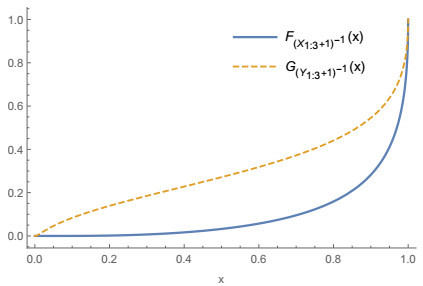
The Covid-19 pandemic continues to cause serious physical and mental problems for health professionals, particularly nurses.
To estimate the prevalence of anxiety and insomnia and to evaluate their possible association with family support received by nurses two years after the onset of the pandemic.
In total, the study participants were 404 nurses (335 females and 69 males) with a mean age of 42.88 (SD = 10.9) years and a mean of 17.96 (SD = 12) years working as nurses. Nurses from five tertiary hospitals in Athens constituted the study population who completed the questionnaires State-Trait Anxiety Inventory (STAI), Athens Insomnia Scale (AIS) and Family Support Scale (FSS), in the months of November and December 2021. Regarding demographic and occupational characteristics, gender, age and years of experience as nurses were recorded.
60.1% of the nurses showed abnormal scores in state anxiety, with 46.8% in trait anxiety, and 61.4% showed insomnia. Women showed higher scores on the two subscales of anxiety and the insomnia scale compared to men (p < 0.01 and p < 0.05 respectively), while they showed a lower score on the FSS without statistical significance (p > 0.05). Positive correlations (p < 0.01) were found between the State Anxiety Inventory, Trait Anxiety Inventory and AIS, while all of them showed a high negative correlation with FSS (p < 0.01). Age showed a negative correlation with Trait Anxiety Inventory (p < 0.05). As shown by the mediation analysis, the relationship between state anxiety and insomnia was mediated by trait anxiety, whereas state anxiety appeared to be dependent on family support.
Nurses continue to experience high levels of anxiety and insomnia and feel less supported by their families than in the first year of the pandemic. Insomnia appears to be dependent on state anxiety, with a significant indirect effect of trait anxiety, while family support seems to affect state anxiety.
Citation: Christos Sikaras, Maria Tsironi, Sofia Zyga, Aspasia Panagiotou. Anxiety, insomnia and family support in nurses, two years after the onset of the pandemic crisis[J]. AIMS Public Health, 2023, 10(2): 252-267. doi: 10.3934/publichealth.2023019
[1] | Bin Lu . Stochastic comparisons of second-order statistics from dependent and heterogeneous modified proportional (reversed) hazard rates scale models. AIMS Mathematics, 2024, 9(4): 8904-8919. doi: 10.3934/math.2024434 |
[2] | Mingxia Yang . Orderings of the second-largest order statistic with modified proportional reversed hazard rate samples. AIMS Mathematics, 2025, 10(1): 311-337. doi: 10.3934/math.2025015 |
[3] | Xiao Zhang, Rongfang Yan . Stochastic comparisons of extreme order statistic from dependent and heterogeneous lower-truncated Weibull variables under Archimedean copula. AIMS Mathematics, 2022, 7(4): 6852-6875. doi: 10.3934/math.2022381 |
[4] | Li Zhang, Rongfang Yan . Stochastic comparisons of series and parallel systems with dependent and heterogeneous Topp-Leone generated components. AIMS Mathematics, 2021, 6(3): 2031-2047. doi: 10.3934/math.2021124 |
[5] | Bin Lu, Rongfang Yan . Ordering results of second order statistics from random and non-random number of random variables with Archimedean copulas. AIMS Mathematics, 2021, 6(6): 6390-6405. doi: 10.3934/math.2021375 |
[6] | H. M. Barakat, M. A. Alawady, I. A. Husseiny, M. Nagy, A. H. Mansi, M. O. Mohamed . Bivariate Epanechnikov-exponential distribution: statistical properties, reliability measures, and applications to computer science data. AIMS Mathematics, 2024, 9(11): 32299-32327. doi: 10.3934/math.20241550 |
[7] | Fawaz K. Alalhareth, Seham M. Al-Mekhlafi, Ahmed Boudaoui, Noura Laksaci, Mohammed H. Alharbi . Numerical treatment for a novel crossover mathematical model of the COVID-19 epidemic. AIMS Mathematics, 2024, 9(3): 5376-5393. doi: 10.3934/math.2024259 |
[8] | Mansour Shrahili, Mohamed Kayid . Laplace transform ordering of bivariate inactivity times. AIMS Mathematics, 2022, 7(7): 13208-13224. doi: 10.3934/math.2022728 |
[9] | Mohamed Kayid, Adel Alrasheedi . Weighted proportional mean inactivity time model. AIMS Mathematics, 2022, 7(3): 4038-4060. doi: 10.3934/math.2022223 |
[10] | Ze Gu . On weakly semiprime segments of ordered semihypergroups. AIMS Mathematics, 2021, 6(9): 9882-9885. doi: 10.3934/math.2021573 |
The Covid-19 pandemic continues to cause serious physical and mental problems for health professionals, particularly nurses.
To estimate the prevalence of anxiety and insomnia and to evaluate their possible association with family support received by nurses two years after the onset of the pandemic.
In total, the study participants were 404 nurses (335 females and 69 males) with a mean age of 42.88 (SD = 10.9) years and a mean of 17.96 (SD = 12) years working as nurses. Nurses from five tertiary hospitals in Athens constituted the study population who completed the questionnaires State-Trait Anxiety Inventory (STAI), Athens Insomnia Scale (AIS) and Family Support Scale (FSS), in the months of November and December 2021. Regarding demographic and occupational characteristics, gender, age and years of experience as nurses were recorded.
60.1% of the nurses showed abnormal scores in state anxiety, with 46.8% in trait anxiety, and 61.4% showed insomnia. Women showed higher scores on the two subscales of anxiety and the insomnia scale compared to men (p < 0.01 and p < 0.05 respectively), while they showed a lower score on the FSS without statistical significance (p > 0.05). Positive correlations (p < 0.01) were found between the State Anxiety Inventory, Trait Anxiety Inventory and AIS, while all of them showed a high negative correlation with FSS (p < 0.01). Age showed a negative correlation with Trait Anxiety Inventory (p < 0.05). As shown by the mediation analysis, the relationship between state anxiety and insomnia was mediated by trait anxiety, whereas state anxiety appeared to be dependent on family support.
Nurses continue to experience high levels of anxiety and insomnia and feel less supported by their families than in the first year of the pandemic. Insomnia appears to be dependent on state anxiety, with a significant indirect effect of trait anxiety, while family support seems to affect state anxiety.
In reliability theory, to model the lifetime data with different hazard shapes, it is desirable to introduce flexible families of distributions, and to this end, there are two methods have been commonly used to characterise lifetime distribution with considerable flexibility. One method is to adopt the well-known families of distributions, for example, Gamma, Weibull and Log-normal, which have been studied quite extensively in the literature, for more discussions on this topic, we refer readers to [1,2,3]. Marshall and Olkin [4] developed a new method to introduce one parameter to a base distribution results in a new family of distribution with more flexibility. For example, for a baseline distribution function F with support R+=(0,∞) and corresponding survival function ˉF, the new distribution functions can be defined as
G(x;α)=F(x)1−ˉαˉF(x),x,α∈R+,ˉα=1−α, | (1.1) |
H(x;α)=αF(x)1−ˉαF(x),x,α∈R+,ˉα=1−α. | (1.2) |
Marshall and Olkin [4] originally proposed the family of distributions in (1.1) and studied it for the case when F is a Weibull distribution. When F has probability density and hazard rate functions as f and hF, respectively, then the hazard rate function of G is given by
hF(x;α)=11−ˉαˉF(x)hF(x),x,α∈R+,ˉα=1−α, |
Therefore, one can observe that if hF(x) is decreasing (increasing) in x, then for 0<α≤1(α≥1), hF(x;α) is also decreasing in x. Moreover, one can observe that hF(x)≤hF(x;α) for 0<α≤1, and hF(x;α)≤hF(x) for α≥1. For this reason, the parameter α in (1.1) is referred to as a tilt parameter (see [5]). Note that (1.1) is equivalent to (1.2) if α in (1.1) is changed to 1/α. The proportional hazard rates (PHR) and the proportional reversed hazard rates (PRHR) models have important applications in reliability and survival analysis. The random variables X1,⋯,Xn are said to follow: (ⅰ) PHR model if Xi has the survival function ˉFXi(x)=ˉFλi(x), i=1,⋯,n, where ˉF is the baseline survival function and (λ1,⋯,λn) is the frailty vector; (ii) PRHR model if Xi has the distribution function FXi(x)=Fβi(x), i=1,⋯,n, where F is the baseline distribution and (β1,⋯,βn) is the resilience vector. It is well-known that the Exponential, Weibull, Lomax and Pareto distributions are special cases of the PHR model, and Fréchet distribution is a special case of the PRHR model. Balakrishnan et al. [6] introduced two new statistical models by adding a parameter to PHR and PRHR models, which are regarded as the baseline distributions in G(x;α) and H(x;α), respectively. The two new models are referred to as the modified proportional hazard rates (MPHR) and modified proportional reversed hazard rates (MPRHR) models, respectively. These are given by
G(x;α,λ)=1−(ˉF(x))λ1−ˉα(ˉF(x))λ,x,α∈R+,ˉα=1−α, | (1.3) |
H(x;α,β)=α(F(x))β1−ˉα(F(x))β,x,α∈R+,ˉα=1−α, | (1.4) |
where λ and β are the proportional hazard rate and proportional reversed hazard rate parameters, respectively. We denote X∼MPHR(α,λ;ˉF) and X∼MPRHR(α,β;F) if X has the distribution functions G(x;α,λ) and H(x;α,β), respectively. For the case λ=β=1, (1.3) and (1.4) simply reduce to (1.1) and (1.2), respectively. For the case α=1, (1.3) and (1.4) simply reduce to the PHR and PRHR models, respectively. According to the Theorem 2.1 of Navarro et al.[14], (1.3) and (1.4) can be rewritten the distorted distribution of h1 and h2, respectively, where
h1(u;α,λ)=1−(u)λ1−ˉα(u)λ,h2(u;α,β)=α(1−u)β1−ˉα(1−u)β,u=ˉF(x),x,α∈R+,ˉα=1−α, | (1.5) |
if λ=β=1, (1.5) just as the distorted distributions of (1.1) and (1.2), respectively. For some special models, please refer to multiple-outlier models ([7], [8]), extended exponential and extend Weibull distribution ([4], [9]), extended Pareto distribution ([10]) and extended Lomax distribution ([11]).
