(τ,Ln−1)−φ2(τ,Ln−2)]dτ‖,‖Φn(t)‖=‖T1n(t)−T1n−1(t)‖≤‖∫t0[φ3(τ,T1n−1)−φ3(τ,T1n−2)]dτ‖,‖Πn(t)‖=‖In(t)−In−1(t)‖≤‖∫t0[φ4(τ,In−1)−φ4(τ,In−2)]dτ‖,‖Θn(t)‖=‖T2n(t)−T2n−1(t)‖≤‖∫t0[φ5(τ,T2n−1)−φ5(τ,T2n−2)]dτ‖,‖Ξn(t)‖=‖Rn(t)−Rn−1(t)‖≤‖∫t0[φ6(τ,Rn−1)−φ6(τ,Rn−2)]dτ‖. | (3.16) |
Since the kernels satisfy the Lipschitz condition (see Theorem 3), we get
‖Sn(t)−Sn−1(t)‖≤q1∫t0‖Sn−1−Sn−2‖dτ,‖Ln(t)−Ln−1(t)‖≤q2∫t0‖Ln−1−Ln−2‖dτ,‖T1n(t)−T1n−1(t)‖≤q3∫t0‖T1n−1−T1n−2‖dτ,‖In(t)−In−1(t)‖≤q4∫t0‖In−1−In−2‖dτ,‖T2n(t)−T2n−1(t)‖≤q5∫t0‖T2n−1−T2n−2‖dτ,‖Rn(t)−Rn−1(t)‖≤q6∫t0‖Rn−1−Rn−2‖dτ. | (3.17) |
Then, using the final inequality, we arrive at:
‖Ψn(t)‖≤q1∫t0‖Ψn−1(τ)‖dτ,‖Δn(t)‖≤q2∫t0‖Δn−1(τ)‖dτ,‖Φn(t)‖≤q3∫t0‖Φn−1(τ)‖dτ,‖Πn(t)‖≤q4∫t0‖Πn−1(τ)‖dτ,‖Θn(t)‖≤q5∫t0‖Θn−1(τ)‖dτ,‖Ξn(t)‖≤q6∫t0‖Ξn−1(τ)‖dτ. | (3.18) |
We derive the following theorem from these findings.
Theorem 4. The suggested TB model has a solution under the presumption that we get tmax holding:
qitmax<1, i=1,2,3,4,5,6. | (3.19) |
Proof 4. Taking into account the functions S(t), L(t), T1(t), I(t), T2(t) and R(t) are bounded and their kernels φ1, φ2, φ3, φ4, φ5 and φ6 hold the Lipschitz condition, we can give the following by taking Eq (3.18) into account,
‖Ψn(t)‖≤‖S0(t)‖{q1tmax}n,‖Δn(t)‖≤‖L0(t)‖{q2tmax}n,‖Φn(t)‖≤‖T10(t)‖{q3tmax}n,‖Πn(t)‖≤‖I0(t)‖{q4tmax}n,‖Θn(t)‖≤‖T20(t)‖{q5tmax}n,‖Ξn(t)‖≤‖R0(t)‖{q6tmax}n. | (3.20) |
The functions in Eq (3.20) are now demonstrated to be the solutions of the specified TB model. We believe
S(t)−S(0)=Sn(t)−ωn(t),L(t)−L(0)=Ln(t)−ϑn(t),T1(t)−T1(0)=T1n(t)−ϰn(t),I(t)−I(0)=In(t)−εn(t),T2(t)−T2(0)=T2n(t)−ςn(t),R(t)−R(0)=Rn(t)−ϱn(t). | (3.21) |
Then we now present that the terms stated in Eq (3.21) maintain that ‖ω∞(t)‖→0, ‖ϑ∞(t)‖→0, ‖ϰ∞(t)‖→0, ‖ε∞(t)‖→0, ‖ς∞(t)‖→0 and ‖ϱ∞(t)‖→0. Because of having
‖ωn(t)‖≤‖∫t0[φ1(τ,S)−φ1(τ,Sn−1)]dτ‖≤∫t0‖φ1(τ,S)−φ1(τ,Sn−1)‖dτ≤tq1‖S−Sn−1‖,‖ϑn(t)‖≤‖∫t0[φ2(τ,L)−φ2(τ,Ln−1)]dτ‖≤∫t0‖φ2(τ,L)−φ2(τ,Ln−1)‖dτ≤tq2‖L−Ln−1‖,‖ϰn(t)‖≤‖∫t0[φ3(τ,T1)−φ3(τ,T1n−1)]dτ‖≤∫t0‖φ3(τ,T1)−φ3(τ,T1n−1)‖dτ≤tq3‖T1−T1n−1‖,‖εn(t)‖≤‖∫t0[φ4(τ,I)−φ4(τ,In−1)]dτ‖≤∫t0‖φ4(τ,I)−φ4(τ,In−1)‖dτ≤tq4‖I−In−1‖,‖ςn(t)‖≤‖∫t0[φ5(τ,T2)−φ5(τ,T2n−1)]dτ‖≤∫t0‖φ5(τ,T2)−φ5(τ,T2n−1)‖dτ≤tq5‖T2−T2n−1‖, | (3.22) |
and
‖ϱn(t)‖≤‖∫t0[φ6(τ,R)−φ6(τ,Rn−1)]dτ‖≤∫t0‖φ6(τ,R)−φ6(τ,Rn−1)‖dτ≤tq6‖R−Rn−1‖, |
recursively carrying out this procedure, we obtain
‖ωn(t)‖≤{t}n+1qn1Υ,‖ϑn(t)‖≤{t}n+1qn2Υ,‖ϰn(t)‖≤{t}n+1qn3Υ,‖εn(t)‖≤{t}n+1qn4Υ,‖ςn(t)‖≤{t}n+1qn5Υ, |
and
‖ϱn(t)‖≤{t}n+1qn6Υ. |
Considering these last two inequalities at tmax point, we have
‖ωn(t)‖≤{tmax}n+1qn1Υ,‖ϑn(t)‖≤{tmax}n+1qn2Υ,‖ϰn(t)‖≤{tmax}n+1qn3Υ,‖εn(t)‖≤{tmax}n+1qn4Υ,‖ςn(t)‖≤{tmax}n+1qn5Υ, |
and
‖ϱn(t)‖≤{tmax}n+1qn6Υ. |
The final step is taken, after applying the limit on both sides of the final inequalities as n→∞, and by taking into account the theorem's 3.3 conclusions, we get ‖ω∞(t)‖→0, ‖ϑ∞(t)‖→0, ‖ϰ∞(t)‖→0, ‖ε∞(t)‖→0, ‖ς∞(t)‖→0 and ‖ϱ∞(t)‖→0.