Order statistics play an important role in reliability theory, auction theory, operations research, and many applied probability areas. Xk:n denotes the kth smallest of random variables X1,…,Xn,k=1,…,n. In reliability theory, Xk:n characterizes the lifetime of a (n−k+1)-out-of-n system, which works if at least n−k+1 of all the n components function normally. Specifically, X1:n and Xn:n denote the lifetimes of series and parallel systems, respectively. In auction theory, X1:n and Xn:n represent the final price of the first-price procurement auction and the first-price sealed-bid auction (see [12]), respectively. In the past decades, researchers devoted themselves to stochastic comparisons of order statistics from heterogeneous independent or dependent samples. For example. Belzunce et al. [13] established some results and applications concerning the likelihood ratio order of random vectors of order statistics in the case of independent but not necessarily identically distributed observations and for the case of possible dependent observations. Navarro et al.[14] obtained ordering properties for coherent systems with possibly dependent identically distributed components. Balakrishnan and Zhao[15] studied the stochastic comparison of order statistics from independent and heterogeneous proportional hazard rates models, gamma variables, geometric variables, and negative binomial variables in the stochastic orders and majorization orders. For more discussions related to order statistics, one may refer to [16,17,18,19,20]. Besides, many authors have studied stochastic comparisons of order statistics from heterogeneous samples following some families of lifetime distributions. For example, Fang et al.[21] conducted stochastic comparisons on sample extremes of dependent and heterogeneous observations from PHR and PRHR models. Balakrishnan et al. [6] introduced MPHR and MPRHR model, and established some stochastic comparisons between the corresponding order statistics with independent samples. Li and Li [22] developed sufficient conditions for the (reversed) hazard rate order on maximums (minimums) of samples following PRHR (PHR) model under Archimedean copula. Das and Kayal [23] introduced a scale parameter into the MPHR and MPRHR models lead to new models, which are called as modified proportional hazard rate scale (MPHRS) and modified proportional reversed hazard rate scale (MPRHRS) models, respectively, and obtained some stochastic comparison results on independent samples in term of the usual stochastic, (reversed) hazard rate orders. Barmalzan et al. [24] discussed the hazard rate order and reversed hazard rate order of series and parallel systems with dependent components following either MPHR or MPRHR models under Archimedean copula. Motivated by the work of Balakrishnan et al.[6], this paper devotes to studying stochastic comparisons of sample extremes arising from heterogeneous and dependent MPHR and MPRHR models, and we derive the usual stochastic, (reversed) hazard rate orders of extremes with the heterogeneity considered in the model parameters.
The remaining part of the paper is organized as follows: Section 2 recalls some basic concepts and notations that will be used in the sequel. Section 3 deals with stochastic comparisons between minimums of MPRHR (MPHR) sample with Archimedean (survival) copula, respectively. Section 4 presents the corresponding results on maximums of the sample. Section 5 summarizes our research findings and future directions.
In this section, let us first review some basic concepts that will be used in the sequel. Let X and Y be two random variables with distribution functions F(x) and G(x), survival functions ˉF(x)=1−F(x) and ˉG(x)=1−G(x), probability density functions f(x) and g(x), the hazard rate functions hX(x)=f(x)/ˉF(x) and hY(x)=g(x)/ˉG(x), and reversed hazard rate functions rX(x)=f(x)/F(x) and rY(x)=g(x)/G(x), respectively.
Definition 1. X is said to be smaller than Y in the sense of
(i) the usual stochastic order (denoted by X≤stY) if ˉF(x)≤ˉG(x) for all x∈R+;
(ii) the hazard rate order (denoted by X≤hrY) if hX(x)≥hY(x) for all x∈R+ or equivalently, if ˉG(x)/ˉF(x) is increasing in x∈R+;
(iii) the reversed hazard rate order (denoted by X≤rhY) if rX(x)≤rY(x) for all x∈R+ or equivalently, if G(x)/F(x) is increasing in x∈R+.
It is well known that the hazard rate and reversed hazard rate orders imply the usual stochastic order, but the converse is not true. For a comprehensive discussion on various stochastic orders and their applications, one may refer to Shaked and Shanthikumar[25] and Li and Li [26].
Next, we introduce the notion of the weak majorization order. For two real vectors x=(x1,...,xn) and y=(y1,...,yn)∈Rn, x(1)≤x(2)≤⋯≤x(n) and y(1)≤y(2)≤⋯≤y(n) denote the increasing arrangement of the components of x and y, respectively. Denote In={1,…,n}.
Definition 2. The vector x is said to be weakly supermajorized by the vector y (write as xw⪯y) if ∑ji=1x(i)≥∑ji=1y(i), for all j∈In.
Now, let us review the concept of Archimedean Copulas.
Definition 3. For a decreasing and continuous function ψ:[0,+∞]↦[0,1] such that ψ(0)=1 and ψ(+∞)=0, let ϕ=ψ−1 be the pseudo-inverse. Then
Cψ(u1,...,un)=ψ(ϕ(u1)+...+ϕ(un)),ui∈[0,1],i∈In, |
is said to be an Archimedean copula with generator ψ if (−1)kψk(x)≥0 for k=0,…,n−2 and (−1)n−2ψn−2(x) is decreasing and convex.
For detailed discussions on copulas and its applications, one may refer to Nelsen [27].
The following lemmas are useful to establish the main results.
Lemma 1. ([28]) Let I⊆R be an open interval, a continuously differentiable h:In→R is Schur-convex(Schur-concave) if and only if h is symmetric on In and for all i≠j
(xi−xj)(∂h(x)∂xi−∂h(x)∂xj)≥(≤)0. |
Lemma 2. ([29]) For a real function h on A⊆Rn, xw⪯y implies h(x)≤h(y) if and only if h is decreasing and Schur-convex on A.
h(x) is Schur-concave on A if and only if −h(x) is Schur-convex. For more details on majorization and Schur-convexity (concavity), please refer to [29].
Lemma 3. For two n-dimensional Archimedean copulas Cψ1 and Cψ2, if ϕ2∘ψ1 is super-additive, then Cψ1(u)≤Cψ2(u) for all u∈[0,1]n.
Throughout this paper, all concerned random variables are assumed to be absolutely continuous and nonnegative, and the terms increasing and decreasing stand for non-decreasing and non-increasing, respectively. Denote ei=(0,…,0,1,⏟i0,…,0⏟n−i) for i∈In, and “sgn=” means equality of sign.
In this section, we carry out stochastic comparison of smallest order statistics from dependent and heterogeneous MPHR and MPRHR samples, and the heterogeneity has been considered in the model parameters.
In this subsection, we deal with the case of MPHR samples in the sense of the usual stochastic and hazard rate orders. Let X=(X1,…,Xn) be the random vector, we denote X∼MPHR(α;λ;ˉF;ψ) the sample follows MPHR model, where ˉF is the baseline survival function, ψ is generator of the associated Archimedean survival copula, and α=(α1,…,αn) and λ=(λ1,…,λn) are the tilt parameter vector and modified proportional hazard rate vector, respectively.
Next, we establish sufficient conditions for the usual stochastic order, whenever the modified proportional hazard rate parameters may be different with the tilt parameters being equal.
Theorem 1. For X∼MPHR(α;λ;ˉF;ψ1) and Y∼MPHR(α;μ;ˉF;ψ2), where 0<α≤1. If ψ1 or ψ2 is log-concave, and ϕ2∘ψ1 is super-additive, then λw⪯μ implies
X1:n≤stY1:n. |
Proof. The survival function of X1:n can be expressed as
ˉFX1:n(x)=ψ1(n∑i=1ϕ1(α(ˉF(x))λi1−ˉα(ˉF(x))λi))=J1(α,λ,ψ1,ˉF(x)),x≥0. |
Assume that ψ1 is log-concave. In order to obtain the required result, according to Lemma 2, it is sufficient to show that J1(α,λ,ψ1,ˉF(x)) is decreasing in λi and Schur-convex in λ for given x≥0 and 0<α≤1. Taking the partial derivative of J1(α,λ,ψ1,ˉF(x)) with respect to λi, i∈In, we have
∂J1(α,λ,ψ1,ˉF(x))∂λi=ψ′1(n∑i=1ϕ1(α(ˉF(x))λi1−ˉα(ˉF(x))λi))lnˉF(x)α(ˉF(x))λi1−ˉα(ˉF(x))λiψ′(ϕ1(α(ˉF(x))λi1−ˉα(ˉF(x))λi))(1−ˉα(ˉF(x))λi)≤0. |
That is, J1(α,λ,ψ1,ˉF(x)) is decreasing in λi. Furthermore, for i≠j, we have
(λi−λj)(∂J1(α,λ,ψ1,ˉF(x))∂λi−∂J1(α,λ,ψ1,ˉF(x))∂λj)=ψ′1(n∑i=1ϕ1(α(ˉF(x))λi1−ˉα(ˉF(x))λi))lnˉF(x)(λi−λj)(h1(λi)−h1(λj)), |
where
h1(λ)=α(ˉF(x))λ1−ˉα(ˉF(x))λψ′1(ϕ1(α(ˉF(x))λ1−ˉα(ˉF(x))λ))(1−ˉα(ˉF(x))λ). |
Since the log-concavity of ψ1 implies the increasing property of ψ1/ψ′1, and consider that ϕ1(α(ˉF(x))λ/(1−ˉα(ˉF(x))λ)) is increasing in λ, then we have
α(ˉF(x))λ1−ˉα(ˉF(x))λψ′1(ϕ1(α(ˉF(x))λ1−ˉα(ˉF(x))λ))=ψ1(ϕ1(α(ˉF(x))λ1−ˉα(ˉF(x))λ))ψ′1(ϕ1(α(ˉF(x))λ1−ˉα(ˉF(x))λ))≤0. |
On the other hand, 1/(1−ˉα(ˉF(x))λ) is nonnegative and decreasing in λ. Consequently, h1(λ) is increasing in λ, which in turn implies that
(λi−λj)(∂J1(α,λ,ψ1,ˉF(x))∂λi−∂J1(α,λ,ψ1,ˉF(x))∂λj)≥0. |
Thus, Schur-convexity of J1(α,λ,ψ1,ˉF(x)) follows from Lemma 1. Due to Lemma 2, λw⪯μ implies J1(α,λ,ψ1,ˉF(x))≤J1(α,μ,ψ1,ˉF(x)), and note that ϕ2∘ψ1 is super-additive, by Lemma 3, we have J1(α,μ,ψ1,ˉF(x))≤J1(α,μ,ψ2,ˉF(x)). Hence, it holds that
J1(α,λ,ψ1,ˉF(x))≤J1(α,μ,ψ1,ˉF(x))≤J1(α,μ,ψ2,ˉF(x)). |
As a consequence, we conclude that X1:n≤stY1:n. For the case of ψ2 is log-concave, the proof can be obtained in a similar way. Then we complete the proof.
Remark 1. When α=1, MPHR model reduces to PHR model, which just the result established in Theorem 4.1 (ii) of [21].
The next example illustrates the result of Theorem 1.
Example 1. Consider the case of n=3. Let ˉF(x)=e−(ax)b, a>0, 0<b≤1, and generators ψ1(x)=e1−exθ1, 0<θ1≤1, ψ2(x)=(θ2x+1)−1/θ2, θ2>0. Set α=0.4,a=1.2,b=0.5,θ1=0.1,θ2=1.2,λ=(0.4,0.5,0.6)w⪯(0.3,0.4,0.5)=μ. One can check chat ψ1(x) is log-concave. It can be seen that [ϕ2∘ψ1(x)]″=ex(e1θ1−exθ1)−θ2(θ1+exθ2)θ1−2≥0, that is, ϕ2∘ψ1(x) is convex function in x, which implies that ϕ2∘ψ1 is super-additive. To plot the whole of survival curves of X1:3 and Y1:3 on [0,∞), we perform the transformation (x+1)−1:[0,∞)⟼[0,1]. Then it is obvious that X1:3≤stY1:3 is equivalent to (Y1:3+1)−1≤st(X1:3+1)−1. As is seen in Figure 1, the distribution curve of (X1:3+1)−1 is always beneath that of (Y1:3+1)−1, that is, X1:3≤stY1:3, which coincides with the result of Theorem 1.
The following counterexample shows that the condition ψ1(x) or ψ2(x) is log-concave given in the above Theorem 1 can't be dropped.