Theorem 5. The TB model constructed in the paper has a unique solution.
Proof 5. Suppose that there is a different systemic solution, such as S1(t), L1(t), T11(t), I1(t), T21(t) and R1(t). After that we get
S(t)−S1(t)=∫t0[φ1(τ,S)−φ1(τ,S1)]dτ,L(t)−L1(t)=∫t0[φ2(τ,L)−φ2(τ,L1)]dτ,T1(t)−T11(t)=∫t0[φ3(τ,T1)−φ3(τ,T11)]dτ,I(t)−I1(t)=∫t0[φ4(τ,I)−φ4(τ,I1)]dτ,T2(t)−T21(t)=∫t0[φ5(τ,T2)−φ5(τ,T21)]dτ,R(t)−R1(t)=∫t0[φ6(τ,R)−φ6(τ,R1)]dτ. | (3.23) |
When both sides of Eq (3.23) are subjected to the norm, we get
‖S(t)−S1(t)‖≤∫t0‖φ1(τ,S)−φ1(τ,S1)‖dτ,‖L(t)−L1(t)‖≤∫t0‖φ2(τ,L)−φ2(τ,L1)‖dτ,‖T1(t)−T11(t)‖≤∫t0‖φ3(τ,T1)−φ3(τ,T11)‖dτ,‖I(t)−I1(t)‖≤∫t0‖φ4(τ,I)−φ4(τ,I1)‖dτ,‖T2(t)−T21(t)‖≤∫t0‖φ5(τ,T2)−φ5(τ,T21)‖dτ,‖R(t)−R1(t)‖≤∫t0‖φ6(τ,R)−φ6(τ,R1)‖dτ. | (3.24) |
As a result of the kernels φ1, φ2, φ3, φ4, φ5 and φ6 satisfying the Lipschitz criterion, we can write
‖S(t)−S1(t)‖≤q1t‖S(t)−S1(t)‖,‖L(t)−L1(t)‖≤q2t‖L(t)−L1(t)‖,‖T1(t)−T11(t)‖≤q3t‖T1(t)−T11(t)‖,‖I(t)−I1(t)‖≤q4t‖I(t)−I1(t)‖,‖T2(t)−T21(t)‖≤q5t‖T2(t)−T21(t)‖,‖R(t)−R1(t)‖≤q6t‖R(t)−R1(t)‖, | (3.25) |
which gives
‖S(t)−S1(t)‖(1−q1t)≤0,‖L(t)−L1(t)‖(1−q2t)≤0,‖T1(t)−T11(t)‖(1−q3t)≤0,‖I(t)−I1(t)‖(1−q4t)≤0,‖T2(t)−T21(t)‖(1−q5t)≤0,‖R(t)−R1(t)‖(1−q6t)≤0. | (3.26) |
Hence, we have ‖S(t)−S1(t)‖=0, ‖L(t)−L1(t)‖=0, ‖T1(t)−T11(t)‖=0, ‖I(t)−I1(t)‖=0, ‖T2(t)−T21(t)‖=0 and ‖R(t)−R1(t)‖=0 which demonstrate S(t)=S1(t), L(t)=L1(t), T1(t)=T11(t), I(t)=I1(t), T2(t)=T21(t) and R(t)=R1(t). As a result, the model is shown to have a unique solution, proving the theorem.
To determine the system (3.7) equilibrium points, we set:
Λ−(βL+αI+μ)S=0,βSL−(γI+f+μ)L=0,fL−(m+μ+σ)T1=0,γLI+αSI−(θ+z+μ+σ)I=0,θI−(c+μ+σ)T2=0,mT1+cT2+zI−μR=0. | (4.1) |
Six steady states are produced by solving system Eq (4.1) collectively. According to their biological importance, we provide these equilibria and describe their local behavior. The disease-free equilibrium (DFE), which is denoted as the first equilibrium point, stated as Ω1=(Λμ,0,0,0,0,0), this indicates that there is no cell population. Co-equilibrium point, which is the second equilibrium point, is given as
Ω2=(ΛμR01,μβ(R01−1),fμβ(μ+m+σ)(R01−1),0,0,fmβ(μ+m+σ)(R01−1)). |
The third equilibrium is another co-equilibrium point presented by
Ω3=(ΛμR02,0,0,μα(R02−1),θμα(μ+c+σ)(R02−1),cθ+cz+μz+σzα(μ+c+σ)(R02−1)). |
Finally, the endemic equilibrium is given as
Ω4=(ΛγM,θ+μ+σ+zγ−αΛM,M(f(−αγΛ−αf(θ+μ+σ+z)))γ(μ+m+σ)+Mf(θ+μ+σ+z)(μ(γ−α)+β(θ+μ+σ)+βz))γ(μ+m+σ),βΛM−f+μγ,−θM(μ2(γ−α)−αf2+f(μ(γ−2α)+β(θ+μ+σ)+βz))γ(c+μ+σ)−θM(β(μ(θ+μ+σ+z)−γΛ))γ(c+μ+σ),−αcf2M(μ+σ)(−θ+m−z)γμ(c+μ+σ)(μ+m+σ)+cM(θ+z)(μ2(γ−α)+β(μ(θ+μ+σ+z)−γΛ))γμ(c+μ+σ)−cfM(μ+σ)(θ+z)(μ(γ−2α)+β(θ+μ+σ)+βz)γμ(c+μ+σ)(μ+m+σ)−cfmM(α(γΛ+μ(−θ+μ+σ−z)))γμ(c+μ+σ)(μ+m+σ)+cfmM((μ+σ)(β(θ+μ+σ)+γμ+βz))γμ(c+μ+σ)(μ+m+σ)−αf2M(μ+σ)(m(θ+μ+σ)−z(μ+σ))γμ(c+μ+σ)(μ+m+σ)−Mz(μ+σ)(μ+m+σ)(μ2(γ−α)+β(μ(θ+μ+σ+z)−γΛ))γμ(c+μ+σ)(μ+m+σ)−fMz(μ+σ)(μ+σ)(μ(γ−2α)+β(θ+μ+σ)+βz)−fmγμ(c+μ+σ)(μ+m+σ)+M(μ+σ)((θ+μ+σ)(μ(γ−α)+β(θ+μ+σ)))γμ(c+μ+σ)(μ+m+σ),−M(μ+σ)(αγΛ+z(αμ+β(θ+μ+σ)))γμ(c+μ+σ)(μ+m+σ)), |
where M=1μ(β+γ)−α(f+μ)+βσ+β(θ+z).
In the next subsection, we proceed with the evaluation of the reproduction number so that one can have some idea about the dynamics of the disease by using this value. Moreover, we show the local stability results of equilibria that have been obtained for the system in the form of theorems and proofs.
The population group that the model we are considering here assumes is heterogeneous, with non-homogeneous individuals that have been grouped. First, we provide the solution set as:
ψ(t)=[S(t),L(t),T1(t),I(t),T2(t),R(t)]T. | (4.2) |
then we use the next generation matrix approach described in [58] to evaluate the system (2.1)'s fundamental reproduction number. Following that, we define the solution set provided by Eq (4.2) as the difference between two matrices:
ψ(t)=F(ψ)−V(ψ), |
where
F(ψ)=(0βSL0(γL+αS)I00),V(ψ)=((βL+αI+μ)S(γI+f+μ)L−fL+(m+μ+σ)T1(θ+z+μ+σ)I−θI+(c+μ+σ)T2−mT1−cT2−zI+μR). |
The matrix generation approach is used to define F=[∂Fi(Ω1)∂tj] and V=[∂Vi(Ω1)∂tj], 1≤i,j≤2 for the F and V matrices of uninfected division at the equilibrium point Ω1. As more explicitly, they can be written in the following forms:
F=(βΛμ00αΛμ),V=(f+μ00θ+z+μ+σ), |
where the matrix V is non-singular and the matrix F is non-negative. With the use of the matrix FV−1's spectral radius at the equilibrium point Ω1, the disease's fundamental reproduction number is computed, which is indicated by two cases namely R01 and R02:
R01=βΛμ(f+μ),R02=αΛμ(θ+z+μ+σ), |
in which
R0=max[R01,R02]. | (4.3) |
Theorem 6. In the epidemic model, the disease-free equilibrium point Ω1 is locally asymptotically stable (LAS) if R0<1, else unstable.
Proof 6. Now, we take into consideration the following Jakobian matrix to highlight the stability criteria at the DFE point indicated as Ω1.
J(Ω1)=(−μ−βΛμ0−αΛμ000(R01−1)(f+μ)00000f−(m+μ+σ)000000(R02−1)(θ+z+μ+σ)00000θ−(c+μ+σ)000mzc−μ). |
The characteristic equation (CE) of matrix J(Ω1) can thus be found as:
Ω1(λ)=(λ+μ)2(λ−(R01−1)(f+μ))(λ+m+μ+σ)×(λ−(R02−1)(θ+z+μ+σ))(λ+c+μ+σ)=0, |
where
λ1,2=−μ,λ3=(R01−1)(f+μ),λ4=−(m+μ+σ),λ5=(R02−1)(θ+z+μ+σ),λ6=−(c+μ+σ), |
are the solutions of the CE. It is obvious that λ1,2,4,6 are negative. Furthermore, we can obtain that λ3 is negative if R01<1 and λ5 is negative as well if R02<1, which means that if R0=max[R01,R02]<1 the DFE is LAS. The proof is finished with this.
Theorem 7. The second equilibrium point Ω2 of the epidemic model is locally asymptotically stable if R01>1, otherwise there is at least one unbounded solution.
Proof 7. In order for the stability of Ω2(λ), we have the following characteristic equation:
Ω2(λ)=1R012R02βμ(−R02γR012μ2+R02γR01μ2+R02βλR01μ+ΛαβR01−ΛR02αβ)×(μR012λ+R01λ2+ΛβR01−Λβ)(λ+μ)×(λ+m+μ+σ)(c+λ+μ+σ)=0, |
where
λ1=−μ,λ2=−(m+μ+σ),λ3=−(c+μ+σ),λ4=γR012R02β2(R01−1)+ΛαR012R02βμ2(1R01−1R02). |
The remaining two roots, namely λ5 and λ6, satisfy the following equation:
λ2+Aλ+B=0, | (4.4) |
in which A=R01μ, B=ΛβR01(R01−1). According to the Routh-Hurwitz stability criteria second order [59,60], the coefficients of the quadratic characteristic equation given in Eq (4.4) must satisfy the following conditions if the roots of the equation have negative real part:
A>0, B>0, | (4.5) |
which means that the following inequalities hold:
R01>1. | (4.6) |
The significance of numerical results for the TB model we developed by taking the consciousness parameter into account is covered in this section. In the present paper, for the simulation, we have taken the parameter values as given in Table 1. In Table 1, we have considered the values as a parameter of "year". We have utilized the parameter value as the year in all of our simulations and graphs.