Counterexample 1. Under the setup of Example 1, let generators ψ1(x)=(θ1x+1)−1/θ1,ψ2(x)=(θ2x+1)−1/θ2, θi>0,i=1,2. Note that [logψi(x)]″=θi(1+θix)−2≥0 for x≥0, thus, ψi(x) is log-convex. Take θ1=10,θ2=1.2. As is seen in Figure 2, the survival function ˉFX1:3(x) is not always beneath that of ˉGY1:3(x), that is, neither X1:3≤stY1:3 nor X1:3≥stY1:3.
The following theorem presents the usual stochastic order on sample minimum, here, we assume that the two samples have common modified proportional hazard rates parameters.
Theorem 2. For X∼MPHR(α;λ;ˉF;ψ1) and Y∼MPHR(β;λ;ˉF;ψ2). If ϕ1∘ψ2 is super-additive, then αw⪯β implies
X1:n≥stY1:n. |
Proof. The survival function of X1:n can be written as
ˉFX1:n(x)=ψ1(n∑i=1ϕ1(αi(ˉF(x))λ1−ˉαi(ˉF(x))λ))=J2(α,λ,ψ1,ˉF(x)),x≥0. |
To obtain the required result, it suffices to show that the J2(α,λ,ψ1,ˉF(x)) is increasing in αi and Schur-concave in α, i∈In. Differentiating J2(α,λ,ψ1,ˉF(x)) with respect to αi, we obtain
∂J2(α,λ,ψ1,ˉF(x))∂αi=ψ′1(n∑i=1ϕ1(αi(ˉF(x))λ1−ˉαi(ˉF(x))λ))(ˉF(x))λ(1−(ˉF(x))λ)ψ′1(ϕ1(αi(ˉF(x))λ1−ˉαi(ˉF(x))λ))(1−ˉαi(ˉF(x))λ)2≥0. |
That is, J2(α,λ,ψ1,ˉF(x)) is increasing in αi. Furthermore, for i≠j, we have
(αi−αj)(∂J2(α,λ,ψ1,ˉF(x))∂αi−∂J2(α,λ,ψ1,ˉF(x))∂αj)=ψ′1(n∑i=1ϕ1(αi(ˉF(x))λ1−ˉαi(ˉF(x))λ))(ˉF(x))λ(1−(ˉF(x))λ)(αi−αj)(1h2(αi)−1h2(αj)), |
where
h2(α)=(1−ˉα(ˉF(x))λ)2ψ′1(ϕ1(α(ˉF(x))λ1−ˉα(ˉF(x))λ)). |
By the decreasing and convexity of ψ1, it holds that
∂h2(α)∂α=2(ˉF(x))λ(1−ˉα(ˉF(x))λ)ψ′1(ϕ1(α(ˉF(x))λ1−ˉα(ˉF(x))λ))+(ˉF(x))λ(1−(ˉF(x))λ)ψ″1(ϕ1(α(ˉF(x))λ1−ˉα(ˉF(x))λ))ψ′1(ϕ1(α(ˉF(x))λ1−ˉα(ˉF(x))λ))≤0. |
Hence, h2(α) is negative and decreasing in α, or equivalently, 1/h2(α) is negative and increasing in α. Then, for i≠j,
(αi−αj)(∂J2(α,λ,ψ1,ˉF(x))∂αi−∂J2(α,λ,ψ1,ˉF(x))∂αj)≤0. |
It follows from Lemma 1 that J2(α,λ,ψ1,ˉF(x)) is Schur-concave, which is equivalent to −J2(α,λ,ψ1,ˉF(x)) is Schur-convex. According to Lemma 2, αw⪯β implies −J2(α,λ,ψ1,ˉF(x))≤−J2(β,λ,ψ1,ˉF(x)), and note that ϕ1∘ψ2 is super-additive, it holds by Lemma 3 that J2(β,λ,ψ1,ˉF(x))≥J2(β,λ,ψ2,ˉF(x)). Hence,
J2(α,λ,ψ1,ˉF(x))≥J2(β,λ,ψ1,ˉF(x))≥J2(β,λ,ψ2,ˉF(x)). |
That is, X1:n≥stY1:n. The desired result then follows.
The following example demonstrates the theoretical result of Theorem 2.
Example 2. Let ˉF(x)=e−(ax)b,a>0, 0<b≤1, and generators ψ1(x)=(θx+1)−1/θ, θ>0,ψ2(x)=e−x. Take n=3,λ=0.4,a=0.5,b=0.8,θ=0.7 and α=(0.3,0.5,0.7)w⪯(0.2,0.4,0.5)=β. It is easy to check that the conditions of Theorem 2 are all satisfied. The distribution functions of (X1:3+1)−1 and (Y1:3+1)−1 are displayed in Figure 3, which confirms X1:3≥stY1:3.
The next theorem gives sufficient conditions guaranteeing the hazard rate order between two modified proportional hazard rates models with the same modified proportional hazard rates parameters and the heterogeneous tilt parameters.
Theorem 3. For X∼MPHR(α;λ;ˉF;ψ) and Y∼MPHR(β;λ;ˉF;ψ) with log-concave ψ, and −ψ′/ψ is log-convex. If αw⪯β, then
X1:n≥hrY1:n. |
Proof. For convenience, let us give the following facts for any x≥0.
ϱ1: ψ(x)≥0 and ψ′(x)≤0.
ϱ2: The log-concave ψ implies the decreasing ψ′/ψ and hence ψ″(x)ψ(x)≤[ψ′(x)]2.
ϱ3: The log-convex −ψ′/ψ implies that {ψ″(x)ψ(x)−[ψ′(x)]2}/ψ(x)ψ′(x)≥0 increases in x≥0.
Denote h(x) the hazard rate function corresponding to the baseline survival function ˉF(x). The survival function of X1:n can be written as
ˉFX1:n(x)=ψ(n∑i=1ϕ(αi(ˉF(x))λ1−ˉαi(ˉF(x))λ)),x≥0, |
and the hazard rate function of X1:n is
hX1:n(x)=ψ′(∑ni=1ϕ(αi(ˉF(x))λ1−ˉαi(ˉF(x))λ))ψ(∑ni=1ϕ(αi(ˉF(x))λ1−ˉαi(ˉF(x))λ))n∑i=1λh(x)1−ˉαi(ˉF(x))λψ(ϕ(αi(ˉF(x))λ1−ˉαi(ˉF(x))λ))ψ′(ϕ(αi(ˉF(x))λ1−ˉαi(ˉF(x))λ))=L1(x,α,λ,ψ). |
Likewise, Y1:n gets the hazard rate function hY1:n(x)=L1(x,β,λ,ψ) for x≥0. Further denote
A1(α(s,t),x)=∑i≠s,tλh(x)1−ˉαi(ˉF(x))λB1(αiei,x),B1(α,x)=ψ(∑ni=1ϕ(αi(ˉF(x))λ1−ˉαi(ˉF(x))λ))ψ′(∑ni=1ϕ(αi(ˉF(x))λ1−ˉαi(ˉF(x))λ)), |
Jψ(x)=ψ″(x)ψ(x)−(ψ′(x))2(ψ(x))2,C1(α,x)=Jψ(n∑i=1ϕ(αi(ˉF(x))λ1−ˉαi(ˉF(x))λ))B1(α,x). |
Then, for any s,t∈In with s≠t and all ui∈[0,1] (i∈In), we have
∂L1(x,α,λ,ψ)∂αs=(ˉF(x))λ(1−(ˉF(x))λ)(1−ˉαs(ˉF(x))λ)2ψ′(ϕ(αs(ˉF(x))λ1−ˉαs(ˉF(x))λ))n∑i=1λh(x)1−ˉαi(ˉF(x))λB1(αiei,x)Jψ(n∑i=1ϕ(αi(ˉF(x))λ1−ˉαi(ˉF(x))λ))−B1(αses,x)B1(α,x)λh(x)(ˉF(x))λ(1−ˉαs(ˉF(x))λ)2−B21(αses,x)B1(α,x)λh(x)(1−ˉαs(ˉF(x))λ)ψ′(ϕ(αs(ˉF(x))λ1−ˉαs(ˉF(x))λ))(ˉF(x))λ(1−(ˉF(x))λ)(1−ˉαs(ˉF(x))λ)2Jψ(ϕ(αs(ˉF(x))λ1−ˉαs(ˉF(x))λ))=[(ˉF(x))λ(1−(ˉF(x))λ)A1(α(s,t),x)(1−ˉαs(ˉF(x))λ)2ψ′(ϕ(αs(ˉF(x))λ1−ˉαs(ˉF(x))λ))+(ˉF(x))λ(1−(ˉF(x))λ)(1−ˉαs(ˉF(x))λ)2ψ′(ϕ(αs(ˉF(x))λ1−ˉαs(ˉF(x))λ))λh(x)B1(αses,x)1−ˉαs(ˉF(x))λ+(ˉF(x))λ(1−(ˉF(x))λ)(1−ˉαs(ˉF(x))λ)2ψ′(ϕ(αs(ˉF(x))λ1−ˉαs(ˉF(x))λ))λh(x)1−ˉαt(ˉF(x))λB1(αtet,x)]⋅Jψ(n∑i=1ϕ(αi(ˉF(x))λ1−ˉαi(ˉF(x))λ))−B1(αses,x)B1(α,x)λh(x)(ˉF(x))λ(1−ˉαs(ˉF(x))λ)2−B1(αses,x)C1(αses,x)B1(α,x)ψ′(ϕ(αs(ˉF(x))λ1−ˉαs(ˉF(x))λ))λh(x)1−ˉαs(ˉF(x))λ(ˉF(x))λ(1−(ˉF(x))λ)(1−ˉαs(ˉF(x))λ)2. |
By ϱ3, it holds that C1(α,x)≥C1(αses,x), and in combination ϱ1 with ϱ2, we have
∂L1(x,α,λ,ψ)∂αs=[(ˉF(x))λ(1−(ˉF(x))λ)A1(α(s,t),x)(1−ˉαs(ˉF(x))λ)2ψ′(ϕ(αs(ˉF(x))λ1−ˉαs(ˉF(x))λ))+(ˉF(x))λ(1−(ˉF(x))λ)(1−ˉαs(ˉF(x))λ)2ψ′(ϕ(αs(ˉF(x))λ1−ˉαs(ˉF(x))λ))λh(x)B1(αtet,x)1−ˉαt(ˉF(x))λ]⋅Jψ(n∑i=1ϕ(αi(ˉF(x))λ1−ˉαi(ˉF(x))λ))−B1(αses,x)B1(α,x)λh(x)(ˉF(x))λ(1−ˉαs(ˉF(x))λ)2+(ˉF(x))λ(1−(ˉF(x))λ)(1−ˉαs(ˉF(x))λ)2ψ′(ϕ(αs(ˉF(x))λ1−ˉαs(ˉF(x))λ))λh(x)1−ˉαs(ˉF(x))λB1(αses,x)(C1(α,x)−C1(αses,x))B1(α,x)≤0. |
Therefore, L1(x,α,λ,ψ) is decreasing in αi for any i∈In. Furthermore, for s,t∈In with s≠t, we obtain
(αs−αt)(∂L1(x,α,λ,ψ)∂αs−∂L1(x,α,λ,ψ)∂αt)=(αs−αt)Jψ(n∑i=1ϕ(αi(ˉF(x))λ1−ˉαi(ˉF(x))λ))(ˉF(x))λ(1−(ˉF(x))λ)A1(α(s,t),x)⋅[1(1−ˉαs(ˉF(x))λ)2ψ′(ϕ(αs(ˉF(x))λ1−ˉαs(ˉF(x))λ))−1(1−ˉαt(ˉF(x))λ)2ψ′(ϕ(αt(ˉF(x))λ1−ˉαt(ˉF(x))λ))]+(αs−αt)(1αs−1αt)Jψ(n∑i=1ϕ(αi(ˉF(x))λ1−ˉαi(ˉF(x))λ))(1−(ˉF(x))λ)λh(x)B1(αses,x)B1(αtet,x)(1−ˉαs(ˉF(x))λ)(1−ˉαt(ˉF(x))λ)+(αs−αt)λh(x)(ˉF(x))λB1(α,x)(B1(αtet,x)(1−ˉαt(ˉF(x))λ)2−B1(αses,x)(1−ˉαs(ˉF(x))λ)2)+(αs−αt)λ h(x)(ˉF(x))λ(1−(ˉF(x))λ)B1(α,x)⋅[B1(αses,x)(C1(α,x)−C1(αses,x))(1−ˉαs(ˉF(x))λ)3ψ′(ϕ(αs(ˉF(x))λ1−ˉαs(ˉF(x))λ))−B1(αtet,x)(C1(α,x)−C1(αtet,x))(1−ˉαt(ˉF(x))λ)3ψ′(ϕ(αt(ˉF(x))λ1−ˉαt(ˉF(x))λ))]sgn=−(αs−αt)C1(α,x)(ˉF(x))λ(1−(ˉF(x))λ)A1(α(s,t),x)⋅[1(1−ˉαs(ˉF(x))λ)2ψ′(ϕ(αs(ˉF(x))λ1−ˉαs(ˉF(x))λ))−1(1−ˉαt(ˉF(x))λ)2ψ′(ϕ(αt(ˉF(x))λ1−ˉαt(ˉF(x))λ))]−(αs−αt)(1αs−1αt)C1(α,x)(1−(ˉF(x))λ)λh(x)B1(αses,x)B1(αtet,x)(1−ˉαs(ˉF(x))λ)(1−ˉαt(ˉF(x))λ)−(αs−αt)λh(x)(ˉF(x))λ(B1(αtet,x)(1−ˉαt(ˉF(x))λ)2−B1(αses,x)(1−ˉαs(ˉF(x))λ)2)−(αs−αt)λh(x)(ˉF(x))λ(1−(ˉF(x))λ)⋅[B1(αses,x)(C1(α,x)−C1(αses,x))(1−ˉαs(ˉF(x))λ)3ψ′(ϕ(αs(ˉF(x))λ1−ˉαs(ˉF(x))λ))−B1(αtet,x)(C1(α,x)−C1(αtet,x))(1−ˉαt(ˉF(x))λ)3ψ′(ϕ(αt(ˉF(x))λ1−ˉαt(ˉF(x))λ))]. |