Par. | Interpretation | Value | Source |
Λ | Recruitment rate | 1562 | assumed |
β | The rate of susceptible individuals to enter the hidden TB compartment | 0.0005 | assumed |
α | Rate of transmission of susceptible individuals to infected compartment | 0.0006 | assumed |
γ | Rate of hidden TB individuals entering the Infected compartment | 0.00027 | assumed |
σ | Disease-related mortality | 0.004 | assumed |
μ | Normal mortality rate | 0.03 | [53] |
f | The rate at which hidden TB individuals enter the consciousness compartment | 0.6 | assumed |
m | Proportion of individuals recovering with preventive treatment | 0.5 | assumed |
θ | Proportion of individuals treated with infected individuals | 0.35 | assumed |
z | Proportion of infected individuals recovering without treatment | 0.4 | assumed |
c | Proportion of individuals recovering by treating infected individuals | 0.06 | assumed |
S(0) | Initial Susceptible population | 30201 | [54] |
L(0) | Initial Latent population | 830 | [54] |
T1(0) | Initial Treatment 1 population | 0 | [54] |
I(0) | Initial Infected population | 801 | [54] |
T2(0) | Initial Treatment 2 population | 0 | assumed |
R(0) | Initial Recovered population | 0 | [54] |
Figure 2 depicts the population dynamics in the model's behaviors and densities. In Figure 3, the impact of the parameter αis depicted, which stands for the rate of transmission of susceptible individuals to infected compartment, on the people who have infected and latent. Considering Figure 3, it is possible to draw the conclusion that when the parameter rises from 0.0006 to 0.00014, when the populations in I increases, the population of L decreases.
We have demonstrated the efficiency of the parameter β in 4, which represents the rate of susceptible individuals to enter the hidden TB compartment, on the latent and infected classes. By considering Figure 4, it can be concluded that as the parameter increases from 0.0008 to 0.0023, when the populations in L increase, the population of I decreases. In Figure 5, we have rededicated the consciousness effect for the number of infected individuals with TB. According to Figure 5, we have concluded that as the parameter f increases from 0.6 to 0.85, the number of infected individuals with TB decreases. This result is very important in terms of determining a parameter that helps to reduce the number of TB cases.
In Figure 6, in relation to the parameter z, we have shown the population density of the R class. The figure makes it evident that as the parameter's values rise from 0.4 to 0.9, the density of the R class rises along with it.
In this study, we have constructed an SLT1IT2R model that contains an effective consciousness strategy for the TB epidemic disease. With the help of the aforementioned model, we have highlighted the usefulness and various facets of consciousness. We have established the biologically possible zone, the solutions' positivity, and their boundlessness. The existence and uniqueness (E & U) of the solutions are demonstrated using the Lipschitz criteria.
The effectiveness of the factors on the population dynamics has been supplied based on the results that are shown in the figures. The dynamics of the population's behaviors and densities in the model are shown in Figure 2. Figures 3 and 4 show, respectively, how the parameters alpha and beta have an impact on latent and infected people. In Figure 5, we have described the dynamics of the consciousness population by taking f values into account. According to Figure 5, we have concluded that as the parameter f increases from 0.6 to 0.85, the number of infected individuals with TB virus decreases. This result is very important in terms of determining a parameter that helps to reduce the number of TB cases. We display the population density of the recovered people in relation to parameter z in Figure 6, which is the proportion of infected individuals recovering without treatment.
The impacts of the treatment and being educated thoughts can be highlighted in future studies by employing appropriate control strategies. Additionally, the fractional order can be taken into consideration when applying the integer-order model.
The authors would like to thank the reviewers and editors of this paper for their careful attention to detail and constructive feedback that improved the presentation of the paper greatly.
The authors were supported by TUBITAK (The Scientific and Technological Research Council of Türkiye). Fatma Özköse was supported by Research Fund of the Erciyes University. Project Number: FDS-2021-11059.
Dr. Mehmet Yavuz is a Guest Editor of special issue "Theoretical and Numerical Study of Nonlinear Models (Dynamical Systems)" for Mathematical Modelling and Control. Dr. Mehmet Yavuz was not involved in the editorial review and the decision to publish this article.
The authors declare that they have no conflicts of interest to report regarding the present study.
[1] | World Health Organization Weakly Report, 2021. Available from: https://www.who.int/publications/i/item/9789240013131. |
[2] |
D. Young, J. Stark, D. Kirschner, Systems Biology of Persistent Infection: Tuberculosis as a Case Study, Nat. Rev. Microbiol., 6 (2008), 520–528. https://doi.org/10.1038/nrmicro1919 doi: 10.1038/nrmicro1919