As 1/h2(α) is increasing in α, it holds that
−(αs−αt)C1(α,x)(ˉF(x))λ(1−(ˉF(x))λ)A1(α(s,t),x)⋅[1(1−ˉαs(ˉF(x))λ)2ψ′(ϕ(αs(ˉF(x))λ1−ˉαs(ˉF(x))λ))−1(1−ˉαt(ˉF(x))λ)2ψ′(ϕ(αt(ˉF(x))λ1−ˉαt(ˉF(x))λ))]≥0. | (3.1) |
Likewise, for αs≥αt, we have
−(αs−αt)(1αs−1αt)C1(α,x)(1−(ˉF(x))λ)λh(x)B1(αses,x)B1(αtet,x)(1−ˉαs(ˉF(x))λ)(1−ˉαt(ˉF(x))λ)≥0. | (3.2) |
Consider that 1/(1−ˉαi(ˉF(x))λ)2≥0 is decreasing in αi, ϱ2 implies that B1(αiei,x) is increasing in αi, and B1(αtet,x)≤B1(αses,x)≤0 for αs≥αt. Then
−(αs−αt)λh(x)(ˉF(x))λ(B1(αtet,x)(1−ˉαt(ˉF(x))λ)2−B1(αses,x)(1−ˉαs(ˉF(x))λ)2)≥0. | (3.3) |
Note that 1/(1−ˉαi(ˉF(x))λ)≥0 is decreasing in αi and 1/h2(αi) is increasing in αi. It is clear that
1(1−ˉαs(ˉF(x))λ)3ψ′(ϕ(αs(ˉF(x))λ1−ˉαs(ˉF(x))λ)) |
is increasing in αi for αs≥αt. By ϱ3, for αs≥αt, we have C1(α,x)−C1(αtet,x)≥C1(α,x)−C1(αses,x)≥0. It holds that
−(αs−αt)λh(x)(ˉF(x))λ(1−(ˉF(x))λ)⋅[B1(αses,x)(C1(α,x)−C1(αses,x))(1−ˉαs(ˉF(x))λ)3ψ′(ϕ(αs(ˉF(x))λ1−ˉαs(ˉF(x))λ))−B1(αtet,x)(C1(α,x)−C1(αtet,x))(1−ˉαt(ˉF(x))λ)3ψ′(ϕ(αt(ˉF(x))λ1−ˉαt(ˉF(x))λ))]≥0. | (3.4) |
Combing (3.1)-(3.4), we conclude that L1(x,α,λ,ψ) is Schur-convex with respect to α. Thus, according to Lemma 1 and Lemma 2, αw⪯β implies hX1:n(x)=L1(x,α,λ,ψ)≤L1(x,β,λ,ψ)=hY1:n(x) for all x, that is, X1:n≥hrY1:n.
Remark 2. For two independent samples, we have ψ(x)=e−x, thus Theorem 3 serves as a generalization of Theorem 3.4 (ii) of [6] to the case of dependent samples with Archimedean survival copulas.
We present the following example to illustrate the result of Theorem 3.
Example 3. Let ˉF(x)=e−(ax)b,a>0, 0<b≤1, and generator ψ(x)=e1−exθ, 0<θ≤0.5(3−√5). Set n=3,λ=0.03,a=5,b=0.007,θ=0.1 and α=(0.8,0.6,0.4)w⪯(0.7,0.5,0.3)=β. One can verify that the conditions of Theorem 3 are all satisfied. Figure 4 plots the reversed hazard rate functions of (X1:3+1)−1 and (Y1:3+1)−1, and it is obvious that X1:3≥hrY1:3 is equivalent to (X1:3+1)−1≤rh(Y1:3+1)−1, which asserts X1:3≥hrY1:3.
In this subsection, we consider the case of MPRHR samples. Let X=(X1,…,Xn) be the random vector, and X∼MPRHR(α;β;F;ψ), where F is the baseline distribution function, ψ is generator of the associated Archimedean copula, and α=(α1,…,αn) and β=(β1,…,βn) are the tilt parameter vector and modified proportional reversed hazard rate vector, respectively.
Next, we present two results on the heterogeneity among parameters in terms of the usual stochastic order. The proofs can be obtained along the same way with that of Theorem 1, and thus omitted here.
Theorem 4. For X∼MPRHR(α;β;F;ψ1) and Y∼MPRHR(α;γ;F;ψ2), where 0<α≤1. If ψ1 or ψ2 is log-concave, and ϕ1∘ψ2 is super-additive, then βw⪯γ implies
X1:n≥stY1:n. |
Theorem 5. For X∼MPRHR(α;β;F;ψ1) and Y∼MPRHR(υ;β;F;ψ2). If ψ1 or ψ2 is log-concave, and ϕ2∘ψ1 is super-additive, then αw⪯υ implies
X1:n≤stY1:n. |
The next example is provided to illustrate the result of Theorem 4.
Example 4. Let ˉF(x)=e−(ax)b,a>0, 0<b≤1, and generators ψ1(x)=(θ1x+1)−1/θ1, θ1>0,ψ2(x)=e1−exθ2, 0<θ2≤1. Set n=3,α=0.3,θ1=0.8,θ2=0.9,a=0.1,b=0.9,β=(0.3,0.3,0.4)w⪯(0.2,0.2,0.3)=γ. It is easy to check that all conditions of Theorem 4 are satisfied. The distribution functions of (X1:3+1)−1 and (Y1:3+1)−1 are plotted in Figure 5, from which we can confirm that X1:3≥stY1:3.
The following theorem compares two sample minimums with common modified proportional reversed hazard rates parameters and heterogeneous tilt parameters in the sense of the hazard rate order. The proof is similar to that of Theorem 3 and hence omitted.
Theorem 6. For X∼MPRHR(α;β;F;ψ) and Y∼MPRHR(υ;β;F;ψ) with log-concave ψ, and −ψ′/ψ is log-convex. If αw⪯υ, then
X1:n≤hrY1:n. |
Remark 3. It is mentioned that Theorem 6 generalizes Theorem 4.5 (ii) of [6] to the case of dependent samples with Archimedean copulas.
Remark 4. According to the Theorem 2.1 of Navarro et al.[10], the coherent system reliability function can be written as ˉFX(x)=h(ˉF(x)), where h only depends on structure function and on the survival copula of (X1,…,Xn). Owing to the complexity of coherent system, we only studied the series system in Section 3 by distored distribution. Based on Archimedean copula K, we obtained the distorted function representations of series system as follows:
h3(u;α,λ)=K(α1(u)λ11−ˉα1(u)λ1,…,αi(u)λi1−ˉαi(u)λi,…,αn(u)λn1−ˉαn(u)λn), | (3.5) |
h4(u;α,β)=K(1−(1−u)β11−ˉα1(1−u)β1,…,1−(1−u)βi1−ˉαi(1−u)βi,…,1−(1−u)βn1−ˉαn(1−u)βn),u=ˉF(x), | (3.6) |
where h3(u;α,λ) and h4(u;α,β) are obtained by MPHR and MPRHR models. In (3.5), if αi=α(i=1,…,n), we can obtain representation of Theorem 1, when λi=λ(i=1,…,n), we can obtain representation of Theorem 2 and Theorem 3. As for in other Theorem 4, Theorem 5, and Theorem 6, we can obtain in similar way by (3.6).
In parallel to the previous section, here, we consider the largest order statistics from dependent and heterogeneous MPHR and MPRHR samples.
In this subsection, we deal with the case of MPHR samples in the sense of the usual stochastic and reversed hazard rate orders. The first theorem establishes sufficient conditions for the usual stochastic order, which can be verified in a similar method with that of Theorem 1, and thus omitted for brevity.
Theorem 7. For X∼MPHR(α;λ;ˉF;ψ1) and Y∼MPHR(α;μ;ˉF;ψ2), where 0<α≤1. If ψ1 or ψ2 is log-concave, and ϕ1∘ψ2 is super-additive, then λw⪯μ implies
Xn:n≤stYn:n. |
In the following, we give a numerical example to illustrate the effectiveness of Theorem 7.
Example 5. Under the setup of Example 4, set n=3,α=0.9,θ1=0.9,θ2=2,a=0.5,b=0.3,λ=(4,5,6)w⪯(3,4,5)=μ. One can check that the conditions of Theorem 7 are satisfied. The survival functions of (X3:3+1)−1 and (Y3:3+1)−1 are plotted in Figure 6, which verifies that FX3:3(x)≥GY3:3(x). That is, \ X3:3≤stY3:3.
The following theorem conducts stochastic comparisons of samples with heterogeneous tilt parameters in the sense of the usual stochastic order.