![]() |
[3] | Ministry of Health, 2021. Available from: https://hsgm.saglik.gov.tr/tr/tuberkuloz-haberler/24-mart-dunya-tuberkuloz-gunu-etkinlikleri.html. |
[4] |
H. Waaler, A. Geser, S. Andersen, The Use of Mathematical Models in the Study of the Epidemiology of Tuberculosis, American Journal of Public Health and the Nations Health, 52 (1962), 1002–1013. https://doi.org/10.2105/ajph.52.6.1002 doi: 10.2105/ajph.52.6.1002
![]() |
[5] |
M. Schulzer, M. P. Radhamani, S. Grzybowski, E. Mak, J. M. Fitzgerald, A Mathematical Model for the Prediction of the İmpact of HIV İnfection on Tuberculosis, Int. J. Epidemiol., 23 (1994), 400–407. https://doi.org/10.1093/ije/23.2.400 doi: 10.1093/ije/23.2.400
![]() |
[6] |
C. Castillo-Chavez, Z. Feng, To Treat or Not to Treat: The Case of Tuberculosis, J. Math. Biol., 35 (1997), 629–656. https://doi.org/10.1007/s002850050069 doi: 10.1007/s002850050069
![]() |
[7] |
Z. Feng, C. Castillo-Chavez, A. F. Capurro, A Model for Tuberculosis with Exogenous Reinfection, Theor. Popul. Biol., 57 (2000), 235–247. https://doi.org/10.1006/tpbi.2000.1451 doi: 10.1006/tpbi.2000.1451
![]() |
[8] |
T. Gumbo, A. Louie, M. R. Deziel, L. M. Parsons, M. Salfinger, G. L. Drusano, Selection of a Moxifloxacin Dose That Suppresses Drug Resistance in Mycobacterium Tuberculosis, by Use of an in Vitro Pharmacodynamic Infection Model and Mathematical Modeling, J. Infect. Dis., 190 (2004), 1642–1651. https://doi.org/10.1086/424849 doi: 10.1086/424849
![]() |
[9] |
D. W. Dowdy, R. E. Chaisson, L. H. Moulton, S. E. Dorman, The Potential Impact of Enhanced Diagnostic Techniques for Tuberculosis Driven by Hiv: A Mathematical Model, Aids, 20 (2006), 751–762. https://doi.org/10.1097/01.aids.0000216376.07185.cc doi: 10.1097/01.aids.0000216376.07185.cc
![]() |
[10] |
D. W. Dowdy, R. E. Chaisson, G. Maartens, E. L. Corbett, S. E. Dorman, Impact of enhanced tuberculosis diagnosis in South Africa: a mathematical model of expanded culture and drug susceptibility testing, Proceedings of the National Academy of Sciences, 105 (2008), 11293–11298. https://doi.org/10.1073/pnas.0800965105 doi: 10.1073/pnas.0800965105
![]() |
[11] |
S. Bowong, J. J. Tewa, Mathematical Analysis of a Tuberculosis Model with Differential Infectivity, Commun. Nonlinear Sci., 14 (2009), 4010–4021. https://doi.org/10.1016/j.cnsns.2009.02.017 doi: 10.1016/j.cnsns.2009.02.017
![]() |
[12] |
J. Liu, T. Zhang, Global Stability for a Tuberculosis Model, Math. Comput. Model., 54 (2011), 836–845. https://doi.org/10.1016/j.mcm.2011.03.033 doi: 10.1016/j.mcm.2011.03.033
![]() |
[13] |
J. J. Tewa, S. Bowong, S. O. Noutchie, Mathematical Analysis of a Two-Patch Model of Tuberculosis Disease with Staged Progression, Appl. Math. Model., 36 (2012), 5792–5807. https://doi.org/10.1016/j.apm.2012.01.026 doi: 10.1016/j.apm.2012.01.026
![]() |
[14] |
J. M. Trauer, J. T. Denholm, E. S. McBryde, Construction of a Mathematical Model for Tuberculosis Transmission in Highly Endemic Regions of the Asia-Pacific, J. Theor. Biol., 358 (2014), 74–84. https://doi.org/10.1016/j.jtbi.2014.05.023 doi: 10.1016/j.jtbi.2014.05.023
![]() |
[15] |
B. K. Mishra, J. Srivastava, Mathematical Model on Pulmonary and Multidrug-Resistant Tuberculosis Patients with Vaccination, Journal of the Egyptian Mathematical Society, 22 (2014), 311–316. https://doi.org/10.1016/j.joems.2013.07.006 doi: 10.1016/j.joems.2013.07.006
![]() |
[16] |
J. Li, Y. Zhang, X. Zhang, Mathematical Modeling of Tuberculosis Data of China, J. Theor. Biol., 365 (2015), 159–163. https://doi.org/10.1016/j.jtbi.2014.10.019 doi: 10.1016/j.jtbi.2014.10.019
![]() |
[17] |
P. J. Dodd, C. Sismanidis, J. A. Seddon, Global Burden of Drug-Resistant Tuberculosis in Children: A Mathematical Modelling Study, The Lancet infectious diseases, 16 (2016), 1193–1201. https://doi.org/10.1016/S1473-3099(16)30132-3 doi: 10.1016/S1473-3099(16)30132-3
![]() |
[18] | A. A. B. Sy, M. L. Diagne, I. Mbaye, O. Seydic, A Mathematical Model for the Impact of Public Health Education Campaign for Tuberculosis, Far East Journal of Applied Mathematics, 100 (2018), 97–138. https://doi.org/10.17654AM100020097 |
[19] |
D. N. Vinh, D. T. M. Ha, N. T. Hanh, G. Thwaites, M. F. Boni, H. E. Clapham, et al., Modeling Tuberculosis Dynamics with the Presence of Hyper-Susceptible Individuals for Ho Chi Minh City from 1996 to 2015, BMC Infect. Dis., 18 (2018), 494. https://doi.org/10.1186/s12879-018-3383-3 doi: 10.1186/s12879-018-3383-3
![]() |
[20] |
K. C. Chong, C. C. Leung, W. W. Yew, B. C. Y. Zee, G. C. H. Tam, M. H. Wang, et al., Mathematical modelling of the impact of treating latent tuberculosis infection in the elderly in a city with intermediate tuberculosis burden, Scientific reports, 9 (2019), 1–11. https://doi.org/10.1038/s41598-019-41256-4 doi: 10.1038/s41598-019-41256-4
![]() |
[21] |
P. A. Naik, M. Yavuz, S. Zu, J. Qureshi, S. Townley, Modeling and analysis of COVID-19 epidemics with treatment in fractional derivatives using real data from Pakistan, The European Physical Journal Plus, 135 (2020), 1–42. https://doi.org/10.1140/epjp/s13360-020-00819-5 doi: 10.1140/epjp/s13360-020-00819-5