Theorem 8. For X∼MPHR(α;λ;ˉF;ψ1) and Y∼MPHR(β;λ;ˉF;ψ2). If ψ1 or ψ2 is log-concave, and ϕ2∘ψ1 is super-additive, then αw⪯β implies
Xn:n≥stYn:n. |
Proof. The distribution function Xn:n is given by
FXn:n(x)=ψ1(n∑i=1ϕ1(1−(ˉF(x))λ1−ˉαi(ˉF(x))λ))=J3(α,λ,ψ1,ˉF(x)),x≥0. |
Without loss of generality, we assume that ψ1 is log-concave. In order to obtain the desired result, we just need to prove that J3(α,λ,ψ1,ˉF(x)) is decreasing in αi and Schur-convex in α, i∈In. Taking the partial derivative of J3(α,λ,ψ1,ˉF(x)) with respect to αi, we get
∂J3(α,λ,ψ1,ˉF(x))∂αi=ψ′1(n∑i=1ϕ1(1−(ˉF(x))λ1−ˉαi(ˉF(x))λ))−(ˉF(x))λ(1−(ˉF(x))λ1−ˉαi(ˉF(x))λ)ψ′1(ϕ1(1−(ˉF(x))λ1−ˉαi(ˉF(x))λ))(1−ˉαi(ˉF(x))λ)≤0. |
That is, J3(α,λ,ψ1,ˉF(x)) is decreasing in αi. Furthermore, for i≠j, it holds that
(αi−αj)(∂J3(α,λ,ψ1,ˉF(x))∂αi−∂J3(α,λ,ψ1,ˉF(x))∂αj)=−ψ′1(n∑i=1ϕ1(1−(ˉF(x))λ1−ˉαi(ˉF(x))λ))(ˉF(x))λ(αi−αj)(h3(αi)−h3(αj)), |
where
h3(α)=1−(ˉF(x))λ1−ˉα(ˉF(x))λψ′1(ϕ1(1−(ˉF(x))λ1−ˉα(ˉF(x))λ))(1−ˉα(ˉF(x))λ). |
Since the log-concavity of ψ1 implies the increasing property of ψ1/ψ′1, and consider that ϕ1(1−(ˉF(x))λ/(1−ˉα(ˉF(x))λ)) is increasing in α, then we have
1−(ˉF(x))λ1−ˉα(ˉF(x))λψ′1(ϕ1(1−(ˉF(x))λ1−ˉα(ˉF(x))λ))=ψ1(ϕ1(1−(ˉF(x))λ1−ˉα(ˉF(x))λ))ψ′1(ϕ1(1−(ˉF(x))λ1−ˉα(ˉF(x))λ)) |
is negative and is increasing in α for every fixed x≥0. In addition, 1/(1−ˉα(ˉF(x))λ)≥0 is decreasing in α. Consequently, h3(α) is increasing in α which in turn implies that
(αi−αj)(∂J3(α,λ,ψ1,ˉF(x))∂αi−∂J3(α,λ,ψ1,ˉF(x))∂αj)≥0. |
Therefore, J3(α,λ,ψ1,ˉF(x)) is decreasing in αi and Schur-convex in α. By Lemma 2, αw⪯β implies J3(α,λ,ψ1,ˉF(x))≤J3(β,λ,ψ1,ˉF(x)), and note that ϕ2∘ψ1 is super-additive, we can conclude by Lemma 3 that J3(β,λ,ψ1,ˉF(x))≤J3(β,λ,ψ2,ˉF(x)). Hence,
J3(α,λ,ψ1,ˉF(x))≤J3(β,λ,ψ1,ˉF(x))≤J3(β,λ,ψ2,ˉF(x)), |
which implies that Xn:n≥stYn:n. The proof can be completed.
The following numerical example is provided to demonstrate the theoretical result of Theorem 8.
Example 6. Let ˉF(x)=e−(ax)b,a>0, 0<b≤1, and generators ψ1(x)=e−x, ψ2(x)=(θx+1)−1/θ, θ>0. Take n=3,λ=0.8,a=0.5,b=0.2,θ=2 and α=(0.3,0.5,0.7)w⪯(0.2,0.4,0.6)=β. One can check chat the conditions of Theorem 8 are satisfied. The survival functions of (X3:3+1)−1 and (Y3:3+1)−1 are plotted in Figure 7, that is X3:3≥stY3:3, which verifies the result of Theorem 8.
In the next theorem, we provide a sufficient condition to obtain the reversed hazard rate order between two modified proportional hazard rates models when the modified proportional hazard rates parameters are equal and the tilt parameters are heterogeneous.
Theorem 9. For X∼MPHR(α;λ;ˉF;ψ) and Y∼MPHR(β;λ;ˉF;ψ) with log-concave ψ. Assume that −ψ′/ψ is log-convex. If αw⪯β, then
Xn:n≥rhYn:n. |
Proof. The distribution function Xn:n is given by
FXn:n(x)=ψ(n∑i=1ϕ(1−(ˉF(x))λ1−ˉαi(ˉF(x))λ)),x≥0, |
and the reversed hazard rate function of Xn:n is
rXn:n(x)=ψ′(∑ni=1ϕ(1−(ˉF(x))λ1−ˉαi(ˉF(x))λ))ψ(∑ni=1ϕ(1−(ˉF(x))λ1−ˉαi(ˉF(x))λ))n∑i=1λ(ˉF(x))λh(x)1−(ˉF(x))λαi1−ˉαi(ˉF(x))λψ(ϕ(1−(ˉF(x))λ1−ˉαi(ˉF(x))λ))ψ′(ϕ(1−(ˉF(x))λ1−ˉαi(ˉF(x))λ))=L2(x,α,λ,ψ). |
Similiarly, Yn:n gets the reversed hazard rate function rYn:n(x)=L2(x,β,λ,ψ) for x≥0. Further denote
A2(α(s,t),x)=∑i≠s,tλ(ˉF(x))λh(x)1−(ˉF(x))λαiB2(αiei,x)1−ˉαi(ˉF(x))λ,B2(α,x)=ψ(∑ni=1ϕ(1−(ˉF(x))λ1−ˉαi(ˉF(x))λ))ψ′(∑ni=1ϕ(1−(ˉF(x))λ1−ˉαi(ˉF(x))λ)), |
Jψ(x)=ψ″(x)ψ(x)−(ψ′(x))2(ψ(x))2,C2(α,x)=Jψ(n∑i=1ϕ(1−(ˉF(x))λ1−ˉαi(ˉF(x))λ))B2(α,x). |
Note that, for s,t∈In with s≠t, and ui∈[0,1] (i∈In), we have
∂L2(x,α,λ,ψ)∂αs=(ˉF(x))λ((ˉF(x))λ−1)(1−ˉαs(ˉF(x))λ)2ψ′(ϕ(1−(ˉF(x))λ1−ˉαs(ˉF(x))λ))n∑i=1λ(ˉF(x))λh(x)1−(ˉF(x))λαiB2(αiei,x)1−ˉαi(ˉF(x))λJψ(n∑i=1ϕ(1−(ˉF(x))λ1−ˉαi(ˉF(x))λ))+B2(αses,x)B2(α,x)λh(x)(ˉF(x))λ(1−ˉαs(ˉF(x))λ)2−B22(αses,x)B2(α,x)(ˉF(x))λ((ˉF(x))λ−1)(1−ˉαs(ˉF(x))λ)3ψ′(ϕ(1−(ˉF(x))λ1−ˉαs(ˉF(x))λ))αsλh(x)(ˉF(x))λ(1−(ˉF(x))λ)Jψ(ϕ(1−(ˉF(x))λ1−ˉαs(ˉF(x))λ))=[(ˉF(x))λ((ˉF(x))λ−1)A2(α(s,t),x)(1−ˉαs(ˉF(x))λ)2ψ′(ϕ(1−(ˉF(x))λ1−ˉαs(ˉF(x))λ))+(ˉF(x))λ((ˉF(x))λ−1)αsB2(αses,x)(1−ˉαs(ˉF(x))λ)3ψ′(ϕ(1−(ˉF(x))λ1−ˉαs(ˉF(x))λ))λ(ˉF(x))λh(x)1−(ˉF(x))λ+(ˉF(x))λ((ˉF(x))λ−1)(1−ˉαs(ˉF(x))λ)2ψ′(ϕ(1−(ˉF(x))λ1−ˉαs(ˉF(x))λ))λ(ˉF(x))λh(x)1−(ˉF(x))λαtB2(αtet,x)1−ˉαt(ˉF(x))λ]Jψ(n∑i=1ϕ(1−(ˉF(x))λ1−ˉαi(ˉF(x))λ))+B2(αses,x)B2(α,x)λh(x)(ˉF(x))λ(1−ˉαs(ˉF(x))λ)2−B2(αses,x)C2(αses,x)B2(α,x)(ˉF(x))λ((ˉF(x))λ−1)(1−ˉαs(ˉF(x))λ)3ψ′(ϕ(1−(ˉF(x))λ1−ˉαs(ˉF(x))λ))αsλh(x)(ˉF(x))λ(1−(ˉF(x))λ). |
By ϱ1, ϱ2 and C1(α,x)≥C1(αses,x), it holds that
∂L2(x,α,λ,ψ)∂αs=[(ˉF(x))λ((ˉF(x))λ−1)A2(α(s,t),x)(1−ˉαs(ˉF(x))λ)2ψ′(ϕ(1−(ˉF(x))λ1−ˉαs(ˉF(x))λ))+(ˉF(x))λ((ˉF(x))λ−1)(1−ˉαs(ˉF(x))λ)2ψ′(ϕ(1−(ˉF(x))λ1−ˉαs(ˉF(x))λ))λ(ˉF(x))λh(x)(1−(ˉF(x))λ)⋅αtB2(αtet,x)(1−ˉαt(ˉF(x))λ)]Jψ(n∑i=1ϕ(1−(ˉF(x))λ1−ˉαi(ˉF(x))λ))+B2(αses,x)B2(α,x)λh(x)(ˉF(x))λ(1−ˉαs(ˉF(x))λ)2+(ˉF(x))λ((ˉF(x))λ−1)(1−ˉαs(ˉF(x))λ)3ψ′(ϕ(1−(ˉF(x))λ1−ˉαs(ˉF(x))λ))αsλh(x)(ˉF(x))λ(1−(ˉF(x))λ)B2(αsesx)(C2(α,x)−C2(αses,x))B2(α,x)≥0. |
As a result, L2(x,α,λ,ψ) is increasing in αi, for any i∈In, and for s,t∈In with s≠t,
(αs−αt)(∂L2(x,α,λ,ψ)∂αs−∂L2(x,α,λ,ψ)∂αt)=−(αs−αt)Jψ(n∑i=1ϕ(1−(ˉF(x))λ1−ˉαi(ˉF(x))λ))(ˉF(x))λA1(α(s,t),x)⋅[(1−(ˉF(x))λ)(1−ˉαs(ˉF(x))λ)2ψ′(ϕ(1−(ˉF(x))λ1−ˉαs(ˉF(x))λ))−(1−(ˉF(x))λ)(1−ˉαt(ˉF(x))λ)2ψ′(ϕ(1−(ˉF(x))λ1−ˉαt(ˉF(x))λ))]+(αs−αt)2Jψ(n∑i=1ϕ(1−(ˉF(x))λ1−ˉαi(ˉF(x))λ))λ(ˉF(x))2λh(x)1−(ˉF(x))λB2(αses,x)B2(αtet,x)(1−ˉαs(ˉF(x))λ)(1−ˉαt(ˉF(x))λ)−(αs−αt)λh(x)(ˉF(x))λB2(α,x)(B2(αtet,x)(1−ˉαt(ˉF(x))λ)2−B2(αses,x)(1−ˉαs(ˉF(x))λ)2)+(αs−αt)λh(x)(ˉF(x))2λ((ˉF(x))λ−1)(1−(ˉF(x))λ)B2(α,x)⋅[αsB2(αses,x)(C2(α,x)−C2(αses,x))(1−ˉαs(ˉF(x))λ)3ψ′(ϕ(1−(ˉF(x))λ1−ˉαs(ˉF(x))λ))−αtB2(αtet,x)(C2(α,x)−C2(αtet,x))(1−ˉαt(ˉF(x))λ)3ψ′(ϕ(1−(ˉF(x))λ1−ˉαt(ˉF(x))λ))]sgn=(αs−αt)C2(α,x)(ˉF(x))λA2(α(s,t),x)⋅[(1−(ˉF(x))λ)(1−ˉαs(ˉF(x))λ)2ψ′(ϕ(1−(ˉF(x))λ1−ˉαs(ˉF(x))λ))−(1−(ˉF(x))λ)(1−ˉαt(ˉF(x))λ)2ψ′(ϕ(1−(ˉF(x))λ1−ˉαt(ˉF(x))λ))]−(αs−αt)2C2(α,x)λ(ˉF(x))2λh(x)1−(ˉF(x))λB2(αses,x)B2(αtet,x)(1−ˉαs(ˉF(x))λ)(1−ˉαt(ˉF(x))λ)+(αs−αt)λh(x)(ˉF(x))λ(B2(αtet,x)(1−ˉαt(ˉF(x))λ)2−B2(αses,x)(1−ˉαs(ˉF(x))λ)2)+(αs−αt)λh(x)(ˉF(x))2λ(1−(ˉF(x))λ)(1−(ˉF(x))λ)⋅[αsB2(αses,x)(C2(α,x)−C2(αses,x))(1−ˉαs(ˉF(x))λ)3ψ′(ϕ(1−(ˉF(x))λ1−ˉαs(ˉF(x))λ))−αtB2(αtet,x)(C2(α,x)−C2(αtet,x))(1−ˉαt(ˉF(x))λ)3ψ′(ϕ(1−(ˉF(x))λ1−ˉαt(ˉF(x))λ))]. |