![]() |
[22] |
S. Allegretti, I. M. Bulai, R. Marino, M. A. Menandro, K. Parisi, Vaccination effect conjoint to fraction of avoided contacts for a Sars-Cov-2 mathematical model, Mathematical Modelling and Numerical Simulation with Applications, 1 (2021), 56–66. https://doi.org/10.53391/mmnsa.2021.01.006 doi: 10.53391/mmnsa.2021.01.006
![]() |
[23] |
F. Özköse, M. Yavuz, Investigation of interactions between COVID-19 and diabetes with hereditary traits using real data: A case study in Turkey, Comput. Biol. Med., 141 (2022), 105044. https://doi.org/10.1016/j.compbiomed.2021.105044 doi: 10.1016/j.compbiomed.2021.105044
![]() |
[24] |
R. Ikram, A. Khan, M. Zahri, A. Saeed, M. Yavuz, P. Kumam, Extinction and stationary distribution of a stochastic COVID-19 epidemic model with time-delay, Comput. Biol. Med., 141 (2022), 105115. https://doi.org/10.1016/j.compbiomed.2021.105115 doi: 10.1016/j.compbiomed.2021.105115
![]() |
[25] |
Y. Sabbar, D. Kiouach, S. P. Rajasekar, S. E. A. El-Idrissi, The influence of quadratic Lévy noise on the dynamic of an SIC contagious illness model: New framework, critical comparison and an application to COVID-19 (SARS-CoV-2) case, Chaos, Solitons & Fractals, 159 (2022), 112110. https://doi.org/10.1016/j.chaos.2022.112110 doi: 10.1016/j.chaos.2022.112110
![]() |
[26] |
M. Higazy, Novel fractional order SIDARTHE mathematical model of COVID-19 pandemic, Chaos, Solitons & Fractals, 138 (2020), 110007. https://doi.org/10.1016/j.chaos.2020.110007 doi: 10.1016/j.chaos.2020.110007
![]() |
[27] |
Z. H. Shen, Y. M. Chu, M. A. Khan, S. Muhammad, O. A. Al-Hartomy, M. Higazy, Mathematical modeling and optimal control of the COVID-19 dynamics, Results Phys., 31 (2021), 105028. https://doi.org/10.1016/j.rinp.2021.105028 doi: 10.1016/j.rinp.2021.105028
![]() |
[28] |
M. Higazy, M. A. Alyami, New Caputo-Fabrizio fractional order SEIASqEqHR model for COVID-19 epidemic transmission with genetic algorithm based control strategy, Alex. Eng. J., 59 (2020), 4719–4736. https://doi.org/10.1016/j.aej.2020.08.034 doi: 10.1016/j.aej.2020.08.034
![]() |
[29] |
M. Higazy, F. M. Allehiany, E. E. Mahmoud, Numerical study of fractional order COVID-19 pandemic transmission model in context of ABO blood group, Results Phys., 22 (2021), 103852. https://doi.org/10.1016/j.rinp.2021.103852 doi: 10.1016/j.rinp.2021.103852
![]() |
[30] |
S. Ahmad, D. Qiu, M. ur Rahman, Dynamics of a fractional-order COVID-19 model under the nonsingular kernel of Caputo-Fabrizio operator, Mathematical Modelling and Numerical Simulation with Applications, 2 (2022), 228–243. https://doi.org/10.53391/mmnsa.2022.019 doi: 10.53391/mmnsa.2022.019
![]() |
[31] |
A. G. C. Pérez, D. A. Oluyori, A model for COVID-19 and bacterial pneumonia coinfection with community-and hospital-acquired infections, Mathematical Modelling and Numerical Simulation with Applications, 2 (2022), 197–210. https://doi.org/10.53391/mmnsa.2022.016 doi: 10.53391/mmnsa.2022.016
![]() |
[32] |
A. O. Atede, A. Omame, A., S. C. Inyama, A fractional order vaccination model for COVID-19 incorporating environmental transmission: a case study using Nigerian data. Bulletin of Biomathematics, 1 (2023), 78–110. https://doi.org/10.59292/bulletinbiomath.2023005 doi: 10.59292/bulletinbiomath.2023005
![]() |
[33] |
F. Özköse, M. T. Şenel, R. Habbireeh, Fractional-order mathematical modelling of cancer cells-cancer stem cells-immune system interaction with chemotherapy, Mathematical Modelling and Numerical Simulation with Applications, 1 (2021), 67–83. https://doi.org/10.53391/mmnsa.2021.01.007 doi: 10.53391/mmnsa.2021.01.007
![]() |
[34] |
A. M. S. Mahdy, M. Higazy, K. A. Gepreel, A. A. A. El-Dahdouh, Optimal control and bifurcation diagram for a model nonlinear fractional SIRC, Alex. Eng. J., 59 (2020), 3481–3501. https://doi.org/10.1016/j.aej.2020.05.028 doi: 10.1016/j.aej.2020.05.028
![]() |
[35] |
F. Evirgen, S. Uçar, N. Özdemir, Z. Hammouch, System response of an alcoholism model under the effect of immigration via non-singular kernel derivative, Discrete Cont. Dyn.-S, 14 (2021), 2199. https://doi.org/10.3934/dcdss.2020145 doi: 10.3934/dcdss.2020145
![]() |
[36] |
P. Kumar, V. S. Erturk, Dynamics of cholera disease by using two recent fractional numerical methods, Mathematical Modelling and Numerical Simulation with Applications, 1 (2021), 102–111. https://doi.org/10.53391/mmnsa.2021.01.010 doi: 10.53391/mmnsa.2021.01.010
![]() |
[37] |
F. Özköse, R. Habbireeh, M. T. Şenel, A novel fractional order model of SARS-CoV-2 and Cholera disease with real data, J. Comput. Appl. Math., 423 (2023), 114969. https://doi.org/10.1016/j.cam.2022.114969 doi: 10.1016/j.cam.2022.114969
![]() |
[38] |
H. Joshi, B. K. Jha, Chaos of calcium diffusion in Parkinson's infectious disease model and treatment mechanism via Hilfer fractional derivative, Mathematical Modelling and Numerical Simulation with Applications, 1 (2021), 84–94. https://doi.org/10.53391/mmnsa.2021.01.008 doi: 10.53391/mmnsa.2021.01.008
![]() |
[39] |
H. Joshi, M. Yavuz, I. Stamova, A fractional order vaccination model for COVID-19 incorporating environmental transmission: a case study using Nigerian data. Bulletin of Biomathematics, 1 (2023), 24–39. https://doi.org/10.59292/bulletinbiomath.2023002 doi: 10.59292/bulletinbiomath.2023002
![]() |
[40] |
P. A. Naik, K. M. Owolabi, M. Yavuz, J. Zu, Chaotic dynamics of a fractional order HIV-1 model involving AIDS-related cancer cells, Chaos, Solitons & Fractals, 140 (2020), 110272. https://doi.org/10.1016/j.chaos.2020.110272 doi: 10.1016/j.chaos.2020.110272
![]() |
[41] |
P. A. Naik, M. Yavuz, J. Zu, The role of prostitution on HIV transmission with memory: a modeling approach, Alex. Eng. J., 59 (2020), 2513–2531. https://doi.org/10.1016/j.aej.2020.04.016 doi: 10.1016/j.aej.2020.04.016
![]() |
[42] |
R. M. Jena, S. Chakraverty, M. Yavuz, T. Abdeljawad, A New Modeling and Existence-Uniqueness Analysis for Babesiosis Disease of Fractional Order, Mod. Phys. Lett. B, (2021). https://doi.org/10.1142/S0217984921504431 doi: 10.1142/S0217984921504431
![]() |
[43] |
M. Yavuz, N. Sene, Stability Analysis and Numerical Computation of the Fractional Predator–Prey Model with the Harvesting Rate, Fractal Fract., 4 (2020), 35. https://doi.org/10.3390/fractalfract4030035 doi: 10.3390/fractalfract4030035
![]() |
[44] |
P. A. Naik, Z. Eskandari, M. Yavuz, J. Zu, Complex dynamics of a discrete-time Bazykin–Berezovskaya prey-predator model with a strong Allee effect, J. Comput. Appl. Math., 413 (2022), 114401. https://doi.org/10.1016/j.cam.2022.114401 doi: 10.1016/j.cam.2022.114401
![]() |
[45] |
M. Naim, Y. Sabbar, A. Zeb, Stability characterization of a fractional-order viral system with the non-cytolytic immune assumption, Mathematical Modelling and Numerical Simulation with Applications, 2 (2022), 164–176. https://doi.org/10.53391/mmnsa.2022.013 doi: 10.53391/mmnsa.2022.013
![]() |
[46] |
F. Evirgen, Transmission of Nipah virus dynamics under Caputo fractional derivative, J. Comput. Appl. Math., 418 (2023), 114654. https://doi.org/10.1016/j.cam.2022.114654 doi: 10.1016/j.cam.2022.114654
![]() |
[47] |
Y. Sabbar, A. Khan, A. Din, D. Kiouach, S. P. Rajasekar, Determining the global threshold of an epidemic model with general interference function and high-order perturbation, AIMS Math., 7 (2022), 19865–19890. https://doi.org/10.3934/math.20221088 doi: 10.3934/math.20221088
![]() |
[48] |
Y. Sabbar, A. Din, D. Kiouach, Predicting potential scenarios for wastewater treatment under unstable physical and chemical laboratory conditions: A mathematical study, Results Phys., 39 (2022), 105717. https://doi.org/10.1016/j.rinp.2022.105717 doi: 10.1016/j.rinp.2022.105717
![]() |
[49] |
A. R. Sheergojri, P. Iqbal, P. Agarwal, N. Ozdemir, Uncertainty-based Gompertz growth model for tumor population and its numerical analysis, An International Journal of Optimization and Control: Theories & Applications (IJOCTA), 12 (2022), 137–150. https://doi.org/10.11121/ijocta.2022.1208 doi: 10.11121/ijocta.2022.1208
![]() |
[50] |
Y. Sabbar, M. Yavuz, F. Özköse, Infection Eradication Criterion in a General Epidemic Model with Logistic Growth, Quarantine Strategy, Media Intrusion, and Quadratic Perturbation, Mathematics, 10 (2022), 4213. https://doi.org/10.3390/math10224213 doi: 10.3390/math10224213
![]() |
[51] |
Z. Hammouch, M. Yavuz, N. Özdemir, Numerical solutions and synchronization of a variable-order fractional chaotic system, Mathematical Modelling and Numerical Simulation with Applications, 1 (2021), 11–23. https://doi.org/10.53391/mmnsa.2021.01.002 doi: 10.53391/mmnsa.2021.01.002
![]() |
[52] |
Y. Sabbar, A. Zeb, D. Kiouach, N. Gul, T. Sitthiwirattham, D. Baleanu, et al., Dynamical bifurcation of a sewage treatment model with general higher-order perturbation, Results Phys., 39 (2022), 105799. https://doi.org/10.1016/j.rinp.2022.105799 doi: 10.1016/j.rinp.2022.105799
![]() |
[53] |
S. Kim, A. A. de los Reyes V, E. Jung, Country-specific intervention strategies for top three TB burden countries using mathematical model, PloS one, 15 (2020), e0230964. https://doi.org/10.1371/journal.pone.0230964 doi: 10.1371/journal.pone.0230964
![]() |
[54] |
S. Ullah, M. A. Khan, M. Farooq, T. Gul, Modeling and analysis of tuberculosis (tb) in Khyber Pakhtunkhwa, Pakistan, Math. Comput. Simulat., 165 (2019), 181–199. https://doi.org/10.1016/j.matcom.2019.03.012 doi: 10.1016/j.matcom.2019.03.012
![]() |
[55] | C. Obasi, G. C. E. Mbah, On the stability analysis of a mathematical model of Lassa fever disease dynamics, Journal of the Nigerian Society for Mathematical Biology, 2 (2019), 135–144. |
[56] | G. Birkhoff, G. C. Rota, Ordinary Differential Equations, Wiley: Hoboken, NJ, USA, 1989. |
[57] |
H. W. Hethcote, The mathematics of infectious diseases, SIAM Rev., 42 (2000), 599–653. https://doi.org/10.1137/S0036144500371907 doi: 10.1137/S0036144500371907
![]() |
[58] |
P. Van den Driessche, J. Watmough, Reproduction numbers and sub-threshold endemic equilibria for compartmental models of disease transmission, Math. Biosci., 180 (2002), 29–48. https://doi.org/10.1016/S0025-5564(02)00108-6 doi: 10.1016/S0025-5564(02)00108-6
![]() |
[59] |
E. Ahmed, A. S. Elgazzar, On fractional order differential equations model for nonlocal epidemics, Physica A, 379 (2007), 607–614. https://doi.org/10.1016/j.physa.2007.01.010 doi: 10.1016/j.physa.2007.01.010