Since h3(α) is increasing in α, it holds that
(αs−αt)C2(α,x)(ˉF(x))λA2(α(s,t),x)⋅[(1−(ˉF(x))λ)(1−ˉαs(ˉF(x))λ)2ψ′(ϕ(1−(ˉF(x))λ1−ˉαs(ˉF(x))λ))−(1−(ˉF(x))λ)(1−ˉαt(ˉF(x))λ)2ψ′(ϕ(1−(ˉF(x))λ1−ˉαt(ˉF(x))λ))]≤0. | (4.1) |
Obviously, for αs≥αt,
−(αs−αt)2C2(α,x)λ(ˉF(x))2λh(x)1−(ˉF(x))λB2(αses,x)B2(αtet,x)(1−ˉαs(ˉF(x))λ)(1−ˉαt(ˉF(x))λ)≤0. | (4.2) |
Note that 1/(1−ˉαi(ˉF(x))λ)2≥0 is decreasing in αi, ϱ2 implies that B1(αiei,x) is increasing in αi, and B1(αtet,x)≤B1(αses,x)≤0 for αs≥αt. Then, we have
(αs−αt)λh(x)(ˉF(x))λ(B2(αtet,x)(1−ˉαt(ˉF(x))λ)2−B2(αses,x)(1−ˉαs(ˉF(x))λ)2)≤0. | (4.3) |
Since αi/(1−ˉαi(ˉF(x))λ) and h3(αi) are increasing in αi, and by ϱ3, we have C1(α,x)−C1(αtet,x)≥C1(α,x)−C1(αses,x)≥0 for αs≥αt. It is verified that
(αs−αt)λh(x)(ˉF(x))2λ(1−(ˉF(x))λ)(1−(ˉF(x))λ)⋅[αsB2(αses,x)(C2(α,x)−C2(αses,x))(1−ˉαs(ˉF(x))λ)3ψ′(ϕ(1−(ˉF(x))λ1−ˉαs(ˉF(x))λ))−αtB2(αtet,x)(C2(α,x)−C2(αtet,x))(1−ˉαt(ˉF(x))λ)3ψ′(ϕ(1−(ˉF(x))λ1−ˉαt(ˉF(x))λ))]≤0. | (4.4) |
By (4.1)-(4.4), it is plain that L2(x,α,λ,ψ) is increasing in αi and Schur-concave with respect to α. According to Lemma 1 and Lemma 2, αw⪯β implies −rXn:n(x)=−L2(x,α,λ,ψ)≤−L2(x,β,λ,ψ)=−rYn:n(x) for all x, that is, Xn:n≥rhYn:n. This completes the proof.
Remark 5. Theorem 9 extends Theorem 3.4 (i) of [6] to the case of dependent samples with Archimedean survival copulas.
The following example illustrates the result of Theorem 9.
Example 7. Let ˉF=e−(ax)b,a>0,0<b≤1, and ψ(x)=e1−exθ, 0<θ≤0.5(3−√5). Set n=3,λ=3,a=6,b=0.08,θ=0.3 and α=(0.8,0.6,0.4)w⪯(0.7,0.5,0.3)=β. These satisfy all conditions of Theorem 9, and the hazard rate functions of (X3:3+1)−1 and (Y3:3+1)−1 are displayed in Figure 8, which confirms that X3:3≥rhY3:3.
In this subsection, we consider the case of MPRHR samples. The first two theorems present the comparison results on the heterogeneity among parameters in terms of the usual stochastic order. The proofs can be completed in a similar way with that of Theorem 1, and thus are omitted.
Theorem 10. For X∼MPRHR(α;β;F;ψ1) and Y∼MPRHR(α;γ;F;ψ2), where 0<α≤1. If ψ1 or ψ2 is log-concave, and ϕ2∘ψ1 is super-additive, then βw⪯γ implies
Xn:n≥stYn:n. |
Proof. The distribution function of Xn:n can be represented as
FXn:n(x)=ψ1(n∑i=1ϕ1(α(F(x))βi1−ˉα(F(x))βi))=J4(α,β,ψ1,F(x)),x≥0. |
Suppose that ψ1 is log-concave. To establish the desired result, along with Lemma 2, it suffices to show that for fixed x≥0 and 0<α≤1. J4(α,β,ψ1,F(x)) is decreasing in βi and Schur-convex in β. The partial derivative of J4(α,β,ψ1,F(x)) with respect to βi is
∂J4(α,β,ψ1,F(x))∂βi=ψ′1(n∑i=1ϕ1(α(F(x))βi1−ˉα(F(x))βi))lnF(x)α(F(x))βi1−ˉα(F(x))βiψ′1(ϕ1(α(F(x))βi1−ˉα(F(x))βi))(1−ˉα(F(x))βi)≤0. |
Which clearly shows that J4(α,β,ψ1,F(x)) is decreasing in βi. Now, for i≠j, we get
(βi−βj)(∂J4(α,β,ψ1,F(x))∂βi−∂J4(α,β,ψ1,F(x))∂βj)=ψ′1(n∑i=1ϕ1(α(F(x))βi1−ˉα(F(x))βi))lnF(x)(βi−βj)(h4(βi)−h4(βj)), |
where
h4(β)=α(F(x))β1−ˉα(F(x))βψ′1(ϕ1(α(F(x))β1−ˉα(F(x))β))(1−ˉα(F(x))β). |
As dicussed in the proof of Theorem 1, for each fixded x>0, we have
α(F(x))β1−ˉα(F(x))βψ′1(ϕ1(α(F(x))β1−ˉα(F(x))β))=ψ1(ϕ1(α(F(x))β1−ˉα(F(x))β))ψ′1(ϕ1(α(F(x))β1−ˉα(F(x))β))≤0. |
Moreover, we readily observe that 1/(1−ˉα(F(x))β) is nonnegative and decreasing in β for 0<α≤1. Upon combining these observations, we find h4(β) to be increasing in β. Consequently
(βi−βj)(∂J4(α,β,ψ1,F(x))∂βi−∂J4(α,β,ψ1,F(x))∂βj)≥0. |
Therefore, Schur-convexity of J4(α,β,ψ1,F(x)) follows from Lemma 1. According to Lemma 2, βw⪯γ implies J4(α,β,ψ1,F(x))≤J4(α,γ,ψ1,F(x)), and the assumption ϕ2∘ψ1 is super-additive, by Lemma 3, we have J4(α,γ,ψ1,F(x))≤J4(α,γ,ψ2,F(x)). So, we obtain
J4(α,β,ψ1,F(x))≤J4(α,γ,ψ1,F(x))≤J4(α,γ,ψ2,F(x)). |
It is clear that we conclude FXn:n(x)≤FYn:n(x), that is, Xn:n≥stYn:n. For the case of ψ2 is log-concave, the proof can be obtained in a similar way. This completes the desired proof.
Remark 6. Theorem 10 generalizes the result of Theorem 5.1 (ii) of [21] to the case of MPHR model.
Theorem 11. For X∼MPRHR(α;β;F;ψ1) and Y∼MPRHR(υ;β;F;ψ2). If ϕ1∘ψ2 is super-additive, then αw⪯υ implies
Xn:n≤stYn:n. |
The following example is provided to illustrate the Theorem 11.
Example 8. Under the setup of Example 2, take n=3,λ=0.8,a=0.5,b=0.4,θ=0.6 and α=(0.4,0.5,0.6)w⪯(0.3,0.4,0.5)=υ. These satisfy all conditions of Theorem 11, and the survival functions of (X3:3+1)−1 and (Y3:3+1)−1 are poltted in Figure 9, which asserts X3:3≤stY3:3.
Now, we present comparison result of sample having common modified proportional reversed hazard rates parameters and heterogeneous tilt parameters in terms of the reversed hazard rate order.
Theorem 12. For X∼MPRHR(α;β;F;ψ) and Y∼MPRHR(υ;β;F;ψ) with log-concave ψ, and −ψ′/ψ is log-convex. If αw⪯υ, then
Xn:n≤rhYn:n. |
Remark 7. It should be pointed out that Theorem 12 extends Theorem 4.5 (i) of [6] to the case of dependent samples with Archimedean copulas.
Remark 8. Similarly, according to the Remark 2, based on Archimedean copula K, we obtained the distorted function representations of parallel system as follows:
h5(u;α,λ)=K(1−(1−u)λ11−ˉα1(1−u)λ1,…,1−(1−u)λi1−ˉαi(1−u)λi,…,1−(1−u)λn1−ˉαn(1−u)λn), | (4.5) |
h6(u;α,β)=K(α1(u)β11−ˉα1(u)β1,…,αi(u)βi1−ˉαi(u)βi,…,αn(u)βn1−ˉαn(u)βn),u=ˉF(x), | (4.6) |
where h5(u;α,λ) and h6(u;α,β) are obtained by MPHR and MPRHR models. In (4.5), when αi=α(i=1,…,n), we can obtain representation of Theorem 7, if λi=λ(i=1,…,n), we can obtain representation of Theorem 8 and Theorem 9. As for in other Theorem 10, Theorem 11, and Theorem 12, we can obtain in similar way by (4.6).
In this paper, we study stochastic comparisons on minimums and maximums from heterogeneous MPRHR (MPHR) samples with Archimedean (survival) copulas. Some ordering results are established for the usual stochastic, hazard rate and reversed rate orders on the smallest and largest order statistics. These results generalize some known results in the literature. As a further study, it is of interest to consider other stochastic orders such as likelihood ratio order, star order and dispersive order.