![]() |
[60] | F. Brauer, C. Castillo-Chavez, Z. Feng, Mathematical models in epidemiology, Vol. 32, 2019, New York: Springer. |
1. | Bijal M. Yeolekar, Radhika D. Dave, Sagar R. Khirsariya, Solution of a cancer treatment model of a drug targeting treatment through nanotechnology using Adomian decomposition Laplace transform method, 2024, 245, 3005-0731, 10.1007/s10751-024-02114-6 | |
2. | Mati ur Rahman, Yeliz Karaca, Ravi P. Agarwal, Sergio Adriani David, Mathematical modelling with computational fractional order for the unfolding dynamics of the communicable diseases, 2024, 32, 2769-0911, 10.1080/27690911.2023.2300330 | |
3. | Manisha Meena, Mridula Purohit, S.D. Purohit, Dumitru Baleanu, D.L. Suthar, A novel fractionalized investigation of tuberculosis disease, 2024, 32, 2769-0911, 10.1080/27690911.2024.2351229 | |
4. | Amr Elsonbaty, Mohammed Alharbi, A. El-Mesady, Waleed Adel, Dynamical analysis of a novel discrete fractional lumpy skin disease model, 2024, 9, 26668181, 100604, 10.1016/j.padiff.2023.100604 | |
5. | Hamid Qureshi, Zahoor Shah, Muhammad Asif Zahoor Raja, Mohammad Y. Alshahrani, Waqar Azeem Khan, Muhammad Shoaib, Machine learning investigation of tuberculosis with medicine immunity impact, 2024, 110, 07328893, 116472, 10.1016/j.diagmicrobio.2024.116472 | |
6. | Francis Oketch Ochieng, Mathematical Modeling of Tuberculosis Transmission Dynamics With Reinfection and Optimal Control, 2024, 2577-8196, 10.1002/eng2.13068 | |
7. | Weiguang Zhou, Guangxin Wang, Jixiao Lu, Hongxin Ruan, Jun Wang, Rui Zhang, On a data model associated with antitrust behaviors, 2024, 4, 2767-8946, 459, 10.3934/mmc.2024036 | |
8. | Kshama Jain, Anuradha Bhattacharjee, Srikumar Krishnamurhty, Mathematical analysis of COVID-19 and TB co-infection dynamics with optimal control, 2025, 11, 2363-6203, 10.1007/s40808-024-02197-8 | |
9. | Kumneger Tadesse Mulugeta, Mohammed Yiha Dawed, Shewafera Wondimagegnhu Teklu, Joshua Kiddy K. Asamoah, Impact of media coverage on the transmission dynamics of TB with vaccines and treatment, 2025, 20, 1932-6203, e0314324, 10.1371/journal.pone.0314324 | |
10. | Shakeel Ahmed, Saif Ullah, Nazma Asghar, Wakeel Ahmed, Computational Analysis of Nipah Virus Transmission Using Mathematical Modelling and Graphical Programming, 2025, 15, 2191-1630, 10.1007/s12668-025-01804-x | |
11. | Nagendra Kumar Yadav, Rajesh Kumar Sinha, Bernstein wavelets based numerical algorithm for solving fractional order lumpy skin disease model, 2025, 2752-2334, 10.1515/jncds-2024-0090 | |
12. | A. El-Mesady, A. M. S. Mahdy, Fatma Özköse, A fractional–order model with prevention and isolation optimal control measures to reduce the transmission of Tuberculosis, 2025, 19, 1658-3655, 10.1080/16583655.2025.2475579 |
Par. | Interpretation | Value | Source |
Λ | Recruitment rate | 1562 | assumed |
β | The rate of susceptible individuals to enter the hidden TB compartment | 0.0005 | assumed |
α | Rate of transmission of susceptible individuals to infected compartment | 0.0006 | assumed |
γ | Rate of hidden TB individuals entering the Infected compartment | 0.00027 | assumed |
σ | Disease-related mortality | 0.004 | assumed |
μ | Normal mortality rate | 0.03 | [53] |
f | The rate at which hidden TB individuals enter the consciousness compartment | 0.6 | assumed |
m | Proportion of individuals recovering with preventive treatment | 0.5 | assumed |
θ | Proportion of individuals treated with infected individuals | 0.35 | assumed |
z | Proportion of infected individuals recovering without treatment | 0.4 | assumed |
c | Proportion of individuals recovering by treating infected individuals | 0.06 | assumed |
S(0) | Initial Susceptible population | 30201 | [54] |
L(0) | Initial Latent population | 830 | [54] |
T1(0) | Initial Treatment 1 population | 0 | [54] |
I(0) | Initial Infected population | 801 | [54] |
T2(0) | Initial Treatment 2 population | 0 | assumed |
R(0) | Initial Recovered population | 0 | [54] |
Par. | Interpretation | Value | Source |
Λ | Recruitment rate | 1562 | assumed |
β | The rate of susceptible individuals to enter the hidden TB compartment | 0.0005 | assumed |
α | Rate of transmission of susceptible individuals to infected compartment | 0.0006 | assumed |
γ | Rate of hidden TB individuals entering the Infected compartment | 0.00027 | assumed |
σ | Disease-related mortality | 0.004 | assumed |
μ | Normal mortality rate | 0.03 | [53] |
f | The rate at which hidden TB individuals enter the consciousness compartment | 0.6 | assumed |
m | Proportion of individuals recovering with preventive treatment | 0.5 | assumed |
θ | Proportion of individuals treated with infected individuals | 0.35 | assumed |
z | Proportion of infected individuals recovering without treatment | 0.4 | assumed |
c | Proportion of individuals recovering by treating infected individuals | 0.06 | assumed |
S(0) | Initial Susceptible population | 30201 | [54] |
L(0) | Initial Latent population | 830 | [54] |
T1(0) | Initial Treatment 1 population | 0 | [54] |
I(0) | Initial Infected population | 801 | [54] |
T2(0) | Initial Treatment 2 population | 0 | assumed |
R(0) | Initial Recovered population | 0 | [54] |