The authors would like to thank the two anonymous reviewers and the Editor for their valuable and constructive comments and suggestions to improve the presentation of this manuscript. This research was supported by the National Natural Science Foundation of China (11861058, 71471148).
The authors declare no conflict of interest.
[1] |
Yuan K, Zheng YB, Wang YJ, et al. (2022) A systematic review and meta-analysis on prevalence of and risk factors associated with depression, anxiety and insomnia in infectious diseases, including COVID-19: a call to action. Mol Psychiatry 27: 3214-3222. https://doi:10.1038/s41380-022-01638-z ![]() |
[2] |
Tselebis A, Pachi A (2022) Primary Mental Health Care in a New Era. Healthcare 10: 2025. https://doi.org/10.3390/healthcare10102025 ![]() |
[3] |
Sikaras C, Ilias I, Tselebis A, et al. (2021) Nursing staff fatigue and burnout during the COVID-19 pandemic in Greece. AIMS Public Health 9: 94-105. https://doi:10.3934/publichealth.2022008 ![]() |
[4] |
Schmuck J, Hiebel N, Rabe M, et al. (2021) Sense of coherence, social support and religiosity as resources for medical personnel during the COVID-19 pandemic: A web-based survey among 4324 health care workers within the German Network University Medicine. PLoS One 16: e0255211. https://doi:10.1371/journal.pone.0255211 ![]() |
[5] |
Dong ZQ, Ma J, Hao YN, et al. (2020) The social psychological impact of the COVID-19 pandemic on medical staff in China: A cross-sectional study. Eur Psychiatry 63: e65. https://doi:10.1192/j.eurpsy.2020.59 ![]() |
[6] |
Marvaldi M, Mallet J, Dubertret C, et al. (2021) Anxiety, depression, trauma-related, and sleep disorders among healthcare workers during the COVID-19 pandemic: A systematic review and meta-analysis. Neurosci Biobehav Rev 126: 252-264. https://doi.org/10.1016/j.neubiorev.2021.03.024 ![]() |
[7] |
Pappa S, Ntella V, Giannakas T, et al. (2020) Prevalence of depression, anxiety, and insomnia among healthcare workers during the COVID-19 pandemic: A systematic review and meta-analysis. Brain Behav Immun 88: 901-907. https://doi.org/10.1016/j.bbi.2020.05.026 ![]() |
[8] |
Perego G, Cugnata F, Brombin C, et al. (2022) The “Healthcare Workers' Wellbeing [Benessere Operatori]” Project: A Longitudinal Evaluation of Psychological Responses of Italian Healthcare Workers during the COVID-19 Pandemic. J Clin Med 11: 2317. https://doi: 10.3390/jcm11092317 ![]() |
[9] |
Varghese A, George G, Kondaguli SV, et al. (2021) Decline in the mental health of nurses across the globe during COVID-19: A systematic review and meta-analysis. J Glob Health 11: 05009. https://doi:10.7189/jogh.11.05009 ![]() |
[10] |
Sarafis P, Rousaki E, Tsounis A, et al. (2016) The impact of occupational stress on nurses' caring behaviors and their health related quality of life. BMC Nurs 27: 56. https://doi.org/10.1186/s12912-016-0178-y ![]() |
[11] |
Sikaras C, Zyga S, Tsironi M, et al. (2023) The Mediating Role of Depression and of State Anxiety οn the Relationship between Trait Anxiety and Fatigue in Nurses during the Pandemic Crisis. Healthcare 11: 367. https://doi.org/10.3390/healthcare11030367 ![]() |
[12] |
Thorsteinsson EB, Brown RF, Owens MT (2019) Modeling the Effects of Stress, Anxiety, and Depression on Rumination, Sleep, and Fatigue in a Nonclinical Sample. J Nerv Ment Dis 207: 355-359. https://doi:10.1097/NMD.0000000000000973 ![]() |
[13] |
Zhang C, Yang L, Liu S, et al. (2020) Survey of Insomnia and Related Social Psychological Factors Among Medical Staff Involved in the 2019 Novel Coronavirus Disease Outbreak. Front Psychiatry 11: 306. https://doi:10.3389/fpsyt.2020.00306 ![]() |
[14] |
Morin CM, Rodrigue S, Ivers H (2003) Role of stress, arousal, and coping skills in primary insomnia. Psychosom Med 65: 259-267. https://doi:10.1097/01.psy.0000030391.09558.a3 ![]() |
[15] |
Yazdi Z, Sadeghniiat-Haghighi K, Javadi AR, et al. (2014) Sleep quality and insomnia in nurses with different circadian chronotypes: morningness and eveningness orientation. Work 47: 561-567. https://doi:10.3233/WOR-131664 ![]() |
[16] |
Knap M, Maciąg D, Trzeciak-Bereza E, et al. (2022) Sleep Disturbances and Health Consequences Induced by the Specificity of Nurses' Work. Int J Environ Res Public Health 19: 9802. https://doi:10.3390/ijerph19169802 ![]() |
[17] |
Jahrami H, BaHammam AS, Bragazzi NL, et al. (2021) Sleep problems during the COVID-19 pandemic by population: a systematic review and meta-analysis. J Clin Sleep Med 17: 299-313. https://doi:10.5664/jcsm.8930 ![]() |
[18] |
Watson NF, Badr MS, et al. (2015) Joint Consensus Statement of the American Academy of Sleep Medicine and Sleep Research Society on the Recommended Amount of Sleep for a Healthy Adult: Methodology and Discussion. Sleep 38: 1161-1183. https://doi.org/10.5665/sleep.4886 ![]() |
[19] |
Hu J, Huang Y, Liu J, et al. (2022) COVID-19 Related Stress and Mental Health Outcomes 1 Year After the Peak of the Pandemic Outbreak in China: the Mediating Effect of Resilience and Social Support. Front Psychiatry 13: 828379. https://doi:10.3389/fpsyt.2022.828379 ![]() |
[20] |
Zeng W, Fang Q, Wang C, et al. (2022) Perceived Family Function and Associated Predictors in Nurses: A Cross-Sectional Study. Front Psychiatry 13: 904581. https://doi:10.3389/fpsyt.2022.904581 ![]() |
[21] |
Tselebis A, Lekka D, Sikaras C, et al. (2020) Insomnia, Perceived Stress, and Family Support among Nursing Staff during the Pandemic Crisis. Healthcare 8: 434. https://doi: 10.3390/healthcare8040434 ![]() |
[22] |
Ilias I, Hatzimichelakis E, Souvatzoglou A, et al. (2001) Perception of family support is correlated with glycemic control in Greeks with diabetes mellitus. Psychol Rep 88: 929-930. https://doi:10.2466/pr0.2001.88.3.929 ![]() |
[23] | Sample size calculator & complete guide, 2020. Available from: https://www.qualtrics.com/blog/calculating-sample-size/. |
[24] | Spielberger CD, Gorsuch RL, Lushene PR, et al. Manual for the State–Trait Spielberger Anxiety Inventory (Form Y). (1983).Consulting Psychologists Press. |
[25] |
Fountoulakis KN, Papadopoulou M, Kleanthous S, et al. (2006) Reliability and psychometric properties of the Greek translation of the State-Trait Anxiety Inventory form Y: preliminary data. Ann Gen Psychiatry 5: 2. https://doi.org/10.1186/1744-859X-5-2 ![]() |
[26] |
Soldatos CR, Dikeos DG, Paparrigopoulos TJ (2003) The diagnostic validity of the Athens Insomnia Scale. J Psychosom Res 55: 263-267. https://doi.org/10.1016/s0022-3999(02)00604-9 ![]() |
[27] |
Soldatos CR, Dikeos DG, Paparrigopoulos TJ (2000) Athens Insomnia Scale: Validation of an instrument based on ICD-10 criteria. J Psychosom Res 48: 555-560. https://doi.org/10.1016/s0022-3999(00)00095-7 ![]() |
[28] |
Tselebis A, Anagnostopoulou T, Bratis D, et al. (2011) The 13 item Family Support Scale: Reliability and validity of the Greek translation in a sample of Greek health care professionals. Asia Pac Fam Med 10: 3. https://doi:10.1186/1447-056X-10-3 ![]() |
[29] | Tselebis A, Bratis D, Pachi A, et al. (2013) Chronic obstructive pulmonary disease: sense of coherence and family support versus anxiety and depression. Psychiatriki 24: 109-116. |
[30] |
Chang EC, Chang OD, Martos T, et al. (2017) Family Support as a Moderator of the Relationship Between Loneliness and Suicide Risk in College Students: Having a Supportive Family Matters!. Family J 25: 257-263. https://doi.org/10.1177/1066480717711102 ![]() |
[31] |
Qi T, Hu T, Ge QQ, et al. (2021) COVID-19 pandemic related long-term chronic stress on the prevalence of depression and anxiety in the general population. BMC Psychiatry 21: 380. https://doi:10.1186/s12888-021-03385-x ![]() |
[32] |
Cyr S, Marcil MJ, Houchi C, et al. (2022) Evolution of burnout and psychological distress in healthcare workers during the COVID-19 pandemic: a 1-year observational study. BMC Psychiatry 22: 809. https://doi:10.1186/s12888-022-04457-2 ![]() |
[33] |
Preti E, Di Mattei V, Perego G, et al. (2020) The Psychological Impact of Epidemic and Pandemic Outbreaks on Healthcare Workers: Rapid Review of the Evidence. Curr Psychiatry Rep 22: 43. https://doi:10.1007/s11920-020-01166-z ![]() |
[34] |
Dragioti E, Tsartsalis D, Mentis M, et al. (2022) Impact of the COVID-19 pandemic on the mental health of hospital staff: An umbrella review of 44 meta-analyses. Int J Nurs Stud 131: 104272. https://doi:10.1016/j.ijnurstu.2022.104272 ![]() |
[35] |
Lai J, Ma S, Wang Y, et al. (2020) Factors Associated With Mental Health Outcomes Among Health Care Workers Exposed to Coronavirus Disease 2019. JAMA Netw Open 3: e203976. https://doi.org/10.1001/jamanetworkopen.2020.3976 ![]() |
[36] |
Morgan R, Tan HL, Oveisi N, et al. (2022) Women healthcare workers' experiences during COVID-19 and other crises: A scoping review. Int J Nurs Stud Adv 4: 100066. https://doi:10.1016/j.ijnsa.2022.100066 ![]() |
[37] |
Li SH, Graham BM (2017) Why are women so vulnerable to anxiety, trauma-related and stress-related disorders? The potential role of sex hormones. Lancet Psychiatry 4: 73-82. https://doi:10.1016/S2215-0366(16)30358-3 ![]() |
[38] |
Morin CM, Jarrin DC (2022) Epidemiology of Insomnia: Prevalence, Course, Risk Factors, and Public Health Burden. Sleep Med Clin 17: 173-191. https://doi:10.1016/j.jsmc.2022.03.003 ![]() |
[39] |
Morin CM, Bjorvatn B, Chung F, et al. (2021) Insomnia, anxiety, and depression during the COVID-19 pandemic: an international collaborative study. Sleep Med 87: 38-45. https://doi:10.1016/j.sleep.2021.07.035 ![]() |
[40] |
Alimoradi Z, Gozal D, Tsang HWH, et al. (2022) Gender-specific estimates of sleep problems during the COVID-19 pandemic: Systematic review and meta-analysis. J Sleep Res 31: e13432. https://doi:10.1111/jsr.13432 ![]() |
[41] |
López-Soto PJ, Fabbian F, Cappadona R, et al. (2019) Chronotype, nursing activity, and gender: A systematic review. J Adv Nurs 75: 734-748. https://doi:10.1111/jan.13876 ![]() |
[42] |
Xin J, Zhang Y, Tang Y, et al. (2019) Brain Differences Between Men and Women: Evidence From Deep Learning. Front Neurosci 13: 185. https://doi: 10.3389/fnins.2019.00185 ![]() |
[43] |
AlRasheed MM, Fekih-Romdhane F, Jahrami H, et al. (2022) The prevalence and severity of insomnia symptoms during COVID-19: A global systematic review and individual participant data meta-analysis. Sleep Med 100: 7-23. https://doi:10.1016/j.sleep.2022.06.020 ![]() |
[44] |
Carmel S, Anson O, Levenson A, et al. (1991) Life events, sense of coherence and health: gender differences on the kibbutz. Soc Sci Med 32: 1089-1096. https://doi.org/10.1016/0277-9536(91)90084-p ![]() |
[45] |
Sriharan A, West KJ, Almost J, et al. (2021) COVID-19-related occupational burnout and moral distress among nurses: a rapid scoping review. Nurs Leadersh 34: 7-19. https://doi:10.12927/cjnl.2021.26459 ![]() |
[46] |
Galanis P, Vraka I, Fragkou D, et al. (2021) Nurses' burnout and associated risk factors during the COVID-19 pandemic: a systematic review and meta-analysis. J Adv Nurs 77: 3286-3302. https://doi:10.1111/jan.14839 ![]() |
[47] |
Italia S, Costa C, Briguglio G, et al. (2021) Quality of Life, Insomnia and Coping Strategies during COVID-19 Pandemic in Hospital Workers. A Cross-Sectional Study. Int J Environ Res Public Health 18: 12466. https://doi:10.3390/ijerph182312466 ![]() |
[48] |
Sahebi A, Abdi K, Moayedi S, et al. (2021) The prevalence of insomnia among health care workers amid the COVID-19 pandemic: An umbrella review of meta-analyses. J Psychosom Res 149: 110597. https://doi:10.1016/j.jpsychores.2021.110597 ![]() |
[49] |
Jahrami H, Haji EA, Saif ZQ, et al. (2022) Sleep Quality Worsens While Perceived Stress Improves in Healthcare Workers over Two Years during the COVID-19 Pandemic: Results of a Longitudinal Study. Healthcare 10: 1588. https://doi:10.3390/healthcare10081588 ![]() |
[50] | Trakada A, Nikolaidis PT, Economou NT, et al. (2022) Comparison of sleep characteristics during the first and second period of restrictive measures due to COVID-19 pandemic in Greece. Eur Rev Med Pharmacol Sci 26: 1382-1387. https://doi:10.26355/eurrev_202202_28131 |
[51] | COVID-19 CORONAVIRUS PANDEMIC, 2023. Available from: https://www.worldometers.info/coronavirus/country/greece/. |
[52] | Health at a Glance: Europe 2020 STATE OF HEALTH IN THE EU CYCLE, 2020. Available from: https://ec.europa.eu/health/system/files/2020-12/2020_healthatglance_rep_en_0.pdf. |
[53] | Zhao Y, Hu B, Liu Q, et al. (2022) Social support and sleep quality in patients with stroke: The mediating roles of depression and anxiety symptoms. Int J Nurs Pract 28: e12939. https://doi:10.1111/ijn.12939 |
[54] | Ye B, Hu J, Im H, et al. (2022) Family Cohesion and Sleep Disturbances During COVID-19: the Mediating Roles of Security and Stress. Int J Ment Health Addict : 1-14. https://doi:10.1007/s11469-022-00753-w |
[55] | Xiao H, Zhang Y, Kong D, et al. (2020) The effects of social support on sleep quality of medical staff treating patients with Coronavirus Disease 2019 (COVID-19) in January and February 2020 in China. Med Sci Monit 26: e923549. https://doi.org/10.12659/MSM.923549 |
[56] |
Bilgiç Ş, Çelikkalp Ü, Mısırlı C (2021) Stress level and sleep quality of nurses during the COVID-19 pandemic. Work 70: 1021-1029. https://doi:10.3233/WOR-210538 ![]() |
[57] |
Bruce HA, Kochunov P, Kvarta MD, et al. (2023) Frontal white matter association with sleep quality and the role of stress. J Sleep Res 32: e13669. https://doi:10.1111/jsr.13669 ![]() |
[58] |
Chan NY, Chan JWY, Li SX, et al. (2021) Non-pharmacological Approaches for Management of Insomnia. Neurotherapeutics 18: 32-43. https://doi:10.1007/s13311-021-01029-2 ![]() |
[59] |
Hertenstein E, Feige B, Gmeiner T, et al. (2019) Insomnia as a predictor of mental disorders: A systematic review and meta-analysis. Sleep Med Rev 43: 96-105. https://doi:10.1016/j.smrv.2018.10.006 ![]() |
[60] |
Meaklim H, Saunders WJ, Byrne ML, et al. (2023) Insomnia is a key risk factor for persistent anxiety and depressive symptoms: A 12-month longitudinal cohort study during the COVID-19 pandemic. J Affect Disord 322: 52-62. https://doi:10.1016/j.jad.2022.11.021 ![]() |
[61] | Acar Sevinc S, Metin S, Balta Basi N, et al. (2022) Anxiety and burnout in anesthetists and intensive care unit nurses during the COVID-19 pandemic: a cross-sectional study. Braz J Anesthesiol 72: 169-175. https://doi:10.1016/j.bjane.2021.07.011 |
[62] |
Roberts NJ, McAloney-Kocaman K, Lippiett K, et al. (2021) Levels of resilience, anxiety and depression in nurses working in respiratory clinical areas during the COVID pandemic. Respir Med 176: 106219. https://doi:10.1016/j.rmed.2020.106219 ![]() |
[63] |
Varma P, Junge M, Meaklim H, et al. (2021) Younger people are more vulnerable to stress, anxiety and depression during COVID-19 pandemic: A global cross-sectional survey. Prog Neuropsychopharmacol Biol Psychiatry 109: 110236. https://doi:10.1016/j.pnpbp.2020.110236 ![]() |
[64] |
Pachi A, Sikaras C, Ilias I, et al. (2022) Burnout, Depression and Sense of Coherence in Nurses during the Pandemic Crisis. Healthcare 10: 134. https://doi:10.3390/healthcare10010134 ![]() |
[65] |
Bratis D, Tselebis A, Sikaras C, et al. (2009) Alexithymia and its association with burnout, depression and family support among Greek nursing staff. Hum Resour Health 7: 72. https://doi:10.1186/1478-4491-7-72 ![]() |
[66] |
Tselebis A, Bratis D, Karkanias A, et al. (2008) Associations on dimensions of burnout and family support for a sample of Greek nurses. Psychol Rep 103: 63-66. https://doi:10.2466/pr0.103.1.63-66 ![]() |
[67] | Lekka D, Pachi A, Tselebis A, et al. (2014) Pain and Anxiety versus Sense of Family Support in Lung Cancer Patients. Pain Res Treat 2014: 312941. https://doi:10.1155/2014/312941 |
[68] |
Noh D, Park S (2022) Mediating Effects of Social Support and Resilience on the Association between COVID-19-Related Stress and Mental Health in Korean Young Adults. Int J Environ Res Public Health 19: 6935. https://doi:10.3390/ijerph19116935 ![]() |
[69] |
Shi LS, Xu RH, Xia Y, et al. (2022) The Impact of COVID-19-Related Work Stress on the Mental Health of Primary Healthcare Workers: The Mediating Effects of Social Support and Resilience. Front Psychol 12: 800183. https://doi:10.3389/fpsyg.2021.800183 ![]() |
[70] |
Mohammed S, Peter E, Killackey T, et al. (2021) The “nurse as hero” discourse in the COVID-19 pandemic: A poststructural discourse analysis. Int J Nurs Stud 117: 103887. https://doi: 10.1016/j.ijnurstu.2021.103887 ![]() |
[71] |
Boulton M, Garnett A, Webster F (2022) A Foucauldian discourse analysis of media reporting on the nurse-as-hero during COVID-19. Nurs Inq 29: e12471. https://doi.org/10.1111/nin.12471 ![]() |
[72] |
Elsayed MEG, El-Abasiri RA, Marzo RR, et al. (2023) Mental health, risk perception, and coping strategies among healthcare workers in Egypt during the COVID-19 pandemic. PLoS One 18: e0282264. https://doi:10.1371/journal.pone.0282264 ![]() |
[73] |
Htay MNN, Marzo RR, Bahari R, et al. (2021) How healthcare workers are coping with mental health challenges during COVID-19 pandemic? - A cross-sectional multi-countries study. Clin Epidemiol Glob Health 11: 100759. https://doi:10.1016/j.cegh.2021.100759 ![]() |
[74] |
Htay MNN, Marzo RR, AlRifai A, et al. (2020) Immediate impact of COVID-19 on mental health and its associated factors among healthcare workers: A global perspective across 31 countries. J Glob Health 10: 020381. https://doi:10.7189/jogh.10.020381 ![]() |
[75] |
Marzo RR, ElSherif M, Abdullah MSAMB, et al. (2022) Demographic and work-related factors associated with burnout, resilience, and quality of life among healthcare workers during the COVID-19 pandemic: A cross sectional study from Malaysia. Front Public Health 10: 1021495. https://doi:10.3389/fpubh.2022.1021495 ![]() |
[76] |
Kamberi F, Sinaj E, Jaho J, et al. (2021) Impact of COVID-19 pandemic on mental health, risk perception and coping strategies among health care workers in Albania - evidence that needs attention. Clin Epidemiol Glob Health 12: 100824. https://doi:10.1016/j.cegh.2021.100824 ![]() |
1. | Bin Lu, Rongfang Yan, Ordering results of second order statistics from random and non-random number of random variables with Archimedean copulas, 2021, 6, 2473-6988, 6390, 10.3934/math.2021375 | |
2. | Rongfang Yan, Jiale Niu, Stochastic comparisons of second-order statistics from dependent and heterogenous modified proportional hazard rate observations, 2023, 0233-1888, 1, 10.1080/02331888.2023.2177999 | |
3. | Xiao Zhang, Rongfang Yan, Stochastic comparisons of extreme order statistic from dependent and heterogeneous lower-truncated Weibull variables under Archimedean copula, 2022, 7, 2473-6988, 6852, 10.3934/math.2022381 | |
4. | Liang Jiao, Rongfang Yan, Stochastic Comparisons of Lifetimes of Series and Parallel Systems with Dependent Heterogeneous MOTL-G Components under Random Shocks, 2021, 13, 2073-8994, 2248, 10.3390/sym13122248 | |
5. | Sangita Das, Suchandan Kayal, Nuria Torrado, Ordering results between extreme order statistics in models with dependence defined by Archimedean [survival] copulas, 2022, 0035-5038, 10.1007/s11587-022-00715-3 | |
6. | Mansour Shrahili, Mohamed Kayid, Mhamed Mesfioui, Relative Orderings of Modified Proportional Hazard Rate and Modified Proportional Reversed Hazard Rate Models, 2023, 11, 2227-7390, 4652, 10.3390/math11224652 | |
7. | Bin Lu, Stochastic comparisons of second-order statistics from dependent and heterogeneous modified proportional (reversed) hazard rates scale models, 2024, 9, 2473-6988, 8904, 10.3934/math.2024434 | |
8. | Mingxia Yang, Orderings of the second-largest order statistic with modified proportional reversed hazard rate samples, 2025, 10, 2473-6988, 311, 10.3934/math.2025015 |